- Research article
- Open access
- Published: 03 February 2021

A review of the quantitative effectiveness evidence synthesis methods used in public health intervention guidelines
- Ellesha A. Smith ORCID: orcid.org/0000-0002-4241-7205 1 ,
- Nicola J. Cooper 1 ,
- Alex J. Sutton 1 ,
- Keith R. Abrams 1 &
- Stephanie J. Hubbard 1
BMC Public Health volume 21 , Article number: 278 ( 2021 ) Cite this article
4084 Accesses
5 Citations
3 Altmetric
Metrics details
The complexity of public health interventions create challenges in evaluating their effectiveness. There have been huge advancements in quantitative evidence synthesis methods development (including meta-analysis) for dealing with heterogeneity of intervention effects, inappropriate ‘lumping’ of interventions, adjusting for different populations and outcomes and the inclusion of various study types. Growing awareness of the importance of using all available evidence has led to the publication of guidance documents for implementing methods to improve decision making by answering policy relevant questions.
The first part of this paper reviews the methods used to synthesise quantitative effectiveness evidence in public health guidelines by the National Institute for Health and Care Excellence (NICE) that had been published or updated since the previous review in 2012 until the 19th August 2019.The second part of this paper provides an update of the statistical methods and explains how they address issues related to evaluating effectiveness evidence of public health interventions.
The proportion of NICE public health guidelines that used a meta-analysis as part of the synthesis of effectiveness evidence has increased since the previous review in 2012 from 23% (9 out of 39) to 31% (14 out of 45). The proportion of NICE guidelines that synthesised the evidence using only a narrative review decreased from 74% (29 out of 39) to 60% (27 out of 45).An application in the prevention of accidents in children at home illustrated how the choice of synthesis methods can enable more informed decision making by defining and estimating the effectiveness of more distinct interventions, including combinations of intervention components, and identifying subgroups in which interventions are most effective.
Conclusions
Despite methodology development and the publication of guidance documents to address issues in public health intervention evaluation since the original review, NICE public health guidelines are not making full use of meta-analysis and other tools that would provide decision makers with fuller information with which to develop policy. There is an evident need to facilitate the translation of the synthesis methods into a public health context and encourage the use of methods to improve decision making.
Peer Review reports
To make well-informed decisions and provide the best guidance in health care policy, it is essential to have a clear framework for synthesising good quality evidence on the effectiveness and cost-effectiveness of health interventions. There is a broad range of methods available for evidence synthesis. Narrative reviews provide a qualitative summary of the effectiveness of the interventions. Meta-analysis is a statistical method that pools evidence from multiple independent sources [ 1 ]. Meta-analysis and more complex variations of meta-analysis have been extensively applied in the appraisals of clinical interventions and treatments, such as drugs, as the interventions and populations are clearly defined and tested in randomised, controlled conditions. In comparison, public health studies are often more complex in design, making synthesis more challenging [ 2 ].
Many challenges are faced in the synthesis of public health interventions. There is often increased methodological heterogeneity due to the inclusion of different study designs. Interventions are often poorly described in the literature which may result in variation within the intervention groups. There can be a wide range of outcomes, whose definitions are not consistent across studies. Intermediate, or surrogate, outcomes are often used in studies evaluating public health interventions [ 3 ]. In addition to these challenges, public health interventions are often also complex meaning that they are made up of multiple, interacting components [ 4 ]. Recent guidance documents have focused on the synthesis of complex interventions [ 2 , 5 , 6 ]. The National Institute for Health and Care Excellence (NICE) guidance manual provides recommendations across all topics that are covered by NICE and there is currently no guidance that focuses specifically on the public health context.
Research questions
A methodological review of NICE public health intervention guidelines by Achana et al. (2014) found that meta-analysis methods were not being used [ 3 ]. The first part of this paper aims to update and compare, to the original review, the meta-analysis methods being used in evidence synthesis of public health intervention appraisals.
The second part of this paper aims to illustrate what methods are available to address the challenges of public health intervention evidence synthesis. Synthesis methods that go beyond a pairwise meta-analysis are illustrated through the application to a case study in public health and are discussed to understand how evidence synthesis methods can enable more informed decision making.
The third part of this paper presents software, guidance documents and web tools for methods that aim to make appropriate evidence synthesis of public health interventions more accessible. Recommendations for future research and guidance production that can improve the uptake of these methods in a public health context are discussed.
Update of NICE public health intervention guidelines review
Nice guidelines.
The National Institute for Health and Care Excellence (NICE) was established in 1999 as a health authority to provide guidance on new medical technologies to the NHS in England and Wales [ 7 ]. Using an evidence-based approach, it provides recommendations based on effectiveness and cost-effectiveness to ensure an open and transparent process of allocating NHS resources [ 8 ]. The remit for NICE guideline production was extended to public health in April 2005 and the first recommendations were published in March 2006. NICE published ‘Developing NICE guidelines: the manual’ in 2006, which has been updated since, with the most recent in 2018 [ 9 ]. It was intended to be a guidance document to aid in the production of NICE guidelines across all NICE topics. In terms of synthesising quantitative evidence, the NICE recommendations state: ‘meta-analysis may be appropriate if treatment estimates of the same outcome from more than 1 study are available’ and ‘when multiple competing options are being appraised, a network meta-analysis should be considered’. The implementation of network meta-analysis (NMA), which is described later, as a recommendation from NICE was introduced into the guidance document in 2014, with a further update in 2018.
Background to the previous review
The paper by Achana et al. (2014) explored the use of evidence synthesis methodology in NICE public health intervention guidelines published between 2006 and 2012 [ 3 ]. The authors conducted a systematic review of the methods used to synthesise quantitative effectiveness evidence within NICE public health guidelines. They found that only 23% of NICE public health guidelines used pairwise meta-analysis as part of the effectiveness review and the remainder used a narrative summary or no synthesis of evidence at all. The authors argued that despite significant advances in the methodology of evidence synthesis, the uptake of methods in public health intervention evaluation is lower than other fields, including clinical treatment evaluation. The paper concluded that more sophisticated methods in evidence synthesis should be considered to aid in decision making in the public health context [ 3 ].
The search strategy used in this paper was equivalent to that in the previous paper by Achana et al. (2014)[ 3 ]. The search was conducted through the NICE website ( https://www.nice.org.uk/guidance ) by searching the ‘Guidance and Advice List’ and filtering by ‘Public Health Guidelines’ [ 10 ]. The search criteria included all guidance documents that had been published from inception (March 2006) until the 19th August 2019. Since the original review, many of the guidelines had been updated with new documents or merged. Guidelines that remained unchanged since the previous review in 2012 were excluded and used for comparison.
The guidelines contained multiple documents that were assessed for relevance. A systematic review is a separate synthesis within a guideline that systematically collates all evidence on a specific research question of interest in the literature. Systematic reviews of quantitative effectiveness, cost-effectiveness evidence and decision modelling reports were all included as relevant. Qualitative reviews, field reports, expert opinions, surveillance reports, review decisions and other supporting documents were excluded at the search stage.
Within the reports, data was extracted on the types of review (narrative summary, pairwise meta-analysis, network meta-analysis (NMA), cost-effectiveness review or decision model), design of included primary studies (randomised controlled trials or non-randomised studies, intermediate or final outcomes, description of outcomes, outcome measure statistic), details of the synthesis methods used in the effectiveness evaluation (type of synthesis, fixed or random effects model, study quality assessment, publication bias assessment, presentation of results, software). Further details of the interventions were also recorded, including whether multiple interventions were lumped together for a pairwise comparison, whether interventions were complex (made up of multiple components) and details of the components. The reports were also assessed for potential use of complex intervention evidence synthesis methodology, meaning that the interventions that were evaluated in the review were made up of components that could potentially be synthesised using an NMA or a component NMA [ 11 ]. Where meta-analysis was not used to synthesis effectiveness evidence, the reasons for this was also recorded.
Search results and types of reviews
There were 67 NICE public health guidelines available on the NICE website. A summary flow diagram describing the literature identification process and the list of guidelines and their reference codes are provided in Additional files 1 and 2 . Since the previous review, 22 guidelines had not been updated. The results from the previous review were used for comparison to the 45 guidelines that were either newly published or updated.
The guidelines consisted of 508 documents that were assessed for relevance. Table 1 shows which types of relevant documents were available in each of the 45 guidelines. The median number of relevant articles per guideline was 3 (minimum = 0, maximum = 10). Two (4%) of the NICE public health guidelines did not report any type of systematic review, cost-effectiveness review or decision model (NG68, NG64) that met the inclusion criteria. 167 documents from 43 NICE public health guidelines were systematic reviews of quantitative effectiveness, cost-effectiveness or decision model reports and met the inclusion criteria.
Narrative reviews of effectiveness were implemented in 41 (91%) of the NICE PH guidelines. 14 (31%) contained a review that used meta-analysis to synthesise the evidence. Only one (1%) NICE guideline contained a review that implemented NMA to synthesise the effectiveness of multiple interventions; this was the same guideline that used NMA in the original review and had been updated. 33 (73%) guidelines contained cost-effectiveness reviews and 34 (76%) developed a decision model.
Comparison of review types to original review
Table 2 compares the results of the update to the original review and shows that the types of reviews and evidence synthesis methodologies remain largely unchanged since 2012. The proportion of guidelines that only contain narrative reviews to synthesise effectiveness or cost-effectiveness evidence has reduced from 74% to 60% and the proportion that included a meta-analysis has increased from 23% to 31%. The proportion of guidelines with reviews that only included evidence from randomised controlled trials and assessed the quality of individual studies remained similar to the original review.
Characteristics of guidelines using meta-analytic methods
Table 3 details the characteristics of the meta-analytic methods implemented in 24 reviews of the 14 guidelines that included one. All of the reviews reported an assessment of study quality, 12 (50%) reviews included only data from randomised controlled trials, 4 (17%) reviews used intermediate outcomes (e.g. uptake of chlamydia screening rather than prevention of chlamydia (PH3)), compared to the 20 (83%) reviews that used final outcomes (e.g. smoking cessation rather than uptake of a smoking cessation programme (NG92)). 2 (8%) reviews only used a fixed effect meta-analysis, 19 (79%) reviews used a random effects meta-analysis and 3 (13%) did not report which they had used.
An evaluation of the intervention information reported in the reviews concluded that 12 (50%) reviews had lumped multiple (more than two) different interventions into a control versus intervention pairwise meta-analysis. Eleven (46%) of the reviews evaluated interventions that are made up of multiple components (e.g. interventions for preventing obesity in PH47 were made up of diet, physical activity and behavioural change components).
21 (88%) of the reviews presented the results of the meta-analysis in the form of a forest plot and 22 (92%) presented the results in the text of the report. 20 (83%) of the reviews used two or more forms of presentation for the results. Only three (13%) reviews assessed publication bias. The most common software to perform meta-analysis was RevMan in 14 (58%) of the reviews.
Reasons for not using meta-analytic methods
The 143 reviews of effectiveness and cost effectiveness that did not use meta-analysis methods to synthesise the quantitative effectiveness evidence were searched for reasons behind this decision. 70 reports (49%) did not give a reason for not synthesising the data using a meta-analysis and 164 reasons were reported which are displayed in Fig. 1 . Out of the remaining reviews, multiple reasons for not using a meta-analysis were given. 53 (37%) of the reviews reported at least one reason due to heterogeneity. 30 (21%) decision model reports did not give a reason and these are categorised separately. 5 (3%) reviews reported that meta-analysis was not applicable or feasible, 1 (1%) reported that they were following NICE guidelines and 5 (3%) reported that there were a lack of studies.
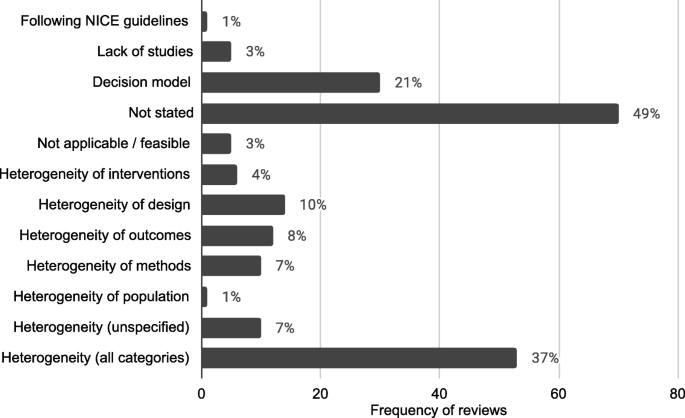
Frequency and proportions of reasons reported for not using statistical methods in quantitative evidence synthesis in NICE PH intervention reviews
The frequency of reviews and guidelines that used meta-analytic methods were plotted against year of publication, which is reported in Fig. 2 . This showed that the number of reviews that used meta-analysis were approximately constant but there is some suggestion that the number of meta-analyses used per guideline increased, particularly in 2018.
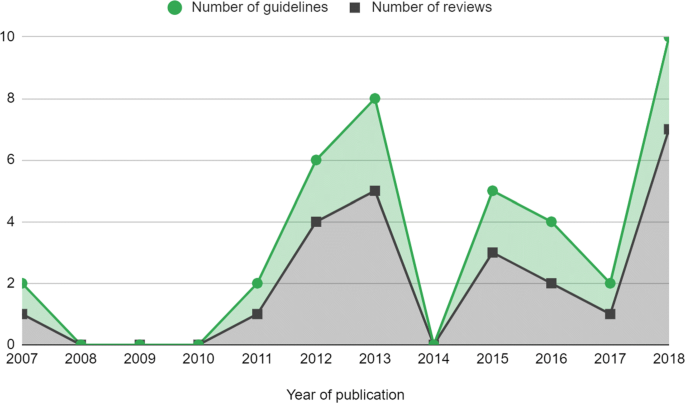
Number of meta-analyses in NICE PH guidelines by year. Guidelines that were published before 2012 had been updated since the previous review by Achana et al. (2014) [ 3 ]
Comparison of meta-analysis characteristics to original review
Table 4 compares the characteristics of the meta-analyses used in the evidence synthesis of NICE public health intervention guidelines to the original review by Achana et al. (2014) [ 3 ]. Overall, the characteristics in the updated review have not much changed from those in the original. These changes demonstrate that the use of meta-analysis in NICE guidelines has increased but remains low. Lumping of interventions still appears to be common in 50% of reviews. The implications of this are discussed in the next section.
Application of evidence synthesis methodology in a public health intervention: motivating example
Since the original review, evidence synthesis methods have been developed and can address some of the challenges of synthesising quantitative effectiveness evidence of public health interventions. Despite this, the previous section shows that the uptake of these methods is still low in NICE public health guidelines - usually limited to a pairwise meta-analysis.
It has been shown in the results above and elsewhere [ 12 ] that heterogeneity is a common reason for not synthesising the quantitative effectiveness evidence available from systematic reviews in public health. Statistical heterogeneity is the variation in the intervention effects between the individual studies. Heterogeneity is problematic in evidence synthesis as it leads to uncertainty in the pooled effect estimates in a meta-analysis which can make it difficult to interpret the pooled results and draw conclusions. Rather than exploring the source of the heterogeneity, often in public health intervention appraisals a random effects model is fitted which assumes that the study intervention effects are not equivalent but come from a common distribution [ 13 , 14 ]. Alternatively, as demonstrated in the review update, heterogeneity is used as a reason to not undertake any quantitative evidence synthesis at all.
Since the size of the intervention effects and the methodological variation in the studies will affect the impact of the heterogeneity on a meta-analysis, it is inappropriate to base the methodological approach of a review on the degree of heterogeneity, especially within public health intervention appraisal where heterogeneity seems inevitable. Ioannidis et al. (2008) argued that there are ‘almost always’ quantitative synthesis options that may offer some useful insights in the presence of heterogeneity, as long as the reviewers interpret the findings with respect to their limitations [ 12 ].
In this section current evidence synthesis methods are applied to a motivating example in public health. This aims to demonstrate that methods beyond pairwise meta-analysis can provide appropriate and pragmatic information to public health decision makers to enable more informed decision making.
Figure 3 summarises the narrative of this part of the paper and illustrates the methods that are discussed. The red boxes represent the challenges in synthesising quantitative effectiveness evidence and refers to the section within the paper for more detail. The blue boxes represent the methods that can be applied to investigate each challenge.
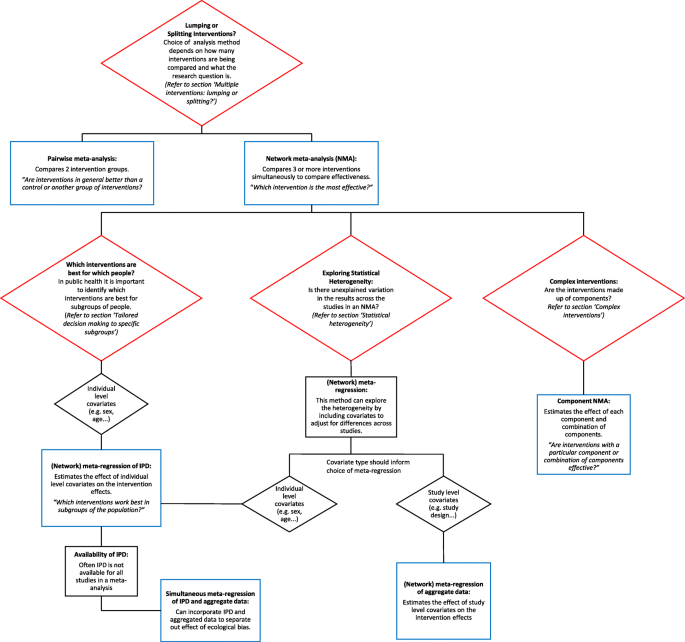
Summary of challenges that are faces in the evidence synthesis of public health interventions and methods that are discussed to overcome these challenges
Evaluating the effect of interventions for promoting the safe storage of cleaning products to prevent childhood poisoning accidents
To illustrate the methodological developments, a motivating example is used from the five year, NIHR funded, Keeping Children Safe Programme [ 15 ]. The project included a Cochrane systematic review that aimed to increase the use of safety equipment to prevent accidents at home in children under five years old. This application is intended to be illustrative of the benefits of new evidence synthesis methods since the previous review. It is not a complete, comprehensive analysis as it only uses a subset of the original dataset and therefore the results are not intended to be used for policy decision making. This example has been chosen as it demonstrates many of the issues in synthesising effectiveness evidence of public health interventions, including different study designs (randomised controlled trials, observational studies and cluster randomised trials), heterogeneity of populations or settings, incomplete individual participant data and complex interventions that contain multiple components.
This analysis will investigate the most effective promotional interventions for the outcome of ‘safe storage of cleaning products’ to prevent childhood poisoning accidents. There are 12 studies included in the dataset, with IPD available from nine of the studies. The covariate, single parent family, is included in the analysis to demonstrate the effect of being a single parent family on the outcome. In this example, all of the interventions are made up of one or more of the following components: education (Ed), free or low cost equipment (Eq), home safety inspection (HSI), and installation of safety equipment (In). A Bayesian approach using WinBUGS was used and therefore credible intervals (CrI) are presented with estimates of the effect sizes [ 16 ].
The original review paper by Achana et al. (2014) demonstrated pairwise meta-analysis and meta-regression using individual and cluster allocated trials, subgroup analyses, meta-regression using individual participant data (IPD) and summary aggregate data and NMA. This paper firstly applies NMA to the motivating example for context, followed by extensions to NMA.
Multiple interventions: lumping or splitting?
Often in public health there are multiple intervention options. However, interventions are often lumped together in a pairwise meta-analysis. Pairwise meta-analysis is a useful tool for two interventions or, alternatively in the presence of lumping interventions, for answering the research question: ‘are interventions in general better than a control or another group of interventions?’. However, when there are multiple interventions, this type of analysis is not appropriate for informing health care providers which intervention should be recommended to the public. ‘Lumping’ is becoming less frequent in other areas of evidence synthesis, such as for clinical interventions, as the use of sophisticated synthesis techniques, such as NMA, increases (Achana et al. 2014) but lumping is still common in public health.
NMA is an extension of the pairwise meta-analysis framework to more than two interventions. Multiple interventions that are lumped into a pairwise meta-analysis are likely to demonstrate high statistical heterogeneity. This does not mean that quantitative synthesis could not be undertaken but that a more appropriate method, NMA, should be implemented. Instead the statistical approach should be based on the research questions of the systematic review. For example, if the research question is ‘are any interventions effective for preventing obesity?’, it would be appropriate to perform a pairwise meta-analysis comparing every intervention in the literature to a control. However, if the research question is ‘which intervention is the most effective for preventing obesity?’, it would be more appropriate and informative to perform a network meta-analysis, which can compare multiple interventions simultaneously and identify the best one.
NMA is a useful statistical method in the context of public health intervention appraisal, where there are often multiple intervention options, as it estimates the relative effectiveness of three or more interventions simultaneously, even if direct study evidence is not available for all intervention comparisons. Using NMA can help to answer the research question ‘what is the effectiveness of each intervention compared to all other interventions in the network?’.
In the motivating example there are six intervention options. The effect of lumping interventions is shown in Fig. 4 , where different interventions in both the intervention and control arms are compared. There is overlap of intervention and control arms across studies and interpretation of the results of a pairwise meta-analysis comparing the effectiveness of the two groups of interventions would not be useful in deciding which intervention to recommend. In comparison, the network plot in Fig. 5 illustrates the evidence base of the prevention of childhood poisonings review comparing six interventions that promote the use of safety equipment in the home. Most of the studies use ‘usual care’ as a baseline and compare this to another intervention. There are also studies in the evidence base that compare pairs of the interventions, such as ‘Education and equipment’ to ‘Equipment’. The plot also demonstrates the absence of direct study evidence between many pairs of interventions, for which the associated treatment effects can be indirectly estimated using NMA.
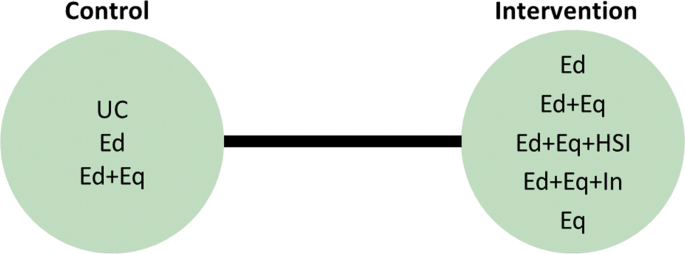
Network plot to illustrate how pairwise meta-analysis groups the interventions in the motivating dataset. Notation UC: Usual care, Ed: Education, Ed+Eq: Education and equipment, Ed+Eq+HSI: Education, equipment, and home safety inspection, Ed+Eq+In: Education, equipment and installation, Eq: Equipment
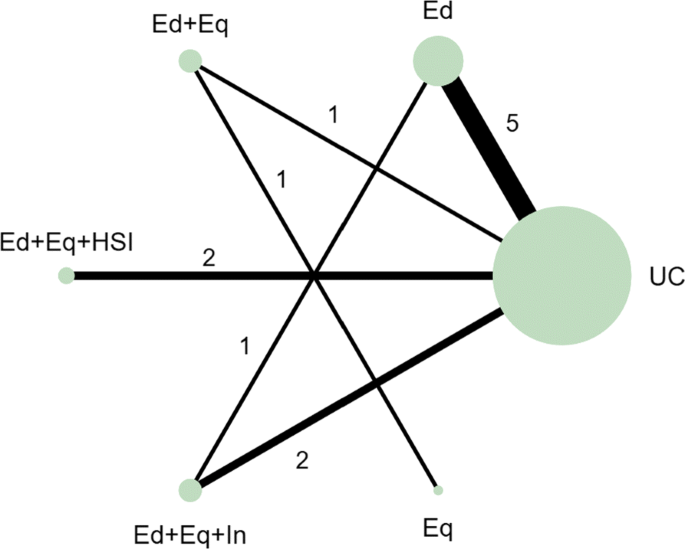
Network plot for the safe storage of cleaning products outcome. Notation UC: Usual care, Ed: Education, Ed+Eq: Education and equipment, Ed+Eq+HSI: Education, equipment, and home safety inspection, Ed+Eq+In: Education, equipment and installation, Eq: Equipment
An NMA was fitted to the motivating example to compare the six interventions in the studies from the review. The results are reported in the ‘triangle table’ in Table 5 [ 17 ]. The top right half of the table shows the direct evidence between pairs of the interventions in the corresponding rows and columns by either pooling the studies as a pairwise meta-analysis or presenting the single study results if evidence is only available from a single study. The bottom left half of the table reports the results of the NMA. The gaps in the top right half of the table arise where no direct study evidence exists to compare the two interventions. For example, there is no direct study evidence comparing ‘Education’ (Ed) to ‘Education, equipment and home safety inspection’ (Ed+Eq+HSI). The NMA, however, can estimate this comparison through the direct study evidence as an odds ratio of 3.80 with a 95% credible interval of (1.16, 12.44). The results suggest that the odds of safely storing cleaning products in the Ed+Eq+HSI intervention group is 3.80 times the odds in the Ed group. The results demonstrate a key benefit of NMA that all intervention effects in a network can be estimated using indirect evidence, even if there is no direct study evidence for some pairwise comparisons. This is based on the consistency assumption (that estimates of intervention effects from direct and indirect evidence are consistent) which should be checked when performing an NMA. This is beyond the scope of this paper and details on this can be found elsewhere [ 18 ].
NMA can also be used to rank the interventions in terms of their effectiveness and estimate the probability that each intervention is likely to be the most effective. This can help to answer the research question ‘which intervention is the best?’ out of all of the interventions that have provided evidence in the network. The rankings and associated probabilities for the motivating example are presented in Table 6 . It can be seen that in this case the ‘education, equipment and home safety inspection’ (Ed+Eq+HSI) intervention is ranked first, with a 0.87 probability of being the best intervention. However, there is overlap of the 95% credible intervals of the median rankings. This overlap reflects the uncertainty in the intervention effect estimates and therefore it is important that the interpretation of these statistics clearly communicates this uncertainty to decision makers.
NMA has the potential to be extremely useful but is underutilised in the evidence synthesis of public health interventions. The ability to compare and rank multiple interventions in an area where there are often multiple intervention options is invaluable in decision making for identifying which intervention to recommend. NMA can also include further literature in the analysis, compared to a pairwise meta-analysis, by expanding the network to improve the uncertainty in the effectiveness estimates.
Statistical heterogeneity
When heterogeneity remains in the results of an NMA, it is useful to explore the reasons for this. Strategies for dealing with heterogeneity involve the inclusion of covariates in a meta-analysis or NMA to adjust for the differences in the covariates across studies [ 19 ]. Meta-regression is a statistical method developed from meta-analysis that includes covariates to potentially explain the between-study heterogeneity ‘with the aim of estimating treatment-covariate interactions’ (Saramago et al. 2012). NMA has been extended to network meta-regression which investigates the effect of trial characteristics on multiple intervention effects. Three ways have been suggested to include covariates in an NMA: single covariate effect, exchangeable covariate effects and independent covariate effects which are discussed in more detail in the NICE Technical Support Document 3 [ 14 ]. This method has the potential to assess the effect of study level covariates on the intervention effects, which is particularly relevant in public health due to the variation across studies.
The most widespread method of meta-regression uses study level data for the inclusion of covariates into meta-regression models. Study level covariate data is when the data from the studies are aggregated, e.g. the proportion of participants in a study that are from single parent families compared to dual parent families. The alternative to study level data is individual participant data (IPD), where the data are available and used as a covariate at the individual level e.g. the parental status of every individual in a study can be used as a covariate. Although IPD is considered to be the gold standard for meta-analysis, aggregated level data is much more commonly used as it is usually available and easily accessible from published research whereas IPD can be hard to obtain from study authors.
There are some limitations to network meta-regression. In our motivating example, using the single parent covariate in a meta-regression would estimate the relative difference in the intervention effects of a population that is made up of 100% single parent families compared to a population that is made up of 100% dual parent families. This interpretation is not as useful as the analysis that uses IPD, which would give the relative difference of the intervention effects in a single parent family compared to a dual parent family. The meta-regression using aggregated data would also be susceptible to ecological bias. Ecological bias is where the effect of the covariate is different at the study level compared to the individual level [ 14 ]. For example, if each study demonstrates a relationship between a covariate and the intervention but the covariate is similar across the studies, a meta-regression of the aggregate data would not demonstrate the effect that is observed within the studies [ 20 ].
Although meta-regression is a useful tool for investigating sources of heterogeneity in the data, caution should be taken when using the results of meta-regression to explain how covariates affect the intervention effects. Meta-regression should only be used to investigate study characteristics, such as the duration of intervention, which will not be susceptible to ecological bias and the interpretation of the results (the effect of intervention duration on intervention effectiveness) would be more meaningful for the development of public health interventions.
Since the covariate of interest in this motivating example is not a study characteristic, meta-regression of aggregated covariate data was not performed. Network meta-regression including IPD and aggregate level data was developed by Samarago et al. (2012) [ 21 ] to overcome the issues with aggregated data network meta-regression, which is discussed in the next section.
Tailored decision making to specific sub-groups
In public health it is important to identify which interventions are best for which people. There has been a recent move towards precision medicine. In the field of public health the ‘concept of precision prevention may [...] be valuable for efficiently targeting preventive strategies to the specific subsets of a population that will derive maximal benefit’ (Khoury and Evans, 2015). Tailoring interventions has the potential to reduce the effect of inequalities in social factors that are influencing the health of the population. Identifying which interventions should be targeted to which subgroups can also lead to better public health outcomes and help to allocate scarce NHS resources. Research interest, therefore, lies in identifying participant level covariate-intervention interactions.
IPD meta-analysis uses data at the individual level to overcome ecological bias. The interpretation of IPD meta-analysis is more relevant in the case of using participant characteristics as covariates since the interpretation of the covariate-intervention interaction is at the individual level rather than the study level. This means that it can answer the research question: ‘which interventions work best in subgroups of the population?’. IPD meta-analyses are considered to be the gold standard for evidence synthesis since it increases the power of the analysis to identify covariate-intervention interactions and it has the ability to reduce the effect of ecological bias compared to aggregated data alone. IPD meta-analysis can also help to overcome scarcity of data issues and has been shown to have higher power and reduce the uncertainty in the estimates compared to analysis including only summary aggregate data [ 22 ].
Despite the advantages of including IPD in a meta-analysis, in reality it is often very time consuming and difficult to collect IPD for all of the studies [ 21 ]. Although data sharing is becoming more common, it remains time consuming and difficult to collect IPD for all studies in a review. This results in IPD being underutilised in meta-analyses. As an intermediate solution, statistical methods have been developed, such as the NMA in Samarago et al. (2012), that incorporates both IPD and aggregate data. Methods that simultaneously include IPD and aggregate level data have been shown to reduce uncertainty in the effect estimates and minimise ecological bias [ 20 , 21 ]. A simulation study by Leahy et al. (2018) found that an increased proportion of IPD resulted in more accurate and precise NMA estimates [ 23 ].
An NMA including IPD, where it is available, was performed, based on the model presented in Samarago et al. (2012) [ 21 ]. The results in Table 7 demonstrates the detail that this type of analysis can provide to base decisions on. More relevant covariate-intervention interaction interpretations can be obtained, for example the regression coefficients for covariate-intervention interactions are the individual level covariate intervention interactions or the ‘within study interactions’ that are interpreted as the effect of being in a single parent family on the effectiveness of each of the interventions. For example, the effect of Ed+Eq compared to UC in a single parent family is 1.66 times the effect of Ed+Eq compared to UC in a dual parent family but this is not an important difference as the credible interval crosses 1. The regression coefficients for the study level covariate-intervention interactions or the ‘between study interactions’ can be interpreted as the relative difference in the intervention effects of a population that is made up of 100% single parent families compared to a population that is made up of 100% dual parent families.
- Complex interventions
In many public health research settings the complex interventions are comprised of a number of components. An NMA can compare all of the interventions in a network as they are implemented in the original trials. However, NMA does not tell us which components of the complex intervention are attributable to this effect. It could be that particular components, or the interacting effect of multiple components, are driving the effectiveness and other components are not as effective. Often, trials have not directly compared every combination of components as there are so many component combination options, it would be inefficient and impractical. Component NMA was developed by Welton et al. (2009) to estimate the effect of each component of the complex interventions and combination of components in a network, in the absence of direct trial evidence and answers the question: ‘are interventions with a particular component or combination of components effective?’ [ 11 ]. For example, for the motivating example, in comparison to Fig. 5 , which demonstrates the interventions that an NMA can estimate effectiveness, Fig. 6 demonstrates all of the possible interventions of which the effectiveness can be estimated in a component NMA, given the components present in the network.
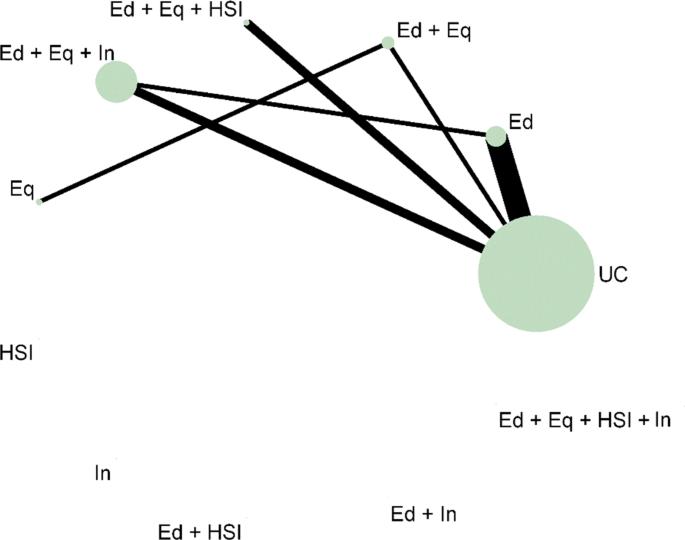
Network plot that illustrates how component network meta-analysis can estimate the effectiveness of intervention components and combinations of components, even when they are not included in the direct evidence. Notation UC: Usual care, Ed: Education, Eq: Equipment, Installation, Ed+Eq: Education and equipment, Ed+HSI: Education and home safety inspection, Ed+In: Education and installation, Eq+HSI: Equipment and home safety inspection, Eq+In: equipment and installation, HSI+In: Home safety inspection and installation, Ed+Eq+HSI: Education, equipment, and home safety inspection, Ed+Eq+In: Education, equipment and installation, Eq+HSI+In: Equipment, home safety inspection and installation, Ed+Eq+HSI+In: Education, equipment, home safety inspection and installation
The results of the analyses of the main effects, two way effects and full effects models are shown in Table 8 . The models, proposed in the original paper by Welton et al. (2009), increase in complexity as the assumptions regarding the component effects relax [ 24 ]. The main effects component NMA assumes that the components in the interventions each have separate, independent effects and intervention effects are the sum of the component effects. The two-way effects models assumes that there are interactions between pairs of the components, so the effects of the interventions are more than the sum of the effects. The full effects model assumes that all of the components and combinations of the components interact. Component NMA did not provide further insight into which components are likely to be the most effective since all of the 95% credible intervals were very wide and overlapped 1. There is a lot of uncertainty in the results, particularly in the 2-way and full effects models. A limitation of component NMA is that there are issues with uncertainty when data is scarce. However, the results demonstrate the potential of component NMA as a useful tool to gain better insights from the available dataset.
In practice, this method has rarely been used since its development [ 24 – 26 ]. It may be challenging to define the components in some areas of public health where many interventions have been studied. However, the use of meta-analysis for planning future studies is rarely discussed and component NMA would provide a useful tool for identifying new component combinations that may be more effective [ 27 ]. This type of analysis has the potential to prioritise future public health research, which is especially useful where there are multiple intervention options, and identify more effective interventions to recommend to the public.
Further methods / other outcomes
The analysis and methods described in this paper only cover a small subset of the methods that have been developed in meta-analysis in recent years. Methods that aim to assess the quality of evidence supporting a NMA and how to quantify how much the evidence could change due to potential biases or sampling variation before the recommendation changes have been developed [ 28 , 29 ]. Models adjusting for baseline risk have been developed to allow for different study populations to have different levels of underlying risk, by using the observed event rate in the control arm [ 30 , 31 ]. Multivariate methods can be used to compare the effect of multiple interventions on two or more outcomes simultaneously [ 32 ]. This area of methodological development is especially appealing within public health where studies assess a broad range of health effects and typically have multiple outcome measures. Multivariate methods offer benefits over univariate models by allowing the borrowing of information across outcomes and modelling the relationships between outcomes which can potentially reduce the uncertainty in the effect estimates [ 33 ]. Methods have also been developed to evaluate interventions with classes or different intervention intensities, known as hierarchical interventions [ 34 ]. These methods were not demonstrated in this paper but can also be useful tools for addressing challenges of appraising public health interventions, such as multiple and surrogate outcomes.
This paper only considered an example with a binary outcome. All of the methods described have also been adapted for other outcome measures. For example, the Technical Support Document 2 proposed a Bayesian generalised linear modelling framework to synthesise other outcome measures. More information and models for continuous and time-to-event data is available elsewhere [ 21 , 35 – 38 ].
Software and guidelines
In the previous section, meta-analytic methods that answer more policy relevant questions were demonstrated. However, as shown by the update to the review, methods such as these are still under-utilised. It is suspected from the NICE public health review that one of the reasons for the lack of uptake of methods in public health could be due to common software choices, such as RevMan, being limited in their flexibility for statistical methods.
Table 9 provides a list of software options and guidance documents that are more flexible than RevMan for implementing the statistical methods illustrated in the previous section to make these methods more accessible to researchers.
In this paper, the network plot in Figs. 5 and 6 were produced using the networkplot command from the mvmeta package [ 39 ] in Stata [ 61 ]. WinBUGS was used to fit the NMA in this paper by adapting the code in the book ‘Evidence Synthesis for Decision Making in Healthcare’ which also provides more detail on Bayesian methods and assessing convergence of Bayesian models [ 45 ]. The model for including IPD and summary aggregate data in an NMA was based on the code in the paper by Saramago et al. (2012). The component NMA in this paper was performed in WinBUGS through R2WinBUGS, [ 47 ] using the code in Welton et al. (2009) [ 11 ].
WinBUGS is a flexible tool for fitting complex models in a Bayesian framework. The NICE Decision Support Unit produced a series of Evidence Synthesis Technical Support Documents [ 46 ] that provide a comprehensive technical guide to methods for evidence synthesis and WinBUGS code is also provided for many of the models. Complex models can also be performed in a frequentist framework. Code and commands for many models are available in R and STATA (see Table 9 ).
The software, R2WinBUGS, was used in the analysis of the motivating example. Increasing numbers of researchers are using R and so packages that can be used to link the two softwares by calling BUGS models in R, packages such as R2WinBUGS, can improve the accessibility of Bayesian methods [ 47 ]. The new R package, BUGSnet, may also help to facilitate the accessibility and improve the reporting of Bayesian NMA [ 48 ]. Webtools have also been developed as a means of enabling researchers to undertake increasingly complex analyses [ 52 , 53 ]. Webtools provide a user-friendly interface to perform statistical analyses and often help in the reporting of the analyses by producing plots, including network plots and forest plots. These tools are very useful for researchers that have a good understanding of the statistical methods they want to implement as part of their review but are inexperienced in statistical software.
This paper has reviewed NICE public health intervention guidelines to identify the methods that are currently being used to synthesise effectiveness evidence to inform public health decision making. A previous review from 2012 was updated to see how method utilisation has changed. Methods have been developed since the previous review and these were applied to an example dataset to show how methods can answer more policy relevant questions. Resources and guidelines for implementing these methods were signposted to encourage uptake.
The review found that the proportion of NICE guidelines containing effectiveness evidence summarised using meta-analysis methods has increased since the original review, but remains low. The majority of the reviews presented only narrative summaries of the evidence - a similar result to the original review. In recent years, there has been an increased awareness of the need to improve decision making by using all of the available evidence. As a result, this has led to the development of new methods, easier application in standard statistical software packages, and guidance documents. Based on this, it would have been expected that their implementation would rise in recent years to reflect this, but the results of the review update showed no such increasing pattern.
A high proportion of NICE guideline reports did not provide a reason for not applying quantitative evidence synthesis methods. Possible explanations for this could be time or resource constraints, lack of statistical expertise, being unaware of the available methods or poor reporting. Reporting guidelines, such as the Preferred Reporting Items for Systematic Reviews and Meta-Analyses (PRISMA), should be updated to emphasise the importance of documenting reasons for not applying methods, as this can direct future research to improve uptake.
Where it was specified, the most common reported reason for not conducting a meta-analysis was heterogeneity. Often in public health, the data is heterogeneous due to the differences between studies in population, design, interventions or outcomes. A common misconception is that the presence of heterogeneity implies that it is not possible to pool the data. Meta-analytic methods can be used to investigate the sources of heterogeneity, as demonstrated in the NMA of the motivating example, and the use of IPD is recommended where possible to improve the precision of the results and reduce the effect of ecological bias. Although caution should be exercised in the interpretation of the results, quantitative synthesis methods provide a stronger basis for making decisions than narrative accounts because they explicitly quantify the heterogeneity and seek to explain it where possible.
The review also found that the most common software to perform the synthesis was RevMan. RevMan is very limited in its ability to perform advanced statistical analyses, beyond that of pairwise meta-analysis, which might explain the above findings. Standard software code is being developed to help make statistical methodology and application more accessible and guidance documents are becoming increasingly available.
The evaluation of public health interventions can be problematic due to the number and complexity of the interventions. NMA methods were applied to a real Cochrane public health review dataset. The methods that were demonstrated showed ways to address some of these issues, including the use of NMA for multiple interventions, the inclusion of covariates as both aggregated data and IPD to explain heterogeneity, and the extension to component network meta-analysis for guiding future research. These analyses illustrated how the choice of synthesis methods can enable more informed decision making by allowing more distinct interventions, and combinations of intervention components, to be defined and their effectiveness estimated. It also demonstrated the potential to target interventions to population subgroups where they are likely to be most effective. However, the application of component NMA to the motivating example has also demonstrated the issues around uncertainty if there are a limited number of studies observing the interventions and intervention components.
The application of methods to the motivating example demonstrated a key benefit of using statistical methods in a public health context compared to only presenting a narrative review – the methods provide a quantitative estimate of the effectiveness of the interventions. The uncertainty from the credible intervals can be used to demonstrate the lack of available evidence. In the context of decision making, having pooled estimates makes it much easier for decision makers to assess the effectiveness of the interventions or identify when more research is required. The posterior distribution of the pooled results from the evidence synthesis can also be incorporated into a comprehensive decision analytic model to determine cost-effectiveness [ 62 ]. Although narrative reviews are useful for describing the evidence base, the results are very difficult to summarise in a decision context.
Although heterogeneity seems to be inevitable within public health interventions due to their complex nature, this review has shown that it is still the main reported reason for not using statistical methods in evidence synthesis. This may be due to guidelines that were originally developed for clinical treatments that are tested in randomised conditions still being applied in public health settings. Guidelines for the choice of methods used in public health intervention appraisals could be updated to take into account the complexities and wide ranging areas in public health. Sophisticated methods may be more appropriate in some cases than simpler models for modelling multiple, complex interventions and their uncertainty, given the limitations are also fully reported [ 19 ]. Synthesis may not be appropriate if statistical heterogeneity remains after adjustment for possible explanatory covariates but details of exploratory analysis and reasons for not synthesising the data should be reported. Future research should focus on the application and dissemination of the advantages of using more advanced methods in public health, identifying circumstances where these methods are likely to be the most beneficial, and ways to make the methods more accessible, for example, the development of packages and web tools.
There is an evident need to facilitate the translation of the synthesis methods into a public health context and encourage the use of methods to improve decision making. This review has shown that the uptake of statistical methods for evaluating the effectiveness of public health interventions is slow, despite advances in methods that address specific issues in public health intervention appraisal and the publication of guidance documents to complement their application.
Availability of data and materials
The dataset supporting the conclusions of this article is included within the article.
Abbreviations
National institute for health and care excellence
- Network meta-analysis
Individual participant data
Home safety inspection
Installation
Credible interval
Preferred reporting items for systematic reviews and meta-analyses
Dias S, Welton NJ, Sutton AJ, Ades A. NICE DSU Technical Support Document 2: A Generalised Linear Modelling Framework for Pairwise and Network Meta-Analysis of Randomised Controlled Trials: National Institute for Health and Clinical Excellence; 2011, p. 98. (Technical Support Document in Evidence Synthesis; TSD2).
Higgins JPT, López-López JA, Becker BJ, et al.Synthesising quantitative evidence in systematic reviews of complex health interventions. BMJ Global Health. 2019; 4(Suppl 1):e000858. https://doi.org/10.1136/bmjgh-2018-000858 .
Article PubMed PubMed Central Google Scholar
Achana F, Hubbard S, Sutton A, Kendrick D, Cooper N. An exploration of synthesis methods in public health evaluations of interventions concludes that the use of modern statistical methods would be beneficial. J Clin Epidemiol. 2014; 67(4):376–90.
Article PubMed Google Scholar
Craig P, Dieppe P, Macintyre S, Michie S, Nazareth I, Petticrew M. Developing and evaluating complex interventions: the new medical research council guidance. Int J Nurs Stud. 2013; 50(5):587–92.
Caldwell DM, Welton NJ. Approaches for synthesising complex mental health interventions in meta-analysis. Evidence-Based Mental Health. 2016; 19(1):16–21.
Melendez-Torres G, Bonell C, Thomas J. Emergent approaches to the meta-analysis of multiple heterogeneous complex interventions. BMC Med Res Methodol. 2015; 15(1):47.
Article CAS PubMed PubMed Central Google Scholar
NICE. NICE: Who We Are. https://www.nice.org.uk/about/who-we-are . Accessed 19 Sept 2019.
Kelly M, Morgan A, Ellis S, Younger T, Huntley J, Swann C. Evidence based public health: a review of the experience of the national institute of health and clinical excellence (NICE) of developing public health guidance in England. Soc Sci Med. 2010; 71(6):1056–62.
NICE. Developing NICE Guidelines: The Manual. https://www.nice.org.uk/process/pmg20/chapter/introduction-and-overview . Accessed 19 Sept 2019.
NICE. Public Health Guidance. https://www.nice.org.uk/guidance/published?type=ph . Accessed 19 Sept 2019.
Welton NJ, Caldwell D, Adamopoulos E, Vedhara K. Mixed treatment comparison meta-analysis of complex interventions: psychological interventions in coronary heart disease. Am J Epidemiol. 2009; 169(9):1158–65.
Ioannidis JP, Patsopoulos NA, Rothstein HR. Reasons or excuses for avoiding meta-analysis in forest plots. BMJ. 2008; 336(7658):1413–5.
Higgins JP, Thompson SG. Quantifying heterogeneity in a meta-analysis. Stat Med. 2002; 21(11):1539–58.
Article Google Scholar
Dias S, Sutton A, Welton N, Ades A. NICE DSU Technical Support Document 3: Heterogeneity: Subgroups, Meta-Regression, Bias and Bias-Adjustment: National Institute for Health and Clinical Excellence; 2011, p. 76.
Kendrick D, Ablewhite J, Achana F, et al.Keeping Children Safe: a multicentre programme of research to increase the evidence base for preventing unintentional injuries in the home in the under-fives. Southampton: NIHR Journals Library; 2017.
Google Scholar
Lunn DJ, Thomas A, Best N, et al.WinBUGS - A Bayesian modelling framework: Concepts, structure, and extensibility. Stat Comput. 2000; 10:325–37. https://doi.org/10.1023/A:1008929526011 .
Dias S, Caldwell DM. Network meta-analysis explained. Arch Dis Child Fetal Neonatal Ed. 2019; 104(1):8–12. https://doi.org/10.1136/archdischild-2018-315224. http://arxiv.org/abs/https://fn.bmj.com/content/104/1/F8.full.pdf.
Dias S, Welton NJ, Sutton AJ, Caldwell DM, Lu G, Ades A. NICE DSU Technical Support Document 4: Inconsistency in Networks of Evidence Based on Randomised Controlled Trials: National Institute for Health and Clinical Excellence; 2011. (NICE DSU Technical Support Document in Evidence Synthesis; TSD4).
Cipriani A, Higgins JP, Geddes JR, Salanti G. Conceptual and technical challenges in network meta-analysis. Ann Intern Med. 2013; 159(2):130–7.
Riley RD, Steyerberg EW. Meta-analysis of a binary outcome using individual participant data and aggregate data. Res Synth Methods. 2010; 1(1):2–19.
Saramago P, Sutton AJ, Cooper NJ, Manca A. Mixed treatment comparisons using aggregate and individual participant level data. Stat Med. 2012; 31(28):3516–36.
Lambert PC, Sutton AJ, Abrams KR, Jones DR. A comparison of summary patient-level covariates in meta-regression with individual patient data meta-analysis. J Clin Epidemiol. 2002; 55(1):86–94.
Article CAS PubMed Google Scholar
Leahy J, O’Leary A, Afdhal N, Gray E, Milligan S, Wehmeyer MH, Walsh C. The impact of individual patient data in a network meta-analysis: an investigation into parameter estimation and model selection. Res Synth Methods. 2018; 9(3):441–69.
Freeman SC, Scott NW, Powell R, Johnston M, Sutton AJ, Cooper NJ. Component network meta-analysis identifies the most effective components of psychological preparation for adults undergoing surgery under general anesthesia. J Clin Epidemiol. 2018; 98:105–16.
Pompoli A, Furukawa TA, Efthimiou O, Imai H, Tajika A, Salanti G. Dismantling cognitive-behaviour therapy for panic disorder: a systematic review and component network meta-analysis. Psychol Med. 2018; 48(12):1945–53.
Rücker G, Schmitz S, Schwarzer G. Component network meta-analysis compared to a matching method in a disconnected network: A case study. Biom J. 2020. https://doi.org/10.1002/bimj.201900339 .
Efthimiou O, Debray TP, van Valkenhoef G, Trelle S, Panayidou K, Moons KG, Reitsma JB, Shang A, Salanti G, Group GMR. GetReal in network meta-analysis: a review of the methodology. Res Synth Methods. 2016; 7(3):236–63.
Salanti G, Del Giovane C, Chaimani A, Caldwell DM, Higgins JP. Evaluating the quality of evidence from a network meta-analysis. PLoS ONE. 2014; 9(7):99682.
Article CAS Google Scholar
Phillippo DM, Dias S, Welton NJ, Caldwell DM, Taske N, Ades A. Threshold analysis as an alternative to grade for assessing confidence in guideline recommendations based on network meta-analyses. Ann Intern Med. 2019; 170(8):538–46.
Dias S, Welton NJ, Sutton AJ, Ades AE. NICE DSU Technical Support Document 5: Evidence Synthesis in the Baseline Natural History Model: National Institute for Health and Clinical Excellence; 2011, p. 29. (NICE DSU Technical Support Document in Evidence Synthesis; TSD5).
Achana FA, Cooper NJ, Dias S, Lu G, Rice SJ, Kendrick D, Sutton AJ. Extending methods for investigating the relationship between treatment effect and baseline risk from pairwise meta-analysis to network meta-analysis. Stat Med. 2013; 32(5):752–71.
Riley RD, Jackson D, Salanti G, Burke DL, Price M, Kirkham J, White IR. Multivariate and network meta-analysis of multiple outcomes and multiple treatments: rationale, concepts, and examples. BMJ (Clinical research ed.) 2017; 358:j3932. https://doi.org/10.1136/bmj.j3932 .
Achana FA, Cooper NJ, Bujkiewicz S, Hubbard SJ, Kendrick D, Jones DR, Sutton AJ. Network meta-analysis of multiple outcome measures accounting for borrowing of information across outcomes. BMC Med Res Methodol. 2014; 14(1):92.
Owen RK, Tincello DG, Keith RA. Network meta-analysis: development of a three-level hierarchical modeling approach incorporating dose-related constraints. Value Health. 2015; 18(1):116–26.
Jansen JP. Network meta-analysis of individual and aggregate level data. Res Synth Methods. 2012; 3(2):177–90.
Donegan S, Williamson P, D’Alessandro U, Garner P, Smith CT. Combining individual patient data and aggregate data in mixed treatment comparison meta-analysis: individual patient data may be beneficial if only for a subset of trials. Stat Med. 2013; 32(6):914–30.
Saramago P, Chuang L-H, Soares MO. Network meta-analysis of (individual patient) time to event data alongside (aggregate) count data. BMC Med Res Methodol. 2014; 14(1):105.
Thom HH, Capkun G, Cerulli A, Nixon RM, Howard LS. Network meta-analysis combining individual patient and aggregate data from a mixture of study designs with an application to pulmonary arterial hypertension. BMC Med Res Methodol. 2015; 15(1):34.
Gasparrini A, Armstrong B, Kenward MG. Multivariate meta-analysis for non-linear and other multi-parameter associations. Stat Med. 2012; 31(29):3821–39.
Chaimani A, Higgins JP, Mavridis D, Spyridonos P, Salanti G. Graphical tools for network meta-analysis in stata. PLoS ONE. 2013; 8(10):76654.
Rücker G, Schwarzer G, Krahn U, König J. netmeta: Network meta-analysis with R. R package version 0.5-0. 2014. R package version 0.5-0. Availiable: http://CRAN.R-project.org/package=netmeta .
van Valkenhoef G, Kuiper J. gemtc: Network Meta-Analysis Using Bayesian Methods. R package version 0.8-2. 2016. Available online at: https://CRAN.R-project.org/package=gemtc .
Lin L, Zhang J, Hodges JS, Chu H. Performing arm-based network meta-analysis in R with the pcnetmeta package. J Stat Softw. 2017; 80(5):1–25. https://doi.org/10.18637/jss.v080.i05 .
Rücker G, Schwarzer G. Automated drawing of network plots in network meta-analysis. Res Synth Methods. 2016; 7(1):94–107.
Welton NJ, Sutton AJ, Cooper N, Abrams KR, Ades A. Evidence Synthesis for Decision Making in Healthcare, vol. 132. UK: Wiley; 2012.
Book Google Scholar
Dias S, Welton NJ, Sutton AJ, Ades AE. Evidence synthesis for decision making 1: introduction. Med Decis Making Int J Soc Med Decis Making. 2013; 33(5):597–606. https://doi.org/10.1177/0272989X13487604 .
Sturtz S, Ligges U, Gelman A. R2WinBUGS: a package for running WinBUGS from R. J Stat Softw. 2005; 12(3):1–16.
Béliveau A, Boyne DJ, Slater J, Brenner D, Arora P. Bugsnet: an r package to facilitate the conduct and reporting of bayesian network meta-analyses. BMC Med Res Methodol. 2019; 19(1):196.
Neupane B, Richer D, Bonner AJ, Kibret T, Beyene J. Network meta-analysis using R: a review of currently available automated packages. PLoS ONE. 2014; 9(12):115065.
White IR. Multivariate random-effects meta-analysis. Stata J. 2009; 9(1):40–56.
Chaimani A, Salanti G. Visualizing assumptions and results in network meta-analysis: the network graphs package. Stata J. 2015; 15(4):905–50.
Owen RK, Bradbury N, Xin Y, Cooper N, Sutton A. MetaInsight: An interactive web-based tool for analyzing, interrogating, and visualizing network meta-analyses using R-shiny and netmeta. Res Synth Methods. 2019; 10(4):569–81. https://doi.org/10.1002/jrsm.1373 .
Freeman SC, Kerby CR, Patel A, Cooper NJ, Quinn T, Sutton AJ. Development of an interactive web-based tool to conduct and interrogate meta-analysis of diagnostic test accuracy studies: MetaDTA. BMC Med Res Methodol. 2019; 19(1):81.
Nikolakopoulou A, Higgins JPT, Papakonstantinou T, Chaimani A, Del Giovane C, Egger M, Salanti G. CINeMA: An approach for assessing confidence in the results of a network meta-analysis. PLoS Med. 2020; 17(4):e1003082. https://doi.org/10.1371/journal.pmed.1003082 .
Viechtbauer W. Conducting meta-analyses in R with the metafor package. J Stat Softw. 2010; 36(3):1–48.
Freeman SC, Carpenter JR. Bayesian one-step ipd network meta-analysis of time-to-event data using royston-parmar models. Res Synth Methods. 2017; 8(4):451–64.
Riley RD, Lambert PC, Staessen JA, Wang J, Gueyffier F, Thijs L, Boutitie F. Meta-analysis of continuous outcomes combining individual patient data and aggregate data. Stat Med. 2008; 27(11):1870–93.
Debray TP, Moons KG, van Valkenhoef G, Efthimiou O, Hummel N, Groenwold RH, Reitsma JB, Group GMR. Get real in individual participant data (ipd) meta-analysis: a review of the methodology. Res Synth Methods. 2015; 6(4):293–309.
Tierney JF, Vale C, Riley R, Smith CT, Stewart L, Clarke M, Rovers M. Individual Participant Data (IPD) Meta-analyses of Randomised Controlled Trials: Guidance on Their Use. PLoS Med. 2015; 12(7):e1001855. https://doi.org/10.1371/journal.pmed.1001855 .
Stewart LA, Clarke M, Rovers M, Riley RD, Simmonds M, Stewart G, Tierney JF. Preferred reporting items for a systematic review and meta-analysis of individual participant data: the prisma-ipd statement. JAMA. 2015; 313(16):1657–65.
StataCorp. Stata Statistical Software: Release 16. College Station: StataCorp LLC; 2019.
Cooper NJ, Sutton AJ, Abrams KR, Turner D, Wailoo A. Comprehensive decision analytical modelling in economic evaluation: a bayesian approach. Health Econ. 2004; 13(3):203–26.
Download references
Acknowledgements
We would like to acknowledge Professor Denise Kendrick as the lead on the NIHR Keeping Children Safe at Home Programme that originally funded the collection of the evidence for the motivating example and some of the analyses illustrated in the paper.
ES is funded by a National Institute for Health Research (NIHR), Doctoral Research Fellow for this research project. This paper presents independent research funded by the National Institute for Health Research (NIHR). The views expressed are those of the authors and not necessarily those of the NHS, the NIHR or the Department of Health and Social Care. The funding bodies played no role in the design of the study and collection, analysis, and interpretation of data and in writing the manuscript.
Author information
Authors and affiliations.
Department of Health Sciences, University of Leicester, Lancaster Road, Leicester, UK
Ellesha A. Smith, Nicola J. Cooper, Alex J. Sutton, Keith R. Abrams & Stephanie J. Hubbard
You can also search for this author in PubMed Google Scholar
Contributions
ES performed the review, analysed the data and wrote the paper. SH supervised the project. SH, KA, NC and AS provided substantial feedback on the manuscript. All authors have read and approved the manuscript.
Corresponding author
Correspondence to Ellesha A. Smith .
Ethics declarations
Ethics approval and consent to participate.
Not applicable.
Consent for publication
Competing interests.
KA is supported by Health Data Research (HDR) UK, the UK National Institute for Health Research (NIHR) Applied Research Collaboration East Midlands (ARC EM), and as a NIHR Senior Investigator Emeritus (NF-SI-0512-10159). The views expressed are those of the author(s) and not necessarily those of the NIHR or the Department of Health and Social Care. KA has served as a paid consultant, providing unrelated methodological advice, to; Abbvie, Amaris, Allergan, Astellas, AstraZeneca, Boehringer Ingelheim, Bristol-Meyers Squibb, Creativ-Ceutical, GSK, ICON/Oxford Outcomes, Ipsen, Janssen, Eli Lilly, Merck, NICE, Novartis, NovoNordisk, Pfizer, PRMA, Roche and Takeda, and has received research funding from Association of the British Pharmaceutical Industry (ABPI), European Federation of Pharmaceutical Industries & Associations (EFPIA), Pfizer, Sanofi and Swiss Precision Diagnostics. He is a Partner and Director of Visible Analytics Limited, a healthcare consultancy company.
Additional information
Publisher’s note.
Springer Nature remains neutral with regard to jurisdictional claims in published maps and institutional affiliations.
Supplementary Information
Additional file 1.
Key for the Nice public health guideline codes. Available in NICEGuidelinesKey.xlsx .
Additional file 2
NICE public health intervention guideline review flowchart for the inclusion and exclusion of documents. Available in Flowchart.JPG .
Rights and permissions
Open Access This article is licensed under a Creative Commons Attribution 4.0 International License, which permits use, sharing, adaptation, distribution and reproduction in any medium or format, as long as you give appropriate credit to the original author(s) and the source, provide a link to the Creative Commons licence, and indicate if changes were made. The images or other third party material in this article are included in the article’s Creative Commons licence, unless indicated otherwise in a credit line to the material. If material is not included in the article’s Creative Commons licence and your intended use is not permitted by statutory regulation or exceeds the permitted use, you will need to obtain permission directly from the copyright holder. To view a copy of this licence, visit http://creativecommons.org/licenses/by/4.0/ . The Creative Commons Public Domain Dedication waiver ( http://creativecommons.org/publicdomain/zero/1.0/ ) applies to the data made available in this article, unless otherwise stated in a credit line to the data.
Reprints and permissions
About this article
Cite this article.
Smith, E.A., Cooper, N.J., Sutton, A.J. et al. A review of the quantitative effectiveness evidence synthesis methods used in public health intervention guidelines. BMC Public Health 21 , 278 (2021). https://doi.org/10.1186/s12889-021-10162-8
Download citation
Received : 22 September 2020
Accepted : 04 January 2021
Published : 03 February 2021
DOI : https://doi.org/10.1186/s12889-021-10162-8
Share this article
Anyone you share the following link with will be able to read this content:
Sorry, a shareable link is not currently available for this article.
Provided by the Springer Nature SharedIt content-sharing initiative
- Meta-analysis
- Systematic review
- Public health
- Decision making
- Evidence synthesis
BMC Public Health
ISSN: 1471-2458
- General enquiries: [email protected]
Click through the PLOS taxonomy to find articles in your field.
For more information about PLOS Subject Areas, click here .
Loading metrics
Open Access
Peer-reviewed
Research Article
Recent quantitative research on determinants of health in high income countries: A scoping review
Roles Conceptualization, Data curation, Formal analysis, Investigation, Methodology, Project administration, Software, Visualization, Writing – original draft, Writing – review & editing
* E-mail: [email protected]
Affiliation Centre for Health Economics Research and Modelling Infectious Diseases, Vaccine and Infectious Disease Institute, University of Antwerp, Antwerp, Belgium

Roles Conceptualization, Data curation, Funding acquisition, Project administration, Resources, Supervision, Validation, Visualization, Writing – review & editing
- Vladimira Varbanova,
- Philippe Beutels
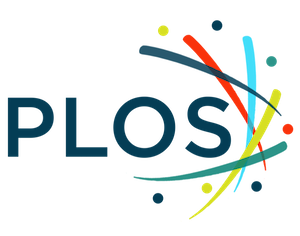
- Published: September 17, 2020
- https://doi.org/10.1371/journal.pone.0239031
- Peer Review
- Reader Comments
Identifying determinants of health and understanding their role in health production constitutes an important research theme. We aimed to document the state of recent multi-country research on this theme in the literature.
We followed the PRISMA-ScR guidelines to systematically identify, triage and review literature (January 2013—July 2019). We searched for studies that performed cross-national statistical analyses aiming to evaluate the impact of one or more aggregate level determinants on one or more general population health outcomes in high-income countries. To assess in which combinations and to what extent individual (or thematically linked) determinants had been studied together, we performed multidimensional scaling and cluster analysis.
Sixty studies were selected, out of an original yield of 3686. Life-expectancy and overall mortality were the most widely used population health indicators, while determinants came from the areas of healthcare, culture, politics, socio-economics, environment, labor, fertility, demographics, life-style, and psychology. The family of regression models was the predominant statistical approach. Results from our multidimensional scaling showed that a relatively tight core of determinants have received much attention, as main covariates of interest or controls, whereas the majority of other determinants were studied in very limited contexts. We consider findings from these studies regarding the importance of any given health determinant inconclusive at present. Across a multitude of model specifications, different country samples, and varying time periods, effects fluctuated between statistically significant and not significant, and between beneficial and detrimental to health.
Conclusions
We conclude that efforts to understand the underlying mechanisms of population health are far from settled, and the present state of research on the topic leaves much to be desired. It is essential that future research considers multiple factors simultaneously and takes advantage of more sophisticated methodology with regards to quantifying health as well as analyzing determinants’ influence.
Citation: Varbanova V, Beutels P (2020) Recent quantitative research on determinants of health in high income countries: A scoping review. PLoS ONE 15(9): e0239031. https://doi.org/10.1371/journal.pone.0239031
Editor: Amir Radfar, University of Central Florida, UNITED STATES
Received: November 14, 2019; Accepted: August 28, 2020; Published: September 17, 2020
Copyright: © 2020 Varbanova, Beutels. This is an open access article distributed under the terms of the Creative Commons Attribution License , which permits unrestricted use, distribution, and reproduction in any medium, provided the original author and source are credited.
Data Availability: All relevant data are within the manuscript and its Supporting Information files.
Funding: This study (and VV) is funded by the Research Foundation Flanders ( https://www.fwo.be/en/ ), FWO project number G0D5917N, award obtained by PB. The funder had no role in study design, data collection and analysis, decision to publish, or preparation of the manuscript.
Competing interests: The authors have declared that no competing interests exist.
Introduction
Identifying the key drivers of population health is a core subject in public health and health economics research. Between-country comparative research on the topic is challenging. In order to be relevant for policy, it requires disentangling different interrelated drivers of “good health”, each having different degrees of importance in different contexts.
“Good health”–physical and psychological, subjective and objective–can be defined and measured using a variety of approaches, depending on which aspect of health is the focus. A major distinction can be made between health measurements at the individual level or some aggregate level, such as a neighborhood, a region or a country. In view of this, a great diversity of specific research topics exists on the drivers of what constitutes individual or aggregate “good health”, including those focusing on health inequalities, the gender gap in longevity, and regional mortality and longevity differences.
The current scoping review focuses on determinants of population health. Stated as such, this topic is quite broad. Indeed, we are interested in the very general question of what methods have been used to make the most of increasingly available region or country-specific databases to understand the drivers of population health through inter-country comparisons. Existing reviews indicate that researchers thus far tend to adopt a narrower focus. Usually, attention is given to only one health outcome at a time, with further geographical and/or population [ 1 , 2 ] restrictions. In some cases, the impact of one or more interventions is at the core of the review [ 3 – 7 ], while in others it is the relationship between health and just one particular predictor, e.g., income inequality, access to healthcare, government mechanisms [ 8 – 13 ]. Some relatively recent reviews on the subject of social determinants of health [ 4 – 6 , 14 – 17 ] have considered a number of indicators potentially influencing health as opposed to a single one. One review defines “social determinants” as “the social, economic, and political conditions that influence the health of individuals and populations” [ 17 ] while another refers even more broadly to “the factors apart from medical care” [ 15 ].
In the present work, we aimed to be more inclusive, setting no limitations on the nature of possible health correlates, as well as making use of a multitude of commonly accepted measures of general population health. The goal of this scoping review was to document the state of the art in the recent published literature on determinants of population health, with a particular focus on the types of determinants selected and the methodology used. In doing so, we also report the main characteristics of the results these studies found. The materials collected in this review are intended to inform our (and potentially other researchers’) future analyses on this topic. Since the production of health is subject to the law of diminishing marginal returns, we focused our review on those studies that included countries where a high standard of wealth has been achieved for some time, i.e., high-income countries belonging to the Organisation for Economic Co-operation and Development (OECD) or Europe. Adding similar reviews for other country income groups is of limited interest to the research we plan to do in this area.
In view of its focus on data and methods, rather than results, a formal protocol was not registered prior to undertaking this review, but the procedure followed the guidelines of the PRISMA statement for scoping reviews [ 18 ].
We focused on multi-country studies investigating the potential associations between any aggregate level (region/city/country) determinant and general measures of population health (e.g., life expectancy, mortality rate).
Within the query itself, we listed well-established population health indicators as well as the six world regions, as defined by the World Health Organization (WHO). We searched only in the publications’ titles in order to keep the number of hits manageable, and the ratio of broadly relevant abstracts over all abstracts in the order of magnitude of 10% (based on a series of time-focused trial runs). The search strategy was developed iteratively between the two authors and is presented in S1 Appendix . The search was performed by VV in PubMed and Web of Science on the 16 th of July, 2019, without any language restrictions, and with a start date set to the 1 st of January, 2013, as we were interested in the latest developments in this area of research.
Eligibility criteria
Records obtained via the search methods described above were screened independently by the two authors. Consistency between inclusion/exclusion decisions was approximately 90% and the 43 instances where uncertainty existed were judged through discussion. Articles were included subject to meeting the following requirements: (a) the paper was a full published report of an original empirical study investigating the impact of at least one aggregate level (city/region/country) factor on at least one health indicator (or self-reported health) of the general population (the only admissible “sub-populations” were those based on gender and/or age); (b) the study employed statistical techniques (calculating correlations, at the very least) and was not purely descriptive or theoretical in nature; (c) the analysis involved at least two countries or at least two regions or cities (or another aggregate level) in at least two different countries; (d) the health outcome was not differentiated according to some socio-economic factor and thus studied in terms of inequality (with the exception of gender and age differentiations); (e) mortality, in case it was one of the health indicators under investigation, was strictly “total” or “all-cause” (no cause-specific or determinant-attributable mortality).
Data extraction
The following pieces of information were extracted in an Excel table from the full text of each eligible study (primarily by VV, consulting with PB in case of doubt): health outcome(s), determinants, statistical methodology, level of analysis, results, type of data, data sources, time period, countries. The evidence is synthesized according to these extracted data (often directly reflected in the section headings), using a narrative form accompanied by a “summary-of-findings” table and a graph.
Search and selection
The initial yield contained 4583 records, reduced to 3686 after removal of duplicates ( Fig 1 ). Based on title and abstract screening, 3271 records were excluded because they focused on specific medical condition(s) or specific populations (based on morbidity or some other factor), dealt with intervention effectiveness, with theoretical or non-health related issues, or with animals or plants. Of the remaining 415 papers, roughly half were disqualified upon full-text consideration, mostly due to using an outcome not of interest to us (e.g., health inequality), measuring and analyzing determinants and outcomes exclusively at the individual level, performing analyses one country at a time, employing indices that are a mixture of both health indicators and health determinants, or not utilizing potential health determinants at all. After this second stage of the screening process, 202 papers were deemed eligible for inclusion. This group was further dichotomized according to level of economic development of the countries or regions under study, using membership of the OECD or Europe as a reference “cut-off” point. Sixty papers were judged to include high-income countries, and the remaining 142 included either low- or middle-income countries or a mix of both these levels of development. The rest of this report outlines findings in relation to high-income countries only, reflecting our own primary research interests. Nonetheless, we chose to report our search yield for the other income groups for two reasons. First, to gauge the relative interest in applied published research for these different income levels; and second, to enable other researchers with a focus on determinants of health in other countries to use the extraction we made here.
- PPT PowerPoint slide
- PNG larger image
- TIFF original image
https://doi.org/10.1371/journal.pone.0239031.g001
Health outcomes
The most frequent population health indicator, life expectancy (LE), was present in 24 of the 60 studies. Apart from “life expectancy at birth” (representing the average life-span a newborn is expected to have if current mortality rates remain constant), also called “period LE” by some [ 19 , 20 ], we encountered as well LE at 40 years of age [ 21 ], at 60 [ 22 ], and at 65 [ 21 , 23 , 24 ]. In two papers, the age-specificity of life expectancy (be it at birth or another age) was not stated [ 25 , 26 ].
Some studies considered male and female LE separately [ 21 , 24 , 25 , 27 – 33 ]. This consideration was also often observed with the second most commonly used health index [ 28 – 30 , 34 – 38 ]–termed “total”, or “overall”, or “all-cause”, mortality rate (MR)–included in 22 of the 60 studies. In addition to gender, this index was also sometimes broken down according to age group [ 30 , 39 , 40 ], as well as gender-age group [ 38 ].
While the majority of studies under review here focused on a single health indicator, 23 out of the 60 studies made use of multiple outcomes, although these outcomes were always considered one at a time, and sometimes not all of them fell within the scope of our review. An easily discernable group of indices that typically went together [ 25 , 37 , 41 ] was that of neonatal (deaths occurring within 28 days postpartum), perinatal (fetal or early neonatal / first-7-days deaths), and post-neonatal (deaths between the 29 th day and completion of one year of life) mortality. More often than not, these indices were also accompanied by “stand-alone” indicators, such as infant mortality (deaths within the first year of life; our third most common index found in 16 of the 60 studies), maternal mortality (deaths during pregnancy or within 42 days of termination of pregnancy), and child mortality rates. Child mortality has conventionally been defined as mortality within the first 5 years of life, thus often also called “under-5 mortality”. Nonetheless, Pritchard & Wallace used the term “child mortality” to denote deaths of children younger than 14 years [ 42 ].
As previously stated, inclusion criteria did allow for self-reported health status to be used as a general measure of population health. Within our final selection of studies, seven utilized some form of subjective health as an outcome variable [ 25 , 43 – 48 ]. Additionally, the Health Human Development Index [ 49 ], healthy life expectancy [ 50 ], old-age survival [ 51 ], potential years of life lost [ 52 ], and disability-adjusted life expectancy [ 25 ] were also used.
We note that while in most cases the indicators mentioned above (and/or the covariates considered, see below) were taken in their absolute or logarithmic form, as a—typically annual—number, sometimes they were used in the form of differences, change rates, averages over a given time period, or even z-scores of rankings [ 19 , 22 , 40 , 42 , 44 , 53 – 57 ].
Regions, countries, and populations
Despite our decision to confine this review to high-income countries, some variation in the countries and regions studied was still present. Selection seemed to be most often conditioned on the European Union, or the European continent more generally, and the Organisation of Economic Co-operation and Development (OECD), though, typically, not all member nations–based on the instances where these were also explicitly listed—were included in a given study. Some of the stated reasons for omitting certain nations included data unavailability [ 30 , 45 , 54 ] or inconsistency [ 20 , 58 ], Gross Domestic Product (GDP) too low [ 40 ], differences in economic development and political stability with the rest of the sampled countries [ 59 ], and national population too small [ 24 , 40 ]. On the other hand, the rationales for selecting a group of countries included having similar above-average infant mortality [ 60 ], similar healthcare systems [ 23 ], and being randomly drawn from a social spending category [ 61 ]. Some researchers were interested explicitly in a specific geographical region, such as Eastern Europe [ 50 ], Central and Eastern Europe [ 48 , 60 ], the Visegrad (V4) group [ 62 ], or the Asia/Pacific area [ 32 ]. In certain instances, national regions or cities, rather than countries, constituted the units of investigation instead [ 31 , 51 , 56 , 62 – 66 ]. In two particular cases, a mix of countries and cities was used [ 35 , 57 ]. In another two [ 28 , 29 ], due to the long time periods under study, some of the included countries no longer exist. Finally, besides “European” and “OECD”, the terms “developed”, “Western”, and “industrialized” were also used to describe the group of selected nations [ 30 , 42 , 52 , 53 , 67 ].
As stated above, it was the health status of the general population that we were interested in, and during screening we made a concerted effort to exclude research using data based on a more narrowly defined group of individuals. All studies included in this review adhere to this general rule, albeit with two caveats. First, as cities (even neighborhoods) were the unit of analysis in three of the studies that made the selection [ 56 , 64 , 65 ], the populations under investigation there can be more accurately described as general urban , instead of just general. Second, oftentimes health indicators were stratified based on gender and/or age, therefore we also admitted one study that, due to its specific research question, focused on men and women of early retirement age [ 35 ] and another that considered adult males only [ 68 ].
Data types and sources
A great diversity of sources was utilized for data collection purposes. The accessible reference databases of the OECD ( https://www.oecd.org/ ), WHO ( https://www.who.int/ ), World Bank ( https://www.worldbank.org/ ), United Nations ( https://www.un.org/en/ ), and Eurostat ( https://ec.europa.eu/eurostat ) were among the top choices. The other international databases included Human Mortality [ 30 , 39 , 50 ], Transparency International [ 40 , 48 , 50 ], Quality of Government [ 28 , 69 ], World Income Inequality [ 30 ], International Labor Organization [ 41 ], International Monetary Fund [ 70 ]. A number of national databases were referred to as well, for example the US Bureau of Statistics [ 42 , 53 ], Korean Statistical Information Services [ 67 ], Statistics Canada [ 67 ], Australian Bureau of Statistics [ 67 ], and Health New Zealand Tobacco control and Health New Zealand Food and Nutrition [ 19 ]. Well-known surveys, such as the World Values Survey [ 25 , 55 ], the European Social Survey [ 25 , 39 , 44 ], the Eurobarometer [ 46 , 56 ], the European Value Survey [ 25 ], and the European Statistics of Income and Living Condition Survey [ 43 , 47 , 70 ] were used as data sources, too. Finally, in some cases [ 25 , 28 , 29 , 35 , 36 , 41 , 69 ], built-for-purpose datasets from previous studies were re-used.
In most of the studies, the level of the data (and analysis) was national. The exceptions were six papers that dealt with Nomenclature of Territorial Units of Statistics (NUTS2) regions [ 31 , 62 , 63 , 66 ], otherwise defined areas [ 51 ] or cities [ 56 ], and seven others that were multilevel designs and utilized both country- and region-level data [ 57 ], individual- and city- or country-level [ 35 ], individual- and country-level [ 44 , 45 , 48 ], individual- and neighborhood-level [ 64 ], and city-region- (NUTS3) and country-level data [ 65 ]. Parallel to that, the data type was predominantly longitudinal, with only a few studies using purely cross-sectional data [ 25 , 33 , 43 , 45 – 48 , 50 , 62 , 67 , 68 , 71 , 72 ], albeit in four of those [ 43 , 48 , 68 , 72 ] two separate points in time were taken (thus resulting in a kind of “double cross-section”), while in another the averages across survey waves were used [ 56 ].
In studies using longitudinal data, the length of the covered time periods varied greatly. Although this was almost always less than 40 years, in one study it covered the entire 20 th century [ 29 ]. Longitudinal data, typically in the form of annual records, was sometimes transformed before usage. For example, some researchers considered data points at 5- [ 34 , 36 , 49 ] or 10-year [ 27 , 29 , 35 ] intervals instead of the traditional 1, or took averages over 3-year periods [ 42 , 53 , 73 ]. In one study concerned with the effect of the Great Recession all data were in a “recession minus expansion change in trends”-form [ 57 ]. Furthermore, there were a few instances where two different time periods were compared to each other [ 42 , 53 ] or when data was divided into 2 to 4 (possibly overlapping) periods which were then analyzed separately [ 24 , 26 , 28 , 29 , 31 , 65 ]. Lastly, owing to data availability issues, discrepancies between the time points or periods of data on the different variables were occasionally observed [ 22 , 35 , 42 , 53 – 55 , 63 ].
Health determinants
Together with other essential details, Table 1 lists the health correlates considered in the selected studies. Several general categories for these correlates can be discerned, including health care, political stability, socio-economics, demographics, psychology, environment, fertility, life-style, culture, labor. All of these, directly or implicitly, have been recognized as holding importance for population health by existing theoretical models of (social) determinants of health [ 74 – 77 ].
https://doi.org/10.1371/journal.pone.0239031.t001
It is worth noting that in a few studies there was just a single aggregate-level covariate investigated in relation to a health outcome of interest to us. In one instance, this was life satisfaction [ 44 ], in another–welfare system typology [ 45 ], but also gender inequality [ 33 ], austerity level [ 70 , 78 ], and deprivation [ 51 ]. Most often though, attention went exclusively to GDP [ 27 , 29 , 46 , 57 , 65 , 71 ]. It was often the case that research had a more particular focus. Among others, minimum wages [ 79 ], hospital payment schemes [ 23 ], cigarette prices [ 63 ], social expenditure [ 20 ], residents’ dissatisfaction [ 56 ], income inequality [ 30 , 69 ], and work leave [ 41 , 58 ] took center stage. Whenever variables outside of these specific areas were also included, they were usually identified as confounders or controls, moderators or mediators.
We visualized the combinations in which the different determinants have been studied in Fig 2 , which was obtained via multidimensional scaling and a subsequent cluster analysis (details outlined in S2 Appendix ). It depicts the spatial positioning of each determinant relative to all others, based on the number of times the effects of each pair of determinants have been studied simultaneously. When interpreting Fig 2 , one should keep in mind that determinants marked with an asterisk represent, in fact, collectives of variables.
Groups of determinants are marked by asterisks (see S1 Table in S1 Appendix ). Diminishing color intensity reflects a decrease in the total number of “connections” for a given determinant. Noteworthy pairwise “connections” are emphasized via lines (solid-dashed-dotted indicates decreasing frequency). Grey contour lines encircle groups of variables that were identified via cluster analysis. Abbreviations: age = population age distribution, associations = membership in associations, AT-index = atherogenic-thrombogenic index, BR = birth rate, CAPB = Cyclically Adjusted Primary Balance, civilian-labor = civilian labor force, C-section = Cesarean delivery rate, credit-info = depth of credit information, dissatisf = residents’ dissatisfaction, distrib.orient = distributional orientation, EDU = education, eHealth = eHealth index at GP-level, exch.rate = exchange rate, fat = fat consumption, GDP = gross domestic product, GFCF = Gross Fixed Capital Formation/Creation, GH-gas = greenhouse gas, GII = gender inequality index, gov = governance index, gov.revenue = government revenues, HC-coverage = healthcare coverage, HE = health(care) expenditure, HHconsump = household consumption, hosp.beds = hospital beds, hosp.payment = hospital payment scheme, hosp.stay = length of hospital stay, IDI = ICT development index, inc.ineq = income inequality, industry-labor = industrial labor force, infant-sex = infant sex ratio, labor-product = labor production, LBW = low birth weight, leave = work leave, life-satisf = life satisfaction, M-age = maternal age, marginal-tax = marginal tax rate, MDs = physicians, mult.preg = multiple pregnancy, NHS = Nation Health System, NO = nitrous oxide emissions, PM10 = particulate matter (PM10) emissions, pop = population size, pop.density = population density, pre-term = pre-term birth rate, prison = prison population, researchE = research&development expenditure, school.ref = compulsory schooling reform, smoke-free = smoke-free places, SO = sulfur oxide emissions, soc.E = social expenditure, soc.workers = social workers, sugar = sugar consumption, terror = terrorism, union = union density, UR = unemployment rate, urban = urbanization, veg-fr = vegetable-and-fruit consumption, welfare = welfare regime, Wwater = wastewater treatment.
https://doi.org/10.1371/journal.pone.0239031.g002
Distances between determinants in Fig 2 are indicative of determinants’ “connectedness” with each other. While the statistical procedure called for higher dimensionality of the model, for demonstration purposes we show here a two-dimensional solution. This simplification unfortunately comes with a caveat. To use the factor smoking as an example, it would appear it stands at a much greater distance from GDP than it does from alcohol. In reality however, smoking was considered together with alcohol consumption [ 21 , 25 , 26 , 52 , 68 ] in just as many studies as it was with GDP [ 21 , 25 , 26 , 52 , 59 ], five. To aid with respect to this apparent shortcoming, we have emphasized the strongest pairwise links. Solid lines connect GDP with health expenditure (HE), unemployment rate (UR), and education (EDU), indicating that the effect of GDP on health, taking into account the effects of the other three determinants as well, was evaluated in between 12 to 16 studies of the 60 included in this review. Tracing the dashed lines, we can also tell that GDP appeared jointly with income inequality, and HE together with either EDU or UR, in anywhere between 8 to 10 of our selected studies. Finally, some weaker but still worth-mentioning “connections” between variables are displayed as well via the dotted lines.
The fact that all notable pairwise “connections” are concentrated within a relatively small region of the plot may be interpreted as low overall “connectedness” among the health indicators studied. GDP is the most widely investigated determinant in relation to general population health. Its total number of “connections” is disproportionately high (159) compared to its runner-up–HE (with 113 “connections”), and then subsequently EDU (with 90) and UR (with 86). In fact, all of these determinants could be thought of as outliers, given that none of the remaining factors have a total count of pairings above 52. This decrease in individual determinants’ overall “connectedness” can be tracked on the graph via the change of color intensity as we move outwards from the symbolic center of GDP and its closest “co-determinants”, to finally reach the other extreme of the ten indicators (welfare regime, household consumption, compulsory school reform, life satisfaction, government revenues, literacy, research expenditure, multiple pregnancy, Cyclically Adjusted Primary Balance, and residents’ dissatisfaction; in white) the effects on health of which were only studied in isolation.
Lastly, we point to the few small but stable clusters of covariates encircled by the grey bubbles on Fig 2 . These groups of determinants were identified as “close” by both statistical procedures used for the production of the graph (see details in S2 Appendix ).
Statistical methodology
There was great variation in the level of statistical detail reported. Some authors provided too vague a description of their analytical approach, necessitating some inference in this section.
The issue of missing data is a challenging reality in this field of research, but few of the studies under review (12/60) explain how they dealt with it. Among the ones that do, three general approaches to handling missingness can be identified, listed in increasing level of sophistication: case-wise deletion, i.e., removal of countries from the sample [ 20 , 45 , 48 , 58 , 59 ], (linear) interpolation [ 28 , 30 , 34 , 58 , 59 , 63 ], and multiple imputation [ 26 , 41 , 52 ].
Correlations, Pearson, Spearman, or unspecified, were the only technique applied with respect to the health outcomes of interest in eight analyses [ 33 , 42 – 44 , 46 , 53 , 57 , 61 ]. Among the more advanced statistical methods, the family of regression models proved to be, by and large, predominant. Before examining this closer, we note the techniques that were, in a way, “unique” within this selection of studies: meta-analyses were performed (random and fixed effects, respectively) on the reduced form and 2-sample two stage least squares (2SLS) estimations done within countries [ 39 ]; difference-in-difference (DiD) analysis was applied in one case [ 23 ]; dynamic time-series methods, among which co-integration, impulse-response function (IRF), and panel vector autoregressive (VAR) modeling, were utilized in one study [ 80 ]; longitudinal generalized estimating equation (GEE) models were developed on two occasions [ 70 , 78 ]; hierarchical Bayesian spatial models [ 51 ] and special autoregressive regression [ 62 ] were also implemented.
Purely cross-sectional data analyses were performed in eight studies [ 25 , 45 , 47 , 50 , 55 , 56 , 67 , 71 ]. These consisted of linear regression (assumed ordinary least squares (OLS)), generalized least squares (GLS) regression, and multilevel analyses. However, six other studies that used longitudinal data in fact had a cross-sectional design, through which they applied regression at multiple time-points separately [ 27 , 29 , 36 , 48 , 68 , 72 ].
Apart from these “multi-point cross-sectional studies”, some other simplistic approaches to longitudinal data analysis were found, involving calculating and regressing 3-year averages of both the response and the predictor variables [ 54 ], taking the average of a few data-points (i.e., survey waves) [ 56 ] or using difference scores over 10-year [ 19 , 29 ] or unspecified time intervals [ 40 , 55 ].
Moving further in the direction of more sensible longitudinal data usage, we turn to the methods widely known among (health) economists as “panel data analysis” or “panel regression”. Most often seen were models with fixed effects for country/region and sometimes also time-point (occasionally including a country-specific trend as well), with robust standard errors for the parameter estimates to take into account correlations among clustered observations [ 20 , 21 , 24 , 28 , 30 , 32 , 34 , 37 , 38 , 41 , 52 , 59 , 60 , 63 , 66 , 69 , 73 , 79 , 81 , 82 ]. The Hausman test [ 83 ] was sometimes mentioned as the tool used to decide between fixed and random effects [ 26 , 49 , 63 , 66 , 73 , 82 ]. A few studies considered the latter more appropriate for their particular analyses, with some further specifying that (feasible) GLS estimation was employed [ 26 , 34 , 49 , 58 , 60 , 73 ]. Apart from these two types of models, the first differences method was encountered once as well [ 31 ]. Across all, the error terms were sometimes assumed to come from a first-order autoregressive process (AR(1)), i.e., they were allowed to be serially correlated [ 20 , 30 , 38 , 58 – 60 , 73 ], and lags of (typically) predictor variables were included in the model specification, too [ 20 , 21 , 37 , 38 , 48 , 69 , 81 ]. Lastly, a somewhat different approach to longitudinal data analysis was undertaken in four studies [ 22 , 35 , 48 , 65 ] in which multilevel–linear or Poisson–models were developed.
Regardless of the exact techniques used, most studies included in this review presented multiple model applications within their main analysis. None attempted to formally compare models in order to identify the “best”, even if goodness-of-fit statistics were occasionally reported. As indicated above, many studies investigated women’s and men’s health separately [ 19 , 21 , 22 , 27 – 29 , 31 , 33 , 35 , 36 , 38 , 39 , 45 , 50 , 51 , 64 , 65 , 69 , 82 ], and covariates were often tested one at a time, including other covariates only incrementally [ 20 , 25 , 28 , 36 , 40 , 50 , 55 , 67 , 73 ]. Furthermore, there were a few instances where analyses within countries were performed as well [ 32 , 39 , 51 ] or where the full time period of interest was divided into a few sub-periods [ 24 , 26 , 28 , 31 ]. There were also cases where different statistical techniques were applied in parallel [ 29 , 55 , 60 , 66 , 69 , 73 , 82 ], sometimes as a form of sensitivity analysis [ 24 , 26 , 30 , 58 , 73 ]. However, the most common approach to sensitivity analysis was to re-run models with somewhat different samples [ 39 , 50 , 59 , 67 , 69 , 80 , 82 ]. Other strategies included different categorization of variables or adding (more/other) controls [ 21 , 23 , 25 , 28 , 37 , 50 , 63 , 69 ], using an alternative main covariate measure [ 59 , 82 ], including lags for predictors or outcomes [ 28 , 30 , 58 , 63 , 65 , 79 ], using weights [ 24 , 67 ] or alternative data sources [ 37 , 69 ], or using non-imputed data [ 41 ].
As the methods and not the findings are the main focus of the current review, and because generic checklists cannot discern the underlying quality in this application field (see also below), we opted to pool all reported findings together, regardless of individual study characteristics or particular outcome(s) used, and speak generally of positive and negative effects on health. For this summary we have adopted the 0.05-significance level and only considered results from multivariate analyses. Strictly birth-related factors are omitted since these potentially only relate to the group of infant mortality indicators and not to any of the other general population health measures.
Starting with the determinants most often studied, higher GDP levels [ 21 , 26 , 27 , 29 , 30 , 32 , 43 , 48 , 52 , 58 , 60 , 66 , 67 , 73 , 79 , 81 , 82 ], higher health [ 21 , 37 , 47 , 49 , 52 , 58 , 59 , 68 , 72 , 82 ] and social [ 20 , 21 , 26 , 38 , 79 ] expenditures, higher education [ 26 , 39 , 52 , 62 , 72 , 73 ], lower unemployment [ 60 , 61 , 66 ], and lower income inequality [ 30 , 42 , 53 , 55 , 73 ] were found to be significantly associated with better population health on a number of occasions. In addition to that, there was also some evidence that democracy [ 36 ] and freedom [ 50 ], higher work compensation [ 43 , 79 ], distributional orientation [ 54 ], cigarette prices [ 63 ], gross national income [ 22 , 72 ], labor productivity [ 26 ], exchange rates [ 32 ], marginal tax rates [ 79 ], vaccination rates [ 52 ], total fertility [ 59 , 66 ], fruit and vegetable [ 68 ], fat [ 52 ] and sugar consumption [ 52 ], as well as bigger depth of credit information [ 22 ] and percentage of civilian labor force [ 79 ], longer work leaves [ 41 , 58 ], more physicians [ 37 , 52 , 72 ], nurses [ 72 ], and hospital beds [ 79 , 82 ], and also membership in associations, perceived corruption and societal trust [ 48 ] were beneficial to health. Higher nitrous oxide (NO) levels [ 52 ], longer average hospital stay [ 48 ], deprivation [ 51 ], dissatisfaction with healthcare and the social environment [ 56 ], corruption [ 40 , 50 ], smoking [ 19 , 26 , 52 , 68 ], alcohol consumption [ 26 , 52 , 68 ] and illegal drug use [ 68 ], poverty [ 64 ], higher percentage of industrial workers [ 26 ], Gross Fixed Capital creation [ 66 ] and older population [ 38 , 66 , 79 ], gender inequality [ 22 ], and fertility [ 26 , 66 ] were detrimental.
It is important to point out that the above-mentioned effects could not be considered stable either across or within studies. Very often, statistical significance of a given covariate fluctuated between the different model specifications tried out within the same study [ 20 , 49 , 59 , 66 , 68 , 69 , 73 , 80 , 82 ], testifying to the importance of control variables and multivariate research (i.e., analyzing multiple independent variables simultaneously) in general. Furthermore, conflicting results were observed even with regards to the “core” determinants given special attention, so to speak, throughout this text. Thus, some studies reported negative effects of health expenditure [ 32 , 82 ], social expenditure [ 58 ], GDP [ 49 , 66 ], and education [ 82 ], and positive effects of income inequality [ 82 ] and unemployment [ 24 , 31 , 32 , 52 , 66 , 68 ]. Interestingly, one study [ 34 ] differentiated between temporary and long-term effects of GDP and unemployment, alluding to possibly much greater complexity of the association with health. It is also worth noting that some gender differences were found, with determinants being more influential for males than for females, or only having statistically significant effects for male health [ 19 , 21 , 28 , 34 , 36 , 37 , 39 , 64 , 65 , 69 ].
The purpose of this scoping review was to examine recent quantitative work on the topic of multi-country analyses of determinants of population health in high-income countries.
Measuring population health via relatively simple mortality-based indicators still seems to be the state of the art. What is more, these indicators are routinely considered one at a time, instead of, for example, employing existing statistical procedures to devise a more general, composite, index of population health, or using some of the established indices, such as disability-adjusted life expectancy (DALE) or quality-adjusted life expectancy (QALE). Although strong arguments for their wider use were already voiced decades ago [ 84 ], such summary measures surface only rarely in this research field.
On a related note, the greater data availability and accessibility that we enjoy today does not automatically equate to data quality. Nonetheless, this is routinely assumed in aggregate level studies. We almost never encountered a discussion on the topic. The non-mundane issue of data missingness, too, goes largely underappreciated. With all recent methodological advancements in this area [ 85 – 88 ], there is no excuse for ignorance; and still, too few of the reviewed studies tackled the matter in any adequate fashion.
Much optimism can be gained considering the abundance of different determinants that have attracted researchers’ attention in relation to population health. We took on a visual approach with regards to these determinants and presented a graph that links spatial distances between determinants with frequencies of being studies together. To facilitate interpretation, we grouped some variables, which resulted in some loss of finer detail. Nevertheless, the graph is helpful in exemplifying how many effects continue to be studied in a very limited context, if any. Since in reality no factor acts in isolation, this oversimplification practice threatens to render the whole exercise meaningless from the outset. The importance of multivariate analysis cannot be stressed enough. While there is no “best method” to be recommended and appropriate techniques vary according to the specifics of the research question and the characteristics of the data at hand [ 89 – 93 ], in the future, in addition to abandoning simplistic univariate approaches, we hope to see a shift from the currently dominating fixed effects to the more flexible random/mixed effects models [ 94 ], as well as wider application of more sophisticated methods, such as principle component regression, partial least squares, covariance structure models (e.g., structural equations), canonical correlations, time-series, and generalized estimating equations.
Finally, there are some limitations of the current scoping review. We searched the two main databases for published research in medical and non-medical sciences (PubMed and Web of Science) since 2013, thus potentially excluding publications and reports that are not indexed in these databases, as well as older indexed publications. These choices were guided by our interest in the most recent (i.e., the current state-of-the-art) and arguably the highest-quality research (i.e., peer-reviewed articles, primarily in indexed non-predatory journals). Furthermore, despite holding a critical stance with regards to some aspects of how determinants-of-health research is currently conducted, we opted out of formally assessing the quality of the individual studies included. The reason for that is two-fold. On the one hand, we are unaware of the existence of a formal and standard tool for quality assessment of ecological designs. And on the other, we consider trying to score the quality of these diverse studies (in terms of regional setting, specific topic, outcome indices, and methodology) undesirable and misleading, particularly since we would sometimes have been rating the quality of only a (small) part of the original studies—the part that was relevant to our review’s goal.
Our aim was to investigate the current state of research on the very broad and general topic of population health, specifically, the way it has been examined in a multi-country context. We learned that data treatment and analytical approach were, in the majority of these recent studies, ill-equipped or insufficiently transparent to provide clarity regarding the underlying mechanisms of population health in high-income countries. Whether due to methodological shortcomings or the inherent complexity of the topic, research so far fails to provide any definitive answers. It is our sincere belief that with the application of more advanced analytical techniques this continuous quest could come to fruition sooner.
Supporting information
S1 checklist. preferred reporting items for systematic reviews and meta-analyses extension for scoping reviews (prisma-scr) checklist..
https://doi.org/10.1371/journal.pone.0239031.s001
S1 Appendix.
https://doi.org/10.1371/journal.pone.0239031.s002
S2 Appendix.
https://doi.org/10.1371/journal.pone.0239031.s003
- View Article
- Google Scholar
- PubMed/NCBI
- 75. Dahlgren G, Whitehead M. Policies and Strategies to Promote Equity in Health. Stockholm, Sweden: Institute for Future Studies; 1991.
- 76. Brunner E, Marmot M. Social Organization, Stress, and Health. In: Marmot M, Wilkinson RG, editors. Social Determinants of Health. Oxford, England: Oxford University Press; 1999.
- 77. Najman JM. A General Model of the Social Origins of Health and Well-being. In: Eckersley R, Dixon J, Douglas B, editors. The Social Origins of Health and Well-being. Cambridge, England: Cambridge University Press; 2001.
- 85. Carpenter JR, Kenward MG. Multiple Imputation and its Application. New York: John Wiley & Sons; 2013.
- 86. Molenberghs G, Fitzmaurice G, Kenward MG, Verbeke G, Tsiatis AA. Handbook of Missing Data Methodology. Boca Raton: Chapman & Hall/CRC; 2014.
- 87. van Buuren S. Flexible Imputation of Missing Data. 2nd ed. Boca Raton: Chapman & Hall/CRC; 2018.
- 88. Enders CK. Applied Missing Data Analysis. New York: Guilford; 2010.
- 89. Shayle R. Searle GC, Charles E. McCulloch. Variance Components: John Wiley & Sons, Inc.; 1992.
- 90. Agresti A. Foundations of Linear and Generalized Linear Models. Hoboken, New Jersey: John Wiley & Sons Inc.; 2015.
- 91. Leyland A. H. (Editor) HGE. Multilevel Modelling of Health Statistics: John Wiley & Sons Inc; 2001.
- 92. Garrett Fitzmaurice MD, Geert Verbeke, Geert Molenberghs. Longitudinal Data Analysis. New York: Chapman and Hall/CRC; 2008.
- 93. Wolfgang Karl Härdle LS. Applied Multivariate Statistical Analysis. Berlin, Heidelberg: Springer; 2015.
Quantitative and Qualitative Research: An Overview of Approaches
- First Online: 03 January 2022
Cite this chapter
- Euclid Seeram 5 , 6 , 7
551 Accesses
In Chap. 1 , the nature and scope of research were outlined and included an overview of quantitative and qualitative research and a brief description of research designs. In this chapter, both quantitative and qualitative research will be described in a little more detail with respect to essential features and characteristics. Furthermore, the research designs used in each of these approaches will be reviewed. Finally, this chapter will conclude with examples of published quantitative and qualitative research in medical imaging and radiation therapy.
This is a preview of subscription content, log in via an institution to check access.
Access this chapter
- Available as PDF
- Read on any device
- Instant download
- Own it forever
- Available as EPUB and PDF
- Compact, lightweight edition
- Dispatched in 3 to 5 business days
- Free shipping worldwide - see info
- Durable hardcover edition
Tax calculation will be finalised at checkout
Purchases are for personal use only
Institutional subscriptions
Anvari, A., Halpern, E. F., & Samir, A. E. (2015). Statistics 101 for radiologists. Radiographics, 35 , 1789–1801.
Article Google Scholar
Battistelli, A., Portoghese, I., Galletta, M., & Pohl, S. (2013). Beyond the tradition: Test of an integrative conceptual model on nurse turnover. International Nursing Review, 60 (1), 103–111. https://doi.org/10.1111/j.1466-7657.2012.01024.x
Article CAS PubMed Google Scholar
Bhattacherjee, A. (2012). Social science research: Principles, methods, and practices . In Textbooks Collection , 3. http://scholarcommons.usf.edu/oa_textbooks/3 . University of South Florida.
Chenail, R. (2011). Ten steps for conceptualizing and conducting qualitative research studies in a pragmatically curious manner. The Qualitative Report, 16 (6), 1713–1730. http://www.nova.edu/ssss/QR/QR16-6/chenail.pdf
Google Scholar
Coyle, M. K. (2012). Depressive symptoms after a myocardial infarction and self-care. Archives of Psychiatric Nursing, 26 (2), 127–134. https://doi.org/10.1016/j.apnu.2011.06.004
Article PubMed Google Scholar
Creswell, J. W., & Guetterman, T. C. (2019). Educational research: Planning, conducting, and evaluating quantitative and qualitative research (6th ed.). Pearson Education.
Curtis, E. A., Comiskey, C., & Dempsey, O. (2016). Importance and use of correlational research. Nurse Researcher, 23 (6), 20–25. https://doi.org/10.7748/nr.2016.e1382
Gibson, D. J., & Davidson, R. A. (2012). Exposure creep in computed radiography: A longitudinal study. Academic Radiology, 19 (4), 458–462. https://doi.org/10.1016/j.acra.2011.12.003 . Epub 2012 Jan 5.
Gray, J. R., Grove, S. K., & Sutherland, S. (2017). The practice of nursing research: Appraisal, synthesis, and generation of evidence . Elsevier.
Miles, M., Hubermann, A., & Saldana, J. (2014). Qualitative data analysis: a methods sourcebook (3rd ed.). Sage.
Munhall, P. L. (2012). Nursing research: A qualitative perspective (5th ed.). Jones and Bartlett.
Munn, Z., & Jordan, Z. (2011). The patient experience of high technology medical imaging: A systematic review of the qualitative evidence. JBI Library of Systematic Reviews, 9 (19), 631–678. https://doi.org/10.11124/01938924-201109190-00001
Munn, Z., Pearson, A., Jordan, Z., Murphy, F., & Pilkington, D. (2013). Action research in radiography: What it is and how it can be conducted. Journal of Medical Radiation Sciences, 60 (2), 47–52. https://doi.org/10.1002/jmrs.8
Article PubMed PubMed Central Google Scholar
O’Regan, T., Robinson, L., Newton-Hughes, A., & Strudwick, R. (2019). A review of visual ethnography: Radiography viewed through a different lens. Radiography, 25 (Supplement 1), S9–S13.
Price, P., Jhangiani, R., & Chiang, I. (2015). Research methods of psychology (2nd Canadian ed.). BC Campus. Retrieved from https://opentextbc.ca/researchmethods/
Seeram, E., Davidson, R., Bushong, S., & Swan, H. (2015). Education and training required for the digital radiography environment: A non-interventional quantitative survey study of radiologic technologists. International Journal of Radiology & Medical Imaging, 2 , 103. https://doi.org/10.15344/ijrmi/2015/103
Seeram, E., Davidson, R., Bushong, S., & Swan, H. (2016). Optimizing the exposure indicator as a dose management strategy in computed radiography. Radiologic Technology, 87 (4), 380–391.
PubMed Google Scholar
Solomon, P., & Draine, J. (2010). An overview of quantitative methods. In B. Thyer (Ed.), The handbook of social work research methods (2nd ed., pp. 26–36). Sage.
Chapter Google Scholar
Suchsland, M. Z., Cruz, M. J., Hardy, V., Jarvik, J., McMillan, G., Brittain, A., & Thompson, M. (2020). Qualitative study to explore radiologist and radiologic technologist perceptions of outcomes patients experience during imaging in the USA. BMJ Open, 10 , e033961. https://doi.org/10.1136/bmjopen-2019-033961
Thomas, L. (2020). An introduction to quasi-experimental designs. Retrieved from Scribbr.com https://www.scribbr.com/methodology/quasi-experimental-design/ . Accessed 8 Jan 2021.
University of Lethbridge (Alberta, Canada). (2020). An introduction to action research. https://www.uleth.ca/education/research/research-centers/action-research/introduction . Accessed 12 Jan 2020.
Download references
Author information
Authors and affiliations.
Medical Imaging and Radiation Sciences, Monash University, Melbourne, VIC, Australia
Euclid Seeram
Faculty of Science, Charles Sturt University, Bathurst, NSW, Australia
Medical Radiation Sciences, Faculty of Health, University of Canberra, Canberra, ACT, Australia
You can also search for this author in PubMed Google Scholar
Editor information
Editors and affiliations.
Medical Imaging, Faculty of Health, University of Canberra, Burnaby, BC, Canada
Faculty of Health, University of Canberra, Canberra, ACT, Australia
Robert Davidson
Brookfield Health Sciences, University College Cork, Cork, Ireland
Andrew England
Mark F. McEntee
Rights and permissions
Reprints and permissions
Copyright information
© 2021 Springer Nature Switzerland AG
About this chapter
Seeram, E. (2021). Quantitative and Qualitative Research: An Overview of Approaches. In: Seeram, E., Davidson, R., England, A., McEntee, M.F. (eds) Research for Medical Imaging and Radiation Sciences. Springer, Cham. https://doi.org/10.1007/978-3-030-79956-4_2
Download citation
DOI : https://doi.org/10.1007/978-3-030-79956-4_2
Published : 03 January 2022
Publisher Name : Springer, Cham
Print ISBN : 978-3-030-79955-7
Online ISBN : 978-3-030-79956-4
eBook Packages : Biomedical and Life Sciences Biomedical and Life Sciences (R0)
Share this chapter
Anyone you share the following link with will be able to read this content:
Sorry, a shareable link is not currently available for this article.
Provided by the Springer Nature SharedIt content-sharing initiative
- Publish with us
Policies and ethics
- Find a journal
- Track your research
Advertisement

- Previous Issue
- Previous Article
- Next Article
Clarifying the Research Purpose
Methodology, measurement, data analysis and interpretation, tools for evaluating the quality of medical education research, research support, competing interests, quantitative research methods in medical education.
Submitted for publication January 8, 2018. Accepted for publication November 29, 2018.
- Split-Screen
- Article contents
- Figures & tables
- Supplementary Data
- Peer Review
- Open the PDF for in another window
- Cite Icon Cite
- Get Permissions
- Search Site
John T. Ratelle , Adam P. Sawatsky , Thomas J. Beckman; Quantitative Research Methods in Medical Education. Anesthesiology 2019; 131:23–35 doi: https://doi.org/10.1097/ALN.0000000000002727
Download citation file:
- Ris (Zotero)
- Reference Manager
There has been a dramatic growth of scholarly articles in medical education in recent years. Evaluating medical education research requires specific orientation to issues related to format and content. Our goal is to review the quantitative aspects of research in medical education so that clinicians may understand these articles with respect to framing the study, recognizing methodologic issues, and utilizing instruments for evaluating the quality of medical education research. This review can be used both as a tool when appraising medical education research articles and as a primer for clinicians interested in pursuing scholarship in medical education.

Image: J. P. Rathmell and Terri Navarette.
There has been an explosion of research in the field of medical education. A search of PubMed demonstrates that more than 40,000 articles have been indexed under the medical subject heading “Medical Education” since 2010, which is more than the total number of articles indexed under this heading in the 1980s and 1990s combined. Keeping up to date requires that practicing clinicians have the skills to interpret and appraise the quality of research articles, especially when serving as editors, reviewers, and consumers of the literature.
While medical education shares many characteristics with other biomedical fields, substantial particularities exist. We recognize that practicing clinicians may not be familiar with the nuances of education research and how to assess its quality. Therefore, our purpose is to provide a review of quantitative research methodologies in medical education. Specifically, we describe a structure that can be used when conducting or evaluating medical education research articles.
Clarifying the research purpose is an essential first step when reading or conducting scholarship in medical education. 1 Medical education research can serve a variety of purposes, from advancing the science of learning to improving the outcomes of medical trainees and the patients they care for. However, a well-designed study has limited value if it addresses vague, redundant, or unimportant medical education research questions.
What is the research topic and why is it important? What is unknown about the research topic? Why is further research necessary?
What is the conceptual framework being used to approach the study?
What is the statement of study intent?
What are the research methodology and study design? Are they appropriate for the study objective(s)?
Which threats to internal validity are most relevant for the study?
What is the outcome and how was it measured?
Can the results be trusted? What is the validity and reliability of the measurements?
How were research subjects selected? Is the research sample representative of the target population?
Was the data analysis appropriate for the study design and type of data?
What is the effect size? Do the results have educational significance?
Fortunately, there are steps to ensure that the purpose of a research study is clear and logical. Table 1 2–5 outlines these steps, which will be described in detail in the following sections. We describe these elements not as a simple “checklist,” but as an advanced organizer that can be used to understand a medical education research study. These steps can also be used by clinician educators who are new to the field of education research and who wish to conduct scholarship in medical education.
Steps in Clarifying the Purpose of a Research Study in Medical Education

Literature Review and Problem Statement
A literature review is the first step in clarifying the purpose of a medical education research article. 2 , 5 , 6 When conducting scholarship in medical education, a literature review helps researchers develop an understanding of their topic of interest. This understanding includes both existing knowledge about the topic as well as key gaps in the literature, which aids the researcher in refining their study question. Additionally, a literature review helps researchers identify conceptual frameworks that have been used to approach the research topic. 2
When reading scholarship in medical education, a successful literature review provides background information so that even someone unfamiliar with the research topic can understand the rationale for the study. Located in the introduction of the manuscript, the literature review guides the reader through what is already known in a manner that highlights the importance of the research topic. The literature review should also identify key gaps in the literature so the reader can understand the need for further research. This gap description includes an explicit problem statement that summarizes the important issues and provides a reason for the study. 2 , 4 The following is one example of a problem statement:
“Identifying gaps in the competency of anesthesia residents in time for intervention is critical to patient safety and an effective learning system… [However], few available instruments relate to complex behavioral performance or provide descriptors…that could inform subsequent feedback, individualized teaching, remediation, and curriculum revision.” 7
This problem statement articulates the research topic (identifying resident performance gaps), why it is important (to intervene for the sake of learning and patient safety), and current gaps in the literature (few tools are available to assess resident performance). The researchers have now underscored why further research is needed and have helped readers anticipate the overarching goals of their study (to develop an instrument to measure anesthesiology resident performance). 4
The Conceptual Framework
Following the literature review and articulation of the problem statement, the next step in clarifying the research purpose is to select a conceptual framework that can be applied to the research topic. Conceptual frameworks are “ways of thinking about a problem or a study, or ways of representing how complex things work.” 3 Just as clinical trials are informed by basic science research in the laboratory, conceptual frameworks often serve as the “basic science” that informs scholarship in medical education. At a fundamental level, conceptual frameworks provide a structured approach to solving the problem identified in the problem statement.
Conceptual frameworks may take the form of theories, principles, or models that help to explain the research problem by identifying its essential variables or elements. Alternatively, conceptual frameworks may represent evidence-based best practices that researchers can apply to an issue identified in the problem statement. 3 Importantly, there is no single best conceptual framework for a particular research topic, although the choice of a conceptual framework is often informed by the literature review and knowing which conceptual frameworks have been used in similar research. 8 For further information on selecting a conceptual framework for research in medical education, we direct readers to the work of Bordage 3 and Irby et al. 9
To illustrate how different conceptual frameworks can be applied to a research problem, suppose you encounter a study to reduce the frequency of communication errors among anesthesiology residents during day-to-night handoff. Table 2 10 , 11 identifies two different conceptual frameworks researchers might use to approach the task. The first framework, cognitive load theory, has been proposed as a conceptual framework to identify potential variables that may lead to handoff errors. 12 Specifically, cognitive load theory identifies the three factors that affect short-term memory and thus may lead to communication errors:
Conceptual Frameworks to Address the Issue of Handoff Errors in the Intensive Care Unit

Intrinsic load: Inherent complexity or difficulty of the information the resident is trying to learn ( e.g. , complex patients).
Extraneous load: Distractions or demands on short-term memory that are not related to the information the resident is trying to learn ( e.g. , background noise, interruptions).
Germane load: Effort or mental strategies used by the resident to organize and understand the information he/she is trying to learn ( e.g. , teach back, note taking).
Using cognitive load theory as a conceptual framework, researchers may design an intervention to reduce extraneous load and help the resident remember the overnight to-do’s. An example might be dedicated, pager-free handoff times where distractions are minimized.
The second framework identified in table 2 , the I-PASS (Illness severity, Patient summary, Action list, Situational awareness and contingency planning, and Synthesis by receiver) handoff mnemonic, 11 is an evidence-based best practice that, when incorporated as part of a handoff bundle, has been shown to reduce handoff errors on pediatric wards. 13 Researchers choosing this conceptual framework may adapt some or all of the I-PASS elements for resident handoffs in the intensive care unit.
Note that both of the conceptual frameworks outlined above provide researchers with a structured approach to addressing the issue of handoff errors; one is not necessarily better than the other. Indeed, it is possible for researchers to use both frameworks when designing their study. Ultimately, we provide this example to demonstrate the necessity of selecting conceptual frameworks to clarify the research purpose. 3 , 8 Readers should look for conceptual frameworks in the introduction section and should be wary of their omission, as commonly seen in less well-developed medical education research articles. 14
Statement of Study Intent
After reviewing the literature, articulating the problem statement, and selecting a conceptual framework to address the research topic, the final step in clarifying the research purpose is the statement of study intent. The statement of study intent is arguably the most important element of framing the study because it makes the research purpose explicit. 2 Consider the following example:
This study aimed to test the hypothesis that the introduction of the BASIC Examination was associated with an accelerated knowledge acquisition during residency training, as measured by increments in annual ITE scores. 15
This statement of study intent succinctly identifies several key study elements including the population (anesthesiology residents), the intervention/independent variable (introduction of the BASIC Examination), the outcome/dependent variable (knowledge acquisition, as measure by in In-training Examination [ITE] scores), and the hypothesized relationship between the independent and dependent variable (the authors hypothesize a positive correlation between the BASIC examination and the speed of knowledge acquisition). 6 , 14
The statement of study intent will sometimes manifest as a research objective, rather than hypothesis or question. In such instances there may not be explicit independent and dependent variables, but the study population and research aim should be clearly identified. The following is an example:
“In this report, we present the results of 3 [years] of course data with respect to the practice improvements proposed by participating anesthesiologists and their success in implementing those plans. Specifically, our primary aim is to assess the frequency and type of improvements that were completed and any factors that influence completion.” 16
The statement of study intent is the logical culmination of the literature review, problem statement, and conceptual framework, and is a transition point between the Introduction and Methods sections of a medical education research report. Nonetheless, a systematic review of experimental research in medical education demonstrated that statements of study intent are absent in the majority of articles. 14 When reading a medical education research article where the statement of study intent is absent, it may be necessary to infer the research aim by gathering information from the Introduction and Methods sections. In these cases, it can be useful to identify the following key elements 6 , 14 , 17 :
Population of interest/type of learner ( e.g. , pain medicine fellow or anesthesiology residents)
Independent/predictor variable ( e.g. , educational intervention or characteristic of the learners)
Dependent/outcome variable ( e.g. , intubation skills or knowledge of anesthetic agents)
Relationship between the variables ( e.g. , “improve” or “mitigate”)
Occasionally, it may be difficult to differentiate the independent study variable from the dependent study variable. 17 For example, consider a study aiming to measure the relationship between burnout and personal debt among anesthesiology residents. Do the researchers believe burnout might lead to high personal debt, or that high personal debt may lead to burnout? This “chicken or egg” conundrum reinforces the importance of the conceptual framework which, if present, should serve as an explanation or rationale for the predicted relationship between study variables.
Research methodology is the “…design or plan that shapes the methods to be used in a study.” 1 Essentially, methodology is the general strategy for answering a research question, whereas methods are the specific steps and techniques that are used to collect data and implement the strategy. Our objective here is to provide an overview of quantitative methodologies ( i.e. , approaches) in medical education research.
The choice of research methodology is made by balancing the approach that best answers the research question against the feasibility of completing the study. There is no perfect methodology because each has its own potential caveats, flaws and/or sources of bias. Before delving into an overview of the methodologies, it is important to highlight common sources of bias in education research. We use the term internal validity to describe the degree to which the findings of a research study represent “the truth,” as opposed to some alternative hypothesis or variables. 18 Table 3 18–20 provides a list of common threats to internal validity in medical education research, along with tactics to mitigate these threats.
Threats to Internal Validity and Strategies to Mitigate Their Effects

Experimental Research
The fundamental tenet of experimental research is the manipulation of an independent or experimental variable to measure its effect on a dependent or outcome variable.
True Experiment
True experimental study designs minimize threats to internal validity by randomizing study subjects to experimental and control groups. Through ensuring that differences between groups are—beyond the intervention/variable of interest—purely due to chance, researchers reduce the internal validity threats related to subject characteristics, time-related maturation, and regression to the mean. 18 , 19
Quasi-experiment
There are many instances in medical education where randomization may not be feasible or ethical. For instance, researchers wanting to test the effect of a new curriculum among medical students may not be able to randomize learners due to competing curricular obligations and schedules. In these cases, researchers may be forced to assign subjects to experimental and control groups based upon some other criterion beyond randomization, such as different classrooms or different sections of the same course. This process, called quasi-randomization, does not inherently lead to internal validity threats, as long as research investigators are mindful of measuring and controlling for extraneous variables between study groups. 19
Single-group Methodologies
All experimental study designs compare two or more groups: experimental and control. A common experimental study design in medical education research is the single-group pretest–posttest design, which compares a group of learners before and after the implementation of an intervention. 21 In essence, a single-group pre–post design compares an experimental group ( i.e. , postintervention) to a “no-intervention” control group ( i.e. , preintervention). 19 This study design is problematic for several reasons. Consider the following hypothetical example: A research article reports the effects of a year-long intubation curriculum for first-year anesthesiology residents. All residents participate in monthly, half-day workshops over the course of an academic year. The article reports a positive effect on residents’ skills as demonstrated by a significant improvement in intubation success rates at the end of the year when compared to the beginning.
This study does little to advance the science of learning among anesthesiology residents. While this hypothetical report demonstrates an improvement in residents’ intubation success before versus after the intervention, it does not tell why the workshop worked, how it compares to other educational interventions, or how it fits in to the broader picture of anesthesia training.
Single-group pre–post study designs open themselves to a myriad of threats to internal validity. 20 In our hypothetical example, the improvement in residents’ intubation skills may have been due to other educational experience(s) ( i.e. , implementation threat) and/or improvement in manual dexterity that occurred naturally with time ( i.e. , maturation threat), rather than the airway curriculum. Consequently, single-group pre–post studies should be interpreted with caution. 18
Repeated testing, before and after the intervention, is one strategy that can be used to reduce the some of the inherent limitations of the single-group study design. Repeated pretesting can mitigate the effect of regression toward the mean, a statistical phenomenon whereby low pretest scores tend to move closer to the mean on subsequent testing (regardless of intervention). 20 Likewise, repeated posttesting at multiple time intervals can provide potentially useful information about the short- and long-term effects of an intervention ( e.g. , the “durability” of the gain in knowledge, skill, or attitude).
Observational Research
Unlike experimental studies, observational research does not involve manipulation of any variables. These studies often involve measuring associations, developing psychometric instruments, or conducting surveys.
Association Research
Association research seeks to identify relationships between two or more variables within a group or groups (correlational research), or similarities/differences between two or more existing groups (causal–comparative research). For example, correlational research might seek to measure the relationship between burnout and educational debt among anesthesiology residents, while causal–comparative research may seek to measure differences in educational debt and/or burnout between anesthesiology and surgery residents. Notably, association research may identify relationships between variables, but does not necessarily support a causal relationship between them.
Psychometric and Survey Research
Psychometric instruments measure a psychologic or cognitive construct such as knowledge, satisfaction, beliefs, and symptoms. Surveys are one type of psychometric instrument, but many other types exist, such as evaluations of direct observation, written examinations, or screening tools. 22 Psychometric instruments are ubiquitous in medical education research and can be used to describe a trait within a study population ( e.g. , rates of depression among medical students) or to measure associations between study variables ( e.g. , association between depression and board scores among medical students).
Psychometric and survey research studies are prone to the internal validity threats listed in table 3 , particularly those relating to mortality, location, and instrumentation. 18 Additionally, readers must ensure that the instrument scores can be trusted to truly represent the construct being measured. For example, suppose you encounter a research article demonstrating a positive association between attending physician teaching effectiveness as measured by a survey of medical students, and the frequency with which the attending physician provides coffee and doughnuts on rounds. Can we be confident that this survey administered to medical students is truly measuring teaching effectiveness? Or is it simply measuring the attending physician’s “likability”? Issues related to measurement and the trustworthiness of data are described in detail in the following section on measurement and the related issues of validity and reliability.
Measurement refers to “the assigning of numbers to individuals in a systematic way as a means of representing properties of the individuals.” 23 Research data can only be trusted insofar as we trust the measurement used to obtain the data. Measurement is of particular importance in medical education research because many of the constructs being measured ( e.g. , knowledge, skill, attitudes) are abstract and subject to measurement error. 24 This section highlights two specific issues related to the trustworthiness of data: the validity and reliability of measurements.
Validity regarding the scores of a measurement instrument “refers to the degree to which evidence and theory support the interpretations of the [instrument’s results] for the proposed use of the [instrument].” 25 In essence, do we believe the results obtained from a measurement really represent what we were trying to measure? Note that validity evidence for the scores of a measurement instrument is separate from the internal validity of a research study. Several frameworks for validity evidence exist. Table 4 2 , 22 , 26 represents the most commonly used framework, developed by Messick, 27 which identifies sources of validity evidence—to support the target construct—from five main categories: content, response process, internal structure, relations to other variables, and consequences.
Sources of Validity Evidence for Measurement Instruments

Reliability
Reliability refers to the consistency of scores for a measurement instrument. 22 , 25 , 28 For an instrument to be reliable, we would anticipate that two individuals rating the same object of measurement in a specific context would provide the same scores. 25 Further, if the scores for an instrument are reliable between raters of the same object of measurement, then we can extrapolate that any difference in scores between two objects represents a true difference across the sample, and is not due to random variation in measurement. 29 Reliability can be demonstrated through a variety of methods such as internal consistency ( e.g. , Cronbach’s alpha), temporal stability ( e.g. , test–retest reliability), interrater agreement ( e.g. , intraclass correlation coefficient), and generalizability theory (generalizability coefficient). 22 , 29
Example of a Validity and Reliability Argument
This section provides an illustration of validity and reliability in medical education. We use the signaling questions outlined in table 4 to make a validity and reliability argument for the Harvard Assessment of Anesthesia Resident Performance (HARP) instrument. 7 The HARP was developed by Blum et al. to measure the performance of anesthesia trainees that is required to provide safe anesthetic care to patients. According to the authors, the HARP is designed to be used “…as part of a multiscenario, simulation-based assessment” of resident performance. 7
Content Validity: Does the Instrument’s Content Represent the Construct Being Measured?
To demonstrate content validity, instrument developers should describe the construct being measured and how the instrument was developed, and justify their approach. 25 The HARP is intended to measure resident performance in the critical domains required to provide safe anesthetic care. As such, investigators note that the HARP items were created through a two-step process. First, the instrument’s developers interviewed anesthesiologists with experience in resident education to identify the key traits needed for successful completion of anesthesia residency training. Second, the authors used a modified Delphi process to synthesize the responses into five key behaviors: (1) formulate a clear anesthetic plan, (2) modify the plan under changing conditions, (3) communicate effectively, (4) identify performance improvement opportunities, and (5) recognize one’s limits. 7 , 30
Response Process Validity: Are Raters Interpreting the Instrument Items as Intended?
In the case of the HARP, the developers included a scoring rubric with behavioral anchors to ensure that faculty raters could clearly identify how resident performance in each domain should be scored. 7
Internal Structure Validity: Do Instrument Items Measuring Similar Constructs Yield Homogenous Results? Do Instrument Items Measuring Different Constructs Yield Heterogeneous Results?
Item-correlation for the HARP demonstrated a high degree of correlation between some items ( e.g. , formulating a plan and modifying the plan under changing conditions) and a lower degree of correlation between other items ( e.g. , formulating a plan and identifying performance improvement opportunities). 30 This finding is expected since the items within the HARP are designed to assess separate performance domains, and we would expect residents’ functioning to vary across domains.
Relationship to Other Variables’ Validity: Do Instrument Scores Correlate with Other Measures of Similar or Different Constructs as Expected?
As it applies to the HARP, one would expect that the performance of anesthesia residents will improve over the course of training. Indeed, HARP scores were found to be generally higher among third-year residents compared to first-year residents. 30
Consequence Validity: Are Instrument Results Being Used as Intended? Are There Unintended or Negative Uses of the Instrument Results?
While investigators did not intentionally seek out consequence validity evidence for the HARP, unanticipated consequences of HARP scores were identified by the authors as follows:
“Data indicated that CA-3s had a lower percentage of worrisome scores (rating 2 or lower) than CA-1s… However, it is concerning that any CA-3s had any worrisome scores…low performance of some CA-3 residents, albeit in the simulated environment, suggests opportunities for training improvement.” 30
That is, using the HARP to measure the performance of CA-3 anesthesia residents had the unintended consequence of identifying the need for improvement in resident training.
Reliability: Are the Instrument’s Scores Reproducible and Consistent between Raters?
The HARP was applied by two raters for every resident in the study across seven different simulation scenarios. The investigators conducted a generalizability study of HARP scores to estimate the variance in assessment scores that was due to the resident, the rater, and the scenario. They found little variance was due to the rater ( i.e. , scores were consistent between raters), indicating a high level of reliability. 7
Sampling refers to the selection of research subjects ( i.e. , the sample) from a larger group of eligible individuals ( i.e. , the population). 31 Effective sampling leads to the inclusion of research subjects who represent the larger population of interest. Alternatively, ineffective sampling may lead to the selection of research subjects who are significantly different from the target population. Imagine that researchers want to explore the relationship between burnout and educational debt among pain medicine specialists. The researchers distribute a survey to 1,000 pain medicine specialists (the population), but only 300 individuals complete the survey (the sample). This result is problematic because the characteristics of those individuals who completed the survey and the entire population of pain medicine specialists may be fundamentally different. It is possible that the 300 study subjects may be experiencing more burnout and/or debt, and thus, were more motivated to complete the survey. Alternatively, the 700 nonresponders might have been too busy to respond and even more burned out than the 300 responders, which would suggest that the study findings were even more amplified than actually observed.
When evaluating a medical education research article, it is important to identify the sampling technique the researchers employed, how it might have influenced the results, and whether the results apply to the target population. 24

Sampling Techniques
Sampling techniques generally fall into two categories: probability- or nonprobability-based. Probability-based sampling ensures that each individual within the target population has an equal opportunity of being selected as a research subject. Most commonly, this is done through random sampling, which should lead to a sample of research subjects that is similar to the target population. If significant differences between sample and population exist, those differences should be due to random chance, rather than systematic bias. The difference between data from a random sample and that from the population is referred to as sampling error. 24
Nonprobability-based sampling involves selecting research participants such that inclusion of some individuals may be more likely than the inclusion of others. 31 Convenience sampling is one such example and involves selection of research subjects based upon ease or opportuneness. Convenience sampling is common in medical education research, but, as outlined in the example at the beginning of this section, it can lead to sampling bias. 24 When evaluating an article that uses nonprobability-based sampling, it is important to look for participation/response rate. In general, a participation rate of less than 75% should be viewed with skepticism. 21 Additionally, it is important to determine whether characteristics of participants and nonparticipants were reported and if significant differences between the two groups exist.
Interpreting medical education research requires a basic understanding of common ways in which quantitative data are analyzed and displayed. In this section, we highlight two broad topics that are of particular importance when evaluating research articles.
The Nature of the Measurement Variable
Measurement variables in quantitative research generally fall into three categories: nominal, ordinal, or interval. 24 Nominal variables (sometimes called categorical variables) involve data that can be placed into discrete categories without a specific order or structure. Examples include sex (male or female) and professional degree (M.D., D.O., M.B.B.S., etc .) where there is no clear hierarchical order to the categories. Ordinal variables can be ranked according to some criterion, but the spacing between categories may not be equal. Examples of ordinal variables may include measurements of satisfaction (satisfied vs . unsatisfied), agreement (disagree vs . agree), and educational experience (medical student, resident, fellow). As it applies to educational experience, it is noteworthy that even though education can be quantified in years, the spacing between years ( i.e. , educational “growth”) remains unequal. For instance, the difference in performance between second- and third-year medical students is dramatically different than third- and fourth-year medical students. Interval variables can also be ranked according to some criteria, but, unlike ordinal variables, the spacing between variable categories is equal. Examples of interval variables include test scores and salary. However, the conceptual boundaries between these measurement variables are not always clear, as in the case where ordinal scales can be assumed to have the properties of an interval scale, so long as the data’s distribution is not substantially skewed. 32
Understanding the nature of the measurement variable is important when evaluating how the data are analyzed and reported. Medical education research commonly uses measurement instruments with items that are rated on Likert-type scales, whereby the respondent is asked to assess their level of agreement with a given statement. The response is often translated into a corresponding number ( e.g. , 1 = strongly disagree, 3 = neutral, 5 = strongly agree). It is remarkable that scores from Likert-type scales are sometimes not normally distributed ( i.e. , are skewed toward one end of the scale), indicating that the spacing between scores is unequal and the variable is ordinal in nature. In these cases, it is recommended to report results as frequencies or medians, rather than means and SDs. 33
Consider an article evaluating medical students’ satisfaction with a new curriculum. Researchers measure satisfaction using a Likert-type scale (1 = very unsatisfied, 2 = unsatisfied, 3 = neutral, 4 = satisfied, 5 = very satisfied). A total of 20 medical students evaluate the curriculum, 10 of whom rate their satisfaction as “satisfied,” and 10 of whom rate it as “very satisfied.” In this case, it does not make much sense to report an average score of 4.5; it makes more sense to report results in terms of frequency ( e.g. , half of the students were “very satisfied” with the curriculum, and half were not).
Effect Size and CIs
In medical education, as in other research disciplines, it is common to report statistically significant results ( i.e. , small P values) in order to increase the likelihood of publication. 34 , 35 However, a significant P value in itself does necessarily represent the educational impact of the study results. A statement like “Intervention x was associated with a significant improvement in learners’ intubation skill compared to education intervention y ( P < 0.05)” tells us that there was a less than 5% chance that the difference in improvement between interventions x and y was due to chance. Yet that does not mean that the study intervention necessarily caused the nonchance results, or indicate whether the between-group difference is educationally significant. Therefore, readers should consider looking beyond the P value to effect size and/or CI when interpreting the study results. 36 , 37
Effect size is “the magnitude of the difference between two groups,” which helps to quantify the educational significance of the research results. 37 Common measures of effect size include Cohen’s d (standardized difference between two means), risk ratio (compares binary outcomes between two groups), and Pearson’s r correlation (linear relationship between two continuous variables). 37 CIs represent “a range of values around a sample mean or proportion” and are a measure of precision. 31 While effect size and CI give more useful information than simple statistical significance, they are commonly omitted from medical education research articles. 35 In such instances, readers should be wary of overinterpreting a P value in isolation. For further information effect size and CI, we direct readers the work of Sullivan and Feinn 37 and Hulley et al. 31
In this final section, we identify instruments that can be used to evaluate the quality of quantitative medical education research articles. To this point, we have focused on framing the study and research methodologies and identifying potential pitfalls to consider when appraising a specific article. This is important because how a study is framed and the choice of methodology require some subjective interpretation. Fortunately, there are several instruments available for evaluating medical education research methods and providing a structured approach to the evaluation process.
The Medical Education Research Study Quality Instrument (MERSQI) 21 and the Newcastle Ottawa Scale-Education (NOS-E) 38 are two commonly used instruments, both of which have an extensive body of validity evidence to support the interpretation of their scores. Table 5 21 , 39 provides more detail regarding the MERSQI, which includes evaluation of study design, sampling, data type, validity, data analysis, and outcomes. We have found that applying the MERSQI to manuscripts, articles, and protocols has intrinsic educational value, because this practice of application familiarizes MERSQI users with fundamental principles of medical education research. One aspect of the MERSQI that deserves special mention is the section on evaluating outcomes based on Kirkpatrick’s widely recognized hierarchy of reaction, learning, behavior, and results ( table 5 ; fig .). 40 Validity evidence for the scores of the MERSQI include its operational definitions to improve response process, excellent reliability, and internal consistency, as well as high correlation with other measures of study quality, likelihood of publication, citation rate, and an association between MERSQI score and the likelihood of study funding. 21 , 41 Additionally, consequence validity for the MERSQI scores has been demonstrated by its utility for identifying and disseminating high-quality research in medical education. 42

Kirkpatrick’s hierarchy of outcomes as applied to education research. Reaction = Level 1, Learning = Level 2, Behavior = Level 3, Results = Level 4. Outcomes become more meaningful, yet more difficult to achieve, when progressing from Level 1 through Level 4. Adapted with permission from Beckman and Cook, 2007. 2
The Medical Education Research Study Quality Instrument for Evaluating the Quality of Medical Education Research

The NOS-E is a newer tool to evaluate the quality of medication education research. It was developed as a modification of the Newcastle-Ottawa Scale 43 for appraising the quality of nonrandomized studies. The NOS-E includes items focusing on the representativeness of the experimental group, selection and compatibility of the control group, missing data/study retention, and blinding of outcome assessors. 38 , 39 Additional validity evidence for NOS-E scores includes operational definitions to improve response process, excellent reliability and internal consistency, and its correlation with other measures of study quality. 39 Notably, the complete NOS-E, along with its scoring rubric, can found in the article by Cook and Reed. 39
A recent comparison of the MERSQI and NOS-E found acceptable interrater reliability and good correlation between the two instruments 39 However, noted differences exist between the MERSQI and NOS-E. Specifically, the MERSQI may be applied to a broad range of study designs, including experimental and cross-sectional research. Additionally, the MERSQI addresses issues related to measurement validity and data analysis, and places emphasis on educational outcomes. On the other hand, the NOS-E focuses specifically on experimental study designs, and on issues related to sampling techniques and outcome assessment. 39 Ultimately, the MERSQI and NOS-E are complementary tools that may be used together when evaluating the quality of medical education research.
Conclusions
This article provides an overview of quantitative research in medical education, underscores the main components of education research, and provides a general framework for evaluating research quality. We highlighted the importance of framing a study with respect to purpose, conceptual framework, and statement of study intent. We reviewed the most common research methodologies, along with threats to the validity of a study and its measurement instruments. Finally, we identified two complementary instruments, the MERSQI and NOS-E, for evaluating the quality of a medical education research study.
Bordage G: Conceptual frameworks to illuminate and magnify. Medical education. 2009; 43(4):312–9.
Cook DA, Beckman TJ: Current concepts in validity and reliability for psychometric instruments: Theory and application. The American journal of medicine. 2006; 119(2):166. e7–166. e116.
Franenkel JR, Wallen NE, Hyun HH: How to Design and Evaluate Research in Education. 9th edition. New York, McGraw-Hill Education, 2015.
Hulley SB, Cummings SR, Browner WS, Grady DG, Newman TB: Designing clinical research. 4th edition. Philadelphia, Lippincott Williams & Wilkins, 2011.
Irby BJ, Brown G, Lara-Alecio R, Jackson S: The Handbook of Educational Theories. Charlotte, NC, Information Age Publishing, Inc., 2015
Standards for Educational and Psychological Testing (American Educational Research Association & American Psychological Association, 2014)
Swanwick T: Understanding medical education: Evidence, theory and practice, 2nd edition. Wiley-Blackwell, 2013.
Sullivan GM, Artino Jr AR: Analyzing and interpreting data from Likert-type scales. Journal of graduate medical education. 2013; 5(4):541–2.
Sullivan GM, Feinn R: Using effect size—or why the P value is not enough. Journal of graduate medical education. 2012; 4(3):279–82.
Tavakol M, Sandars J: Quantitative and qualitative methods in medical education research: AMEE Guide No 90: Part II. Medical teacher. 2014; 36(10):838–48.
Support was provided solely from institutional and/or departmental sources.
The authors declare no competing interests.
Citing articles via
Most viewed, email alerts, related articles, social media, affiliations.
- ASA Practice Parameters
- Online First
- Author Resource Center
- About the Journal
- Editorial Board
- Rights & Permissions
- Online ISSN 1528-1175
- Print ISSN 0003-3022
- Anesthesiology
- ASA Monitor

- Terms & Conditions Privacy Policy
- Manage Cookie Preferences
- © Copyright 2024 American Society of Anesthesiologists
This Feature Is Available To Subscribers Only
Sign In or Create an Account

An official website of the United States government
The .gov means it’s official. Federal government websites often end in .gov or .mil. Before sharing sensitive information, make sure you’re on a federal government site.
The site is secure. The https:// ensures that you are connecting to the official website and that any information you provide is encrypted and transmitted securely.
- Publications
- Account settings
Preview improvements coming to the PMC website in October 2024. Learn More or Try it out now .
- Advanced Search
- Journal List
- v.55; Jan-Dec 2018
A Quantitative Observational Study of Physician Influence on Hospital Costs
Herbert wong.
1 U.S. Department of Health and Human Services, Agency for Healthcare Research and Quality, Rockville, MD, USA
Zeynal Karaca
Teresa b. gibson.
2 IBM Watson Health, Ann Arbor, MI, USA
Physicians serve as the nexus of treatment decision-making in hospitalized patients; however, little empirical evidence describes the influence of individual physicians on hospital costs. In this study, we examine the extent to which hospital costs vary across physicians and physician characteristics. We used all-payer data from 2 states representing 15 237 physicians and 2.5 million hospital visits. Regression analysis and propensity score matching were used to understand the role of observable provider characteristics on hospital costs controlling for patient demographics, socioeconomic characteristics, clinical risk, and hospital characteristics. We used hierarchical models to estimate the amount of variation attributable to physicians. We found that the average cost of hospital inpatient stays registered to female physicians was consistently lower across all empirical specifications when compared with male physicians. We also found a negative association between physicians’ years of experience and the average costs. The average cost of hospital inpatient stays registered to foreign-trained physicians was lower than US-trained physicians. We observed sizable variation in average costs of hospital inpatient stays across medical specialties. In addition, we used hierarchical methods and estimated the amount of remaining variation attributable to physicians and found that it was nonnegligible (intraclass correlation coefficient [ICC]: 0.33 in the full sample). Historically, most physicians have been reimbursed separately from hospitals, and our study shows that physicians play a role in influencing hospital costs. Future policies and practices should acknowledge these important dependencies. This study lends further support for alignment of physician and hospital incentives to control costs and improve outcomes.
- What do we already know about this topic?
- Specific physician characteristics influence a physician’s practice style as well as health care cost, delivery of care and outcomes.
- How does your research contribute to the field?
- Our research expands the current literature by performing an all-payer (vs single payer) analysis, using hierarchical models to estimate the amount of variation attributable to individual physicians, and partitioning the variation in hospital costs to understand the extent of influence attributable to physicians.
- What are your research’s implications toward theory, practice, or policy?
- We found substantial variation in hospital costs with observable physician characteristics, lending further support for payment and organizational models that align physician and hospital incentives that seek to control costs and improve outcomes.
Introduction
It has been well established that health care spending varies with geography. 1 - 3 The source of this variation has been often questioned—whether it is arising from area practice patterns, patient health status, patient characteristics, price, and/or individual provider decision making. 3 , 4
An Institute of Medicine (IoM) Committee examining geographic variations in Medicare spending convened earlier this decade concluded that individual providers of care had a great deal of influence on spending. 5 The Committee found post-acute care and inpatient care had the largest amounts of variation in spending, and discovered large variations in provider behavior. 4 Recommendations from the IoM Committee stated that evidence pointed away from geographic or small area spending signatures and toward health care decision-makers. Similarly, Gottleib and colleagues 6 performed a study of spending variation controlling for patient demographic characteristics, health status, and prices between regions, and found that price contributed only a small fraction of variation in spending although patients with similar characteristics received different levels of care from providers.
Previous studies have long demonstrated that specific physician characteristics influence a physician’s practice style. 7 - 10 Several studies have assessed how well physician characteristics explain the variation in hospital resource use. 1 , 11 - 13 Other researchers profiled physicians by analyzing and comparing the effects of their characteristics on health care cost, delivery, and outcomes. 14 - 19 A recent study by Tsugawa and colleagues 20 demonstrated the existence of physician influence on Part B Medicare spending and the extent of spending attributable to physicians.
Other recent studies have examined the relationship between observable characteristics of physicians and health care spending and outcomes. For example, patients treated by graduates of foreign medical schools had lower mortality but higher Medicare Part B payments than those graduating from US medical schools. 21 Elderly patients with a female physician had lower mortality and readmission rates than male physicians. 22 In a separate study, no clear pattern was found between patient mortality and physician age for elderly patients, but patients with an older physician had higher Medicare Part B payments. 23 Also, Southern and colleagues 24 found that tenure in practice was positively associated with higher risk of mortality and longer lengths of stay in a local hospital system.
In this study, we use all-payer inpatient data from 2 states, Arizona and Florida, to analyze and quantify the extent of physician influence on inpatient hospital costs other than professional services. Hospital care accounts for 32% of national health care expenditures and is the largest expense category in 2015. 25 In addition, physicians are responsible for selecting the course of care provision and treatment, thereby influencing hospital costs of care.
Our research has 2 aims. First, we describe the relationship between hospital costs and observable characteristics of physicians including physician gender and foreign medical school graduation while controlling for patient demographics, socioeconomic characteristics, clinical risk, and hospital characteristics, although cost in this analysis cannot be distinguished between patients and payers. Second, we measure the fraction of variation in costs of hospital inpatient visits due to individual physicians, controlling for observable physician characteristics, patient demographics, socioeconomic characteristics, clinical risk, and hospital characteristics.
This article complements and expands upon the existing empirical literature in several important ways. First, our data are all-payer and do not limit the analysis to a specific payer group or patient group. This extends the previous literature as most recent studies have focused on the physician role in Part B spending variation in large Medicare samples 20 - 23 or within small, local samples. 24 Most physicians have a mix of patients covered by Medicare, Medicaid, private payers, and the uninsured, and we seek to understand their role in influencing hospital costs across all-payer groups. In addition, we use hierarchical models to estimate the amount of variation attributable to individual physicians, controlling for patient demographics, socioeconomic characteristics, clinical risk, and hospital characteristics, allowing us to partition the variation in hospital costs and to understand the extent of influence attributable to physicians. Finally, we use regression analysis and propensity score matching to further understand the role of providers on hospital costs.
We used the Healthcare Cost and Utilization Project (HCUP) 2008 State Inpatient Databases (SID) for Arizona and Florida. These HCUP SID files include all inpatient hospitalizations for nearly all acute care nonfederal hospitals in the subject states. The SID provide detailed diagnoses and procedures, total charges, and patient demographics including gender, age, race, and expected payment source (ie, Medicare, Medicaid, private insurance, other insurance, and self-pay). Physician characteristic information (eg, specialty, year of graduation from medical school, and the name of the medical school) was obtained from the Arizona Board of Medical Examiners and the Florida Department of Health. With permissions from all data partners, information was linked to the Arizona SID using both physician license number and physician name, and to the Florida SID using physician license numbers as the Florida SID do not provide physician name. i The physician represented the surgeon (operating physician), if a surgery was performed, otherwise, the attending physician who is responsible for overall care from admission to discharge. In addition, supplemental hospital characteristic and area characteristic information were obtained, respectively, from the American Hospital Association (AHA) and Area Resource Files. The total number of hospital inpatient visits during 2008 in Arizona and Florida was about 3.31 million, and about 5% were missing physician identifiers. We successfully linked 2.53 million of these visits to physician licensure databases and AHA hospital survey data. All investigators signed a Data Use Agreement. Because HCUP does not involve human subjects, institutional review board approval was not required for this study.
Our key covariates of interest were the physician’s gender, years of experience, board certified specialties, and whether they graduated from a medical school outside of the United States. We calculated years of experience as the difference between 2008 and the year the physician graduated from medical school. We created a series of dummy variables to represent the physician’s specialties of surgery, internal medicine, obstetrics and gynecology, neurology, psychiatry, pediatrics, cardiology, family medicine and general practitioners, and urology. The effect of being foreign-trained was examined by including a separate dummy variable for physicians if they graduated from a medical school outside of the United States. While physicians’ names and the name of their medical school are included in physicians’ licensure databases, physicians’ gender and the location of their medical schools are not readily available. We obtained these from various data sources and online search engines including http://doctor.webmd.com , http://www.aamc.org , http://www.babynames.com , and http://www.google.com . For physician gender, we followed a systematic assignment process requiring matching information from at least 2 independent data sources. Complete information regarding major physician characteristics was obtained for 2.53 million discharges studied in this study.
Hospital costs represent the underlying expenses to produce the hospital services. Since hospitals differ in their markup from costs to charges, we first reduced the charge for each case based on the hospital’s all-payer, inpatient cost-to-charge ratio. ii We applied hospital-specific all-payer cost-to-charge ratios, and replaced all-payer cost-to-charge ratios with group-average all-payer inpatient cost-to-charge ratios when hospital-specific all-payer inpatient cost-to-charge ratios were missing. Next, we adjusted these costs with the area wage index iii computed by the Centers for Medicare and Medicaid Services (CMS) to control for price factors beyond the hospital’s control. We also obtained information about hospital characteristics (eg, teaching status and bed size) using the AHA Annual Survey Database.
Empirical Models
Our study’s empirical models employ a hierarchical framework 26 to assess the effects of physician characteristics on the costs of hospital inpatient visits; we developed a model that controls for physician characteristics, patient demographics, socioeconomic characteristics, clinical risk, and hospital characteristics.
We reassessed the impacts of physician characteristics on costs of hospital inpatient visits using multilevel regression analysis where hospital inpatient visits were clustered by physician.
Our empirical model takes the following general form:
where variation in the intercept is predicted at level 2 by
Substituting (2) into Equation (1) yields the following single multilevel equation:
where i indexes the hospital inpatient visits and j indexes the physicians who treated the i th visit, and LogCost ij is the natural log value of the total hospital inpatient cost associated with the i th visit in the j th physician unit. Physician j is a vector of physicians’ characteristics that includes physicians’ years of experience measured as the difference between 2008 and their year of graduation from medical school, a set of dummy variables for physicians’ board certified specialties (surgery, internal medicine, obstetrics and gynecology, neurology, psychiatry, pediatrics, cardiology, family medicine and general practitioners, urology), for physicians’ gender, and for physicians who graduated from a medical school outside of the United States. Demographic i is a vector of observable patient demographic characteristics, which include age (in age/10 scale), and dummy variables for race and gender. Socioecon i includes a set of county-level dummy variables for income (low, low-medium, medium-high, and high) and for patients’ primary insurance providers (ie, Medicare, Medicaid, private, and other). Risk i includes dummy variables for the Elixhauser comorbidity index. 27 Severity i is the high-severity-measure dummy variable (with value 1 for the patient when All Patient Refined Diagnosis Related Groups (APR-DRG) severity index takes a value of 3 or 4). 28 Hospital i includes a set of dummy variables related to hospital characteristics—including teaching status, ownership type, bed size, and state (Arizona or Florida)—that may also represent unmeasured severity of illness for a patient referred to a highly capable institution. Finally, γ j represents departures of the j th physician from the overall mean that serves to shift the overall regression line representing the population average up or down according to each physician, and ξ ij is the level 1 random error. The random components of this model provide information about intraclass correlation coefficients (ICCs), which enables us to understand variation in costs of hospital inpatient visits associated with physicians’ characteristics. Our level 1 predictor variables are dummy variables except for age, which we standardized by dividing by 10. In our case, centering around the grand mean or using raw metric values did not change the direction of estimates. Therefore, we used raw metric values in our regression analysis. iv We present findings overall, and for teaching and nonteaching hospitals, as physician mix and patient complexity may vary between these types of facilities.
Sensitivity Analysis
We also developed various scenarios to test the robustness of our results. Specifically, we enhanced our model by incorporating level 2 variation not only in intercept but also in slope. Under this model, we assumed that patients had certain preferences in their choice of physician. We ran 3 models with level 2 variations within physicians by these patient characteristics: gender, severity of illness, and gender and severity of illness. Our empirical findings in these 3 models, where both intercepts and slopes varied in level 2, were parallel to our model with level 2 intercept-only variations. For the purpose of clarity, we provide the results for our base model where level 2 variations are only observed through intercepts that represent departures for each physician from the overall mean.
Some researchers claim that there is an implicit relationship between patient gender and physician gender 7 , 29 - 32 or physicians’ practice style and their graduating medical school, 34 which could introduce some degree of endogeneity into our empirical model as presented above. Although our multilevel model substantially reduces the unobservable endogeneity by clustering patients across physicians, we employed propensity score matching techniques 33 to address the potential endogeneity issues when estimating the impact of physicians’ practice style on hospital inpatient costs. We employed the propensity score nearest neighbor (NN) matching without replacement method to create subsamples of physicians based on their observable characteristics. We created our first subsample of physicians by matching female physicians with male physicians based on their observable characteristic of medical specialties, experience, foreign- versus US-trained, state (Arizona or Florida), and whether physicians practiced at both teaching and nonteaching hospitals. Then, we reestimated our multilevel model using hospital inpatient visits registered to these matched cohorts of physicians. The new estimates provide more robust findings regarding the impact of practice styles of female physicians on hospital inpatient costs when compared with their matching male cohorts. Next, we created our second subsample of physicians by matching foreign-trained physicians with US-trained physicians based on their observable characteristics of medical specialties, experience, gender, state, and whether physicians practiced at both teaching and nonteaching hospitals and reestimated our multilevel model.
The average cost of hospital inpatient visits was $9172 for all visits, $9492 for visits to teaching hospitals, and $8679 for visits to nonteaching hospitals (see Appendix Table A1 for visit characteristics). There were 7993 physicians who worked only at teaching hospitals, 4249 physicians who worked only at nonteaching hospitals, and 2995 physicians who worked in both settings for a total of 15 237 physicians ( Table 1 ). The physicians had an average of 24 years of experience. The proportion of female physicians was 26.5%, and the relative distribution working only at teaching hospitals or nonteaching hospitals, or at both, were comparable. About a third of the physicians graduated from medical schools outside of the United States, and we observed a higher prevalence at nonteaching hospitals when compared with teaching hospitals. We also observed that 16.4% of physicians in our sample were board certified surgeons and 31.7% of physicians had board certification in internal medicine. The percentage of physicians with other board certified specialties was lower: obstetrics and gynecology (8.0), neurology (2.3), psychiatry (1.2), pediatrics (12.7), cardiology (7.0), family medicine and general practitioners (7.3), urology (2.5).
Profile of Physicians at Hospital Inpatient Settings.
Note. Data include all hospital inpatient stays incurred during 2008 in Arizona and Florida. We excluded all records associated with physicians with 12 or fewer observations during 2008, which is about 1% of entire sample.
Table 1 also presents the average cost per hospital inpatient visit by physician characteristics. The average cost of hospital inpatient visits for patients visiting female physicians was $2264 lower when compared with costs for patients visiting male physicians. This difference was larger in teaching hospitals when compared with nonteaching hospitals. Similarly, we observed the average cost per hospital visit treated by foreign-trained physicians was $1191 less when compared with physicians who graduated from a medical college in the United States. Although we observed a larger difference in average hospital inpatient costs between foreign-trained and US-trained physicians who work only at teaching hospitals, there was only about $64 difference for physicians working only at nonteaching hospitals. We found sizable variation in the average cost of a hospital inpatient visit across physicians’ specialties. Patients treated by physicians with specialties in surgery, neurology, and cardiology had relatively higher average costs per hospital visit, which were $17 431, $16 496, and $14 714, respectively.
We also documented the distribution of patients’ severity of illness by physician characteristics. The results presented in Table 1 show that the percentage with high severity of illness was higher for male patients than for female patients regardless of the hospital setting. We also observed that foreign-trained physicians had a relatively higher share of high-severity patients at teaching hospitals when compared with nonteaching hospitals. Finally, we found that the relative share of high-severity patients was greater for physicians with specialties in internal medicine or family medicine and general practitioners working at nonteaching hospitals when compared with physicians with the same specialties working at teaching hospitals. However, for most of the remaining physicians working only at teaching hospitals, we observed a higher share of patients with high severity of illness when compared with physicians working only at nonteaching hospitals.
Regression Results
Linear regression results presented in column 1 of Table 2 show that the average cost of hospital inpatient visits for patients visiting female physicians was 0.1% lower than male physicians and was 0.5% lower for patients visiting foreign-trained physicians versus US-trained physicians. Each additional year of experience was associated with 4.3% lower costs. We also observed sizable variation in average costs of hospital inpatient visits across medical specialties where surgeons and cardiologists were associated with the highest average cost and pediatricians and psychiatrists were associated with the lowest average cost per hospital inpatient visit. The regression results based on hospital inpatient visits to teaching hospitals were parallel to our main results for all key covariates ( Table 2 , column 2). We also found similar results for nonteaching hospitals ( Table 2 , column 3).
Estimated Effects of Physician Characteristics on Log Inpatient Cost Per Visit.
Note. Data include all hospital inpatient stays incurred during 2008 in Arizona and Florida. We excluded all records associated with physicians with 12 or fewer observations during 2008, which is about 1% of the entire sample. All regression models include patient’s primary payers, median household income for residences in patient’s ZIP Code, and the Elixhauser comorbidity index. Level 1 is visit level and level 2 is physician level. Percent impact is calculated as (exp(coefficient) – 1) × 100. Standard errors are in parentheses.
Columns 4 to 6 of Table 2 present the results of multilevel regressions estimated separately for hospital inpatient visits to all hospitals, to teaching hospitals, and to nonteaching hospitals to assess the robustness of our earlier results derived from single-level linear regression. The average cost of hospital inpatient visits for patients visiting female physicians was 11% lower than male physicians and was 3.6% lower for patients visiting foreign-trained physicians versus US-trained physicians. Each additional year of experience was associated with 0.10% lower costs. Similar to our earlier results, we found substantial variation in costs of hospital inpatient visits across medical specialties. The multilevel regression results based on inpatient visits to teaching hospitals and nonteaching hospitals retained the same sign and statistical significance, which enhanced the validity and robustness of our results, specifically how physician characteristics impact the cost per hospital inpatient visits. v
Table 2 presents the estimates separately for 2 cohorts of physicians where the first cohort includes equal numbers of male and female physicians with a similar distribution of other characteristics, and the second cohort includes equal numbers of foreign-trained and US-trained physicians with a similar distribution of other characteristics (see matching results in Appendix Table A2 ). The estimated coefficients on key physician characteristics are highly statistically significant and have the same direction as our earlier results. In our female-male matched cohort, the regression results show that the hospital inpatient costs registered to female physicians are 10.8% lower when compared with hospital inpatient visits registered to male physicians. Similarly, the estimated effect of foreign-trained physicians on hospital inpatient costs is 3.8% lower in our second cohort where each foreign-trained physician is matched with a US-trained physician. The coefficients on physicians’ experience and medical specialties remain statistically significant and parallel to our earlier findings in the hierarchical models.
The multilevel regression results presented in Tables 2 and and3 3 also enable us to empirically measure the average correlation of patients registered to the same physicians. ICC, which is calculated by dividing the level 1 variance by the sum of the level 1 and level 2 variations, describes how strongly hospital inpatient visits registered to the same physicians are correlated with each other. In general, if ICC approaches to value zero, then one might chose to ignore multilevel estimation models and analyze the data in standard ways. On the contrary, if the ICC approaches the value one, there is no variation among patients registered to same physicians, so one might aggregate the data at the physician level and run a single-level linear regression model on aggregated data. For our case, the ICC values ranged from 0.329 (0.241 / [0.241 + 0.419]) (nonteaching hospitals) to 0.364 (teaching hospitals) ( Table 2 ) before matching and 0.605 (female models) to 0.643 (0.481 / [0.481 + 0.267]) (foreign-trained physician models) ( Table 3 ) after matching which indicates modest to sizable variation among visits registered to the same physician. The ICC range of our multilevel model also empirically validates our discussion around the necessity of using a multilevel model rather than single-level linear regression model.
Estimated Effects of Physician Characteristics on Log Inpatient Spending Per Visit.
Note. Level 1 is visit level and level 2 is physician level. Percent impact is calculated as (exp(coefficient) – 1) × 100. NN = nearest neighbor. Absolute values of t -ratios are in parentheses.
In this examination of all-payer data from two states, we found substantial variation in the costs of producing these hospital services with observable physician characteristics such as physician age, gender, foreign training, and physician specialty. We found that the average cost of hospital inpatient stays registered to female physicians was consistently lower across all empirical specifications when compared with the average cost of hospital inpatient stays registered to male physicians. We also found a negative association between physicians’ years of experience and the average costs of hospital inpatient stays. Similarly, the average cost of hospital inpatient stays registered to foreign-trained physicians was significantly lower when compared with the average cost of hospital inpatient stays registered to US-trained physicians. Finally, we observed sizable variation in average costs of hospital inpatient stays across medical specialties where surgeons and cardiologists were generally associated with higher average costs and pediatricians and psychiatrists were generally associated with lower average costs. Further research should investigate the sources of the differences associated with physician characteristics.
Using hierarchical methods and random effects, we estimated the percentage of remaining variation attributable to individual physicians. Using the entire sample, the ICC was approximately 0.35, or one third of the variation was attributable to physicians. Our approach partitions the variation in hospital costs and allows physicians to practice at multiple hospitals. Other studies have employed hospital fixed effects and partitioned the remaining variation in physician costs effectively comparing physicians within the same hospital. 21 This is an important distinction in approaches and could result in slightly different conclusions based on the variation that is being partitioned (total or net of hospital fixed effects).
Our data are all-payer and focus on the underlying costs of providing care. These differ from reimbursement amounts which may be relatively standardized across hospitals through Diagnosis Related Groups (DRG) payments within payers. Our results confirm that physician behavior is associated with variation in hospital costs other than professional services and this could occur through variations in physician practice styles and treatment decision-making.
Our study is limited to data from 2 large US states, and analysis of physician behavior in other states or countries may differ. This study relies on accurate attribution of individual physicians to hospital discharges. Our study is not experimental, and is observational, revealing a retrospective view of the association between physicians and hospital costs. Physicians were not randomized to patients, so potential endogeneity exists in patient selection of physicians. We attempted to minimize the impact of potential endogeneity in physician gender and foreign-trained physicians by creating matched samples of physicians and found that the ICC increased substantially, exceeding 0.60. This result is likely due to the retention of more similar samples of physicians, where residual variation is lower, and the percentage of variation attributable to physicians is higher.
When compared with recent studies, our findings are consistent with Tsugawa and colleagues 22 who found that female physicians treating Medicare patients had lower Part B payments. However, while we found that foreign medical graduates had slightly lower hospital costs, Tsugawa and colleagues 21 also found that foreign medical graduates had slightly higher Part B spending ($47 per discharge). The difference may be in the data used; ours is all-payer and focuses on hospital costs, and Tsugawa and colleagues 21 analyze Medicare enrollees and Medicare Part B payments as well as a methodological difference. Tsugawa and colleagues employ hospital fixed effects which compares physicians practicing at the same hospital.
Historically, physician and hospitals have been reimbursed via separate mechanisms, and our results quantify the physician role in the provision of care in hospital facilities. Our study lends support to the interconnected relationship between physicians and facilities in providing care to patients. Future policies, practices, and training processes for hospital administrators and physicians should acknowledge and address these important dependencies.
This study predates large systemic changes to align incentives of physicians and hospitals including some types of Alternative Payment Models and Accountable Care Organizations, and allows a window into physician influence on hospital costs prior to the expansion of these initiatives. At the time of this study, physicians generally had fewer incentives to control hospital costs. As aligned incentives expand, repeating this analysis will be important to understand trends in cost variation and physician influence. Our study also lends further support for payment and organizational models that align physician and hospital incentives that seek to control costs and improve outcomes.
Acknowledgments
Arizona Department of Health Services and Florida Department of Health granted special permission to access physician identifiers used by the research team. The authors would like to acknowledge the following Healthcare Cost and Utilization Project (HCUP) Partner organizations for contributing data to the HCUP State Inpatient Databases (SID) used in this study: Arizona Department of Health Services and Florida Agency for Health Care Administration. A full list of HCUP Data Partners can be found at www.hcup-us.ahrq.gov/db/hcupdatapartners.jsp .
Profile of Hospital Inpatient Visits.
Note. Data include all hospital inpatient stays incurred during 2008 in Arizona and Florida. We excluded all records associated with physicians with 12 or fewer observations during 2008, which was about 1% of the entire sample.
Analytic Framework for Hierarchical Models
Following existing studies on multilevel models ( Bryk and Raudenbush 1992 , Rice and Jones 1997 , Carey 2000 , Diez-Roux 2000 ), a basic formal 2-level model is presented, with a single level 1 predictor and a single level 2 predictor with the intercept modeled to vary randomly at level 2. The level 1 model takes the form
Response variable Y represents hospital inpatient cost per visit, X is a predictor that varies with hospital inpatient visits, and subscripts i and j reference hospital inpatient visits and physicians, respectively. Residual ξ i j is the random error for the i th hospital inpatient visit in the j th physician unit. At level 2, variation in the intercept is predicted by
The terms β 02 represent fixed elements and β 12 , the coefficients on P j , varies for each physician. The terms γ j are the random error components and along with ξ ij are assumed to be normally distributed with zero mean. Furthermore,
Substituting (2) into Equation (1) yields the single 2-level multilevel equation:
The first 3 terms on the right-hand side make up the deterministic part of the model. The 2 terms in parentheses comprise the stochastic or residual portion, which, in this example, contains 2 random variables. Components γ j represent departures of the j th physician from the overall mean; ξ i j is the hospital-inpatient-visit-level random error. Equation (3) requires the estimation of 3 fixed coefficients, 2 variances, and one covariance component. The presence of more than one residual term distinguishes this model from standard regression models. It is straightforward to enhance this model with more predictors and higher levels.
We present the descriptive characteristics for the 2 separate subsamples of physicians obtained from the propensity score nearest neighbor (NN) matching without replacement method as explained earlier. The descriptive results presented in Table A2 shows that the distribution of physician characteristics in the matching cohorts are very similar. For example, 3978 female physicians were individually matched with 3978 male physicians based on their observable characteristics. In this sample, the relative distribution of medical specialties and mean value for experience among female physicians were very similar to those of their male physician counterparts. Similarly, Table A2 shows that 5298 foreign-trained physicians were matched with 5298 US-trained physicians. The relative distributions of medical specialties, gender, and mean value for experience for foreign-trained physicians were very close to those statistics among the US-trained physicians.
Profile of Physicians Matched Through Propensity Score NN Matching Without Replacement Method.
Note. NN = nearest neighbor.
- Bryk A, Raudenbush S. Hierarchical Linear Models . Newbury Park, CA: Sage; 1992. [ Google Scholar ]
- Carey K. A multilevel modelling approach to analysis of patient costs under managed care . Health Econ . 2000; 9 :435-446. [ PubMed ] [ Google Scholar ]
- Diez-Roux A. Multilevel analysis in public health research . Annu Rev Public Health . 2000; 21 :171-192. [ PubMed ] [ Google Scholar ]
- Rice N, Jones A. Multilevel models and health economics . Health Econ . 1997; 6 :561-575. [ PubMed ] [ Google Scholar ]
i. Arizona Department of Health and Florida Agency for Health Care Administration granted special permission to access physician identifiers used by the research team
ii. The methodology uses the hospital’s accounting report covering all patients submitted to Centers for Medicare and Medicaid Services (CMS) and is described in user guides at http://www.hcup-us.ahrq.gov/db/state/costtocharge.jsp (accessed October 10, 2012).
iii. Costs throughout this article are inflation-adjusted. The methodology is described in user guides at http://www.hcup-us.ahrq.gov/db/state/costtocharge.jsp (accessed October 10, 2012).
iv. We also used grand mean centering for all level 1 variables (except age, which was scaled as age/10), and we found the direction and significance of results remained same.
v. Some researchers may suggest further clustering instead of estimating 2-level multilevel regression model separately using patients’ discharge data from teaching hospitals and nonteaching hospitals. We added further clustering by hospital’s teaching status and regression results for 3-level multilevel regression model were parallel to our earlier 2-level multilevel regression results.
Declaration of Conflicting Interests: The author(s) declared no potential conflicts of interest with respect to the research, authorship, and/or publication of this article.
Funding: The author(s) disclosed receipt of the following financial support for the research, authorship, and/or publication of this article: This study was funded by the Agency for Healthcare Research and Quality (AHRQ) under contract HHSA-290-2013-00002-C and through AHRQ intramural research funds. The views expressed herein are those of the authors and do not necessarily reflect those of the AHRQ Quality or the US Department of Department of Health and Human Services. No official endorsement by any agency of the federal or state governments or IBM Watson Health is intended or should be inferred.
IRB Statement: The Healthcare Cost and Utilization Project (HCUP) databases are consistent with the definition of limited data sets under the Health Insurance Portability and Accountability Act Privacy Rule. The Agency for Healthcare Research and Quality (AHRQ) Institutional Review Board considers research using HCUP data to have exempt status.

Log in using your username and password
- Search More Search for this keyword Advanced search
- Latest content
- Current issue
- For authors
- New editors
- BMJ Journals More You are viewing from: Google Indexer
You are here
- Volume 58, Issue 11
- Research in specialist sport and exercise medicine training
- Article Text
- Article info
- Citation Tools
- Rapid Responses
- Article metrics

- Bruce Hamilton 1 , 2 ,
- http://orcid.org/0000-0002-8413-2814 Larissa Trease 3 , 4 ,
- Corey Cunningham 4 , 5
- 1 Sports Medicine , High Performance Sport New Zealand AUT Millennium Institute of Sport and Health , Auckland , New Zealand
- 2 SPRINZ , Auckland University of Technology , Auckland , New Zealand
- 3 La Trobe Sport and Exercise Medicine Research Centre (LASEM) , La Trobe University , Bundoora , Victoria , Australia
- 4 Australasian College of Sport and Exercise Physicians , Melbourne , Victoria , Australia
- 5 New South Wales Institute of Sport , Sydney Olympic Park , New South Wales , Australia
- Correspondence to Dr Bruce Hamilton, Sports Medicine, High Performance Sport New Zealand AUT Millennium Institute of Sport and Health, Auckland, New Zealand; bruce.hamilton{at}hpsnz.org.nz
https://doi.org/10.1136/bjsports-2024-108554
Statistics from Altmetric.com
Request permissions.
If you wish to reuse any or all of this article please use the link below which will take you to the Copyright Clearance Center’s RightsLink service. You will be able to get a quick price and instant permission to reuse the content in many different ways.
- Sports medicine
Over 20 years ago, Thomas Best and Domhnall MacAuley rhetorically posited that evidence-based sports medicine was potentially a ‘contradiction in terms’. 1 In 2010, Evert Verhagen and Willem van Mechelen stated that ‘most individuals involved in sports medicine are not thoroughly trained in epidemiological and methodological rigour’. 2 Despite these somewhat disparaging views, research has long been recognised as an important component of specialist training in sport and exercise medicine, 3 4 at least in part as a result of academic medical centres demonstrating better patient outcomes. 5 Indeed, the Australasian College of Sport and Exercise Physicians (ACSEP) has centralised the role of research in sports medicine training since its inception in 1985, incorporating a requirement to complete original research as part of fellowship training. 6 Until 2023, in order to graduate from the training programme, registrars were required to complete a series of mandatory research modules and undertake ‘an original research project, and [be] published as first author in an international refereed journal’. 7
As part of an internal 2022 review of the college’s research requirements, several limitations with this research approach were identified including:
A focus on publication in a high-level journal as a binary outcome, rather than the process of research.
Inconsistency with the research requirements with other specialist training programmes in Australia and New Zealand. 8
A reliance on the nuances and publication imperatives of academic journals to determine registrar research outcomes, with resultant delays, difficulties in publishing and an inability to complete the fellowship requirements.
A lack of focus on identifying and developing research competencies.
A lack of access for registrars to research environments, resources and technical capability.
Registrar dissatisfaction, frustration and disengagement with research activities.
The review highlighted a conflict between the desirability of incorporating research requirements into specialist sports medicine training, and the unavoidable challenges of performing quality research. Reflecting this, the Medical Council of New Zealand specifically highlights the importance of ‘enquiry, intellectual curiosity and evidence-based practice’ in specialist training, but also acknowledges that ‘not all trainees will have the inclination, opportunity or aptitude for an extended period of research activity’. 9
Following the 2022 review, the ACSEP ‘doubled down’ on its desire to develop specialists who were competent in critically interpreting, applying and undertaking sports medicine research. While recognising that trainee approaches to research engagement may vary, all trainees are required to contribute to or lead a research study. 8 In essence, the college recognised that while not all specialist sport and exercise registrars were destined to be researchers, all specialists must be able to engage positively in research activity. One size does not fit all. Subsequently, the college overhauled the training programme approach to research with the goal of achieving greater research engagement from both registrars and fellows.
In 2023, the ACSEP formally evolved its training requirements to a competency-based assessment with the removal of the singular publication outcome requirement and providing a range of means by which registrars could complete their individualised ‘research-based activity (RBA)’. While the participation in original research remained a requirement, evidence of developing research competencies such as the formulation of research questions and hypotheses, literature reviews and the development of a research methodology allowed registrars to establish a broad research portfolio in order to complete the training requirements. Furthermore, evidence of ongoing involvement in research and the demonstration of the translation of novel research in sporting or clinical environments can contribute to a registrar’s RBA portfolio.
In recognition of the constraints many registrars face in linking with effective research environments and supervisors, the ACSEP has recruited a technical advisor to support registrars in developing appropriate projects and to guide them towards national and international research support networks. Finally, the college has committed to promoting research from its registrars and fellows with a view to ensuring the ongoing involvement in research is seen as a viable and rewarding professional pathway for sport and exercise physicians.
For the specialty of sport and exercise medicine to thrive requires highly skilled and informed clinicians who are able to interpret and use a broad range of 21st century research techniques. In modernising its research curriculum, the ACSEP hopes to be at the forefront of clinical and evidence-based sports medicine in the decades to come. Evidence-based sports medicine should not be a contradiction.
Ethics statements
Patient consent for publication.
Not applicable.
Ethics approval
- MacAuley D ,
- Verhagen E ,
- van Mechelen W
- Humphries D ,
- Dijkstra HP , et al
- Khullar D ,
- Orav EJ , et al
- Brukner PD ,
- Crichton KJ ,
- Stehlik P ,
- Brandenburg C , et al
X @DrLarissaTrease
Contributors All authors contributed to the development of this editorial.
Funding The authors have not declared a specific grant for this research from any funding agency in the public, commercial or not-for-profit sectors.
Competing interests CC is the president of the Australasian College of Sport and Exercise Physicians (ACSEP) and BH is the chair of the Research Committee of ACSEP.
Provenance and peer review Not commissioned; externally peer reviewed.
Read the full text or download the PDF:
- Open access
- Published: 30 May 2024
Differential attainment in assessment of postgraduate surgical trainees: a scoping review
- Rebecca L. Jones 1 , 2 ,
- Suwimol Prusmetikul 1 , 3 &
- Sarah Whitehorn 1
BMC Medical Education volume 24 , Article number: 597 ( 2024 ) Cite this article
66 Accesses
Metrics details
Introduction
Solving disparities in assessments is crucial to a successful surgical training programme. The first step in levelling these inequalities is recognising in what contexts they occur, and what protected characteristics are potentially implicated.
This scoping review was based on Arksey & O’Malley’s guiding principles. OVID and Embase were used to identify articles, which were then screened by three reviewers.
From an initial 358 articles, 53 reported on the presence of differential attainment in postgraduate surgical assessments. The majority were quantitative studies (77.4%), using retrospective designs. 11.3% were qualitative. Differential attainment affects a varied range of protected characteristics. The characteristics most likely to be investigated were gender (85%), ethnicity (37%) and socioeconomic background (7.5%). Evidence of inequalities are present in many types of assessment, including: academic achievements, assessments of progression in training, workplace-based assessments, logs of surgical experience and tests of technical skills.
Attainment gaps have been demonstrated in many types of assessment, including supposedly “objective” written assessments and at revalidation. Further research is necessary to delineate the most effective methods to eliminate bias in higher surgical training. Surgical curriculum providers should be informed by the available literature on inequalities in surgical training, as well as other neighbouring specialties such as medicine or general practice, when designing assessments and considering how to mitigate for potential causes of differential attainment.
Peer Review reports
Diversity in the surgical workforce has been a hot topic for the last 10 years, increasing in traction following the BlackLivesMatter movement in 2016 [ 1 ]. In the UK this culminated in publication of the Kennedy report in 2021 [ 2 ]. Before this the focus was principally on gender imbalance in surgery, with the 2010 Surgical Workforce report only reporting gender percentages by speciality, with no comment on racial profile, sexuality distribution, disability occurrence, or socioeconomic background [ 3 ].
Gender is not the only protected characteristic deserving of equity in surgery; many groups find themselves at a disadvantage during postgraduate surgical examinations [ 4 ] and at revalidation [ 5 ]. This phenomenon is termed ‘differential attainment’ (DA), in which disparities in educational outcomes, progression rates, or achievements between groups with protected characteristics occur [ 4 ]. This may be due to the assessors’ subconscious bias, or a deficit in training and education before assessment.
One of the four pillars of medical ethics is “justice”, emphasising that healthcare should be provided in a fair, equitable, and ethical manner, benefiting all individuals and promoting the well-being of society as a whole. This applies not only to our patients but also to our colleagues; training should be provided in a fair, equitable, and ethical manner, benefiting all. By applying the principle of justice to surgical trainees, we can create an environment that is supportive, inclusive, and conducive to professional growth and well-being.
A diverse consultant body is crucial for providing high-quality healthcare to a diverse patient population. It has been shown that patients are happier when cared for by a doctor with the same ethnic background [ 6 ]. Takeshita et al. [ 6 ] proposed this is due to a greater likelihood of mutual understanding of cultural values, beliefs, and preferences and is therefore more likely to cultivate a trusting relationship, leading to accurate diagnosis, treatment adherence and improved patient understanding. As such, ensuring that all trainees are justly educated and assessed throughout their training may contribute to improving patient care by diversifying the consultant body.
Surgery is well known to have its own specific culture, language, and social rules which are unique even within the world of medicine [ 7 , 8 ]. Through training, graduates develop into surgeons, distinct from other physicians and practitioners [ 9 ]. As such, research conducted in other medical domains is not automatically applicable to surgery, and behavioural interventions focused on reducing or eliminating bias in training need to be tailored specifically to surgical settings.
Consequently, it’s important that the surgical community asks the questions:
Does DA exist in postgraduate surgical training, and to what extent?
Why does DA occur?
What groups or assessments are under-researched?
How can we apply this knowledge, or acquire new knowledge, to provide equity for trainees?
The following scoping review hopes to provide the surgical community with robust answers for future of surgical training.
Aims and research question
The aim of this scoping review is to understand the breadth of research about the presence of DA in postgraduate surgical education and to determine themes pertaining to causes of inequalities. A scoping review was chosen to provide a means to map the available literature, including published peer-reviewed primary research and grey literature.
Following the methodological framework set out by Arksey and O’Malley [ 10 ], our research was intended to characterise the literature addressing DA in HST, including Ophthalmology, Obstetrics & Gynaecology (O&G). We included literature from English-language speaking countries, including the UK and USA.
Search strategy
We used search terms tailored to our target population characteristics (e.g., gender, ethnicity), concept (i.e., DA) and context (i.e., assessment in postgraduate surgical education). Medline and Embase were searched with the assistance of a research librarian, with addition of synonyms. This was conducted in May 2023, and was exported to Microsoft Excel for further review. The reference lists of included articles were also searched to find any relevant data sources that had yet to be considered. In addition, to identify grey literature, a search was performed for the term “differential attainment” and “disparity” on the relevant stakeholders’ websites (See supplemental Table 1 for full listing). Stakeholders were included on the basis of their involvement in governance or training of surgical trainees.
Study selection
To start we excluded conference abstracts that were subsequently published as full papers to avoid duplications ( n = 337). After an initial screen by title to exclude obviously irrelevant articles, articles were filtered to meet our inclusion and exclusion criteria (Table 1 ). The remaining articles ( n = 47) were then reviewed in their entirety, with the addition of five reports found in grey literature. Following the screening process, 45 studies were recruited for scoping review (Fig. 1 ).
Charting the data
The extracted data included literature title, authors, year of publication, country of study, study design, population characteristic, case number, context, type of assessment, research question and main findings (Appendix 1). Extraction was performed initially by a single author and then subsequently by a second author to ensure thorough review. Group discussion was conducted in case of any disagreements. As charting occurred, papers were discovered within reference lists of included studies which were eligible for inclusion; these were assimilated into the data charting table and included in the data extraction ( n = 8).
Collating, summarizing and reporting the results
The included studies were not formally assessed in their quality or risk of bias, consistent with a scoping review approach [ 10 ]. However, group discussion was conducted during charting to aid argumentation and identify themes and trends.
We conducted a descriptive numerical summary to describe the characteristics of included studies. Then thematic analysis was implemented to examine key details and organise the attainment quality and population characteristics based on their description. The coding of themes was an iterative process and involved discussion between authors, to identify and refine codes to group into themes.
We categorised the main themes as gender, ethnicity, country of graduation, individual and family background in education, socioeconomic background, age, and disability. The number of articles in each theme is demonstrated in Table 2 . Data was reviewed and organised into subtopics based on assessment types included: academic achievement (e.g., MRCS, FRCS), assessments for progression (e.g., ARCP), workplace-based assessment (e.g., EPA, feedback), surgical experience (e.g., case volume), and technical skills (e.g., visuo-spatial tasks).
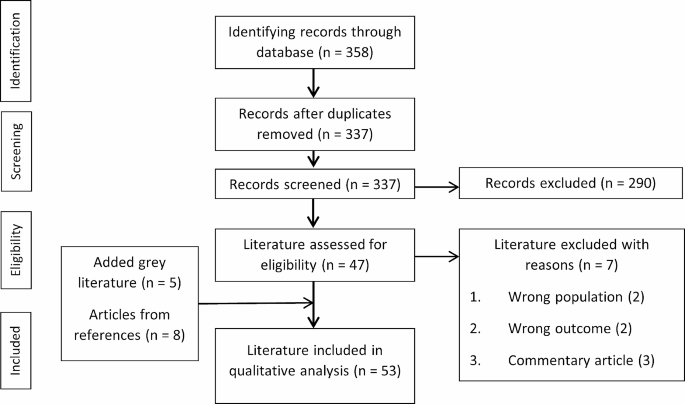
PRISMA flow diagram
44 articles defined the number of included participants (89,399 participants in total; range of participants across individual studies 16–34,755). Two articles reported the number of included studies for their meta-analysis (18 and 63 included articles respectively). Two reports from grey literature did not define the number of participants they included in their analysis. The characteristics of the included articles are displayed in Table 2 .
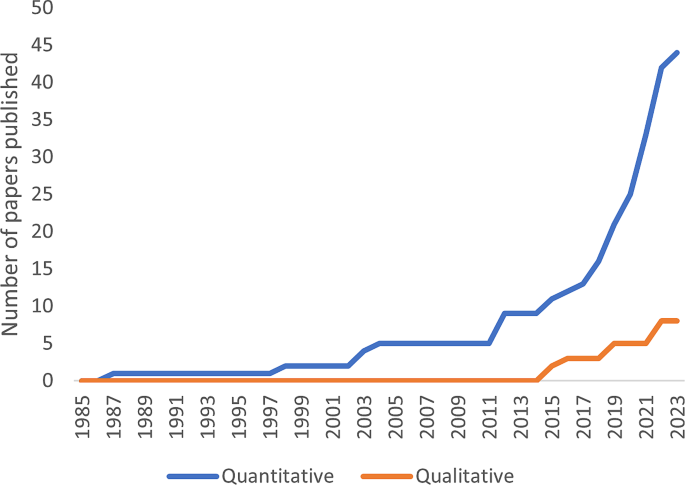
Growth in published literature on differential attainment over the past 40 years
Academic achievement
In the American Board of Surgery Certifying Exam (ABSCE), Maker [ 11 ] found there to be no significant differences in terms of gender when comparing those who passed on their first attempt and those who did not in general surgery training, a finding supported by Ong et al. [ 12 ]. Pico et al. [ 13 ] reported that in Orthopaedic training, Orthopaedic In-Training Examination (OITE) and American Board of Orthopaedic Surgery (ABOS) Part 1 scores were similar between genders, but that female trainees took more attempts in order to pass. In the UK, two studies reported significantly lower Membership of the Royal College of Surgeons (MRCS) pass rates for female trainees compared to males [ 4 , 14 ]. However, Robinson et al. [ 15 ] presented no significant gender differences in MRCS success rates. A study assessing Fellowship of the Royal College of Surgeons (FRCS) examination results found no significant gender disparities in pass rates [ 16 ]. In MRCOG examination, no significant gender differences were found in Part 1 scores, but women had higher pass rates and scores in Part 2 [ 17 ].
Assessment for Progression
ARCP is the annual process of revalidation that UK doctors must perform to progress through training. A satisfactory progress outcome (“outcome 1”) allows trainees to advance through to the next training year, whereas non-satisfactory outcomes (“2–5”) suggest inadequate progress and recommends solutions, such as further time in training or being released from the training programme. Two studies reported that women received 60% more non-satisfactory outcomes than men [ 16 , 18 ]. In contrast, in O&G men had higher non-satisfactory ARCP outcomes without explicit reasons for this given [ 19 ].
Regarding Milestone evaluations based from the US Accreditation Council for Graduate Medical Education (ACGME), Anderson et al. [ 20 ] reported men had higher ratings of knowledge of diseases at postgraduate year 5 (PGY-5), while women had lower mean score achievements. This was similar to another study finding that men and women had similar competencies at PGY-1 to 3, and that it was only at PGY-5 that women were evaluated lower than men [ 21 ]. However, Kwasny et al. [ 22 ] found no difference in trainers’ ratings between genders, but women self-rated themselves lower. Salles et al. [ 23 ] demonstrated significant improvement in scoring in women following a value-affirmation intervention, while this intervention did not affect men.
Workplace-based Assessment
Galvin et al. [ 24 ] reported better evaluation scores from nurses for PGY-2 male trainees, while females received fewer positive and more negative comments. Gerull et al. [ 25 ] demonstrated men received compliments with superlatives or standout words, whereas women were more likely to receive compliments with mitigating phrases (e.g., excellent vs. quite competent).
Hayward et al. [ 26 ] investigated assessment of attributes of clinical performance (ethics, judgement, technical skills, knowledge and interpersonal skills) and found similar scoring between genders.
Several authors have studied autonomy given to trainees in theatre [ 27 , 28 , 29 , 30 , 31 ]. Two groups found no difference in level of granted autonomy between genders but that women rated lower perceived autonomy on self-evaluation [ 27 , 28 ]. Other studies found that assessors consistently gave female trainees lower autonomy ratings, but only in one paper was this replicated in lower performance scores [ 29 , 30 , 31 ].
Padilla et al. [ 32 ] reported no difference in entrustable professional activity assessment (EPA) levels between genders, yet women rated themselves much lower, which they regarded as evidence of imposter syndrome amongst female trainees. Cooney et al. [ 33 ] found that male trainers scored EPAs for women significantly lower than men, while female trainers rated both genders similarly. Conversely, Roshan et al. [ 34 ] found that male assessors were more positive in feedback comments to female trainees than male trainees, whereas they also found that comments from female assessors were comparable for each gender.
Surgical Experience
Gong et al. [ 35 ] found significantly fewer cataract operations were performed by women in ophthalmology residency programmes, which they suggested could be due to trainers being more likely to give cases to male trainees. Female trainees also participated in fewer robotic colorectal procedures, with less operative time on the robotic console afforded [ 36 ]. Similarly, a systematic review highlighted female trainees in various specialties performed fewer cases per week and potentially had limited access to training facilities [ 37 ]. Eruchalu et al. [ 38 ] found that female trainees performed fewer cases, that is, until gender parity was reached, after which case logs were equivalent.
Technical skills
Antonoff et al. [ 39 ] found higher scores for men in coronary anastomosis skills, with women receiving more “fail” assessments. Dill-Macky et al. [ 40 ] analysed laparoscopic skill assessment using blinded videos of trainees and unblinded assessments. While there was no difference in blinded scores between genders, when comparing blinded and unblinded scores individually, assessors were less likely to agree on the scores of women compared to men. However, another study about laparoscopic skills by Skjold-Ødegaard et al. [ 41 ] reported higher performance scores in female residents, particularly when rated by women. The lowest score was shown in male trainees rated by men. While some studies showed disparities in assessment, several studies reported no difference in technical skill assessments (arthroscopic, knot tying, and suturing skills) between genders [ 42 , 43 , 44 , 45 , 46 ].
Several studies investigated trainees’ abilities to complete isolated tasks associated with surgical skills. In laparoscopic tasks, men were initially more skilful in peg transfer and intracorporeal knot tying than women. Following training, the performance was not different between genders [ 47 ]. A study on microsurgical skills reported better initial visual-spatial and perceptual ability in men, while women had better fine motor psychomotor ability. However, these differences were not significant, and all trainees improved significantly after training [ 48 ]. A study by Milam et al. [ 49 ] revealed men performed better in mental rotation tasks and women outperformed in working memory. They hypothesised that female trainees would experience stereotype threat, fear of being reduced to a stereotype, which would impair their performance. They found no evidence of stereotype threat influencing female performance, disproving their hypothesis, a finding supported by Myers et al. [ 50 ].
Ethnicity and country of graduation
Most papers reported ethnicity and country of graduation concurrently, for example grouping trainees as White UK graduates (WUKG), Black and minority ethnicity UK graduates (BME UKG), and international medical graduates (IMG). Therefore, these areas will be addressed together in the following section.
When assessing the likelihood of passing American Board of Surgery (ABS) examinations on first attempt, Yeo et al. [ 51 ] found that White trainees were more likely than non-White. They found that the influence of ethnicity was more significant in the end-of-training certifying exam than in the start-of-training qualifying exam. This finding was corroborated in a study of both the OITE and ABOS certifying exam, suggesting widening inequalities during training [ 52 ].
Two UK-based studies reported significantly higher MRCS pass rates in White trainees compared to BMEs [ 4 , 14 ]. BMEs were less likely to pass MRCS Part A and B, though this was not true for Part A when variations in socioeconomic background were corrected for [ 14 ]. However, Robinson et al. [ 53 ] found no difference in MRCS pass rates based on ethnicity. Another study by Robinson et al. [ 15 ] demonstrated similar pass rates between WUKGs and BME UKGs, but IMGs had significantly lower pass rates than all UKGs. The FRCS pass rates of WUKGs, BME UKGs and IMGs were 76.9%, 52.9%, and 53.9%, respectively, though these percentages were not statistically significantly different [ 16 ].
There was no difference in MRCOG results based on ethnicity, but higher success rates were found in UKGs [ 19 ]. In FRCOphth, WUKGs had a pass rate of 70%, higher than other groups of trainees, with a pass rate of only 45% for White IMGs [ 52 ].
By gathering data from training programmes reporting little to no DA due to ethnicity, Roe et al. [ 54 ] were able to provide a list of factors they felt were protective against DA, such as having supportive supervisors and developing peer networks.
Assessment for progression
RCOphth [ 55 ] found higher rates of satisfactory ARCP outcomes for WUKGs compared to BME UKGs, followed by IMGs. RCOG [ 19 ] discovered higher rates of non-satisfactory ARCP outcomes from non-UK graduates, particularly amongst BMEs and those from the European Economic Area (EEA). Tiffin et al. [ 56 ] considered the difference in experience between UK graduates and UK nationals whose primary medical qualification was gained outside of the UK, and found that the latter were more likely to receive a non-satisfactory ARCP outcome, even when compared to non-UK nationals.
Woolf et al. [ 57 ] explored reasons behind DA by conducting interview studies with trainees. They investigated trainees’ perceptions of fairness in evaluation and found that trainees felt relationships developed with colleagues who gave feedback could affect ARCP results, and might be challenging for BME UKGs and IMGs who have less in common with their trainers.
Workplace-based assessment
Brooks et al. [ 58 ] surveyed the prevalence of microaggressions against Black orthopaedic surgeons during assessment and found 87% of participants experienced some level of racial discrimination during workplace-based performance feedback. Black women reported having more racially focused and devaluing statements from their seniors than men.
Surgical experience
Eruchalu et al. [ 38 ] found that white trainees performed more major surgical cases and more cases as a supervisor than did their BME counterparts.
Dill-Macky et al. [ 40 ] reported no significant difference in laparoscopic surgery assessments between ethnicities.
Individual and family background in education
Two studies [ 4 , 16 ] concentrated on educational background, considering factors such as parental occupation and attendance of a fee-paying school. MRCS part A pass rate was significantly higher for trainees for whom Medicine was their first Degree, those with university-educated parents, higher POLAR (Participation In Local Areas classification group) quintile, and those from fee-paying schools. Higher part B pass rate was associated with graduating from non-Graduate Entry Medicine programmes and parents with managerial or professional occupations [ 4 ]. Trainees with higher degrees were associated with an almost fivefold increase in FRCS success and seven times more scientific publications than their counterparts [ 16 ].
Socioeconomic background
Two studies used Index of Multiple Deprivation quintile, the official measure of relative deprivation in England based on geographical areas for grading socioeconomic level. The area was defined at the time of medical school application. Deprivation quintiles (DQ) were calculated, ranging from DQ1 (most deprived) to DQ5 (least deprived) [ 4 , 14 ].
Trainees with history of less deprivation were associated with higher MRCS part A pass rate. More success in part B was associated with history of no requirement for income support and less deprived areas [ 4 ]. Trainees from DQ1 and DQ2 had lower pass rates and higher number of attempts to pass [ 14 ]. A general trend of better outcomes in examination was found from O&G trainees in less deprived quintiles [ 19 ].
Trainees from DQ1 and DQ2 received significantly more non-satisfactory ARCP outcomes (24.4%) than DQ4 and DQ5 (14.2%) [ 14 ].
Trainees who graduated at age less than 29 years old were more likely to pass MRCS than their counterparts [ 4 ].
Authors [ 18 , 56 ] found that older trainees received more non-satisfactory ARCP outcomes. Likewise, there was higher percentage of non-satisfactory ARCP outcomes in O&G trainees aged over 45 compared with those aged 25–29 regardless of gender [ 19 ].
Trainees with disability had significantly lower pass rates in MRCS part A compared to candidates without disability. However, the difference was not significant for part B [ 59 ].
What have we learnt from the literature?
It is heartening to note the recent increase in interest in DA (27 studies in the last 4 years, compared to 26 in the preceding 40) (Fig. 2 ). The vast majority (77%) of studies are quantitative, based in the US or UK (89%), focus on gender (85%) and relate to clinical assessments (51%) rather than examination results. Therefore, the surgical community has invested primarily in researching the experience of women in the USA and UK.
Interestingly, a report by RCOG [ 19 ] showed that men were more likely to receive non-satisfactory ARCP outcomes than women, and a study by Rushd et al. [ 17 ] found that women were more likely to pass part 2 of MRCOG than men. This may be because within O&G men are the “out-group” (a social group or category characterised by marginalisation or exclusion by the dominant cultural group) as 75% of O&G trainees are female [ 60 ].
This contrasts with other specialities in which men are the in-group and women are seen to underperform. Outside of O&G, in comparison to men, women are less likely to pass MRCS [ 4 , 14 ], receive satisfactory ARCP outcome [ 16 , 18 ], or receive positive feedback [ 24 ], whilst not performing the same number of procedures as men [ 34 , 35 ]. This often leads to poor self-confidence in women [ 32 ], which can then worsen performance [ 21 ].
It proves difficult to comment on DA for many groups due to a lack of evidence. The current research suggests that being older, having a disability, graduate entry to medicine, low parental education, and living in a lower socioeconomic area at the time of entering medical school are all associated with lower MRCS pass rates. Being older and having a lower socioeconomic background are also associated with non-satisfactory ARCP outcomes, slowing progression through training.
These characteristics may provide a compounding negative effect – for example having a previous degree will automatically make a trainee older, and living in a lower socioeconomic area makes it more likely their parents will have a non-professional job and not hold a higher degree. When multiple protected characteristics interact to produce a compounded negative effect for a person, it is often referred to as “intersectional discrimination” or “intersectionality” [ 61 ]. This is a concept which remains underrepresented in the current literature.
The literature is not yet in agreement over the presence of DA due to ethnicity. There are many studies that report perceived discrimination, however the data for exam and clinical assessment outcomes is equivocal. This may be due to the fluctuating nature of in-groups and out-groups, and multiple intersecting characteristics. Despite this, the lived experience of BME surgeons should not be ignored and requires further investigation.
What are the gaps in the literature?
The overwhelming majority of literature exploring DA addresses issues of gender, ethnicity or country of medical qualification. Whilst bias related to these characteristics is crucial to recognise, studies into other protected characteristics are few and far between. The only paper on disability reported striking differences in attainment between disabled and non-disabled registrars [ 59 ]. There has also been increased awareness about neurodiversity amongst doctors and yet an exploration into the experience of neurodiverse surgeons and their progress through training has yet to be published [ 62 ].
The implications of being LGBTQ + in surgical training have not been recognised nor formally addressed in the literature. Promisingly, the experiences of LGBTQ + medical students have been recognised at an undergraduate level, so one can hope that this will be translated into postgraduate education [ 63 , 64 ]. While this is deeply entwined with experiences of gender discrimination, it is an important characteristic that the surgical community would benefit from addressing, along with disability. To a lesser extent, the effect of socioeconomic background and age have also been overlooked.
Characterising trainees for the purpose of research
Ethnicity is deeply personal, self-defined, and may change over time as personal identity evolves, and therefore arbitrarily grouping diverse ethnic backgrounds is unlikely to capture an accurate representation of experiences. There are levels of discrimination even within minority groups; colourism in India means dark-skinned Indians will experience more discrimination than light-skinned Indians, even from those within in their own ethnic group [ 65 ]. Therefore, although the studies included in the scoping review accepted self-definitions of ethnicity, this is likely not enough to fully capture the nuances of bias and discrimination present in society. For example, Ellis et al. [ 4 ] grouped participants as “White”, “Mixed”, “Asian”, “Black” and “Other”, however they could have also assigned a skin tone value such as the NIS Skin Colour Scale [ 66 ], thus providing more detail.
Ethnicity is more than genetic heritage; it is also cultural expression. The experience of an IMG in UK postgraduate training will differ from that of a UKG, an Indian UKG who grew up in India, and an Indian UKG who grew up in the UK. These are important distinctions which are noted in the literature (e.g. by Woolf et al., 2016 [ 57 ]) however some do not distinguish between ethnicity and graduate status [ 15 ] and none delve into an individual’s cultural expression (e.g., clothing choice) and how this affects the perception of their assessors.
Reasons for DA
Despite the recognition of inequalities in all specialties of surgery, there is a paucity of data explicitly addressing why DA occurs. Reasons behind the phenomenon must be explored to enable change and eliminate biases. Qualitative research is more attuned to capturing the complexities of DA through observation or interview-based studies. Currently most published data is quantitative, and relies on performance metrics to demonstrate the presence of DA while ignoring the causes. Promisingly, there are a gradually increasing number of qualitative, predominantly interview-based, studies (Fig. 2 ).
To create a map of DA in all its guises, an analysis of the themes reported to be contributory to its development is helpful. In our review of the literature, four themes have been identified:
Training culture
In higher surgical training, for there to be equality in outcomes, there needs to be equity in opportunities. Ellis et al. [ 4 ] recognised that variation in training experiences, such as accessibility of supportive peers and senior role models, can have implications on attainment. Trainees would benefit from targeted support at times of transition, such as induction or at examinations, and it may be that currently the needs of certain groups are being met before others, reinforcing differential attainment [ 4 ].
Experience of assessment
Most literature in DA relates to the presence (or lack of) an attainment gap in assessments, such as ARCP or MRCS. It is assumed that these assessments of trainee development are objective and free of bias, and indeed several authors have described a lack of bias in these high-stakes examinations (e.g., Ong et al., 2019 [ 12 ]; Robinson et al., 2019 [ 53 ]). However, in some populations, such as disabled trainees, there are differences in attainment [ 59 ]. This is demonstrated despite legislation requiring professional bodies to make reasonable adjustments to examinations for disabled candidates, such as additional time, text formatting amendments, or wheelchair-accessible venues [ 67 ]. Therefore it would be beneficial to investigate the implementation of these adjustments across higher surgical examinations and identify any deficits.
Social networks
Relationships between colleagues may influence DA in multiple ways. Several studies identified that a lack of a relatable and inspiring mentor may explain why female or BME doctors fail to excel in surgery [ 4 , 55 ]. Certain groups may receive preferential treatment due to their perceived familiarity to seniors [ 35 ]. Robinson et al. [ 15 ] recognised that peer-to-peer relationships were also implicated in professional development, and the lack thereof could lead to poor learning outcomes. Therefore, a non-discriminatory culture and inclusion of trainees within the social network of training is posited as beneficial.
Personal characteristics
Finally, personal factors directly related to protected characteristics have been suggested as a cause of DA. For example, IMGs may perform worse in examinations due to language barriers, and those from disadvantaged backgrounds may have less opportunity to attend expensive courses [ 14 , 16 ]. Although it is impossible to exclude these innate deficits from training, we may mitigate their influence by recognising their presence and providing solutions.
The causes of DA may also be grouped into three levels, as described by Regan de Bere et al. [ 68 ]: macro (the implications of high-level policy), meso (focusing on institutional or working environments) and micro (the influence of individual factors). This can intersect with the four themes identified above, as training culture can be enshrined at both an institutional and individual level, influencing decisions that relate to opportunities for trainees, or at a macro level, such as in the decisions made on nationwide recruitment processes. These three levels can be used to more deeply explore each of the four themes to enrich the discovery of causes of DA.
Discussions outside of surgery
Authors in General Practice (e.g., Unwin et al., 2019 [ 69 ]; Pattinson et al., 2019 [ 70 ]), postgraduate medical training (e.g., Andrews, Chartash, and Hay, 2021 [ 71 ]), and undergraduate medical education (e.g., Yeates et al., 2017 [ 72 ]; Woolf et al., 2013 [ 73 ]) have published more extensively in the aetiology of DA. A study by Hope et al. [ 74 ] evaluating the bias present in MRCP exams used differential item functioning to identify individual questions which demonstrated an attainment gap between male and female and Caucasian and non-Caucasian medical trainees. Conclusions drawn about MRCP Part 1 examinations may be generalisable to MRCS Part A or FRCOphth Part 1: they are all multiple-choice examinations testing applied basic science and usually taken within the first few years of postgraduate training. Therefore it is advisable that differential item functioning should also be applied to these examinations. However, it is possible that findings in some subspecialities may not be generalisable to others, as training environments can vary profoundly. The RCOphth [ 55 ] reported that in 2021, 53% of ophthalmic trainees identified as male, whereas in Orthopaedics 85% identified as male, suggesting different training environments [ 5 ]. It is useful to identify commonalities of DA between surgical specialties and in the wider scope of medical training.
Limitations of our paper
Firstly, whilst aiming to provide a review focussed on the experience of surgical trainees, four papers contained data about either non-surgical trainees or medical students. It is difficult to draw out the surgeons from this data and therefore it is possible that there are issues with generalisability. Furthermore, we did not consider the background of each paper’s authors, as their own lived experience of attainment gap could form the lens through which they commented on surgical education, colouring their interpretation. Despite intending to include as many protected characteristics as possible, inevitably there will be lived experiences missed. Lastly, the experience of surgical trainees outside of the English-speaking world were omitted. No studies were found that originated outside of Europe or North America and therefore the presence or characteristics of DA outside of this area cannot be assumed.
Experiences of inequality in surgical assessment are prevalent in all surgical subspecialities. In order to further investigate DA, researchers should ensure all protected characteristics are considered - and how these interact - to gain insight into intersectionality. Given the paucity of current evidence, particular focus should be given to the implications of disability, and specifically neurodiversity, in progress through training as they are yet to be explored in depth. In defining protected characteristics, future authors should be explicit and should avoid generalisation of cultural backgrounds to allow authentic appreciation of attainment gap. Few authors have considered the driving forces between bias in assessment and DA, and therefore qualitative studies should be prioritised to uncover causes for and protective factors against DA. Once these influences have been identified, educational designers can develop new assessment methods that ensure equity across surgical trainees.
Data availability
All data provided during this study are included in the supplementary information files.
Abbreviations
Accreditation Council for Graduate Medical Education
American Board of Orthopaedic Surgery
American Board of Surgery
American Board of Surgery Certifying Exam
Annual Review of Competence Progression
Black, Asian, and Minority Ethnicity
Council on Resident Education in Obstetrics and Gynecology
Differential Attainment
Deprivation Quintile
European Economic Area
Entrustable Professional Activities
Fellowship of The Royal College of Ophthalmologists
Fellow of the Royal College of Surgeons
General Medical Council
Higher Surgical Training
International Medical Graduate
In-Training Evaluation Report
Member of the Royal College of Obstetricians and Gynaecologists
Member of the Royal College of Physicians
Member of the Royal College of Surgeons
Obstetrics and Gynaecology
Orthopaedic In-Training Examination
Participation In Local Areas
Postgraduate Year
The Royal College of Ophthalmologists
The Royal College of Obstetricians and Gynaecologists
The Royal College of Surgeons of England
United Kingdom Graduate
White United Kingdom Graduate
Joseph JP, Joseph AO, Jayanthi NVG, et al. BAME Underrepresentation in Surgery Leadership in the UK and Ireland in 2020: An Uncomfortable Truth. The Bulletin of the Royal College of Surgeons of England. 2020; 102 (6): 232–33.
Royal College of Surgeons of England. The Royal College – Our Professional Home. An independent review on diversity and inclusion for the Royal College of Surgeons of England. Review conducted by Baroness Helena Kennedy QC. RCS England. 2021.
Sarafidou K, Greatorex R. Surgical workforce: planning today for the workforce of the future. Bull Royal Coll Surg Engl. 2011;93(2):48–9. https://doi.org/10.1308/147363511X552575 .
Article Google Scholar
Ellis R, Brennan P, Lee AJ, et al. Differential attainment at MRCS according to gender, ethnicity, age and socioeconomic factors: a retrospective cohort study. J R Soc Med. 2022;115(7):257–72. https://doi.org/10.1177/01410768221079018 .
Hope C, Humes D, Griffiths G, et al. Personal Characteristics Associated with Progression in Trauma and Orthopaedic Specialty Training: A Longitudinal Cohort Study.Journal of Surgical Education 2022; 79 (1): 253–59. doi:10.1016/j.jsurg.2021.06.027.
Takeshita J, Wang S, Loren AW, et al. Association of Racial/Ethnic and Gender Concordance Between Patients and Physicians With Patient Experience Ratings. JAMA Network Open. 2022; 3(11). doi:10.1001/jamanetworkopen.2020.24583.
Katz, P. The Scalpel’s Edge: The Culture of Surgeons. Allyn and Bacon, 1999.
Tørring B, Gittell JH, Laursen M, et al. (2019) Communication and relationship dynamics in surgical teams in the operating room: an ethnographic study. BMC Health Services Research. 2019;19, 528. doi:10.1186/s12913-019-4362-0.
Veazey Brooks J & Bosk CL. (2012) Remaking surgical socialization: work hour restrictions, rites of passage, and occupational identity. Social Science & Medicine. 2012;75(9):1625-32. doi: 10.1016/j.socscimed.2012.07.007.
Arksey H & OʼMalley L. Scoping studies: Towards a methodological framework. International Journal of Social Research Methodology. 2005;8(1), 19–32.
Maker VK, Marco MZ, Dana V, et al. Can We Predict Which Residents Are Going to Pass/Fail the Oral Boards? Journal of Surgical Education. 2012;69 (6): 705–13.
Ong TQ, Kopp JP, Jones AT, et al. Is there gender Bias on the American Board of Surgery general surgery certifying examination? J Surg Res. 2019;237:131–5. https://doi.org/10.1016/j.jss.2018.06.014 .
Pico K, Gioe TJ, Vanheest A, et al. Do men outperform women during orthopaedic residency training? Clin Orthop Relat Res. 2010;468(7):1804–8. https://doi.org/10.1007/s11999-010-1318-4 .
Vinnicombe Z, Little M, Super J, et al. Differential attainment, socioeconomic factors and surgical training. Ann R Coll Surg Engl. 2022;104(8):577–82. https://doi.org/10.1308/rcsann.2021.0255 .
Robinson DBT, Hopkins L, James OP, et al. Egalitarianism in surgical training: let equity prevail. Postgraduate Medical Journal. 2020;96 (1141), 650–654. doi:10.1136/postgradmedj-2020-137563.
Luton OW, Mellor K, Robinson DBT, et al. Differential attainment in higher surgical training: scoping pan-specialty spectra. Postgraduate Medical Journal. 2022;99(1174),849–854. doi:10.1136/postgradmedj-2022-141638.
Rushd S, Landau AB, Khan JA, Allgar V & Lindow SW. An analysis of the performance of UK medical graduates in the MRCOG Part 1 and Part 2 written examinations. Postgraduate Medical Journal. 2012;88 (1039), 249–254. doi:10.1136/postgradmedj-2011-130479.
Hope C, Lund J, Griffiths G, et al. Differences in ARCP outcome by surgical specialty: a longitudinal cohort study. Br J Surg. 2021;108. https://doi.org/10.1093/bjs/znab282.051 .
Royal College of Obstetricians and Gynaecologists. Report Differential Attainment 2019. https://www.rcog.org.uk/media/jscgfgwr/differential-attainment-tef-report-2019.pdf [Last accessed 28/12/23].
Anderson JE, Zern NK, Calhoun KE, et al. Assessment of Potential Gender Bias in General Surgery Resident Milestone Evaluations. JAMA Surgery. 2022;157 (12), 1164–1166. doi:10.1001/jamasurg.2022.3929.
Landau SI, Syvyk S, Wirtalla C, et al. Trainee Sex and Accreditation Council for Graduate Medical Education Milestone Assessments during general surgery residency. JAMA Surg. 2021;156(10):925–31. https://doi.org/10.1001/jamasurg.2021.3005 .
Kwasny L, Shebrain S, Munene G, et al. Is there a gender bias in milestones evaluations in general surgery residency training? Am J Surg. 2021;221(3):505–8. https://doi.org/10.1016/j.amjsurg.2020.12.020 .
Salles A, Mueller CM & Cohen GL. A Values Affirmation Intervention to Improve Female Residents’ Surgical Performance. Journal of Graduate Medical Education. 2016;8 (3), 378–383. doi:10.4300/JGME-D-15-00214.1.
Galvin S, Parlier A, Martino E, et al. Gender Bias in nurse evaluations of residents in Obstetrics and Gynecology. Obstet Gynecol. 2015;126(7S–12S). https://doi.org/10.1097/AOG.0000000000001044 .
Gerull KM, Loe M, Seiler K, et al. Assessing gender bias in qualitative evaluations of surgical residents. Am J Surg. 2019;217(2):306–13. https://doi.org/10.1016/j.amjsurg.2018.09.029 .
Hayward CZ, Sachdeva A, Clarke JR. Is there gender bias in the evaluation of surgical residents? Surgery. 1987;102(2):297–9.
Google Scholar
Cookenmaster C, Shebrain S, Vos D, et al. Gender perception bias of operative autonomy evaluations among residents and faculty in general surgery training. Am J Surg. 2021;221(3):515–20. https://doi.org/10.1016/j.amjsurg.2020.11.016 .
Olumolade OO, Rollins PD, Daignault-Newton S, et al. Closing the Gap: Evaluation of Gender Disparities in Urology Resident Operative Autonomy and Performance.Journal of Surgical Education.2022;79 (2), 524–530. doi.org/10.1016/j.jsurg.2021.10.010.
Chen JX, Chang EH, Deng F, et al. Autonomy in the Operating Room: A Multicenter Study of Gender Disparities During Surgical Training. Journal of Graduate Medical Education. 2021;13(5), 666–672. doi: 10.4300/JGME-D-21-00217.1.
Meyerson SL, Sternbach JM, Zwischenberger JB, & Bender EM. The Effect of Gender on Resident Autonomy in the Operating room. Journal of Surgical Education. 2017. 74(6), e111–e118. doi.org/10.1016/j.jsurg.2017.06.014.
Hoops H, Heston A, Dewey E, et al. Resident autonomy in the operating room: Does gender matter? The AmericanJournalofSurgery. 2019; 217(2), 301–305. doi.org/10.1016/j.amjsurg.2018.12.023.
Padilla EP, Stahl CC, Jung SA, et al. Gender Differences in Entrustable Professional Activity Evaluations of General Surgery Residents. Annals of Surgery. 2022;275 (2), 222–229. doi:10.1097/SLA.0000000000004905.
Cooney CM, Aravind P, Hultman CS, et al. An Analysis of Gender Bias in Plastic Surgery Resident Assessment. Journal of Graduate Medical Education. 2021;13 (4), 500–506. doi:10.4300/JGME-D-20-01394.1.
Roshan A, Farooq A, Acai A, et al. The effect of gender dyads on the quality of narrative assessments of general surgery trainees. The American Journal of Surgery. 2022; 224 (1A), 179–184. doi.org/10.1016/j.amjsurg.2021.12.001.
Gong D, Winn BJ, Beal CJ, et al. Gender Differences in Case Volume Among Ophthalmology Residents. Archives of Ophthalmology. 2019;137 (9), 1015–1020. doi:10.1001/jamaophthalmol.2019.2427.
Foley KE, Izquierdo KM, von Muchow MG, et al. Colon and Rectal Surgery Robotic Training Programs: An Evaluation of Gender Disparities. Diseases of the Colon and Rectum. 2020; 63(7), 974–979. doi.org/10.1097/DCR.0000000000001625.
Ali A, Subhi Y, Ringsted C et al. Gender differences in the acquisition of surgical skills: a systematic review. Surgical Endoscopy. 2015;29 (11), 3065–3073. doi:10.1007/s00464-015-4092-2.
Eruchalu CN, He K, Etheridge JC, et al. Gender and Racial/Ethnic Disparities in Operative Volumes of Graduating General Surgery Residents.The Journal of Surgical Research. 2022; 279, 104–112. doi.org/10.1016/j.jss.2022.05.020.
Antonoff MB, Feldman H, Luc JGY, et al. Gender Bias in the Evaluation of Surgical Performance: Results of a Prospective Randomized Trial. Annals of Surgery. 2023;277 (2), 206–213. doi:10.1097/SLA.0000000000005015.
Dill-Macky A, Hsu C, Neumayer LA, et al. The Role of Implicit Bias in Surgical Resident Evaluations. Journal of Surgical Education. 2022;79 (3), 761–768. doi:10.1016/j.jsurg.2021.12.003.
Skjold-Ødegaard B, Ersdal HL, Assmus J et al. Comparison of Performance Score for Female and Male Residents in General Surgery Doing Supervised Real-Life Laparoscopic Appendectomy: Is There a Norse Shield-Maiden Effect? World Journal of Surgery. 2021;45 (4), 997–1005. doi:10.1007/s00268-020-05921-4.
Leape CP, Hawken JB, Geng X, et al. An investigation into gender bias in the evaluation of orthopedic trainee arthroscopic skills. Journal of Shoulder and Elbow Surgery. 2022;31 (11), 2402–2409. doi:10.1016/j.jse.2022.05.024.
Vogt VY, Givens VM, Keathley CA, et al. Is a resident’s score on a videotaped objective structured assessment of technical skills affected by revealing the resident’s identity? American Journal of Obstetrics and Gynecology. 2023;189 (3), 688–691. doi:10.1067/S0002-9378(03)00887-1.
Fjørtoft K, Konge L, Christensen J et al. Overcoming Gender Bias in Assessment of Surgical Skills. Journal of Surgical Education. 2022;79 (3), 753–760. doi:10.1016/j.jsurg.2022.01.006.
Grantcharov TP, Bardram L, Funch-Jensen P, et al. Impact of Hand Dominance, Gender, and Experience with Computer Games on Performance in Virtual Reality Laparoscopy. Surgical Endoscopy 2003;17 (7): 1082–85.
Rosser Jr JC, Rosser LE & Savalgi RS. Objective Evaluation of a Laparoscopic Surgical Skill Program for Residents and Senior Surgeons. Archives of Surgery. 1998; 133 (6): 657–61.
White MT & Welch K. Does gender predict performance of novices undergoing Fundamentals of Laparoscopic Surgery (FLS) training? The American Journal of Surgery. 2012;203 (3), 397–400. doi:10.1016/j.amjsurg.2011.09.020.
Nugent E, Joyce C, Perez-Abadia G, et al. Factors influencing microsurgical skill acquisition during a dedicated training course. Microsurgery. 2012;32 (8), 649–656. doi:10.1002/micr.22047.
Milam LA, Cohen GL, Mueller C et al. Stereotype threat and working memory among surgical residents. The American Journal of Surgery. 2018;216 (4), 824–829. doi:10.1016/j.amjsurg.2018.07.064.
Myers SP, Dasari M, Brown JB, et al. Effects of Gender Bias and Stereotypes in Surgical Training: A Randomized Clinical Trial. JAMA Surgery. 2020; 155(7), 552–560. doi.org/10.1001/jamasurg.2020.1127.
Yeo HL, Patrick TD, Jialin M, et al. Association of Demographic and Program Factors With American Board of Surgery Qualifying and Certifying Examinations Pass Rates. JAMA Surgery 2020; 155 (1): 22–30. doi:0.1001/jamasurg.2019.4081.
Foster N, Meghan P, Bettger JP, et al. Objective Test Scores Throughout Orthopedic Surgery Residency Suggest Disparities in Training Experience. Journal of Surgical Education 2021;78 (5): 1400–1405. doi:10.1016/j.jsurg.2021.01.003.
Robinson DBT, Hopkins L, Brown C, et al. Prognostic Significance of Ethnicity on Differential Attainment in Core Surgical Training (CST). Journal of the American College of Surgeons. 2019;229 (4), e191. doi:10.1016/j.jamcollsurg.2019.08.1254.
Roe V, Patterson F, Kerrin M, et al. What supported your success in training? A qualitative exploration of the factors associated with an absence of an ethnic attainment gap in post-graduate specialty training. General Medical Council. 2019. https://www.gmc-uk.org/-/media/documents/gmc-da-final-report-success-factors-in-training-211119_pdf-80914221.pdf [Last accessed 28/12/23].
Royal College of Ophthalmologists. Data on Differential attainment in ophthalmology and monitoring equality, diversity, and inclusion: Recommendations to the RCOphth. London, Royal College of Ophthalmologists. 2022. https://www.rcophth.ac.uk/wp-content/uploads/2023/01/Differential-Attainment-Report-2022.pdf [Last accessed 28/12/23].
Tiffin PA, Orr J, Paton LW, et al. UK nationals who received their medical degrees abroad: selection into, and subsequent performance in postgraduate training: a national data linkage study. BMJ Open. 2018;8:e023060. doi: 10.1136/bmjopen-2018-023060.
Woolf K, Rich A, Viney R, et al. Perceived causes of differential attainment in UK postgraduate medical training: a national qualitative study. BMJ Open. 2016;6 (11), e013429. doi:10.1136/bmjopen-2016-013429.
Brooks JT, Porter SE, Middleton KK, et al. The Majority of Black Orthopaedic Surgeons Report Experiencing Racial Microaggressions During Their Residency Training. Clinical Orthopaedics and Related Research. 2023;481 (4), 675–686. doi:10.1097/CORR.0000000000002455.
Ellis R, Cleland J, Scrimgeour D, et al. The impact of disability on performance in a high-stakes postgraduate surgical examination: a retrospective cohort study. Journal of the Royal Society of Medicine. 2022;115 (2), 58–68. doi:10.1177/01410768211032573.
Royal College of Obstetricians & Gynaecologists. RCOGWorkforceReport2022. Available at: https://www.rcog.org.uk/media/fdtlufuh/workforce-report-july-2022-update.pdf [Last accessed 28/12/23].
Crenshaw KW. On Intersectionality: Essential Writings. Faculty Books. 2017; 255.
Brennan CM & Harrison W. The Dyslexic Surgeon. The Bulletin of the Royal College of Surgeons of England. 2020;102 (3): 72–75. doi:10.1308/rcsbull.2020.72.
Toman L. Navigating medical culture and LGBTQ identity. Clinical Teacher. 2019;16: 335–338. doi:10.1111/tct.13078.
Torales J, Castaldelli-Maia JM & Ventriglio A. LGBT + medical students and disclosure of their sexual orientation: more than in and out of the closet. International Review of Psychiatry. 2022;34:3–4, 402–406. doi:10.1080/09540261.2022.2101881.
Guda VA & Kundu RV. India’s Fair Skin Phenomena. SKINmed. 2021;19(3), 177–178.
Massey D & Martin JA. The NIS skin color scale. Princeton University Press. 2003.
Intercollegiate Committee for Basic Surgical Examinations.AccessArrangementsandReasonableAdjustmentsPolicyforCandidateswithaDisabilityorSpecificLearningdifficulty. 2020. https://www.intercollegiatemrcsexams.org.uk/-/media/files/imrcs/mrcs/mrcs-regulations/access-arrangements-and-reasonable-adjustments-january-2020.pdf [Last accessed 28/12/23].
Regan de Bere S, Nunn S & Nasser M. Understanding differential attainment across medical training pathways: A rapid review of the literature. General Medical Council. 2015. https://www.gmc-uk.org/-/media/documents/gmc-understanding-differential-attainment_pdf-63533431.pdf [Last accessed 28/12/23].
Unwin E, Woolf K, Dacre J, et al. Sex Differences in Fitness to Practise Test Scores: A Cohort Study of GPs. The British Journal of General Practice: The Journal of the Royal College of General Practitioners. 2019; 69 (681): e287–93. doi:10.3399/bjgp19X701789.
Pattinson J, Blow C, Sinha B et al. Exploring Reasons for Differences in Performance between UK and International Medical Graduates in the Membership of the Royal College of General Practitioners Applied Knowledge Test: A Cognitive Interview Study. BMJ Open. 2019;9 (5): e030341. doi:10.1136/bmjopen-2019-030341.
Andrews J, Chartash D & Hay S. Gender Bias in Resident Evaluations: Natural Language Processing and Competency Evaluation. Medical Education. 2021;55 (12): 1383–87. doi:10.1111/medu.14593.
Yeates P, Woolf K, Benbow E, et al. A Randomised Trial of the Influence of Racial Stereotype Bias on Examiners’ Scores, Feedback and Recollections in Undergraduate Clinical Exams. BMC Medicine 2017;15 (1): 179. doi:10.1186/s12916-017-0943-0.
Woolf K, McManus IC, Potts HWW et al. The Mediators of Minority Ethnic Underperformance in Final Medical School Examinations. British Journal of Educational Psychology. 2013; 83 (1): 135–59. doi:10.1111/j.2044-8279.2011.02060.x.
Hope D, Adamson K, McManus IC, et al. Using Differential Item Functioning to Evaluate Potential Bias in a High Stakes Postgraduate Knowledge Based Assessment. BMC Medical Education. 2018;18 (1): 64. doi:10.1186/s12909-018-1143-0.
Download references
No sources of funding to be declared.
Author information
Authors and affiliations.
Department of Surgery and Cancer, Imperial College London, London, UK
Rebecca L. Jones, Suwimol Prusmetikul & Sarah Whitehorn
Department of Ophthalmology, Cheltenham General Hospital, Gloucestershire Hospitals NHS Foundation Trust, Alexandra House, Sandford Road, Cheltenham, GL53 7AN, UK
Rebecca L. Jones
Department of Orthopaedics, Faculty of Medicine, Ramathibodi Hospital, Mahidol University, Bangkok, Thailand
Suwimol Prusmetikul
You can also search for this author in PubMed Google Scholar
Contributions
RJ, SP and SW conceived the study. RJ carried out the search. RJ, SP and SW reviewed and appraised articles. RJ, SP and SW extracted data and synthesized results from articles. RJ, SP and SW prepared the original draft of the manuscript. RJ and SP prepared Figs. 1 and 2. All authors reviewed and edited the manuscript and agreed to the final version.
Corresponding author
Correspondence to Rebecca L. Jones .
Ethics declarations
Ethics approval and consent to participate.
Not required for this scoping review.
Consent for publication
Not applicable.
Competing interests
The authors declare no competing interests.
Additional information
Publisher’s note.
Springer Nature remains neutral with regard to jurisdictional claims in published maps and institutional affiliations.
Electronic supplementary material
Below is the link to the electronic supplementary material.
Supplementary Material 1
Supplementary material 2, rights and permissions.
Open Access This article is licensed under a Creative Commons Attribution 4.0 International License, which permits use, sharing, adaptation, distribution and reproduction in any medium or format, as long as you give appropriate credit to the original author(s) and the source, provide a link to the Creative Commons licence, and indicate if changes were made. The images or other third party material in this article are included in the article’s Creative Commons licence, unless indicated otherwise in a credit line to the material. If material is not included in the article’s Creative Commons licence and your intended use is not permitted by statutory regulation or exceeds the permitted use, you will need to obtain permission directly from the copyright holder. To view a copy of this licence, visit http://creativecommons.org/licenses/by/4.0/ . The Creative Commons Public Domain Dedication waiver ( http://creativecommons.org/publicdomain/zero/1.0/ ) applies to the data made available in this article, unless otherwise stated in a credit line to the data.
Reprints and permissions
About this article
Cite this article.
Jones, R.L., Prusmetikul, S. & Whitehorn, S. Differential attainment in assessment of postgraduate surgical trainees: a scoping review. BMC Med Educ 24 , 597 (2024). https://doi.org/10.1186/s12909-024-05580-2
Download citation
Received : 27 February 2024
Accepted : 20 May 2024
Published : 30 May 2024
DOI : https://doi.org/10.1186/s12909-024-05580-2
Share this article
Anyone you share the following link with will be able to read this content:
Sorry, a shareable link is not currently available for this article.
Provided by the Springer Nature SharedIt content-sharing initiative
- Differential attainment
- Postgraduate
BMC Medical Education
ISSN: 1472-6920
- Submission enquiries: [email protected]
- General enquiries: [email protected]
- Access Member Benefits
- Exercise is Medicine

ACSM and CDC recommendations state that:
- All healthy adults aged 18–65 years should participate in moderate intensity aerobic physical activity for a minimum of 30 minutes on five days per week, or vigorous intensity aerobic activity for a minimum of 20 minutes on three days per week.
- Every adult should perform activities that maintain or increase muscular strength and endurance for a minimum of two days per week.
Physical Activity Guidelines for Americans, 2nd Edition
The Physical Activity Guidelines for Americans, 2nd Edition, were published in the fall of 2018. Learn what the recommendations are here.
- Bill Kraus, M.D., FACSM, Introduces ACSM's New Scientific Pronouncements 2019
- Behind the Scenes of ACSM’s Collection of Scientific Pronouncements | Physical Activity Guidelines
Online Learning
- CEPA Webinar: The 2018 Physical Activity Guidelines for Americans: What’s New? | Continuing Education Course
- 2018 Physical Activity Guidelines – How to Meet the Goals in Everyday Activities
- Daily Steps and Health | Walking Your Way to Better Health
- Five Frequently Asked Questions About the Physical Activity Guidelines for Americans, 2nd Edition
- High-Intensity Interval Training: For Fitness, for Health or Both?
- New ACSM Pronouncements Make the Case, Find the Gaps | Introduction to the Physical Activity Guidelines for Americans, 2nd edition and the accompanying ACSM pronouncements
- Paradigm Shift in Physical Activity Research: Do Bouts Matter?
- Physical Activity: A Key Lifestyle Behavior for Prevention of Weight Gain and Obesity
- Physical Activity and Function in Older Age: It’s Never too Late to Start!
- Physical Activity and Health: Does Sedentary Behavior Make a Difference?
- Physical activity, decreased risk for all-cause mortality and cardiovascular disease: No longer any doubt and short bouts count
- Physical Activity for the Prevention and Treatment of Cancer
- What’s New in the ACSM Pronouncement on Exercise and Hypertension?
General Activity and Health Recommendations
- Physical Activity in School-Aged Children | Blog | Infographic
- Being Active as a Teen | Handout
- Being Active as We Get Older | Handout
- Being Active for a Better Life | Handout
- Being Active with Your Young Child | Handout
- Exercise Preparticipation Health Screening Process | Infographic
- Exercise Prescription Form | Form
- Monitoring Aerobic Exercise Intensity | Infographic
- PAR-Q+/ Physical Activity Readiness Questionnaire | Form
- Physical Activity Vital Sign | Form
- Resistance Training for Health | Infographic
- Sit Less, Move More | Handout
Chronic Disease and Special Populations
Exercise is Medicine ® offers many handouts on being active with a variety of medical conditions as a part of their Exercise Rx Series .
- Being Active When You Have Cancer | Handout
- Exercise for Cancer Prevention and Treatment | Infographic
- Moving Through Cancer, Exercise Prescription Form | Form
- Physical Activity Guidelines: Cancer | Infographic
Hypertension
- American Heart Association Updates Blood Pressure Guidelines | Blog
- Being Active with High Blood Pressure | Handout
- Exercise for the Prevention and Treatment of Hypertension - Implications and Application | Blog
- FITT Recommendations for Hypertension | Infographic
- What’s Changed: New High Blood Pressure Guidelines | Blog
- Being Active During Pregnancy | Handout
- Fit Pregnancy Guidelines, A Simple Guide | Blog
- Pregnancy and Physical Activity | Handout
- Pregnancy, Physical Activity Recommendations | Infographic
- ACSM's Guidelines for Exercise Testing and Prescription, 10th Edition
- ACSM's Health-Related Physical Fitness Assessment Manual
- ACSM’s Exercise Testing and Prescription
- ACSM’s Health/Fitness Facility Standards and Guidelines, Fifth Edition
Official Positions
ACSM is pleased to present the scientific reviews underlying the second edition of the Physical Activity Guidelines . Health professionals, scientists, community organizations and policymakers can use the papers included in the ACSM Scientific Pronouncements: Physical Activity Guidelines for Americans, 2nd Edition to promote more active, healthier lifestyles for individuals and communities. All papers were published in Medicine & Science in Sports & Exercise .
- The U.S. Physical Activity Guidelines Advisory Committee Report—Introduction
- Physical Activity Promotion: Highlights from the 2018 PAGAC Systematic Review
- Daily Step Counts for Measuring Physical Activity Exposure and Its Relation to Health
- Association between Bout Duration of Physical Activity and Health: Systematic Review
- High-Intensity Interval Training (HIIT) for Cardiometabolic Disease Prevention
- Sedentary Behavior and Health: Update from the 2018 Physical Activity Guidelines Advisory Committee
- Physical Activity, Cognition and Brain Outcomes: A Review of the 2018 Physical Activity Guidelines
- Physical Activity in Cancer Prevention and Survival: A Systematic Review
- Physical Activity and the Prevention of Weight Gain in Adults: A Systematic Review
- Physical Activity, All-Cause and Cardiovascular Mortality, and Cardiovascular Disease
- Physical Activity and Health in Children under 6 Years of Age: A Systematic Review
- Benefits of Physical Activity during Pregnancy and Postpartum: An Umbrella Review
- Physical Activity, Injurious Falls and Physical Function in Aging: An Umbrella Review
- Physical Activity to Prevent and Treat Hypertension: A Systematic Review
- Effects of Physical Activity in Knee and Hip Osteoarthritis: A Systematic Umbrella Review
Earlier Papers
- Quantity and Quality of Exercise for Developing and Maintaining Cardiorespiratory, Musculoskeletal, and Neuromotor Fitness in Apparently Healthy Adults: Guidance for Prescribing Exercise

Guidelines for Physical Activity and Health: Evolution Over 50 Years
Presented as the D.B. Dill Historical Lecture at the 2019 ACSM Annual Meeting, William Haskell, PhD, FACSM, and ACSM past president, presented a timeline of the developing science behind the Physical Activity Guidelines for Americans.
- 2024 Election Results
- News Archive
- Certification Task Force
- Advertise with ACSM
- Current Partners
- American Fitness Index
- National Youth Sports Health & Safety Institute
- Annual Report 2022
- Annual Report 2023
- Annual Report 2024
- Honor & Citation Awards
- Strategic Plan
- Student Membership
- Alliance Membership
- Professional-in-Training Membership
- Professional Membership
- Renew Membership
- Regional Chapters
- Member Code of Ethics
- ACSM Member Spotlight
- Group Exercise Instructor
- ACSM Personal Trainer Prep
- ACSM Exercise Physiologist Prep
- ACSM Clinical Exercise Physiologist Prep
- Beijing Institute of Sports Medicine
- Wellness Academy
- Frequently Asked Questions
- Recertification FAQs
- Find an ACSM Certified Professional
- Certified Professional of the Year
- Wellcoaches
- Certified Professional Discounts
- Hire ACSM Certified Professionals
- Specialty Certificate Programs
- ceOnline FAQs
- Approved Providers
- Current Sports Medicine Reports
- Exercise and Sport Sciences Reviews
- Exercise, Sport, and Movement
- Health & Fitness Journal
- Medicine & Science in Sports & Exercise
- Translational Journal
- Paper of the Year Awards
- ACSM's Guidelines for Exercise Testing and Prescription
- ACSM's Resources for the Personal Trainer
- ACSM's Resources for the Exercise Physiologist
- ACSM's Clinical Exercise Physiology
- ACSM's Resources for the Group Exercise Instructor
- ACSM's Certification Review
- ACSM's Foundations of Strength Training and Conditioning
- ACSM's Nutrition Exercise Science
- ACSM's Essentials of Youth Fitness
- ACSM's Introduction to Exercise Science
- ACSM's Health/Fitness Facility Standards and Guidelines
- ACSM’s Body Composition Assessment
- ACSM's Complete Guide to Fitness and Health
- Preparticipation Physical Evaluation (PPE) Monograph, 5th Edition
- ACSM's Fitness Assessment Manual
- ACSMs Exercise Testing and Prescription
- Textbook Adoption
- Translated Position Stands
- ACSM Official Statements
- Team Physician Consensus Statements
- Resource Library
- ACSM Fitness Trends
- Autism and Exercise
- Sudden Cardiac Arrest
- Mental Health
- Physical Activity Guidelines
- Reducing Sedentary Behavior
- Faculty Resources
- EIM Clinical Resources
- Black History Month
- ACSM's Brown Bag Series (archived)
- Sex Differences
- Emerging Physician Leaders Pilot Program
- Annual Meeting
- IDEA & ACSM Health & Fitness Summit
- Advanced Team Physician Course
- Sports Medicine Essentials
- Integrative Physiology of Exercise Conference
- International Team Physician Course
- Regional Chapter Meetings
- Meeting Exhibits and Sponsors
- Research & Program Grants
- Howard G. "Skip" Knuttgen Scholar Award
- Travel and Research Awards
- ACSM Research Grant Recipients
- Dedicated Endowments & Funds
- Planned Giving / Discovery Society
- Article Information
Shown are the 28-day risk period rates of the 28 included adverse events of special interest to COVID-19 vaccines following XBB.1.5-containing mRNA vaccine immunization as a fifth dose compared with reference period rates in Danish people aged 65 years and older from October 1, 2023, to January 8, 2024. The 28-day risk period outcome rates following fifth dose vaccination with an XBB.1.5-containing mRNA vaccine was compared with reference period rates from day 43 after the fourth or fifth dose and onward. Individuals could contribute with person-time during both the 28-day risk period and the 2 reference periods while the number of events and person-time from the 2 reference periods were aggregated. Each outcome was studied separately, which is why there may be slight differences in the denominators due to different exclusions. The arrows indicate that the 95% CI exceeds the upper or lower limits on the x-axis. IRR indicates incidence rate ratio; NE, not estimable; and TIA, transient ischemic attack.
eTable. Eligibility Criteria and Outcome and Covariates Definitions
eFigure. Schematic Figure of the Study Design
eReferences
Data Sharing Statement
See More About
Select your interests.
Customize your JAMA Network experience by selecting one or more topics from the list below.
- Academic Medicine
- Acid Base, Electrolytes, Fluids
- Allergy and Clinical Immunology
- American Indian or Alaska Natives
- Anesthesiology
- Anticoagulation
- Art and Images in Psychiatry
- Artificial Intelligence
- Assisted Reproduction
- Bleeding and Transfusion
- Caring for the Critically Ill Patient
- Challenges in Clinical Electrocardiography
- Climate and Health
- Climate Change
- Clinical Challenge
- Clinical Decision Support
- Clinical Implications of Basic Neuroscience
- Clinical Pharmacy and Pharmacology
- Complementary and Alternative Medicine
- Consensus Statements
- Coronavirus (COVID-19)
- Critical Care Medicine
- Cultural Competency
- Dental Medicine
- Dermatology
- Diabetes and Endocrinology
- Diagnostic Test Interpretation
- Drug Development
- Electronic Health Records
- Emergency Medicine
- End of Life, Hospice, Palliative Care
- Environmental Health
- Equity, Diversity, and Inclusion
- Facial Plastic Surgery
- Gastroenterology and Hepatology
- Genetics and Genomics
- Genomics and Precision Health
- Global Health
- Guide to Statistics and Methods
- Hair Disorders
- Health Care Delivery Models
- Health Care Economics, Insurance, Payment
- Health Care Quality
- Health Care Reform
- Health Care Safety
- Health Care Workforce
- Health Disparities
- Health Inequities
- Health Policy
- Health Systems Science
- History of Medicine
- Hypertension
- Images in Neurology
- Implementation Science
- Infectious Diseases
- Innovations in Health Care Delivery
- JAMA Infographic
- Law and Medicine
- Leading Change
- Less is More
- LGBTQIA Medicine
- Lifestyle Behaviors
- Medical Coding
- Medical Devices and Equipment
- Medical Education
- Medical Education and Training
- Medical Journals and Publishing
- Mobile Health and Telemedicine
- Narrative Medicine
- Neuroscience and Psychiatry
- Notable Notes
- Nutrition, Obesity, Exercise
- Obstetrics and Gynecology
- Occupational Health
- Ophthalmology
- Orthopedics
- Otolaryngology
- Pain Medicine
- Palliative Care
- Pathology and Laboratory Medicine
- Patient Care
- Patient Information
- Performance Improvement
- Performance Measures
- Perioperative Care and Consultation
- Pharmacoeconomics
- Pharmacoepidemiology
- Pharmacogenetics
- Pharmacy and Clinical Pharmacology
- Physical Medicine and Rehabilitation
- Physical Therapy
- Physician Leadership
- Population Health
- Primary Care
- Professional Well-being
- Professionalism
- Psychiatry and Behavioral Health
- Public Health
- Pulmonary Medicine
- Regulatory Agencies
- Reproductive Health
- Research, Methods, Statistics
- Resuscitation
- Rheumatology
- Risk Management
- Scientific Discovery and the Future of Medicine
- Shared Decision Making and Communication
- Sleep Medicine
- Sports Medicine
- Stem Cell Transplantation
- Substance Use and Addiction Medicine
- Surgical Innovation
- Surgical Pearls
- Teachable Moment
- Technology and Finance
- The Art of JAMA
- The Arts and Medicine
- The Rational Clinical Examination
- Tobacco and e-Cigarettes
- Translational Medicine
- Trauma and Injury
- Treatment Adherence
- Ultrasonography
- Users' Guide to the Medical Literature
- Vaccination
- Venous Thromboembolism
- Veterans Health
- Women's Health
- Workflow and Process
- Wound Care, Infection, Healing
Others Also Liked
- Download PDF
- X Facebook More LinkedIn
Andersson NW , Thiesson EM , Hviid A. Adverse Events After XBB.1.5-Containing COVID-19 mRNA Vaccines. JAMA. 2024;331(12):1057–1059. doi:10.1001/jama.2024.1036
Manage citations:
© 2024
- Permissions
Adverse Events After XBB.1.5-Containing COVID-19 mRNA Vaccines
- 1 Department of Epidemiology Research, Statens Serum Institut, Copenhagen, Denmark
The monovalent Omicron XBB.1.5–containing COVID-19 mRNA vaccines were authorized in the US and Europe for use in autumn and winter 2023-2024. 1 , 2 In Denmark, the XBB.1.5-containing vaccines were recommended as a fifth COVID-19 vaccine dose to individuals aged 65 years and older beginning October 1, 2023. However, data to support safety evaluations are lacking.
We investigated the association between the XBB.1.5-containing vaccine administered as a fifth COVID-19 vaccine dose and the risk of 28 adverse events.
A study cohort of all individuals in Denmark aged 65 and older who had received 4 COVID-19 vaccine doses was established by cross-linking nationwide health care and demography registers on an individual level. The study period was September 15, 2022 (ie, the national rollout date of the fourth dose), to January 8, 2024, and vaccination status was classified in a time-varying manner (the eTable in Supplement 1 provides further details). The 28 adverse events were adapted from prioritized lists of adverse events of special interest to COVID-19 vaccines (eTable in Supplement 1 ). 3 - 5 Each outcome was studied separately and identified as any first hospital contact where an outcome diagnosis was recorded. The diagnosis date served as the event date.
Individuals were followed up from day 43 after the fourth dose (days 29-42 considered a buffer period) until first outcome event while censoring upon emigration, death, receipt of a sixth vaccine dose (as such a dose was not rolled out to the general Danish population during the study period), or end of the study period (eFigure in Supplement 1 ). Outcome rates within the risk period of 28 days following XBB.1.5-containing vaccine administration as a fifth dose was compared with reference period rates from day 43 after a fourth or fifth dose and onward as previously described; the number of events and person-time from the 2 reference periods were aggregated. 5 Individuals could contribute person-time both during the 28-day risk period and the 2 reference periods; individuals not receiving the XBB.1.5-containing vaccine only contributed to reference period person-time. Using Poisson regression, the risk and reference period outcome rates were compared by incidence rate ratios, adjusted for sex, age, region of residence, considered at high risk of severe COVID-19, health care worker, calendar time, and number of comorbidities. Statistical tests were 2-sided and conducted in R (version 4.1.1; R Project for Statistical Computing). A 95% CI that did not cross 1 was defined as statistically significant. Analysis was performed as surveillance activities as part of the advisory tasks of the governmental institution Statens Serum Institut (SSI), which monitors the spread of disease in accordance with §222 of the Danish Health Act, for the Danish Ministry of Health. According to Danish law, national surveillance activities conducted by SSI do not require approval from an ethics committee.
Among the 1 076 531 included individuals (mean [SD] age, 74.7 [7.4] years; 53.8% female), 902 803 received an XBB.1.5-containing vaccine as a fifth dose during follow-up ( Table ).
Receipt of an XBB.1.5-containing vaccine was not associated with a statistically significant increased rate of hospital contacts for any of the 28 different adverse events within 28 days after vaccination compared with the reference period rates ( Figure ). For example, the incidence rate ratio was 0.96 (95% CI, 0.87-1.07) for an ischemic cardiac event, 0.87 (95% CI, 0.79-0.96) for a cerebral infarction, and 0.60 (95% CI, 0.14-2.66) for myocarditis. Some outcomes were very rare during follow-up (eg, cerebral venous thrombosis), resulting in lower statistical precision; however, for 18 of the 28 adverse events examined, the upper bound of the CI was inconsistent with moderate to large increases in relative risk of 1.4 or greater.
In a nationwide cohort of more than 1 million adults aged 65 years and older, no increased risk of 28 adverse events was observed following vaccination with a monovalent XBB.1.5-containing vaccine.
Limitations of this study include potential residual confounding; differences in ascertainment of adverse events between compared periods cannot be excluded, but, in contrast to what was observed, would bias toward increased risks if present. This was mitigated by comparing the 28-day risk period rates following a fifth dose vaccination with an XBB.1.5-containing vaccine with reference period rates from 43 days or more after the fourth and fifth vaccine dose as opposed to never vaccinated period rates. Additionally, analyses were not adjusted for multiple testing, and some results showed lower risk for XBB.1.5-containing vaccines; yet, a time-varying healthy vaccinee effect cannot be excluded. Also, no medical record review of cases was done, but any outcome misclassification would most likely be nondifferential.
Accepted for Publication: January 23, 2024.
Published Online: February 26, 2024. doi:10.1001/jama.2024.1036
Corresponding Author: Niklas Worm Andersson, MD, Department of Epidemiology Research, Statens Serum Institut, Artillerivej 5, Copenhagen S 2300, Denmark ( [email protected] ).
Author Contributions: Dr Andersson had full access to all of the data in the study and takes responsibility for the integrity of the data and the accuracy of the data analysis.
Concept and design: All authors.
Acquisition, analysis, or interpretation of data: All authors.
Drafting of the manuscript: Andersson.
Critical review of the manuscript for important intellectual content: All authors.
Statistical analysis: Andersson, Thiesson.
Supervision: Hviid.
Conflict of Interest Disclosures: Dr Hviid reported receiving grants from Lundbeck Foundation, Novo Nordisk Foundation, and Danish Medical Research Council and being a scientific advisory board member for VAC4EU. No other disclosures were reported.
Data Sharing Statement: See Supplement 2 .
- Register for email alerts with links to free full-text articles
- Access PDFs of free articles
- Manage your interests
- Save searches and receive search alerts

COMMENTS
Background The complexity of public health interventions create challenges in evaluating their effectiveness. There have been huge advancements in quantitative evidence synthesis methods development (including meta-analysis) for dealing with heterogeneity of intervention effects, inappropriate 'lumping' of interventions, adjusting for different populations and outcomes and the inclusion of ...
methodology that can adequately respond to the study's research question or objective. This initial step will be based on the theoretical orientation, i.e. on the selected research paradigm, from which the methods and techniques for analysing the data collected during the study of a given phenomenon will be defined.
Introduction. The Accreditation Council for Graduate Medical Education requires that internal medicine (IM) residency programs provide a didactic curriculum based on the core knowledge content. 1 The ability of this curriculum to motivate independent study among residents is not known. 2 - 9 A study in continuing medical education found that lecture effectiveness was correlated with the ...
faction using a Likert-type scale (1 = very unsatis ed, 2 =. unsatis ed, 3 = neutral, 4 = satis ed, 5 = very satis ed). total of 20 medical students evaluate the curriculum, 10 of. whom rate their ...
Background Identifying determinants of health and understanding their role in health production constitutes an important research theme. We aimed to document the state of recent multi-country research on this theme in the literature. Methods We followed the PRISMA-ScR guidelines to systematically identify, triage and review literature (January 2013—July 2019). We searched for studies that ...
The rise of quantitative medicine. Quantitative medicine is a paradigm shift in the practice of medicine that emphasizes the use of quantitative data and mathematical models to understand and treat disease. 20 This approach is based on the idea that the human body can be studied as a complex system, with many interconnected parts that can be modeled and simulated using mathematical and ...
All subjects Allied Health Cardiology & Cardiovascular Medicine Dentistry Emergency Medicine & Critical Care Endocrinology & Metabolism Environmental Science General Medicine Geriatrics Infectious ... Quantitative Research Excellence: Study Design and Reliable and Valid Measurement of Variables ... PDF/ePub View PDF/ePub Full Text View Full ...
Experimental research is a quantitative research method that deals specically with numbers. Specically, an experiment is designed to investigate if a causal relation-ship exists between two variables (Gray et al., 2017; Price et al., 2015). These vari-ables are the independent variable and the dependent variable. The denitions of
Public and patient involvement (PPI) in health research has been defined as research being carried out "with" or "by" members of the public rather than "to," "about" or "for" them. 1 PPI covers a diverse range of approaches from "one off" information gathering to sustained partnerships. Tritter's conceptual framework for ...
Chronic diseases have an impact on and change patients' lives, and the way they experience their bodies alters. Patients may struggle with identity and self-esteem, a shrinking lifeworld and a challenging reality. 1 The chronic diseases become part of the patients' lives, whether they affect their physical health and functions, autonomy, freedom and identity, or threaten their life. 2 The ...
It provides some guidance for those trying to better understand the variety of quantitative methods available. The chapter distinguishes four research traditions - experimental, epidemiological, psychometric, and correlational - exploring some basic principles of measurement and statistical inference along the way.
Clarifying the research purpose is an essential first step when reading or conducting scholarship in medical education. 1 Medical education research can serve a variety of purposes, from advancing the science of learning to improving the outcomes of medical trainees and the patients they care for. However, a well-designed study has limited ...
Most students (74.7%) agreed the pandemic had significantly disrupted their medical education, and believed they should continue with normal clinical rotations during this pandemic (61.3%). When asked if they would accept the risk of infection with COVID-19 if they returned to the clinical setting, 83.4% agreed.
Research articles of translational medicine (n=1662) Non-research articles (n=1499) Research articles within non-medical fields (n=103) Figure 2. The flow chart of the quantitative evaluation of translational medicine. Research articles on translational medicine from 2011 to 2013 Descriptive finding (the number of articles and citations ...
Background As a community of practice (CoP), medical education depends on its research literature to communicate new knowledge, examine alternative perspectives, and share methodological innovations. As a key route of communication, the medical education CoP must be concerned about the rigor and validity of its research literature, but prior studies have suggested the need to improve medical ...
Mixed-methods research is a flexible approach, where the research design is determined by what we want to find out rather than by any predetermined epistemological position. In mixed-methods research, qualitative or quantitative components can predominate, or both can have equal status. 1.4. Units and variables.
Description. Quantitative Research in Human Biology and Medicine reflects the author's past activities and experiences in the field of medical statistics. The book presents statistical material from a variety of medical fields. The text contains chapters that deal with different aspects of vital statistics. It provides statistical surveys of ...
INTRODUCTION. Scientific research is usually initiated by posing evidenced-based research questions which are then explicitly restated as hypotheses.1,2 The hypotheses provide directions to guide the study, solutions, explanations, and expected results.3,4 Both research questions and hypotheses are essentially formulated based on conventional theories and real-world processes, which allow the ...
quantitative proteomics in DMPK research and precision medicine. The proceedings of this . workshop were publish. ed in a. white. paper, summarizing the consensus on methodology and . application. s. of quantitative proteomics in translational pharmacology and precision medicine (Prasad . et al., 2019). Five years after the publication of the ...
The chapter describes the quantitative research methods of meta‐analysis and systematic reviews. It contrasts these strategies with those of reviews that are better defined as critical and theory‐oriented. The chapter examines various issues related to selecting a particular research design.
2.0 Quantitative Research. Quantitative research is regarded as the organized inquiry about phenomenon through collection. of numer ical data and execution of statistical, mathematical or ...
Introduction. It has been well established that health care spending varies with geography. 1-3 The source of this variation has been often questioned—whether it is arising from area practice patterns, patient health status, patient characteristics, price, and/or individual provider decision making. 3,4 An Institute of Medicine (IoM) Committee examining geographic variations in Medicare ...
Over 20 years ago, Thomas Best and Domhnall MacAuley rhetorically posited that evidence-based sports medicine was potentially a 'contradiction in terms'.1 In 2010, Evert Verhagen and Willem van Mechelen stated that 'most individuals involved in sports medicine are not thoroughly trained in epidemiological and methodological rigour'.2 Despite these somewhat disparaging views, research ...
The Department of Tropical Medicine, Medical Microbiology, and Pharmacology offers graduate programs leading to the MS and PhD in Biomedical Sciences (Tropical Medicine). Faculty conduct extensive research on pathogenic microorganisms and the diseases they cause using laboratory-based, field-based and clinic-based techniques.
The majority were quantitative studies (77.4%), using retrospective designs. 11.3% were qualitative. ... As such, research conducted in other medical domains is not automatically applicable to surgery, and behavioural interventions focused on reducing or eliminating bias in training need to be tailored specifically to surgical settings ...
All healthy adults aged 18-65 years should participate in moderate intensity aerobic physical activity for a minimum of 30 minutes on five days per week, or vigorous intensity aerobic activity for a minimum of 20 minutes on three days per week. Every adult should perform activities that maintain or increase muscular strength and endurance for ...
Summary This chapter contains sections titled: The Quantitative Paradigm The Research Question Research Designs The Experimental Tradition The Epidemiologic Tradition The Psychometric Tradition The...
The monovalent Omicron XBB.1.5-containing COVID-19 mRNA vaccines were authorized in the US and Europe for use in autumn and winter 2023-2024. 1,2 In Denmark, the XBB.1.5-containing vaccines were recommended as a fifth COVID-19 vaccine dose to individuals aged 65 years and older beginning October 1, 2023. However, data to support safety evaluations are lacking.