- Privacy Policy

Home » What is a Hypothesis – Types, Examples and Writing Guide

What is a Hypothesis – Types, Examples and Writing Guide
Table of Contents

Definition:
Hypothesis is an educated guess or proposed explanation for a phenomenon, based on some initial observations or data. It is a tentative statement that can be tested and potentially proven or disproven through further investigation and experimentation.
Hypothesis is often used in scientific research to guide the design of experiments and the collection and analysis of data. It is an essential element of the scientific method, as it allows researchers to make predictions about the outcome of their experiments and to test those predictions to determine their accuracy.
Types of Hypothesis
Types of Hypothesis are as follows:
Research Hypothesis
A research hypothesis is a statement that predicts a relationship between variables. It is usually formulated as a specific statement that can be tested through research, and it is often used in scientific research to guide the design of experiments.
Null Hypothesis
The null hypothesis is a statement that assumes there is no significant difference or relationship between variables. It is often used as a starting point for testing the research hypothesis, and if the results of the study reject the null hypothesis, it suggests that there is a significant difference or relationship between variables.
Alternative Hypothesis
An alternative hypothesis is a statement that assumes there is a significant difference or relationship between variables. It is often used as an alternative to the null hypothesis and is tested against the null hypothesis to determine which statement is more accurate.
Directional Hypothesis
A directional hypothesis is a statement that predicts the direction of the relationship between variables. For example, a researcher might predict that increasing the amount of exercise will result in a decrease in body weight.
Non-directional Hypothesis
A non-directional hypothesis is a statement that predicts the relationship between variables but does not specify the direction. For example, a researcher might predict that there is a relationship between the amount of exercise and body weight, but they do not specify whether increasing or decreasing exercise will affect body weight.
Statistical Hypothesis
A statistical hypothesis is a statement that assumes a particular statistical model or distribution for the data. It is often used in statistical analysis to test the significance of a particular result.
Composite Hypothesis
A composite hypothesis is a statement that assumes more than one condition or outcome. It can be divided into several sub-hypotheses, each of which represents a different possible outcome.
Empirical Hypothesis
An empirical hypothesis is a statement that is based on observed phenomena or data. It is often used in scientific research to develop theories or models that explain the observed phenomena.
Simple Hypothesis
A simple hypothesis is a statement that assumes only one outcome or condition. It is often used in scientific research to test a single variable or factor.
Complex Hypothesis
A complex hypothesis is a statement that assumes multiple outcomes or conditions. It is often used in scientific research to test the effects of multiple variables or factors on a particular outcome.
Applications of Hypothesis
Hypotheses are used in various fields to guide research and make predictions about the outcomes of experiments or observations. Here are some examples of how hypotheses are applied in different fields:
- Science : In scientific research, hypotheses are used to test the validity of theories and models that explain natural phenomena. For example, a hypothesis might be formulated to test the effects of a particular variable on a natural system, such as the effects of climate change on an ecosystem.
- Medicine : In medical research, hypotheses are used to test the effectiveness of treatments and therapies for specific conditions. For example, a hypothesis might be formulated to test the effects of a new drug on a particular disease.
- Psychology : In psychology, hypotheses are used to test theories and models of human behavior and cognition. For example, a hypothesis might be formulated to test the effects of a particular stimulus on the brain or behavior.
- Sociology : In sociology, hypotheses are used to test theories and models of social phenomena, such as the effects of social structures or institutions on human behavior. For example, a hypothesis might be formulated to test the effects of income inequality on crime rates.
- Business : In business research, hypotheses are used to test the validity of theories and models that explain business phenomena, such as consumer behavior or market trends. For example, a hypothesis might be formulated to test the effects of a new marketing campaign on consumer buying behavior.
- Engineering : In engineering, hypotheses are used to test the effectiveness of new technologies or designs. For example, a hypothesis might be formulated to test the efficiency of a new solar panel design.
How to write a Hypothesis
Here are the steps to follow when writing a hypothesis:
Identify the Research Question
The first step is to identify the research question that you want to answer through your study. This question should be clear, specific, and focused. It should be something that can be investigated empirically and that has some relevance or significance in the field.
Conduct a Literature Review
Before writing your hypothesis, it’s essential to conduct a thorough literature review to understand what is already known about the topic. This will help you to identify the research gap and formulate a hypothesis that builds on existing knowledge.
Determine the Variables
The next step is to identify the variables involved in the research question. A variable is any characteristic or factor that can vary or change. There are two types of variables: independent and dependent. The independent variable is the one that is manipulated or changed by the researcher, while the dependent variable is the one that is measured or observed as a result of the independent variable.
Formulate the Hypothesis
Based on the research question and the variables involved, you can now formulate your hypothesis. A hypothesis should be a clear and concise statement that predicts the relationship between the variables. It should be testable through empirical research and based on existing theory or evidence.
Write the Null Hypothesis
The null hypothesis is the opposite of the alternative hypothesis, which is the hypothesis that you are testing. The null hypothesis states that there is no significant difference or relationship between the variables. It is important to write the null hypothesis because it allows you to compare your results with what would be expected by chance.
Refine the Hypothesis
After formulating the hypothesis, it’s important to refine it and make it more precise. This may involve clarifying the variables, specifying the direction of the relationship, or making the hypothesis more testable.
Examples of Hypothesis
Here are a few examples of hypotheses in different fields:
- Psychology : “Increased exposure to violent video games leads to increased aggressive behavior in adolescents.”
- Biology : “Higher levels of carbon dioxide in the atmosphere will lead to increased plant growth.”
- Sociology : “Individuals who grow up in households with higher socioeconomic status will have higher levels of education and income as adults.”
- Education : “Implementing a new teaching method will result in higher student achievement scores.”
- Marketing : “Customers who receive a personalized email will be more likely to make a purchase than those who receive a generic email.”
- Physics : “An increase in temperature will cause an increase in the volume of a gas, assuming all other variables remain constant.”
- Medicine : “Consuming a diet high in saturated fats will increase the risk of developing heart disease.”
Purpose of Hypothesis
The purpose of a hypothesis is to provide a testable explanation for an observed phenomenon or a prediction of a future outcome based on existing knowledge or theories. A hypothesis is an essential part of the scientific method and helps to guide the research process by providing a clear focus for investigation. It enables scientists to design experiments or studies to gather evidence and data that can support or refute the proposed explanation or prediction.
The formulation of a hypothesis is based on existing knowledge, observations, and theories, and it should be specific, testable, and falsifiable. A specific hypothesis helps to define the research question, which is important in the research process as it guides the selection of an appropriate research design and methodology. Testability of the hypothesis means that it can be proven or disproven through empirical data collection and analysis. Falsifiability means that the hypothesis should be formulated in such a way that it can be proven wrong if it is incorrect.
In addition to guiding the research process, the testing of hypotheses can lead to new discoveries and advancements in scientific knowledge. When a hypothesis is supported by the data, it can be used to develop new theories or models to explain the observed phenomenon. When a hypothesis is not supported by the data, it can help to refine existing theories or prompt the development of new hypotheses to explain the phenomenon.
When to use Hypothesis
Here are some common situations in which hypotheses are used:
- In scientific research , hypotheses are used to guide the design of experiments and to help researchers make predictions about the outcomes of those experiments.
- In social science research , hypotheses are used to test theories about human behavior, social relationships, and other phenomena.
- I n business , hypotheses can be used to guide decisions about marketing, product development, and other areas. For example, a hypothesis might be that a new product will sell well in a particular market, and this hypothesis can be tested through market research.
Characteristics of Hypothesis
Here are some common characteristics of a hypothesis:
- Testable : A hypothesis must be able to be tested through observation or experimentation. This means that it must be possible to collect data that will either support or refute the hypothesis.
- Falsifiable : A hypothesis must be able to be proven false if it is not supported by the data. If a hypothesis cannot be falsified, then it is not a scientific hypothesis.
- Clear and concise : A hypothesis should be stated in a clear and concise manner so that it can be easily understood and tested.
- Based on existing knowledge : A hypothesis should be based on existing knowledge and research in the field. It should not be based on personal beliefs or opinions.
- Specific : A hypothesis should be specific in terms of the variables being tested and the predicted outcome. This will help to ensure that the research is focused and well-designed.
- Tentative: A hypothesis is a tentative statement or assumption that requires further testing and evidence to be confirmed or refuted. It is not a final conclusion or assertion.
- Relevant : A hypothesis should be relevant to the research question or problem being studied. It should address a gap in knowledge or provide a new perspective on the issue.
Advantages of Hypothesis
Hypotheses have several advantages in scientific research and experimentation:
- Guides research: A hypothesis provides a clear and specific direction for research. It helps to focus the research question, select appropriate methods and variables, and interpret the results.
- Predictive powe r: A hypothesis makes predictions about the outcome of research, which can be tested through experimentation. This allows researchers to evaluate the validity of the hypothesis and make new discoveries.
- Facilitates communication: A hypothesis provides a common language and framework for scientists to communicate with one another about their research. This helps to facilitate the exchange of ideas and promotes collaboration.
- Efficient use of resources: A hypothesis helps researchers to use their time, resources, and funding efficiently by directing them towards specific research questions and methods that are most likely to yield results.
- Provides a basis for further research: A hypothesis that is supported by data provides a basis for further research and exploration. It can lead to new hypotheses, theories, and discoveries.
- Increases objectivity: A hypothesis can help to increase objectivity in research by providing a clear and specific framework for testing and interpreting results. This can reduce bias and increase the reliability of research findings.
Limitations of Hypothesis
Some Limitations of the Hypothesis are as follows:
- Limited to observable phenomena: Hypotheses are limited to observable phenomena and cannot account for unobservable or intangible factors. This means that some research questions may not be amenable to hypothesis testing.
- May be inaccurate or incomplete: Hypotheses are based on existing knowledge and research, which may be incomplete or inaccurate. This can lead to flawed hypotheses and erroneous conclusions.
- May be biased: Hypotheses may be biased by the researcher’s own beliefs, values, or assumptions. This can lead to selective interpretation of data and a lack of objectivity in research.
- Cannot prove causation: A hypothesis can only show a correlation between variables, but it cannot prove causation. This requires further experimentation and analysis.
- Limited to specific contexts: Hypotheses are limited to specific contexts and may not be generalizable to other situations or populations. This means that results may not be applicable in other contexts or may require further testing.
- May be affected by chance : Hypotheses may be affected by chance or random variation, which can obscure or distort the true relationship between variables.
About the author
Muhammad Hassan
Researcher, Academic Writer, Web developer
You may also like

Data Collection – Methods Types and Examples

Delimitations in Research – Types, Examples and...

Research Process – Steps, Examples and Tips

Research Design – Types, Methods and Examples

Institutional Review Board – Application Sample...

Evaluating Research – Process, Examples and...
Have a language expert improve your writing
Run a free plagiarism check in 10 minutes, automatically generate references for free.
- Knowledge Base
- Methodology
- How to Write a Strong Hypothesis | Guide & Examples
How to Write a Strong Hypothesis | Guide & Examples
Published on 6 May 2022 by Shona McCombes .
A hypothesis is a statement that can be tested by scientific research. If you want to test a relationship between two or more variables, you need to write hypotheses before you start your experiment or data collection.
Table of contents
What is a hypothesis, developing a hypothesis (with example), hypothesis examples, frequently asked questions about writing hypotheses.
A hypothesis states your predictions about what your research will find. It is a tentative answer to your research question that has not yet been tested. For some research projects, you might have to write several hypotheses that address different aspects of your research question.
A hypothesis is not just a guess – it should be based on existing theories and knowledge. It also has to be testable, which means you can support or refute it through scientific research methods (such as experiments, observations, and statistical analysis of data).
Variables in hypotheses
Hypotheses propose a relationship between two or more variables . An independent variable is something the researcher changes or controls. A dependent variable is something the researcher observes and measures.
In this example, the independent variable is exposure to the sun – the assumed cause . The dependent variable is the level of happiness – the assumed effect .
Prevent plagiarism, run a free check.
Step 1: ask a question.
Writing a hypothesis begins with a research question that you want to answer. The question should be focused, specific, and researchable within the constraints of your project.
Step 2: Do some preliminary research
Your initial answer to the question should be based on what is already known about the topic. Look for theories and previous studies to help you form educated assumptions about what your research will find.
At this stage, you might construct a conceptual framework to identify which variables you will study and what you think the relationships are between them. Sometimes, you’ll have to operationalise more complex constructs.
Step 3: Formulate your hypothesis
Now you should have some idea of what you expect to find. Write your initial answer to the question in a clear, concise sentence.
Step 4: Refine your hypothesis
You need to make sure your hypothesis is specific and testable. There are various ways of phrasing a hypothesis, but all the terms you use should have clear definitions, and the hypothesis should contain:
- The relevant variables
- The specific group being studied
- The predicted outcome of the experiment or analysis
Step 5: Phrase your hypothesis in three ways
To identify the variables, you can write a simple prediction in if … then form. The first part of the sentence states the independent variable and the second part states the dependent variable.
In academic research, hypotheses are more commonly phrased in terms of correlations or effects, where you directly state the predicted relationship between variables.
If you are comparing two groups, the hypothesis can state what difference you expect to find between them.
Step 6. Write a null hypothesis
If your research involves statistical hypothesis testing , you will also have to write a null hypothesis. The null hypothesis is the default position that there is no association between the variables. The null hypothesis is written as H 0 , while the alternative hypothesis is H 1 or H a .
Hypothesis testing is a formal procedure for investigating our ideas about the world using statistics. It is used by scientists to test specific predictions, called hypotheses , by calculating how likely it is that a pattern or relationship between variables could have arisen by chance.
A hypothesis is not just a guess. It should be based on existing theories and knowledge. It also has to be testable, which means you can support or refute it through scientific research methods (such as experiments, observations, and statistical analysis of data).
A research hypothesis is your proposed answer to your research question. The research hypothesis usually includes an explanation (‘ x affects y because …’).
A statistical hypothesis, on the other hand, is a mathematical statement about a population parameter. Statistical hypotheses always come in pairs: the null and alternative hypotheses. In a well-designed study , the statistical hypotheses correspond logically to the research hypothesis.
Cite this Scribbr article
If you want to cite this source, you can copy and paste the citation or click the ‘Cite this Scribbr article’ button to automatically add the citation to our free Reference Generator.
McCombes, S. (2022, May 06). How to Write a Strong Hypothesis | Guide & Examples. Scribbr. Retrieved 6 May 2024, from https://www.scribbr.co.uk/research-methods/hypothesis-writing/
Is this article helpful?
Shona McCombes
Other students also liked, operationalisation | a guide with examples, pros & cons, what is a conceptual framework | tips & examples, a quick guide to experimental design | 5 steps & examples.
- Bipolar Disorder
- Therapy Center
- When To See a Therapist
- Types of Therapy
- Best Online Therapy
- Best Couples Therapy
- Best Family Therapy
- Managing Stress
- Sleep and Dreaming
- Understanding Emotions
- Self-Improvement
- Healthy Relationships
- Student Resources
- Personality Types
- Guided Meditations
- Verywell Mind Insights
- 2024 Verywell Mind 25
- Mental Health in the Classroom
- Editorial Process
- Meet Our Review Board
- Crisis Support
How to Write a Great Hypothesis
Hypothesis Definition, Format, Examples, and Tips
Kendra Cherry, MS, is a psychosocial rehabilitation specialist, psychology educator, and author of the "Everything Psychology Book."
:max_bytes(150000):strip_icc():format(webp)/IMG_9791-89504ab694d54b66bbd72cb84ffb860e.jpg)
Amy Morin, LCSW, is a psychotherapist and international bestselling author. Her books, including "13 Things Mentally Strong People Don't Do," have been translated into more than 40 languages. Her TEDx talk, "The Secret of Becoming Mentally Strong," is one of the most viewed talks of all time.
:max_bytes(150000):strip_icc():format(webp)/VW-MIND-Amy-2b338105f1ee493f94d7e333e410fa76.jpg)
Verywell / Alex Dos Diaz
- The Scientific Method
Hypothesis Format
Falsifiability of a hypothesis.
- Operationalization
Hypothesis Types
Hypotheses examples.
- Collecting Data
A hypothesis is a tentative statement about the relationship between two or more variables. It is a specific, testable prediction about what you expect to happen in a study. It is a preliminary answer to your question that helps guide the research process.
Consider a study designed to examine the relationship between sleep deprivation and test performance. The hypothesis might be: "This study is designed to assess the hypothesis that sleep-deprived people will perform worse on a test than individuals who are not sleep-deprived."
At a Glance
A hypothesis is crucial to scientific research because it offers a clear direction for what the researchers are looking to find. This allows them to design experiments to test their predictions and add to our scientific knowledge about the world. This article explores how a hypothesis is used in psychology research, how to write a good hypothesis, and the different types of hypotheses you might use.
The Hypothesis in the Scientific Method
In the scientific method , whether it involves research in psychology, biology, or some other area, a hypothesis represents what the researchers think will happen in an experiment. The scientific method involves the following steps:
- Forming a question
- Performing background research
- Creating a hypothesis
- Designing an experiment
- Collecting data
- Analyzing the results
- Drawing conclusions
- Communicating the results
The hypothesis is a prediction, but it involves more than a guess. Most of the time, the hypothesis begins with a question which is then explored through background research. At this point, researchers then begin to develop a testable hypothesis.
Unless you are creating an exploratory study, your hypothesis should always explain what you expect to happen.
In a study exploring the effects of a particular drug, the hypothesis might be that researchers expect the drug to have some type of effect on the symptoms of a specific illness. In psychology, the hypothesis might focus on how a certain aspect of the environment might influence a particular behavior.
Remember, a hypothesis does not have to be correct. While the hypothesis predicts what the researchers expect to see, the goal of the research is to determine whether this guess is right or wrong. When conducting an experiment, researchers might explore numerous factors to determine which ones might contribute to the ultimate outcome.
In many cases, researchers may find that the results of an experiment do not support the original hypothesis. When writing up these results, the researchers might suggest other options that should be explored in future studies.
In many cases, researchers might draw a hypothesis from a specific theory or build on previous research. For example, prior research has shown that stress can impact the immune system. So a researcher might hypothesize: "People with high-stress levels will be more likely to contract a common cold after being exposed to the virus than people who have low-stress levels."
In other instances, researchers might look at commonly held beliefs or folk wisdom. "Birds of a feather flock together" is one example of folk adage that a psychologist might try to investigate. The researcher might pose a specific hypothesis that "People tend to select romantic partners who are similar to them in interests and educational level."
Elements of a Good Hypothesis
So how do you write a good hypothesis? When trying to come up with a hypothesis for your research or experiments, ask yourself the following questions:
- Is your hypothesis based on your research on a topic?
- Can your hypothesis be tested?
- Does your hypothesis include independent and dependent variables?
Before you come up with a specific hypothesis, spend some time doing background research. Once you have completed a literature review, start thinking about potential questions you still have. Pay attention to the discussion section in the journal articles you read . Many authors will suggest questions that still need to be explored.
How to Formulate a Good Hypothesis
To form a hypothesis, you should take these steps:
- Collect as many observations about a topic or problem as you can.
- Evaluate these observations and look for possible causes of the problem.
- Create a list of possible explanations that you might want to explore.
- After you have developed some possible hypotheses, think of ways that you could confirm or disprove each hypothesis through experimentation. This is known as falsifiability.
In the scientific method , falsifiability is an important part of any valid hypothesis. In order to test a claim scientifically, it must be possible that the claim could be proven false.
Students sometimes confuse the idea of falsifiability with the idea that it means that something is false, which is not the case. What falsifiability means is that if something was false, then it is possible to demonstrate that it is false.
One of the hallmarks of pseudoscience is that it makes claims that cannot be refuted or proven false.
The Importance of Operational Definitions
A variable is a factor or element that can be changed and manipulated in ways that are observable and measurable. However, the researcher must also define how the variable will be manipulated and measured in the study.
Operational definitions are specific definitions for all relevant factors in a study. This process helps make vague or ambiguous concepts detailed and measurable.
For example, a researcher might operationally define the variable " test anxiety " as the results of a self-report measure of anxiety experienced during an exam. A "study habits" variable might be defined by the amount of studying that actually occurs as measured by time.
These precise descriptions are important because many things can be measured in various ways. Clearly defining these variables and how they are measured helps ensure that other researchers can replicate your results.
Replicability
One of the basic principles of any type of scientific research is that the results must be replicable.
Replication means repeating an experiment in the same way to produce the same results. By clearly detailing the specifics of how the variables were measured and manipulated, other researchers can better understand the results and repeat the study if needed.
Some variables are more difficult than others to define. For example, how would you operationally define a variable such as aggression ? For obvious ethical reasons, researchers cannot create a situation in which a person behaves aggressively toward others.
To measure this variable, the researcher must devise a measurement that assesses aggressive behavior without harming others. The researcher might utilize a simulated task to measure aggressiveness in this situation.
Hypothesis Checklist
- Does your hypothesis focus on something that you can actually test?
- Does your hypothesis include both an independent and dependent variable?
- Can you manipulate the variables?
- Can your hypothesis be tested without violating ethical standards?
The hypothesis you use will depend on what you are investigating and hoping to find. Some of the main types of hypotheses that you might use include:
- Simple hypothesis : This type of hypothesis suggests there is a relationship between one independent variable and one dependent variable.
- Complex hypothesis : This type suggests a relationship between three or more variables, such as two independent and dependent variables.
- Null hypothesis : This hypothesis suggests no relationship exists between two or more variables.
- Alternative hypothesis : This hypothesis states the opposite of the null hypothesis.
- Statistical hypothesis : This hypothesis uses statistical analysis to evaluate a representative population sample and then generalizes the findings to the larger group.
- Logical hypothesis : This hypothesis assumes a relationship between variables without collecting data or evidence.
A hypothesis often follows a basic format of "If {this happens} then {this will happen}." One way to structure your hypothesis is to describe what will happen to the dependent variable if you change the independent variable .
The basic format might be: "If {these changes are made to a certain independent variable}, then we will observe {a change in a specific dependent variable}."
A few examples of simple hypotheses:
- "Students who eat breakfast will perform better on a math exam than students who do not eat breakfast."
- "Students who experience test anxiety before an English exam will get lower scores than students who do not experience test anxiety."
- "Motorists who talk on the phone while driving will be more likely to make errors on a driving course than those who do not talk on the phone."
- "Children who receive a new reading intervention will have higher reading scores than students who do not receive the intervention."
Examples of a complex hypothesis include:
- "People with high-sugar diets and sedentary activity levels are more likely to develop depression."
- "Younger people who are regularly exposed to green, outdoor areas have better subjective well-being than older adults who have limited exposure to green spaces."
Examples of a null hypothesis include:
- "There is no difference in anxiety levels between people who take St. John's wort supplements and those who do not."
- "There is no difference in scores on a memory recall task between children and adults."
- "There is no difference in aggression levels between children who play first-person shooter games and those who do not."
Examples of an alternative hypothesis:
- "People who take St. John's wort supplements will have less anxiety than those who do not."
- "Adults will perform better on a memory task than children."
- "Children who play first-person shooter games will show higher levels of aggression than children who do not."
Collecting Data on Your Hypothesis
Once a researcher has formed a testable hypothesis, the next step is to select a research design and start collecting data. The research method depends largely on exactly what they are studying. There are two basic types of research methods: descriptive research and experimental research.
Descriptive Research Methods
Descriptive research such as case studies , naturalistic observations , and surveys are often used when conducting an experiment is difficult or impossible. These methods are best used to describe different aspects of a behavior or psychological phenomenon.
Once a researcher has collected data using descriptive methods, a correlational study can examine how the variables are related. This research method might be used to investigate a hypothesis that is difficult to test experimentally.
Experimental Research Methods
Experimental methods are used to demonstrate causal relationships between variables. In an experiment, the researcher systematically manipulates a variable of interest (known as the independent variable) and measures the effect on another variable (known as the dependent variable).
Unlike correlational studies, which can only be used to determine if there is a relationship between two variables, experimental methods can be used to determine the actual nature of the relationship—whether changes in one variable actually cause another to change.
The hypothesis is a critical part of any scientific exploration. It represents what researchers expect to find in a study or experiment. In situations where the hypothesis is unsupported by the research, the research still has value. Such research helps us better understand how different aspects of the natural world relate to one another. It also helps us develop new hypotheses that can then be tested in the future.
Thompson WH, Skau S. On the scope of scientific hypotheses . R Soc Open Sci . 2023;10(8):230607. doi:10.1098/rsos.230607
Taran S, Adhikari NKJ, Fan E. Falsifiability in medicine: what clinicians can learn from Karl Popper [published correction appears in Intensive Care Med. 2021 Jun 17;:]. Intensive Care Med . 2021;47(9):1054-1056. doi:10.1007/s00134-021-06432-z
Eyler AA. Research Methods for Public Health . 1st ed. Springer Publishing Company; 2020. doi:10.1891/9780826182067.0004
Nosek BA, Errington TM. What is replication ? PLoS Biol . 2020;18(3):e3000691. doi:10.1371/journal.pbio.3000691
Aggarwal R, Ranganathan P. Study designs: Part 2 - Descriptive studies . Perspect Clin Res . 2019;10(1):34-36. doi:10.4103/picr.PICR_154_18
Nevid J. Psychology: Concepts and Applications. Wadworth, 2013.
By Kendra Cherry, MSEd Kendra Cherry, MS, is a psychosocial rehabilitation specialist, psychology educator, and author of the "Everything Psychology Book."

What Is A Research (Scientific) Hypothesis? A plain-language explainer + examples
By: Derek Jansen (MBA) | Reviewed By: Dr Eunice Rautenbach | June 2020
If you’re new to the world of research, or it’s your first time writing a dissertation or thesis, you’re probably noticing that the words “research hypothesis” and “scientific hypothesis” are used quite a bit, and you’re wondering what they mean in a research context .
“Hypothesis” is one of those words that people use loosely, thinking they understand what it means. However, it has a very specific meaning within academic research. So, it’s important to understand the exact meaning before you start hypothesizing.
Research Hypothesis 101
- What is a hypothesis ?
- What is a research hypothesis (scientific hypothesis)?
- Requirements for a research hypothesis
- Definition of a research hypothesis
- The null hypothesis
What is a hypothesis?
Let’s start with the general definition of a hypothesis (not a research hypothesis or scientific hypothesis), according to the Cambridge Dictionary:
Hypothesis: an idea or explanation for something that is based on known facts but has not yet been proved.
In other words, it’s a statement that provides an explanation for why or how something works, based on facts (or some reasonable assumptions), but that has not yet been specifically tested . For example, a hypothesis might look something like this:
Hypothesis: sleep impacts academic performance.
This statement predicts that academic performance will be influenced by the amount and/or quality of sleep a student engages in – sounds reasonable, right? It’s based on reasonable assumptions , underpinned by what we currently know about sleep and health (from the existing literature). So, loosely speaking, we could call it a hypothesis, at least by the dictionary definition.
But that’s not good enough…
Unfortunately, that’s not quite sophisticated enough to describe a research hypothesis (also sometimes called a scientific hypothesis), and it wouldn’t be acceptable in a dissertation, thesis or research paper . In the world of academic research, a statement needs a few more criteria to constitute a true research hypothesis .
What is a research hypothesis?
A research hypothesis (also called a scientific hypothesis) is a statement about the expected outcome of a study (for example, a dissertation or thesis). To constitute a quality hypothesis, the statement needs to have three attributes – specificity , clarity and testability .
Let’s take a look at these more closely.
Need a helping hand?
Hypothesis Essential #1: Specificity & Clarity
A good research hypothesis needs to be extremely clear and articulate about both what’ s being assessed (who or what variables are involved ) and the expected outcome (for example, a difference between groups, a relationship between variables, etc.).
Let’s stick with our sleepy students example and look at how this statement could be more specific and clear.
Hypothesis: Students who sleep at least 8 hours per night will, on average, achieve higher grades in standardised tests than students who sleep less than 8 hours a night.
As you can see, the statement is very specific as it identifies the variables involved (sleep hours and test grades), the parties involved (two groups of students), as well as the predicted relationship type (a positive relationship). There’s no ambiguity or uncertainty about who or what is involved in the statement, and the expected outcome is clear.
Contrast that to the original hypothesis we looked at – “Sleep impacts academic performance” – and you can see the difference. “Sleep” and “academic performance” are both comparatively vague , and there’s no indication of what the expected relationship direction is (more sleep or less sleep). As you can see, specificity and clarity are key.
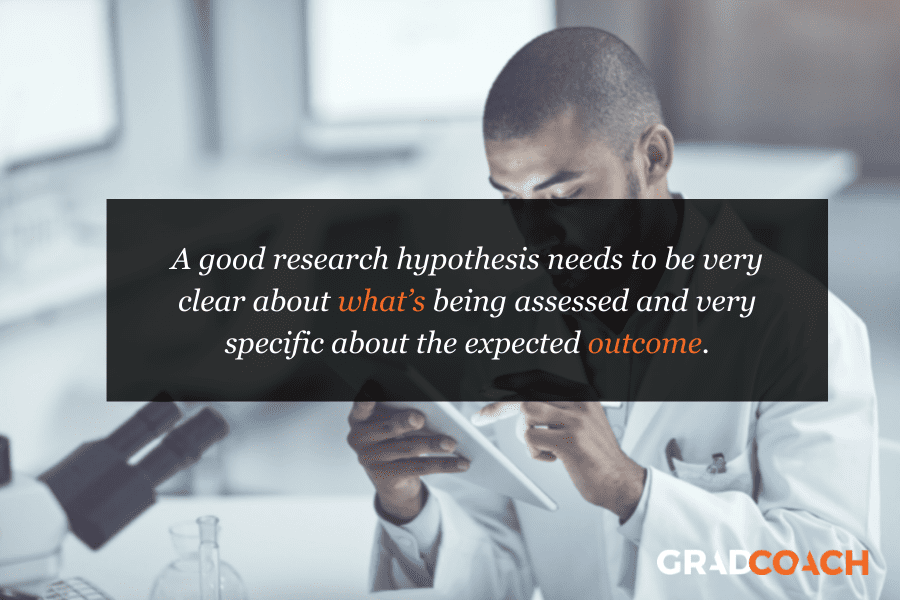
Hypothesis Essential #2: Testability (Provability)
A statement must be testable to qualify as a research hypothesis. In other words, there needs to be a way to prove (or disprove) the statement. If it’s not testable, it’s not a hypothesis – simple as that.
For example, consider the hypothesis we mentioned earlier:
Hypothesis: Students who sleep at least 8 hours per night will, on average, achieve higher grades in standardised tests than students who sleep less than 8 hours a night.
We could test this statement by undertaking a quantitative study involving two groups of students, one that gets 8 or more hours of sleep per night for a fixed period, and one that gets less. We could then compare the standardised test results for both groups to see if there’s a statistically significant difference.
Again, if you compare this to the original hypothesis we looked at – “Sleep impacts academic performance” – you can see that it would be quite difficult to test that statement, primarily because it isn’t specific enough. How much sleep? By who? What type of academic performance?
So, remember the mantra – if you can’t test it, it’s not a hypothesis 🙂
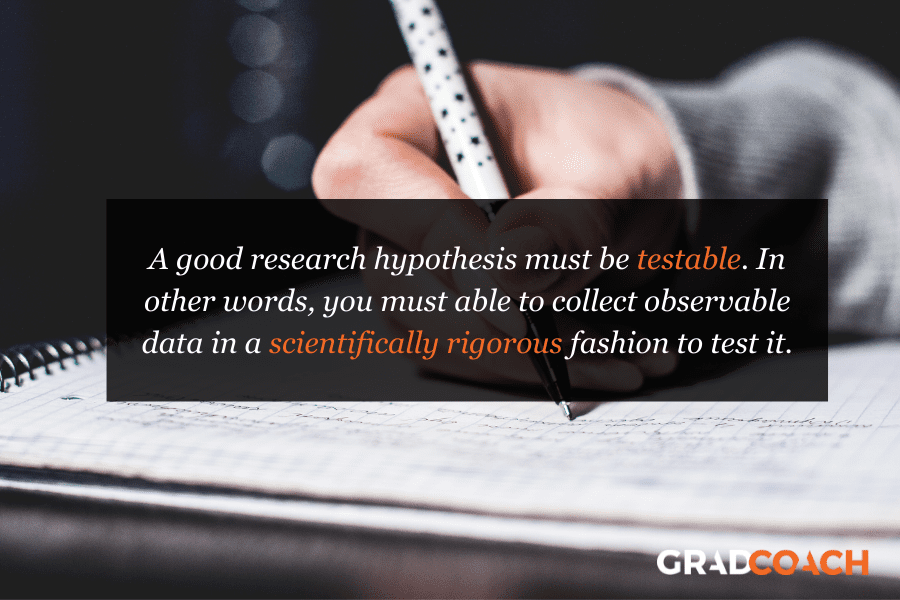
Defining A Research Hypothesis
You’re still with us? Great! Let’s recap and pin down a clear definition of a hypothesis.
A research hypothesis (or scientific hypothesis) is a statement about an expected relationship between variables, or explanation of an occurrence, that is clear, specific and testable.
So, when you write up hypotheses for your dissertation or thesis, make sure that they meet all these criteria. If you do, you’ll not only have rock-solid hypotheses but you’ll also ensure a clear focus for your entire research project.
What about the null hypothesis?
You may have also heard the terms null hypothesis , alternative hypothesis, or H-zero thrown around. At a simple level, the null hypothesis is the counter-proposal to the original hypothesis.
For example, if the hypothesis predicts that there is a relationship between two variables (for example, sleep and academic performance), the null hypothesis would predict that there is no relationship between those variables.
At a more technical level, the null hypothesis proposes that no statistical significance exists in a set of given observations and that any differences are due to chance alone.
And there you have it – hypotheses in a nutshell.
If you have any questions, be sure to leave a comment below and we’ll do our best to help you. If you need hands-on help developing and testing your hypotheses, consider our private coaching service , where we hold your hand through the research journey.
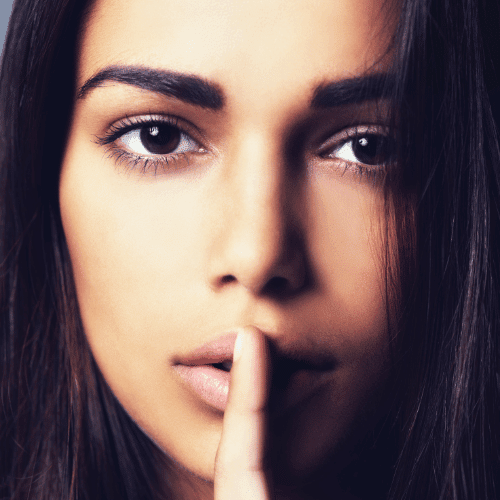
Psst... there’s more!
This post was based on one of our popular Research Bootcamps . If you're working on a research project, you'll definitely want to check this out ...
You Might Also Like:
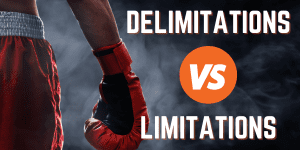
16 Comments
Very useful information. I benefit more from getting more information in this regard.
Very great insight,educative and informative. Please give meet deep critics on many research data of public international Law like human rights, environment, natural resources, law of the sea etc
In a book I read a distinction is made between null, research, and alternative hypothesis. As far as I understand, alternative and research hypotheses are the same. Can you please elaborate? Best Afshin
This is a self explanatory, easy going site. I will recommend this to my friends and colleagues.
Very good definition. How can I cite your definition in my thesis? Thank you. Is nul hypothesis compulsory in a research?
It’s a counter-proposal to be proven as a rejection
Please what is the difference between alternate hypothesis and research hypothesis?
It is a very good explanation. However, it limits hypotheses to statistically tasteable ideas. What about for qualitative researches or other researches that involve quantitative data that don’t need statistical tests?
In qualitative research, one typically uses propositions, not hypotheses.
could you please elaborate it more
I’ve benefited greatly from these notes, thank you.
This is very helpful
well articulated ideas are presented here, thank you for being reliable sources of information
Excellent. Thanks for being clear and sound about the research methodology and hypothesis (quantitative research)
I have only a simple question regarding the null hypothesis. – Is the null hypothesis (Ho) known as the reversible hypothesis of the alternative hypothesis (H1? – How to test it in academic research?
this is very important note help me much more
Trackbacks/Pingbacks
- What Is Research Methodology? Simple Definition (With Examples) - Grad Coach - […] Contrasted to this, a quantitative methodology is typically used when the research aims and objectives are confirmatory in nature. For example,…
Submit a Comment Cancel reply
Your email address will not be published. Required fields are marked *
Save my name, email, and website in this browser for the next time I comment.
- Print Friendly
- Skip to main content
- Skip to primary sidebar
- Skip to footer
- QuestionPro

- Solutions Industries Gaming Automotive Sports and events Education Government Travel & Hospitality Financial Services Healthcare Cannabis Technology Use Case NPS+ Communities Audience Contactless surveys Mobile LivePolls Member Experience GDPR Positive People Science 360 Feedback Surveys
- Resources Blog eBooks Survey Templates Case Studies Training Help center

Home Market Research
Research Hypothesis: What It Is, Types + How to Develop?
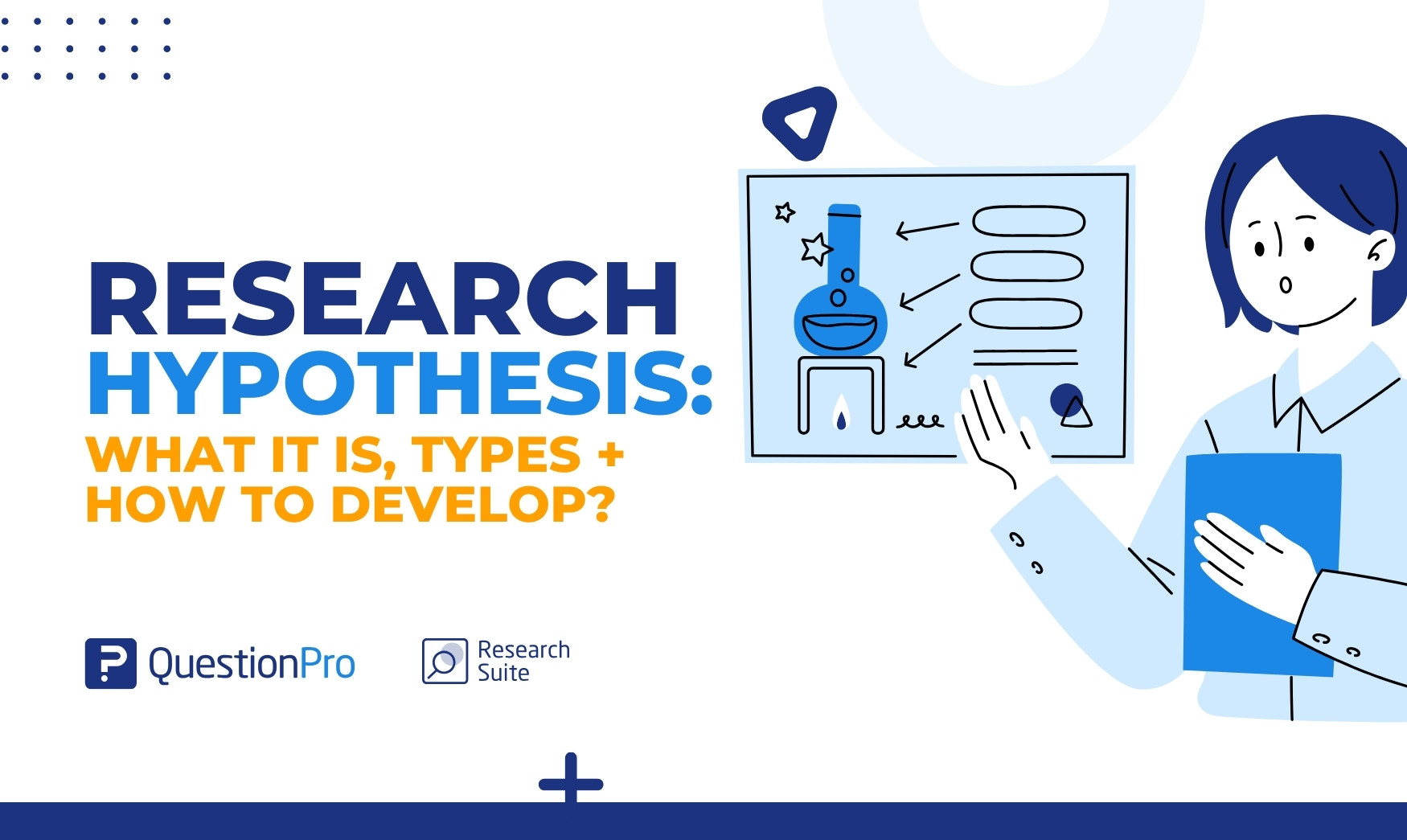
A research study starts with a question. Researchers worldwide ask questions and create research hypotheses. The effectiveness of research relies on developing a good research hypothesis. Examples of research hypotheses can guide researchers in writing effective ones.
In this blog, we’ll learn what a research hypothesis is, why it’s important in research, and the different types used in science. We’ll also guide you through creating your research hypothesis and discussing ways to test and evaluate it.
What is a Research Hypothesis?
A hypothesis is like a guess or idea that you suggest to check if it’s true. A research hypothesis is a statement that brings up a question and predicts what might happen.
It’s really important in the scientific method and is used in experiments to figure things out. Essentially, it’s an educated guess about how things are connected in the research.
A research hypothesis usually includes pointing out the independent variable (the thing they’re changing or studying) and the dependent variable (the result they’re measuring or watching). It helps plan how to gather and analyze data to see if there’s evidence to support or deny the expected connection between these variables.
Importance of Hypothesis in Research
Hypotheses are really important in research. They help design studies, allow for practical testing, and add to our scientific knowledge. Their main role is to organize research projects, making them purposeful, focused, and valuable to the scientific community. Let’s look at some key reasons why they matter:
- A research hypothesis helps test theories.
A hypothesis plays a pivotal role in the scientific method by providing a basis for testing existing theories. For example, a hypothesis might test the predictive power of a psychological theory on human behavior.
- It serves as a great platform for investigation activities.
It serves as a launching pad for investigation activities, which offers researchers a clear starting point. A research hypothesis can explore the relationship between exercise and stress reduction.
- Hypothesis guides the research work or study.
A well-formulated hypothesis guides the entire research process. It ensures that the study remains focused and purposeful. For instance, a hypothesis about the impact of social media on interpersonal relationships provides clear guidance for a study.
- Hypothesis sometimes suggests theories.
In some cases, a hypothesis can suggest new theories or modifications to existing ones. For example, a hypothesis testing the effectiveness of a new drug might prompt a reconsideration of current medical theories.
- It helps in knowing the data needs.
A hypothesis clarifies the data requirements for a study, ensuring that researchers collect the necessary information—a hypothesis guiding the collection of demographic data to analyze the influence of age on a particular phenomenon.
- The hypothesis explains social phenomena.
Hypotheses are instrumental in explaining complex social phenomena. For instance, a hypothesis might explore the relationship between economic factors and crime rates in a given community.
- Hypothesis provides a relationship between phenomena for empirical Testing.
Hypotheses establish clear relationships between phenomena, paving the way for empirical testing. An example could be a hypothesis exploring the correlation between sleep patterns and academic performance.
- It helps in knowing the most suitable analysis technique.
A hypothesis guides researchers in selecting the most appropriate analysis techniques for their data. For example, a hypothesis focusing on the effectiveness of a teaching method may lead to the choice of statistical analyses best suited for educational research.
Characteristics of a Good Research Hypothesis
A hypothesis is a specific idea that you can test in a study. It often comes from looking at past research and theories. A good hypothesis usually starts with a research question that you can explore through background research. For it to be effective, consider these key characteristics:
- Clear and Focused Language: A good hypothesis uses clear and focused language to avoid confusion and ensure everyone understands it.
- Related to the Research Topic: The hypothesis should directly relate to the research topic, acting as a bridge between the specific question and the broader study.
- Testable: An effective hypothesis can be tested, meaning its prediction can be checked with real data to support or challenge the proposed relationship.
- Potential for Exploration: A good hypothesis often comes from a research question that invites further exploration. Doing background research helps find gaps and potential areas to investigate.
- Includes Variables: The hypothesis should clearly state both the independent and dependent variables, specifying the factors being studied and the expected outcomes.
- Ethical Considerations: Check if variables can be manipulated without breaking ethical standards. It’s crucial to maintain ethical research practices.
- Predicts Outcomes: The hypothesis should predict the expected relationship and outcome, acting as a roadmap for the study and guiding data collection and analysis.
- Simple and Concise: A good hypothesis avoids unnecessary complexity and is simple and concise, expressing the essence of the proposed relationship clearly.
- Clear and Assumption-Free: The hypothesis should be clear and free from assumptions about the reader’s prior knowledge, ensuring universal understanding.
- Observable and Testable Results: A strong hypothesis implies research that produces observable and testable results, making sure the study’s outcomes can be effectively measured and analyzed.
When you use these characteristics as a checklist, it can help you create a good research hypothesis. It’ll guide improving and strengthening the hypothesis, identifying any weaknesses, and making necessary changes. Crafting a hypothesis with these features helps you conduct a thorough and insightful research study.
Types of Research Hypotheses
The research hypothesis comes in various types, each serving a specific purpose in guiding the scientific investigation. Knowing the differences will make it easier for you to create your own hypothesis. Here’s an overview of the common types:
01. Null Hypothesis
The null hypothesis states that there is no connection between two considered variables or that two groups are unrelated. As discussed earlier, a hypothesis is an unproven assumption lacking sufficient supporting data. It serves as the statement researchers aim to disprove. It is testable, verifiable, and can be rejected.
For example, if you’re studying the relationship between Project A and Project B, assuming both projects are of equal standard is your null hypothesis. It needs to be specific for your study.
02. Alternative Hypothesis
The alternative hypothesis is basically another option to the null hypothesis. It involves looking for a significant change or alternative that could lead you to reject the null hypothesis. It’s a different idea compared to the null hypothesis.
When you create a null hypothesis, you’re making an educated guess about whether something is true or if there’s a connection between that thing and another variable. If the null view suggests something is correct, the alternative hypothesis says it’s incorrect.
For instance, if your null hypothesis is “I’m going to be $1000 richer,” the alternative hypothesis would be “I’m not going to get $1000 or be richer.”
03. Directional Hypothesis
The directional hypothesis predicts the direction of the relationship between independent and dependent variables. They specify whether the effect will be positive or negative.
If you increase your study hours, you will experience a positive association with your exam scores. This hypothesis suggests that as you increase the independent variable (study hours), there will also be an increase in the dependent variable (exam scores).
04. Non-directional Hypothesis
The non-directional hypothesis predicts the existence of a relationship between variables but does not specify the direction of the effect. It suggests that there will be a significant difference or relationship, but it does not predict the nature of that difference.
For example, you will find no notable difference in test scores between students who receive the educational intervention and those who do not. However, once you compare the test scores of the two groups, you will notice an important difference.
05. Simple Hypothesis
A simple hypothesis predicts a relationship between one dependent variable and one independent variable without specifying the nature of that relationship. It’s simple and usually used when we don’t know much about how the two things are connected.
For example, if you adopt effective study habits, you will achieve higher exam scores than those with poor study habits.
06. Complex Hypothesis
A complex hypothesis is an idea that specifies a relationship between multiple independent and dependent variables. It is a more detailed idea than a simple hypothesis.
While a simple view suggests a straightforward cause-and-effect relationship between two things, a complex hypothesis involves many factors and how they’re connected to each other.
For example, when you increase your study time, you tend to achieve higher exam scores. The connection between your study time and exam performance is affected by various factors, including the quality of your sleep, your motivation levels, and the effectiveness of your study techniques.
If you sleep well, stay highly motivated, and use effective study strategies, you may observe a more robust positive correlation between the time you spend studying and your exam scores, unlike those who may lack these factors.
07. Associative Hypothesis
An associative hypothesis proposes a connection between two things without saying that one causes the other. Basically, it suggests that when one thing changes, the other changes too, but it doesn’t claim that one thing is causing the change in the other.
For example, you will likely notice higher exam scores when you increase your study time. You can recognize an association between your study time and exam scores in this scenario.
Your hypothesis acknowledges a relationship between the two variables—your study time and exam scores—without asserting that increased study time directly causes higher exam scores. You need to consider that other factors, like motivation or learning style, could affect the observed association.
08. Causal Hypothesis
A causal hypothesis proposes a cause-and-effect relationship between two variables. It suggests that changes in one variable directly cause changes in another variable.
For example, when you increase your study time, you experience higher exam scores. This hypothesis suggests a direct cause-and-effect relationship, indicating that the more time you spend studying, the higher your exam scores. It assumes that changes in your study time directly influence changes in your exam performance.
09. Empirical Hypothesis
An empirical hypothesis is a statement based on things we can see and measure. It comes from direct observation or experiments and can be tested with real-world evidence. If an experiment proves a theory, it supports the idea and shows it’s not just a guess. This makes the statement more reliable than a wild guess.
For example, if you increase the dosage of a certain medication, you might observe a quicker recovery time for patients. Imagine you’re in charge of a clinical trial. In this trial, patients are given varying dosages of the medication, and you measure and compare their recovery times. This allows you to directly see the effects of different dosages on how fast patients recover.
This way, you can create a research hypothesis: “Increasing the dosage of a certain medication will lead to a faster recovery time for patients.”
10. Statistical Hypothesis
A statistical hypothesis is a statement or assumption about a population parameter that is the subject of an investigation. It serves as the basis for statistical analysis and testing. It is often tested using statistical methods to draw inferences about the larger population.
In a hypothesis test, statistical evidence is collected to either reject the null hypothesis in favor of the alternative hypothesis or fail to reject the null hypothesis due to insufficient evidence.
For example, let’s say you’re testing a new medicine. Your hypothesis could be that the medicine doesn’t really help patients get better. So, you collect data and use statistics to see if your guess is right or if the medicine actually makes a difference.
If the data strongly shows that the medicine does help, you say your guess was wrong, and the medicine does make a difference. But if the proof isn’t strong enough, you can stick with your original guess because you didn’t get enough evidence to change your mind.
How to Develop a Research Hypotheses?
Step 1: identify your research problem or topic..
Define the area of interest or the problem you want to investigate. Make sure it’s clear and well-defined.
Start by asking a question about your chosen topic. Consider the limitations of your research and create a straightforward problem related to your topic. Once you’ve done that, you can develop and test a hypothesis with evidence.
Step 2: Conduct a literature review
Review existing literature related to your research problem. This will help you understand the current state of knowledge in the field, identify gaps, and build a foundation for your hypothesis. Consider the following questions:
- What existing research has been conducted on your chosen topic?
- Are there any gaps or unanswered questions in the current literature?
- How will the existing literature contribute to the foundation of your research?
Step 3: Formulate your research question
Based on your literature review, create a specific and concise research question that addresses your identified problem. Your research question should be clear, focused, and relevant to your field of study.
Step 4: Identify variables
Determine the key variables involved in your research question. Variables are the factors or phenomena that you will study and manipulate to test your hypothesis.
- Independent Variable: The variable you manipulate or control.
- Dependent Variable: The variable you measure to observe the effect of the independent variable.
Step 5: State the Null hypothesis
The null hypothesis is a statement that there is no significant difference or effect. It serves as a baseline for comparison with the alternative hypothesis.
Step 6: Select appropriate methods for testing the hypothesis
Choose research methods that align with your study objectives, such as experiments, surveys, or observational studies. The selected methods enable you to test your research hypothesis effectively.
Creating a research hypothesis usually takes more than one try. Expect to make changes as you collect data. It’s normal to test and say no to a few hypotheses before you find the right answer to your research question.
Testing and Evaluating Hypotheses
Testing hypotheses is a really important part of research. It’s like the practical side of things. Here, real-world evidence will help you determine how different things are connected. Let’s explore the main steps in hypothesis testing:
- State your research hypothesis.
Before testing, clearly articulate your research hypothesis. This involves framing both a null hypothesis, suggesting no significant effect or relationship, and an alternative hypothesis, proposing the expected outcome.
- Collect data strategically.
Plan how you will gather information in a way that fits your study. Make sure your data collection method matches the things you’re studying.
Whether through surveys, observations, or experiments, this step demands precision and adherence to the established methodology. The quality of data collected directly influences the credibility of study outcomes.
- Perform an appropriate statistical test.
Choose a statistical test that aligns with the nature of your data and the hypotheses being tested. Whether it’s a t-test, chi-square test, ANOVA, or regression analysis, selecting the right statistical tool is paramount for accurate and reliable results.
- Decide if your idea was right or wrong.
Following the statistical analysis, evaluate the results in the context of your null hypothesis. You need to decide if you should reject your null hypothesis or not.
- Share what you found.
When discussing what you found in your research, be clear and organized. Say whether your idea was supported or not, and talk about what your results mean. Also, mention any limits to your study and suggest ideas for future research.
The Role of QuestionPro to Develop a Good Research Hypothesis
QuestionPro is a survey and research platform that provides tools for creating, distributing, and analyzing surveys. It plays a crucial role in the research process, especially when you’re in the initial stages of hypothesis development. Here’s how QuestionPro can help you to develop a good research hypothesis:
- Survey design and data collection: You can use the platform to create targeted questions that help you gather relevant data.
- Exploratory research: Through surveys and feedback mechanisms on QuestionPro, you can conduct exploratory research to understand the landscape of a particular subject.
- Literature review and background research: QuestionPro surveys can collect sample population opinions, experiences, and preferences. This data and a thorough literature evaluation can help you generate a well-grounded hypothesis by improving your research knowledge.
- Identifying variables: Using targeted survey questions, you can identify relevant variables related to their research topic.
- Testing assumptions: You can use surveys to informally test certain assumptions or hypotheses before formalizing a research hypothesis.
- Data analysis tools: QuestionPro provides tools for analyzing survey data. You can use these tools to identify the collected data’s patterns, correlations, or trends.
- Refining your hypotheses: As you collect data through QuestionPro, you can adjust your hypotheses based on the real-world responses you receive.
A research hypothesis is like a guide for researchers in science. It’s a well-thought-out idea that has been thoroughly tested. This idea is crucial as researchers can explore different fields, such as medicine, social sciences, and natural sciences. The research hypothesis links theories to real-world evidence and gives researchers a clear path to explore and make discoveries.
QuestionPro Research Suite is a helpful tool for researchers. It makes creating surveys, collecting data, and analyzing information easily. It supports all kinds of research, from exploring new ideas to forming hypotheses. With a focus on using data, it helps researchers do their best work.
Are you interested in learning more about QuestionPro Research Suite? Take advantage of QuestionPro’s free trial to get an initial look at its capabilities and realize the full potential of your research efforts.
LEARN MORE FREE TRIAL
MORE LIKE THIS
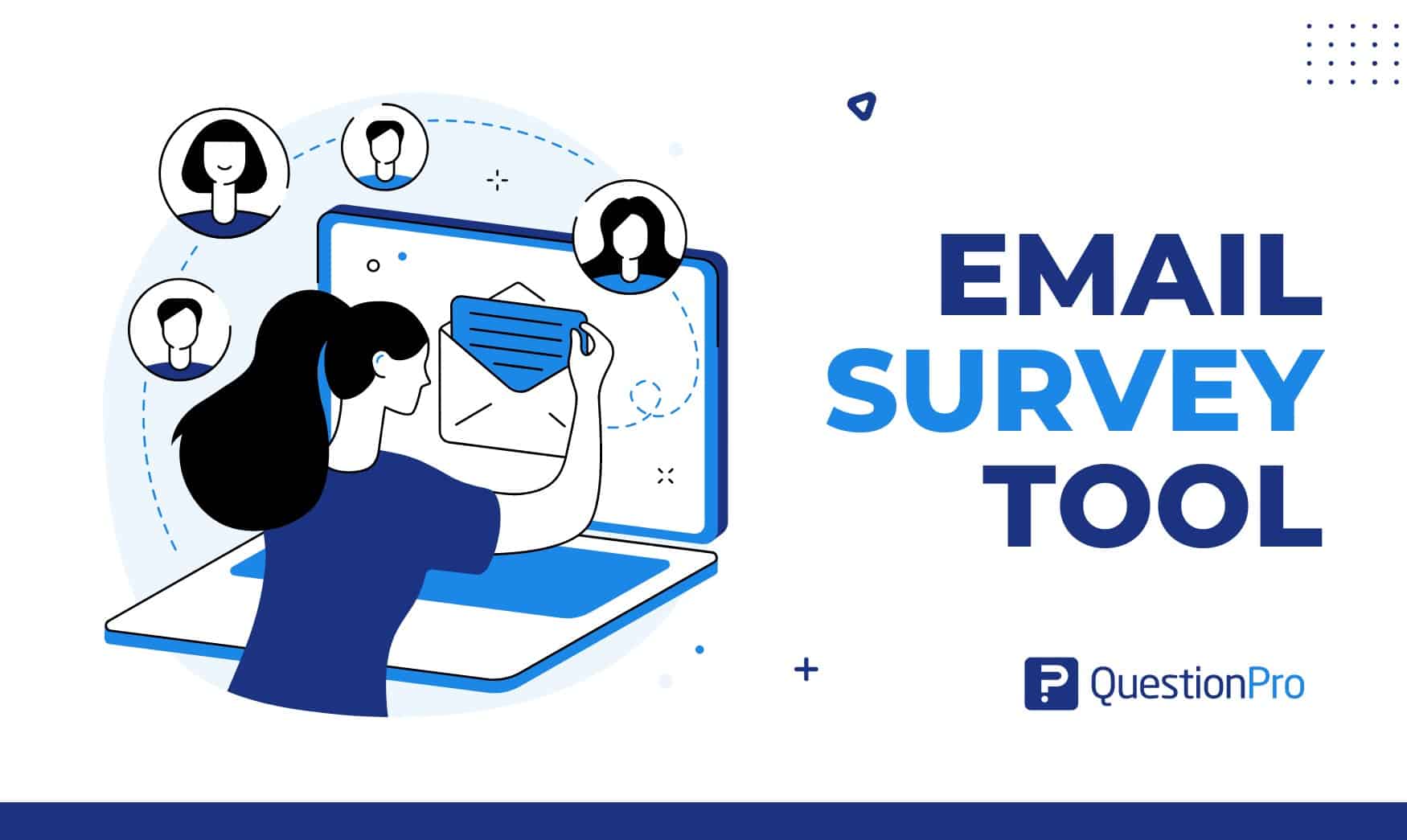
The Best Email Survey Tool to Boost Your Feedback Game
May 7, 2024
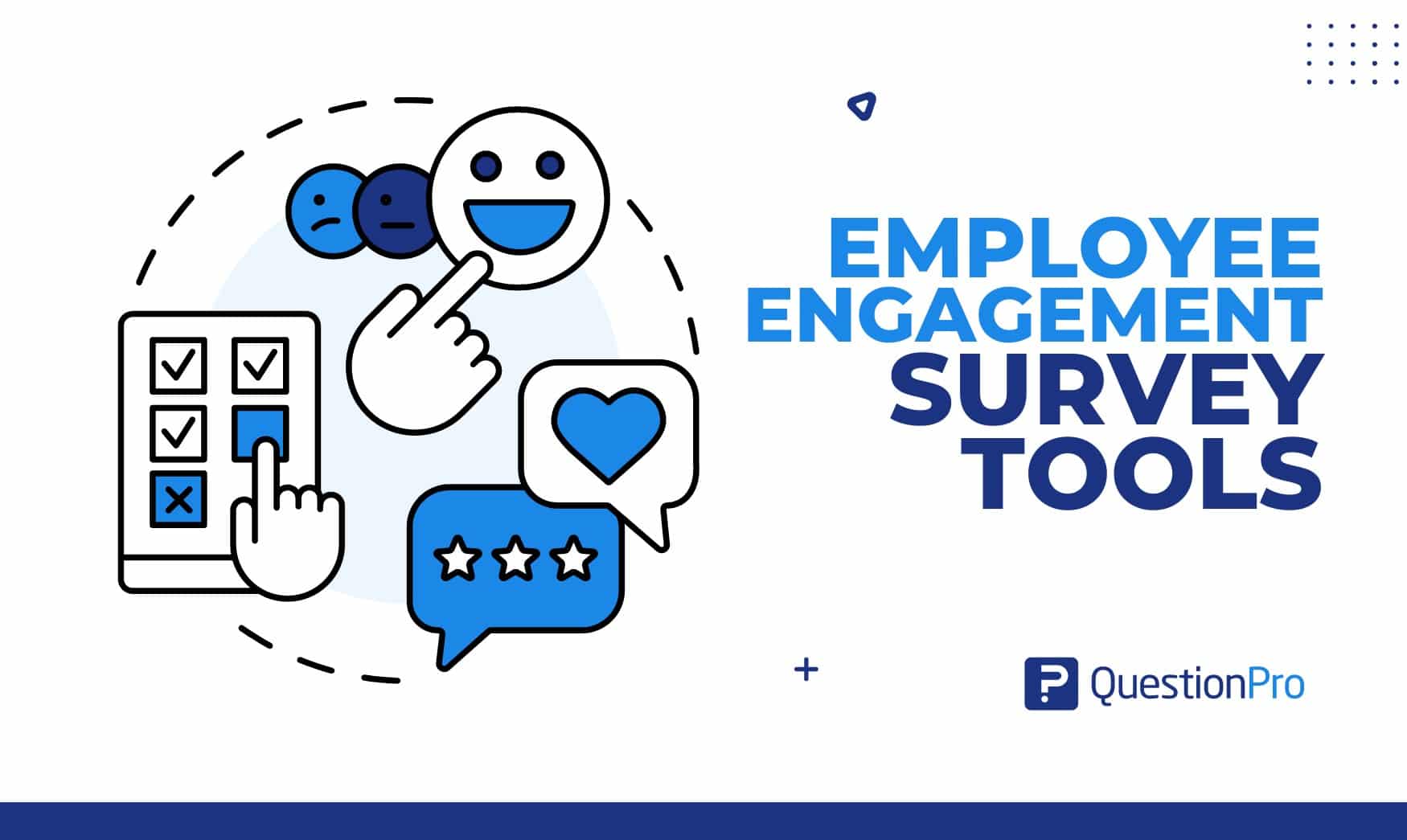
Top 10 Employee Engagement Survey Tools
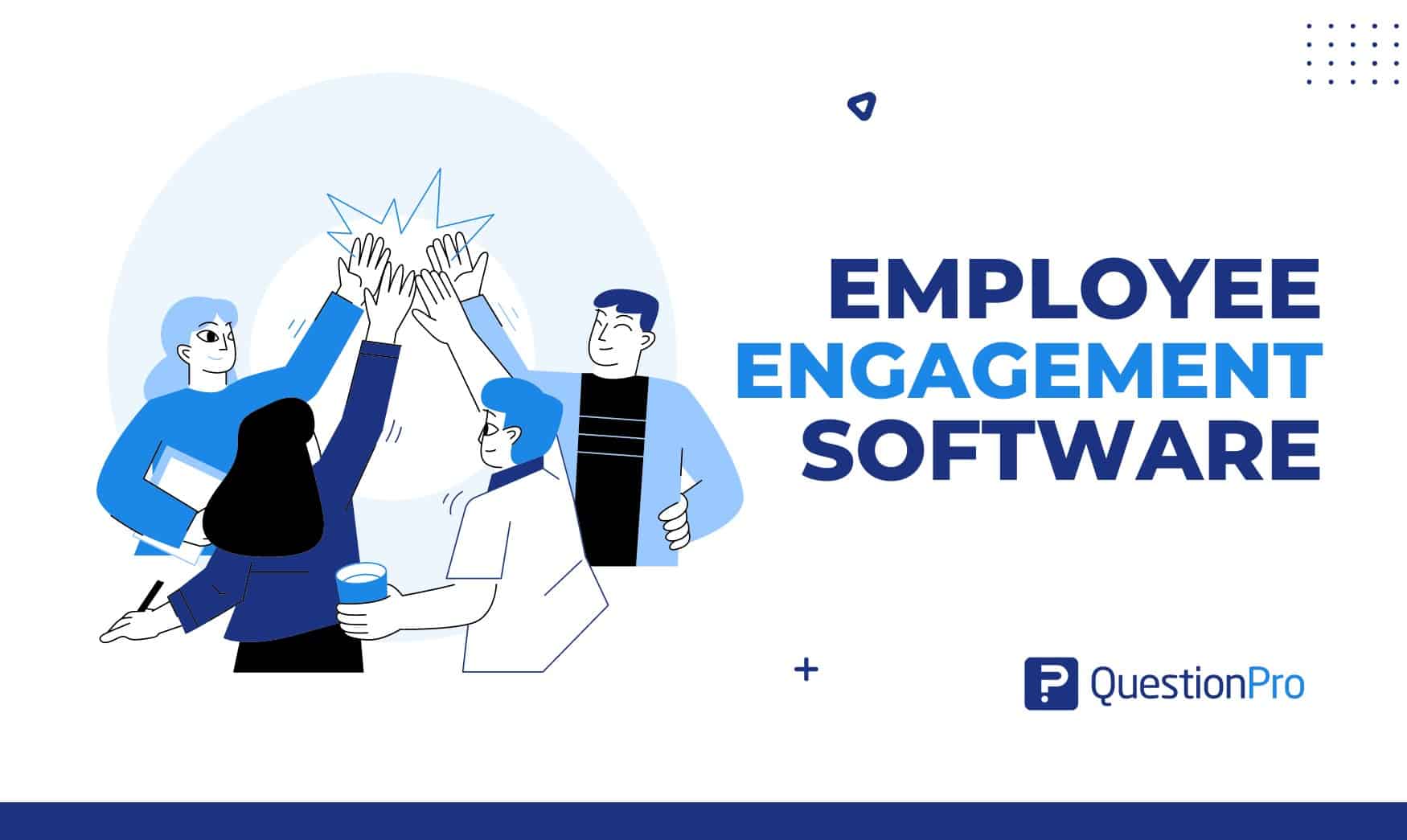
Top 20 Employee Engagement Software Solutions
May 3, 2024
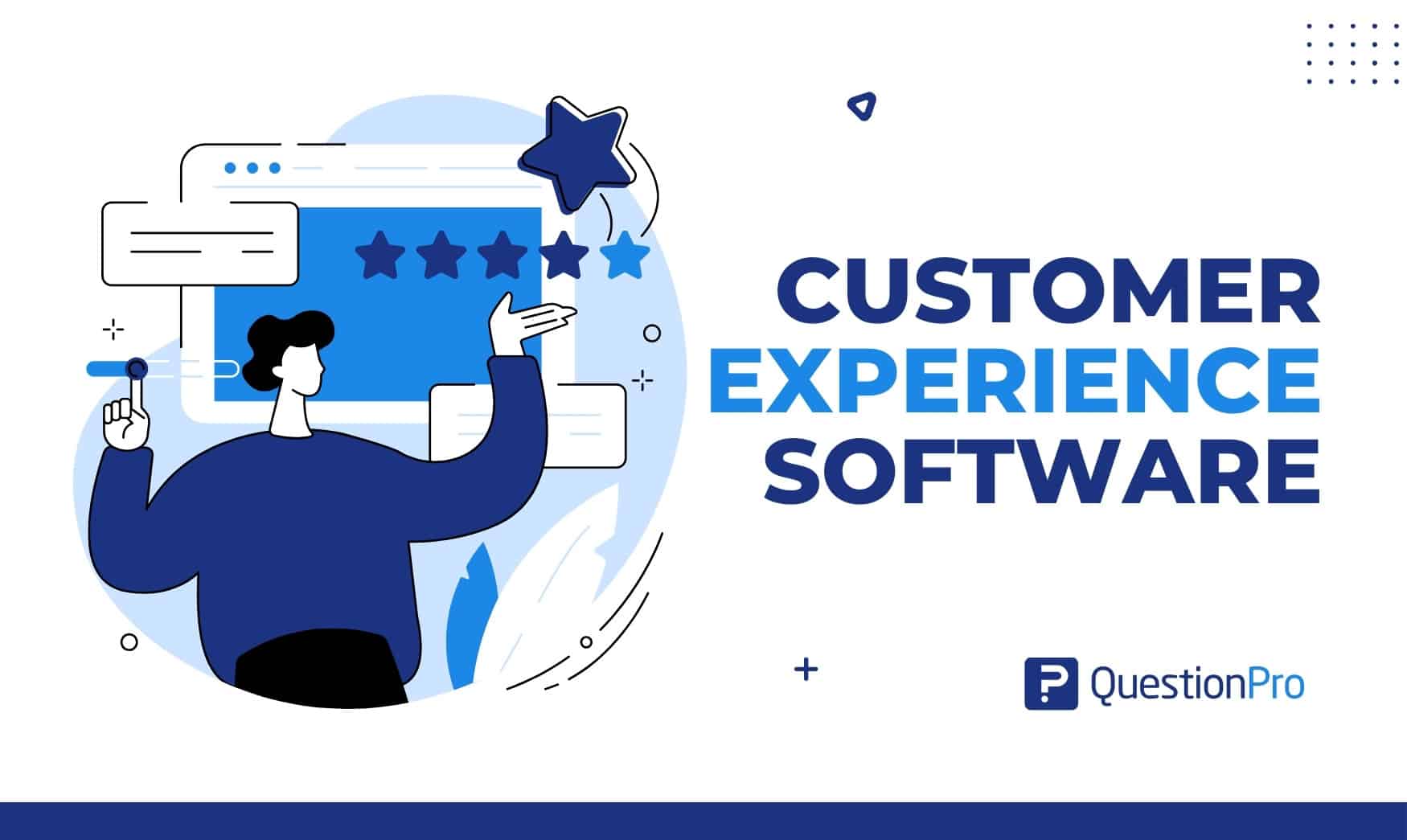
15 Best Customer Experience Software of 2024
May 2, 2024
Other categories
- Academic Research
- Artificial Intelligence
- Assessments
- Brand Awareness
- Case Studies
- Communities
- Consumer Insights
- Customer effort score
- Customer Engagement
- Customer Experience
- Customer Loyalty
- Customer Research
- Customer Satisfaction
- Employee Benefits
- Employee Engagement
- Employee Retention
- Friday Five
- General Data Protection Regulation
- Insights Hub
- Life@QuestionPro
- Market Research
- Mobile diaries
- Mobile Surveys
- New Features
- Online Communities
- Question Types
- Questionnaire
- QuestionPro Products
- Release Notes
- Research Tools and Apps
- Revenue at Risk
- Survey Templates
- Training Tips
- Uncategorized
- Video Learning Series
- What’s Coming Up
- Workforce Intelligence
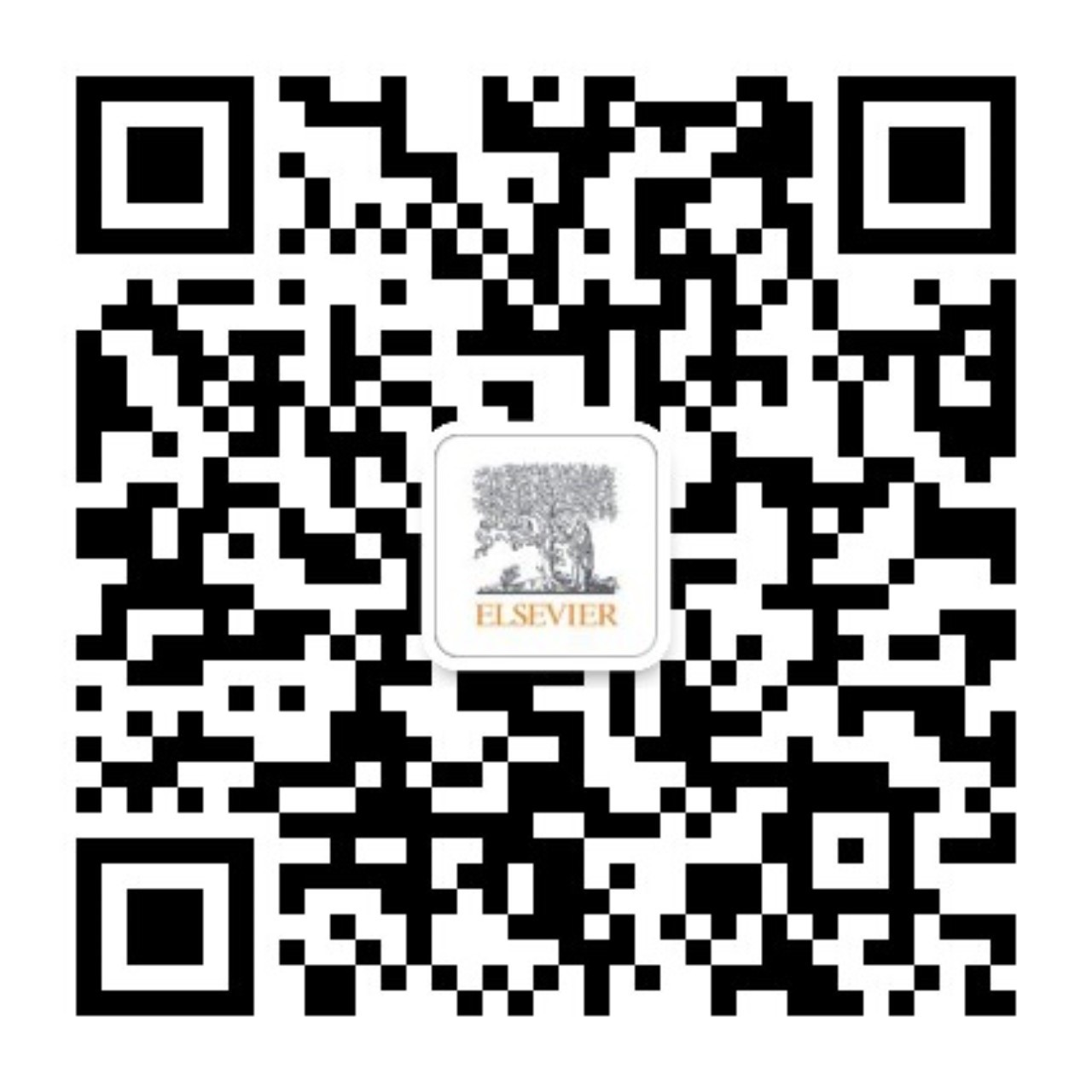
- Manuscript Preparation
What is and How to Write a Good Hypothesis in Research?
- 4 minute read
- 309.6K views
Table of Contents
One of the most important aspects of conducting research is constructing a strong hypothesis. But what makes a hypothesis in research effective? In this article, we’ll look at the difference between a hypothesis and a research question, as well as the elements of a good hypothesis in research. We’ll also include some examples of effective hypotheses, and what pitfalls to avoid.
What is a Hypothesis in Research?
Simply put, a hypothesis is a research question that also includes the predicted or expected result of the research. Without a hypothesis, there can be no basis for a scientific or research experiment. As such, it is critical that you carefully construct your hypothesis by being deliberate and thorough, even before you set pen to paper. Unless your hypothesis is clearly and carefully constructed, any flaw can have an adverse, and even grave, effect on the quality of your experiment and its subsequent results.
Research Question vs Hypothesis
It’s easy to confuse research questions with hypotheses, and vice versa. While they’re both critical to the Scientific Method, they have very specific differences. Primarily, a research question, just like a hypothesis, is focused and concise. But a hypothesis includes a prediction based on the proposed research, and is designed to forecast the relationship of and between two (or more) variables. Research questions are open-ended, and invite debate and discussion, while hypotheses are closed, e.g. “The relationship between A and B will be C.”
A hypothesis is generally used if your research topic is fairly well established, and you are relatively certain about the relationship between the variables that will be presented in your research. Since a hypothesis is ideally suited for experimental studies, it will, by its very existence, affect the design of your experiment. The research question is typically used for new topics that have not yet been researched extensively. Here, the relationship between different variables is less known. There is no prediction made, but there may be variables explored. The research question can be casual in nature, simply trying to understand if a relationship even exists, descriptive or comparative.
How to Write Hypothesis in Research
Writing an effective hypothesis starts before you even begin to type. Like any task, preparation is key, so you start first by conducting research yourself, and reading all you can about the topic that you plan to research. From there, you’ll gain the knowledge you need to understand where your focus within the topic will lie.
Remember that a hypothesis is a prediction of the relationship that exists between two or more variables. Your job is to write a hypothesis, and design the research, to “prove” whether or not your prediction is correct. A common pitfall is to use judgments that are subjective and inappropriate for the construction of a hypothesis. It’s important to keep the focus and language of your hypothesis objective.
An effective hypothesis in research is clearly and concisely written, and any terms or definitions clarified and defined. Specific language must also be used to avoid any generalities or assumptions.
Use the following points as a checklist to evaluate the effectiveness of your research hypothesis:
- Predicts the relationship and outcome
- Simple and concise – avoid wordiness
- Clear with no ambiguity or assumptions about the readers’ knowledge
- Observable and testable results
- Relevant and specific to the research question or problem
Research Hypothesis Example
Perhaps the best way to evaluate whether or not your hypothesis is effective is to compare it to those of your colleagues in the field. There is no need to reinvent the wheel when it comes to writing a powerful research hypothesis. As you’re reading and preparing your hypothesis, you’ll also read other hypotheses. These can help guide you on what works, and what doesn’t, when it comes to writing a strong research hypothesis.
Here are a few generic examples to get you started.
Eating an apple each day, after the age of 60, will result in a reduction of frequency of physician visits.
Budget airlines are more likely to receive more customer complaints. A budget airline is defined as an airline that offers lower fares and fewer amenities than a traditional full-service airline. (Note that the term “budget airline” is included in the hypothesis.
Workplaces that offer flexible working hours report higher levels of employee job satisfaction than workplaces with fixed hours.
Each of the above examples are specific, observable and measurable, and the statement of prediction can be verified or shown to be false by utilizing standard experimental practices. It should be noted, however, that often your hypothesis will change as your research progresses.
Language Editing Plus
Elsevier’s Language Editing Plus service can help ensure that your research hypothesis is well-designed, and articulates your research and conclusions. Our most comprehensive editing package, you can count on a thorough language review by native-English speakers who are PhDs or PhD candidates. We’ll check for effective logic and flow of your manuscript, as well as document formatting for your chosen journal, reference checks, and much more.
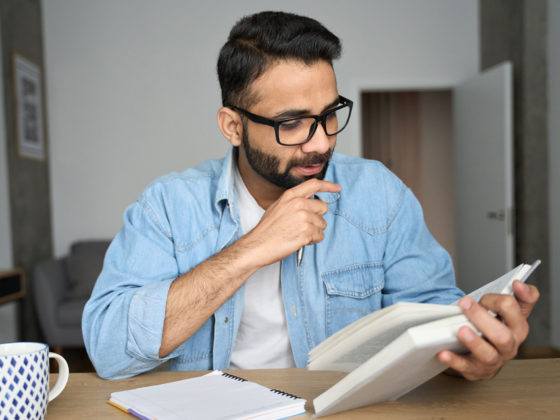
- Research Process
Systematic Literature Review or Literature Review?
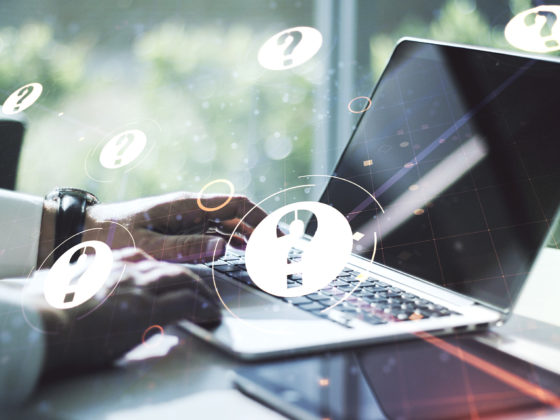
What is a Problem Statement? [with examples]
You may also like.
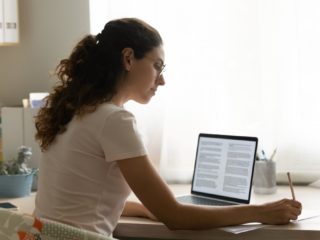
Make Hook, Line, and Sinker: The Art of Crafting Engaging Introductions
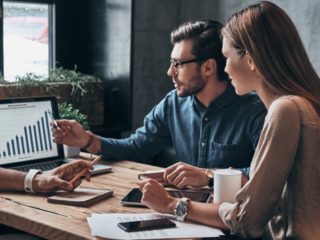
Can Describing Study Limitations Improve the Quality of Your Paper?
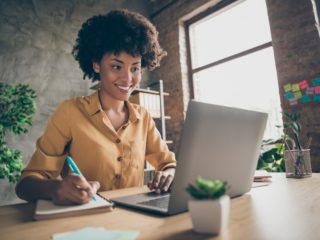
A Guide to Crafting Shorter, Impactful Sentences in Academic Writing
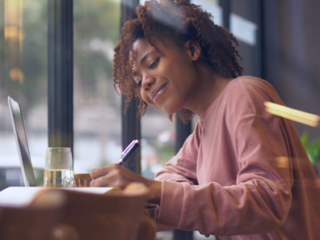

6 Steps to Write an Excellent Discussion in Your Manuscript
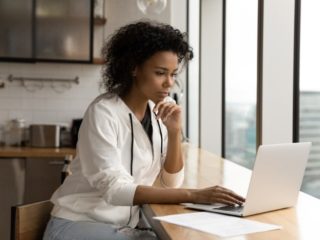
How to Write Clear and Crisp Civil Engineering Papers? Here are 5 Key Tips to Consider
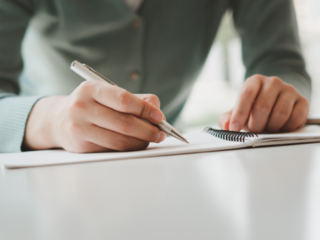
The Clear Path to An Impactful Paper: ②
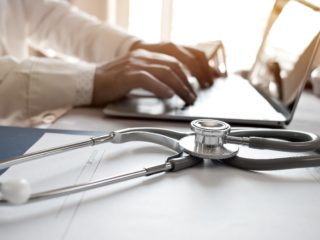
The Essentials of Writing to Communicate Research in Medicine
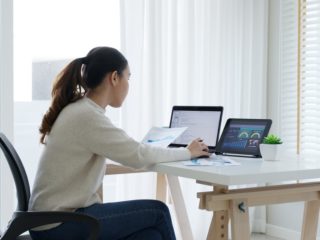
Changing Lines: Sentence Patterns in Academic Writing
Input your search keywords and press Enter.

How to Develop a Good Research Hypothesis
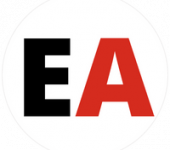
The story of a research study begins by asking a question. Researchers all around the globe are asking curious questions and formulating research hypothesis. However, whether the research study provides an effective conclusion depends on how well one develops a good research hypothesis. Research hypothesis examples could help researchers get an idea as to how to write a good research hypothesis.
This blog will help you understand what is a research hypothesis, its characteristics and, how to formulate a research hypothesis
Table of Contents
What is Hypothesis?
Hypothesis is an assumption or an idea proposed for the sake of argument so that it can be tested. It is a precise, testable statement of what the researchers predict will be outcome of the study. Hypothesis usually involves proposing a relationship between two variables: the independent variable (what the researchers change) and the dependent variable (what the research measures).
What is a Research Hypothesis?
Research hypothesis is a statement that introduces a research question and proposes an expected result. It is an integral part of the scientific method that forms the basis of scientific experiments. Therefore, you need to be careful and thorough when building your research hypothesis. A minor flaw in the construction of your hypothesis could have an adverse effect on your experiment. In research, there is a convention that the hypothesis is written in two forms, the null hypothesis, and the alternative hypothesis (called the experimental hypothesis when the method of investigation is an experiment).
Characteristics of a Good Research Hypothesis
As the hypothesis is specific, there is a testable prediction about what you expect to happen in a study. You may consider drawing hypothesis from previously published research based on the theory.
A good research hypothesis involves more effort than just a guess. In particular, your hypothesis may begin with a question that could be further explored through background research.
To help you formulate a promising research hypothesis, you should ask yourself the following questions:
- Is the language clear and focused?
- What is the relationship between your hypothesis and your research topic?
- Is your hypothesis testable? If yes, then how?
- What are the possible explanations that you might want to explore?
- Does your hypothesis include both an independent and dependent variable?
- Can you manipulate your variables without hampering the ethical standards?
- Does your research predict the relationship and outcome?
- Is your research simple and concise (avoids wordiness)?
- Is it clear with no ambiguity or assumptions about the readers’ knowledge
- Is your research observable and testable results?
- Is it relevant and specific to the research question or problem?
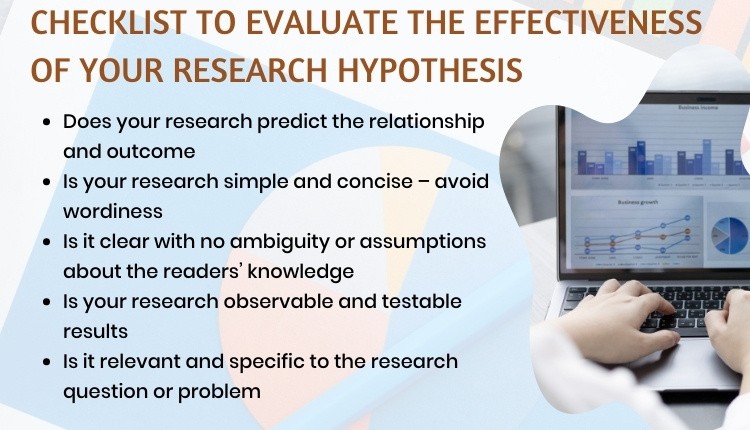
The questions listed above can be used as a checklist to make sure your hypothesis is based on a solid foundation. Furthermore, it can help you identify weaknesses in your hypothesis and revise it if necessary.
Source: Educational Hub
How to formulate a research hypothesis.
A testable hypothesis is not a simple statement. It is rather an intricate statement that needs to offer a clear introduction to a scientific experiment, its intentions, and the possible outcomes. However, there are some important things to consider when building a compelling hypothesis.
1. State the problem that you are trying to solve.
Make sure that the hypothesis clearly defines the topic and the focus of the experiment.
2. Try to write the hypothesis as an if-then statement.
Follow this template: If a specific action is taken, then a certain outcome is expected.
3. Define the variables
Independent variables are the ones that are manipulated, controlled, or changed. Independent variables are isolated from other factors of the study.
Dependent variables , as the name suggests are dependent on other factors of the study. They are influenced by the change in independent variable.
4. Scrutinize the hypothesis
Evaluate assumptions, predictions, and evidence rigorously to refine your understanding.
Types of Research Hypothesis
The types of research hypothesis are stated below:
1. Simple Hypothesis
It predicts the relationship between a single dependent variable and a single independent variable.
2. Complex Hypothesis
It predicts the relationship between two or more independent and dependent variables.
3. Directional Hypothesis
It specifies the expected direction to be followed to determine the relationship between variables and is derived from theory. Furthermore, it implies the researcher’s intellectual commitment to a particular outcome.
4. Non-directional Hypothesis
It does not predict the exact direction or nature of the relationship between the two variables. The non-directional hypothesis is used when there is no theory involved or when findings contradict previous research.
5. Associative and Causal Hypothesis
The associative hypothesis defines interdependency between variables. A change in one variable results in the change of the other variable. On the other hand, the causal hypothesis proposes an effect on the dependent due to manipulation of the independent variable.
6. Null Hypothesis
Null hypothesis states a negative statement to support the researcher’s findings that there is no relationship between two variables. There will be no changes in the dependent variable due the manipulation of the independent variable. Furthermore, it states results are due to chance and are not significant in terms of supporting the idea being investigated.
7. Alternative Hypothesis
It states that there is a relationship between the two variables of the study and that the results are significant to the research topic. An experimental hypothesis predicts what changes will take place in the dependent variable when the independent variable is manipulated. Also, it states that the results are not due to chance and that they are significant in terms of supporting the theory being investigated.
Research Hypothesis Examples of Independent and Dependent Variables
Research Hypothesis Example 1 The greater number of coal plants in a region (independent variable) increases water pollution (dependent variable). If you change the independent variable (building more coal factories), it will change the dependent variable (amount of water pollution).
Research Hypothesis Example 2 What is the effect of diet or regular soda (independent variable) on blood sugar levels (dependent variable)? If you change the independent variable (the type of soda you consume), it will change the dependent variable (blood sugar levels)
You should not ignore the importance of the above steps. The validity of your experiment and its results rely on a robust testable hypothesis. Developing a strong testable hypothesis has few advantages, it compels us to think intensely and specifically about the outcomes of a study. Consequently, it enables us to understand the implication of the question and the different variables involved in the study. Furthermore, it helps us to make precise predictions based on prior research. Hence, forming a hypothesis would be of great value to the research. Here are some good examples of testable hypotheses.
More importantly, you need to build a robust testable research hypothesis for your scientific experiments. A testable hypothesis is a hypothesis that can be proved or disproved as a result of experimentation.
Importance of a Testable Hypothesis
To devise and perform an experiment using scientific method, you need to make sure that your hypothesis is testable. To be considered testable, some essential criteria must be met:
- There must be a possibility to prove that the hypothesis is true.
- There must be a possibility to prove that the hypothesis is false.
- The results of the hypothesis must be reproducible.
Without these criteria, the hypothesis and the results will be vague. As a result, the experiment will not prove or disprove anything significant.
What are your experiences with building hypotheses for scientific experiments? What challenges did you face? How did you overcome these challenges? Please share your thoughts with us in the comments section.
Frequently Asked Questions
The steps to write a research hypothesis are: 1. Stating the problem: Ensure that the hypothesis defines the research problem 2. Writing a hypothesis as an 'if-then' statement: Include the action and the expected outcome of your study by following a ‘if-then’ structure. 3. Defining the variables: Define the variables as Dependent or Independent based on their dependency to other factors. 4. Scrutinizing the hypothesis: Identify the type of your hypothesis
Hypothesis testing is a statistical tool which is used to make inferences about a population data to draw conclusions for a particular hypothesis.
Hypothesis in statistics is a formal statement about the nature of a population within a structured framework of a statistical model. It is used to test an existing hypothesis by studying a population.
Research hypothesis is a statement that introduces a research question and proposes an expected result. It forms the basis of scientific experiments.
The different types of hypothesis in research are: • Null hypothesis: Null hypothesis is a negative statement to support the researcher’s findings that there is no relationship between two variables. • Alternate hypothesis: Alternate hypothesis predicts the relationship between the two variables of the study. • Directional hypothesis: Directional hypothesis specifies the expected direction to be followed to determine the relationship between variables. • Non-directional hypothesis: Non-directional hypothesis does not predict the exact direction or nature of the relationship between the two variables. • Simple hypothesis: Simple hypothesis predicts the relationship between a single dependent variable and a single independent variable. • Complex hypothesis: Complex hypothesis predicts the relationship between two or more independent and dependent variables. • Associative and casual hypothesis: Associative and casual hypothesis predicts the relationship between two or more independent and dependent variables. • Empirical hypothesis: Empirical hypothesis can be tested via experiments and observation. • Statistical hypothesis: A statistical hypothesis utilizes statistical models to draw conclusions about broader populations.

Wow! You really simplified your explanation that even dummies would find it easy to comprehend. Thank you so much.
Thanks a lot for your valuable guidance.
I enjoy reading the post. Hypotheses are actually an intrinsic part in a study. It bridges the research question and the methodology of the study.
Useful piece!
This is awesome.Wow.
It very interesting to read the topic, can you guide me any specific example of hypothesis process establish throw the Demand and supply of the specific product in market
Nicely explained
It is really a useful for me Kindly give some examples of hypothesis
It was a well explained content ,can you please give me an example with the null and alternative hypothesis illustrated
clear and concise. thanks.
So Good so Amazing
Good to learn
Thanks a lot for explaining to my level of understanding
Explained well and in simple terms. Quick read! Thank you
It awesome. It has really positioned me in my research project
Rate this article Cancel Reply
Your email address will not be published.
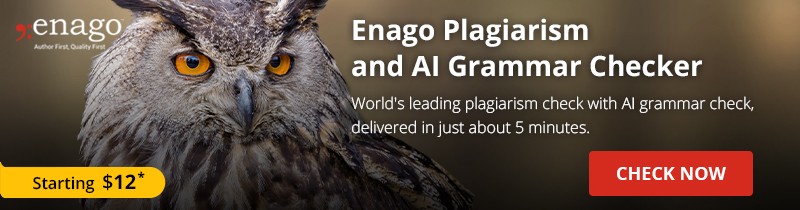
Enago Academy's Most Popular Articles
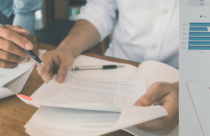
- Reporting Research
Choosing the Right Analytical Approach: Thematic analysis vs. content analysis for data interpretation
In research, choosing the right approach to understand data is crucial for deriving meaningful insights.…
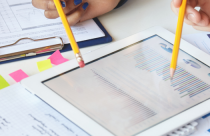
Comparing Cross Sectional and Longitudinal Studies: 5 steps for choosing the right approach
The process of choosing the right research design can put ourselves at the crossroads of…
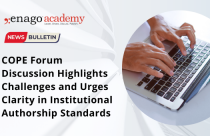
- Industry News
COPE Forum Discussion Highlights Challenges and Urges Clarity in Institutional Authorship Standards
The COPE forum discussion held in December 2023 initiated with a fundamental question — is…
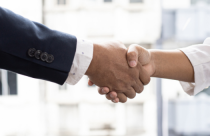
- Career Corner
Unlocking the Power of Networking in Academic Conferences
Embarking on your first academic conference experience? Fear not, we got you covered! Academic conferences…
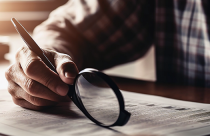
Research Recommendations – Guiding policy-makers for evidence-based decision making
Research recommendations play a crucial role in guiding scholars and researchers toward fruitful avenues of…
Choosing the Right Analytical Approach: Thematic analysis vs. content analysis for…
Comparing Cross Sectional and Longitudinal Studies: 5 steps for choosing the right…
How to Design Effective Research Questionnaires for Robust Findings

Sign-up to read more
Subscribe for free to get unrestricted access to all our resources on research writing and academic publishing including:
- 2000+ blog articles
- 50+ Webinars
- 10+ Expert podcasts
- 50+ Infographics
- 10+ Checklists
- Research Guides
We hate spam too. We promise to protect your privacy and never spam you.
I am looking for Editing/ Proofreading services for my manuscript Tentative date of next journal submission:
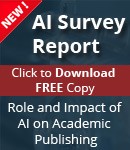
As a researcher, what do you consider most when choosing an image manipulation detector?
You are using an outdated browser. Please upgrade your browser .
T4Tutorials.com
Research hypothesis – types, examples characteristics, and sources, research hypothesis.
A research hypothesis is referred to as a scientific hypothesis. This is a clear, specific, and testable statement that predicts the expected result in a scientific study. It is a prediction, reasonable guess, and logical supposition about the relationship between the variables. A research hypothesis is an integral and central part of research whether it is exploratory or explanatory, qualitative or quantitative. It creates the base of scientific experiments. So, you must be very careful while building any hypothesis.
A hypothesis can be correct or wrong. It is tested through experiments or research to determine whether it is correct or incorrect.
Functions of research hypothesis
There are major functions of research hypothesis that are as follow:
- It helps in making observations and experiments possible.
- It is the basic point for the research.
- It verifies the observations.
- It leads the inquiries in the right regulation.
- It provides the extension of knowledge.
- It helps to explore different aspects of the research.
- It introduces different research techniques.
- It ensures the precision and accuracy of the results of the research.
- It enables the researcher to be focused. Because without a hypothesis, he may focus on unnecessary aspects and wastes his resources like time, money, and effort.
Sources of hypothesis
Following are the sources of the hypothesis:
- Scientific theories
- Personal experience
- Observation
- Imagination and thinking
- Previous study
- General patterns
Characteristics of an effective research hypothesis
Following are the characteristics of an effective research hypothesis:
- It must be logical.
- It must be simple and clear.
- It needs to be precise.
- It must identify the research objectives.
- It must be empirically testable with experimentation and research.
- It must be manageable.
- It must be relevant and specific to the theme of the research.
- It must be predictable.
- It must be falsifiable.
- It must be neither specific nor general.
- It must be considered valuable even if it proves false.
Types of research hypothesis
Following are the types of research hypotheses.
- Simple hypothesis
It shows a relationship between a single dependent variable and an independent variable. For instance, if you take in more carbs and fats, you will gain obesity. Here taking more carbs and fats are an independent variable and gaining weight is the dependent variable.
- Complex hypothesis
It predicts the relationship between two or more independent variables and dependent variables. For example, we can say that taking in more carbs and fats can cause obesity along with other problems like high blood pressure, heart disease, and so on.
- Directional hypothesis
Typically, directional hypotheses are derived from theory. This type of hypothesis shows the researcher’s intellectual commitment towards a specific outcome. The researcher predicts the existence and nature of a relationship between variables.
- Non-directional hypothesis
The non-directional hypothesis is used when there is no theory and the findings of studies are contradictory. It shows the relationship between two variables but does not set down the expected direction or nature of the relationship.
- Null hypothesis
Null hypotheses are made when there is no empirical and adequate theoretical information to show a hypothesis. The null hypothesis negates the relationship between variables. It is denoted by Ho. This hypothesis is made when the researcher wants to reject or disapprove the null hypothesis. It is contrary to what an experimenter or investigator expects. The purpose is to confirm the existence of a relationship between the variables.
The null hypothesis can be:
- Associative or causal
- Simple or complex
1. Alternative hypothesis
When a hypothesis is rejected, then another hypothesis is made to be tested and show the desired results. This is called an alternative hypothesis. It is opposite to the null hypothesis and is made to disprove that hypothesis. This hypothesis is denoted by H1.
2. Statistical hypothesis
As the name mentions, this hypothesis has the quality to be verified statistically. It is tested by using quantitative techniques. The variables in this hypothesis are quantifiable and can also transform into quantifiable indicators to verify it statistically.
- Empirical hypothesis
This hypothesis is used when a theory is tested with observation and experiment. It is just a notion or idea. This hypothesis goes through trial and error by changing independent variables. The series of trial and error helps to find the best result. The outcomes of these experiments can be proven over time.
- Associative and causal hypothesis
The associative hypothesis shows interdependency between variables. Any change in one variable causes the change in another variable. Whereas, the causal hypothesis shows a cause and effect between variables.
How to formulate a research hypothesis
There are some important points you must consider while formulating a hypothesis:
- Ask a question
The first and foremost thing for creating a research hypothesis is to generate a research question. The question should be specific, focused, and researchable within the limitations of your project.
- Do preliminary research
Now try to find the answer to your question. The initial answer must be based on previous knowledge about the topic. Concern theories and previous studies and try to form assumptions about what you will find in your research.
Create a conceptual framework about different variables you are going to study and the relationships between them.
- Formulate the hypothesis
Now you have an idea of what you are expecting to find. Make a clear and concise answer to the question.
- Refine your hypothesis
Now check whether your hypothesis is testable. There must be clear definitions of your hypothesis while phrasing. It should contain:
- The relevant variables
The particular group being studied.
The predicted result of the analysis or experiment
- Phrase your hypothesis in three ways
To recognize the variables, write a prediction in (if-then) form. Like, if a particular action is taken, a certain result is expected. The first part of the phrase shows the independent variable while the second part shows the dependent variable.
- Write a null hypothesis
If the research requires statistical hypothesis testing, you must have to make a null hypothesis and an alternative hypothesis.
Now test your hypothesis through observations, techniques, and experiments by keeping necessary things and resources in consideration.
Related Posts:
- Correlational Research Design [Examples, Types, Advantages, Disadvantages, Characteristics]
- Which of the following is the first step in hypothesis testing?
- Which of the following is considered one of the earliest literary sources in human history?
- Sources of Islamic law (Islamic Study) MCQs
- Quantitative research Examples, Methods, Characteristics, Advantages, Disadvantages
- Hierarchical Model with examples and characteristics

What is Hypothesis? Definition, Meaning, Characteristics, Sources
- Post last modified: 10 January 2022
- Reading time: 18 mins read
- Post category: Research Methodology

What is Hypothesis?
Hypothesis is a prediction of the outcome of a study. Hypotheses are drawn from theories and research questions or from direct observations. In fact, a research problem can be formulated as a hypothesis. To test the hypothesis we need to formulate it in terms that can actually be analysed with statistical tools.
As an example, if we want to explore whether using a specific teaching method at school will result in better school marks (research question), the hypothesis could be that the mean school marks of students being taught with that specific teaching method will be higher than of those being taught using other methods.
In this example, we stated a hypothesis about the expected differences between groups. Other hypotheses may refer to correlations between variables.
Table of Content
- 1 What is Hypothesis?
- 2 Hypothesis Definition
- 3 Meaning of Hypothesis
- 4.1 Conceptual Clarity
- 4.2 Need of empirical referents
- 4.3 Hypothesis should be specific
- 4.4 Hypothesis should be within the ambit of the available research techniques
- 4.5 Hypothesis should be consistent with the theory
- 4.6 Hypothesis should be concerned with observable facts and empirical events
- 4.7 Hypothesis should be simple
- 5.1 Observation
- 5.2 Analogies
- 5.4 State of Knowledge
- 5.5 Culture
- 5.6 Continuity of Research
- 6.1 Null Hypothesis
- 6.2 Alternative Hypothesis
Thus, to formulate a hypothesis, we need to refer to the descriptive statistics (such as the mean final marks), and specify a set of conditions about these statistics (such as a difference between the means, or in a different example, a positive or negative correlation). The hypothesis we formulate applies to the population of interest.
The null hypothesis makes a statement that no difference exists (see Pyrczak, 1995, pp. 75-84).
Hypothesis Definition
A hypothesis is ‘a guess or supposition as to the existence of some fact or law which will serve to explain a connection of facts already known to exist.’ – J. E. Creighton & H. R. Smart
Hypothesis is ‘a proposition not known to be definitely true or false, examined for the sake of determining the consequences which would follow from its truth.’ – Max Black
Hypothesis is ‘a proposition which can be put to a test to determine validity and is useful for further research.’ – W. J. Goode and P. K. Hatt
A hypothesis is a proposition, condition or principle which is assumed, perhaps without belief, in order to draw out its logical consequences and by this method to test its accord with facts which are known or may be determined. – Webster’s New International Dictionary of the English Language (1956)
Meaning of Hypothesis
From the above mentioned definitions of hypothesis, its meaning can be explained in the following ways.
- At the primary level, a hypothesis is the possible and probable explanation of the sequence of happenings or data.
- Sometimes, hypothesis may emerge from an imagination, common sense or a sudden event.
- Hypothesis can be a probable answer to the research problem undertaken for study. 4. Hypothesis may not always be true. It can get disproven. In other words, hypothesis need not always be a true proposition.
- Hypothesis, in a sense, is an attempt to present the interrelations that exist in the available data or information.
- Hypothesis is not an individual opinion or community thought. Instead, it is a philosophical means which is to be used for research purpose. Hypothesis is not to be considered as the ultimate objective; rather it is to be taken as the means of explaining scientifically the prevailing situation.
The concept of hypothesis can further be explained with the help of some examples. Lord Keynes, in his theory of national income determination, made a hypothesis about the consumption function. He stated that the consumption expenditure of an individual or an economy as a whole is dependent on the level of income and changes in a certain proportion.
Later, this proposition was proved in the statistical research carried out by Prof. Simon Kuznets. Matthus, while studying the population, formulated a hypothesis that population increases faster than the supply of food grains. Population studies of several countries revealed that this hypothesis is true.
Validation of the Malthus’ hypothesis turned it into a theory and when it was tested in many other countries it became the famous Malthus’ Law of Population. It thus emerges that when a hypothesis is tested and proven, it becomes a theory. The theory, when found true in different times and at different places, becomes the law. Having understood the concept of hypothesis, few hypotheses can be formulated in the areas of commerce and economics.
- Population growth moderates with the rise in per capita income.
- Sales growth is positively linked with the availability of credit.
- Commerce education increases the employability of the graduate students.
- High rates of direct taxes prompt people to evade taxes.
- Good working conditions improve the productivity of employees.
- Advertising is the most effecting way of promoting sales than any other scheme.
- Higher Debt-Equity Ratio increases the probability of insolvency.
- Economic reforms in India have made the public sector banks more efficient and competent.
- Foreign direct investment in India has moved in those sectors which offer higher rate of profit.
- There is no significant association between credit rating and investment of fund.
Characteristics of Hypothesis
Not all the hypotheses are good and useful from the point of view of research. It is only a few hypotheses satisfying certain criteria that are good, useful and directive in the research work undertaken. The characteristics of such a useful hypothesis can be listed as below:
Conceptual Clarity
Need of empirical referents, hypothesis should be specific, hypothesis should be within the ambit of the available research techniques, hypothesis should be consistent with the theory, hypothesis should be concerned with observable facts and empirical events, hypothesis should be simple.
The concepts used while framing hypothesis should be crystal clear and unambiguous. Such concepts must be clearly defined so that they become lucid and acceptable to everyone. How are the newly developed concepts interrelated and how are they linked with the old one is to be very clear so that the hypothesis framed on their basis also carries the same clarity.
A hypothesis embodying unclear and ambiguous concepts can to a great extent undermine the successful completion of the research work.
A hypothesis can be useful in the research work undertaken only when it has links with some empirical referents. Hypothesis based on moral values and ideals are useless as they cannot be tested. Similarly, hypothesis containing opinions as good and bad or expectation with respect to something are not testable and therefore useless.
For example, ‘current account deficit can be lowered if people change their attitude towards gold’ is a hypothesis encompassing expectation. In case of such a hypothesis, the attitude towards gold is something which cannot clearly be described and therefore a hypothesis which embodies such an unclean thing cannot be tested and proved or disproved. In short, the hypothesis should be linked with some testable referents.
For the successful conduction of research, it is necessary that the hypothesis is specific and presented in a precise manner. Hypothesis which is general, too ambitious and grandiose in scope is not to be made as such hypothesis cannot be easily put to test. A hypothesis is to be based on such concepts which are precise and empirical in nature. A hypothesis should give a clear idea about the indicators which are to be used.
For example, a hypothesis that economic power is increasingly getting concentrated in a few hands in India should enable us to define the concept of economic power. It should be explicated in terms of measurable indicator like income, wealth, etc. Such specificity in the formulation of a hypothesis ensures that the research is practicable and significant.
While framing the hypothesis, the researcher should be aware of the available research techniques and should see that the hypothesis framed is testable on the basis of them. In other words, a hypothesis should be researchable and for this it is important that a due thought has been given to the methods and techniques which can be used to measure the concepts and variables embodied in the hypothesis.
It does not however mean that hypotheses which are not testable with the available techniques of research are not to be made. If the problem is too significant and therefore the hypothesis framed becomes too ambitious and complex, it’s testing becomes possible with the development of new research techniques or the hypothesis itself leads to the development of new research techniques.
A hypothesis must be related to the existing theory or should have a theoretical orientation. The growth of knowledge takes place in the sequence of facts, hypothesis, theory and law or principles. It means the hypothesis should have a correspondence with the existing facts and theory.
If the hypothesis is related to some theory, the research work will enable us to support, modify or refute the existing theory. Theoretical orientation of the hypothesis ensures that it becomes scientifically useful. According to Prof. Goode and Prof. Hatt, research work can contribute to the existing knowledge only when the hypothesis is related with some theory.
This enables us to explain the observed facts and situations and also verify the framed hypothesis. In the words of Prof. Cohen and Prof. Nagel, “hypothesis must be formulated in such a manner that deduction can be made from it and that consequently a decision can be reached as to whether it does or does not explain the facts considered.”
If the research work based on a hypothesis is to be successful, it is necessary that the later is as simple and easy as possible. An ambition of finding out something new may lead the researcher to frame an unrealistic and unclear hypothesis. Such a temptation is to be avoided. Framing a simple, easy and testable hypothesis requires that the researcher is well acquainted with the related concepts.
Sources of Hypothesis
Hypotheses can be derived from various sources. Some of the sources is given below:
Observation
State of knowledge, continuity of research.
Hypotheses can be derived from observation from the observation of price behavior in a market. For example the relationship between the price and demand for an article is hypothesized.
Analogies are another source of useful hypotheses. Julian Huxley has pointed out that casual observations in nature or in the framework of another science may be a fertile source of hypotheses. For example, the hypotheses that similar human types or activities may be found in similar geophysical regions come from plant ecology.
This is one of the main sources of hypotheses. It gives direction to research by stating what is known logical deduction from theory lead to new hypotheses. For example, profit / wealth maximization is considered as the goal of private enterprises. From this assumption various hypotheses are derived’.
An important source of hypotheses is the state of knowledge in any particular science where formal theories exist hypotheses can be deduced. If the hypotheses are rejected theories are scarce hypotheses are generated from conception frameworks.
Another source of hypotheses is the culture on which the researcher was nurtured. Western culture has induced the emergence of sociology as an academic discipline over the past decade, a large part of the hypotheses on American society examined by researchers were connected with violence. This interest is related to the considerable increase in the level of violence in America.
The continuity of research in a field itself constitutes an important source of hypotheses. The rejection of some hypotheses leads to the formulation of new ones capable of explaining dependent variables in subsequent research on the same subject.
Null and Alternative Hypothesis
Null hypothesis.
The hypothesis that are proposed with the intent of receiving a rejection for them are called Null Hypothesis . This requires that we hypothesize the opposite of what is desired to be proved. For example, if we want to show that sales and advertisement expenditure are related, we formulate the null hypothesis that they are not related.
Similarly, if we want to conclude that the new sales training programme is effective, we formulate the null hypothesis that the new training programme is not effective, and if we want to prove that the average wages of skilled workers in town 1 is greater than that of town 2, we formulate the null hypotheses that there is no difference in the average wages of the skilled workers in both the towns.
Since we hypothesize that sales and advertisement are not related, new training programme is not effective and the average wages of skilled workers in both the towns are equal, we call such hypotheses null hypotheses and denote them as H 0 .
Alternative Hypothesis
Rejection of null hypotheses leads to the acceptance of alternative hypothesis . The rejection of null hypothesis indicates that the relationship between variables (e.g., sales and advertisement expenditure) or the difference between means (e.g., wages of skilled workers in town 1 and town 2) or the difference between proportions have statistical significance and the acceptance of the null hypotheses indicates that these differences are due to chance.
As already mentioned, the alternative hypotheses specify that values/relation which the researcher believes hold true. The alternative hypotheses can cover a whole range of values rather than a single point. The alternative hypotheses are denoted by H 1 .
Business Ethics
( Click on Topic to Read )
- What is Ethics?
- What is Business Ethics?
- Values, Norms, Beliefs and Standards in Business Ethics
- Indian Ethos in Management
- Ethical Issues in Marketing
- Ethical Issues in HRM
- Ethical Issues in IT
- Ethical Issues in Production and Operations Management
- Ethical Issues in Finance and Accounting
- What is Corporate Governance?
- What is Ownership Concentration?
- What is Ownership Composition?
- Types of Companies in India
- Internal Corporate Governance
- External Corporate Governance
- Corporate Governance in India
- What is Enterprise Risk Management (ERM)?
- What is Assessment of Risk?
- What is Risk Register?
- Risk Management Committee
Corporate social responsibility (CSR)
- Theories of CSR
- Arguments Against CSR
- Business Case for CSR
- Importance of CSR in India
- Drivers of Corporate Social Responsibility
- Developing a CSR Strategy
- Implement CSR Commitments
- CSR Marketplace
- CSR at Workplace
- Environmental CSR
- CSR with Communities and in Supply Chain
- Community Interventions
- CSR Monitoring
- CSR Reporting
- Voluntary Codes in CSR
- What is Corporate Ethics?
Lean Six Sigma
- What is Six Sigma?
- What is Lean Six Sigma?
- Value and Waste in Lean Six Sigma
- Six Sigma Team
- MAIC Six Sigma
- Six Sigma in Supply Chains
- What is Binomial, Poisson, Normal Distribution?
- What is Sigma Level?
- What is DMAIC in Six Sigma?
- What is DMADV in Six Sigma?
- Six Sigma Project Charter
- Project Decomposition in Six Sigma
- Critical to Quality (CTQ) Six Sigma
- Process Mapping Six Sigma
- Flowchart and SIPOC
- Gage Repeatability and Reproducibility
- Statistical Diagram
- Lean Techniques for Optimisation Flow
- Failure Modes and Effects Analysis (FMEA)
- What is Process Audits?
- Six Sigma Implementation at Ford
- IBM Uses Six Sigma to Drive Behaviour Change
- Research Methodology
- What is Research?
- Sampling Method
- Research Methods
- Data Collection in Research
Methods of Collecting Data
- Application of Business Research
- Levels of Measurement
- What is Sampling?
- Hypothesis Testing
- Research Report
- What is Management?
- Planning in Management
- Decision Making in Management
- What is Controlling?
- What is Coordination?
- What is Staffing?
- Organization Structure
- What is Departmentation?
- Span of Control
- What is Authority?
- Centralization vs Decentralization
- Organizing in Management
- Schools of Management Thought
- Classical Management Approach
- Is Management an Art or Science?
- Who is a Manager?
Operations Research
- What is Operations Research?
- Operation Research Models
- Linear Programming
- Linear Programming Graphic Solution
- Linear Programming Simplex Method
- Linear Programming Artificial Variable Technique
- Duality in Linear Programming
- Transportation Problem Initial Basic Feasible Solution
- Transportation Problem Finding Optimal Solution
- Project Network Analysis with Critical Path Method
- Project Network Analysis Methods
- Project Evaluation and Review Technique (PERT)
- Simulation in Operation Research
- Replacement Models in Operation Research
Operation Management
- What is Strategy?
- What is Operations Strategy?
- Operations Competitive Dimensions
- Operations Strategy Formulation Process
- What is Strategic Fit?
- Strategic Design Process
- Focused Operations Strategy
- Corporate Level Strategy
- Expansion Strategies
- Stability Strategies
- Retrenchment Strategies
- Competitive Advantage
- Strategic Choice and Strategic Alternatives
- What is Production Process?
- What is Process Technology?
- What is Process Improvement?
- Strategic Capacity Management
- Production and Logistics Strategy
- Taxonomy of Supply Chain Strategies
- Factors Considered in Supply Chain Planning
- Operational and Strategic Issues in Global Logistics
- Logistics Outsourcing Strategy
- What is Supply Chain Mapping?
- Supply Chain Process Restructuring
- Points of Differentiation
- Re-engineering Improvement in SCM
- What is Supply Chain Drivers?
- Supply Chain Operations Reference (SCOR) Model
- Customer Service and Cost Trade Off
- Internal and External Performance Measures
- Linking Supply Chain and Business Performance
- Netflix’s Niche Focused Strategy
- Disney and Pixar Merger
- Process Planning at Mcdonald’s
Service Operations Management
- What is Service?
- What is Service Operations Management?
- What is Service Design?
- Service Design Process
- Service Delivery
- What is Service Quality?
- Gap Model of Service Quality
- Juran Trilogy
- Service Performance Measurement
- Service Decoupling
- IT Service Operation
- Service Operations Management in Different Sector
Procurement Management
- What is Procurement Management?
- Procurement Negotiation
- Types of Requisition
- RFX in Procurement
- What is Purchasing Cycle?
- Vendor Managed Inventory
- Internal Conflict During Purchasing Operation
- Spend Analysis in Procurement
- Sourcing in Procurement
- Supplier Evaluation and Selection in Procurement
- Blacklisting of Suppliers in Procurement
- Total Cost of Ownership in Procurement
- Incoterms in Procurement
- Documents Used in International Procurement
- Transportation and Logistics Strategy
- What is Capital Equipment?
- Procurement Process of Capital Equipment
- Acquisition of Technology in Procurement
- What is E-Procurement?
- E-marketplace and Online Catalogues
- Fixed Price and Cost Reimbursement Contracts
- Contract Cancellation in Procurement
- Ethics in Procurement
- Legal Aspects of Procurement
- Global Sourcing in Procurement
- Intermediaries and Countertrade in Procurement
Strategic Management
- What is Strategic Management?
- What is Value Chain Analysis?
- Mission Statement
- Business Level Strategy
- What is SWOT Analysis?
- What is Competitive Advantage?
- What is Vision?
- What is Ansoff Matrix?
- Prahalad and Gary Hammel
- Strategic Management In Global Environment
- Competitor Analysis Framework
- Competitive Rivalry Analysis
- Competitive Dynamics
- What is Competitive Rivalry?
- Five Competitive Forces That Shape Strategy
- What is PESTLE Analysis?
- Fragmentation and Consolidation Of Industries
- What is Technology Life Cycle?
- What is Diversification Strategy?
- What is Corporate Restructuring Strategy?
- Resources and Capabilities of Organization
- Role of Leaders In Functional-Level Strategic Management
- Functional Structure In Functional Level Strategy Formulation
- Information And Control System
- What is Strategy Gap Analysis?
- Issues In Strategy Implementation
- Matrix Organizational Structure
- What is Strategic Management Process?
Supply Chain
- What is Supply Chain Management?
- Supply Chain Planning and Measuring Strategy Performance
- What is Warehousing?
- What is Packaging?
- What is Inventory Management?
- What is Material Handling?
- What is Order Picking?
- Receiving and Dispatch, Processes
- What is Warehouse Design?
- What is Warehousing Costs?
You Might Also Like
What is research types, purpose, characteristics, process, what is measurement scales, types, criteria and developing measurement tools, steps in questionnaire design, what is research design features, components, types of hypotheses, measures of relationship, data analysis in research, research process | types, what is descriptive research types, features, primary data and secondary data, leave a reply cancel reply.
You must be logged in to post a comment.
World's Best Online Courses at One Place
We’ve spent the time in finding, so you can spend your time in learning
Digital Marketing
Personal growth.
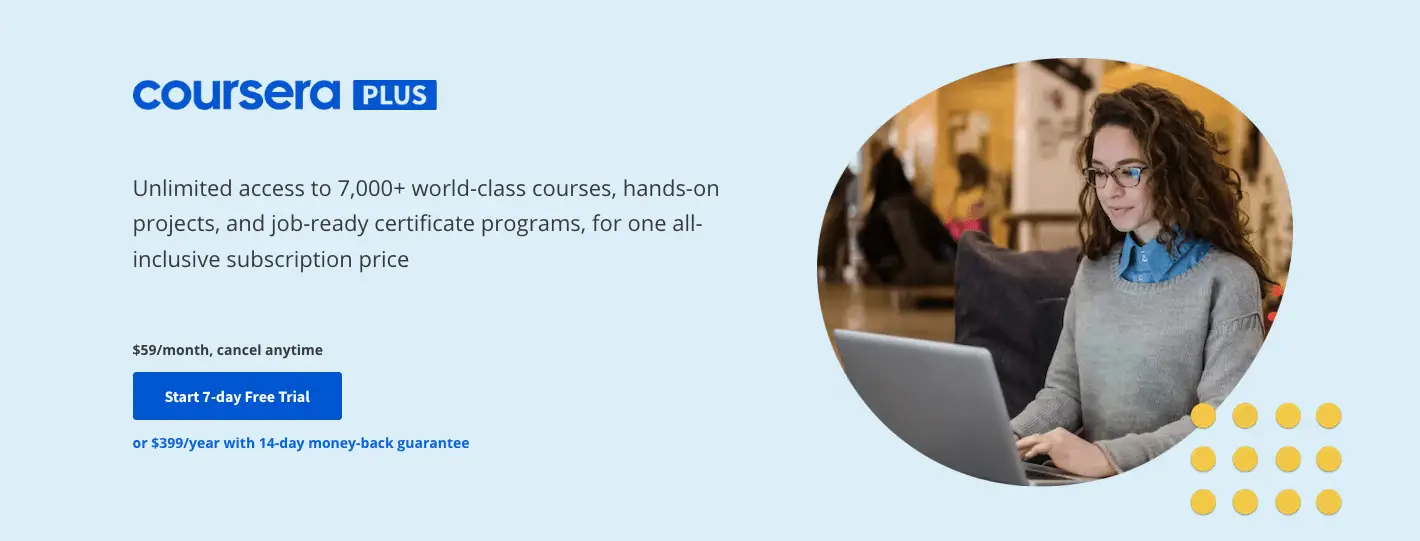
Development
MBA Knowledge Base
Business • Management • Technology
Home » Research Methodology » Sources of Hypothesis in Research
Sources of Hypothesis in Research
A hypothesis is an assumption about:
- The relationship between/among variables or
- The level of influence of independent variables on the dependent variable or
- The value of population parameter.
Thus relationship between income and brand preference, between education and habit of savings, between cost of capital and capital structure, between motivation and productivity, etc., are hypotheses of the first type. The influence of sales on profit, of profit on value of business, of reward on performance and such other things are the second type. The third type involves putting values on population parameters such as the mean monthly salary of students who graduated from a particular university in the first year of their employment is 1000$ in 2012 or on an average management graduates wait for 6 months after graduation to get job or 40% management graduates get administrative jobs and so on.
Sources of Hypothesis
There are diverse sources of hypothesis in research . First, an explorative research work might lead to the establishment of hypothesis . Second, the environment is a source of hypothesis , because environment portrays broad relationship across factors which form the basis for drawing an inference. Third, analogies are a source of hypothesis . The term analogies refer to parallelism. Though human system and animal system are different, there is some parallelism. That is why medicines are tried first on rats or monkeys then used for human consumption. So, hypothesis on animal behavior can be done based on proven behavior of human and vice versa. Similarly, between thermodynamics and group dynamics, biological system and social system, nervous system and central processing unit of a computer , parallelism can be thought of and spring hypotheses therefrom. Fourth, previous research studies are a great source of hypotheses . That is why review of literature is made. Fifth, assumptions of certain theories become a source of hypothesis in research . Similarly, exceptions to certain theory are ground for new hypotheses. Sixth, personal experiences and experiences of others are another source of hypotheses . Everyone encounters numerous experiences in day to day life in relation to one’s avocation. From these glimpses of hypothetical relations between events, variables, etc emanate. These are, therefore, bases for establishment of possible hypotheses. Seventh, social, physical and other theories and laws provide for hypotheses. Newton’s laws of motion might be a source of hypotheses , in social science, say behavior and reward and the like. Finally, for the research mind, the whole universe is a source of hypotheses . Yes. the searching mind fathoms out new hypotheses from seemingly events of insignificance.
Related posts:
- Documentary Sources of Information in Research
- Secondary Data Sources for Research
- Standard Error in Hypothesis Testing
- Hypothesis and Procedure for its Testing
- The Efficient Markets Hypothesis (EMH)
- The Research Problem
- The Purpose of Research
- Research Design
- Significance of Research
- Referencing a Research Report
Leave a Reply Cancel reply
Your email address will not be published. Required fields are marked *

Concept, Characteristics, Types and Sources of Hypothesis in Research Methodology
Back to: Introduction to Educational Research Methodology
A hypothesis is used for explaining a phenomenon. Hypothesis is essential to discover cause-and-effect relationships. It provides direction for research and prevents from collecting unnecessary and useless data.
Selection of a research problem highly depends on the researcher’s knowledge, skills and motivation on the subject matter. To develop a proper hypothesis, the researcher needs to have a high interest in the topic. The level of expertise on the topic is very crucial as well. The researcher needs to make sure that there is enough data available on the topic before getting started. Controversial and narrow topics should be avoided.
Characteristics of Hypothesis
1. It states what findings are going to be done through the research.
2. It guides data collection and interpretation.
3. It helps in designing the research and what results can be expected.
4. It is helpful in acquiring useful and relevant data.
5. It helps in doing valid and reasonable research.
6. It helps in data analysis and interpretation.
7. It is helpful for discovering relationships between variables and theoretical guidelines.
Types of Hypothesis
1. simple hypothesis.
It portrays the relationship between two variables, that is independent variable or cause and dependent variable or effect.
2. Complex Hypothesis
This hypothesis contains several dependent and independent variables.
3. Null Hypothesis
This hypothesis is used to prove that there is no relationship between dependent and independent variables. It rejects the relationship between variables.
4. Alternative Hypothesis
As the name suggests, numerous hypothesis are put forward and the most effective one is chosen from them. If one hypothesis is rejected, another is taken to be tested.
Sources of Hypothesis
1. personal experiences .
One can get a lot of information from their own experiences in life. Personal experiences can be an important source of hypothesis.
2. Previous Study
Looking at the previous works on the topic of hypothesis will be beneficial in making it of good quality.
3. Thinking and Imagination
The creative thinking of researchers can help in preparing a good hypothesis. A researchers’ thinking process can be another source of hypothesis.
Conclusion
A hypothesis needs earlier evidence and should be testable. The hypothesis should be stated clearly and briefly for readers to understand it easily. To develop a hypothesis, the researcher needs to have an extensive and broad knowledge on the topic and look deeply into the past articles and journals on the same topic. The researcher should be able to limit the problem while formulating the hypothesis.
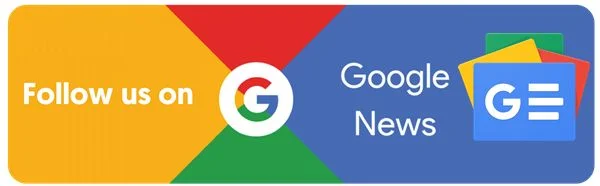
- Scientific Methods
What is Hypothesis?
We have heard of many hypotheses which have led to great inventions in science. Assumptions that are made on the basis of some evidence are known as hypotheses. In this article, let us learn in detail about the hypothesis and the type of hypothesis with examples.
A hypothesis is an assumption that is made based on some evidence. This is the initial point of any investigation that translates the research questions into predictions. It includes components like variables, population and the relation between the variables. A research hypothesis is a hypothesis that is used to test the relationship between two or more variables.
Characteristics of Hypothesis
Following are the characteristics of the hypothesis:
- The hypothesis should be clear and precise to consider it to be reliable.
- If the hypothesis is a relational hypothesis, then it should be stating the relationship between variables.
- The hypothesis must be specific and should have scope for conducting more tests.
- The way of explanation of the hypothesis must be very simple and it should also be understood that the simplicity of the hypothesis is not related to its significance.
Sources of Hypothesis
Following are the sources of hypothesis:
- The resemblance between the phenomenon.
- Observations from past studies, present-day experiences and from the competitors.
- Scientific theories.
- General patterns that influence the thinking process of people.
Types of Hypothesis
There are six forms of hypothesis and they are:
- Simple hypothesis
- Complex hypothesis
- Directional hypothesis
- Non-directional hypothesis
- Null hypothesis
- Associative and casual hypothesis
Simple Hypothesis
It shows a relationship between one dependent variable and a single independent variable. For example – If you eat more vegetables, you will lose weight faster. Here, eating more vegetables is an independent variable, while losing weight is the dependent variable.
Complex Hypothesis
It shows the relationship between two or more dependent variables and two or more independent variables. Eating more vegetables and fruits leads to weight loss, glowing skin, and reduces the risk of many diseases such as heart disease.
Directional Hypothesis
It shows how a researcher is intellectual and committed to a particular outcome. The relationship between the variables can also predict its nature. For example- children aged four years eating proper food over a five-year period are having higher IQ levels than children not having a proper meal. This shows the effect and direction of the effect.
Non-directional Hypothesis
It is used when there is no theory involved. It is a statement that a relationship exists between two variables, without predicting the exact nature (direction) of the relationship.
Null Hypothesis
It provides a statement which is contrary to the hypothesis. It’s a negative statement, and there is no relationship between independent and dependent variables. The symbol is denoted by “H O ”.
Associative and Causal Hypothesis
Associative hypothesis occurs when there is a change in one variable resulting in a change in the other variable. Whereas, the causal hypothesis proposes a cause and effect interaction between two or more variables.
Examples of Hypothesis
Following are the examples of hypotheses based on their types:
- Consumption of sugary drinks every day leads to obesity is an example of a simple hypothesis.
- All lilies have the same number of petals is an example of a null hypothesis.
- If a person gets 7 hours of sleep, then he will feel less fatigue than if he sleeps less. It is an example of a directional hypothesis.
Functions of Hypothesis
Following are the functions performed by the hypothesis:
- Hypothesis helps in making an observation and experiments possible.
- It becomes the start point for the investigation.
- Hypothesis helps in verifying the observations.
- It helps in directing the inquiries in the right direction.
How will Hypothesis help in the Scientific Method?
Researchers use hypotheses to put down their thoughts directing how the experiment would take place. Following are the steps that are involved in the scientific method:
- Formation of question
- Doing background research
- Creation of hypothesis
- Designing an experiment
- Collection of data
- Result analysis
- Summarizing the experiment
- Communicating the results
Frequently Asked Questions – FAQs
What is hypothesis.
A hypothesis is an assumption made based on some evidence.
Give an example of simple hypothesis?
What are the types of hypothesis.
Types of hypothesis are:
- Associative and Casual hypothesis
State true or false: Hypothesis is the initial point of any investigation that translates the research questions into a prediction.
Define complex hypothesis..
A complex hypothesis shows the relationship between two or more dependent variables and two or more independent variables.
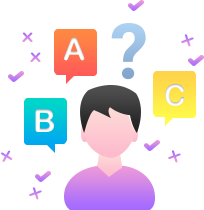
Put your understanding of this concept to test by answering a few MCQs. Click ‘Start Quiz’ to begin!
Select the correct answer and click on the “Finish” button Check your score and answers at the end of the quiz
Visit BYJU’S for all Physics related queries and study materials
Your result is as below
Request OTP on Voice Call
Leave a Comment Cancel reply
Your Mobile number and Email id will not be published. Required fields are marked *
Post My Comment

- Share Share
Register with BYJU'S & Download Free PDFs
Register with byju's & watch live videos.


Model Answers
Q: Discuss the importance and sources of hypothesis in social research.
Question asked in UPSC Sociology 2020 Paper 1. Download our app for last 20 year question with model answers.
Model Answer:
Importance of Hypothesis in Social Research
Hypothesis in social research refers to a tentative statement or assumption about the relationship between two or more variables. It is a testable prediction that serves as a starting point for conducting a study. Hypotheses are important in social research for several reasons:
1. Direction and focus: Hypotheses provide a clear direction and focus for the research. They help researchers identify the variables that need to be studied and the relationships that need to be explored. For example, a hypothesis might state that “ higher levels of education lead to higher income levels. ” This statement provides a clear direction for the researcher to investigate the relationship between education and income.
2. Basis for research design: Hypotheses serve as the foundation for designing a research study. They help researchers choose the appropriate methods, techniques, and tools to collect and analyze data. For instance, if a researcher wants to test the hypothesis that “ participation in sports reduces the likelihood of engaging in criminal behavior, ” they might design a study that compares crime rates among individuals who participate in sports and those who do not.
3. Testability: Hypotheses are testable statements that can be either supported or refuted by empirical evidence. This testability is crucial for the scientific process, as it allows researchers to build on existing knowledge and contribute to the understanding of social phenomena. For example, if a hypothesis states that “ social media use increases feelings of loneliness, ” researchers can collect data on social media usage and loneliness levels to test this assumption.
4. Explanation and prediction: Hypotheses help researchers explain and predict social phenomena. By identifying relationships between variables, hypotheses can provide insights into the underlying mechanisms and processes that drive social behavior. For instance, a hypothesis that “ unemployment leads to increased crime rates, ” might suggest that addressing unemployment could help reduce crime.
Sources of hypothesis in social research:
1. Theory: Hypotheses can be derived from existing theories in the field. Theories provide a framework for understanding social phenomena and can suggest relationships between variables that can be tested through research. For example, social learning theory might suggest the hypothesis that “ children who witness violence in their homes are more likely to exhibit aggressive behavior. “
2. Previous research: Hypotheses can be based on the findings of previous studies. Researchers can build on existing knowledge by testing new relationships or exploring the same relationships in different contexts. For example, if a previous study found a relationship between poverty and crime in urban areas, a researcher might hypothesize that the same relationship exists in rural areas.
3. Observations and personal experiences: Researchers can develop hypotheses based on their own observations and experiences. These insights can provide a starting point for investigating social phenomena. For example, a researcher who notices a high rate of teenage pregnancy in their community might hypothesize that a lack of access to sexual education is a contributing factor.
4. Expert opinions and literature reviews: Consulting experts in the field and reviewing existing literature can help researchers identify gaps in knowledge and generate hypotheses. For instance, a review of research on the effects of social media on mental health might reveal conflicting findings, leading a researcher to hypothesize that certain factors, such as the type of social media platform or the amount of time spent online, might moderate these effects.
In conclusion, hypotheses play a crucial role in social research by providing direction, focus, and a basis for research design. They are derived from various sources, including theory, previous research, observations, and expert opinions. By testing hypotheses, researchers can contribute to the understanding of social phenomena and inform policies and interventions aimed at addressing social issues.
Download our app for UPSC Sociology Optional - Syllabus, NCERT Books, IGNOU Books, Past Paper with Model Answers, Topper Notes & Answer Sheet.
ORIGINAL RESEARCH article
Integrating non-renewable energy consumption, geopolitical risks, economic development with the ecological intensity of well-being: evidence from quantile regression analysis provisionally accepted.
- 1 COMSATS University, Islamabad Campus, Pakistan
- 2 Medgar Evers College, United States
The final, formatted version of the article will be published soon.
This study delves into the intricate relationship between non-renewable energy sources, economic advancement, and the ecological footprint of well-being in Pakistan, spanning the years from 1980 to 2021. Employing the quantile regression model, we analyzed the co-integrating dynamics among the variables under scrutiny. Non-renewable energy sources were dissected into four distinct components-namely, gas, electricity, and oil consumption-facilitating a granular examination of their impacts. Our empirical investigations reveal that coal, gas, and electricity consumption exhibit a negative correlation with the ecological footprint of well-being. Conversely, coal consumption and overall energy consumption show a positive association with the ecological footprint of well-being.Additionally, the study underscores the detrimental impact of geopolitical risks on the ecological footprint of well-being. Our findings align with the Environmental Kuznets Curve (EKC) hypothesis, positing that environmental degradation initially surges with economic development, subsequently declining as a nation progresses economically. Consequently, our research advocates for Pakistan's imperative to prioritize the adoption of renewable energy sources as it traverses its developmental trajectory. This strategic pivot towards renewables, encompassing hydroelectric, wind, and solar energy, not only seeks to curtail environmental degradation but also endeavors to foster a cleaner and safer ecological milieu.
Keywords: Non-renewable energy consumption, environmental kuznet hypothesis, Geopolitical risks, Economic Development, Pakistan
Received: 26 Feb 2024; Accepted: 10 May 2024.
Copyright: © 2024 Khurshid, Egbe and Akram. This is an open-access article distributed under the terms of the Creative Commons Attribution License (CC BY) . The use, distribution or reproduction in other forums is permitted, provided the original author(s) or licensor are credited and that the original publication in this journal is cited, in accordance with accepted academic practice. No use, distribution or reproduction is permitted which does not comply with these terms.
* Correspondence: Mx. Nabila Khurshid, COMSATS University, Islamabad Campus, Islamabad, Pakistan
People also looked at
The crucial roles of ICT, renewable energy sources, industrialization, and institutional quality in achieving environmental sustainability in BRICS
- Research Article
- Open access
- Published: 08 May 2024
Cite this article
You have full access to this open access article
- Charles Shaaba Saba ORCID: orcid.org/0000-0001-6230-7292 1 ,
- Charles Raoul Tchuinkam Djemo ORCID: orcid.org/0000-0001-7999-448X 1 &
- Nicholas Ngepah ORCID: orcid.org/0000-0002-1947-0008 1
The BRICS countries—Brazil, Russia, India, China, and South Africa—are committed to achieving United Nations Sustainable Development Goal 13, which focuses on mitigating climate change. To attain this goal, it is crucial to emphasize the significance of ICT, renewable energy sources, industrialization, and institutional quality. This study contributes to the literature by examining the potential role of these factors in environmental sustainability in the BRICS economies from 2000 to 2021, utilizing cross-sectional augmented autoregressive distributed lag (CS-ARDL) estimation and other novel econometric techniques. Accordingly, the study suggests that BRICS governments and policymakers prioritize the use of ICT in the industrial and institutional sectors to achieve faster environmental sustainability in the short-run, as per the CS-ARDL results. However, the study advises caution in the long-term as the interaction between ICT and renewable energy sources, industrialization, and institutional quality may not favour environmental quality. Although the renewable energy sources interaction with ICT may not yield immediate progress, strong measures need to be taken to ensure that short-term gains are not nullified. In conclusion, the study highlights the potential of ICT, renewable energy sources, industrialization, and institutional quality in achieving environmental sustainability in the BRICS countries, while recommending cautious measures in the long run to safeguard the progress made.
Similar content being viewed by others
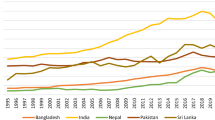
Analysing the role of globalisation, institutional qualities, and renewable energy consumption in environmental degradation mitigation: the SAARC experience

Can undergoing renewable energy transition assist the BRICS countries in achieving environmental sustainability?
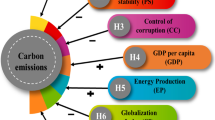
Globalization, institutions, and environmental quality in Middle East and North African countries
Avoid common mistakes on your manuscript.
Introduction
This study’s position of inquiry is driven by four primary considerations, namely: (i) information and communication technology (ICT) diffusion potentials in all the sectors of Brazil, Russia, India, China and South Africa (BRICS) economies; (ii) the challenge of achieving Sustainable Development Goals (SDGs) 13 and 7 (i.e. SDGs 13 (combating climate change) and 7 (affordable and clean energy)) in BRICS countries; (iii) the vital role of industrialization, renewable energy sources and institutional quality in either propulsive or mitigating environmental pollution (for example, carbon dioxide (CO 2 ) emissions); and (iv) there are research gaps in the empirical literature on the environment that require attention. According to the International Energy Agency (IEA) report from 2022, when compared to other regions of the world, the combined emissions per capita of the BRICS economies are among the highest, and they contribute more than three percent of global energy-related CO 2 emissions to date (see the graphical trends in Fig. 1 as an evidence). The continuous use of fossil fuels by BRICS countries poses a serious threat to environment quality. Therefore, it has become important to explore all possible avenues/measures to combat climate change and its impact in BRICS.
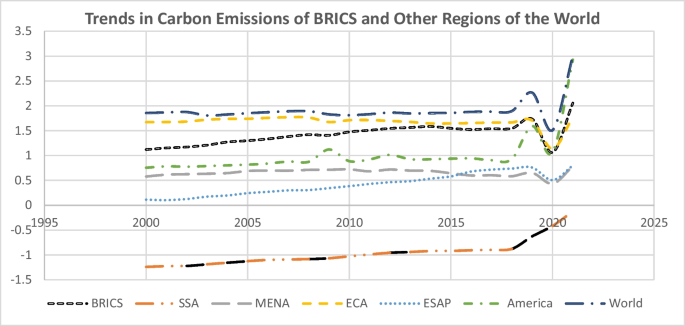
Source : Author’s computation using World Development Indicators (WDI) data
Average trend in CO 2 emissions across regions according to World Bank classification (Sub-Saharan Africa (SSA), Middle East and North Africa (MENA), Europe & Central Asia (ECA), East & South Asia and the Pacific (ESAP) and America) and BRICS from 2000–2021.
According to previous ICT studies, there is a significant amount of opportunity for ICT diffusion in BRICS when contrasted with more developed regions such as in Europe, North America etc., where ICT diffusion has been well established (see Pénard et al. 2012 ; Mirza et al. 2020 ). This is because policymakers can utilize ICT to address obvious policy concerns around sustainable development (for example, environmental pollution and climate change/global warming) given the potential role it plays in every society.
Since environmental sustainability/combating climate change became top policy agenda after the post-2015 development era, therefore, we should be worried about environmental sustainability in BRICS economies for at least four key reasons:
the BRICS countries have achieved high levels of economic growth and are among the fastest growing economies globally, particularly when compared to other developing countries. For example, according to the World Bank, the BRICS countries collectively grew at an average annual rate of 5.8% between 2000 and 2010, compared to 2.6% for high-income countries even with all the fluctuations that took place;
the continuous crisis/challenges of investing, accessing and utilizing renewable energy in this era of achieving sustainable development;
the countries have not reached perfection in energy management; and
the post-2015 sustainable development agenda's main concerns center on the harmful effects of climate change. Huxster et al. ( 2015 ) assert that the unsustainable use of fossil fuels is the root cause of these issues. In addition, Shah et al. ( 2022 ) argued that Africa would experience the worst effects of climate change.
The role of ICT in managing/promoting environmental resources/sustainability sparks a new discussion among policymakers on how to advance technology and reduce high mass CO 2 emissions globally. On the one hand, ICT penetration have the potential to contribute significantly to environmental sustainability (Khan et al. 2022a , b ). This is because ICT can be used to promote sustainable practices, monitor and manage natural resources, reduce energy consumption, and mitigate the impacts of climate change ( Inter alia : Yap 2011 ; Titifanue et al. 2017 ; Qureshi 2019 ; Lahouel et al. 2021 ; Amari et al. 2022 ).
Some of the ways in which ICT can contribute to environmental sustainability across the globe as identified in the literature include: electronic waste (e-waste) management through proper disposal and recycling of electronic devices (Nnorom and Osibanjo 2008 ; Nivedha and Sutha 2020 ; Shahabuddin et al. 2022 ); promoting the use of renewable energy sources such as solar, wind, and hydro power (Saba and Biyase 2022 ; Chang et al. 2022 ); promoting sustainable agricultural practices such as precision farming, which reduces the use of pesticides and fertilizers, and to monitor and manage water resources for irrigation (smart agriculture) (Meena et al. 2012 ; Adamides et al 2020 ); managing natural disasters such as floods, droughts, and wildfires by providing early warning systems, real-time monitoring of affected areas, and coordinating relief efforts (disaster management) (Yap 2011 ; Md Hassan 2015 ; Cacciotti et al. 2021 ); and promoting sustainable transport systems such as public transport, carpooling, and cycling, reducing the use of fossil fuels and air pollution ( Inter alia : Agarwal and Alam 2018 ; Gössling 2018 ; Chatti 2021 ). Even though ICT have the potential to also contribute significantly to environmental sustainability in BRICS countries, however, for it to be effective in promoting environmental sustainability, it must be integrated into policies and strategies aimed at achieving sustainable development in the economic bloc. On the other hand, ICT could play a significant role in carbon emissions due to its energy consumption, which contributes to greenhouse gas emissions. The use of ICTs, including smartphones, laptops, servers, and data centers, requires electricity to operate, which is mostly generated from non-renewable sources of energy. As a result, the ICT sector could contribute to carbon emissions. According to a report by the International Energy Agency (IEA) ( 2020 ), the ICT sector was responsible for 1.4% of global carbon emissions in 2020. This figure is projected to increase to 3.5% by 2025 if no action is taken to reduce the energy consumption of ICTs. The energy consumption of ICT infrastructure, such as data centers, servers, and network equipment, is a major contributor to carbon emissions and also the production, transportation, and disposal of ICT devices also contribute to carbon emissions (Higón et al. 2017 ).
Recent studies on ICT and environmental sustainability across various global regions underscore ICT's potential to drive sustainable development and tackle environmental issues. Reports from international organizations like the World Bank ( 2021 ), United Nations Development Programme (UNDP) and the International Finance Corporation (IFC) ( 2021 ) and African Development Bank (AfDB) ( 2021 ) discuss ICT's role in enhancing climate resilience in different countries. These reports highlight ICT solutions such as weather forecasting and early warning systems that aid communities in adapting to climate change. They also stress the importance of rigorous empirical research to guide policies and regulations supporting the implementation of these solutions.
Several studies have examined the potential of renewable energy sources (RESS) to contribute to environmental sustainability both at panel and country-specific levels. For example, a study in South Africa found that the deployment of wind energy could lead to significant emissions reductions and improve air quality (Mubiru et al. 2019 ). In a study on BRICS economies, Shah et al. ( 2022 ) explore the link between renewable energy, agriculture, GDP, and CO 2 emissions. They conclude that renewable energy can influence the Environmental Kuznets Curve hypothesis. Additionally, Bano et al. ( 2022 ) propose a policy framework for these economies to shift towards renewable energy, supporting green tourism development. The study explores the role of aging, industrial innovations, and ICT on tourism and renewable energy consumption and finds that aging, industrial innovations, and ICT play a significant role in promoting renewable energy and sustainable tourism development in BRICS economies. Industrialization and CO 2 emissions are two important issues in BRICS given the levels of industrial development in the countries. The recent study of Voumik and Sultana ( 2022 ) examines the impact of industrialization, urbanization, electrification, and renewable energy on the environment in BRICS countries. The study finds that these factors have a significant effect on protecting water, land, and forest resources while lowering carbon emissions.
Institutional quality is widely recognized as a critical factor in promoting sustainable development and reducing greenhouse gas emissions. This is because strong institutions can create an enabling environment for the adoption of environmentally friendly policies and practices, and promote accountability and transparency in environmental governance. Several studies, including those of Zhang et al. ( 2019 ), Gyamfi and Zhou ( 2020 ), Olaniran and Adeyemo ( 2020 ), Jiang et al. ( 2022 ) among others, have investigated the relationship between institutional quality and CO 2 emissions. These studies have highlighted the fact that strengthening institutions is an ongoing task that countries must continue to undertake to achieve meaningful progress in society. This underscores the importance of examining institutional quality-ICT induced impact on environmental sustainability in the BRICS economy given that institutions also make use of ICT infrastructures/services for their day-today activities.
The central research question that previous studies have not addressed, and which we aim to explore, is how BRICS nations can achieve environmental sustainability by utilizing their sustainable policy tools in the areas of ICT, renewable energy sources, industrialization, and institutional quality. The specific sub-research questions are as follows: does the interaction between ICT and renewable energy sources impact CO 2 emissions in BRICS countries?; does the interaction between ICT and industrialization affect CO 2 emissions in BRICS countries?; and does the interaction between ICT and institutional quality influence CO 2 emissions in BRICS countries? These questions guide the study's objectives and contributions. The overarching goal is to examine how ICT complements industrialization, renewable energy sources, and institutional quality to influence environmental sustainability in the BRICS economies from 2000 to 2021.
Our research on BRICS contributes to the existing literature in several ways compared to previous empirical studies. Firstly, it investigates the impact of the interaction between ICT and industrialization on CO 2 emissions. Secondly, it examines the impact of the interaction between ICT and renewable energy sources on CO 2 emissions. Thirdly, it investigates the impact of the interaction between ICT and institutional quality on CO 2 emissions. These were investigated and the results were obtained within the context of short and long run estimates.
This study holds both academic and policy relevance due to the objectives outlined above. The primary theoretical contribution of this study is that it demonstrates how a modified Cobb–Douglas production function (Cobb and Douglas 1928 ), following previous empirical studies, can be used to investigate the objectives of this study. Under the research topic for this study, our findings suggested mitigation methods from a variable interaction approach at the panel level. We also take into account the issues of homogeneity and cross-sectional dependence (CD) in the series under the unit root and cointegration techniques. Most panel series in the literature assume that the residual terms are unrelated, which ignores the issues of CD between countries. Accounting for the issues of CD in the series is essential as BRICS countries possess varying levels of economic tiles. We applied the Dumitrescu and Hurlin ( 2012 ) panel causality technique that considers heterogeneity among countries. Additionally, to the best of our knowledge, this is the first study to simultaneously employ the novel Cross-Sectional Autoregressive-Distributed Lag (hereafter, CS-ARDL) technique proposed by Chudik and Pesaran ( 2015 ), as well as Augmented Mean Group (henceforth, AMG) and dynamic Common Correlated Effects Mean Group (CCEMG) estimation techniques, to analyze panel data from 2000–2021. While we used the AMG and CCEMG estimators to validate our results, we primarily relied on the CS-ARDL approach, given its advantages over the others. The CS-ARDL method has several advantages over other panel data estimators. It yields robust results in the presence of cross-sectional dependence and can be applied to series with different orders of integration, including I(0 ), I(1) , or both. Additionally, it produces accurate results in cases of weak exogeneity and allows for both pooled, mean group, and pooled-mean group estimates based on the homogeneity or heterogeneity of slope coefficients.
Based on the CS-ARDL results, which were further validated by the use of AMG and CCEMG approaches, this study recommends that BRICS governments and policymakers prioritize the use of ICT in the industrial and institutional sectors to achieve faster environmental sustainability in the short run. While the study advises caution in the long term as the interaction between ICT and renewable energy sources, industrialization, and institutional quality may not favour environmental quality. In conclusion, the study highlights the potential of ICT, renewable energy sources, industrialization, and institutional quality in achieving environmental sustainability in the BRICS countries, while recommending cautious measures in the long run to safeguard the progress made.
The organization of this paper is as follows: Sect. 2 presents the literature review. Section 3 presents the methodology and data. Results from the empirical analysis are presented and discussed in Sect. 4, while policy implications are found in Sect. 5. Finally, Sect. 6 concludes the study.
Literature review, theoretical framework and hypothesis development
Theoretical framework and hypothesis development.
The existing literature on the value of ICT at the country level primarily indicates that ICT investment positively impacts productivity in developed countries, but not in developing ones. However, with continued investment in ICT infrastructure, it is crucial to reassess its benefits in developing countries. Dedrick et al. ( 2013 ) explored this by analyzing data from 45 countries between 1994–2007, comparing it to the 1985–1993 period. They found that upper-income developing countries experienced significant productivity gains from ICT investment in the more recent period, with the effects of ICT on productivity being influenced by factors such as human resources and openness to foreign investment. Beyond productivity, ICT also holds potential for environmental sustainability, such as reducing emissions through smarter cities and optimizing production processes. Watson et al. ( 2010 ) highlight ICT's transformative ability to foster an environmentally sustainable society. This broadens the scope of ICT value research to include environmental aspects, aligning with the triple bottom line (TBL) concept that encompasses economic, environmental, and social dimensions of value.
The study is grounded in the Environmental Kuznets Curve (EKC) theory, which suggests that environmental degradation initially worsens with economic development, peaks at a certain point, and then improves as growth continues. The EKC framework posits that technological advancements and energy conservation can enhance environmental sustainability. Information and Communication Technologies (ICTs) are identified as a key factor in promoting environmental sustainability, impacting it in three ways: directly through consumption, indirectly through substitution, and systemically through rebound effects. The direct negative impacts of ICTs include the use of non-renewable energy in manufacturing ICT equipment and the disposal of e-waste (Park et al. 2018 ). However, ICTs can also positively influence environmental sustainability through green ICT initiatives, smarter cities, digital education, e-health, e-commerce, and virtual meetings (Shabani and Shahnazi 2019 ). Nonetheless, energy-intensive ICT activities can increase energy consumption, and the rebound effect—where the increased efficiency of ICT goods and services leads to greater usage—can exacerbate pollution (Danish et al. 2018a , b ). Additionally, the expansion of business activities and communication networks facilitated by ICT can negatively affect environmental quality (Ozcan and Apergis 2018 ).
The relationship between renewable energy sources and environmental sustainability is a critical area of study in the quest for sustainable development. Renewable energy sources, such as solar, wind, hydro, and biomass, are considered vital for achieving environmental sustainability due to their lower environmental impact compared to fossil fuels. Renewable energy sources produce significantly lower greenhouse gas emissions compared to fossil fuels, thereby mitigating climate change. For example, solar and wind energy have near-zero emissions during operation (IPCC 2021 ). Unlike fossil fuels, renewable energy sources are abundant and replenishable, reducing the strain on finite natural resources and ensuring long-term energy security (Goldemberg and Lucon 2009 ). Renewable energy technologies generate minimal air and water pollutants, improving air quality and reducing health risks associated with pollution (Jacobson et al. 2018 ). By reducing habitat destruction and pollution, renewable energy sources can help protect biodiversity and ecosystems (Sovacool and Dworkin 2014 ). The deployment of renewable energy can drive economic development through job creation, technology innovation, and energy access in remote areas (IRENA 2020 ). However, the transition to renewable energy is not without challenges, such as variability, storage, and the need for grid integration. Addressing these challenges requires technological advancements, policy support, and investment in renewable energy infrastructure.
The success of environmental policy measures is heavily influenced by the effectiveness of the institutions responsible for their implementation. Strong institutions can enforce laws that protect the environment and promote sustainable growth (Azam et al. 2021a , b ). However, political instability can undermine the government's ability to enforce pollution control policies, and political preferences on environmental issues can shift (Mrabet et al. 2021 ). Furthermore, weak institutions may be exploited by rent-seeking investors, leading to the failure of environmental policies (Baloch and Wang 2019 ).
Theoretical literature suggests that industrial development can impact environmental degradation. According to the theory of ecological modernization, industrial activities are part of a social transformation process that promotes modernization but can also lead to environmental issues. However, the negative environmental effects of industrial development may lessen due to technological advancement, urbanization, and a shift from a manufacturing-based economy to a service-based economy (Mol and Spaargaren 2000 ). The urban environmental transition theory similarly suggests that while manufacturing expansion can increase wealth, it may also intensify environmental pollution. Nevertheless, as a society becomes wealthier, pollution may decrease due to technological improvements or changes in the economic sectors' composition or structure (Hussain and Zhou 2022 ). Ehigiamusoe ( 2023 ) argued that industrial activities can emit carbon and other greenhouse gases, while Wen et al. ( 2014 ) noted that industrial development might not worsen carbon emissions if there is environmental awareness and the adoption of energy-efficient technologies. Drawing from the theoretical discussion above, this study examines the formulated hypotheses as follows:
\(Hypothesis {H}_{aii}:\) ICT development, renewable energy sources, institutional quality and industrialization causes environmental sustainability path in BRICS.
\(Hypothesis {H}_{bii}:\) ICT development, renewable energy sources, institutional quality and industrialization promotes environmental sustainability path in BRICS.
\(Hypothesis {H}_{cii}:\) The joint impact between ICT development and renewable energy sources, promotes environmental sustainability path in BRICS.
\(Hypothesis {H}_{dii}:\) The joint impact of ICT development and institutional quality promotes environmental sustainability path in BRICS.
\(Hypothesis {H}_{eii}:\) The joint impact of ICT development and industrialization promotes environmental sustainability path in BRICS.
Empirical literature
Global warming, precipitation variability, and significant climate changes pose a serious threat to sustainable development in BRICS countries, particularly affecting agricultural production and food and water supplies. This can directly impact investor behavior, financial markets, welfare, the environment, and economic growth. The increasing trends of extreme temperatures and rainfall in BRICS countries heighten the risks and uncertainties of environmental, climatic, and economic instability. Governments, farmers, and scholars are increasingly concerned about climate change risks to the economy, and adaptation strategies have become a crucial focus for researchers and policymakers. Understanding the nexus between CO2 emissions, renewable energy sources, ICT, and institutional quality is vital for the economic and environmental sustainability of BRICS countries. Some literature has explored the connections between pollution, agriculture, and economic development, as well as agriculture, energy consumption, and industrialization in developing and emerging countries. This research aims to provide policymakers with the necessary tools to mitigate climate risks and implement adaptation strategies.
Despite ongoing empirical research in environment and energy, climate change challenges keep the topic relevant, especially for developing nations. Many studies have examined the link between environmental and macroeconomic variables, such as agricultural performance and economic growth, often finding a bidirectional causal relationship between pollution and agricultural value added in regions like the EU and North Africa. (see for example, Bekun et al. 2019 ; Jebli and Youssef 2017 among others). In contrast, Asumadu-Sarkodie and Owusu ( 2016 ) sought to investigate the interconnections among CO 2 emissions, electricity consumption, industrialization, and economic development in Benin. The results of the long-run analysis show the existence of a positive relationship between industrialization and CO 2 emissions. In a study focused on Zambia’s economy, Phiri et al. ( 2021 ) highlighted the influence of agricultural practices on pollution. They also examined the relationship between agriculturalization, industrialization, and economic growth, finding that these factors are interrelated and tend to stabilize over time. However, their research is limited to certain African countries and does not explore other factors crucial for a sustainable environment, such as the interplay between ICT, renewable energy sources, and institutional quality.
For instance, limited empirical studies (Karim et al. 2022 ; Avom et al. 2020 ) focusing on the impact of institutional quality and other environmental factors in Sub-Saharan African countries suggest that strong institutional quality significantly reduces environmental pollution. Conversely, Acheampong et al. ( 2021 ) contend that there is no causal relationship between institutional quality and economic development. It is essential to categorize existing research on environmental sustainability into three distinct groups for a clearer understanding. Primarily, studies have focused on evaluating the role of the financial sector and its development (FD), information systems (ICTs), and renewable energy consumption (REC) as key factors in environmental pollution. For instance, Lahouel et al. ( 2021 ) examined Tunisia, Shabani and Shahnazi ( 2019 ) looked at Iran, Higón et al. ( 2017 ) covered selected developing and 26 industrialized economies, N’dri et al. ( 2021 ) focused on developing countries, Sahoo et al. ( 2021 ) studied India, Gyamfi et al. ( 2022 ) analyzed G7 countries, and Shobande and Ogbeifun ( 2022 ) investigated 24 OECD countries. The findings on the impact of ICTs on CO 2 emissions or environmental degradation remain inconclusive, with Shabani and Shahnazi ( 2019 ) noting a unidirectional causal relationship between ICT, economic development, and energy consumption with CO 2 emissions in the long term.
Conversely, Lahouel et al. ( 2021 ) observed a nonlinear relationship between CO 2 emissions and ICT, suggesting that ICT plays a crucial role in mitigating climate risk and promoting economic growth. Higón et al. ( 2017 ) explored the connection between environmental variables such as CO 2 emissions, economic growth (GDP), and ICT, finding a positive correlation between pollution and ICT, indicated by an inverted U-shaped curve. Similarly, N’dri et al. ( 2021 ) found that ICTs can reduce pollution in the long term. Sahoo et al. ( 2021 ) extended their analysis to India, including financial development and electricity alongside economic growth and CO 2 emissions, and argued that ICT negatively affects environmental sustainability while monetary expansion is inversely related to CO 2 emissions. Shobande and Ogbeifun ( 2022 ) also assert that ICT promotes ecological sustainability, highlighting the contribution of ICT and financial development to ecosystem sustainability.
A secondary group of research studies delves into the impact of institutional quality on environmental quality. Riti et al. ( 2021 ) investigated this relationship in ten countries with high press freedom, while Goel et al. ( 2013 ) focused on MENA economies. Chien et al. ( 2021 ) and Hussain and Dogan ( 2021 ) directed their studies towards BRICS economies, and Karim et al. ( 2022 ) examined 30 Sub-Saharan African countries. These studies collectively aim to understand the link between the quality of institutions and environmental outcomes in various global regions. Goel et al. ( 2013 ) examined the effects of corruption and the informal sector on environmental pollution, discovering that high corruption levels and the presence of a shadow sector can obscure actual CO 2 emissions. This underscores the importance of institutional management in mitigating environmental pollution.
Similarly, Riti et al. ( 2021 ) assessed the role of press freedom in influencing environmental degradation across ten countries, finding that greater press freedom is likely to reduce environmental degradation. Karim et al. ( 2022 ) evaluated the impact of institutional quality on CO 2 emissions in Sub-Saharan Africa and found that measures like corruption control, legislative quality, and rule of law significantly lower CO 2 emissions in the region. Furthermore, they find the existence of two-way causality between all institutional quality indices and CO 2 emissions. Henceforth, effective governance policies and regulations mitigate climate risk. Aligning the result with previous papers concentrating on the nexus between institutional quality and ecological pollution, Hussian and Dogan ( 2021 ) conclude that institutional quality and environmental-related technology negatively impact the environmental footprint in the BRICS economies. Jebli et al. ( 2020 ) investigated the nexus between CO 2 emissions, renewable energy consumption and economic growth using the generalized method of moments model. This study applied the econometric method to data from 1990 to 2015 for 102 countries classified by income level. The findings reveal a causality link between renewable energy consumption and other variables in all countries under study, decreasing CO 2 emissions.
Radmehr et al. ( 2021 ) focused on the EU’s regional economic zone, using the generalized spatial two-stage least square (GS2SLS) method to find that financial performance and renewable energy consumption have a unidirectional causal relationship and are positively correlated across countries. They also found a bidirectional link between CO 2 emissions and economic growth and renewable energy consumption. Acheampong et al. ( 2021 ) used a different approach, the GMM-based panel vector autoregressive technique, to study the intertemporal causality between economic growth, renewable energy, institutional quality, and CO 2 emissions. They found a bidirectional causality between renewable energy consumption and economic growth in the long run but no causality between economic growth and institutional quality. This contrasts with findings from Gyamfi et al. ( 2022 ), Riti et al. ( 2021 ), and Goel et al. ( 2013 ), who suggested that institutional quality can lead to environmental sustainability.
Furthermore, several studies have investigated the relationship between industrialization and environmental degradation, particularly focusing on CO 2 emissions. For example, an analysis of 99 countries across different income levels found that industrialization increases CO 2 emissions in all groups. Li and Lin ( 2015 ) confirmed this detrimental impact using data from 76 countries, while Sohag et al. ( 2017 ) reported similar findings for 86 nations. Opoku and Aluko ( 2021 ) employed quantile regression to examine the impact of industrialization on the ecological footprint in 37 African countries, finding mixed effects across different quantiles. Kahouli et al. ( 2022 ) identified a long-run unidirectional causal relationship from industrialization to CO 2 emissions in Saudi Arabia, with bidirectional causality in the short run. Similar unidirectional causality was reported by Nasir et al. ( 2021 ) in Australia, where Rahman and Alam ( 2022 ) also found bidirectional causality.
Usman and Balsalobre-Lorente ( 2022 ) observed that industrialization contributes to the ecological footprint in 10 newly industrialized countries. However, Naudé ( 2011 ) argued that industrialization could reduce emissions associated with agriculture by shifting labor to more energy-efficient and cleaner industrial sectors. Rafiq et al. ( 2016 ) found that industrialization reduces CO 2 emissions in high-income countries but has an insignificant effect in low- and middle-income countries. Zhou et al. ( 2013 ) discovered that industrial adjustment can mitigate CO 2 emissions in China, while Lin et al. ( 2015 ) and Ehigiamusoe ( 2023 ) found no adverse effects of industrialization on CO 2 emissions in Nigeria and ASEAN + China, respectively.
Renewable energy is increasingly recognized as a means to promote environmental and ecological sustainability alongside economic growth (Nazir 2023 ). However, empirical studies exploring the relationship between renewable energy consumption and industrial growth are limited. Dey et al. ( 2023 ) and Van Hoang ( 2020 ) are among the few who have examined this relationship. Van Hoang ( 2020 ) found that in the United States, renewable energy consumption not only supports industrial production, but the type of energy also plays a significant role. Specifically, biomass energy had a more pronounced effect on industrial production compared to hydroelectric and geothermal energy, though its impact takes time to manifest. The study also identified a bidirectional causality between renewable energy consumption and industrialization.
Dey et al. ( 2023 ) analyzed the effects of various renewable energy sources, including biomass, solar, wind, bagasse, small hydropower (SHP), and waste, on sustainable industrialization in India. Using quantile regression, the study found that SHP and bagasse, in particular, contributed to increased industrial production. The research also revealed bidirectional non-linear causality between biomass, waste heat, and industrial production, and unidirectional non-linear causality with other energy sources. Mentel et al. ( 2022 ) investigated the relationship between industrialization and CO 2 emissions in Central Asia and Europe, considering the moderating effect of renewable energy. Employing the two-step GMM method and data from 2000 to 2018, the study concluded that industrial development exacerbates CO 2 emissions, while the moderating effect of renewable energy is negative.
Fossil fuels, which account for nearly one-third of global pollution, are considered one of the least sustainable energy sources and contribute significantly to environmental damage (Kumar and Majid 2022 ). Rapier ( 2020 ) notes that energy from natural resources fulfilled 84% of the total energy demand up to 2019, highlighting their role as major pollutants. To achieve environmental sustainability, transitioning to renewable energy consumption is essential as it can meet energy demands with fewer environmental impacts (He et al. 2023 ). Nasreen et al. ( 2017 ) emphasize that unsustainable energy sources like coal and fuel have serious environmental consequences. Conversely, renewable energy is key to reversing environmental damage and attaining environmental sustainability. Wada et al. ( 2021 ) argue that natural resources-powered fuels such as coal and gas increase pollution levels, whereas renewable energy offers a viable solution to this issue.
Iorember et al. ( 2020 ) find that renewable energy can reduce CO 2 emissions and suggest that governments should develop policies promoting long-term renewable energy use. Achuo et al. ( 2022 ) note that energy efficiency through increased renewable energy can significantly reduce environmental pollution and achieve environmental sustainability. Asongu et al. ( 2020 ) highlight the dangers of continued fossil fuel use and advocate for a shift towards renewable energy. Liu et al. ( 2022 ) suggest that alternative energy can reduce pollution in thermal machines. Overall, renewable energy is crucial for promoting environmental sustainability.
Asumadu and Owusu ( 2016 ) explored the causal relationship in African countries, specifically in Benin's economy, using the ARDL approach. They discovered that a slight 1% increase in industrialization and electricity consumption leads to a rise in CO 2 emissions. However, the paper did not assess the impact of ICT on CO 2 emissions nor provided policies for sustainable environmental management. Sustainable development is a key agenda for the BRICS union, achievable through policies promoting ecological sustainability. Thus, it is essential to extend previous research to investigate the role of ICT in enhancing environmental sustainability in BRICS economies. In the light of the above previous literature, we further carried out a literature survey on the nexus between ICT, renewable energy sources, industrialization, institutional quality and CO 2 emissions which is examined from four perspectives: ICT impact on CO 2 emissions (both direct and indirect impacts); institutional quality-CO 2 emissions nexus; renewable energy - CO 2 emissions nexus; and industrialization-CO 2 emissions nexus (see Table 1 ). The results reveal various scenarios and insights derived from these linkages.
Despite empirical literature on environmental protection and quality, to the best of our knowledge, no studies have examined how ICT can distinctively complement renewable energy sources, institutional quality, and industrialization concurrently in mitigating CO 2 emissions in BRICS countries using the novel CS-ARDL approach. It is on this basis that we embark on this study for the purpose of recommending policies that will help the economic bloc achieve faster and sustained environmental quality.
Empirical methodology and data
Empirical strategy.
The preliminary empirical strategy used in this study include principal components approach/analysis (PCA), descriptive analysis, scatter plot (graph), panel unit root test (first-and second-generation), slope homogeneity test, cross-sectional dependence (CD) test, CIPS panel unit root tests, panel cointegration test (first- and second-generation), fully modified ordinary least square (FMOLS) and the dynamic OLS (DOLS), Dumitrescu and Hurlin ( 2012 ) panel causality, AMG and CCEMG estimation techniques.
This study employs a comprehensive econometric approach to analyze the data, following a systematic sequence of steps designed to ensure robustness and accuracy in the results. The methodology is outlined as follows: The analysis begins with Principal Component Analysis (PCA), which is used to reduce the dimensionality of the dataset while retaining the variability present in the data. This step helps in identifying the key components that explain the majority of the variance in the data. Following PCA, descriptive statistics are computed to provide a summary of the central tendency, dispersion, and shape of the dataset's distribution. This step offers a preliminary insight into the data's characteristics. Scatter plots are employed to visually examine the relationships between variables. This step aids in identifying any potential linear or nonlinear patterns, outliers, or clusters in the data. The slope homogeneity test is conducted to assess whether the slopes of the regression lines are homogeneous across different panels or groups in the dataset. This step is crucial for determining the appropriateness of pooling the data for further analysis.
The Cross-sectional Dependence (CD) test is performed to detect the presence of cross-sectional dependence in the panel data. This step is essential to ensure that the subsequent econometric techniques account for any interdependencies between cross-sectional units. The panel unit root test is applied to ascertain the stationarity properties of the variables. This step is necessary to avoid spurious regression results and to determine the appropriate level of differencing required for the analysis. Once the variables are confirmed to be stationary, a cointegration test is conducted to examine the long-run equilibrium relationships among the variables. This step helps in identifying any long-term associations that exist in the panel data. The panel causality test is employed to investigate the direction of causality between variables. This step provides insights into the dynamic interactions and causal relationships among the variables under study. Finally, the study employs panel Cross-sectionally Augmented Autoregressive Distributed Lag (CS-ARDL), Augmented Mean Group (AMG), and Common Correlated Effects Mean Group (CCEMG) estimations to analyze both the short-run and long-run relationships between the variables. The Cross-Sectional Augmented Autoregressive Distributed Lag (CS-ARDL) estimation method accounts for differences across countries in a panel data estimation (Chudik and Pesaran 2015 ). This approach is an extension of the ARDL model to panel data, and it is designed to handle heterogeneity across cross-sectional units (e.g., countries) by allowing for country-specific short-run dynamics and long-run relationships (Saba and Monkam 2024 ).
In the CS-ARDL model, the cross-sectional dependence among countries is addressed by augmenting the model with the cross-sectional averages of the dependent and independent variables (Chudik and Pesaran 2015 ). This approach helps to capture common factors that influence all countries in the panel, thereby controlling for cross-sectional dependence and heterogeneity (Chudik and Pesaran 2015 ). By incorporating country-specific effects and dynamics, the CS-ARDL model provides a more nuanced understanding of the relationships in the data, taking into account the unique characteristics and circumstances of each country in the panel (Chudik and Pesaran 2015 ).
These advanced econometric techniques account for cross-sectional dependence, heterogeneity, and dynamic interactions in the panel data. By following this methodological sequence, the study aims to provide a comprehensive and robust analysis of the relationships between the variables of interest, ensuring the validity and reliability of the econometric findings. To save space, we did not provide all the estimated equations for the aforementioned econometric techniques because they are readily available in other empirical literatures. But we rather focused our attention on the main estimation technique which is the CS-ARDL econometric technique. Figure 2 provides a visual representation of the methodological approach for the readers' ease of comprehension.
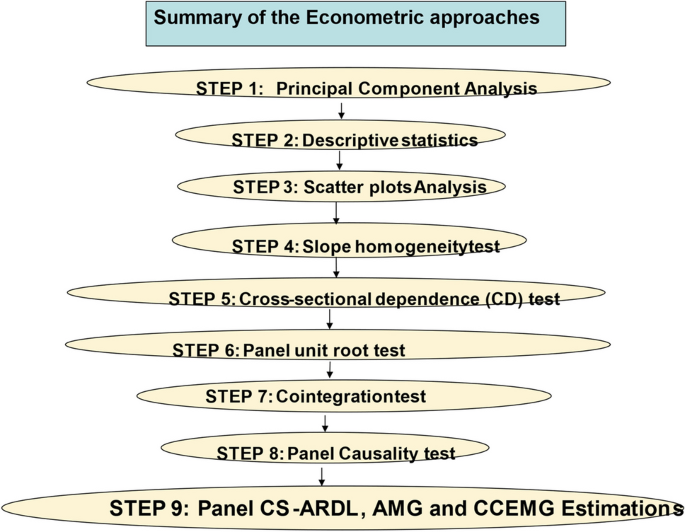
Summary of the Econometric approaches
Brief theoretical framework and empirical model specification
Theoretically, this paper utilizes the Cobb–Douglas production function (Cobb and Douglas 1928 ) with necessary modification for the purpose of investigating the relationship between ICT, renewable energy sources, industrialization, institutional quality and CO 2 emissions within the context of BRICS economies. The aggregate growth function can be illustrated using a typical Cobb–Douglas production function with a constant rate of returns which is as follows:
where \(Y, k and L\) is GDP, capital and effective labor, respectively. Theoretically, levels of income and CO 2 emissions are related since it is widely believed that as economic activities take place in a country, it equally leads to the release of CO 2 emissions in the economy (Kuznets 1955 ; Saba 2023a , 2023b ). Moreover, rapid economic expansion is associated with a whole lot of factors (such as the use of ICT, energy, institutions etc.) during the production process, while increasing the proportion of renewable energy sources in total energy use such that it contributes to environmental sustainability by curbing CO 2 emissions from dirty energy sources. Therefore, the augmented CO 2 emission function can be expressed as:
where \(LCO2EM\) and \(X\) represents log of CO 2 emissions (proxy for environmental sustainability) and regressors, Footnote 1 respectively. The basic econometric models which were later transformed to CS-ARDL model and estimated can be found below:
Model 1 :where \(\beta\) , \({\beth }_{1},\dots ,{\beth }_{4},\) and \({\upepsilon }_{it}\) represents the constants, coefficient and the error term, respectively. Model 1 excludes the interaction terms between ICT and LIND, LICT and RESS, LICT and INSQTY, while the rest of the models (that is, model 5–8) does in a systemic manner one after the other.
Model 2: Capturing the interaction between LICT and LIND
Model 3: Capturing the interaction between ICT and RESS
Model 4: Capturing the interaction between ICT and INSQTY
We specify the CS-ARDL model below which took it bearing from the above equations:
where \(\Delta LCO2EM,\) \({X}_{i,t}\) , \({\overline{LCO2EM}}_{t-1}\) & \({\overline{X}}_{t-1}\) , \({\Delta LCO2EM}_{i,t-j} \& {\Delta X}_{i,t-j}\) , \(\Delta {\overline{LCO2EM}}_{t} \& \Delta {\overline{X}}_{t}\) and \({u}_{it}\) are dependent variable, all independent variables during the long run, mean of the dependent and explanatory variables in the long run, dependent and independent variables in the short run, mean dependent and independent variables during the short run and the error term, respectively. Furthermore, where j, t, \({\beth }_{1i}\) , \({\gamma }_{1i}\) , \({\Gamma }_{ij}\) , \({\varnothing }_{1i}\) and \({\varnothing }_{2i}\) denotes cross-sectional dimension, time, coefficients of the independent variables, short-run coefficient of the dependent variable, short-run coefficients of the independent variables, mean of dependent variables and mean of independent variables in the short-run, respectively. The details of the dependent and independents variables can be found in Table 2 . We used the AMG and CCEMG for robustness checks.
Data and variables description
This study utilized annual panel data for 5 BRICS countries (that is Brazil, China, India, Russia and South Africa) covering the period from 2000 to 2021. The data were sourced from three main databases, namely, the International Telecommunication Union (ITU), the World Bank's World Development Indicators (WDI), and the World Governance Indicators (WGI). The time span and countries used were selected based on data availability. The variables ICT, renewable energy sources, and institutional quality were obtained from the indicators listed in Tables 1 and 2 through the utilization of PCA. Table 2 list the variables used in this study. The justification for including the regressors in the model is explained briefly below:
Renewable energy sources (RESS) : Renewable energy has been identified in the environmental and energy literature as a potent tool that can help to combat environmental degradation and promote environmental sustainability, as the world grapples with the worsening impact of climate change across different sectors of the economy. Several studies that support this notion include Sharif et al. ( 2021 ), You and Kakinaka ( 2022 ), Saba and Ngepah ( 2022a , b ), Saba and Biyase ( 2022 ), and Apergis et al. ( 2023 ), among others. Therefore, it is important to investigate the critical role that renewable energy has played in achieving environmental sustainability by the BRICS’s energy sector.
Industrial value added (proxy for industrialization) (INDU) : Literature has shown that historically, industries have been a significant source of pollution due to their continuous demand for and use of fossil fuels in their production processes. The continuous use of energy pollutes the environment by increasing CO 2 emissions, which indirectly affects economic growth (Anwar and Elfaki 2021 ; Elfaki et al. 2022 ). Therefore, it is important to investigate the role that the industrial sector plays in the path towards environmental sustainability in BRICS, especially when the variable is interacted with ICT. This is because the industrial sector utilizes ICT infrastructure in its production processes.
GDP per capita (proxy for levels of income/economic growth) (GDPC) : The empirical literature has shown that the levels of income/output/economic growth contribute to environmental degradation at country-specific, regional, and global levels (see among others: Aslam et al. 2021 ; Saba 2023b ; Boukhelkhal 2022 ). This is why the challenge of how to limit environmental pollution while preserving growth is a concern for policymakers today, and it is especially crucial for BRICS. Hence, further investigation into the role that economic growth plays using more advanced econometric approaches is crucial for providing policy directions.
Information and communications technology (ICT) : Policymakers can leverage ICT diffusion to address critical policy concerns related to sustainable development goals, such as improving access to affordable and clean energy and mitigating global warming (climate action). This is because ICT is strongly associated with sustainable development as it helps reduce CO 2 emissions by (a) lowering unnecessary transportation costs, (b) enhancing quality of life, (c) increasing productivity and financial affairs for households and firms, and (d) facilitating easy access to resources and knowledge, thereby reducing information asymmetry levels (Faisal et al. 2020 ; Chi and Meng 2022 ). Therefore, we are examining the role of ICT in promoting environmental sustainability in BRICS.
Institutional quality (INSQ) : In the extant literature, institutional quality has been strongly linked to environmental quality, as it influences every sector of the economy. The policies that political institutions adopt to provide the cultural and legal framework for the successful implementation of programs, projects, and societal activities could be tied to institutional quality (Yang et al. 2022 ; Jahanger et al. 2022 ). Therefore, examining its importance to environmental quality cannot be overemphasized, as it showcases the government's capacity and willingness to implement rules and laws meant to promote society in all its spheres (Islam et al. 2021 ; Haldar and Sethi 2021 ). Environmental policies cannot operate outside the established institutions in a society.
Empirical results and discussion
Preliminary analysis, principal component analysis.
Table 3 presents the principal component approach and correlation matrix results for institutional quality (INSQTY), LICT and renewable energy sources (RESS) variables. We first started by testing whether or not there are some degree of association between the indicators used to generate an index for each of the variables, that is, INSQTY, LICT and RESS. The results in Panel A, B and C show that the indicators are strongly correlated, hence, we proceeded to the estimation of the PCA given that the condition of the indicators being correlated was filled (Saba and Ngepah 2022a , 2022b , 2022c ). To create a composite index for institutional quality, we selected the first principal component that explains the highest percentage of the total variation. We followed the same approach for LICT and RESS. We selected the first component for the INSQTY, LICT, and RESS variables because its eigenvalue accounts for the highest percentage of the total variation, which is 4.03%, 2.08%, and 1.34%, respectively. The scree plots in Fig. 3 further supports our results.
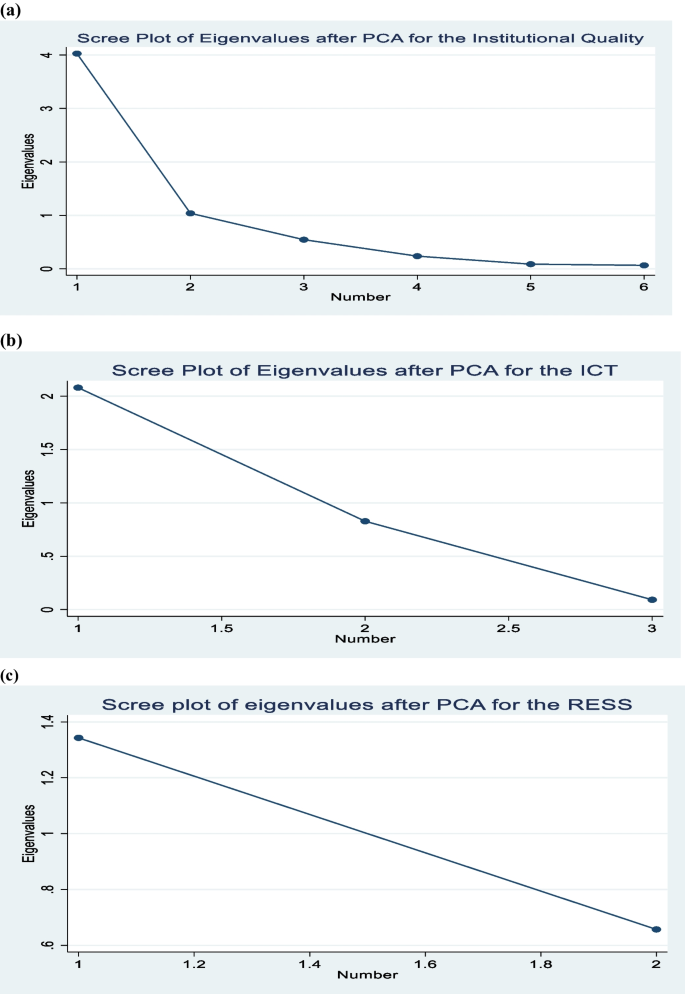
(a) Scree plot for institutional quality; ( b ) Scree plot for ICT; and ( c ) Scree plot for renewable energy
Summary statistics and scatter plot analysis
Table 4 (Panel A) presents the summary statistics results for the variables. We observed that for the series, the mean (or median) value of LCO2EM, LIND, LGDPC, LICT, RESS and INSQTY is around 1.419 (1.846), 3.389 (3.355), 8.568 (8.900), 0.100 (0.474), -0.023 (0.032) and 2.20E-09 (-0.254), respectively. The maximum and minimum values of the variables are found to be approximately between 9.392 and -5.045, respectively. 0.841, 0.240, 0.814, 1.361, 1.164 and 2.007 for LCO2EM, LIND, LGDPC, LICT, RESS and INSQTY, respectively, which indicate the variation in series. The series with the negative and positive values of skewness shows a negatively and positively skewed distribution for the variables, respectively.
The summary statistics presented in Table 4 (Panel A) provide insights into the distribution of the variables in the study, which include CO 2 emissions (LCO2EM), industrialization (LIND), GDP per capita (LGDPC), information and communications technology (ICT), renewable energy sources (RESS), and institutional quality (INSQTY). The mean (average) and median (middle value) of the variables provide a sense of the central tendency. For example, the mean value of CO 2 emissions is 1.419, and the median is 1.846, indicating that the distribution is skewed since the mean is less than the median. The range of values, indicated by the maximum and minimum, shows the extent of variation in the data. For instance, CO 2 emissions vary between -5.045 and 9.392, suggesting a wide range of emissions levels across the observations. The standard deviation values (0.841 for LCO2EM, 0.240 for LIND, etc.) measure the dispersion of the data around the mean. A higher standard deviation indicates greater variability. For example, ICT has a relatively high standard deviation of 1.361, suggesting diverse levels of ICT adoption among the observations. The skewness values indicate the asymmetry of the distribution. A positive skewness (e.g., 0.465 for LIND) means the tail on the right side of the distribution is longer, while a negative skewness (e.g., -0.337 for LCO2EM) indicates a longer tail on the left side. This information helps understand the distribution's shape and potential outliers. The kurtosis values measure the 'tailedness' of the distribution. A kurtosis greater than 3 (e.g., 4.566 for ICT) indicates a distribution with heavier tails and a sharper peak compared to a normal distribution, suggesting a higher likelihood of extreme values. The variability in CO 2 emissions, GDP per capita, and ICT suggests differing levels of economic development and technological adoption among the countries in the sample.
Based on the summary statistics provided, we can make some descriptive observations about the levels of environmental sustainability, ICT, renewable energy, industrialization, and institutional quality in the dataset: Firstly , the mean and median values of CO 2 emissions (LCO2EM) suggest that there is a wide range of emissions levels across the observations, with some countries having significantly higher emissions than others. The negative skewness indicates that the distribution is skewed towards lower emissions, but the presence of high kurtosis suggests that there are also countries with extremely high emissions levels. Secondly , the mean and median values for ICT are relatively low, indicating that overall ICT adoption may be limited in the sample. However, the high standard deviation and kurtosis suggest that there is significant variability, with some countries having much higher levels of ICT adoption.
Thirdly , the mean value of renewable energy sources is slightly negative, while the median is positive, indicating a skewed distribution with a majority of countries having low levels of renewable energy usage. However, the presence of countries with higher usage is also indicated by the positive skewness and kurtosis. Fourthly , the mean and median values for industrialization are relatively close, suggesting a more symmetric distribution. However, the positive skewness and high kurtosis indicate that there are countries with significantly higher levels of industrialization, contributing to the tail on the right side of the distribution. Fifthly , the mean value is very close to zero, and the median is negative, suggesting that overall institutional quality may be low in the sample. The positive skewness and kurtosis indicate that while most countries have lower institutional quality, there are a few countries with much higher quality.
Overall, the levels of environmental sustainability, ICT, renewable energy, industrialization, and institutional quality vary widely across the countries in the sample. There are indications of both low and high levels of each variable, with significant variability and skewness in the distributions. These descriptive insights serve as a foundation for deeper analysis and the application of the various econometric techniques utilized in this study to elucidate the relationships among these variables and their effects on environmental sustainability.
Figure 4 presents scatter plots that visually demonstrate the relationship between CO 2 emissions and the explanatory variables. The scatter plot graphs reveal that CO 2 emissions has a positive relationship with industrialization, GDPC and ICT, while it has a negative relationship with renewable energy sources and institutional quality. Therefore, this initial relationship was provided only to give an indication of the potential relationship that may exist between CO 2 emissions and the other explanatory variables in BRICS within the time periods examined. It should be noted that the positive relationship between CO 2 emissions and some of the explanatory variables cannot be properly validated since the scatter plots graphical approach does not account for cross-sectional dependence issues.
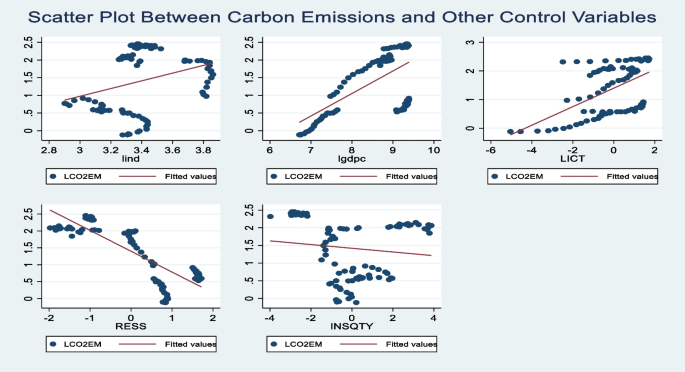
Scatter plots revealing the relationship between CO2 emissions and the regressors
Slope homogeneity, cross-sectional dependence (CD) , panel unit root, and cointegration analysis
To begin our study, we conducted a slope homogeneity test suggested by Pesaran and Yamagata ( 2008 ), and the results of the test can be found in Table 5 . Here both test statistics (delt and adjusted delta) and probability values seem to reject the assumption of homogenous slope coefficients at the 1% significance level. This would suggest the presence of strong country heterogeneity (in the long run) for the variables under consideration. To account for cross-sectional dependence, we tested for cross-sectional dependence in the data using Pesaran ( 2004 ) and Breusch and Pagan ( 1980 ) LM test techniques. The two approaches result in Table 6 show that there is evidence of cross-sectional dependence in the series, with p- values for the statistic being statistically significant at 1%. Therefore, the null hypothesis (cross-sectional independence) is rejected. This implies that levels of all the variables in one member of BRICS depend on the movements of these aspects in at least one other member within the BRICS.
Although the asymptotic results of all first-generation panel unit root (that is, Levin-Lin-Chu (LLC) (Levin et al. 2002 ) and Im-Pesaran-Shin (IPS) (Im et al. 2003 )) tests rely on the assumption of cross-sectional independence, we still tested the first-generation panel unit root. However, their reliability was less important. Table 7 shows the results at levels and first differences to determine whether the variables were integrated of order zero or one. The stationarity tests for the two techniques had a null hypothesis of a unit root. While some variables were not stationary at levels, a critical examination of the results in the last column of Table 7 shows that all variables had an integrated order of 1 at a significance level of at least 1%, indicating perfect stationarity at first differences (except for two variables). Like the first-generation panel unit root results, the second-generation panel unit root (CIPS) results of Pesaran ( 2007 ) showed that the series were integrated of order 1 at least at a 1% significance level (see Table 8 ). This indicates that we can proceed to test the long-run equilibrium relationship between the series using the second-generation cointegration approach. More importantly, based on these estimates and the aforementioned unit root and cross-dependency tests, it does confirm the adequacy of the use of the panel CS-ARDL estimator to determine the existence of a possible relationship between all the variables used in this study.
To investigate the long-run equilibrium relationship between the variables, we used the Pedroni and Westerlund panel cointegration tests of cointegration. The results of the Pedroni cointegration test (refer to Table 9 ) reveal that there exists a long-run equilibrium relationship between the variables since the values of the rho -statistics, PP -statistics, and ADF -statistics were statistically significant at least at the 10% significance level for the Within-dimension estimates. While for the Between-dimension estimates the values of the PP -statistics, and ADF -statistics were statistically significant at least at the 10% significance level which implies that there is long-run equilibrium relationship between the variables. To perform robustness checks within the context of cointegration test, and account for the presence of cross-sectional dependence in our data, we employed the Westerlund (2007) cointegration test. The results of the test (refer to Table 10 ) reveal that the values of \({G}_{t}\) , \({G}_{a}\) , \({P}_{t}\) and \({P}_{a}\) statistics were statistically significant at least at the 10% significance level which implies that there is also long-run equilibrium relationship between the variables despite the presence of cross-sectional dependence in the data.
We used FMOLS and DOLS techniques proposed by Pedroni ( 2001 , 2004 ) to estimate the long-run coefficients of the explanatory variables, as these techniques address serial correlation and endogeneity issues better than OLS. The R-squared and Adjusted R-squared values, which were above 80% for both approaches, demonstrate that our models were correctly specified. Therefore, we can proceed to interpret our estimated results. For Column 1, in the long run, renewable energy sources and institutional quality (except for DOLS) significantly contribute to reducing CO 2 emissions, while ICT, industrialization, and GDP per capita have the opposite effect. These results are consistent with the findings of Adebayo ( 2020 ), Teng et al. ( 2021 ), Adebayo and Kalmaz ( 2021 ), Azam et al. ( 2021a , b , 2022 ), Raihan and Tuspekova ( 2022a , b , c , d ), among others. This underscores the importance of exploring the role that ICT could play when it is interacted with the other explanatory variables. When LICT is interacted with industrialization (refer to Column 2 of Table 11 ), the results reveal that both variables reduce CO 2 emissions. However, for the impact of the interaction between LICT and renewable energy on CO 2 emissions, the opposite is observed in the long-run cointegration (refer to Column 3 of Table 11 ). While the impact of the interaction between LICT and institutional quality on CO 2 emissions also reduces CO 2 emissions (refer to Column 4 of Table 11 ). This leads us to investigate the causality relationships that may exist between our variables of interest.
Panel causality and CS-ARDL, AMG and CCEMG estimates analysis
This section analysed the causal relationship between the series under review. Table 12 presents the panel causality test results. In Table 12 , two-way causality exists between GDP per capita and CO 2 emissions. This implies that the two variables depend on each other, and it is consistent with Esso and Keho ( 2016 ) and Boukhelkhal’s ( 2022 ) findings but contradicts Acheampong's ( 2018 ) study. While unidirectional causality runs from: CO 2 emissions to industrialization which is in line with the findings of Shahbaz et al. ( 2014 ); ICT to CO 2 emissions which is consistent with the findings of Faisal et al. ( 2020 ); and renewable energy sources to CO 2 emissions.
One of the reasons that could be responsible for this is that renewable energy sources like wind or solar power may be located in remote areas in the BRICS countries where the electricity grid infrastructure is not well-developed. In these cases, the energy generated from renewable sources may need to be transported long distances, which can result in some carbon emissions from the transportation of equipment, materials, and personnel. There was no causality between institutional quality and CO 2 emissions which implies that they are independent of each other in BRICS economy. Even if institutions exist to regulate carbon emissions, they may not be enforced effectively. This could be due to corruption, lack of resources, or other factors that limit the ability of institutions to implement and enforce regulations. We rejected/accepted the null hypothesis that there was no causation for each Chi square-value statistic since their p-values were less/greater than the 10% significance level.
The causal relationships observed in the panel causality test results can be justified using various economic and environmental theories: firstly , the two-way causality between GDP per capita and CO 2 emissions can be explained by the Environmental Kuznets Curve (EKC) theory. The EKC hypothesis suggests that environmental degradation initially increases with economic growth, reaches a peak, and then declines as the economy continues to grow (Grossman and Krueger 1991 ). This theory supports the bidirectional causality found in this study and aligns with the findings of Esso and Keho ( 2016 ) and Boukhelkhal ( 2022 ) as mentioned earlier. Secondly , the unidirectional causality from CO 2 emissions to industrialization can be explained by the Pollution Haven Hypothesis (PHH). According to the PHH, countries with less stringent environmental regulations attract more pollution-intensive industries, leading to higher CO 2 emissions (Copeland and Taylor 2017 ). This causal relationship is consistent with the findings of Shahbaz et al. ( 2014 ) as mentioned earlier.
Thirdly , the causality from ICT to CO 2 emissions can be explained by the theory of ecological modernization. This theory suggests that technological advancements, such as ICT, can lead to more efficient and less resource-intensive production processes, thereby reducing environmental impacts (Mol and Spaargaren 2000 ). This is consistent with the findings of Faisal et al. ( 2020 ) as mentioned earlier. Lastly , the unidirectional causality from renewable energy sources to CO 2 emissions is supported by the theory of sustainable development. Renewable energy sources, such as solar and wind, are cleaner and produce fewer emissions compared to fossil fuels. The adoption of renewable energy can lead to a reduction in CO 2 emissions, supporting the transition to a more sustainable energy system (Holden et al. 2014 ). Figure 5 presents the visual summary of the Dumitrescu and Hurlin ( 2012 ) panel causality test results.
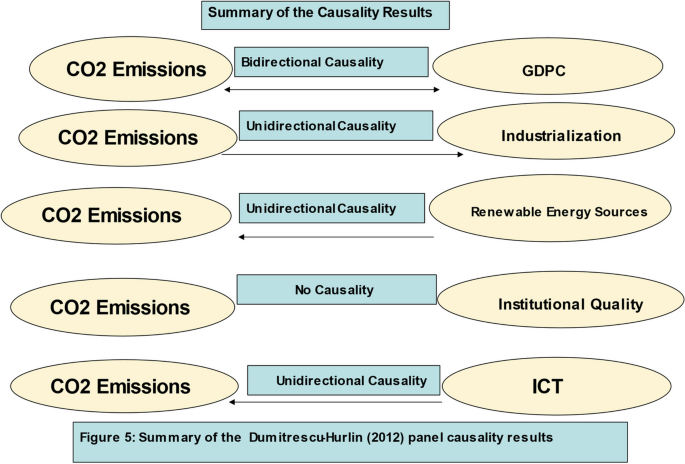
Dumitrescu and Hurlin ( 2012 ) panel causality test results
Table 13 presents the panel CS-ARDL results. Beginning with the estimates of the Error Correction Term (ECT) for all regression models, the values are -1.344 (refer to Column 1), -1.413 (refer to Column 2), -1.061 (refer to Column 3), and -1.390 (refer to Column 4), which are significant at least at the 1% level of significance. These values suggest that there is a strong negative relationship between the deviations from the long-run equilibrium and the short-run changes in the CO 2 emissions variable. Specifically, the negative ECT values indicate that any deviations from the long-run equilibrium will be corrected at a rate of approximately 1.344, 1.413, 1.061, and 1.390 units per period, respectively, suggesting that the CO 2 emissions variable will adjust back towards its equilibrium level relatively quickly. Additionally, the R-squared values for all the models are above 50%, which implies that our models are correctly specified.
For the first model which shows the results without the interaction term variables, in Column 1 of Table 13 , the CS-ARDL results show that both in the short and long run, at a 10% level of significance, the impact of industrialization and GDP per capita on CO 2 emissions is significant and positive. Specifically in the short run, a 1% increase in industrialization and GDP per capita led to a 0.43% and 0.45% increase in CO 2 emissions, respectively. These findings are consistent with the studies of Ike et al. ( 2020 ), Aslam et al. ( 2021 ) and Zafar et al. ( 2022 ). In the short and long run, it is only renewable energy sources that has a significant and negative impact on CO 2 emissions at least at 5% level of significance. In the short and long run, a 1% increase in renewable energy sources leads -0.36% and -0.27% decrease in CO 2 emissions, respectively. This implies that both in the short and long run renewable energy sources promotes environmental sustainability in BRICS countries. Both the ICT and institutional quality variables in the short and long run does not significantly impact CO 2 emissions. This contradicts the findings of Lu ( 2018 ) and Haseeb et al. ( 2019 ) Warsame et al. ( 2022 ) because they used different methodologies and focused on different regions.
For the second model, which shows the impact of the interaction between industrialization and ICT on CO 2 emissions reveal that in Column 2 of Table 13 , the CS-ARDL results show that in the short run the interaction between industrialization and ICT has a negative and significant impact on CO 2 emissions. This indicate that a 1% increase in the interaction between industrialization and ICT leads to -0.09% decrease in CO 2 emission, while in the long run the interaction between the two variables does not have a significant impact on CO 2 emission. This suggests that industrialization supported by ICT can help to reduce CO 2 emissions in BRICS in the short run. This concurs with Asumadu-Sarkodie and Owusu ( 2017 ) and Elfaki, et al.’s ( 2022 ) studies and counters with the study of Asumadu-Sarkodie and Owusu ( 2016 ). In the long run, the interaction between the two variables does not have significant impact on CO 2 emission. This further implies that the interaction between the two variables is environmentally sustainable in the short run.
For the third model, which shows the impact of the interaction between renewable energy sources and ICT on CO 2 emissions, it is revealed that in Column 3 of Table 13 , the CS-ARDL results show that in the short and long run, the interaction between renewable energy sources and ICT has a positive and significant impact on CO2 emissions. This indicate that a 1% increase in the interaction between renewable energy sources and ICT in the short and long run leads to 0.15% and 0.15% increase in CO 2 emission, respectively. This implies that the interaction between the two variables contributes to the increase in CO 2 emission both in the short and long run. This finding is not consistent with the studies conducted by Ozcan and Apergis ( 2018 ) for emerging countries, Salahuddin and Alam ( 2015 ) for Australia, Ibrahim and Waziri ( 2020 ) for SSA, and Mehrjo et al. ( 2022 ) for Iran. The differences in the findings could be due to various factors, such as differences in the time period studied, the theoretical framework used, econometrics techniques and other related factors. This is not as expected for the BRICS economy, and some possible reasons for the short- and long-run results could be that: (i) the increased renewable energy consumption of ICT infrastructures has not been sufficient to contribute to the reduction of CO 2 emissions given that the energy use in the BRICS countries is mostly generated from fossil fuels/non-renewable sources, on which they heavily rely. (ii) Their production and disposal of ICT devices and equipment could have led to significant environmental damage and carbon emissions. This includes the energy used in the production of ICT devices, as well as the carbon emissions generated by the disposal of electronic/ICT devices. (iii) the possible poor/inadequate link between the manufacturing and transportation of renewable energy equipment and the concurrent use of ICT infrastructures. This is especially true if the manufacturing and transportation of renewable energy equipment, such as solar panels, wind turbines, etc., processes are not properly optimized with the use of ICT infrastructures to reduce carbon emissions (Lee et al. 2017 ; Godil et al. 2021 ; Khan et al. 2022a , b ; Batool et al. 2022 ).
For the fourth model, which shows the institutional quality-ICT induced impact on CO 2 emissions reveal that in Column 4 of Table 13 , the CS-ARDL results show that in the short run, the interaction between the two variables has a negative and significant impact on CO 2 emissions. This indicate that a 1% increase in the interaction between the two variables leads to -0.02% decrease in CO 2 emission. This agrees with the findings of Cansino et al. ( 2019 ) Haldar and Sethi ( 2021 ) and Mahjabeen et al. ( 2020 ). While in the long run the interaction between the two variables does not have a significant impact on CO 2 emission. This implies that the interaction between institutional quality and ICT contributes to the reduction of CO 2 emission in the short run, while in the long run, it does not have a significant impact on CO 2 emission. This further implies that the interaction between the two variables is environmentally sustainable in the short run.
Based on the results, the negative impact of the interaction between ICT and institutional quality on CO 2 emissions in the short run is significant. This can be attributed to the fact that BRICS countries, with their growing economies, large populations, and increasing global political influence, have more advanced institutional development compared to many other developing countries. However, it is important to recognize that each BRICS country faces its own unique challenges and opportunities for development, with progress varying significantly within each nation. The success of policies related to industry, ICT, the environment, and renewable energy sources is heavily influenced by the effectiveness of domestic institutions. Without supportive institutions in the long term, the government's ability to enact and enforce policies in these sectors can be severely hampered. Therefore, it is crucial to implement and review strategies and initiatives that promote the six governance indicators used to compute institutional quality, to ensure ICT development and mitigate CO 2 emissions for environmental sustainability in the BRICS countries. Additionally, BRICS governments should consider imposing long-term CO 2 emission caps on the economic, ICT, and industrial sectors to keep environmental pollution levels under control. This is crucial because, in theory, ICT could promote environmental pollution through the production of machinery and devices, as well as the recycling of electronic waste. Furthermore, polluters are less likely to engage in pollution-causing behavior if they fear facing harsh punishment, which could take any form adopted by governments. Given that industrial production processes in BRICS still heavily rely on dirty energy, it is important for industrialists to consider integrating and promoting the use of ICT to achieve environmental sustainability.
Robustness checks
As a means of testing the robustness of the CS-ARDL results, the study followed similar approaches adopted in recent literature (Wang 2007 ; Sharif et al. 2022 ) by using models that could account for cross-sectional dependence in the long run. In doing so, AMG and CCEMG estimation were applied to the variables under consideration. Results for both these tests are reported in Table 14 . Upon reflection of the estimates provided in Table 14 , we were able to establish that the signs for all coefficients for both the AMG and CCEMG for the respective control variables, were relatively consistent with those reported in the CS-ARDL estimations. The results of AMG and CCEMG for the interacted variables (i.e., the interaction between industrialization and ICT, renewable energy sources and ICT, institutional quality and ICT) are consistent with the long run results of CS-ARDL.
Policy implications
Based on the results, the policymakers and governments should utilize the levels of ICT development to gain insights into the risks associated with environmental degradation and promote sustainable values for environmental sustainability in BRICS. Governments should invest in BRICS's ICT, renewable energy sources, industrial and institutions to promote environmental sustainability. This can be achieved by enhancing positive behaviours and modifying behaviour in BRICS countries. In order to encourage and support BRICS countries to actively participate in the fight against environmental degradation/global warming, one of the challenges they may face is accessing the global ICT market.
To effectively reduce CO 2 emissions through the use of renewable energy sources, it is imperative that BRICS countries prioritize and enhance the integration of ICT in the production processes of renewable energy products. Given the budgetary constraints faced by these nations, it is recommended that investments and resource allocations in the ICT and renewable energy sectors be pursued in collaboration with the private sector. Furthermore, BRICS governments should develop and implement comprehensive policies for ICT and renewable energy, outlining specific objectives and providing financial incentives for investments in these areas. This strategic approach will not only elevate the development of ICT and increase the share of renewable energy in the energy mix but will also contribute significantly to the achievement of Sustainable Development Goals 7 (Affordable and Clean Energy) and 9 (Industry, Innovation, and Infrastructure), thereby promoting environmental sustainability and fostering innovation within the BRICS nations.
The detrimental effect of the interplay between ICT and institutional quality on CO 2 emissions highlights the need for policymakers to concentrate on bolstering institutions through pertinent policies. Such actions would aid in the adoption of technologies, ultimately paving the way for long-term environmental sustainability. Potential institutional policies to consider include enhancing transparency and accountability, improving regulatory frameworks, fostering public–private partnerships, and investing in education and training to build capacity for technology adoption. Furthermore, policies that strengthens institutions that facilitate technological property right protections in the renewable energy sources portfolio will be crucial for reducing CO 2 emissions since it facilitates technological innovation.
Based on the CS-ARDL estimates, this study recommends that BRICS governments and policymakers can achieve environmental sustainability more quickly in the short term by utilizing ICT in conjunction with levels of industrialization and institutional quality. The core theoretical contribution of this study is that ICT could acts as an information sharing mechanism if proper measures are put in place by promoting industrialization and institutional quality in curbing CO 2 emissions. The managerial implications of this study within the context of the industrial, renewable and institutional sectors are as follows, firstly, managers in the industrial sector can integrate ICT in the production process to reduce energy consumption, optimize resource usage, and minimize waste generation. By using ICT services/infrastructures industrial processes can be made more efficient, thereby reducing their carbon emissions. Secondly, managers in the institutional sector can use ICT to improve communication and collaboration among different levels of government and agencies. This can facilitate the implementation of policies and programs that promote environmental sustainability, such as the use of renewable energy sources, waste reduction, and conservation. In addition, the use of ICT can provide innovative solutions to help managers in these sectors reduce their negative environmental impact while improving their operations and profitability in BRICS economies.
This study advises caution in the long term as the interaction between ICT and renewable energy sources, industrialization, and institutional quality may not favour environmental quality. Although the renewable energy sources interaction with ICT may not yield immediate progress, strong measures need to be taken to ensure that short-term gains are not nullified. In conclusion, the study highlights the potential of ICT, renewable energy sources, industrialization, and institutional quality in achieving environmental sustainability in the BRICS countries, while recommending cautious measures in the long run to safeguard the progress made.
As BRICS countries—Brazil, Russia, India, China, and South Africa—strive to achieve the United Nations Sustainable Development Goal 13, which is climate change mitigation, there is a growing need to harness ICT, renewable energy sources, levels of industrialization and institutional quality to achieve this goal. Therefore, it is crucial to explore the potential role that ICT development, renewable energy sources, industrialization, and institutional quality can play in promoting environmental sustainability in BRICS economy. The study covers the period from 2000 to 2021 and utilized a range of innovative econometric techniques, which are outlined in the third section of this study. This research is unique compared to previous literature because it examines the impact of the interaction between ICT and industrialization, ICT and renewable energy sources, ICT and institutional quality on CO 2 emissions. This is because globally, regionally and economic bloc wise, the use of ICT services and infrastructures has permeated all sectors of the economy. Consequently, formulating integrated policies that are informed by the findings of this study has become critical in the BRICS context.
This study contributes to the literature by providing the following results. Firstly, a long-run equilibrium relationship was established among the variables, while the causality results reveal that there is a two-way causality between GDP per capita and CO 2 emissions. While unidirectional causality runs from: CO 2 emissions to industrialization; ICT to CO 2 emissions; and renewable energy sources to CO 2 emissions. Secondly, for the long run coefficient estimates, the results from the FMOLS and the DOLS reveal that ICT and industrialization, ICT and institutional quality significantly contribute to the reduction of CO 2 emissions.
Thirdly, In Model 1, CS-ARDL results show a positive and significant impact of GDP per capita and industrialization on CO 2 emissions, a negative and insignificant impact of institutional quality on CO 2 emissions, and a positive and insignificant impact of ICT on CO 2 emissions. In the remaining Models, CS-ARDL short run results indicate that the interaction between industrialization and ICT has a significant and negative impact on CO 2 emissions, the interaction between renewable energy sources and ICT has a significant and positive impact on CO 2 emissions, and the interaction between institutional quality and ICT has a significant and negative impact on CO 2 emissions. In the long run, the interaction between industrialization and ICT has an insignificant and negative impact on CO 2 emissions, the interaction between renewable energy sources and ICT has a significant and positive impact on CO 2 emissions, and the interaction between institutional quality and ICT has an insignificant and negative impact on CO 2 emissions. Lastly, our research is crucial for the strategic development of climate change policies designed to lessen the effects of climate change in BRICS, because ICT development interaction with renewable energy sources, industrialization and institutional quality must be taken seriously to ensure environmental sustainability.
Our study focuses on BRICS economies and utilizes a panel dataset covering the period from 2000 to 2021, primarily due to data limitations. Future research endeavors incorporating more extensive data that includes recently added countries to the BRICS and other regions, such as Europe & Central Asian (ECA), East & South Asia and the Pacific (ESAP), and the Americas, would provide valuable insights for governments and policymakers on leveraging ICT development, renewable energy, agriculture, industry, and institutional sectors to promote environmental sustainability. This is especially relevant as governments in these regions confront the challenge of achieving Sustainable Development Goal 13. Furthermore, future research should examine whether the established conclusions in this study withstand empirical scrutiny within country-specific or other economic bloc settings to enhance the current understanding of this study's research topic. This is essential for more country-specific policy implications.
Data availability
All data generated or analysed during this study are not included in this submission but can be made available upon reasonable request.
Due to the governing rules, it is important for the reader to take note that we did not log variables with negative values.
Studies that have used these techniques include those of Saba & Ngepah ( 2019a , 2019b , 2019c , 2019d , 2022a, 2022b, 2022c, 2022d, 2023), Saba ( 2020a , 2020b , 2020c , 2021a , 2021b , 2023a , 2023b ), Saba et al. ( 2023a , 2023b , 2024a, 2024b), Ngepah et al. ( 2024 ), Saba and Biyase ( 2022 ) and Saba and Pretorius ( 2024 ).
Abid M (2017) Does economic, financial and institutional developments matter for environmental quality? A comparative analysis of EU and MEA countries. J Environ Manage 188:183–194
Article Google Scholar
Acheampong AO (2018) Economic growth, CO2 emissions and energy consumption: what causes what and where? Energy Economics 74:677–692
Acheampong AO, Dzator J, Savage DA (2021) Renewable energy, CO2 emissions and economic growth in sub-Saharan Africa: does institutional quality matter? J Policy Model 43(5):1070–1093
Achuo ED, Miamo CW, Nchofoung TN (2022) Energy consumption and environmental sustainability: What lessons for posterity? Energy Rep 8:12491–12502
Adamides G, Kalatzis N, Stylianou A, Marianos N, Chatzipapadopoulos F, Giannakopoulou M ... Neocleous D (2020) Smart farming techniques for climate change adaptation in Cyprus. Atmosphere, 11(6), 557
Adebayo TS (2020) Revisiting the EKC hypothesis in an emerging market: an application of ARDL-based bounds and wavelet coherence approaches. SN Appl Sci 2(12):1945
Adebayo TS, Beton Kalmaz D (2021) Determinants of CO 2 emissions: empirical evidence from Egypt. Environ Ecol Stat 28:239–262
Article CAS Google Scholar
African Development Bank (AfDB) (2021). Leveraging ICT for climate resilience in Africa. Accessed on 07 th March 2023. Available at : https://www.afdb.org/sites/default/files/documents/publications/leveraging-ict-for-climate-resilience-in-africa.pdf
Agarwal, P., & Alam, M. A. (2018). Use of ICT for sustainable transportation. In IOP Conference Series: Earth and Environmental Science (Vol. 150, No. 1, p. 012032). IOP Publishing
Ahmed Z, Le HP (2021) Linking Information Communication Technology, trade globalization index, and CO 2 emissions: evidence from advanced panel techniques. Environ Sci Pollut Res 28:8770–8781
Amari M, Mouakhar K, Jarboui A (2022) ICT development, governance quality and the environmental performance: Avoidable thresholds from the lower and lower-middle-income countries. Manage Environ Qual Intl J 33(2):125–140
Anwar N, Elfaki KE (2021) Examining the Relationship Between Energy Consumption, Economic Growth, and Environmental Degradation in Indonesia: Do Capital and Trade Openness Matter?. Intl J Renew Energy Dev, 10(4)
Apergis N, Payne JE (2014) Renewable energy, output, CO2 emissions, and fossil fuel prices in Central America: Evidence from a nonlinear panel smooth transition vector error correction model. Energy Economics 42:226–232
Apergis N, Kuziboev B, Abdullaev I, Rajabov A (2023) Investigating the association among CO2 emissions, renewable and non-renewable energy consumption in Uzbekistan: an ARDL approach. Environ Sci Pollut Res, 1–14
Aslam B, Hu J, Majeed MT, Andlib Z, Ullah S (2021) Asymmetric macroeconomic determinants of CO2 emission in China and policy approaches. Environ Sci Pollut Res 28(31):41923–41936
Asongu SA, Agboola MO, Alola AA, Bekun FV (2020) The criticality of growth, urbanization, electricity and fossil fuel consumption to environment sustainability in Africa. Sci Total Environ 712:136376
Asumadu-Sarkodie S, Owusu PA (2016) Carbon dioxide emission, electricity consumption, industrialization, and economic growth nexus: The Beninese case. Energy Sources Part B 11(11):1089–1096
Asumadu-Sarkodie S, Owusu PA (2017) The causal effect of carbon dioxide emissions, electricity consumption, economic growth, and industrialization in Sierra Leone. Energy Sources Part B 12(1):32–39
Avom D, Nkengfack H, Fotio HK, Totouom A (2020) ICT and environmental quality in Sub-Saharan Africa: Effects and transmission channels. Technol Forecast Soc Chang 155:120028
Azam A, Rafiq M, Shafique M, Yuan J (2021a) An empirical analysis of the non-linear effects of natural gas, nuclear energy, renewable energy and ICT-Trade in leading CO2 emitter countries: Policy towards CO2 mitigation and economic sustainability. J Environ Manage 286:112232
Azam A, Rafiq M, Shafique M, Yuan J (2022) Towards achieving environmental sustainability: the role of nuclear energy, renewable energy, and ICT in the top-five carbon emitting countries. Front Energy Res 9:971
Azam M, Liu L, Ahmad N (2021b) Impact of institutional quality on environment and energy consumption: evidence from developing world. Environ Dev Sustain 23:1646–1667
Baloch MA, Wang B (2019) Analyzing the role of governance in CO2 emissions mitigation: the BRICS experience. Struct Chang Econ Dyn 51:119–125
Bano S, Liu L, Khan A (2022) Dynamic influence of aging, industrial innovations, and ICT on tourism development and renewable energy consumption in BRICS economies. Renewable Energy 192:431–442
Batool Z, Raza SMF, Ali S, Abidin SZU (2022) ICT, renewable energy, financial development, and CO2 emissions in developing countries of East and South Asia. Environ Sci Pollut Res 29(23):35025–35035
Bekun FV, Alola AA, Sarkodie SA (2019) Toward a sustainable environment: Nexus between CO2 emissions, resource rent, renewable and nonrenewable energy in 16-EU countries. Sci Total Environ 657:1023–1029
Ben Lahouel B, Taleb L, Managi S, Guesmi K (2022) The threshold effects of ICT on CO2 emissions: evidence from the MENA countries. Environ Econ Policy Stud, 1–21
Boontome P, Therdyothin A, Chontanawat J (2017) Investigating the causal relationship between non-renewable and renewable energy consumption, CO2 emissions and economic growth in Thailand. Energy Procedia 138:925–930
Boukhelkhal A (2022) Energy use, economic growth and CO2 emissions in Africa: does the environmental Kuznets curve hypothesis exist? New evidence from heterogeneous panel under cross-sectional dependence. Environ Dev Sustain 24(11):13083–13110
Breusch TS, Pagan AR (1980) The Lagrange multiplier test and its applications to model specification in econometrics. Rev Econ Stud 47(1):239–253
Cacciotti R, Kaiser A, Sardella A, De Nuntiis P, Drdácký M, Hanus C, Bonazza A (2021) Climate change-induced disasters and cultural heritage: Optimizing management strategies in Central Europe. Clim Risk Manag 32:100301
Cansino JM, Román-Collado R, Molina JC (2019) Quality of institutions, technological progress, and pollution havens in Latin America. An analysis of the environmental Kuznets curve hypothesis. Sustainability, 11(13), 3708
Chang L, Taghizadeh-Hesary F, Saydaliev HB (2022) How do ICT and renewable energy impact sustainable development? Renewable Energy 199:123–131
Chatti W (2021) Moving towards environmental sustainability: information and communication technology (ICT), freight transport, and CO2 emissions. Heliyon 7(10):e08190
Chi F, Meng Z (2022) The effects of ICT and FDI on CO2 emissions in China. Environ Sci Pollut Res, 1–13
Chien F, Anwar A, Hsu CC, Sharif A, Razzaq A, Sinha A (2021) The role of information and communication technology in encountering environmental degradation: proposing an SDG framework for the BRICS countries. Technol Soc 65:101587
Chudik A, Pesaran MH (2015) Common correlated effects estimation of heterogeneous dynamic panel data models with weakly exogenous regressors. J Econometr 188(2):393–420
Cobb CW, Douglas PH (1928) A theory of production. Am Econ Rev Rev 18:139–165
Google Scholar
Copeland BR, Taylor MS (2017) North-South trade and the environment. In International Trade and the Environment (pp. 205–238). Routledge
Danish, Khan N, Baloch MA, Saud S, Fatima T (2018) The effect of ICT on CO2 emissions in emerging economies: does the level of income matters?. Environ Sci Pollut Res, 25, 22850-22860
Danish, Khan N, Baloch MA, Saud S, Fatima T (2018) The effect of ICT on CO 2 emissions in emerging economies: does the level of income matters?. Environ Sci Pollut Re, 25, 22850-22860 22860
Dedrick J, Kraemer KL, Shih E (2013) Information technology and productivity in developed and developing countries. J Manag Inf Syst 30(1):97–122
Dey K, Dubey AK, Sharma S (2023) Can renewable energy drive industrial growth in developing economies? Evidence from India. Int J Energy Sect Manage 17(5):950–971
Dumitrescu EI, Hurlin C (2012) Testing for Granger non-causality in heterogeneous panels. Econ Model 29(4):1450–1460
Ehigiamusoe KU (2023) The drivers of environmental degradation in ASEAN+ China: Do financial development and urbanization have any moderating effect? Singapore Econ Rev 68(05):1671–1714
Elfaki KE, Khan Z, Kirikkaleli D, Khan N (2022) On the nexus between industrialization and carbon emissions: evidence from ASEAN+ 3 economies. Environ Sci Pollut Res 29(21):31476–31485
Esso LJ, Keho Y (2016) Energy consumption, economic growth and carbon emissions: Cointegration and causality evidence from selected African countries. Energy 114:492–497
Faisal F, Tursoy T, Pervaiz R (2020) Does ICT lessen CO2 emissions for fast-emerging economies? An application of the heterogeneous panel estimations. Environ Sci Pollut Res 27(10):10778–10789
Godil DI, Sharif A, Agha H, Jermsittiparsert K (2020) The dynamic nonlinear influence of ICT, financial development, and institutional quality on CO2 emission in Pakistan: new insights from QARDL approach. Environ Sci Pollut Res 27:24190–24200
Godil DI, Yu Z, Sharif A, Usman R, Khan SAR (2021) Investigate the role of technology innovation and renewable energy in reducing transport sector CO2 emission in China: a path toward sustainable development. Sustain Dev 29(4):694–707
Goel RK, Herrala R, Mazhar U (2013) Institutional quality and environmental pollution: MENA countries versus the rest of the world. Econ Syst 37(4):508–521
Goldemberg J, Lucon O (2009) Energy, environment and development. Routledge
Book Google Scholar
Gössling S (2018) ICT and transport behavior: A conceptual review. Int J Sustain Transp 12(3):153–164
Grossman GM, Krueger AB (1991) Environmental impacts of a North American free trade agreement. NBER Working Paper No. 3914. Access on 12/11/2023, available at: https://www.nber.org/system/files/working_papers/w3914/w3914.pdf
Gyamfi BA, Ampomah AB, Bekun FV, Asongu SA (2022) Can information and communication technology and institutional quality help mitigate climate change in E7 economies? An environmental Kuznets curve extension. J Econ Struct 11(1):1–20
Gyamfi S, Zhou Y (2020) Does institutional quality matter for environmental sustainability? Evidence from African countries. Environ Sci Pollut Res 27(22):27232–27243. https://doi.org/10.1007/s11356-020-09379-5
Haldar A, Sethi N (2021) Effect of institutional quality and renewable energy consumption on CO2 emissions− an empirical investigation for developing countries. Environ Sci Pollut Res 28(12):15485–15503
Haseeb A, Xia E, Saud S, Ahmad A, Khurshid H (2019) Does information and communication technologies improve environmental quality in the era of globalization? An empirical analysis. Environ Sci Pollut Res 26:8594–8608
He J, Iqbal W, Su F (2023) Nexus between renewable energy investment, green finance, and sustainable development: Role of industrial structure and technical innovations. Renewable Energy 210:715–724
Higón DA, Gholami R, Shirazi F (2017) ICT and environmental sustainability: A global perspective. Telematics Inform 34(4):85–95
Holden E, Linnerud K, Banister D (2014) Sustainable development: Our common future revisited. Glob Environ Chang 26:130–139
Hussain J, Zhou K (2022) Globalization, industrialization, and urbanization in Belt and Road Initiative countries: implications for environmental sustainability and energy demand. Environ Sci Pollut Res 29(53):80549–80567
Hussain M, Dogan E (2021) The role of institutional quality and environment-related technologies in environmental degradation for BRICS. J Clean Prod 304:127059
Huxster JK, Uribe-Zarain X, Kempton W (2015) Undergraduate understanding of climate change: The influences of college major and environmental group membership on survey knowledge scores. J Environ Educ 46(3):149–165
Ibrahim KM, Waziri SI (2020) Improving ICT and renewable energy for environmental sustainability in sub-Saharan Africa. J Res Emerg Markets 2(3):82–90
Ike GN, Usman O, Alola AA, Sarkodie SA (2020) Environmental quality effects of income, energy prices and trade: the role of renewable energy consumption in G-7 countries. Sci Total Environ 721:137813
Im KS, Pesaran MH, Shin Y (2003) Testing for unit roots in heterogeneous panels. J Econometr 115(1):53–74
Intergovernmental Panel on Climate Change (IPCC) (2021). Climate Change 2021: The Physical Science Basis. Cambridge University Press. Accessed on 24/10/2023, available at: https://reliefweb.int/report/world/climate-change-2021-physical-science-basis?gad_source=1&gclid=CjwKCAjwtqmwBhBVEiwAL-WAYRg5l5-hpU_DBAYXyB4r-tmZUGK1THyQD9XYg88Tse4SyD-THvxpshoCvZoQAvD_BwE
International Energy Agency (IEA) (2020). Digitalization and energy. Accessed on 07 th March 2023 Available at: https://www.iea.org/reports/digitalization-and-energy
International Energy Agency (IEA) (2022). Africa Energy Outlook 2022. Access on 3 rd of March 2023. Available at: https://iea.blob.core.windows.net/assets/27f568cc-1f9e-4c5b-9b09-b18a55fc850b/AfricaEnergyOutlook2022.pdf
International Renewable Energy Agency (IRENA) (2020). Renewable Energy and Jobs: Annual Review 2020. IRENA. Accessed on 24/10/2023, available at: https://www.irena.org/-/media/Files/IRENA/Agency/Publication/2020/Sep/IRENA_RE_Jobs_2020.pdf?rev=db153791a7744a33913b553e02a1e5b0
Iorember PT, Goshit GG, Dabwor DT (2020) Testing the nexus between renewable energy consumption and environmental quality in Nigeria: The role of broad-based financial development. Afr Dev Rev 32(2):163–175
Islam MM, Khan MK, Tareque M, Jehan N, Dagar V (2021) Impact of globalization, foreign direct investment, and energy consumption on CO2 emissions in Bangladesh: Does institutional quality matter? Environ Sci Pollut Res 28(35):48851–48871
Jacobson MZ, Delucchi MA, Cameron MA, Mathiesen BV (2018) Matching demand with supply at low cost in 139 countries among 20 world regions with 100% intermittent wind, water, and sunlight (WWS) for all purposes. Renewable Energy 123:236–248
Jahanger A, Usman M, Ahmad P (2022) Investigating the effects of natural resources and institutional quality on CO2 emissions during globalization mode in developing countries. Intl J Environ Sci Technol, 1–20
Jebli MB, Youssef SB (2017) The role of renewable energy and agriculture in reducing CO2 emissions: Evidence for North Africa countries. Ecol Ind 74:295–301
Jebli MB, Farhani S, Guesmi K (2020) Renewable energy, CO2 emissions and value added: Empirical evidence from countries with different income levels. Struct Chang Econ Dyn 53:402–410
Jiang Q, Rahman ZU, Zhang X, Guo Z, Xie Q (2022) An assessment of the impact of natural resources, energy, institutional quality, and financial development on CO2 emissions: Evidence from the B&R nations. Resour Policy 76:102716
Kahouli B, Miled K, Aloui Z (2022) Do energy consumption, urbanization, and industrialization play a role in environmental degradation in the case of Saudi Arabia? Energ Strat Rev 40:100814
Karim S, Appiah M, Naeem MA, Lucey BM, Li M (2022) Modelling the role of institutional quality on carbon emissions in Sub-Saharan African countries. Renew Energy 198:213–221
Khan FN, Sana A, Arif U (2020) Information and communication technology (ICT) and environmental sustainability: a panel data analysis. Environ Sci Pollut Res 27:36718–36731
Khan H, Weili L, Khan I (2022a) Examining the effect of information and communication technology, innovations, and renewable energy consumption on CO2 emission: evidence from BRICS countries. Environ Sci Pollut Res 29(31):47696–47712
Khan Y, Oubaih H, Elgourrami FZ (2022b) The effect of renewable energy sources on carbon dioxide emissions: Evaluating the role of governance, and ICT in Morocco. Renewable Energy 190:752–763
Kumar CR, Majid MA (2022) Renewable energy for sustainable development in India: Current status, future prospects, challenges, employment, and investment opportunities. TIDEE: TERI Information Digest on Energy and Environment, 21(1), 33–33
Kuznets S (1955) Economic growth and income inequality. Am Econ Rev 45(1):1–28
Lahouel BB, Taleb L, Zaied YB, Managi S (2021) Does ICT change the relationship between total factor productivity and CO2 emissions? Evidence based on a nonlinear model. Energy Economics 101:105406
Le HP, Ozturk I (2020) The impacts of globalization, financial development, government expenditures, and institutional quality on CO 2 emissions in the presence of environmental Kuznets curve. Environ Sci Pollut Res 27:22680–22697
Lee CT, Hashim H, Ho CS, Van Fan Y, Klemeš JJ (2017) Sustaining the low-carbon emission development in Asia and beyond: Sustainable energy, water, transportation and low-carbon emission technology. J Clean Prod 146:1–13
Levin A, Lin CF, Chu CSJ (2002) Unit root tests in panel data: asymptotic and finite-sample properties. J Econometr 108(1):1–24
Li K, Lin B (2015) Impacts of urbanization and industrialization on energy consumption/CO2 emissions: does the level of development matter? Renew Sustain Energy Rev 52:1107–1122
Lin B, Zhu J (2017) Energy and carbon intensity in China during the urbanization and industrialization process: A panel VAR approach. J Clean Prod 168:780–790
Lin B, Omoju OE, Okonkwo JU (2015) Impact of industrialisation on CO2 emissions in Nigeria. Renew Sustain Energy Rev 52:1228–1239
Liu K, Deng B, Shen Q, Yang J, Li Y (2022) Optimization based on genetic algorithms on energy conservation potential of a high speed SI engine fueled with butanol–gasoline blends. Energy Rep 8:69–80
Lu WC (2017) Renewable energy, carbon emissions, and economic growth in 24 Asian countries: evidence from panel cointegration analysis. Environ Sci Pollut Res 24:26006–26015
Lu WC (2018) The impacts of information and communication technology, energy consumption, financial development, and economic growth on carbon dioxide emissions in 12 Asian countries. Mitig Adapt Strat Glob Change 23:1351–1365
Mahjabeen N, Shah SZ, Chughtai S, Simonetti B (2020) Renewable energy, institutional stability, environment and economic growth nexus of D-8 countries. Energ Strat Rev 29:100484
Md Hassan AA (2015) Role of ICT in natural disaster management of Bangladesh (Doctoral dissertation, BRAC University). Accessed on 07 th March 2023. Available at https://dspace.bracu.ac.bd/xmlui/bitstream/handle/10361/4485/14168008.pdf?sequence=1&isAllowed=y
Meena MS, Singh KM, Singh R (2012) ICT-Enabled Extension in Agriculture Sector: Opportunities and Challenges in Climate Change Situation. ICTs for agricultural development under changing climate, KM Singh, MS Meena, eds., Narendra Publishing House. Accessed on 07 th March 2023. Available at SSRN: https://ssrn.com/abstract=2027803
Mehrjo A, Satari Yuzbashkandi S, Eskandari Nasab MH, Gudarzipor H (2022) Economic complexity, ICT, biomass energy consumption, and environmental degradation: evidence from Iran. Environ Sci Pollut Res 29(46):69888–69902
Mentel G, Tarczyński W, Dylewski M, Salahodjaev R (2022) Does renewable energy sector affect industrialization-CO2 emissions nexus in Europe and Central Asia? Energies 15(16):5877
Mirza FM, Ansar S, Ullah K, Maqsood F (2020) The impact of information and communication technologies, CO2 emissions, and energy consumption on inclusive development in developing countries. Environ Sci Pollut Res 27:3143–3155
Mol AP, Spaargaren G (2000) Ecological modernisation theory in debate: A review. Environ Politics 9(1):17–49
Mrabet Z, Alsamara M, Mimouni K, Mnasri A (2021) Can human development and political stability improve environmental quality? New evidence from the MENA region. Econ Model 94:28–44
Mubiru J, Bahaj AS, James PAB (2019) Life cycle assessment of wind energy systems in South Africa. Renewable Energy 130:53–61. https://doi.org/10.1016/j.renene.2018.06.103
N’dri LM, Islam M, Kakinaka M (2021) ICT and environmental sustainability: any differences in developing countries?. J Clean Prod, 297, p.126642
Nasir MA, Canh NP, Le TNL (2021) Environmental degradation & role of financialisation, economic development, industrialisation and trade liberalisation. J Environ Manage 277:111471
Nasreen S, Anwar S, Ozturk I (2017) Financial stability, energy consumption and environmental quality: Evidence from South Asian economies. Renew Sustain Energy Rev 67:1105–1122
Naudé W (2011) Climate Change and Industrial Policy. Sustainability 3(7):1003–1021
Nazir U (2023) The winds of change are blowing: globalization's impact on renewable energy and environmental challenges. Nazir, Umar.(2023). The Winds of Change are Blowing: Globalization's Impact on Renewable Energy and Environmental Challenges. Archives of the Social Sciences: A Journal of Collaborative Memory, 2(1), 78–93
Ngepah N, Saba CS, Kajewole DO (2024) The impact of industry 4.0 on South Africa’s manufacturing sector. Journal of Open Innovation: Technology, Market, and Complexity, 10(1), 100226
Nivedha R, Sutha DAI (2020) The challenges of electronic waste (e-waste) management in India. Eur J Mol Clin Med 7(3):4583–4588
Nnorom IC, Osibanjo O (2008) Electronic waste (e-waste): Material flows and management practices in Nigeria. Waste Manage 28(8):1472–1479
Olaniran OJ, Adeyemo RO (2020) Institutional quality and environmental sustainability in Nigeria: Empirical analysis. Environ Sci Pollut Res 27(28):35501–35513. https://doi.org/10.1007/s11356-020-10003-9
Opoku EEO, Aluko OA (2021) Heterogeneous effects of industrialization on the environment: Evidence from panel quantile regression. Struct Chang Econ Dyn 59:174–184
Ozcan B, Apergis N (2018) The impact of internet use on air pollution: Evidence from emerging countries. Environ Sci Pollut Res 25:4174–4189
Park Y, Meng F, Baloch MA (2018) The effect of ICT, financial development, growth, and trade openness on CO 2 emissions: an empirical analysis. Environ Sci Pollut Res 25:30708–30719
Pedroni P (2001) Purchasing power parity tests in cointegrated panels. Rev Econ Stat 83(4):727–731
Pedroni P (2004) Panel cointegration: asymptotic and finite sample properties of pooled time series tests with an application to the PPP hypothesis. Economet Theor 20(3):597–625
Pénard T, Poussing N, Zomo Yebe G, Ella N (2012) Comparing the determinants of internet and cell phone use in Africa: evidence from Gabon. Commun Strateg 86:65–83
Pesaran MH (2004) General diagnostic tests for cross section dependence in panels. Cambridge Working Papers. Economics, 1240(1), 1
Pesaran MH (2007) A simple panel unit root test in the presence of cross-section dependence. J Appl Economet 22(2):265–312
Pesaran MH, Yamagata T (2008) Testing slope homogeneity in large panels. J Econometr 142(1):50–93
Phiri J, Malec K, Kapuka A, Maitah M, Appiah-Kubi SNK, Gebeltová Z, Bowa M, Maitah K (2021) Impact of agriculture and energy on co2 emissions in Zambia. Energies 14(24):8339
Qureshi S (2019) Climate change adaptation for sustainable development: The information and communication technology (ICT) paradox. Inf Technol Dev 25(4):625–629
Radmehr R, Henneberry SR, Shayanmehr S (2021) Renewable energy consumption, CO2 emissions, and economic growth nexus: a simultaneity spatial modeling analysis of EU countries. Struct Chang Econ Dyn 57:13–27
Rafiq S, Salim R, Apergis N (2016) Agriculture, trade openness and emissions: an empirical analysis and policy options. Austr J Agric Resour Econ 60(3):348–365
Raheem ID, Tiwari AK, Balsalobre-Lorente D (2020) The role of ICT and financial development in CO2 emissions and economic growth. Environ Sci Pollut Res 27(2):1912–1922
Rahman MM, Alam K (2022) Impact of industrialization and non-renewable energy on environmental pollution in Australia: do renewable energy and financial development play a mitigating role? Renewable Energy 195:203–213
Raihan A, Tuspekova A (2022a) The nexus between economic growth, renewable energy use, agricultural land expansion, and carbon emissions: new insights from Peru. Energy Nexus 6:100067
Raihan A, Tuspekova A (2022b) Nexus between economic growth, energy use, agricultural productivity, and carbon dioxide emissions: new evidence from Nepal. Energy Nexus 7:100113
Raihan A, Tuspekova A (2022c) Nexus between emission reduction factors and anthropogenic carbon emissions in India. Anthropocene Sci 1(2):295–310
Raihan A, Tuspekova A (2022d) Dynamic impacts of economic growth, energy use, urbanization, tourism, agricultural value-added, and forested area on carbon dioxide emissions in Brazil. J Environ Stud Sci 12(4):794–814
Rapier R (2020) Fossil Fuels Still Supply 84 Percent of World Energy — and Other Eye Openers from BP’s Annual Review. Forbes. https://www.forbes.com/sites/rrapier /2020/06/20/bp-review-new-highs-in-global-energy-consumption-and-carbon-em issions-in-2019/?sh=408de9d766a1
Riti JS, Shu Y, Kamah M (2021) Institutional quality and environmental sustainability: The role of freedom of press in most freedom of press countries. Environ Impact Assess Rev 91:106656
Saba CS, Ngepah N (2019a) Military expenditure and economic growth: evidence from a heterogeneous panel of African countries. Econ Res-Ekonomska Istraživanja 32(1):3586–3606
Saba CS, Ngepah N (2022a) Convergence in renewable energy sources and the dynamics of their determinants: An insight from a club clustering algorithm. Energy Rep 78:116–125. https://doi.org/10.1016/j.egyr.2022.01.190
Saba CS, Ngepah N (2022b) Convergence in renewable energy consumption and their influencing factors across regions: evidence from convergence algorithm approach. Environ Sci Pollut Res. https://doi.org/10.1007/s11356-022-19731-9
Saba CS, Ngepah N (2022d) Nexus between telecommunication infrastructures, defence and economic growth: a global evidence. NETNOMICS: Economic Research and Electronic Networking, https://doi.org/10.1007/s11066-022-09151-9
Saba CS (2020a) Security as an outcome for promoting economic prosperity in the regional economic communities of Africa: Evidence from a panel data analysis. Afr Secur Rev, 29(4), 376–400. Available at: https://doi.org/10.1080/10246029.2020.1835681
Saba CS (2020b) Military expenditure and security outcome convergence in Africa: an application of club clustering approach. African Security. available at: https://doi.org/10.1080/19392206.2020.1808418
Saba CS (2020c) Convergence or Divergence Patterns in Global Defence Spending: Further Evidence from a Nonlinear Single Factor Model. Peace Economics, Peace Science and Public Policy, available at DOI https://doi.org/10.1515/peps-2020-0012
Saba CS (2021a) Convergence and transition paths in transportation: fresh insights from a club clustering algorithm. Transp Policy 112:80–93. https://doi.org/10.1016/j.tranpol.2021.08.008
Saba CS (2021b) Defence spending and economic growth in South Africa: evidence from cointegration and co-feature analysis. Peace Econ Peace Sci Public Policy 28(1):51–100. https://doi.org/10.1515/peps-2021-0017
Saba CS (2023a) Nexus between CO 2 emissions, renewable energy consumption, militarisation, and economic growth in South Africa: evidence from using novel dynamic ARDL simulations. Renewable Energy 205:349–365
Saba CS (2023b) CO 2 emissions-energy consumption-militarisation-growth nexus in South Africa: evidence from novel dynamic ARDL simulations. Environ Sci Pollut Res 30(7):18123–18155
Saba CS, Biyase M (2022) Determinants of renewable electricity development in Europe: Do Governance indicators and institutional quality matter? Energy Rep 8:13914–13938
Saba CS, Monkam N (2024) Leveraging the potential of artificial intelligence (AI) in exploring the interplay among tax revenue, institutional quality, and economic growth in the G-7 countries. AI & SOCIETY, 1–23
Saba CS, Ngepah N (2019b) Military expenditure and security outcome convergence in African regional economic communities: Evidence from the convergence club algorithm. Peace Econ Peace Sci Public Policy 26(1):1–28
Saba CS, Ngepah N (2019c) Empirical analysis of military expenditure and industrialisation nexus: A regional approach for Africa. Intl Econ J, 34(1), 1–27.
Saba CS, Ngepah N (2019d) A cross-regional analysis of military expenditure, state fragility and economic growth in Africa. Qual Quant 53(6):2885–2915
Saba CS, Ngepah N (2022d) ICT diffusion, industrialisation and economic growth nexus: An international cross-country analysis. J Knowl Econ 13(3):2030–2069
Saba CS, Ngepah N (2023) Empirics of convergence in industrialisation and their determinants: global evidence. Discover Sustainability 4(1):25
Saba CS, Pretorius M (2024) The impact of artificial intelligence (AI) investment on human well-being in G-7 countries: Does the moderating role of governance matter? Sustainable Futures 7:100156
Saba CS, Asongu SA, Ngepah N, Ngoungou YE (2024a) Governance in the exploration of global and regional determinants of ICT development. Intl J Innov Stud 8(2):132–153. https://doi.org/10.1016/j.ijis.2024.02.002
Saba CS, David OO, Voto TP (2024b) Institutional quality effect of ICT penetration: Global and regional perspectives. South African J Econ Manage Sci 27(1):5180
Saba CS, Djemo CRT, Eita JH, Ngepah N (2023a) Towards environmental sustainability path in Africa: The critical role of ICT, renewable energy sources, agriculturalization, industrialization and institutional quality. Energy Rep 10:4025–4050
Saba CS, Ngepah N, Odhiambo NM (2023b) Information and Communication Technology (ICT), Growth and Development in Developing Regions: Evidence from a Comparative Analysis and a New Approach. J Knowl Econ, 1–49. https://doi.org/10.1007/s13132-023-01571-8
Sadiq S (2023) Balancing economic growth with environmental and healthcare considerations: insights from Pakistan’s development trajectory. Research Letters 1(1):17–26
Sahoo M, Gupta M, Srivastava P (2021) Does information and communication technology and financial development lead to environmental sustainability in India? An Empirical Insight. Telem Inform 60:101598
Salahuddin M, Alam K (2015) Internet usage, electricity consumption and economic growth in Australia: A time series evidence. Telematics Inform 32(4):862–878
Salahuddin M, Alam K, Ozturk I (2016) Is rapid growth in Internet usage environmentally sustainable for Australia? An empirical investigation. Environ Sci Pollut Res 23:4700–4713
Salman M, Long X, Dauda L, Mensah CN (2019a) The impact of institutional quality on economic growth and carbon emissions: Evidence from Indonesia, South Korea and Thailand. J Clean Prod 241:118331
Salman M, Long X, Dauda L, Mensah CN, Muhammad S (2019b) Different impacts of export and import on carbon emissions across 7 ASEAN countries: a panel quantile regression approach. Sci Total Environ 686:1019–1029
Sana A, Khan FN, Arif U (2021) ICT diffusion and climate change: The role of economic growth, financial development and trade openness. NETNOMICS: Economic Research and Electronic Networking, 22(2–3), 179–194.
Sebri M, Ben-Salha O (2014) On the causal dynamics between economic growth, renewable energy consumption, CO2 emissions and trade openness: Fresh evidence from BRICS countries. Renew Sustain Energy Rev 39:14–23
Shabani ZD, Shahnazi R (2019) Energy consumption, carbon dioxide emissions, information and communications technology, and gross domestic product in Iranian economic sectors: A panel causality analysis. Energy 169:1064–1078
Shah MI, AbdulKareem HK, Abbas S (2022) Examining the agriculture induced Environmental Kuznets Curve hypothesis in BRICS economies: The role of renewable energy as a moderator. Renewable Energy 198:343–351
Shahabuddin M, Uddin MN, Chowdhury JI, Ahmed SF, Uddin MN, Mofijur M, Uddin MA (2022) A review of the recent development, challenges, and opportunities of electronic waste (e-waste). Intl J Environ Sci Technol, 1–8
Shahbaz M, Uddin GS, Rehman IU, Imran K (2014) Industrialization, electricity consumption and CO2 emissions in Bangladesh. Renew Sustain Energy Rev 31:575–586
Shahnazi R, Dehghan Shabani Z (2019) The effects of spatial spillover information and communications technology on carbon dioxide emissions in Iran. Environ Sci Pollut Res 26:24198–24212
Sharif A, Bhattacharya M, Afshan S, Shahbaz M (2021) Disaggregated renewable energy sources in mitigating CO2 emissions: new evidence from the USA using quantile regressions. Environ Sci Pollut Res 28(41):57582–57601
Sharif A, Saqib N, Dong K, Khan SAR (2022) Nexus between green technology innovation, green financing, and CO 2 emissions in the G7 countries: the moderating role of social globalisation. Sustain Dev 2022(1):1–13
Shobande OA, Ogbeifun L (2022) Has information and communication technology improved environmental quality in the OECD?—a dynamic panel analysis. Int J Sust Dev World 29(1):39–49
Sohag K, Al Mamun M, Uddin GS, Ahmed AM (2017) Sectoral output, energy use, and CO 2 emission in middle-income countries. Environ Sci Pollut Res 24:9754–9764
Sovacool BK, Dworkin MH (2014) Global energy justice. Cambridge University Press
Teng JZ, Khan MK, Khan MI, Chishti MZ, Khan MO (2021) Effect of foreign direct investment on CO 2 emission with the role of globalization, institutional quality with pooled mean group panel ARDL. Environ Sci Pollut Res 28:5271–5282
Titifanue J, Kant R, Finau G, Tarai J (2017) Climate change advocacy in the Pacific: The role of information and communication technologies. Pac Journal Rev 23(1):133–149
Tsimisaraka RSM, Xiang L, Andrianarivo ARNA, Josoa EZ, Khan N, Hanif MS ... Limongi R (2023) Impact of Financial Inclusion, Globalization, Renewable Energy, ICT, and Economic Growth on CO2 Emission in OBOR Countries. Sustainability, 15(8), 6534
United Nations Development Programme and International Finance Corporation (2021). Tech for green: Fostering green entrepreneurship and innovation in Sub-Saharan Africa. Accessed on 07 th March 2023. Available at : https://www.undp.org/publications/tech-green-fostering-green-entrepreneurship-and-innovation-sub-saharan-africa
Usman M, Balsalobre-Lorente D (2022) Environmental concern in the era of industrialization: can financial development, renewable energy and natural resources alleviate some load? Energy Policy 162:112780
Van Hoang TH, Shahzad SJH, Czudaj RL (2020) Renewable energy consumption and industrial production: A disaggregated time-frequency analysis for the US. Energy Economics 85:104433
Voumik LC, Sultana T (2022) Impact of urbanization, industrialization, electrification and renewable energy on the environment in BRICS: fresh evidence from novel CS-ARDL model. Heliyon 8(11):e11457
Wada I, Faizulayev A, Bekun FV (2021) Exploring the role of conventional energy consumption on environmental quality in Brazil: Evidence from cointegration and conditional causality. Gondwana Res 98:244–256
Wang Q, Su M (2019) The effects of urbanization and industrialization on decoupling economic growth from carbon emission–a case study of China. Sustain Cities Soc 51:101758
Wang Q, Su M, Li R (2018) Toward to economic growth without emission growth: The role of urbanization and industrialization in China and India. J Clean Prod 205:499–511
Wang Y (2007) Globalisation enhances cultural identity. Intercultural Commun Stud 16(1):83–86
Warsame AA, Sheik-Ali IA, Mohamed J, Sarkodie SA (2022) Renewables and institutional quality mitigate environmental degradation in Somalia. Renewable Energy 194:1184–1191
Watson RT, Boudreau MC, Chen AJ (2010) Information systems and environmentally sustainable development: energy informatics and new directions for the IS community. MIS quarterly, 23–38
Weili L, Khan H, Khan I, Han L (2022) The impact of information and communication technology, financial development, and energy consumption on carbon dioxide emission: evidence from the Belt and Road countries. Environ Sci Pollut Res, 1–16
Wen Z, Meng F, Chen M (2014) Estimates of the potential for energy conservation and CO2 emissions mitigation based on Asian-Pacific Integrated Model (AIM): the case of the iron and steel industry in China. J Clean Prod 65:120–130
World Bank (2021) Leveraging technology for climate adaptation and resilience in Sub-Saharan Africa. Accessed on 07 th March 2023. Available at : https://openknowledge.worldbank.org/handle/10986/36177
Yang B, Ali M, Hashmi SH, Jahanger A (2022) Do income inequality and institutional quality affect CO2 emissions in developing economies? Environ Sci Pollut Res 29(28):42720–42741
Yap NT (2011) Disaster management, developing country communities & climate change: The role of ICTs. Manchester: Report, eds. R. Heeks and A. Ospina for IDRC, Centre for Development Informatics, Insitute for Development Policy and Management, University of Manchester. Accessed 07 th March 2023. Available at: https://d1wqtxts1xzle7.cloudfront.net/30298266/yapdisastermanagementdevelopmenticts-libre.pdf?1390883515=&response-content-disposition=inline%3B+filename%3DDisaster_management_developing_country_c.pdf&Expires=1678180675&Signature=RFam6DJb7RwwaI8J3-1vfeM7J2n8Omy9778u1PorwBVkIn~Cgf6qgsZYafBdsgWPr5RqeIo2u-kZyhsS-YXiEGxfL9Tjpu0QbPBmphgKW11WxrIPk20hdbgPvFB0uL4Sb9ZfKhmmSNmhtB6CXPRzo0-R~uSXohDma7dan9mtvD1UZAHL5lAtuco5FgOjsHAD7yNYWMNk0fJ1Ml~DsIU78HNi3WwT~M1Bjx3tM8jnIGbtgNdyPQo4ji~RkwfkmKAnvzLifUpL0CiK-WS1uoAZzadc7DyGjKnz7Ub1qc-adQlku-hp2XbNDscA7nK61TPrJ4YUkaQCl0Caic9kE8soiQ__&Key-Pair-Id=APKAJLOHF5GGSLRBV4ZA
You V, Kakinaka M (2022) Modern and traditional renewable energy sources and CO2 emissions in emerging countries. Environ Sci Pollut Res 29(12):17695–17708
Zafar MW, Saleem MM, Destek MA, Caglar AE (2022) The dynamic linkage between remittances, export diversification, education, renewable energy consumption, economic growth, and CO2 emissions in top remittance-receiving countries. Sustain Dev 30(1):165–175
Zakaria M, Bibi S (2019) Financial development and environment in South Asia: the role of institutional quality. Environ Sci Pollut Res 26:7926–7937
Zhang C, Yang Y, Tian X (2019) The impact of institutional quality on carbon emissions in African countries. Environ Sci Pollut Res 26(24):24696–24711. https://doi.org/10.1007/s11356-019-05506-8
Zhou X, Zhang J, Li J (2013) Industrial structural transformation and carbon dioxide emissions in China. Energy Policy 57:43–51
Download references
Acknowledgements
We appreciate the editor(s) and anonymous reviewer(s) for their valuable comments that helped improve the quality of this study. The usual disclaimer applies.
Open access funding provided by University of Johannesburg. The author(s) received no financial support for the research, authorship, and/or publication of this article.
Author information
Authors and affiliations.
School of Economics, College of Business and Economics, University of Johannesburg, Auckland Park Kingsway Campus, PO Box 524, Johannesburg, Auckland Park, South Africa
Charles Shaaba Saba, Charles Raoul Tchuinkam Djemo & Nicholas Ngepah
You can also search for this author in PubMed Google Scholar
Contributions
CSS, CRTD and NN conceptualised the study idea, drafted the paper, collected data, analysed data, wrote the introduction section, organised the literature review, drafted the methodology section, interpreted the results and provided the discussions, concluded the study with policy implications, and organised the reference list. CSS, CRTD and NN equally proofread, edited, and finalised the article.
Corresponding author
Correspondence to Charles Shaaba Saba .
Ethics declarations
Conflict of interest.
The author(s) declared no potential conflict of interest with respect to the research, authorship, and/or publication of this article.
Ethical approval
This article does not contain any studies with human participants or animals performed by the author.
Consent to Participate
Not applicable.
Consent for Publication
This manuscript is an original work produced by the author(s). CSS, CRTD and NN is aware of its content and approves its submission. It is also important to mention that the manuscript has not been published elsewhere in part or in entirety and is not under consideration by another journal. The author(s) has given consent for this article to be submitted for publication in Environmental Science and Pollution Research .
Additional information
Responsible Editor: Roula Inglesi-Lotz
Publisher's Note
Springer Nature remains neutral with regard to jurisdictional claims in published maps and institutional affiliations.
Rights and permissions
Open Access This article is licensed under a Creative Commons Attribution 4.0 International License, which permits use, sharing, adaptation, distribution and reproduction in any medium or format, as long as you give appropriate credit to the original author(s) and the source, provide a link to the Creative Commons licence, and indicate if changes were made. The images or other third party material in this article are included in the article's Creative Commons licence, unless indicated otherwise in a credit line to the material. If material is not included in the article's Creative Commons licence and your intended use is not permitted by statutory regulation or exceeds the permitted use, you will need to obtain permission directly from the copyright holder. To view a copy of this licence, visit http://creativecommons.org/licenses/by/4.0/ .
Reprints and permissions
About this article
Saba, C.S., Djemo, C.R.T. & Ngepah, N. The crucial roles of ICT, renewable energy sources, industrialization, and institutional quality in achieving environmental sustainability in BRICS. Environ Sci Pollut Res (2024). https://doi.org/10.1007/s11356-024-33479-4
Download citation
Received : 05 May 2023
Accepted : 23 April 2024
Published : 08 May 2024
DOI : https://doi.org/10.1007/s11356-024-33479-4
Share this article
Anyone you share the following link with will be able to read this content:
Sorry, a shareable link is not currently available for this article.
Provided by the Springer Nature SharedIt content-sharing initiative
- Information and communications technology (ICT)
- Renewable energy sources
- Industrialization
- Environmental sustainability
- Institutional quality
- CS-ARDL panel analysis
JEL Classification
- Find a journal
- Publish with us
- Track your research

IMAGES
VIDEO
COMMENTS
Simple hypothesis. A simple hypothesis is a statement made to reflect the relation between exactly two variables. One independent and one dependent. Consider the example, "Smoking is a prominent cause of lung cancer." The dependent variable, lung cancer, is dependent on the independent variable, smoking. 4.
A research hypothesis is a statement that proposes a possible explanation for an observable phenomenon or pattern. It guides the direction of a study and predicts the outcome of the investigation. A research hypothesis is testable, i.e., it can be supported or disproven through experimentation or observation. Characteristics of a good hypothesis
Developing a hypothesis (with example) Step 1. Ask a question. Writing a hypothesis begins with a research question that you want to answer. The question should be focused, specific, and researchable within the constraints of your project. Example: Research question.
One of the main differences between scientific hypothesis and review articles relates to the volume of supportive literature sources (Table 1). In fact, hypothesis is usually formulated by referring to a few scientific facts or compelling evidence derived from a handful of literature sources.19 By contrast, reviews require analyses of a large ...
Definition: Hypothesis is an educated guess or proposed explanation for a phenomenon, based on some initial observations or data. It is a tentative statement that can be tested and potentially proven or disproven through further investigation and experimentation. Hypothesis is often used in scientific research to guide the design of experiments ...
A research hypothesis is your proposed answer to your research question. The research hypothesis usually includes an explanation ('x affects y because …'). A statistical hypothesis, on the other hand, is a mathematical statement about a population parameter. Statistical hypotheses always come in pairs: the null and alternative hypotheses.
A hypothesis is a tentative statement about the relationship between two or more variables. It is a specific, testable prediction about what you expect to happen in a study. It is a preliminary answer to your question that helps guide the research process. Consider a study designed to examine the relationship between sleep deprivation and test ...
A research hypothesis (also called a scientific hypothesis) is a statement about the expected outcome of a study (for example, a dissertation or thesis). To constitute a quality hypothesis, the statement needs to have three attributes - specificity, clarity and testability. Let's take a look at these more closely.
A research hypothesis, in its plural form "hypotheses," is a specific, testable prediction about the anticipated results of a study, established at its outset. It is a key component of the scientific method. Hypotheses connect theory to data and guide the research process towards expanding scientific understanding.
Abstract. A hypothesis is a logical construct, interposed between a problem and its solution, which represents a proposed answer to a research question. It gives direction to the investigator's thinking about the problem and, therefore, facilitates a solution. There are three primary modes of inference by which hypotheses are developed ...
A research hypothesis helps test theories. A hypothesis plays a pivotal role in the scientific method by providing a basis for testing existing theories. For example, a hypothesis might test the predictive power of a psychological theory on human behavior. It serves as a great platform for investigation activities.
INTRODUCTION. Scientific research is usually initiated by posing evidenced-based research questions which are then explicitly restated as hypotheses.1,2 The hypotheses provide directions to guide the study, solutions, explanations, and expected results.3,4 Both research questions and hypotheses are essentially formulated based on conventional theories and real-world processes, which allow the ...
An effective hypothesis in research is clearly and concisely written, and any terms or definitions clarified and defined. Specific language must also be used to avoid any generalities or assumptions. Use the following points as a checklist to evaluate the effectiveness of your research hypothesis: Predicts the relationship and outcome.
Formulating Hypotheses for Different Study Designs. Generating a testable working hypothesis is the first step towards conducting original research. Such research may prove or disprove the proposed hypothesis. Case reports, case series, online surveys and other observational studies, clinical trials, and narrative reviews help to generate ...
There are 5 main steps in hypothesis testing: State your research hypothesis as a null hypothesis and alternate hypothesis (H o) and (H a or H 1 ). Collect data in a way designed to test the hypothesis. Perform an appropriate statistical test. Decide whether to reject or fail to reject your null hypothesis. Present the findings in your results ...
The steps to write a research hypothesis are: 1. Stating the problem: Ensure that the hypothesis defines the research problem. 2. Writing a hypothesis as an 'if-then' statement: Include the action and the expected outcome of your study by following a 'if-then' structure. 3.
A research hypothesis is referred to as a scientific hypothesis. This is a clear, specific, and testable statement that predicts the expected result in a scientific study. It is a prediction, reasonable guess, and logical supposition about the relationship between the variables. A research hypothesis is an integral and central part of research ...
Hypothesis is a prediction of the outcome of a study. Hypotheses are drawn from theories and research questions or from direct observations. In fact, a research problem can be formulated as a hypothesis. To test the hypothesis we need to formulate it in terms that can actually be analysed with statistical tools.
CHAPTER - 4. FORMULATING AND T ESTING HYPOTHESIS. Topics Covered. 4.1 Definition of Hypothesis. 4.2 Assumption, Postulate and Hypothesis. 4.3 Nature of Hypothesis. 4.4 Functions/ Roles of ...
Sources of Hypothesis. There are diverse sources of hypothesis in research. First, an explorative research work might lead to the establishment of hypothesis. Second, the environment is a source of hypothesis, because environment portrays broad relationship across factors which form the basis for drawing an inference. Third, analogies are a ...
Back to: Introduction to Educational Research Methodology Concept, Characteristics, Types and Sources of Hypothesis in Research Methodology. A hypothesis is used for explaining a phenomenon. Hypothesis is essential to discover cause-and-effect relationships.
It includes components like variables, population and the relation between the variables. A research hypothesis is a hypothesis that is used to test the relationship between two or more variables. ... Following are the sources of hypothesis: The resemblance between the phenomenon. Observations from past studies, present-day experiences and from ...
Hypothesis in social research refers to a tentative statement or assumption about the relationship between two or more variables. It is a testable prediction that serves as a starting point for conducting a study. Hypotheses are important in social research for several reasons: 1. Direction and focus: Hypotheses provide a clear direction and ...
Research hypothesis. The primary research question should be driven by the hypothesis rather than the data. 1, 2 That is, the research question and hypothesis should be developed before the start of the study. This sounds intuitive; however, if we take, for example, a database of information, it is potentially possible to perform multiple ...
Gr11 Research Task 2024; RM 00 01 Wolfe; ... Evidence of secondary sources: (e a newspaper article) ... Geography Grade 12 Research TASK 2024; Hypothesis on Load Shedding; Geography; Research Gr12 2024-1-1; 2024 Geo Grade 12 Research Task; GR 10 Essay Project Based Learning Teachers Guide; English (ZA) South Africa.
In a hypothesis test, sample data is evaluated in order to arrive at a decision about some type of claim. If certain conditions about the sample are satisfied, then the claim can be evaluated for a population in a hypothesis test, we: a. Evaluate the null hypothesis, typically denoted with H0.The null is not rejected unless the hypothesis test shows otherwise.
In business development, research is a critical component for success. A well-defined hypothesis acts as a compass, guiding you through the complexities of market trends, consumer behavior, and ...
This study delves into the intricate relationship between non-renewable energy sources, economic advancement, and the ecological footprint of well-being in Pakistan, spanning the years from 1980 to 2021. Employing the quantile regression model, we analyzed the co-integrating dynamics among the variables under scrutiny. Non-renewable energy sources were dissected into four distinct components ...
The BRICS countries—Brazil, Russia, India, China, and South Africa—are committed to achieving United Nations Sustainable Development Goal 13, which focuses on mitigating climate change. To attain this goal, it is crucial to emphasize the significance of ICT, renewable energy sources, industrialization, and institutional quality. This study contributes to the literature by examining the ...