The week's pick
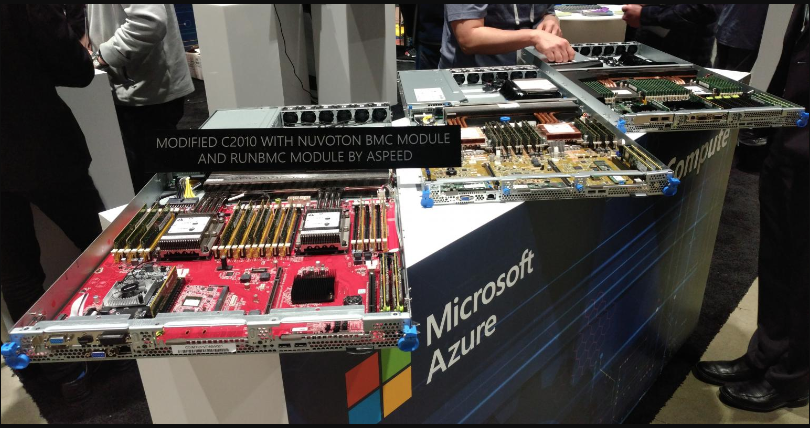

Random Articles
- Design and Implementation of a Medium Interaction Honeypot May 2013 An Approach for Recommender System by Combining Collaborative Filtering with User Demographics and Items Genres October 2015 Study of Entity Detection and Identification using Deep Learning Techniques a Survey Mar 2020 Article:A Multi-Agent System for Management of Supplier Selection Process in a Fuzzy Supply Chain June 2011
Reseach Article
Improvement of crm using data mining: a case study at corporate telecom sector.
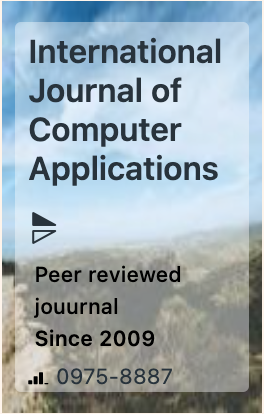
Sanjib Kumar Routray, Sanjit Kumar Dash, Sasmita Mishra . Improvement of CRM using Data Mining: A Case study at Corporate Telecom Sector. International Journal of Computer Applications. 178, 53 ( Sep 2019), 12-20. DOI=10.5120/ijca2019919413
As Customer relationship management (CRM) is a well established concept and its practice to enable the realization of successful Telecommunication system, data mining techniques is developed for improving the customer relationship management part mainly in Corporate Telecom Sector. Considering the existing methodology, a well established methodology with data mining is needed for development of good integrated approach with growth in time and space complexities. The aim is to find the strategic point on the essential part of Telecommunication industry by exploring the techniques of data mining. Then the focus is on presenting a new methodology in case of mobile services on the perceptions of customers of Telecommunication basing on applicability of data mining techniques to CRM databases by generating Association rules from frequent item sets on the proposed approach F-MFPG (Fast Modified Frequent Pattern Growth) by using FFIM (Fast Frequent Item sets Mining) Algorithm under concept of data mining and predicting the profit of Corporate Telecom Sector and predicting the churn for retention of customers for efficient managerial decision for reaching the ultimate goals by proposing a suitable classification techniques in data mining algorithms.
- Adam, Braff., Passmore, William,J. and Simpson, Michael (2006) “Going the distance with telecom customers” In the proceedings of The Mckinsey Quarterly,No.4, (pp.83).
- Ananthi, D., Karthiga, E., Vithiskumar, R.(2013) “Optimizing the CRM process in Product Market place by Association Rule Mining Technology” In the proceeding of Journal of Environmental Science Engineering & Technology, Vol-2 (pp.412-416)
- Alawin, Ayman., Mohmmad, Al., aitah, ma., Balqua, Al (2014) “ Proposed ranking for point of sales using data mining for Telecom Operations” In the proceeding of International Journal of Database management system,Vol-6.
- Bagozzi, Richard P.(1995). ”Reflections on Relationship marketing in Consumer markets” In proceeding of Journal of the Academy of Marketing Science, 23(Fall), (pp. 272-277).
- Berry, l., Shostack, G., and .Upah, G. (2003). “Emerging perspectives on service marketing”,Chacago,IL, American marketing Association (pp.25-28).
- Bhave, Ashish (2002). “Customer Satisfaction Measurement” in the proceeding of Quality and Productive journal, ( pp.562-572).
- Chaturvedi, Mukesh., Chaturvedi, Abhinav (2005) “Customer Relationship Management-An India Perspective” In the proceeding of Excel Books,ISBN 10: 8174464565, ISBN 13: 9788174464560,New Delhi (pp-36).
- Dahiya, Kiran., Talwar, Kanika (2015) “Customer Churn Prediction in Telecommunication Industry using Data mining technique-A Review” In the proceeding of International Journal of Advanced Research in Computer Science and Software Engineering, Vol-5, Issue-4.
- Heling, Jiang., Yang, An., Yan, Fengyun.,Hang, Miao (2016) “Research on pattern Analysis and data Classification methodology of Data mining and knowledge Recovery”, In the proceeding of International journal of Hybrid Information Technology, IEEE, Vol-9( pp.179-186).
- Khan, M N., Rahman, Abdur (2017) “An Assessment of data mining based CRM techniques for enhancing profitability” In proceeding of Association of Modern Education & Computer science, (pp.30-40).
- Meltzer, Michael.“Are your customer profitable and segment your customer based on profitability”
- Pradnya, A., Shirasth, Verma, Vijaya Kumar (2013) “ A recent Survey on Incremental Temporal Association Rule Mining” In the proceeding of International Journal of Innovative Technology & Exploring Engineering, Vol-3,Issue-1( pp.88-90).
- Sasikala (2006) “Telecom Services: Measurement of Customer satisfaction” In the proceeding of The ICFAI, Journal of Management Research,Vol-5,Issue-10 (pp.35-53).
- Srivastava, R., Bhangde, Jatin., Bhatt, Nirav., Gogri, Kunal and Marfatia, Hemat (2006) “ Role of Competition in Growing Markets: Telecom Sector” In the proceeding of Indian Journal of Marketing ( pp-8).
- Senkamalavalll, R., Dr. Bhubaneswari,T (2013)“Data mining techniques for CRM” In the proceeding of International journal of Scientific & Engineering Research, Vol-4, Issue-4, (pp. 509-513).
- Tejaswini, Hilage, Kulkarni, R.V (2012)” Review the literature of Data Mining” In the proceeding of IJRRS, Vol-II ( PP.107-114).
Index Terms
Computer Science Information Sciences
Customer relationship management Fast Modified Frequent Pattern Growth Fast Frequent Item sets Mining Association Classification True Positive False Positive and Telecommunication
The application of Data Mining in CRM
Ieee account.
- Change Username/Password
- Update Address
Purchase Details
- Payment Options
- Order History
- View Purchased Documents
Profile Information
- Communications Preferences
- Profession and Education
- Technical Interests
- US & Canada: +1 800 678 4333
- Worldwide: +1 732 981 0060
- Contact & Support
- About IEEE Xplore
- Accessibility
- Terms of Use
- Nondiscrimination Policy
- Privacy & Opting Out of Cookies
A not-for-profit organization, IEEE is the world's largest technical professional organization dedicated to advancing technology for the benefit of humanity. © Copyright 2024 IEEE - All rights reserved. Use of this web site signifies your agreement to the terms and conditions.
Academia.edu no longer supports Internet Explorer.
To browse Academia.edu and the wider internet faster and more securely, please take a few seconds to upgrade your browser .
Enter the email address you signed up with and we'll email you a reset link.
- We're Hiring!
- Help Center
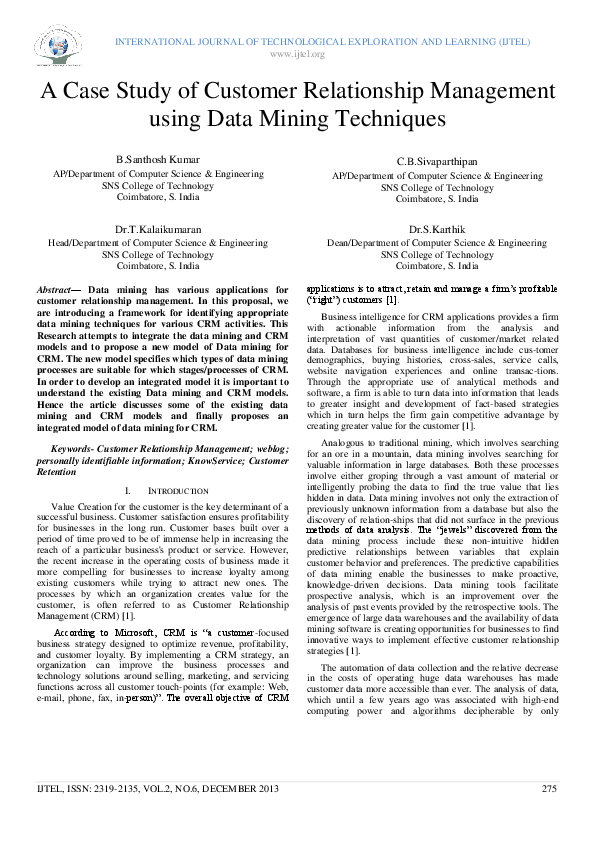
A Case Study of Customer Relationship Management using Data Mining Techniques

Related Papers
Moe Sedighi
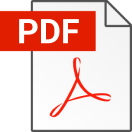
priya jhmat
Business Process Management Journal
Rai Priyanka Vinod
Mohamed Kabayare
Customer relationship management (CRM) is a combination of people, processes and technology that seeks to understand a company's customers. It is an integrated approach to managing relationships by focusing on customer retention and relationship development. CRM has evolved from advances in information technology and organizational changes in customer-centric processes. Companies that successfully implement CRM will reap the rewards in customer loyalty and long run profitability. However, successful implementation is elusive to many companies, mostly because they do not understand that CRM requires company-wide, cross-functional, customer-focused business process re-engineering. Although a large portion of CRM is technology, viewing CRM as a technology-only solution is likely to fail. Managing a successful CRM implementation requires an integrated and balanced approach to technology, process, and people. 1. Introduction In the mid-twentieth century, mass production techniques and mass marketing changed the competitive landscape by increasing product availability for consumers. However, the purchasing process that allowed the shopkeeper and customer to spend quality time getting to know each other was also fundamentally changed. Customers lost their uniqueness, as they became an “account number” and shopkeepers lost track of their customers’ individual needs as the market became full of product and service options. Many companies today are racing to re-establish their connections to new as well as existing customers to boost long-term customer loyalty. Some companies are competing effectively and winning this race through the implementation of relationship marketing principles using strategic and technology-based customer relationship management (CRM) applications. CRM technology applications link front office (e.g. sales, marketing and customer service) and back office (e.g. financial, operations, logistics and human resources) functions with the company's customer “touch points” (Fickel, 1999). A company's touch points can include the Internet, e-mail, sales, direct mail, telemarketing operations, call centers, advertising, fax, pagers, stores, and kiosks. Often, these touch points are controlled by separate information systems. CRM integrates touch points around a common view of the customer (Eckerson and Watson, 2000). Figure 1 demonstrates the relationship between customer touch points with front and back office operations. In some organizations, CRM is simply a technology solution that extends separate databases and sales force automation tools to bridge sales and marketing functions in order to improve targeting efforts. Other organizations consider CRM as a tool
Namadhila Shapaka
Rolph Anderson
Journal of Interactive Marketing
Shankar Ganesan , Peter Verhoef
RELATED TOPICS
- We're Hiring!
- Help Center
- Find new research papers in:
- Health Sciences
- Earth Sciences
- Cognitive Science
- Mathematics
- Computer Science
- Academia ©2024
- Data Center
- Applications
- Open Source
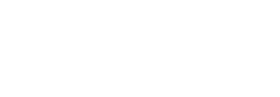
Datamation content and product recommendations are editorially independent. We may make money when you click on links to our partners. Learn More .
Companies understand that data mining can provide insights to improve the organization. Yet, many struggle with the right types of data to collect, where to start, or what project may benefit from data mining.
Examining the data mining success of others in a variety of circumstances illuminates how certain methods and software in the market can assist companies. See below how five organizations benefited from data mining in different industries: cybersecurity, finance, health care, logistics, and media.
See more: What is Data Mining? Types & Examples
1. Cerner Corporation
Over 14,000 hospitals, physician’s offices, and other medical facilities use Cerner Corporation’s software solutions.
Cerner’s access allows them to combine patient medical records and medical device data to create an integrated medical database and improve health care.
Using Cloudera’s data mining allows different devices to feed into a common database and predict medical conditions.
“In our first attempts to build this common platform, we immediately ran into roadblocks,” says Ryan Brush, senior director and distinguished engineer at Cerner.
“Our clients are reporting that the new system has actually saved hundreds of lives by being able to predict if a patient is septic more effectively than they could before.”
Industry: Health care
Data mining provider: Cloudera
- Collect data from unlimited and different sources
- Enhance operational and financial performance for health care facilities
- Improve patient diagnosis and save lives
Read the Cerner Corporation and Cloudera, Inc. case study.
DHL Temperature Management Solutions provides temperature controlled pharmaceutical logistics to ensure pharmaceutical and biological goods stay within required temperature ranges to retain potency.
Previously, DHL transferred data into spreadsheets that took a week to compile and would only contain a portion of the potential information.
Moving to DOMO’s data mining platform allows for real-time reporting of a broader set of data categories to improve insight.
“We’re able to pinpoint issues that we couldn’t see before. For example, a certain product, on a certain lane, at a certain station is experiencing an issue repeatedly,” says Dina Bunn, global head of central operations and IT for DHL Temperature Management Solutions.
Industry: Logistics
Data mining provider: DOMO
- Real-time versus week-old logistics information
- More insight into sources of delays or problems at both a high and a detailed level
- More customer engagement
Read the DHL and DOMO case study.
See more: Current Trends & Future Scope of Data Mining
The Nasdaq electronic stock exchange integrates Sisense’s data mining capabilities into their IR Insight software to help customers analyze huge data sets.
“Our customers rely on a range of content sets, including information that they license from others, as well as data that they input themselves,” says James Tickner, head of data analytics for Nasdaq Corporate Solutions.
“Being able to layer those together and attain a new level of value from content that they’ve been looking at for years but in another context.”
The combined application provides real-time analysis and clear reports easy for customers to understand and communicate internally.
Industry: Finance
Data mining provider: Sisense
- Meets rigorous data security regulations
- Quickly processes huge data sets from a variety of sources
- Provides clients with new ways to visualize and interpret data to extract new value
Read or watch the Nasdaq and Sisense case study.
The Public Broadcasting System (PBS) of the U.S. manages an online website to service 353 PBS member stations and their viewers. Their 330 million sessions, 800 million page views, and 17.5 million episode plays generate enormous data that the PBS team struggled to analyze.
PBS worked with LunaMetrics to perform data mining on the Google Analytics 360 platform to speed up insights into PBS customers.
Dan Haggerty, director of digital analytics for PBS, says “that was the coolest thing about it. A machine took our data without prior assumptions and reaffirmed and strengthened ideas that subject matter experts already suspected about our audiences based on our contextual knowledge.”
Industry: Media
Data mining provider: Google Analytics and LunaMetrics
- Identified seven key audience segments based on web behaviors
- Developed in-depth personas per segment through data mining
- Insights help direct future content and feature development
Read the PBS, LunaMetrics, and Google Analytics case study.
5. The Pegasus Group
Cyber attackers compromised and targeted the data mining system (DMS) of a major network client of The Pegasus Group and launched a distributed denial-of-service (DDoS) attack against 1,500 services.
Under extreme time pressure, The Pegasus Group needed to find a way to use data mining to analyze up to 35GB of data with no prior knowledge of the data contents.
“[I analyzed] the first three million lines and [used RapidMiner’s data mining to perform] a stratified sampling to see which ones [were] benign, which packets [were] really part of the network, and which packets were part of the attack,” says Rodrigo Fuentealba Cartes of The Pegasus Group.
“In just 15 minutes … I used this amazing simulator to see what kinds of parameters I could use to filter packets … and in another two hours, the attack was stopped.”
Industry: Cybersecurity
Data mining provider: RapidMinder
- Uploaded and analyzed three million lines of data
- Recommended analysis models provided answers within 15 minutes
- Data analysis suggested solutions that stopped the attack within two hours
Watch The Pegasus Group and RapidMiner case study.
See more: Top Data Mining Tools
Subscribe to Data Insider
Learn the latest news and best practices about data science, big data analytics, artificial intelligence, data security, and more.
Similar articles
8 best data analytics tools: gain data-driven advantage in 2024, common data visualization examples: transform numbers into narratives, what is data management a guide to systems, processes, and tools, get the free newsletter.
Subscribe to Data Insider for top news, trends & analysis
Latest Articles
8 best data analytics..., common data visualization examples:..., what is data management..., how to run a....
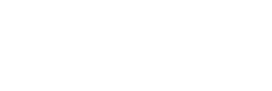
Data Mining Case Studies & Benefits

- Key Takeaways
Data mining has improved the decision-making process for over 80% of companies. (Source: Gartner).
Statista reports that global spending on robotic process automation (RPA) is projected to reach $98 billion by 2024, indicating a significant investment in automation technologies.
According to Grand View Research, the global data mining market will reach $16,9 billion in 2027.
Ethical Data Mining preserves individual rights and fosters trust.
A successful implementation requires defining clear goals, choosing data wisely, and constant adaptation.
Data mining case studies help businesses explore data for smart decision-making. It’s about finding valuable insights from big datasets. This is crucial for businesses in all industries as data guides strategic planning. By spotting patterns in data, businesses gain intelligence to innovate and stay competitive. Real examples show how data mining improves marketing and healthcare. Data mining isn’t just about analyzing data; it’s about using it wisely for meaningful changes.
The Importance of Data Mining for Modern Business:

Data mining has taken on a central role in the modern world of business. Data is a major issue for businesses today. Making informed decisions with this data can be crucial to staying competitive. This article explores the many aspects of data mining and its impact on decisions.
- Unraveling Data Landscape
Businesses generate a staggering amount of data, including customer interactions, market patterns, and internal operations. Decision-makers face an information overload without effective tools for sorting through all this data.
Data mining is a process which not only organizes, structures and extracts patterns and insights from this vast amount of data. It acts as a compass to guide decision makers through the complex landscape of data.
- Empowering Strategic Decision Making
Data mining is a powerful tool for strategic decision making. Businesses can predict future trends and market behavior by analyzing historical data. This insight allows businesses to better align their strategies with predicted shifts.
Data mining can provide the strategic insights required for successful decision making, whether it is launching a product, optimizing supply chain, or adjusting pricing strategies.
- Customer-Centric Determining
Understanding and meeting the needs of customers is paramount in an era where customer-centricity reigns. Data mining is crucial in determining customer preferences, behaviors, and feedback.
This information allows businesses to customize products and services in order to meet the expectations of customers, increase satisfaction and build lasting relationships. With customer-centric insights, decision-makers can make choices that resonate with their target audiences and foster loyalty and brand advocacy.
Data Mining: Applications across industries
Data mining is transforming the way companies operate and make business decisions. This article explores the various applications of data-mining, highlighting case studies that illuminate its impact in the healthcare, retail, and finance sectors.
- Healthcare Case Studies:

Data mining is a powerful tool in the healthcare industry. It can improve patient outcomes and treatment plans. Discover compelling case studies in which data mining played a crucial role in predicting patterns of disease, optimizing treatment and improving patient care. These examples, which range from early detection of health risks to personalized medicines, show the impact that data mining has had on the healthcare industry.
State of Technology 2024
Humanity's Quantum Leap Forward
Explore 'State of Technology 2024' for strategic insights into 7 emerging technologies reshaping 10 critical industries. Dive into sector-wide transformations and global tech dynamics, offering critical analysis for tech leaders and enthusiasts alike, on how to navigate the future's technology landscape.
- Retail Success stories:
Retail is at the forefront of leveraging data mining to enhance customer experiences and streamline operations. Discover success stories of how data mining empowered businesses to better understand consumer behavior, optimize their inventory management and create personalized marketing strategies.
These case studies, which range from e-commerce giants and brick-and-mortar shops, show how data mining can boost sales, improve customer satisfaction, transform the retail landscape, etc.
- Financial Sector Examples:
Data mining is a valuable tool in the finance industry, where precision and risk assessment are key. Explore case studies that demonstrate how data mining can be used for fraud detection and risk assessment. These examples demonstrate how financial institutions use data mining to make better decisions, protect against fraud, and customize services to their clients’ needs.
- Data Mining and Education:
Data mining has been used in the education sector to enhance learning beyond healthcare, retail and finance. Learn how educational institutions use data mining to optimize learning outcomes, analyze student performance and personalize materials. These examples, ranging from adaptive learning platforms and predictive analytics to predictive modeling, demonstrate the potential for data mining to revolutionize how we approach education.
- Manufacturing efficiency:

Data mining is a powerful tool for streamlining manufacturing processes. Examine case studies that demonstrate how data mining can be used to improve supply chain management, predict maintenance requirements, and increase overall operational efficiency. These examples show how data-driven insights can lead to cost savings, increased productivity, and a competitive advantage in manufacturing.
Data mining is a key component in each of these applications. It unlocks insights, streamlines operations, and shapes the future of decisions. Data mining is transforming the landscapes of many industries, including healthcare, retail, education, finance, and manufacturing.
Data Mining Techniques
Data mining techniques help businesses gain an edge by extracting valuable insights and information from large datasets. This exploration will provide an overview of the most popular data mining methods, and back each one with insightful case studies.
- Popular Data Mining Techniques
Clustering Analysis
The clustering technique involves grouping data points based on a set of criteria. This method is useful for detecting patterns in data sets and can be used to segment customers, detect anomalies, or recognize patterns. The case studies will show how clustering can be used to improve marketing strategies, streamline products, and increase overall operational efficiency.
Association Rule Mining
Association rule mining reveals relationships between variables within large datasets. Market basket analysis is a common application of association rule mining, which identifies patterns in co-occurring products in transactions. Real-world examples of how association rule mining is used in retail to improve product placements, increase sales, and enhance the customer experience.
Decision Tree Analysis
The decision tree is a visual representation of the process of making decisions. This technique is a powerful tool for classification tasks. It helps businesses make decisions using a set of criteria. Through case studies, you will learn how decision tree analyses have been used in the healthcare industry for disease diagnosis and fraud detection, as well as predictive maintenance in manufacturing.
Regression Analysis
Regression analysis is a way to explore the relationship between variables. This allows businesses to predict and understand how one variable affects another. Discover case studies that demonstrate how regression analysis is used to predict customer behavior, forecast sales trends, and optimize pricing strategies.
Benefits and ROI:
Businesses are increasingly realizing the benefits of data mining in the current dynamic environment. The benefits are numerous and tangible, ranging from improved decision-making to increased operational efficiency. We’ll explore these benefits, and how businesses can leverage data mining to achieve significant gains.
- Enhancing Decision Making
Data mining provides businesses with actionable insight derived from massive datasets. Analyzing patterns and trends allows organizations to make more informed decisions. This reduces uncertainty and increases the chances of success. There are many case studies that show how data mining has transformed the decision-making process of businesses in various sectors.
- Operational Efficiency
Data mining is essential to achieving efficiency, which is the cornerstone of any successful business. Organizations can improve their efficiency by optimizing processes, identifying bottlenecks, and streamlining operations. These real-world examples show how businesses have made remarkable improvements in their operations, leading to savings and resource optimization.
- Personalized Customer Experiences
Data mining has the ability to customize experiences for customers. Businesses can increase customer satisfaction and loyalty by analyzing the behavior and preferences of their customers. Discover case studies that show how data mining has been used to create engaging and personalized customer journeys.
- Competitive Advantage
Gaining a competitive advantage is essential in today’s highly competitive environment. Data mining gives businesses insights into the market, competitor strategies, and customer expectations. These insights can give organizations a competitive edge and help them achieve success. Look at case studies that show how companies have outperformed their competitors by using data mining.
Calculating ROI and Benefits
To justify investments, businesses must also quantify their return on investment. Calculating ROI for data mining initiatives requires a thorough analysis of the costs, benefits, and long-term impacts. Let’s examine the complexities of ROI within the context of data-mining.
- Cost-Benefit Analysis
Prior to focusing on ROI, companies must perform a cost-benefit assessment of their data mining projects. It involves comparing the costs associated with implementing data-mining tools, training staff, and maintaining infrastructure to the benefits anticipated, such as higher revenue, cost savings and better decision-making. Case studies from real-world situations provide insight into cost-benefit analysis.
- Quantifying Tangible and intangible benefits
Data mining initiatives can yield tangible and intangible benefits. Quantifying tangible benefits such as an increase in sales or a reduction in operational costs is easier. Intangible benefits such as improved brand reputation or customer satisfaction are also important, but they may require a nuanced measurement approach. Examine case studies that quantify both types.
- Long-term Impact Assessment
ROI calculations should not be restricted to immediate gains. Businesses need to assess the impact their data mining projects will have in the future. Consider factors like sustainability, scalability, and ongoing benefits. Case studies that demonstrate the success of data-mining strategies over time can provide valuable insight into long-term impact assessment.
- Key Performance Indicators for ROI
Businesses must establish KPIs that are aligned with their goals in order to measure ROI. KPIs can be used to evaluate the success of data-mining initiatives, whether it is tracking sales growth, customer satisfaction rates, or operational efficiency. Explore case studies to learn how to select and monitor KPIs strategically for ROI measurement.
Data Mining Ethics
Data mining is a field where ethical considerations are crucial to ensuring transparent and responsible practices. It is important to carefully navigate the ethical landscape as organizations use data to extract valuable insights. This section examines ethical issues in data mining and highlights cases that demonstrate ethical practices.
- Understanding Ethical Considerations
Data mining ethics revolves around privacy, consent, and responsible information use. Businesses are faced with the question of how they use and collect data. Ethics also includes the biases in data and the fairness of algorithms.
- Balance Innovation and Privacy
Finding the right balance between privacy and innovation is a major ethical issue in data mining. In order to gain an edge in the market through data insights and to innovate, organizations must walk a tightrope between innovation and privacy. Case studies will illuminate how companies have successfully balanced innovation and privacy.
- Transparency and informed consent
Transparency in the processes is another important aspect of ethical data mining. This is to ensure that individuals are informed and consented before their data is used. This subtopic will explore the importance of transparency in data collection and processing, with case studies that highlight instances where organizations have established exemplary standards to obtain informed consent.
Exploring Data Mining Ethics is crucial as data usage evolves. Businesses must balance innovation, privacy, and transparency while gaining informed consent. Real-world cases show how ethical data mining protects privacy and builds trust.
Implementing Data Mining is complex yet rewarding. This guide helps set goals, choose data sources, and use algorithms effectively. Challenges like data security and resistance to change are common but manageable.
Considering ethics while implementing data mining shows responsibility and opens new opportunities. Organizations prioritizing ethical practices become industry leaders, mitigating risks and achieving positive impacts on business, society, and technology. Ethics and implementation synergize in data mining, unlocking its true potential.
- Q. What ethical considerations are important in data mining?
Privacy and consent are important ethical considerations for data mining.
- Q. How can companies avoid common pitfalls when implementing data mining?
By ensuring the security of data, addressing cultural opposition, and encouraging continuous learning and adaptation.
- Q. Why is transparency important in data mining?
Transparency and consent to use collected data ethically are key elements of building trust.
- Q. What are the main steps to implement data mining in businesses?
Define your objectives, select data sources, select algorithms and monitor continuously.
- Q. How can successful organizations use data mining to gain a strategic advantage?
By taking informed decisions, improving operations and staying on top of the competition.

Related Post
What is data harmonization: key concepts, benefits and more, clickstream data: understanding user behavior on your website, intent data explained: uses and benefits in marketing, what is data segmentation: a comprehensive guide, advanced techniques for query optimization in 2024, exploring alternative data and top use cases of it in finance, table of contents.
Expand My Business is Asia's largest marketplace platform which helps you find various IT Services like Web and App Development, Digital Marketing Services and all others.
- IT Staff Augmentation
- Data & AI
- E-commerce Development
Article Categories
- Technology 698
- Business 329
- Digital Marketing 302
- Social Media Marketing 129
- E-Commerce 129
- Website Development 108
- Software 104
Sitemap / Glossary
Copyright © 2024 Mantarav Private Limited. All Rights Reserved.

- Privacy Overview
- Strictly Necessary Cookies
This website uses cookies so that we can provide you with the best user experience possible. Cookie information is stored in your browser and performs functions such as recognising you when you return to our website and helping our team to understand which sections of the website you find most interesting and useful.
Strictly Necessary Cookie should be enabled at all times so that we can save your preferences for cookie settings.
If you disable this cookie, we will not be able to save your preferences. This means that every time you visit this website you will need to enable or disable cookies again.

CRM Case Studies With Examples
What is a crm case study.

- Customer service
- Project development
- Human resources (HR)
What’s Included in CRM Case Studies?
- Client profile— company type, industry, and a brief history and description of the company
- The situation— the circumstances that led the company to become interested in a CRM solution
- The challenges— the problems and issues the company was facing that a CRM solution could solve
- The process— the heart of the case study, showing how the CRM vendor or service provider performed its services in a way that met or exceeded the client’s goals and expectations
- The results— the benefits to the client at the end of the day
- Vendor/service provider profile— brief description of the vendor or service provider including contact information
The Benefits of a CRM Case Study in the Selection Process
How tec's crm case studies can help you to find the best-fit crm system, tec’s software selection process.
- Assess : Assess the client’s business processes and goals, gaps in key processes, and discover their functional requirements
- Review : Review CRM solutions and vendor capabilities to meet the client’s business needs
- Identify : Identify the shortlist of CRM vendors and their partner(s)
- Demonstration : Assess CRM solution demonstrations scripted to the client’s business processes
- Proposal : Create and distribute CRM request for proposal (RFP) to vendors to clarify deliverables and project TCO (total cost of ownership)
- Reference : Evaluate CRM vendor and their partner(s) through reference checks from real-world clients on previous projects
- Contract : Perform contract review and price negotiations on behalf of the client for cost savings
- Implementation : Perform oversight and monitor the implementation of the CRM application(s) to enable successful transformation and business growth
Access Our Free Library of Case Studies to Learn More About CRM
TEC provides an extensive library of case studies across all types of enterprise software , including CRM . Below we have selected two TEC case studies that showcase how TEC has helped organizations evaluate CRM software systems and select the ideal CRM system for their current and future business needs: Honor Credit Union (HCU)—A Finance & Banking CRM Case Study Honor Credit Union (HCU), a not-for-profit financial institution, faced challenges with a disparate IT setup and lack of unified CRM system, hindering its customer service efforts. Partnering with TEC, HCU underwent a comprehensive process of business assessment, CRM market review, vendor shortlisting, evaluation, contract review and negotiation, leading to the selection and successful implementation of a CRM system that streamlined back-office processes and enhanced customer experience. Read the case study . Acumed—A Manufacturing CRM Case Study Acumed, a global orthopedic and medical solutions provider, faced challenges with their previous CRM system that lacked functionality tailored to their unique sales needs, impacting visibility into sales performance and consignment-based inventory. With TEC's guidance, Acumed went through a comprehensive evaluation process, including requirement assessments, product demonstrations, and contract negotiations, to select a CRM system that addressed the company's complex needs, facilitated consignment-based sales and inventory tracking, and improved management of customer data. Read the case study .
These enterprise software case studies provide insight into the way business solutions work to optimize key processes through best-fit CRM software systems . For companies interested in more information about CRM selection and implementation, or assistance with their CRM project, please contact us today. Get your free business software case study today!
Refine Results
- Enterprise Resource Planning (ERP) 536
- Discrete Manufacturing ERP 127
- Process Manufacturing ERP 122
- Mixed-Mode ERP 112
- ERP for Small Manufacturing Business 100
- Distribution ERP 83
- ERP for Service Industry 35
- ERP for Small Business 33
- Engineer-to-Order (ETO) 121
- Supply Chain & Logistics 299
- Inventory Management 87
- Manufacturing Execution Systems (MES) 57
- Merchandising Software 18
- Supply Relationship Management (SRM) 29
- Transportation Management Systems (TMS) 25
- Demand Management Software 27
- RFID Software 5
- Warehouse Management Systems (WMS) 97
- Supply Chain Management (SCM) 158
- Business Intelligence & Data Analytics 247
- Spreadsheet 1
- Business Process Management (BPM) 100
- Enterprise Performance Management 60
- Business Intelligence (BI) and Data Management 181
- Customer Relationship Management & Support 219
- Customer Relationship Management (CRM) 185
- Call Center 6
- Event Management 1
- Proposal Management 10
- Contract Management 2
- Sales Force Automation Software 21
- Field Service Management (FSM) 80
- Configure Price Quote (CPQ) 33
- Benefits Administration 12
- Compensation Management 6
- Human Resources 24
- Performance Management 14
- Time & Attendance 11
- Workforce Management 15
- Learning Management Systems (LMS) 75
- Talent Acquisition Software 14
- Talent Management Systems 43
- Human Capital Management (HCM) 83
- Financial 130
- Billing & Invoicing 17
- Point of Sale (POS) 2
- Accounting and Financial Software 85
- Asset Management 122
- Facility Management 6
- Computerized Maintenance Management System 109
- Asset Management (EAM) 97
- Product Lifecycle Management (PLM) 115
- Process PLM 15
- Discrete PLM 16
- Regulatory and Compliance PLM 26
- Fashion PLM 22
- Retail PLM 48
- Information & Document Management 69
- Document Management Systems (DMS) 58
- Enterprise Content Management (ECM) 52
- Content Management System (CMS) 17
- IT & IT Security 57
- Help Desk 6
- Virtual Private Network (VPN) 1
- Remote Access 3
- Network Security 16
- E-commerce 31
- Web Content Management (WCM) 6
- Communication & Collaboration 31
- Collaboration 2
- Project Management 8
- Project and Process Management (PPM) 23
- Marketing 23
- Marketing Automation 23
- Design/Creative 9
- Computer-Aided Design (CAD) 9
- Industry-Specific 3
- Property Management 3
- Manufacturing 25
- Wholesale and Retail Trade 18
- Finance and Banking 11
- Industry Independent 10
- Computer, IT, and Software 7
- Telecommunications 6
- Education 4
- Food and Beverage Products 4
- Business Services and Consulting 3
- Health Care and Social Work 3
- Chemical Products 2
- Construction 2
- Electronics and High-tech Components 2
- Insurance 2
- Life and Natural Sciences 2
- Publishing and Media 2
- Real Estate 2
- Recreational, Cultural, and Sporting Activities 2
- Agriculture and Forestry 1
- Engineering and Architecture 1
- Non Profit Organization 1
- Public Administration and Defense 1
- Transportation 1
- Utilities 1
Publication Types
- White Papers 1,818
- Blog Posts 881
- Case Studies 185
- Brochures 105
- Industry Reports 79
- Software Reviews 27
- Datasheets 21
- Buyer's Guides 13
- Accreditation Reports 7
- Microsoft 15
- Technology Evaluation Centers 6
- Maximizer 5
- Pronto Software 5
- Salesforce.com 5
- ServiceMax 5
- BigCommerce 4
- Habanero Consulting Group 4
- Strategy Companion 4
- InfusionSoft 3
- Nucleus Research 3
- SAP Sales Cloud (formerly CallidusCloud) 3
- AppFinity Software Corp. 2
- Cameleon Software 2
- Cincom Systems 2
- Everest Software Inc. 2
- GeoMetrix Data Systems 2
- Huron Consulting Group Inc 2
- Moxie Software 2
- Nimble Storage 2
- ProjectLocker 2
- Riverbed Technology 2
- Know the option you’re looking for? Type in the search field.

Top Publications

Understanding Data Mining With the Help Of Case Studies On Data Mining In Market Analysis
Data | 0 comments
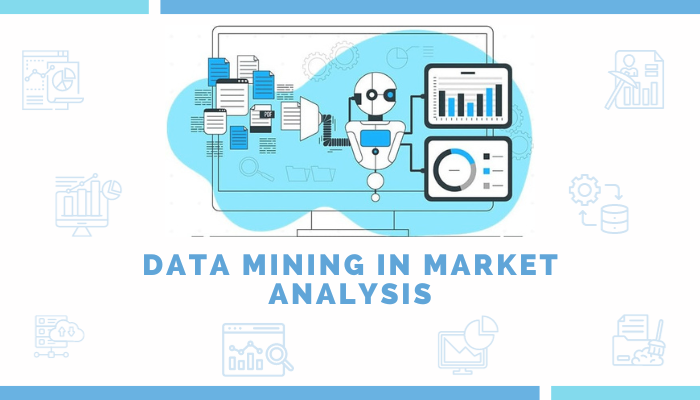
The modern generation of the 21st century witnessed a massive growth of businesses and companies around the globe. Every other day you would find a business lifting its head into existence. The arrival of so many businesses together leveled up the game of the competition. The competition is for the sense of existence in the market. One of the major technologies that gained immense popularity and is growing rapidly among businesses is Data mining.
Data mining technology assists the various companies to look into the compilation of huge quantities of data they brought together and use them to develop collaboration and improve relationships to boost its efficiency. It helps them gather accurate and detailed information about customers, which helps them strategize an influential plan and make better decisions.
Let us first understand what Data Mining is before we delve deep into the case studies in data mining applications or the various data mining case study examples.
What Is Data Mining?
Data mining is a mechanical tool used by companies that helps extract all the information from a compilation of data. Such information helps in making predictions and acting as per the same. Various statistical and mathematical calculations are used to remove the cover from relations and trends among the huge quantities of data stored in the company database. Data mining is a perfect combination of statistics, data warehousing, artificial intelligence technology, and machine learning.
Statistics resembled the starting point of data mining. Regression analysis, standard deviation, and variance are the statistical functions that act as tools in facilitating people’s study of relationships between data and their reliability. Statistics is one of the pillars of data mining technology, as most data mining actions function according to it.
Data warehouses saw their birth in the 1970s when they used large mainframe systems and the COBOL techniques of programming to store data. All these saw the arrival of big databases that we now know as data warehouses. These warehouses are for the management, retrieval, and storage of data. Megabytes and terabytes of data get data management systems reflecting sophistication. Such storage is an integral part of data mining as it helps the company to manipulate organized data.
Artificial intelligence is another basic pillar of data mining like data warehouses and statistics. The beginning of artificial intelligence in the 1980s gave a set of algorithms with a design to help the computer learn by itself. The passage of time helped massive development that led to algorithms becoming data manipulation tools, with applications in large sets of data.
Data mining collaborated with artificial intelligence instead of applying an early-defined hypothesis that helped generate relationships between the data. Artificial intelligence helped analyze the data and find collaborations between the data, thus developing models to help the developers assume diverse relationships.
Artificial intelligence paved the way for machine learning. Experts define machine learning as the machine’s capability to improve its performance after assessing its earlier results. Machine learning comes right after artificial intelligence as it works towards bringing together the study of trial and error with the help of statistical analysis. It provides an opportunity for the software to learn things by itself and makes all the data without any external help.
Tasks Of Data Mining

Classification
Classification refers to a procedure of finding out a model that explains the classes and concepts of data. The primary objective of classification is to predict the class objects whose identities are still behind the curtain. This derivation of this model works on the analytical results of the training sets of data.
You can understand it better with the following example;
- Political parties are assigning the voters in their known buckets.
- Including new customers into an existing customer group.
When we talk about statistical modeling, regression analysis comes first in discussion. It refers to a statistical process for estimating the relationship among the diverse variables. Regression comes with a lot of analyzing and modeling techniques for many variables. Here the spotlight is on the relationship between a dependent variable and one or more than one independent variable.
Following are some examples;
- Predictions concerning the unemployment rates for the following year.
- Making estimates about the insurance premium.
Detection Of Anomaly
Anomalies refer to the problems and issues within the software. This is a process of identifying the events, items, or observations that do not fall in line with the patterns or items expected in the dataset.
Example: Fraud transactions in your credit card account.
Time Series
A time series refers to a series of data points that are in a list, graph, or index, depending on the order of time. Commonly speaking, time-series signifies the action sequence taken along successive points of time, placed at regular intervals. Therefore, it is a discrete sequence of data.
Example: Production forecasting, forecasting of sales, etc.
Clustering refers to sorting the objects into diverse groups, where each group would consist of objects having similar characteristics. The features of the objects in one group will differ from the objects in another group. Following is a static example of clustering;
- Searching the customer segments in a company, depending on their transactions, customer calls, and web.
Analysis Of Association
Association is one of those data mining functions that finds out the possibility of an item recurring while in a collection. Association rules explain the rules of relationship between the items that co-occur.
Example: Search for the various opportunities for cross-selling for a retailer, as per his transaction history.
Expanding And Exploring Business
As we already know, data mining refers to a process in businesses where large chunks of data get explored to find meaningful rules and patterns. Companies can use data mining to gain that competitive edge over their fellow companies and push their business to better heights.
History Of Data Mining
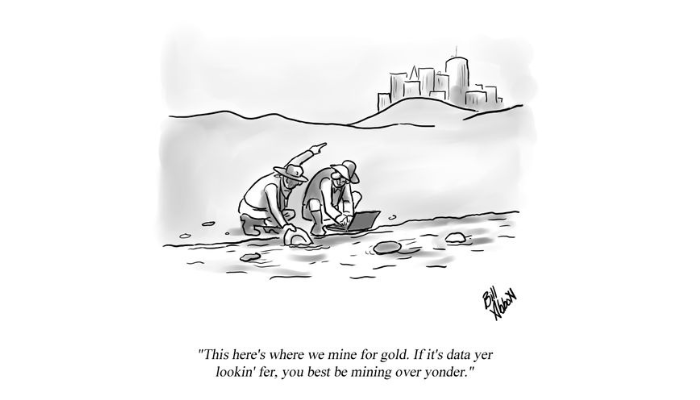
You might feel that data mining is a new concept. This term might be a new one, but the concept has been around for quite a few years. Classical statistics, artificial intelligence, and machine learning together led to the development of data mining . Everything began in the 1960s when the concept of data collection surfaced. It refers to the storage of data and information in computers. Tapes, disks, and computers were the technology available during that time.
Next, we saw the arrival of the concept of Data access in the 1980s. The concept of data access brought the introduction of relational databases and structured languages for the query. Both these helped in educating us humans more about data. Dynamic availability of data at a record level came with Data access.
Decision reports and Data warehousing came in the 1990s, which unveiled the procedure of management and retrieval of centralized data. This came with the following characteristics;
- Maintenance of a central address for keeping all the data concerning the organization.
- It will help you in analyzing the data and concentrating on the specific characteristics.
- Dynamic delivery of data at multiple levels.
The present data mining is all about making predictions and generalizing the patterns.
Influential Events And Personalities In Data Mining
Data mining saw the inception of its mention in the hands of John Herry Holland in 1975, who wrote the book “Adaptation in Natural and Artificial Systems.” This book was a thesis on genetic algorithms. However, the term “Data Mining” came into the limelight in the 1990s, with its mention in the database community for the first time. Moving further, William S . Cleveland brought forward Data mining as an independent concept in 2001. Data mining gained its epitome of prominence in February 2015, when The White House of the United States Of America hired D.J. Patil as their data scientist.
Methods Of Analysis In Data Mining

There are different methods of analytical observation in Data mining. They are as follows;
Artificial Neural Networks
These are the non-linear models of prediction that have a stark resemblance with the neural networks in biology when it comes to structuring.
Genetic Algorithms
Genetic algorithms refer to optimizing that utilizes the combination of genetics, natural selection, and mutation based on the concept of natural evolution.
Induction Of Rules
The application of all the rules found via extraction is the ones found from the significance of statistics.
Decision Trees
These are structures with the shape of trees that help in showing a set of decisions. These decisions help in generating rules for classifying a dataset.
- Chi-square Automatic Interaction Detection (CHAID), Classification and Regression Trees (CART).
- Classification and Regression tree works towards segmentation of the dataset by creating a spill that goes two ways.
- Chi-square Automatic Interaction Detection uses the chi-square tests to create splits in multiple directions.
Method Of Nearest Neighbour
The nearest neighbor technique works towards classifying each record within a dataset. It depends on combining the classes of the k-records that have the highest similarity with the dataset. (K=1)
Visualization Of Data
- Data visualization refers to the interpretation of complex relationships via visuals within data with multi-dimensions.
- One of the substantial examples is the usage of graphical tools for the illustration purposes of data relationships.
Also Read: Top 10 Data Mining Techniques for Business Success
Application Of Data Mining
You can establish data mining for your company via the feature of modeling. Modeling refers to the act of building a model that has an application to a particular situation, following which you can use that model in a different situation that does not have any existing model. Such models help you in making predictions regarding the patterns.
Data Mining In The Field Of Marketing
Companies garnered huge benefits and returns in their marketing area when considering data mining and working on its advancement. Companies use all the data gathered via data mining to push their efforts towards tailoring their discount coupons, and gift vouchers and working on their sales and advertisements to target their customers.
When you devise your marketing strategy through such mined data, you can devise a better marketing strategy and increase your sales effectiveness. Your company will also save a lot of money depending on such information.
Case Studies On Data Mining
Let us now go through the various case studies of data mining applications that will help us understand the importance of this procedure in various companies and businesses;
Case Study No.1: Target
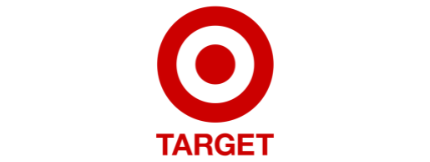
The first data mining case study example is that of the company named ‘ Target .’ Target vouchers use data mining for tailoring their discount coupons. They hope that sending such discount coupons to the customers will make them buy their products regularly. The strategists of Target assume this as an effective mechanism to prevent the customers from changing their loyalties to other brands or companies.
- When most customers are vulnerable to changing brand loyalties , due to varied choices and changing preferences. Like in tech sphere, innovative features like longer battery life and a more advanced camera system can be some factors.
- The stores like Target try to utilize such time to exploit the opportunity and lure customers into making purchases from their brand. Not just temporarily, but they try to engage them till the very end.
The company puts all its data into useful, which they collect while you are in its store for purchase or on its online website for buying something. They also collect such data by purchasing it from other companies. Duhigg states that Target has been collecting data for decades through the customers who walk into their stores regularly. They assign distinctive, unique codes to diverse customers from time to time.
This unique code is the Guest ID number within the working of Target, which keeps track of everything they purchase. Later, they use this very data to analyze the tastes and preferences of the customers and take effective steps to boost their marketing strategy.
Andrew Pole, an analyst, started his analysis of the “Pregnancy prediction model” by going through the history of the company’s baby shower registry. He tried to use this data to predict a woman’s changes regarding their shopping habits when they are expecting a baby. He formulated a list of 25 items based on this information to determine whether a woman is pregnant or not. This model successfully predicted whether a customer was pregnant or not but also assumed the date of delivery.
Case Study No.2: Amazon
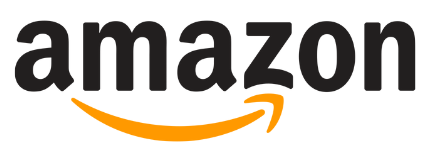
Here we are going to talk about Amazon . The case study of Amazon is one of the best case studies on data mining in market analysis. Amazon tries to use all the data they mined so that they can improve their customer service. The data mined consists of the customer’s name, home address, and personal details. Such data also consists of the customer’s preferences and the issues they are dealing with to find a solution.
They try to collect all the data regarding the customer from the various departments of the firm. Once they have all the necessary data, they synchronize and compile them for sending them to the human representative. The human representative then uses the data to have a great personalized conversation with the particular customer.
The employees of the customer service department of Amazon have all such needful information in their hands. This adds to making the conversation with the customer a lot more convenient. The employees of customer service have enough information about you which helps them make the conversation personal. However, there are no worries about the conversation being creepy.
Also check: Amazon AWS data partner marketplace.
Case Study No.3: Starbucks

Starbucks is one of the leading coffee shops with innumerable branches around the globe. Their case study will be a perfect example of the case studies on data mining in market analysis. Starbucks indulges in data mining to determine the perfect locations for setting up its stores. Tactics of Data mining and modeling assist the numerous Starbucks locations within proximity.
They try to analyze the data based on the locations, the population composition of the location, and traffic in the streets around to predict whether setting up a store there will be successful or not. Starbucks seeks assistance from a data platform named ArcGIS, developed by a company named Esri. They help them gather all the necessary information about the concerned location, demographic structure, the presence of Customer homes around, work, and other outings. All these data supplement the monitoring and boosting of their sales.
This particular company named Esri gathers a lot of data from Starbucks and, after ingrained analysis, positions them on platforms that are easily understandable for the employees there.
Case Study No.4: Usage Of Association Rule Mining In the Systems Of Recommendation
Recommender systems gained immense popularity among various fields of the industry at the current time. Music, movies, books, search queries, research books, social tags, etc., are widely-known fields. These recommendation systems assist enterprises by combining ideas from intelligent systems, information retrieval, and machine learning to make assumptions regarding the customer’s behavior. Recommender systems have two distinct approaches for their functioning;
Collaborative Filtering
The method of collaborative filtering indulges in collecting and analyzing a huge chunk of information regarding the user’s preferences, behavior, activities, etc. this will help them predict the like of a user in accordance with the other users. One of the approaches here is the usage of the Apriori algorithm.
Here you would know how the Apriori algorithm is used to squeeze out data concerning association rules from the user profiles. PVT is one of the prominent examples in this regard. PVT is one of those recommender systems that recommend various TV channels to the viewers, depending on their viewing experience. Channels with both positive and negative reviews work under the management of PVT. It treats TV viewers as transactions and the program ratings as itemsets.
One can use the Apriori algorithm to find out a set of rules and attached confidence levels between the programs. The confidence values resemble the similarity scores, and the system uses them to fill a program similarity matrix. There is an initiative to create a bridge between two TV shows. One who watches Splitsville or Roadies will not take an interest in shows like Kaun Banega Crorepati. However, if there is a line drawn between MTV Spitsvilla and Kaun Banega Crorepati, it will result in pattern watching.
Case Study No. 5: Model Of Classification For Selection of Targets In Direct Marketing
Historical data of purchase helped develop a prediction model of response along with data mining techniques. This development was to make predictions about whether a customer of the Ebedi Microfinance Bank of Nigeria would revert to a promotional offer or not. Data mining techniques helped develop a prediction model with the help of data regarding the customer’s purchase history. The data found its storage in a data warehouse to assist the decision of the management. The customer’s purchases in history and his demographic dataset helped in formulating a response model.
Then the development of the model took inputs from the following purchase variables;
Recent Purchases
It refers to the number of months from the time of the first purchase till the time the customer made the last purchase. It is one of the most powerful weapons to predict whether the promotional offer will succeed or fail. It is quite a logical observation. The primary statement through this point is that if you made some recent purchases, you are more likely to respond to the offer as against the fact that your last purchase was way back.
Frequency stands for the number of purchases the customer made. This data concerning the number of purchases can be within a definite period or all the purchases to date. This characteristic feature comes second to the factor of recent purchases when it comes to making predictions.
Monetary value
Monetary value resembles the total amount spent by the customer on making purchases from the company. You can draw some resemblance with frequency as such data can have a definite period or the total money spent to date. It is the least favorable tool to predict the steps of the customer.
However, when all three characteristic tools come together, it can sharpen the chances of the prediction being correct.
The customer’s demographic information includes his features and details concerning his sex, postal address, age, occupation, etc. The Bayesian algorithm, rather than the Naïve Bayesian algorithm, was a primary ingredient in constructing the classifier system. The selection techniques of both wrapper and filter features were in an application for selecting the inputs of this model.
The data results revealed that the Ebedi Microfinance Bank of Nigeria could plan effective strategies for marketing their goods and services. They can do it by making a detailed report on the status of customers that will guide their path to making correct decisions regarding the disbursement of funds. Therefore, they can use those funds on useful marketing tactics rather than waste them on failed strategies.
Read More: Case Study: Walmart

The Future Of Data Mining
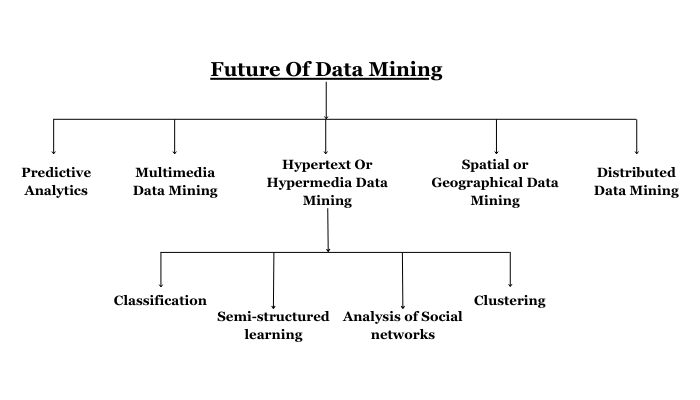
You can perceive the future of data mining through the following characteristics;
Predictive Analytics
Predictive analytics states that you can achieve “one-click data mining” through a simple and more efficient data mining process.
- You should allow the application of advanced analytics across various subjects.
- The area that will bring the highest revolution will be medicine. The researchers can analyze prediction to determine the factors associated with a particular disease and what medicine might work wonders on the affected patient.
Distributed Data Mining
Distributed data mining refers to the act of mining data spread across different locations. Combine the facilities of local data analysis and a global data model to get the best results out of data mining.
Hypertext Or Hypermedia Data Mining
This type of data mining includes hyperlinks, texts, marked texts, and any other form of information related to hypermedia. It has the following techniques;
- Classification.
- Clustering.
- Semi-structured learning.
- Analysis of Social Networks.
Multimedia Data Mining
The data from Multimedia data mining includes multimedia like videos, images, animation, audio, etc. This form of data requires a separate representation compared to traditional data.
Spatial or Geographical Data Mining
Data mining consisting of space or geography includes the analytical information concerning satellite images, natural resources, and all data from topography. All of the data comes from diverse locations, with most of them being pictures.
Things That Bother About Data Mining
There are a few drawbacks or rather concerns that experts have regarding data mining. They are as follows;
Assurance Of Privacy
Data mining is gaining immense popularity and momentum among various industries. This results in the collection of more information about every individual. When such accurate and personal information comes to the public domain, the chances of exploitation increase by manifolds. Some people or applications can use this information to meet their narrow desires. So you can very well understand that data mining allows easy accessibility of data, thus exposing it to the ills of exploitation. People can steal your identity and use it to fraud someone else.
Issues With User Interface
The experts dealing with data mining studies are skeptical about whether visualization tools can expose the true knowledge any data holds. The chances of people understanding any visual data and discovering the true meaning are difficult at times.
Problems Concerning The Performance Of Data Mining
When we talk about data mining tools, numerous statistics, and other analytical methods had a design for discovering smaller quantities of data. Such tools might fail to accommodate the rising gravity and depth of information.
There is no guarantee that the collection of data and its mining will mitigate the risks in the future. Therefore, data mining is still not a flawless procedure.
The above information can help you better understand data mining through the various case studies in data mining applications. Data mining can be a boon for various industries as that will help them secure more relevant information about the customers and boost all strategies for garnering better customer experiences.
When we talk about the few drawbacks of data mining, various companies’ advanced technology and software developments can mitigate the risks. Moreover, no company will sacrifice its brand name for the sake of some cheap benefits. You can get the best data solution services from http://bizprospex.com , which can help you with their AML Sanctions List , PEP List , Data Appending , and Skip Tracing services to gather the best and most authentic data available.
Submit a Comment Cancel reply
Your email address will not be published. Required fields are marked *
This site uses Akismet to reduce spam. Learn how your comment data is processed .
Don't miss updates from us!
Test us free, maybe.
We are very sure of our services and quality of work. How sure if you may ask?
We offer 100% money back with a 10-hour free trial.*
Recent Posts
- Why does your business need a big data marketplace?
- Exploring the role of data marketplace in your business’s success
- Leverage data to secure funding for your business
- Using data analytics solutions for business decision-making
- How jobs feed data can unlock market trends?
- CASE STUDIES (6)
- Data mining (31)
- data solution (13)
- marketing (26)
Free sample data available
Process Mining for Analyzing Customer Relationship Management Systems: A Case Study
- First Online: 29 July 2018
Cite this chapter
- Ahmed Fares 3 ,
- João Gama 3 , 4 &
- Pedro Campos 3 , 4
Part of the book series: Studies in Big Data ((SBD,volume 41))
3 Citations
Process Mining aims to discover and evaluate As-Is processes from sets of sequential events, by examining different instances of the same process and building models that can detect patterns and behaviors. In the meanwhile, organizational perspective is being considered in Process Mining by taking advantage of the ability to extract social networks that represent different kinds of relations between resources performing the process. The case study tries to describe how Process Mining could be applied in order to detect and improve “Customer Relationship Management” process and extract some kind of social networks that represent the relations between the employees(resources) of National Institute of Statistics of Portugal (INE) using event logs.
This is a preview of subscription content, log in via an institution to check access.
Access this chapter
- Available as EPUB and PDF
- Read on any device
- Instant download
- Own it forever
- Compact, lightweight edition
- Dispatched in 3 to 5 business days
- Free shipping worldwide - see info
- Durable hardcover edition
Tax calculation will be finalised at checkout
Purchases are for personal use only
Institutional subscriptions
Van der Aalst, W.M.P.: Process Mining: Discovery, Conformance and Enhancement of Business Processes. Springer, Berlin (2011)
Book Google Scholar
ProM: Prom 6 tutorial. http://www.promtools.org/prom6/downloads/prom-6.0-tutorial.pdf (2010)
Van der Aalst, W., Song, M.: Mining social networks: uncovering interaction patterns in business processes. In: International Conference on Business Process Management. Springer, Berlin (2004)
Google Scholar
Mans, R.S., Schonenberg, M.H., Song, M., et al.: Application of process mining in healthcare : a case study in a Dutch hospital. Biomed. Eng. Syst. Technol. 25 , 425–438 (2009)
Article Google Scholar
van der Aalst, W.M.P., Reijers, H.A., Weijters, A., et al.: Business process mining: an industrial application. Inf. Syst. 32 (5), 713–732 (2007)
INE: Statistics Portugal. https://www.ine.pt/xportal/xmain?xpgid=ine_main&xpid=INE (2015)
Buijs, J.C.A.M., van Dongen, B.F., van der Aalst, W.M.P.: On the role of fitness, precision, generalization and simplicity in process discovery. In: OTM 2012: On the Move to Meaningful Internet Systems: OTM 2012, pp. 305–322. Springer, Berlin (2012)
Weijters, A.J.M.M., Ribeiro, J.T.S.: Flexible heuristics miner (fhm). In: IEEE Symposium on Computational Intelligence and Data 565 Mining (CIDM), pp. 310–317 (2011)
Van Eck, M. L., Buijs, J.C.A.M., van Dongen, B.F.: Genetic process mining: alignment-based process model mutation. In: Business Process Management Workshops, pp. 291–303. Springer International Publishing, Cham (2015)
CROSS, R.: Knowing what we know: supporting knowledge creation and sharing in social networks. Organ. Dyn. 30 , 100120 (2001)
Song, M., van der Aalst, W.: Towards comprehensive support for organizational mining. Decis. Support. Syst. 46 (1), 300317 (2008)
Download references
Acknowledgements
This work was supported by the research project TEC4Growth—Pervasive Intelligence, Enhancers and Proofs of Concept with Industrial Impact/NORTE-01-0145-FEDER-000020, , North Portugal Regional Operational Programme (NORTE 2020), under the PORTUGAL 2020 Partnership Agreement, and through the European Regional Development Fund (ERDF) and the ERDF European Regional Development Fund through the Operational Programme for Competitiveness and Internationalization—COMPETE 2020 Programme within project POCI-01-0145-FEDER-006961, and by National Funds through the FCT Fundao para a Cincia e a Tecnologia (Portuguese Foundation for Science and Technology) as part of project UID/EEA/50014/2013.
Author information
Authors and affiliations.
LIAAD-INESC TEC, Porto, Portugal
Ahmed Fares, João Gama & Pedro Campos
Faculty of Economics, University of Porto, Porto, Portugal
João Gama & Pedro Campos
You can also search for this author in PubMed Google Scholar
Corresponding author
Correspondence to Ahmed Fares .
Editor information
Editors and affiliations.
Institute Mines-Telecom Lille Douai, Douai, France
Moamar Sayed-Mouchaweh
Rights and permissions
Reprints and permissions
Copyright information
© 2019 Springer International Publishing AG, part of Springer Nature
About this chapter
Fares, A., Gama, J., Campos, P. (2019). Process Mining for Analyzing Customer Relationship Management Systems: A Case Study. In: Sayed-Mouchaweh, M. (eds) Learning from Data Streams in Evolving Environments. Studies in Big Data, vol 41. Springer, Cham. https://doi.org/10.1007/978-3-319-89803-2_9
Download citation
DOI : https://doi.org/10.1007/978-3-319-89803-2_9
Published : 29 July 2018
Publisher Name : Springer, Cham
Print ISBN : 978-3-319-89802-5
Online ISBN : 978-3-319-89803-2
eBook Packages : Engineering Engineering (R0)
Share this chapter
Anyone you share the following link with will be able to read this content:
Sorry, a shareable link is not currently available for this article.
Provided by the Springer Nature SharedIt content-sharing initiative
- Publish with us
Policies and ethics
- Find a journal
- Track your research
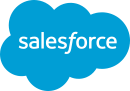
Salesforce is closed for new business in your area.
Thank you for visiting nature.com. You are using a browser version with limited support for CSS. To obtain the best experience, we recommend you use a more up to date browser (or turn off compatibility mode in Internet Explorer). In the meantime, to ensure continued support, we are displaying the site without styles and JavaScript.
- View all journals
- My Account Login
- Explore content
- About the journal
- Publish with us
- Sign up for alerts
- Open access
- Published: 27 May 2024
Coupling control technology of anchoring and unloading in deep intense-mining and large-deformation roadway: a case study
- En Wang ORCID: orcid.org/0000-0002-5455-3116 1 , 2 , 3 ,
- Shuaifeng Yin 1 ,
- Qingtao Kang 1 ,
- Xubo Zhao 1 ,
- Qiankun Lan 1 ,
- Hongyuan Sheng 1 &
- Huiyang Liang 1
Scientific Reports volume 14 , Article number: 12075 ( 2024 ) Cite this article
Metrics details
- Civil engineering
- Engineering
In order to control the deformation of surrounding rock in deep high-stress and intense-mining roadways, taking a deep coal roadway with continuous deformation as an example, the characteristics of crustal stress, coal strength, and mining influence of roadway are obtained by underground tests. The combined failure mechanism of coal roadway surrounding-rock is revealed by differential stress of deep and shallow anchor cables. We propose that the improvement of surrounding rock control for coal roadway is adopting the coupling control technology of anchoring and unloading. The stress distribution and evolution laws of lateral surrounding rock of unloading holes are obtained by numerical simulation and theoretical calculation, and reasonable unloading-hole spacing of 4.0 m is comprehensively determined. A mechanical model of roadway roof beam under fixed support at both ends is constructed and the important role of anchor cable beam-truss in controlling the stability of coal roadway is obtained. The rationality of coupling control technology of anchoring and unloading and parameters has been verified by engineering test and mine pressure observation, providing technical references for surrounding rock control in deep intense-mining and large-deformation roadways.
Introduction
After coal mine enters deep mining, it often leads to large deformation and damage of roadway surrounding-rock, which seriously restricts the safe and efficient mining of deep coal resources 1 , 2 , 3 . The control of large-deformation roadways in deep coal mines has always been a hot research topic. Exploring appropriate control technology in deep high-stress and complex conditions plays an important role in achieving the stability of roadway surrounding-rock 4 .
Deep does not mean that the depth, but a mechanical state, which is jointly determined by surrounding rock properties, mining stress and its crustal stress. It refers to the depth of nonlinear physical and mechanical phenomena exhibited by engineering coal and rock masses and the depth range below it 5 , 6 , 7 . In terms of failure mechanism of surrounding rock in deep mining roadway, Xue et al. 8 revealed that stress evolution and crack development of roadway surrounding-rock are influenced by weathering and horizontal structural stress, and elucidated the asymmetric distortion characteristic of roadway. Yang et al. 9 , 10 analyzed the characteristics of deformation, stress, and crack propagation in deep and soft rock-roadway under deviatoric stress, and elucidated the evolution process of deformation and failure in roadway surrounding-rock. Li et al. 2 analyzed the main failure characteristic and mechanism of surrounding rock in deep thick-coal-seam roadway, revealing that the central roof is key control point of deformation and failure. Sun et al. 11 explored the micro mechanism of roadway failure, elucidated the relationship between deformation, rock dip angle, and the position of weak rock masses, and revealed the mechanism of deformation and failure in soft rock-roadway. Sahoo and Palei 12 come up with a novel control technology for roadway surrounding-rock by adopting risk-based maintenance. Scholars have also used deviatoric stress 13 , 14 , 15 , 16 to study the deformation and failure mechanism of coal roadway surrounding-rock. In terms of unloading and control technology for deep large-deformation roadways, Zang et al. 17 explored the layered failure characteristic of roadway from shallow to deep, and proposed a combined support technology of "bolt table mesh steel ladder" for deep roadway. Zhao et al. 18 proposed a support technology of shotcreting, grouting anchor bolt, anchor bolt, and grouting anchor cable for deep soft rock-roadway. Yao et al. 19 proposed a regional support technology for controlling large deformation of roadway passing through a fault. Scholars have put forward a variety of unloading technical measures such as borehole unloading 20 , 21 , roof cutting 22 , 23 , 24 , blasting 25 , hydraulic fracturing 26 , as well as asymmetric differential support technology 27 , 28 , 29 , 30 , 31 , 32 to resist large deformation of coal roadway surrounding-rock under complex conditions of deep high-stress and maintain the stability of surrounding rock in deep roadway.
Given the high stress and complex conditions in deep coal mines, it is difficult to control the deformation of surrounding rock in large-deformation roadways, and it is arduous to effectively control the roadway displacement using a single strengthening support or grouting modification technology. Based on the test of crustal stress and coal strength in deep-mining coal roadway, combined with the differential stress characteristics of deep and shallow anchor cables, this study reveals the deformation and failure mechanism of surrounding rock migration of coal roadway, and puts forward a coupling control technology of anchoring and unloading for coal roadway surrounding-rock. The reasonable spacing of internal unloading-holes of coal roadway is comprehensively determined by numerical simulation and theoretical calculation. A mechanical model of roadway roof beam under fixed support at both ends is constructed, and the important role of anchor cable beam-truss structure in maintaining the stability of coal roadway is obtained. Reasonable coupling control parameters of anchoring and unloading are proposed. The important role of coupling control technology in maintaining the stability of deep-mining coal roadway has been verified by engineering test and mine pressure observation, providing technical references for the control of complex and large-deformation surrounding rock in deep-mining roadways.
Mine pressure characteristics in mining roadway
Taking the Dongpang Coal Mine of Jizhong Energy Co., Ltd., China as engineering project, this section introduces the engineering overview, deformation and failure characteristics, control difficulties, and mining characteristics of coal roadway. It further reveals the mechanism of continuous deformation and failure, and points out the improvement strategy of surrounding rock control in coal roadway.
Engineering overview and failure characteristics
The total thickness of No. 2 coal seam in Dongpang Coal Mine is 4.60–6.50 m, with an average of 5.40 m. The average inclination angle of the coal seam is 5°, and the average burial depth is 660 m. The direct roof is 2.32 m siltstone, the basic roof is 8.90 m fine sandstone, and the direct floor is 1.06 m fine sandstone. The chamber of centralized liquid supply pumping station of No. 12 mining area in No. 2 coal seam is a coal roadway with 5.0 m width and 3.0 m height. It is arranged along the roof of the coal seam and is 75 m away from the main track roadway in No.12 mining area and the design final-mining-line of 21,215 coal face. The coal around the roadway exhibits typical characteristics such as looseness and fragmentation, leading to significant deformation throughout the year. Expansion and repair are required every six months to a year, making it difficult to achieve long-term effective control of large-deformation coal roadway.
When not affected by the disturbance of 21,215 high-mining coal face, two ribs’ surrounding rock of coal roadway undergoes continuous deformation and damage all year round. A special construction team needs to be arranged to continuously expand and renovate it (Fig. 1 a) to maintain the basic operation of the roadway, resulting in high support costs. During the mining process of 21,215 high-mining coal face, the carriage roadway located at the same level between the designed final-mining-line of the coal face and coal roadway has been completely closed. The observation results of surrounding rock displacement in two ribs of haulage roadway in the front section of the coal face are shown in Fig. 1 b. During the mining process of 21,215 high-mining coal face, the displacement of both ribs within the range of 130 m in front of the coal face exceeds 1.0 m, and the deformation of both ribs at 75 m in front of the coal face exceeds 2.0 m. Therefore, due to the severe disturbance of 21,215 high-mining coal face, the mine pressure in roadway surrounding-rock beyond the front section of the coal face exhibits severe disturbance, and the mining influence range on surrounding rock far exceeds 130 m.
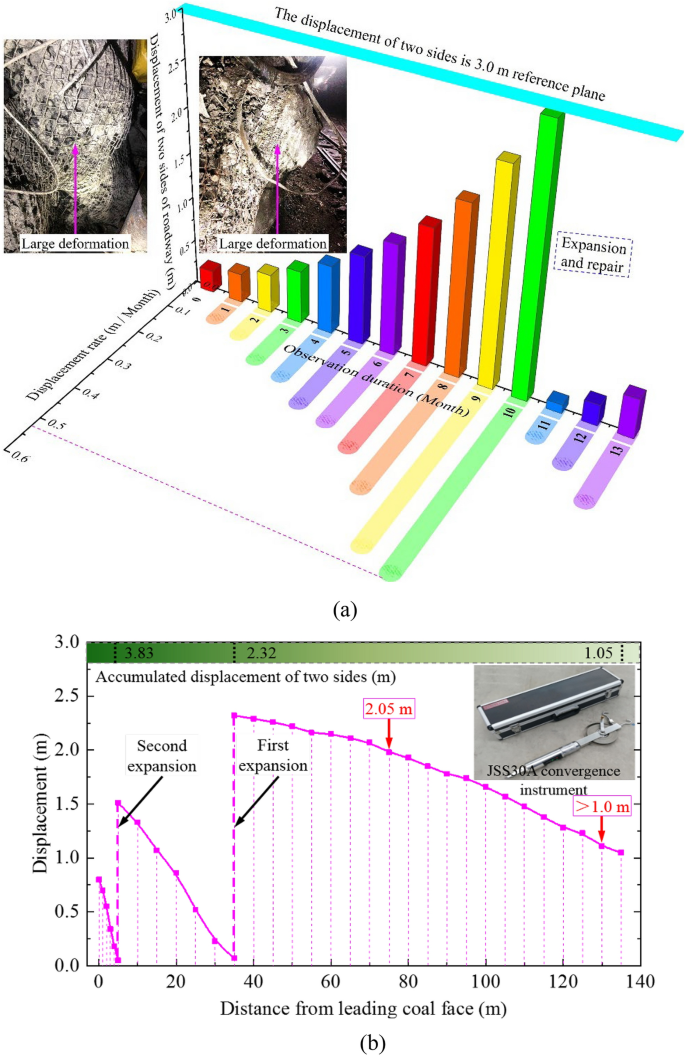
Observation results of roadway deformation around 21,215 coal face. ( a ) Deformation of roadway surrounding-rock without disturbance of coal face, ( b ) Roadway displacement at different positions ahead of the coal face.
Crustal stress and coal strength test of roadway
Mastering the stress environment of roadway surrounding-rock is necessary for analyzing its physical and mechanical properties of surrounding rock and simulating roadway stability. Therefore, two aspects of study on physical and mechanical characteristics of coal roadway are carried out: crustal stress test for deep coal roadway and physical and mechanical test for laboratory coal. It can provide measured data support for analyzing large deformation and failure mechanisms of coal roadway.
Crustal stress test
The crustal stress of surrounding rock near the coal roadway is measured by stress relief hollow inclusion method. Three typical measuring stations are selected near the coal roadway and hollow inclusions are arranged for crustal stress test. The drilling depth of each measuring point is 12 m, and the stress relief depth is 320 mm. The maximum horizontal principal stress around the coal roadway is 22.18 MPa, the minimum horizontal principal stress is 11.45 MPa, and the vertical stress is 16.72 MPa after averaging the crustal stress.
Coal strength test
The joints and fractures in No.2 coal seam of Dongpang Mine are extremely developed, and the coal structure is loose and fragmented. Almost all the original structure has been destroyed, showing significant characteristics of loose, soft, and fragmented in deep coal mines. The mechanical strength of 9 sets of samples is tested in the laboratory, and the results show that the maximum uniaxial compressive strength of the coal around the roadway is 8.763 MPa, the minimum is 2.632 MPa, and the average strength is 5.405 MPa. Therefore, the ratios of average coal strength around the roadway to three principal stresses mentioned above are 0.24, 0.47, and 0.32, respectively. The minimum principal stress has exceeded the compressive strength of coal roadway (up to 2.12 times), and the maximum principal stress is 4.10 times of uniaxial compressive coal-strength. Therefore, the coal roadway is prone to plasticization and destruction under deep and high ground stress.
Mining characteristics of coal roadway
The borehole stress test is adopted to dynamically monitor the mining stress of roadway surrounding-rock in mining process. Each measuring station is equipped with different numbers and depths of borehole stress meters (Fig. 2 a), and three-dimensional spatial stress of surrounding rock converted into the mining-stress concentration coefficient is shown in Fig. 2 b. The stress concentration factor is equal to the ratio of on-site measured stress to original borehole stress.
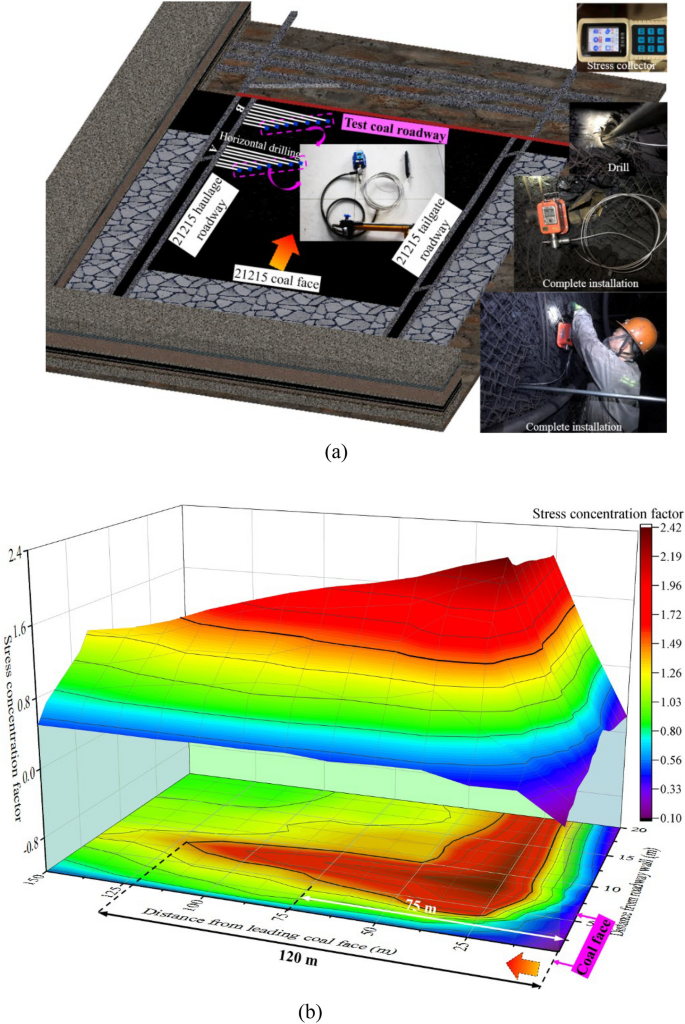
Observation and distribution in advance section of surrounding rock stress around 21,215 coal face. ( a ) Testing plan and process for borehole stress, ( b ) Spatial distribution of surrounding rock stress in advance section of the coal face.
There are two peak-stress concentration areas in surrounding rock of the front section of 21,215 coal face: (1) the peak zone of abutment pressure in front of the coal wall parallel to the coal face, about 26 m; (2) the peak zone of abutment pressure perpendicular to the coal face and parallel to the axial direction of the roadway. When the mining face reaches 150 m away from the measuring station, the roadway enters the disturbance influence-area; and when the mining face reaches about 120 m away from the measuring station, the roadway has obviously entered the mining influence-area. When the coal face is 75 m away from the measuring station, the stress concentration coefficient of the roadway is more than 1.60, indicating that surrounding rock at the position 75 m in front of the coal face is strongly influenced by the mining of large-mining-height coal face. Therefore, when the coal face is mined to the design final-mining-line (75 m away from the coal roadway), a larger range of coal roadway damage will occur under the intense-mining influence of the coal face with large-mining height. The intense-mining influence is an important characteristic of coal roadway at present.
Failure mechanism of coal roadway distortion
In order to explore the deformation and failure mechanisms of continuous large-deformation of two ribs in deep coal roadway, the dynamic observation of anchor cables stress in different lengths during continuous deformation was carried out by field test. The observation scheme is as follows: the anchor cables of the same height and different lengths (4.0–14.0 m) are arranged in two ribs of coal roadway, and the stress variation laws of anchor cables during surrounding rock deformation are continuously monitored. The results are shown in Fig. 3 .
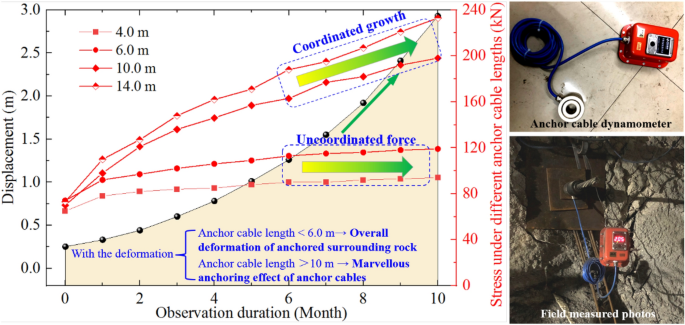
Stress curves of anchor cables with different lengths in the deformation process of roadway.
In the process of continuous deformation of two ribs in coal roadway, the stress variation laws of anchor cables with different lengths are completely different. (1) When anchor cable lengths are 4.0 m and 6.0 m, the stresses are not coordinated with surrounding rock deformation, that is, anchor cable stress maintains a relatively stable state in the process of continuous increase of surrounding rock deformation, which is obviously not synchronous and inconsistent with the mine pressure of roadway surrounding-rock. (2) When anchor cable length increases to 10.0 m, the stress also increases to a certain extent with continuous deformation process of surrounding rock. The anchor cables can anchor the surrounding rock and limit the deformation of coal roadway. (3) As anchor cable length increases to 14.0 m, the stress changes greatly compared with that when anchor cable length is 10.0 m, and the maximum stress can reach 238 kN, showing the coordinated characteristic consistent with the deformation trend of two ribs’ surrounding rock of coal roadway. It is concluded that anchor cable with length of 14 m plays a marvelous anchoring role in roadway surrounding-rock significantly.
In summary, when anchor cable length is small (< 6 m), the damage range of surrounding rock in both ribs of coal roadway exceeds the scope of anchor cable support. The surrounding rock in shallow anchoring rock of two ribs will be squeezed out as a whole by deep coal body, which means that shallow anchoring rock will undergo overall structural extrusion deformation. When anchor cable length is greater than 10.0 m, it can play a certain anchoring role, and when anchor cable length increases to 14.0 m, the anchoring effect is better. It can be concluded that the failure depth of surrounding rock in two ribs of coal roadway is large (beyond the support range of anchor cables), and the whole surrounding rock of shallow anchorage supporting structure is extruded, which leads to continuous large-deformation of surrounding rock. The joint failure mechanism of large deformation is revealed and formed, in which entire coal mass in the deep area migrates into the coal roadway and acts on the anchoring support system.
Improvement strategy for coal roadway control
The surrounding rock control of deep coal roadway in Dongpang Mine exists the following problems: (1) unclear understanding of deformation and failure mechanism; (2) unclear understanding of the interaction between surrounding rock and support; (3) the control idea of surrounding rock is not suitable. Based on the failure mechanism of continuous movement of coal in the deep area of two ribs, which acts on the anchoring surrounding rock to cause overall deformation, it is analyzed that only conventional strengthening support or grouting modification technology is no longer suitable for roadway surrounding-rock control. Starting from the essence and mechanism of continuous deformation and failure of surrounding rock in intense-mining roadway, this study proposes a coupling control technology of anchoring and unloading that can improve the stress environment of roadway surrounding-rock. The technical framework is shown in Fig. 4 .
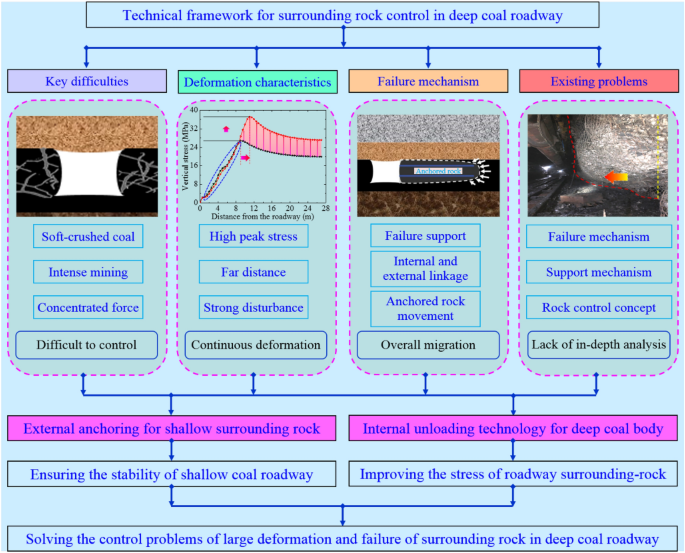
Technical framework of surrounding rock control in deep coal roadway.
In the coupling control technology of anchoring and unloading for deep coal-roadway surrounding-rock, anchoring refers to the comprehensive control measures such as strengthening support with anchor cable beam-truss and grouting modification in the shallow of coal roadway to improve the strength and bearing capacity of coal roadway surrounding-rock. And unloading refers to the implementation of cave-making and pressure relief technology in two ribs of coal roadway to transfer the high concentrated-stress to the deep, thereby improving the overall stress environment of coal roadway surrounding-rock.
Determination of internal unloading-hole spacing of coal roadway
Based on the determination of reasonable internal unloading-hole position, unloading-hole length and other key technical parameters, this section mainly analyzes the three-level classification and standard of unloading-hole position on stability of coal roadway, and comprehensively determines reasonable spacing of internal unloading-holes by theoretical calculation and numerical simulation.
Basic parameters of internal unloading for coal roadway surrounding-rock
In this section, a three-dimensional numerical model of FLAC for surrounding rock of deep coal roadway in Dongpan Mine is established, which is based on the Hoek–Brown criterion for processing laboratory rock mechanics and numerical simulation mechanics parameters 33 . The load on the upper model is 16.50 MPa, and the lateral pressure coefficient is 1.2. The Mohr–Coulomb model is used as the constitutive model of coal roadway deformation and failure. Based on the existing research 34 , 35 , the reasonable internal unloading parameters are determined as follows: (1) the unloading-hole position is 10.0 m away from the roadway wall; (2) the unloading-hole length is 5.0 m. This section mainly studies the response laws of unloading-hole spacing to the stability of coal roadway and determines reasonable unloading-hole spacing.
Based on the stress distribution, expansion, and evolution laws of surrounding rock under different internal unloading-hole positions and lengths in two ribs of coal roadway by numerical simulation, a three-level classification and standard for different unloading-hole positions are obtained (Fig. 5 ). (1) Good unloading zone: located within the peak-stress zone within 2.0 m of two ribs of coal roadway (the original peak position extends 1.0 m inward and 1.0 m outwards, forming Zone B), especially when unloading is carried out outside the original peak-stress line (near solid coal side), it can significantly transfer the original high-concentrated stress of roadway to a deeper depth, without affecting the stability of roadway surrounding-rock in shallow anchoring support. (2) Unloading failure zone: as unloading position is in the inner side of peak-stress zone (zone A) within the range of 2.0 m, the stress transfer effect of coal roadway surrounding-rock is not obvious, and it is easy to damage the integrity of shallow anchoring surrounding-rock of coal roadway, which is easy to induce the loss of bearing capacity of surrounding rock in two-rib anchoring area. (3) Insufficient unloading zone: as unloading position of two ribs is outside the peak-stress zone (zone C) within the range of 2.0 m, the magnitude and location of internal peak-stress are basically consistent with that before unloading, resulting in insufficient unloading of roadway surrounding-rock.
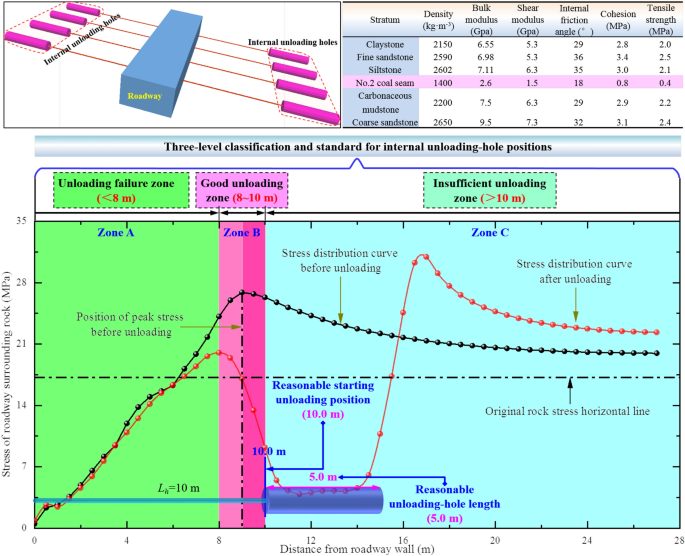
Numerical model and three-level division of different internal unloading-hole positions.
Numerical analysis of internal unloading-hole spacing
Based on determining reasonable internal unloading-hole position and length, the maximum shear stress of roadway surrounding-rock under different unloading-hole spacing is studied by numerical simulation, as shown in Fig. 6 . When unloading-hole spacing is less than 4.0 m, the hole surrounding-rock is in a low-stress state, which can ensure that anchoring surrounding rock of coal roadway is not deteriorated on the basis of realizing significant transfer of high concentration stress to the deep. It is conducive to the overall stability of internal unloading-holes and roadway surrounding-rock. As unloading-hole spacing increases to 5.0 m, a new high-concentrated stress zone appears between unloading holes, and the maximum concentrated stress increases by 27.68% compared with the spacing of 4.0 m. The surrounding rock between cavitation holes is inadequate pressure relief. When unloading-hole spacing is larger, the surrounding rock will induce secondary failure under the high stress, which is not conducive to the stability control of coal roadway surrounding-rock. Therefore, internal unloading-hole spacing should be ≤ 4.0 m.
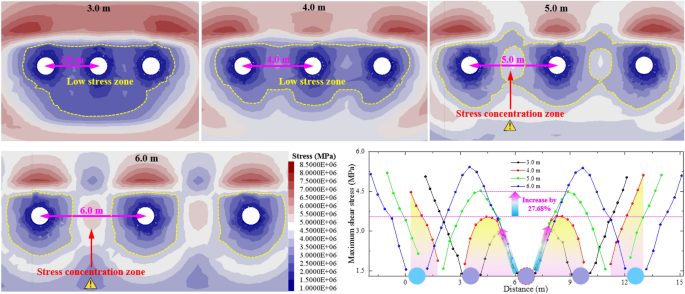
Stress distribution of surrounding rock under different unloading-hole spacing.
Theoretical calculation and analysis of internal unloading-hole spacing
Due to the complex boundary conditions of underground roadways in coal mines, which are heterogeneous, discontinuous, and nonlinear geometric bodies, it is currently impossible to accurately solve the stress state of surrounding rock using mathematical and mechanical methods. To simplify the calculation, it is assumed that surrounding rock in coal mine is homogeneous, isotropic, linear elastic, and without creep or viscous characteristic. The original rock stress is in an isotropic and isobaric state, and thus the plane strain problem 36 , 37 can be used to solve internal circular unloading-hole spacing. Figure 7 shows the stress distribution of unit body around internal unloading hole, which can be used to list the equilibrium equation of unloading hole as follows:
where, σ r and σ t are the radial stress and tangential stress of unloading-hole surrounding-rock, respectively. r and θ are the radius and coordinate angle of unit body, respectively.
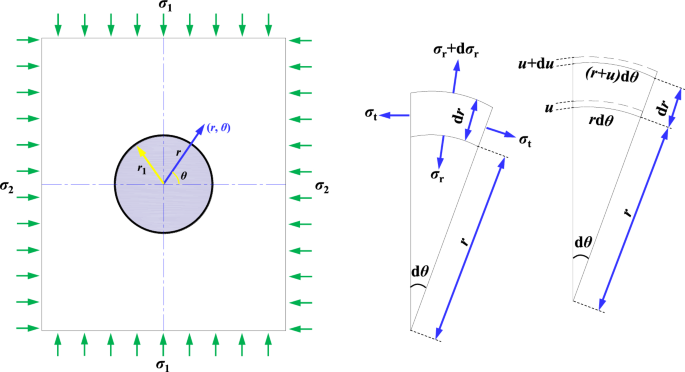
Stress distribution of unit body around internal unloading circular-hole.
Considering that the value of \(\frac{{{\text{d}}\theta }}{2}\) is very small, it can be approximated as \(\sin \frac{{{\text{d}}\theta }}{2} = \frac{{{\text{d}}\theta }}{2}\) , then:
The geometric equation for surrounding rock in circular unloading-hole is as follows: as radial deformation of unit body is in the process of u → u + d u , the expression of radial strain is:
The expression of tangential strain ɛ t resulting from r d θ → ( r + u )d θ in the course of tangential deformation of unit body is:
From the generalized Hooke's law:
where, σ z is the axial stress of surrounding rock of internal unloading-hole.
If the expression ( 2 ) and ( 10 ) are combined, σ 1 = γH , the expression of σ r and σ t at any point of unloading circular-hole can be expressed as follows:
where, r 1 is the radius of internal unloading circular-hole.
In Fig. 7 above, the static equilibrium equation of limiting equilibrium zone of surrounding rock of internal unloading circular-hole is:
The expression of limit equilibrium condition is:
where, σ r and σ t are the radial stress and tangential stress of surrounding rock of unloading hole in limit equilibrium zone, respectively. C and φ are the cohesion and internal friction angle of coal around unloading holes, respectively. r is the radius of surrounding rock corresponding to a point in limit equilibrium zone.
By substituting Eq. ( 14 ) into Eq. ( 13 ), we can get:
where, A is the integral constant. According to r = r 1 , there is σ r = 0, then:
The radial stress and tangential stress of surrounding rock of unloading hole in limit equilibrium zone can be expressed as follows:
Accordingly, the stress distribution in both ribs of circular hole to surrounding rock is shown in Fig. 8 .
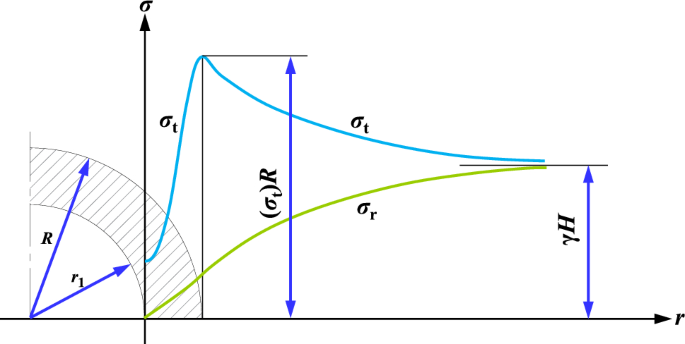
Tangential stress and radial stress distribution of lateral rock of circular hole.
Assuming that the in-situ stress of surrounding rock of unloading hole is the hydrostatic field, that is, λ = 1. At the boundary R of limit equilibrium zone, equation \(\sigma_{{\text{r}}} + \sigma_{{\text{t}}} = 2\sigma_{{1}}\) is followed. If \(\sigma_{{1}} = \lambda H\) , then:
According to the coal parameters and its internal unloading-hole around coal roadway of Dongpang Mine: unloading-hole radius r 1 = 0.5 m, coal bulk density γ = 25 kN/m 3 , coal seam burial depth H = 660 m, coal cohesion C = 0.8 MPa, internal friction angle φ = 18°. Substituting Eq. ( 23 ) above, the limit equilibrium zone range R = 3.21 m for surrounding rock of internal unloading hole is obtained, so the actual plastic zone distance r 2 = 2.71 m after excluding the radius of unloading hole.
Basic composition and micromorphology of high-water material
The segmented expression of tangential stress in lateral surrounding rock of internal unloading-hole of two ribs in coal roadway is: (1) the tangential stress in limit equilibrium zone in shallow part of unloading hole is shown in Eq. ( 21 ); (2) the tangential stress of surrounding rock in deep elastic zone is shown in Eq. ( 12 ). The Matlab software is used to obtain tangential stress of lateral surrounding rock at different position r and different spacing of unloading hole, as shown in Fig. 9 .

Abutment pressure distribution of surrounding rock under different unloading-hole spaces. ( a ) Unloading-hole spacing is large (≥ 13.08 m), ( b ) 13.08 m > Unloading-hole spacing ≥ 8.46 m, ( c ) 8.46 m > Unloading-hole spacing ≥ 4.25 m, ( d ) Unloading-hole spacing < 4.25 m.
From Fig. 9 , it shows that: (1) when internal unloading-hole spacing is too large (≥ 13.08 m), the surrounding rock load at central position between unloading holes is approximately a uniform distribution of in-situ stress and unloading effect is not ideal. (2) when internal unloading-hole spacing is large (13.08 m > spacing ≥ 4.25 m), the abutment pressure of surrounding rock located at central position between unloading holes is superimposed to a certain extent, and the stress in the middle is greater than the in-situ stress, which does not achieve effective pressure relief of unloading-hole surrounding rock. (3) as internal unloading-hole spacing is less than 4.25 m, there is a single-peak distribution of abutment pressure between adjacent unloading holes, and the superimposed abutment pressure between unloading holes is less than the in-situ stress, thus achieving effective pressure relief of coal roadway surrounding-rock and internal unloading holes. At the same time, based on the progress of internal excavation during on-site engineering construction, it has been comprehensively determined that the reasonable spacing between internal unloading-holes should be less than 4.25 m and should not be too small.
Reasonable internal unloading-hole spacing
In summary, it has been found that when the spacing between internal unloading-holes is greater than 5.0 m by numerical simulation, there is a clear peak-stress area near unloading holes, and the large concentrated stress makes unloading-hole surrounding-rock not fully depressurized. As unloading-hole spacing is 2 ~ 4 m, the surrounding-rock is in a low stress environment, which can achieve marvelous pressure relief effect. Meanwhile, combined with distribution laws of lateral abutment pressure in surrounding rock of unloading circular-hole obtained by theoretical calculation, it is concluded that reasonable internal unloading-hole spacing in two ribs of coal roadway should be less than 4.25 m. The reasonable internal unloading-hole spacing is determined to be 4.0 m combined with on-site construction and comprehensive economic benefits of the coal mine.
Engineering test
Based on determining reasonable internal unloading parameters, a rock-beam mechanical model is constructed under fixed support at both ends of coal roadway roof. The stability of coal roadway surrounding-rock under different support forms is solved, and the important role of anchor cable beam-truss in controlling surrounding rock deformation is elucidated. A coupling control technology of anchoring and unloading of coal roadway is proposed, and engineering tests are conducted on the stability control of roadway surrounding-rock in Dongpang Mine to verify the rationality of coupling control technology of anchoring and unloading and parameters.
Analysis of stress and bending moment of roadway roof under different supports
In order to accurately construct the mechanical model of coal roadway roof-beam with fixed supports at both ends, the following assumptions should be made: (1) the roof is a beam structure model with fixed supports at both ends; (2) the roof is the homogeneous rock mass bearing dead weight; (3) the roof strata are continuous materials without gaps; (4) the roof has isotropic characteristic along different directions.
The roof beam with fixed support at both ends belongs to statically indeterminate structure problem, which can be solved by force method or displacement method in structural mechanics 38 . Among them, the displacement method takes the nodal displacement of model structure as an unknown quantity to solve the model stress state. The classical equation of displacement method is as follows:
where, Z i and direction represent the key displacement and direction of original structure; r ij is the additional constraint reaction in Z i direction caused by the element displacement Z j = 1, also known as stiffness coefficient; R iP is the additional constraint reaction in Z i direction caused by load, also known as free term.
According to the calculation expression of bending moment at the end of the statically indeterminate bar of constant section by displacement method, the stress and bending moment of coal roadway roof-beam under different supports are obtained. The stress and bending moment of roof rock-beam supported by single anchor cables and reinforced ladder beam are shown in Fig. 10 .
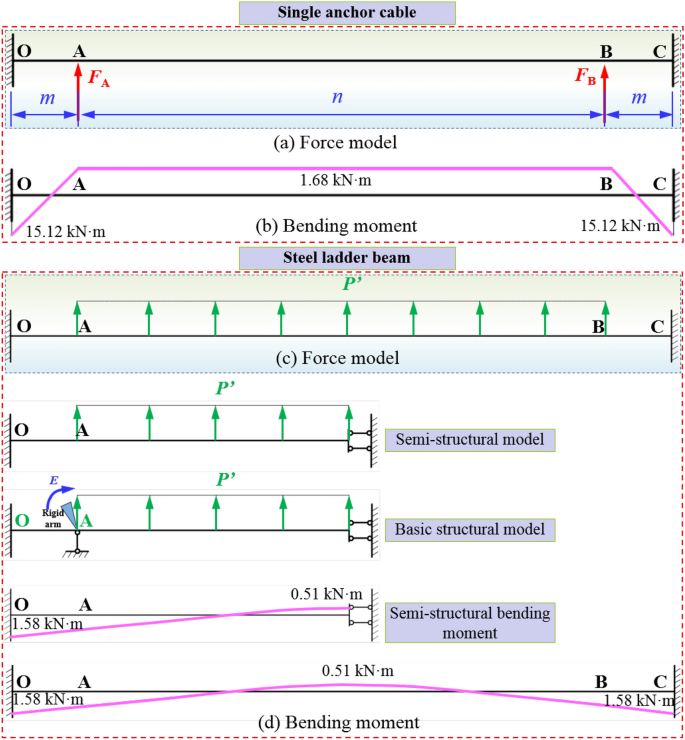
Force and bending moment of single anchor cables and steel ladder beam in roadway roof.
The support system of anchor cable beam-truss is mainly composed of anchor cables, double steel ladder beams and square tray. A comparison diagram of stress and bending moment of roof rock-beam in coal roadway under the conditions of anchor cable beam-truss, single anchor cables and no support is obtained, as shown in Fig. 11 . Under the condition of fixed support at both ends of coal roadway roof with no support, the maximum bending moment is located on both ribs of fixed side section, the maximum bending moment is 43.50 kN·m, and the middle section bending moment of the roof is 21.75 kN·m. The bending moment of the roof is greater than the other two types of support. When single anchor cables are adopted on both ribs of the roof, the maximum bending moment at fixed side support of the roof is 28.38 kN·m, and the bending moment at middle roof section is 20.04 kN·m. The bending moment is reduced to a certain extent compared with no support. When the roof adopts the anchor cable beam-truss support, the roof bending moment on both ribs of fixed support and the central are reduced, the maximum bending moment at fixed support on both ribs is reduced to 26.80 kN·m, and the bending moment of rock-beam in the middle roof is reduced to 19.56 kN·m. Both ribs of roof support and bending moment at the central cross section are significantly reduced, which is conducive to maintaining the roof stability of coal roadway, and verifies the important role of anchor cable beam-truss system in controlling the roof stability.
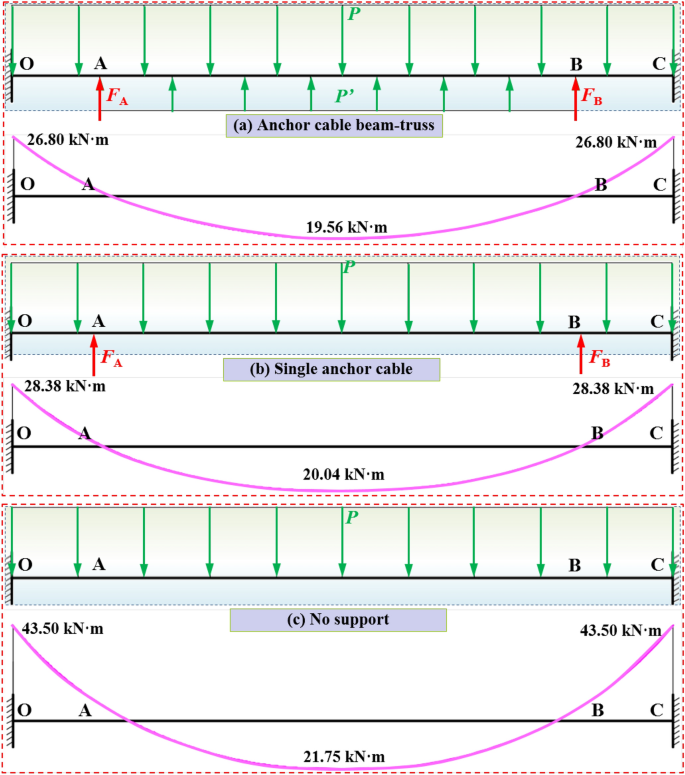
Force and bending moment of roadway roof with different supports under fixed support at both ends.
In order to further analyze the changes of maximum bending moments at different positions of coal roadway roof under different supports, the maximum bending moments of rock beam at both ends of roof and central cross section under different supports are shown in Fig. 12 .
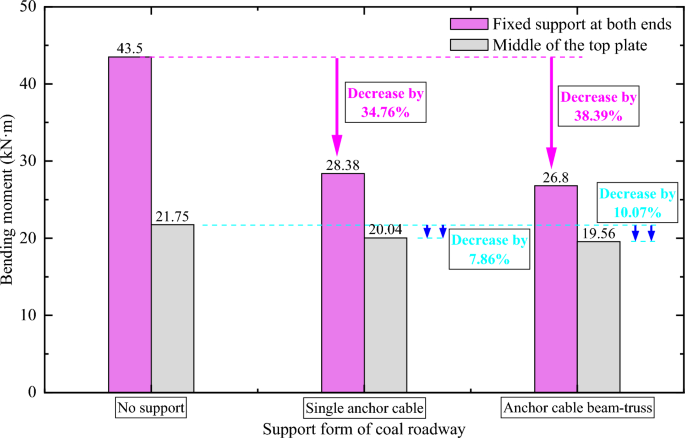
Bending moment for different supports of roadway roof under fixed supports at both ends.
It can be seen from Fig. 12 that when roadway roof is supported by single anchor cables at both ends, the maximum bending moments at both ends of roof support and the central section decrease by 34.76% and 7.86%, respectively compared with no support. The maximum bending moments at these positions decrease by 38.39% and 10.07%, respectively as anchor cable beam-truss structure is adopted. Therefore, the anchor cable beam-truss structure significantly reduces the maximum bending moment of coal roadway roof. The structure has a more significant control effect on surrounding rock compared with single anchor cables, improving the stress state of roadway surrounding-rock and promoting the stability of coal roadway.
Coupling control parameters of anchoring and unloading for coal roadway
Two grouting anchor cables of Φ21.8 × 10,500 mm are added to each row of coal roadway roof for strengthening support, and the row spacing is 2.40 m and 3.20 m. The grouting pipe extends into the anchor cable hole and uses chemical slurry to modify surrounding rock, with grouting pressure of 3.0 MPa. After chemical slurry has solidified, the anchor cables should be tensioned in a timely manner, and the pre tightening force of anchor cables on roadway roof should not be less than 130 kN. Three grouting anchor cables of Φ21.8 × 6500 mm are added to each row of two ribs of coal roadway, and the row spacing is 1.20 m and 1.60 m. When anchor cables are stabilized to the bottom of anchor cable hole, the grouting pipe is extended to anchor cable hole and surrounding rock is grouting modified with chemical material. The grouting pressure is 3.0 MPa, and the pre-tightening force of anchor cables in two ribs of coal roadway is not less than 100 kN. Meanwhile, according to the engineering geology of coal roadway in No.12 mining area of Dongpang Mine and the working attributes of crawler hydraulic drill, the reasonable unloading parameters of coal roadway are comprehensively determined as follows: The unloading holes are approximately cylindrical holes with a diameter of 1.0 m and a length of 5.0 m. The height of unloading hole is not less than 1.20 m from the bottom. The unloading holes of coal roadway are 10.0 m from roadway wall, and internal unloading-holes spacing is 4.0 m. In order to resist large deformation and failure of roadway surrounding-rock, comprehensive control technologies such as supporting, grouting modification and internal unloading are strengthened. The technical parameters and construction of coupling control of coal roadway in No.12 mining area of Dongpang Mine are shown in Fig. 13 .
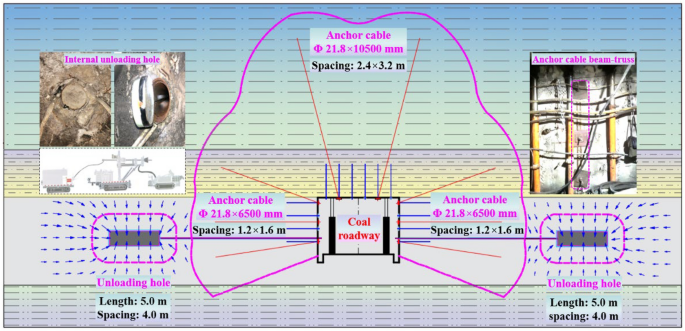
Coupling control parameters of anchoring and unloading for coal roadway.
Observation results and analysis of roadway mine-pressure
Displacement and roof separation of coal roadway.
The observation results of surrounding rock displacement and roof subsidence are shown in Fig. 14 a. The roof separation and displacement of coal roadway tend to be stable after unloading. The maximum displacement is not more than 390 mm, and the maximum roof separation is not more than 13 mm. Therefore, the coupling control technology of anchoring and unloading can significantly control large deformation of surrounding rock in deep coal roadway.
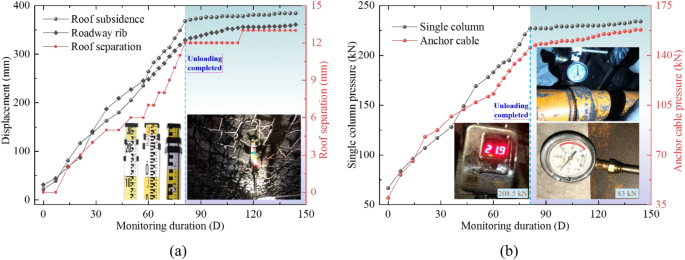
Observation results of mine pressure in coal roadway. ( a ) Displacement and roof separation, ( b ) Pressure of single columns and anchor cables.
Pressure of single columns and anchor cables
Figure 14 b shows the pressure curves of single columns and anchor cables in coal roadway before and after unloading. The pressure continues to increase before the coupling control technology of anchoring and unloading, which is easy to cause instability and failure of coal roadway. The pressure growth rate of single columns and anchor cables in coal roadway surrounding-rock slows down obviously after adopting the coupling control technology, and finally tends to be stable. The pressure of single column is no more than 235 kN and the pressure of anchor cable is no more than 160 kN after stability, which is approximately 52.29% of the anchor breaking load. The coupling technology improves the stress state of coal roadway surrounding-rock, ensures the safety and stability of coal roadway during its service period, and verifies the applicability of coupling control technology of anchoring and unloading to resist large deformation of deep coal roadway surrounding-rock.
Conclusions
The underground test results show that the minimum principal stress of roadway surrounding-rock in Dongpang Mine has exceeded the compressive strength of coal, up to 3.25 times of coal strength, and the maximum principal stress is 6.30 times of uniaxial compressive strength of coal, which indicates that coal roadway is prone to plastination and failure under deep high-stress state.
The joint failure mechanism of coal mass in the deep area migrates into coal roadway and acts on the anchoring support system is analyzed and expounded. It is concluded that conventional strengthening support and grouting modification techniques are no longer suitable for roadway surrounding-rock control in integrated extrusion deformation of coal mass structure. The improvement strategy named coupling control technology of anchoring and unloading for coal roadway is proposed.
Based on the distribution laws of surrounding rock stress under different unloading-hole spacing, it is concluded that surrounding rock stress will be superimposed as unloading-hole spacing is more than 4.25 m, and the roadway surrounding-rock has no obvious unloading effect. As unloading-hole spacing is less than 4.25 m, the maximum abutment pressure does not exceed the in-situ stress. The reasonable spacing of internal unloading-holes is determined to be 4.0 m combined with numerical simulation.
It is obtained that anchor cable beam-truss support is beneficial to maintain the stability of coal roadway by constructing a mechanical model of roof beam. The technical parameters named grouting anchor cable beam-truss support and internal-cavitation unloading for coal roadway are put forward. The experimental results of Dongpang Mine show that coupling control technology can significantly improve the stress state of roadway surrounding-rock, which verifies that coupling control technology of anchoring and unloading plays an important role in resisting large deformation of deep coal-roadway.
Data availability
All data and models or used during the study appear in the submitted article.
Yang, S. Q., Chen, M., Jing, H. W., Chen, K. F. & Meng, B. A case study on large deformation failure mechanism of deep soft rock roadway in Xin’An coal mine. China. Eng. Geol. 217 , 89–101. https://doi.org/10.1016/j.enggeo.2016.12.012 (2017).
Article Google Scholar
Li, S. C. et al. Model test study on surrounding rock deformation and failure mechanisms of deep roadways with thick top coal. Tunn. Undergr. Space Tech. 47 , 52–63. https://doi.org/10.1016/j.tust.2014.12.013 (2015).
Article ADS CAS Google Scholar
Xie, H. P. et al. Study on the mechanical properties and mechanical response of coal mining at 1000 m or deeper. R. Mech. R. Eng. 52 (5), 1475–1490. https://doi.org/10.1007/s00603-018-1509-y (2019).
Shen, B. T. Coal mine roadway stability in soft rock: a case study. R. Mech. Rock Eng. 47 (6), 2225–2238. https://doi.org/10.1007/s00603-013-0528-y (2014).
Sears, M. M. et al. Coal rib response during bench mining: A case study. Int. J. Min. Sci. Tech. 28 (1), 107–113. https://doi.org/10.1016/j.ijmst.2017.12.010 (2018).
Article MathSciNet Google Scholar
Kang, H. P., Gao, F. Q., Xu, G. & Ren, H. W. Mechanical behaviors of coal measures and ground control technologies for China’s deep coal mines—A review. J. Rock Mech. Geotech. 15 (1), 37–65. https://doi.org/10.1016/j.jrmge.2022.11.004 (2023).
He, M. C. Conception system and evaluation indexes for deep engineering. Chinese J. R. Mech. Eng. 24 (16), 2854–2858 (2005).
Google Scholar
Xue, G. Z., Gu, C., Fang, X. Q. & Wei, T. A case study on large deformation failure mechanism and control techniques for soft rock roadways in tectonic stress areas. Sustainability 11 (13), 3510. https://doi.org/10.3390/su11133510 (2019).
Article CAS Google Scholar
Yang, X. J., Wang, E. Y., Wang, Y. J., Gao, Y. B. & Wang, P. A study of the large deformation mechanism and control techniques for deep soft rock roadways. Sustainability 10 (4), 1100. https://doi.org/10.3390/su10041100 (2018).
Chen, D. D. et al. Roadway surrounding rock under multi-coal-seam mining: deviatoric stress evolution and control technology. Adv. Civ. Eng. 2020 , 9891825. https://doi.org/10.1155/2020/9891825 (2020).
Article ADS Google Scholar
Sun, X. M., Zhao, W. C., Shen, F. X., Zhang, Y. & Jiang, M. Study on failure mechanism of deep soft rock roadway and high prestress compensation support countermeasure. Eng. Fail. Anal. https://doi.org/10.1016/j.engfailanal.2022.106857 (2023).
Sahoo, B. R. & Palei, S. K. Study on new type of roadway side support technology in coal mines. J. Min. Sci. 56 (4), 616–630. https://doi.org/10.1134/S1062739120046909 (2020).
Wang, E. & Xie, S. R. Determination of coal pillar width for gob-side entry driving in isolated coal face and its control in deep soft-broken coal seam: A case study. Energy Sci. Eng. 10 (7), 2305–2316. https://doi.org/10.1002/ese3.1139 (2022).
Yang, Y. J., Zhang, Y. & Huang, G. Research on stress distribution regularity and support optimization of an “umbrella” coal pillar in a gob-side roadway based on irregular gob. Energies 15 (11), 3932. https://doi.org/10.1002/ese3.1139 (2022).
Yang, H. Y. et al. A caving self-stabilization bearing structure of advancing cutting roof for gob-side entry retaining with hard roof stratum. Geomech. Eng. 21 (1), 23–33. https://doi.org/10.12989/gae.2020.21.1.023 (2020).
Chen, D. D. et al. Mining-induced failure characteristics and surrounding rock control of gob-side entry driving adjacent to filling working face in the deep coal mine. Energy Sci. Eng. 10 (8), 2593–2611. https://doi.org/10.1002/ese3.1214 (2022).
Zang, C. W. et al. Research on the failure process and stability control technology in a deep roadway: Numerical simulation and field test. Energy Sci. Eng. 8 (7), 2297–2310. https://doi.org/10.1002/ese3.664 (2020).
Zhao, C. X., Li, Y. M., Liu, G. & Meng, X. R. Mechanism analysis and control technology of surrounding rock failure in deep soft rock roadway. Eng. Fail. Anal. https://doi.org/10.1016/j.engfailanal.2020.104611 (2020).
Yao, Q. L., Li, X. H., Pan, F., Wang, T. & Wang, G. Deformation and failure mechanism of roadway sensitive to stress disturbance and its zonal support technology. Shock Vib. 2016 , 1812768. https://doi.org/10.1155/2016/1812768 (2016).
Zhang, L. et al. Relief mechanism of segmented hole reaming and stress distribution characteristics of drilling holes in deep coal mine. Processes 10 (8), 1566. https://doi.org/10.3390/pr10081566 (2022).
Gu, S. T., Chen, C. P., Jiang, B. Y., Ding, K. & Xiao, H. J. Study on the pressure relief mechanism and engineering application of segmented enlarged-diameter boreholes. Sustainability 14 (9), 5234. https://doi.org/10.3390/su14095234 (2022).
Wang, Y. J. et al. Test of a liquid directional roof-cutting technology for pressure-relief entry retaining mining. J. Geophys. Eng. 16 (3), 620–638. https://doi.org/10.1093/jge/gxz041 (2019).
Wang, M. Z., Zheng, H. H., Ma, Z. Q., Mu, H. & Feng, X. L. Control technology of roof-cutting and pressure relief for roadway excavation with strong mining small coal pillar. Sustainability 15 (3), 2046. https://doi.org/10.3390/su15032046 (2023).
Tu, M., Zhao, G. M., Zhang, X. Y., Bu, Q. W. & Dang, J. X. Fracture evolution between blasting roof cutting holes in a mining stress environment. Minerals 12 (4), 418. https://doi.org/10.3390/min12040418 (2022).
Liu, H., Dai, J., Jiang, J. Q., Wang, P. & Yang, J. Q. Analysis of overburden structure and pressure-relief effect of hard roof blasting and cutting. Adv. Civ. Eng. 2019 , 1354652. https://doi.org/10.1155/2019/1354652 (2019).
Zhang, X. & Kang, H. P. Pressure relief mechanism of directional hydraulic fracturing for gob-side entry retaining and its application. Shock Vib. 2021 , 6690654. https://doi.org/10.1155/2021/6690654 (2021).
Mukherjee, D., Selvi, V. A., Ganguly, J., Ram, L. C. & Masto, R. E. Exploratory study of archaebacteria and their habitat in underground, opencast coal mines and coal cine fire areas of Dhanbad. J. Geol. Soc. India 91 (5), 575–582 (2018).
Klishin, V. I., Opruk, GYu., Pavlova, L. D. & Fryanov, V. N. Active prefracture methods in top coal caving technologies for thick and gently dipping seams. J. Min. Sci. 56 (3), 395–403. https://doi.org/10.1134/S1062739120036689 (2020).
Yang, H. Z., Wang, D. P., Ju, W. J., Yuan, W. M. & Su, C. Asymmetric damage mechanisms and prevention technology in large-Section Gob-side entry retaining. Sustainability 15 (1), 739. https://doi.org/10.3390/su15010739 (2023).
Wu, P. et al. Surrounding rock stability control technology of roadway in large inclination seam with weak structural plane in roof. Minerals 11 (8), 881. https://doi.org/10.3390/min11080881 (2021).
Tian, M. L. et al. Asymmetric deformation failure mechanism and support technology of roadways under non-uniform pressure from a mining disturbance. B. Eng. Geol. Environ. 81 (5), 211. https://doi.org/10.1007/s10064-022-02710-2 (2022).
Xiong, X. Y., Jun, D., Chen, X. N. & Ouyang, Y. B. Complex function solution for deformation and failure mechanism of inclined coal seam roadway. Sci. Rep. 12 (1), 7147. https://doi.org/10.1038/s41598-022-11277-7 (2022).
Article ADS CAS PubMed PubMed Central Google Scholar
Xie, S. R. et al. Stability analysis and control technology of gob-side entry retaining with double roadways by filling with high-water material in gently inclined coal seam. Int. J. Coal Sci. Technol. 9 , 55. https://doi.org/10.1007/s40789-022-00524-x (2022).
Xie, S. R., Jiang, Z. S., Chen, D. D., Wang, E. & Lv, F. A new pressure relief technology by internal hole-making to protect roadway in two sides of deep coal roadway: a case study. Rock Mech. Rock Eng. 56 (2), 1537–1561. https://doi.org/10.1007/s00603-022-03135-9 (2022).
Xie, S. R., Jiang, Z. S., Chen, D. D., Wang, E. & Lv, F. Failure mechanism of continuous large deformation and a novel pressure relief control technology on the two sides of deep coal roadway. Eng. Fail. Anal. 144 , 106941. https://doi.org/10.1016/j.engfailanal.2022.106941 (2023).
Zheng, W. X., Bu, Q. W. & Hu, Y. Q. Plastic failure analysis of roadway floor surrounding rocks based on unified strength theory. Adv. Civ. Eng. 2018 , 7475698. https://doi.org/10.1155/2018/7475698 (2018).
Wu, G. S. et al. Experimental investigation on rockburst behavior of the rock-coal-bolt specimen under different stress conditions. Sci. Rep. 10 (1), 7556. https://doi.org/10.1038/s41598-020-64513-3 (2020).
Wen, Z. J., Rinne, M., Han, Z. Z., Song, Z. & Shi, Y. K. Structure model of roadway with large deformation and its basic research into engineering theories. Teh. Vjesn. 21 (5), 1065–1071 (2014).
Download references
Acknowledgements
This work was financially supported by the National Natural Science Foundation of China (Grant no. 52274081), the Natural Science Foundation of Hebei Province of China (Grant no. E2021508011), the Science and Technology Project of Hebei Education Department (Grant no. QN2024235), the Fundamental Research Funds for the Central Universities (3142024013).
Author information
Authors and affiliations.
School of Mine Safety, North China Institute of Science and Technology, Langfang, 065201, China
En Wang, Shuaifeng Yin, Qingtao Kang, Xubo Zhao, Qiankun Lan, Hongyuan Sheng & Huiyang Liang
Hebei Key Laboratory of Mine Intelligent Unmanned Mining Technology, North China Institute of Science and Technology, Beijing, 101601, China
School of Energy and Mining Engineering, China University of Mining and Technology-Beijing, Beijing, 100083, China
You can also search for this author in PubMed Google Scholar
Contributions
E.W.: Investigation, Conceptualization, Data curation, Writing—original draft. S.Y.: Conceptualization, Methodology, Funding acquisition, Writing—review & editing. Q.K.: Supervision, Software, Visualization. X.Z.: Resources, Visualization. Q.L.: Software, Validation. H.S.: Validation. H.L.: Visualization.
Corresponding author
Correspondence to Shuaifeng Yin .
Ethics declarations
Competing interests.
The authors declare no competing interests.
Additional information
Publisher's note.
Springer Nature remains neutral with regard to jurisdictional claims in published maps and institutional affiliations.
Rights and permissions
Open Access This article is licensed under a Creative Commons Attribution 4.0 International License, which permits use, sharing, adaptation, distribution and reproduction in any medium or format, as long as you give appropriate credit to the original author(s) and the source, provide a link to the Creative Commons licence, and indicate if changes were made. The images or other third party material in this article are included in the article's Creative Commons licence, unless indicated otherwise in a credit line to the material. If material is not included in the article's Creative Commons licence and your intended use is not permitted by statutory regulation or exceeds the permitted use, you will need to obtain permission directly from the copyright holder. To view a copy of this licence, visit http://creativecommons.org/licenses/by/4.0/ .
Reprints and permissions
About this article
Cite this article.
Wang, E., Yin, S., Kang, Q. et al. Coupling control technology of anchoring and unloading in deep intense-mining and large-deformation roadway: a case study. Sci Rep 14 , 12075 (2024). https://doi.org/10.1038/s41598-024-61029-y
Download citation
Received : 04 January 2024
Accepted : 30 April 2024
Published : 27 May 2024
DOI : https://doi.org/10.1038/s41598-024-61029-y
Share this article
Anyone you share the following link with will be able to read this content:
Sorry, a shareable link is not currently available for this article.
Provided by the Springer Nature SharedIt content-sharing initiative
By submitting a comment you agree to abide by our Terms and Community Guidelines . If you find something abusive or that does not comply with our terms or guidelines please flag it as inappropriate.
Quick links
- Explore articles by subject
- Guide to authors
- Editorial policies
Sign up for the Nature Briefing newsletter — what matters in science, free to your inbox daily.


IMAGES
VIDEO
COMMENTS
The tools and technologies of data warehousing, data mining, and other customer relationship management (CRM) techniques afford new opportunities for businesses to act on the concepts of relationship marketing. The old model of "design-build-sell" (a product-oriented view) is being replaced by "sell-build-redesign" (a customer-oriented ...
Abstract and Figures. Data mining has various applications for customer relationship management. In this article, we introduce a framework for identifying appropriate data mining techniques for ...
Abstract and Figures. Data mining has various applications for customer relationship management. In this proposal, we are introducing a framework for identifying appropriate data mining techniques ...
Scores of researchers have paid attention to empirical and conceptual dimensions of Customer relationship management (CRM). A few studies summarise the research output of CRM focusing on a specific industry. Nevertheless, there is scant literature summarising the research output of CRM in contrast to the data mining-based CRM. This study presents a scientometric analysis that evaluates CRM ...
Data Mining in CRM - a Case Study for an Online Gaming Company Project Work submitted in partial Fulfillment of the Requirements for the Degree of Master of Science in Information and Media Technologies at the Technical University of Hamburg-Harburg Project Work Examiner: Prof. Dr. Ralf Moeller Submitted by: Natalya Furmanova
As Customer relationship management (CRM) is a well established concept and its practice to enable the realization of successful Telecommunication system, data mining techniques is developed for improving the customer relationship management part mainly in Corporate Telecom Sector. Considering the …
A step-by-step guide to data mining applications in CRM. Following a handbook approach, this book bridges the gap between analytics and their use in everyday marketing, providing guidance on solving real business problems using data mining techniques. ... RapidMiner and Data Mining for Excel. Case studies from industries including banking ...
A complete and comprehensive handbook for the application of data mining techniques in marketing and customer relationship management. It combines a technical and a business perspective, bridging the gap between data mining and its use in marketing.It guides readers through all the phases of the data mining process, presenting a solid data mining methodology, data mining best practices and ...
Many organisations across a variety of industries are engaging in the process of data mining as part of an overall strategy for business intelligence, customer relationship management (CRM), including churn prevention. This paper provides an overview of the data mining process and illustrates a case study in which data mining is utilised as a churn prevention tool for a major Midwest USA ...
Many small online retailers and new entrants to the online retail sector are keen to practice data mining and consumer-centric marketing in their businesses yet technically lack the necessary knowledge and expertise to do so. In this article a case study of using data mining techniques in customer-centric business intelligence for an online retailer is presented. The main purpose of this ...
A new methodology in case of mobile services on the perceptions of customers of Telecommunication basing on applicability of data mining techniques to CRM databases is presented by generating Association rules from frequent item sets on the proposed approach F-MFPG. As Customer relationship management (CRM) is a well established concept and its practice to enable the realization of successful ...
Abstract: CRM is a crucial way in today's marketing, using customer knowledge to sustain business growth. Data Mining plays an important part in getting the detail information from a large number of customer database. This essay discuss how the DM function in CRM and give a case study of a famous cosmetics retailing brand to show the role of DM in marketing strategy.
From marketing to sales and customer service, the industry is full of CRM case studies highlighting how chatbots and virtual assistants help businesses improve the customer journey. It's not just AI, though; companies are using data mining and personalization technology and overhauling their customer service channels to better interact with ...
INTERNATIONAL JOURNAL OF TECHNOLOGICAL EXPLORATION AND LEARNING (IJTEL) www.ijtel.org A Case Study of Customer Relationship Management using Data Mining Techniques B.Santhosh Kumar C.B.Sivaparthipan AP/Department of Computer Science & Engineering SNS College of Technology Coimbatore, S. India AP/Department of Computer Science & Engineering SNS College of Technology Coimbatore, S. India Dr.T ...
Read the PBS, LunaMetrics, and Google Analytics case study. 5. The Pegasus Group. Cyber attackers compromised and targeted the data mining system (DMS) of a major network client of The Pegasus Group and launched a distributed denial-of-service (DDoS) attack against 1,500 services. Under extreme time pressure, The Pegasus Group needed to find a ...
A successful implementation requires defining clear goals, choosing data wisely, and constant adaptation. Data mining case studies help businesses explore data for smart decision-making. It's about finding valuable insights from big datasets. This is crucial for businesses in all industries as data guides strategic planning.
Sequential Sentiment Pattern Mining to Predict Churn in CRM Systems: A Case Study with Telecom Data. In Proceedings of the XV Brazilian Symposium on Information Systems (Aracaju, Brazil) (SBSI '19). ... Sergio Muñoz-Romero, and José Luis Rojo-Álvarez. 2018. Using big data from Customer Relationship Management information systems to ...
A few studies summarise the research output of CRM focusing on a specic industry. Nevertheless, there is scant literature summarising the research output of CRM in contrast to the data mining-based CRM. This study presents a scientometric analysis that evaluates CRM research output with a special focus on data mining-based CRM. Bibliometric ...
Fraud detection by analyzing past fraudulent transactions and taking corrective measures Techniques of Data Mining in CRM 1. Clustering - Identify similar data sets and understand both similarities and differences in data to increase conversion rates 2. Classification - Gather all information about a data set and classify it into proper ...
CRM case studies describe how CRM vendors and service providers were able to help clients increase the efficiency and effectiveness of their customer service and sales and marketing efforts. Examples of CRM case studies would include a professional services firm selecting a CRM solution or a manufacturer undertaking a CRM implementation.
Case Study No.3: Starbucks. Starbucks is one of the leading coffee shops with innumerable branches around the globe. Their case study will be a perfect example of the case studies on data mining in market analysis. Starbucks indulges in data mining to determine the perfect locations for setting up its stores.
Treatment effectiveness, Management of healthcare, Detection of fraud and abuse, Customer relationship management [1]. Jayanthi Ranjan presents how data mining discovers and extracts useful patterns of this large data to find observable patterns. This paper demonstrates the ability of Data mining in improving the quality of the
The case study tries to describe how Process Mining could be applied in order to detect and improve "Customer Relationship Management" process and extract some kind of social networks that represent the relations between the employees (resources) of National Institute of Statistics of Portugal (INE) using event logs. Download chapter PDF.
Boost your sales efficiency and growth with Salesforce, the trusted AI-powered sales solution. Learn more and try it for free.
It can provide measured data support for analyzing large deformation and failure mechanisms of coal roadway. ... Sears, M. M. et al. Coal rib response during bench mining: A case study. Int. J ...