Space robotics — Present and past challenges
Ieee account.
- Change Username/Password
- Update Address

Purchase Details
- Payment Options
- Order History
- View Purchased Documents
Profile Information
- Communications Preferences
- Profession and Education
- Technical Interests
- US & Canada: +1 800 678 4333
- Worldwide: +1 732 981 0060
- Contact & Support
- About IEEE Xplore
- Accessibility
- Terms of Use
- Nondiscrimination Policy
- Privacy & Opting Out of Cookies
A not-for-profit organization, IEEE is the world's largest technical professional organization dedicated to advancing technology for the benefit of humanity. © Copyright 2024 IEEE - All rights reserved. Use of this web site signifies your agreement to the terms and conditions.
Space Robotics: A Comprehensive Study of Major Challenges and Proposed Solutions
- Conference paper
- First Online: 06 October 2022
- Cite this conference paper
- Abhishek Shrivastava 13 &
- Vijay Kumar Dalla 13
Part of the book series: Lecture Notes in Mechanical Engineering ((LNME))
738 Accesses
1 Altmetric
Space robotics is a relatively new exploring field in science mainly utilized for space exploration and space missions. Space robotics field is also utilized in space debris removal from earth orbit and preventing meteorites from hitting earth planet. Many authorized techniques were explored in past four decades and several technology advancement missions to space were also demonstrated by The National Aeronautics and Space Administration (NASA), European Space Agency (ESA), and The Indian Space Research Organization (ISRO). Several manned space missions were already demonstrated but fully autonomous, unmanned space missions are needed to be explored facing some technical issues in it. The major difficulty in space mission is to perform servicing of non-cooperative satellite with fully autonomous control, detumbling of non-cooperative satellites through impedance control, and dynamic control of autonomous moving targets with minimum rendezvous attitude. To inspire and assist advance research development in space technology, this paper provides a comprehensive study of the key challenges and projected solutions resembling to the obstacle avoidance, on-orbit servicing, and impedance control for fully autonomous space missions in near future. At last, possible directions for future research are discussed in conclusion part.
This is a preview of subscription content, log in via an institution to check access.
Access this chapter
- Available as PDF
- Read on any device
- Instant download
- Own it forever
- Available as EPUB and PDF
- Compact, lightweight edition
- Dispatched in 3 to 5 business days
- Free shipping worldwide - see info
- Durable hardcover edition
Tax calculation will be finalised at checkout
Purchases are for personal use only
Institutional subscriptions
Ahmed, S., Dalla, V.K., Prasad, N.: Motion planning control of cooperative two redundant space robots. In: 5th International Conference on Advances in Robotics, June 30–July 4 2021, IIT Kanpur, ACM, New York, NY, USA, 6 pp.
Google Scholar
Catlin, D., Blamires, M.: Designing robots for special needs education. Technol. Knowl. Learn. 24 (2), 291–313 (2019)
Article Google Scholar
Fong, T., Rochlis Zumbado, J., Currie, N., Mishkin, A., Akin, D.L.: Space telerobotics: unique challenges to human–robot collaboration in space. Rev. Hum. Factors Ergon. 9 (1), 6–56 (2013)
Yoshida, K.: Achievements in space robotics. IEEE Robot. Autom. Mag. 16 (4), 20–28 (2009)
Sanguino, T.D.: 50 years of rovers for planetary exploration: a retrospective review for future directions. Robot. Auton. Syst. 1 (94), 172–185 (2017)
Woffinden, D.C., Geller, D.K.: Navigating the road to autonomous orbital rendezvous. J. Spacecr. Rocket. 44 (4), 898–909 (2007)
Hirzinger, G., Landzettel, K., Brunner, B., Fischer, M., Preusche, C., Reintsema, D., Albu-Schäffer, A., Schreiber, G., Steinmetz, B.M.: DLR’s robotics technologies for on-orbit servicing. Adv. Robot. 18 (2), 139–174 (2004)
Jung, S., Hsia, T.C., Bonitz, R.G.: Force tracking impedance control of robot manipulators under unknown environment. IEEE Trans. Control Syst. Technol. 12 (3), 474–483 (2004)
Yoo, S., Lee, J., Choi, J., Chung, G., Chung, W.K.: Development of rotary hydro-elastic actuator with robust internal-loop-compensator-based torque control and cross-parallel connection spring. Mechatronics 1 (43), 112–123 (2017)
Zhao, Y., Chai, X., Gao, F., Qi, C.: Obstacle avoidance and motion planning scheme for a hexapod robot Octopus-III. Robot. Auton. Syst. 1 (103), 199–212 (2018)
Uyama, N., Narumi, T.: Hybrid impedance/position control of a free-flying space robot for detumbling a noncooperative satellite. IFAC-Papers On Line 49 (17), 230–235 (2016)
Qian, X., Altché, F., Bender, P., Stiller, C., de La Fortelle, A.: Optimal trajectory planning for autonomous driving integrating logical constraints: an MIQP perspective. In: 2016 IEEE 19th International Conference on Intelligent Transportation Systems (ITSC) 1 Nov 2016, pp. 205–210. IEEE (2016)
Dalla, V.K., Pathak, P.M.: Reconfiguration of joint locked hyper-redundant space manipulator. In: Proceedings of the 2015 Conference on Advances in Robotics 2 Jul 2015, pp. 1–6 (2015)
Hörmann, A., Meier, W., Schloen, J.: A control architecture for an advanced fault-tolerant robot system. Robot. Auton. Syst. 7 (2–3), 211–225 (1991)
Rahmann, H., Hilbig, R., Flemming, J., Slenzka, K.: Influence of long-term altered gravity on the swimming performance of developing cichlid fish: including results from the 2nd German Spacelab Mission D-2. Adv. Space Res. 17 (6–7), 121–124 (1996)
Oda, M.: ETS-VII: achievements, troubles and future. In: Proceedings of the 6th International Symposium on Artificial Intelligence and Robotics & Automation in Space: ISAIRAS 2001, Jun 2001
Oda, M.: Experiences and lessons learned from the ETS-VII robot satellite. In: Proceedings 2000 ICRA. Millennium Conference. IEEE International Conference on Robotics and Automation. Symposia Proceedings (Cat. No. 00CH37065) 24 Apr 2000, vol. 1, pp. 914–919). IEEE (2000)
Shan, M., Guo, J., Gill, E.: Review and comparison of active space debris capturing and removal methods. Prog. Aerosp. Sci. 1 (80), 18–32 (2016)
Dalla, V.K., Pathak, P.M.: Trajectory tracking control of a group of cooperative planar space robot systems. Proc. Inst. Mech. Eng. Part I: J. Syst. Control Eng. 229 (10), 885–901 (2015)
Garg, H., Sharma, S.P.: Multi-objective reliability-redundancy allocation problem using particle swarm optimization. Comput. Ind. Eng. 64 (1), 247–255 (2013)
Guo, W., Li, Y., Li, Y.Z., Wang, S.N., Zhong, M.L., Wang, J.X., Zhang, J.X.: Construction and experimental verification of a novel flexible thermal control system configuration for the autonomous on-orbit services of space missions. Energy Convers. Manag. 15 (138), 273–285 (2017)
Dalla, V.K., Pathak, P.M.: Curve-constrained collision-free trajectory control of hyper-redundant planar space robot. Proc. Inst. Mech. Eng. Part I: J. Syst. Control Eng. 231 (4), 282–298 (2017)
Shrivastava, A., Dalla, V.K.: Failure control and energy optimization of multi-axes space manipulator through genetic algorithm approach. J. Braz. Soc. Mech. Sci. Eng. 43 (10), 1–7 (2021)
Dalla, V.K., Pathak, P.M.: Docking operation by multiple space robots for minimum attitude disturbance. Int. J. Model. Simul. 38 (1), 38–49 (2018)
Zhengcang, C., Yuquan, L., Luo, H.: Analysis of dynamics of a floating-base N-DOF space manipulator system. In: 2016 2nd International Conference on Control, Automation and Robotics (ICCAR), 28 Apr 2016, pp. 233–237. IEEE (2016)
Zhan, B., Jin, M., Yang, G., Zhang, C.: A novel strategy for space manipulator detumbling a non-cooperative target with collision avoidance. Adv. Space Res. 66 (4), 785–799 (2020)
Meng, Q., Zhao, C., Ji, H., Liang, J.: Identify the full inertial parameters of a non-cooperative target with eddy current detumbling. Adv. Space Res. 66 (7), 1792–1802 (2020)
Luo, J., Xu, R., Wang, M.: Detumbling and stabilization of a tumbling target using a space manipulator with joint-velocity limits. Adv. Space Res. 66 (7), 1689–1699 (2020)
Han, D., Huang, P., Liu, X., Yang, Y.: Combined spacecraft stabilization control after multiple impacts during the capture of a tumbling target by a space robot. Acta Astron. (2020)
Liu, Y.Q., Yu, Z.W., Liu, X.F., Cai, G.P.: Active detumbling technology for high dynamic non-cooperative space targets. Multibody Syst. Dyn. 47 (1), 21–41 (2019)
Article MathSciNet MATH Google Scholar
Bennett, S.: Development of the PID controller. IEEE Control Syst. Mag. 13 (6), 58–62 (1993)
Uyama, N., Narumi, T.: Hybrid impedance/position control of a free-flying space robot for detumbling a non-cooperative satellite. IFAC-PapersOnLine 49 (17), 230–235 (2016)
Pathak, P.M., Mukherjee, A., Dasgupta, A.: Impedance control of space robots using passive degrees of freedom in controller domain
Dalla, V.K., Pathak, P.M.: Impedance control in multiple cooperative space robots pulling a flexible wire. Proc. Inst. Mech. Eng. C J. Mech. Eng. Sci. 233 (6), 2190–2205 (2019)
Zong, L., Luo, J., Wang, M., Yuan, J.: Obstacle avoidance handling and mixed integer predictive control for space robots. Adv. Space Res. 61 (8), 1997–2009 (2018)
Bao, D.Q., Zelinka, I.: Obstacle avoidance for swarm robot based on self-organizing migrating algorithm. Procedia Comput. Sci. 1 (150), 425–432 (2019)
Llamazares, Á., Molinos, E., Ocaña, M., Ivan, V.: Improved dynamic obstacle mapping (iDOMap). Sensors 20 (19), 5520 (2020)
Dalla, V.K., Pathak, P.M.: Power-optimized motion planning of reconfigured redundant space robot. Proc. Inst. Mech. Eng. Part I: J. Syst. Control Eng. 233 (8), 1030–1044 (2019)
Wang, M., Luo, J., Walter, U.: A non-linear model predictive controller with obstacle avoidance for a space robot. Adv. Space Res. 57 (8), 1737–1746 (2016)
Chen, Y., Peng, H., Grizzle, J.: Obstacle avoidance for low-speed autonomous vehicles with barrier function. IEEE Trans. Control Syst. Technol. 26 (1), 194–206 (2017)
Menon, M.S., Ravi, V.C., Ghosal, A.: Trajectory planning and obstacle avoidance for hyper-redundant serial robots. J. Mech. Robot. 9 (4) (2017)
Rostami, S.M., Sangaiah, A.K., Wang, J., Liu, X.: Obstacle avoidance of mobile robots using modified artificial potential field algorithm. EURASIP J. Wirel. Commun. Netw. 2019 (1), 70 (2019)
Kim, J.O., Khosla, P.: Real-time obstacle avoidance using harmonic potential functions
Kamegawa, T., Akiyama, T., Suzuki, Y., Kishutani, T., Gofuku, A.: Three-dimensional reflexive behavior by a snake robot with full circumference pressure sensors. In: 2020 IEEE/SICE International Symposium on System Integration (SII), 12 Jan 2020, pp. 897–902. IEEE (2020)
Parra, P., Polo, Ó.R., Carrasco, A., da Silva, A., Martínez, A., Sánchez, S.: Model-driven environment for configuration control and deployment of on-board satellite software. Acta Astron. (2020)
Fan, Y., Liu, X.: Two-stage auxiliary model gradient-based iterative algorithm for the input nonlinear controlled autoregressive system with variable-gain nonlinearity. Int. J. Robust Nonlinear Control 30 (14), 5492–5509 (2020)
Yao, Q., Zheng, Z., Qi, L., Yuan, H., Guo, X., Zhao, M., Liu, Z., Yang, T.: Path planning method with improved artificial potential field—a reinforcement learning perspective. IEEE Access 22 (8), 135513–135523 (2020)
Rösmann, C., Makarow, A., Bertram, T.: Online motion planning based on nonlinear model predictive control with non-Euclidean rotation groups. arXiv preprint arXiv:2006.03534 (2020)
Rybus, T.: Point-to-point motion planning of a free-floating space manipulator using the rapidly-exploring random trees (RRT) method. Robotica 38 (6), 957–982 (2020)
Khadem, M., O’Neill. J., Mitros, Z., da Cruz, L., Bergeles, C.: Autonomous steering of concentric tube robots via nonlinear model predictive control. IEEE Trans. Robot. (2020)
Zhao, Y., Liu, H., Gao, K.: An evacuation simulation method based on an improved artificial bee colony algorithm and a social force model. Appl. Intell. 6 , 1–24 (2020)
Nourbakhsh, I.R., Sycara, K., Koes, M., Yong, M., Lewis, M., Burion, S.: Human-robot teaming for search and rescue. IEEE Pervasive Comput. 4 (1), 72–79 (2005)
De Visser, E., Parasuraman, R.: Adaptive aiding of human-robot teaming: effects of imperfect automation on performance, trust, and workload. J. Cogn. Eng. Decis. Mak. 5 (2), 209–231 (2011)
Dalla, V.K., Pathak, P.M.: Trajectory control of curve constrained hyper-redundant space manipulator. In: Proceedings of the 14th IFToMM World Congress, 4 Nov 2015, pp. 367–374. 國立臺灣大學機械系
Download references
Author information
Authors and affiliations.
National Institute of Technology Jamshedpur, Jamshedpur, 831014, India
Abhishek Shrivastava & Vijay Kumar Dalla
You can also search for this author in PubMed Google Scholar
Corresponding author
Correspondence to Abhishek Shrivastava .
Editor information
Editors and affiliations.
Department of Industrial Design, National Institute of Technology Rourkel, Rourkela, India
B.B.V.L. Deepak
Department of Mechanical Engineering, National Institute of Technology, Puducherry, India
M.V.A. Raju Bahubalendruni
Head Professor (HAG),Mechanical Eng, National Institute of Technology Rourkel, Rourkela, India
D.R.K. Parhi
Mechanical Engineering, National Institute of Technology Meghala, Laitumkhrah, Shillong, India
Bibhuti Bhusan Biswal
Rights and permissions
Reprints and permissions
Copyright information
© 2023 The Author(s), under exclusive license to Springer Nature Singapore Pte Ltd.
About this paper
Cite this paper.
Shrivastava, A., Dalla, V.K. (2023). Space Robotics: A Comprehensive Study of Major Challenges and Proposed Solutions. In: Deepak, B., Bahubalendruni, M.R., Parhi, D., Biswal, B.B. (eds) Recent Trends in Product Design and Intelligent Manufacturing Systems. Lecture Notes in Mechanical Engineering. Springer, Singapore. https://doi.org/10.1007/978-981-19-4606-6_87
Download citation
DOI : https://doi.org/10.1007/978-981-19-4606-6_87
Published : 06 October 2022
Publisher Name : Springer, Singapore
Print ISBN : 978-981-19-4605-9
Online ISBN : 978-981-19-4606-6
eBook Packages : Engineering Engineering (R0)
Share this paper
Anyone you share the following link with will be able to read this content:
Sorry, a shareable link is not currently available for this article.
Provided by the Springer Nature SharedIt content-sharing initiative
- Publish with us
Policies and ethics
- Find a journal
- Track your research
Thank you for visiting nature.com. You are using a browser version with limited support for CSS. To obtain the best experience, we recommend you use a more up to date browser (or turn off compatibility mode in Internet Explorer). In the meantime, to ensure continued support, we are displaying the site without styles and JavaScript.
- View all journals
- Explore content
- About the journal
- Publish with us
- Sign up for alerts
- Published: 02 May 2024
Maximum diffusion reinforcement learning
- Thomas A. Berrueta ORCID: orcid.org/0000-0002-3781-0934 1 ,
- Allison Pinosky ORCID: orcid.org/0000-0002-3095-8856 1 &
- Todd D. Murphey ORCID: orcid.org/0000-0003-2262-8176 1
Nature Machine Intelligence ( 2024 ) Cite this article
2185 Accesses
138 Altmetric
Metrics details
- Computational science
- Computer science
A preprint version of the article is available at arXiv.
Robots and animals both experience the world through their bodies and senses. Their embodiment constrains their experiences, ensuring that they unfold continuously in space and time. As a result, the experiences of embodied agents are intrinsically correlated. Correlations create fundamental challenges for machine learning, as most techniques rely on the assumption that data are independent and identically distributed. In reinforcement learning, where data are directly collected from an agent’s sequential experiences, violations of this assumption are often unavoidable. Here we derive a method that overcomes this issue by exploiting the statistical mechanics of ergodic processes, which we term maximum diffusion reinforcement learning. By decorrelating agent experiences, our approach provably enables single-shot learning in continuous deployments over the course of individual task attempts. Moreover, we prove our approach generalizes well-known maximum entropy techniques and robustly exceeds state-of-the-art performance across popular benchmarks. Our results at the nexus of physics, learning and control form a foundation for transparent and reliable decision-making in embodied reinforcement learning agents.
This is a preview of subscription content, access via your institution
Access options
Access Nature and 54 other Nature Portfolio journals
Get Nature+, our best-value online-access subscription
24,99 € / 30 days
cancel any time
Subscribe to this journal
Receive 12 digital issues and online access to articles
111,21 € per year
only 9,27 € per issue
Buy this article
- Purchase on Springer Link
- Instant access to full article PDF
Prices may be subject to local taxes which are calculated during checkout
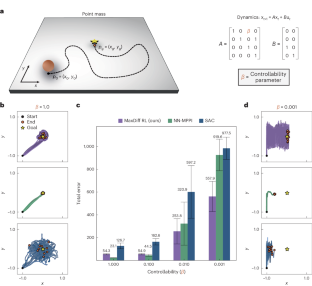
Similar content being viewed by others
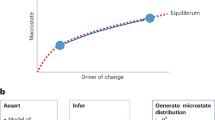
Entropy, irreversibility and inference at the foundations of statistical physics
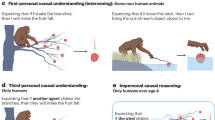
The development of human causal learning and reasoning
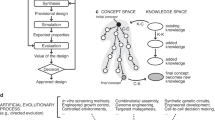
Engineering is evolution: a perspective on design processes to engineer biology
Data availability.
Data supporting the findings of this study are available via Zenodo at https://doi.org/10.5281/zenodo.10723320 (ref. 71 ).
Code availability
Code supporting the findings of this study is available via Zenodo at https://doi.org/10.5281/zenodo.10723320 (ref. 71 ).
Degrave, J. et al. Magnetic control of tokamak plasmas through deep reinforcement learning. Nature 602 , 414–419 (2022).
Article Google Scholar
Won, D.-O., Müller, K.-R. & Lee, S.-W. An adaptive deep reinforcement learning framework enables curling robots with human-like performance in real-world conditions. Sci. Robot. 5 , eabb9764 (2020).
Irpan, A. Deep reinforcement learning doesn’t work yet. Sorta Insightful www.alexirpan.com/2018/02/14/rl-hard.html (2018).
Henderson, P. et al. Deep reinforcement learning that matters. In Proc. 32nd AAAI Conference on Artificial Intelligence (eds McIlraith, S. & Weinberger, K.) 3207–3214 (AAAI, 2018).
Ibarz, J. et al. How to train your robot with deep reinforcement learning: lessons we have learned. Int. J. Rob. Res. 40 , 698–721 (2021).
Lillicrap, T. P. et al. Proc. 4th International Conference on Learning Representations (ICLR, 2016).
Haarnoja, T., Zhou, A., Abbeel, P. & Levine, S. Soft actor-critic: off-policy maximum entropy deep reinforcement learning with a stochastic actor. In Proc. 35th International Conference on Machine Learning (eds Dy, J. & Krause, A.) 1861–1870 (PMLR, 2018).
Plappert, M. et al. Proc. 6th International Conference on Learning Representations (ICLR, 2018).
Lin, L.-J. Self-improving reactive agents based on reinforcement learning, planning and teaching. Mach. Learn. 8 , 293–321 (1992).
Schaul, T., Quan, J., Antonoglou, I. & Silver, D. Proc. 4th International Conference on Learning Representations (ICLR, 2016).
Andrychowicz, M. et al. Hindsight experience replay. In Proc. Advances in Neural Information Processing Systems 30 (eds Guyon, I. et al.) 5049–5059 (Curran Associates, 2017).
Zhang, S. & Sutton, R. S. A deeper look at experience replay. Preprint at https://arxiv.org/abs/1712.01275 (2017).
Wang, Z. et al. Proc. 5th International Conference on Learning Representations (ICLR, 2017).
Hessel, M. et al. Rainbow: combining improvements in deep reinforcement learning. In Proc. 32nd AAAI Conference on Artificial Intelligence (eds McIlraith, S. and Weinberger, K.) 3215–3222 (AAAI Press, 2018).
Fedus, W. et al. Revisiting fundamentals of experience replay. In Proc. 37th International Conference on Machine Learning (eds Daumé III, H. & Singh, A.) 3061–3071 (JMLR.org, 2020).
Mnih, V. et al. Human-level control through deep reinforcement learning. Nature 518 , 529–533 (2015).
Ziebart, B. D., Maas, A. L., Bagnell, J. A. & Dey, A. K. Maximum entropy inverse reinforcement learning. In Proc. 23rd AAAI Conference on Artificial Intelligence (ed. Cohn, A.) 1433–1438 (AAAI, 2008).
Ziebart, B. D., Bagnell, J. A. & Dey, A. K. Modeling interaction via the principle of maximum causal entropy. In Proc. 27th International Conference on Machine Learning (eds Fürnkranz, J. & Joachims, T.) 1255–1262 (Omnipress, 2010).
Ziebart, B. D. Modeling Purposeful Adaptive Behavior with the Principle of Maximum Causal Entropy. PhD thesis, Carnegie Mellon Univ. (2010).
Todorov, E. Efficient computation of optimal actions. Proc. Natl Acad. Sci. USA 106 , 11478–11483 (2009).
Toussaint, M. Robot trajectory optimization using approximate inference. In Proc. 26th International Conference on Machine Learning (eds Bottou, L. & Littman, M.) 1049–1056 (ACM, 2009).
Rawlik, K., Toussaint, M. & Vijayakumar, S. On stochastic optimal control and reinforcement learning by approximate inference. In Proc. Robotics: Science and Systems VIII (eds Roy, N. et al.) 353–361 (MIT, 2012).
Levine, S. & Koltun, V. Guided policy search. In Proc. 30th International Conference on Machine Learning (eds Dasgupta, S. & McAllester, D.) 1–9 (JMLR.org, 2013).
Haarnoja, T., Tang, H., Abbeel, P. & Levine, S. Reinforcement learning with deep energy-based policies. In Proc. 34th International Conference on Machine Learning (eds Precup, D. & Teh, Y. W.) 1352–1361 (JMLR.org, 2017).
Haarnoja, T. et al. Learning to walk via deep reinforcement learning. In Proc. Robotics: Science and Systems XV (eds Bicchi, A. et al.) (RSS, 2019).
Eysenbach, B. & Levine, S. Proc. 10th International Conference on Learning Representations (ICLR, 2022).
Chen, M. et al. Top-K off-policy correction for a REINFORCE recommender system. In Proc. 12th ACM International Conference on Web Search and Data Mining (eds Bennett, P. N. & Lerman, K.) 456–464 (ACM, 2019).
Afsar, M. M., Crump, T. & Far, B. Reinforcement learning based recommender systems: a survey. ACM Comput. Surv. 55 , 1–38 (2022).
Chen, X., Yao, L., McAuley, J., Zhou, G. & Wang, X. Deep reinforcement learning in recommender systems: a survey and new perspectives. Knowl. Based Syst. 264 , 110335 (2023).
Sontag, E. D. Mathematical Control Theory: Deterministic Finite Dimensional Systems (Springer, 2013).
Hespanha, J. P. Linear Systems Theory 2nd edn (Princeton Univ. Press, 2018).
Mitra, D. W - matrix and the geometry of model equivalence and reduction. Proc. Inst. Electr. Eng. 116 , 1101–1106 (1969).
Article MathSciNet Google Scholar
Dean, S., Mania, H., Matni, N., Recht, B. & Tu, S. On the sample complexity of the linear quadratic regulator. Found. Comput. Math. 20 , 633–679 (2020).
Tsiamis, A. & Pappas, G. J. Linear systems can be hard to learn. In Proc. 60th IEEE Conference on Decision and Control (ed. Prandini, M.) 2903–2910 (IEEE, 2021).
Tsiamis, A., Ziemann, I. M., Morari, M., Matni, N. & Pappas, G. J. Learning to control linear systems can be hard. In Proc. 35th Conference on Learning Theory (eds Loh, P.-L. & Raginsky, M.) 3820–3857 (PMLR, 2022).
Williams, G. et al. Information theoretic MPC for model-based reinforcement learning. In Proc. IEEE International Conference on Robotics and Automation (ed. Nakamura, Y.) 1714–1721 (IEEE, 2017).
So, O., Wang, Z. & Theodorou, E. A. Maximum entropy differential dynamic programming. In Proc. IEEE International Conference on Robotics and Automation (ed. Kress-Gazit, H.) 3422–3428 (IEEE, 2022).
Thrun, S. B. Efficient Exploration in Reinforcement Learning . Technical report (Carnegie Mellon Univ., 1992).
Amin, S., Gomrokchi, M., Satija, H., van Hoof, H. & Precup, D. A survey of exploration methods in reinforcement learning. Preprint at https://arXiv.org/2109.00157 (2021).
Jaynes, E. T. Information theory and statistical mechanics. Phys. Rev. 106 , 620–630 (1957).
Dixit, P. D. et al. Perspective: maximum caliber is a general variational principle for dynamical systems. J. Chem. Phys. 148 , 010901 (2018).
Chvykov, P. et al. Low rattling: a predictive principle for self-organization in active collectives. Science 371 , 90–95 (2021).
Kapur, J. N. Maximum Entropy Models in Science and Engineering (Wiley, 1989).
Moore, C. C. Ergodic theorem, ergodic theory, and statistical mechanics. Proc. Natl Acad. Sci. USA 112 , 1907–1911 (2015).
Taylor, A. T., Berrueta, T. A. & Murphey, T. D. Active learning in robotics: a review of control principles. Mechatronics 77 , 102576 (2021).
Seo, Y. et al. State entropy maximization with random encoders for efficient exploration. In Proc. 38th International Conference on Machine Learning, Virtual (eds Meila, M. & Zhang, T.) 9443–9454 (ICML, 2021).
Prabhakar, A. & Murphey, T. Mechanical intelligence for learning embodied sensor-object relationships. Nat. Commun. 13 , 4108 (2022).
Chentanez, N., Barto, A. & Singh, S. Intrinsically motivated reinforcement learning. In Proc. Advances in Neural Information Processing Systems 17 (eds Saul, L. et al.) 1281–1288 (MIT, 2004).
Pathak, D., Agrawal, P., Efros, A. A. & Darrell, T. Curiosity-driven exploration by self-supervised prediction. In Proc. 34th International Conference on Machine Learning (eds Precup, D. & Teh, Y. W.) 2778–2787 (JLMR.org, 2017).
Taiga, A. A., Fedus, W., Machado, M. C., Courville, A. & Bellemare, M. G. Proc. 8th International Conference on Learning Representations (ICLR, 2020).
Wang, X., Deng, W. & Chen, Y. Ergodic properties of heterogeneous diffusion processes in a potential well. J. Chem. Phys. 150 , 164121 (2019).
Palmer, R. G. Broken ergodicity. Adv. Phys. 31 , 669–735 (1982).
Islam, R., Henderson, P., Gomrokchi, M. & Precup, D. Reproducibility of benchmarked deep reinforcement learning tasks for continuous control. Preprint at https://arXiv.org/1708.04133 (2017).
Moos, J. et al. Robust reinforcement learning: a review of foundations and recent advances. Mach. Learn. Knowl. Extr. 4 , 276–315 (2022).
Strehl, A. L., Li, L., Wiewiora, E., Langford, J. & Littman, M. L. PAC model-free reinforcement learning. In Proc. 23rd International Conference on Machine Learning (eds Cohen, W. W. & Moore, A.) 881–888 (ICML, 2006).
Strehl, A. L., Li, L. & Littman, M. L. Reinforcement learning in finite MDPs: PAC analysis. J. Mach. Learn. Res. 10 , 2413–2444 (2009).
Kirk, R., Zhang, A., Grefenstette, E. & Rocktäaschel, T. A survey of zero-shot generalisation in deep reinforcement learning. J. Artif. Intell. Res. 76 , 201–264 (2023).
Oh, J., Singh, S., Lee, H. & Kohli, P. Zero-shot task generalization with multi-task deep reinforcement learning. In Proc. 34th International Conference on Machine Learning (eds Precup, D. & Teh, Y. W.) 2661–2670 (JLMR.org, 2017).
Krakauer, J. W., Hadjiosif, A. M., Xu, J., Wong, A. L. & Haith, A. M. Motor learning. Compr. Physiol. 9 , 613–663 (2019).
Lu, K., Grover, A., Abbeel, P. & Mordatch, I. Proc. 9th International Conference on Learning Representations (ICLR, 2021).
Chen, A., Sharma, A., Levine, S. & Finn, C. You only live once: single-life reinforcement learning. In Proc. Advances in Neural Information Processing Systems 35 (eds Koyejo, S. et al.) 14784–14797 (NeurIPS, 2022).
Ames, A., Grizzle, J. & Tabuada, P. Control barrier function based quadratic programs with application to adaptive cruise control. In Proc. 53rd IEEE Conference on Decision and Control 6271–6278 (IEEE, 2014).
Taylor, A., Singletary, A., Yue, Y. & Ames, A. Learning for safety-critical control with control barrier functions. In Proc. 2nd Conference on Learning for Dynamics and Control (eds Bayen, A. et al.) 708–717 (PLMR, 2020).
Xiao, W. et al. BarrierNet: differentiable control barrier functions for learning of safe robot control. IEEE Trans. Robot. 39 , 2289–2307 (2023).
Seung, H. S., Sompolinsky, H. & Tishby, N. Statistical mechanics of learning from examples. Phys. Rev. A 45 , 6056–6091 (1992).
Chen, C., Murphey, T. D. & MacIver, M. A. Tuning movement for sensing in an uncertain world. eLife 9 , e52371 (2020).
Song, S. et al. Deep reinforcement learning for modeling human locomotion control in neuromechanical simulation. J. Neuroeng. Rehabil. 18 , 126 (2021).
Berrueta, T. A., Murphey, T. D. & Truby, R. L. Materializing autonomy in soft robots across scales. Adv. Intell. Syst. 6 , 2300111 (2024).
Sutton, R. S. & Barto, A. G. Reinforcement Learning: An Introduction (MIT, 2018).
Virtanen, P. et al. SciPy 1.0: fundamental algorithms for scientific computing in Python. Nat. Methods 17 , 261–272 (2020).
Berrueta, T. A., Pinosky, A. & Murphey, T. D. Maximum diffusion reinforcement learning repository. Zenodo https://doi.org/10.5281/zenodo.10723320 (2024).
Download references
Acknowledgements
We thank A. T. Taylor, J. Weber and P. Chvykov for their comments on early drafts of this work. We acknowledge funding from the US Army Research Office MURI grant no. W911NF-19-1-0233 and the US Office of Naval Research grant no. N00014-21-1-2706. We also acknowledge hardware loans and technical support from Intel Corporation, and T.A.B. is partially supported by the Northwestern University Presidential Fellowship.

Author information
Authors and affiliations.
Department of Mechanical Engineering, Northwestern University, Evanston, IL, USA
Thomas A. Berrueta, Allison Pinosky & Todd D. Murphey
You can also search for this author in PubMed Google Scholar
Contributions
T.A.B. derived all theoretical results, performed supplementary data analyses and control experiments, supported RL experiments and wrote the manuscript. A.P. developed and tested RL algorithms, carried out all RL experiments and supported manuscript writing. T.D.M. secured funding and guided the research programme.
Corresponding authors
Correspondence to Thomas A. Berrueta or Todd D. Murphey .
Ethics declarations
Competing interests.
The authors declare no competing interests.
Peer review
Peer review information.
Nature Machine Intelligence thanks the anonymous reviewers for their contribution to the peer review of this work.
Additional information
Publisher’s note Springer Nature remains neutral with regard to jurisdictional claims in published maps and institutional affiliations.
Supplementary information
Supplementary information.
Supplementary Notes 1–4, Tables 1 and 2 and Figs. 1–9.
Supplementary Video 1
Depicts an application of MaxDiff RL to MuJoCo’s swimmer environment. We explore the role of the temperature parameter’s performance by varying it across three orders of magnitude.
Supplementary Video 2
Depicts an application of MaxDiff RL to MuJoCo’s swimmer environment, with comparisons to NN-MPPI and SAC. The performance of MaxDiff RL does not vary across seeds. This is tested across two different system conditions: one with a light-tailed and more controllable swimmer and one with a heavy-tailed and less controllable swimmer.
Supplementary Video 3
Depicts an application of MaxDiff RL to MuJoCo’s swimmer environment. We perform a transfer learning experiment in which neural representations are learned on a system with a given set of properties and then deployed on a system with different properties. MaxDiff RL remains task-capable across agent embodiments.
Supplementary Video 4
Depicts an application of MaxDiff RL to MuJoCo’s swimmer environment under a substantial modification. Agents cannot reset their environment, which requires solving the task in a single deployment. First, representative snapshots of single-shot deployments are shown. A complete playback of an individual MaxDiff RL single-shot learning trial is shown. Playback is staggered such that the first swimmer covers environment steps 1–2,000, the next one 2,001–4,000, and so on, for a total of 20,000 environment steps.
Rights and permissions
Springer Nature or its licensor (e.g. a society or other partner) holds exclusive rights to this article under a publishing agreement with the author(s) or other rightsholder(s); author self-archiving of the accepted manuscript version of this article is solely governed by the terms of such publishing agreement and applicable law.
Reprints and permissions
About this article
Cite this article.
Berrueta, T.A., Pinosky, A. & Murphey, T.D. Maximum diffusion reinforcement learning. Nat Mach Intell (2024). https://doi.org/10.1038/s42256-024-00829-3
Download citation
Received : 03 August 2023
Accepted : 19 March 2024
Published : 02 May 2024
DOI : https://doi.org/10.1038/s42256-024-00829-3
Share this article
Anyone you share the following link with will be able to read this content:
Sorry, a shareable link is not currently available for this article.
Provided by the Springer Nature SharedIt content-sharing initiative
Quick links
- Explore articles by subject
- Guide to authors
- Editorial policies
Sign up for the Nature Briefing: AI and Robotics newsletter — what matters in AI and robotics research, free to your inbox weekly.

Help | Advanced Search
Computer Science > Robotics
Title: field notes on deploying research robots in public spaces.
Abstract: Human-robot interaction requires to be studied in the wild. In the summers of 2022 and 2023, we deployed two trash barrel service robots through the wizard-of-oz protocol in public spaces to study human-robot interactions in urban settings. We deployed the robots at two different public plazas in downtown Manhattan and Brooklyn for a collective of 20 hours of field time. To date, relatively few long-term human-robot interaction studies have been conducted in shared public spaces. To support researchers aiming to fill this gap, we would like to share some of our insights and learned lessons that would benefit both researchers and practitioners on how to deploy robots in public spaces. We share best practices and lessons learned with the HRI research community to encourage more in-the-wild research of robots in public spaces and call for the community to share their lessons learned to a GitHub repository.
Submission history
Access paper:.
- HTML (experimental)
- Other Formats

References & Citations
- Google Scholar
- Semantic Scholar
BibTeX formatted citation

Bibliographic and Citation Tools
Code, data and media associated with this article, recommenders and search tools.
- Institution
arXivLabs: experimental projects with community collaborators
arXivLabs is a framework that allows collaborators to develop and share new arXiv features directly on our website.
Both individuals and organizations that work with arXivLabs have embraced and accepted our values of openness, community, excellence, and user data privacy. arXiv is committed to these values and only works with partners that adhere to them.
Have an idea for a project that will add value for arXiv's community? Learn more about arXivLabs .

IMAGES
VIDEO
COMMENTS
Space robotics plays a critical role in current and future space exploration missions and enables mission-defined machines that are capable of surviving in the space environment and performing exploration, assembly, construction, maintenance, or service tasks. Modern space robotics represents a multidisciplinary emerging field that builds on ...
After reviewing representative space robotic programs and analyzing the primary key technologies and challenges, the following seven topics are recommended for further space robot research. 4.1 Advanced Control Strategies for Nonlinear Rigid-Flexible Coupling Systems. Space robots are time-varying nonlinear rigid-flexible coupling systems.
The critical role that robotics and autonomous systems can play in enabling the exploration of planetary surfaces has been projected for many decades and was foreseen by a NASA study group on "Robotics and Machine Intelligence" in 1980 led by Carl Sagan [].As of this writing, we are only 2 years away from achieving a continuous robotic presence on Mars for one-quarter century.
This paper introduces recent space missions and surveys hot topics on new research activities and developed technology for the near future missions. ... His research interests include AI in space, Robotics, and Image-based navigation. He is a member of the Robotics Society of Japan, the Society of Instrument and Control Engineers of Japan, IEEE ...
This paper presents a review of modular and reconfigurable space robot systems intended for use in orbital and planetary applications. Modular autonomous robotic systems promise to be efficient, versatile, and resilient compared with conventional and monolithic robots, and have the potential to outperform traditional systems with a fixed morphology when carrying out tasks that require a high ...
The paper reviews space robots' technological progress and development trends, which provides a good reference for further technical research in this field. Acknowledgments We warmly thank all authors of the numerous manuscripts submitted to this Special Issue.
Because research pertaining to space robotics is generally interdisciplinary in nature, we include articles not only from roboticists but also from physicists and planetary scientists. ... The two review papers in this special section discuss robotic attachment mechanisms (2100063) and soft robots (2200071) for space applications. In particular ...
Advanced robotic hardware, software and autonomous capabilities are key for space exploration missions on the surface of extraterrestrial objects, such as distant planets or moons (planetary robotics). They are also key ingredients to military, government, and commercial spacecraft missions like on-orbit resupply, inspection, repair, and de ...
Purpose of Review This review provides an overview of the motivation, challenges, state-of-the-art, and recent research for human-robot interaction (HRI) in space. For context, we focus on NASA space missions, use cases, and systems (both flight and research). However, the discussion is broadly applicable to all activities in space that require or make use of human-robot teams. Recent Findings ...
focused on terrestrial applications. Research on soft space robots is still in its infancy. Soft robots used in space should satisfy the special requirements of space environments. More specifically, softspacerobots mustadapttolow-gravityand ultrahighvacuum environments and have the ability to withstand extreme temper-atures and space radiation.
Purpose of Review: The article provides an extensive overview on the resilient autonomy advances made across various missions, orbital or deep-space, that captures the current research approaches while investigating the possible future direction of resiliency in space autonomy. Recent Findings: In recent years, the need for several automated operations in space applications has been rising ...
This paper recalls some of the major robotics missions in space and explains technologies related to them. Space robotic manipulators, especially flexible link and flexible joint robots are discussed.
Space Robotics is a relatively new field of science and engineering that was developed as an answer to growing needs created by space exploration and space missions. New technologies had to be invented and designed in order to meet demands in extremely hostile environments. Those technologies have to work in a gravityless environment, rarefied atmosphere and often in high temperature. This ...
Search for more papers by this author. Pengchun Li, Pengchun Li. State Key Laboratory of Robotics and System, Harbin Institute of Technology, Harbin, 150001 China ... Research on soft space robots is still in its infancy. Soft robots used in space should satisfy the special requirements of space environments. More specifically, soft space ...
Abstract and Figures. In this work, we provide the findings of a NASA survey that was designed to assess the current status of space robotics and forecast future robotic capabilities under either ...
This paper reviews some key application areas of robotics that involve interactions with harsh environments (such as search and rescue, space exploration, and deep-sea operations), gives an ...
Abstract. In this paper we summarize a survey conducted by. 1. Introduction NASA to determine the state-of-the-art in space Robotic systems began the era of space exploration robotics and to predict future robotic capabilities under with series of spacecraft including Mariner, Ranger, either nominal and intensive development effort.
One important aspect for the use of robots in space environments is the way they are operated. The Lunokhod 1 and Lunokhod 2 [] rovers were tele-operated in the 1970s, and travelled around 10 km and 37 km respectively on the surface of the moon.A team of operators gave direct driving commands based on slow scan TV cameras with update rates of one frame every 3 s.
The research of space station robot can also promote advanced crossover such as human factor engineering and technology research. Therefore, the use of space robots to carry out on-orbit assembly technology research has the dual significance of promoting the development of the aerospace field and pulling related technological breakthroughs ...
A space robotic system (also referred to as space manipulator or space robot) for an OOS mission typically consists of three major components: the base spacecraft or servicing satellite, an n-degree-of-freedom (n-DOF) robot manipulator attached to the servicing satellite, and the target spacecraft to be serviced.A spacecraft-manipulator servicing vehicle (illustrated in Fig. 1) is sometimes ...
Italy SPACE ROBOTICS G. HIRZINGER German Aerospace Research Establishment (DLR) Institute for Robotics and System Dynamics, Oberpfaffenhofen, D-82234 Wessling, Germany, fax: +49 8153 28-1134, email: [email protected] Abstract. The paper tries to outline the state of the art in space robotics. It discusses the technologies used in ROTEX ...
Outer space has a harsh environment, high temperature, and radiation so human access is very difficult. To assist human research activity in space for developing a space module and planetary surface research, robots are contributing to the research of solar system [].Space servicing is an important task to be performed in the area of space science.
Robots and animals both experience the world through their bodies and senses. Their embodiment constrains their experiences, ensuring that they unfold continuously in space and time. As a result ...
Space Robotics and its Challenges. Jurek Sasiadek. Carleton University, Ottawa, Ontario, K1S 5B6, Canada. Abstract. Space robotics is a fascinating field that was developed for space explorations ...
Human-robot interaction requires to be studied in the wild. In the summers of 2022 and 2023, we deployed two trash barrel service robots through the wizard-of-oz protocol in public spaces to study human-robot interactions in urban settings. We deployed the robots at two different public plazas in downtown Manhattan and Brooklyn for a collective of 20 hours of field time. To date, relatively ...