- History, Facts & Figures
- YSM Dean & Deputy Deans
- YSM Administration
- Department Chairs
- YSM Executive Group
- YSM Board of Permanent Officers
- FAC Documents
- Current FAC Members
- Appointments & Promotions Committees
- Ad Hoc Committees and Working Groups
- Chair Searches
- Leadership Searches
- Organization Charts
- Faculty Demographic Data
- Professionalism Reporting Data
- 2022 Diversity Engagement Survey
- State of the School Archive
- Faculty Climate Survey: YSM Results
- Strategic Planning
- Mission Statement & Process
- Beyond Sterling Hall
- COVID-19 Series Workshops
- Previous Workshops
- Departments & Centers
- Find People
- Biomedical Data Science
- Health Equity
- Inflammation
- Neuroscience
- Global Health
- Diabetes and Metabolism
- Policies & Procedures
- Media Relations
- A to Z YSM Lab Websites
- A-Z Faculty List
- A-Z Staff List
- A to Z Abbreviations
- Dept. Diversity Vice Chairs & Champions
- Dean’s Advisory Council on Lesbian, Gay, Bisexual, Transgender, Queer and Intersex Affairs Website
- Minority Organization for Retention and Expansion Website
- Office for Women in Medicine and Science
- Committee on the Status of Women in Medicine Website
- Director of Scientist Diversity and Inclusion
- Diversity Supplements
- Frequently Asked Questions
- Recruitment
- By Department & Program
- News & Events
- Executive Committee
- Aperture: Women in Medicine
- Self-Reflection
- Portraits of Strength
- Mindful: Mental Health Through Art
- Event Photo Galleries
- Additional Support
- MD-PhD Program
- PA Online Program
- Joint MD Programs
- How to Apply
- Advanced Health Sciences Research
- Clinical Informatics & Data Science
- Clinical Investigation
- Medical Education
- Visiting Student Programs
- Special Programs & Student Opportunities
- Residency & Fellowship Programs
- Center for Med Ed
- Organizational Chart
- Leadership & Staff
- Committee Procedural Info (Login Required)
- Faculty Affairs Department Teams
- Recent Appointments & Promotions
- Academic Clinician Track
- Clinician Educator-Scholar Track
- Clinican-Scientist Track
- Investigator Track
- Traditional Track
- Research Ranks
- Instructor/Lecturer
- Social Work Ranks
- Voluntary Ranks
- Adjunct Ranks
- Other Appt Types
- Appointments
- Reappointments
- Transfer of Track
- Term Extensions
- Timeline for A&P Processes
- Interfolio Faculty Search
- Interfolio A&P Processes
- Yale CV Part 1 (CV1)
- Yale CV Part 2 (CV2)
- Samples of Scholarship
- Teaching Evaluations
- Letters of Evaluation
- Dept A&P Narrative
- A&P Voting
- Faculty Affairs Staff Pages
- OAPD Faculty Workshops
- Leadership & Development Seminars
- List of Faculty Mentors
- Incoming Faculty Orientation
- Faculty Onboarding
- Past YSM Award Recipients
- Past PA Award Recipients
- Past YM Award Recipients
- International Award Recipients
- Nominations Calendar
- OAPD Newsletter
- Fostering a Shared Vision of Professionalism
- Academic Integrity
- Addressing Professionalism Concerns
- Consultation Support for Chairs & Section Chiefs
- Policies & Codes of Conduct
- First Fridays
- Fund for Physician-Scientist Mentorship
- Grant Library
- Grant Writing Course
- Mock Study Section
- Research Paper Writing
- Establishing a Thriving Research Program
- Funding Opportunities
- Join Our Voluntary Faculty
- Child Mental Health: Fostering Wellness in Children
- Faculty Resources
- Research by Keyword
- Research by Department
- Research by Global Location
- Translational Research
- Research Cores & Services
- Program for the Promotion of Interdisciplinary Team Science (POINTS)
- CEnR Steering Committee
- Experiential Learning Subcommittee
- Goals & Objectives
- Faculty & Staff
- Issues List
- Print Magazine PDFs
- Print Newsletter PDFs
- YSM Events Newsletter
- Social Media
- Patient Care
INFORMATION FOR
- Residents & Fellows
- Researchers

Reimagining Medical Education: Using Technology to Educate, Innovate, and Captivate
Reimagining medical education.
Technology is changing many aspects of life and work, as well as the way Yale School of Medicine (YSM) students learn.
“For alumni and students, you know that the Yale System is the core of medical education at Yale. But today we have creative and novel technologies that enable that system in new ways,” said Nancy J. Brown, MD, Jean and David W. Wallace Dean and C.N.H. Long Professor of Internal Medicine, as she welcomed YSM alumni, students, faculty, and staff to the inaugural webinar, Reimagining Medical Education: Using Technology to Educate, Innovate, and Captivate , in the school’s Innovation in Medical Education series.
The four-part series that began on November 4 is designed to showcase “how that innovation enhances our already unique education,” Brown explained. The next session, on January 26, will focus on how simulation is used in medical education at YSM, followed by discussions of the adoption of telehealth in YSM’s clinical skills program and how innovation is being incorporated into the school’s pharmacology training.
The theme of the series aligns with one of the medical education priorities of Jessica Illuzzi, MD, MS, deputy dean for education and Harold W. Jockers Professor of Medical Education—to develop innovative approaches to medical education that are irrevocably engaging and compelling, enhanced by increased use of simulation and evolving technologies and resources. In her remarks, Illuzzi referenced how medicine looks a lot different than it did even a decade ago and will continue to evolve along with technology. In addition, she stated, “the learning style of our students has evolved as they become ever more technologically-savvy."
The panel’s moderator, Associate Dean for Curriculum Michael Schwartz, PhD, was appointed the inaugural director for innovation in medical education in July 2021, demonstrating the school’s commitment to enhancing medical education through technology. Schwartz shared the history of how YSM has been using technology to enhance student learning through devices like iPads and iPad Minis since 2010.
One theme of Schwartz’s remarks was the important role of students in decision-making about what technology to incorporate, since students know what will be useful, versus leadership and faculty trying to anticipate what might be useful. He noted the importance of ensuring students with different levels of tech-savviness pilot technology, since it has to work well for all users.
When a participant asked how faculty stay ahead of changing technology, Schwartz again pointed to students, explaining they “become technology educators for our faculty.” When students first had iPad Minis on the wards in 2013, during rounding, residents and attendings often turned to students to quickly access patient data in EPIC and other online information. This helped to integrate students into teams and allowed for bi-directional teaching and learning. As mobile devices have become more common in clinical spaces, residents and attendings are increasingly modeling their use in patient care.
Schwartz described how use of iPad Minis on the wards also improves interactions with patients and shared how during COVID-19, when telehealth was being used extensively, the iPad Minis importantly gave students access to electronic medical records on and off-site.
The learning style of our students has evolved as they become ever more technologically-savvy. Jessica Illuzzi, MD, MS, deputy dean for education and Harold W. Jockers Professor of Medical Education
In 2010, when YSM first introduced iPads in the pre-clerkship curriculum, it was one of three medical schools to use the technology. By fall 2011, the entire curriculum was delivered through iPads, allowing students to receive faculty members’ most current lecture notes and presentations and to annotate these course materials.
Four faculty panelists shared how they have been using technology to enhance their teaching. William Stewart, PhD, associate professor of surgery (gross anatomy), described how the anatomy lab now has 40 dissection tables with networked iMacs on an adjustable arm. Additionally, he and a team have created 24 interactive iBooks for use in anatomy that are also available to other institutions through the Apple bookstore.
Associate Professor of Emergency Medicine Rachel Liu, BAO, MBBCh, who is the director of point-of-care ultrasound (POCUS) education, noted that since 2015, POCUS transducers paired with iPads and other tablet devices have been available in all YSM educational physical exam practice rooms. Each year, Liu explained, 16 students are trained in the use of these devices and how to teach their peers. This has enabled students to practice POCUS on their own and enhance their ability to learn normal anatomy, physiology, and pathology. Using POCUS, a student will “see the spleen for the first time in their medical career,” Liu stated.
Technology altered how clinical skills were taught during the pandemic, changes that according to Jaideep Talwalkar, MD, associate professor of internal medicine (general medicine) and pediatrics and director of clinical skills, will have staying power because they enhanced learning. Pre-pandemic, small groups of students practiced clinical skills with each other in the clinical skills practice rooms, with faculty providing feedback. COVID restrictions forced creative adaptation. Students practiced on roommates, family members, or even mannequins, using mounted iPads at home if they did not have access to the school’s cameras, with faculty observing remotely providing formative feedback. Students engaged with standardized patients on iPads as they practiced the actual physical exam on a mannequin. Faculty brought iPads to hospital rooms so that students, who could not be physically present, could talk with patients and their team members and participate in care discussions remotely.
The portability and versatility of these tech-driven solutions improved clinical skills training because students were more engaged, there was increased access to faculty and patients, and more opportunities for physical exam practice and feedback.
Deliberate practice—premised on Dr. Anders Ericsson’s concept that people get better at a skill when they practice toward a discrete goal, receive immediate feedback, and have the chance to practice again—led Christine J. Ko, MD, professor of dermatology and pathology, to develop an app that trains people to visually recognize skin cancer. People look at images of skin lesions in a range of skin types and are asked to identify which lesions are skin cancer. Ko has visions of the app expanding to other diseases, specialties, and physical examination skills. Schwartz hopes that in his new role as director for innovation in medical education he will be able to encourage and support more new applications of technology to enhance learning.
Featured in this article
- Nancy J. Brown, MD Jean and David W. Wallace Dean of the Yale School of Medicine and C.N.H. Long Professor of Internal Medicine
- Jessica Illuzzi, MD, MS, FACOG Deputy Dean for Education and Harold W. Jockers Professor of Medical Education and Professor of Obstetrics, Gynecology and Reproductive Sciences
- Christine Ko, MD Professor of Dermatology and Pathology
- Rachel Liu, BAO, MBBCh, FACEP, FAIUM Associate Professor of Emergency Medicine; Director, Point of Care Ultrasound Education, Office of Curriculum; Fellowship Director of Emergency Ultrasound, Emergency Medicine; Faculty Associate, Point of Care Ultrasound Education, Center for Medical Education; Director, Advanced Training Period , Office of Curriculum; Associate Director of the Emergency Ultrasound Section, Emergency Medicine
- Michael Schwartz, PhD Associate Dean for Curriculum; Director of Innovation in Medical Education, MD Program; Emeritus Associate Professor, Neuroscience; Senior Research Scientist, Neuroscience; Director, Medical Studies, Neuroscience
- William Stewart, PhD Associate Professor of Surgery (Gross Anatomy); Section Chief
- Jaideep S. Talwalkar, MD Associate Professor of Internal Medicine (General Medicine); Assistant Dean for Education, Medical Education; Associate Program Director, Yale Combined Med-Peds Residency Program; Director of Clinical Skills, Office of Education; Associate Professor, Pediatrics; Editor, Yale Primary Care Pediatrics Curriculum, Pediatrics
Related Links
- Video: Yale School of Medicine: "Reimagining Medical Education"
- DOI: 10.15694/mep.2020.000137.1
- Corpus ID: 225666116
The use of technology in healthcare education: a literature review
- Tom Grimwood , L. Snell
- Published 25 June 2020
- Education, Medicine, Computer Science
- MedEdPublish
Ask This Paper
By using this feature, you agree to AI2's terms and conditions and that you will not submit any sensitive or confidential info.
AI2 may include your prompts and inputs in a public dataset for future AI research and development. Please check the box to opt-out.
Ask a question about " "
Supporting statements, figures from this paper.
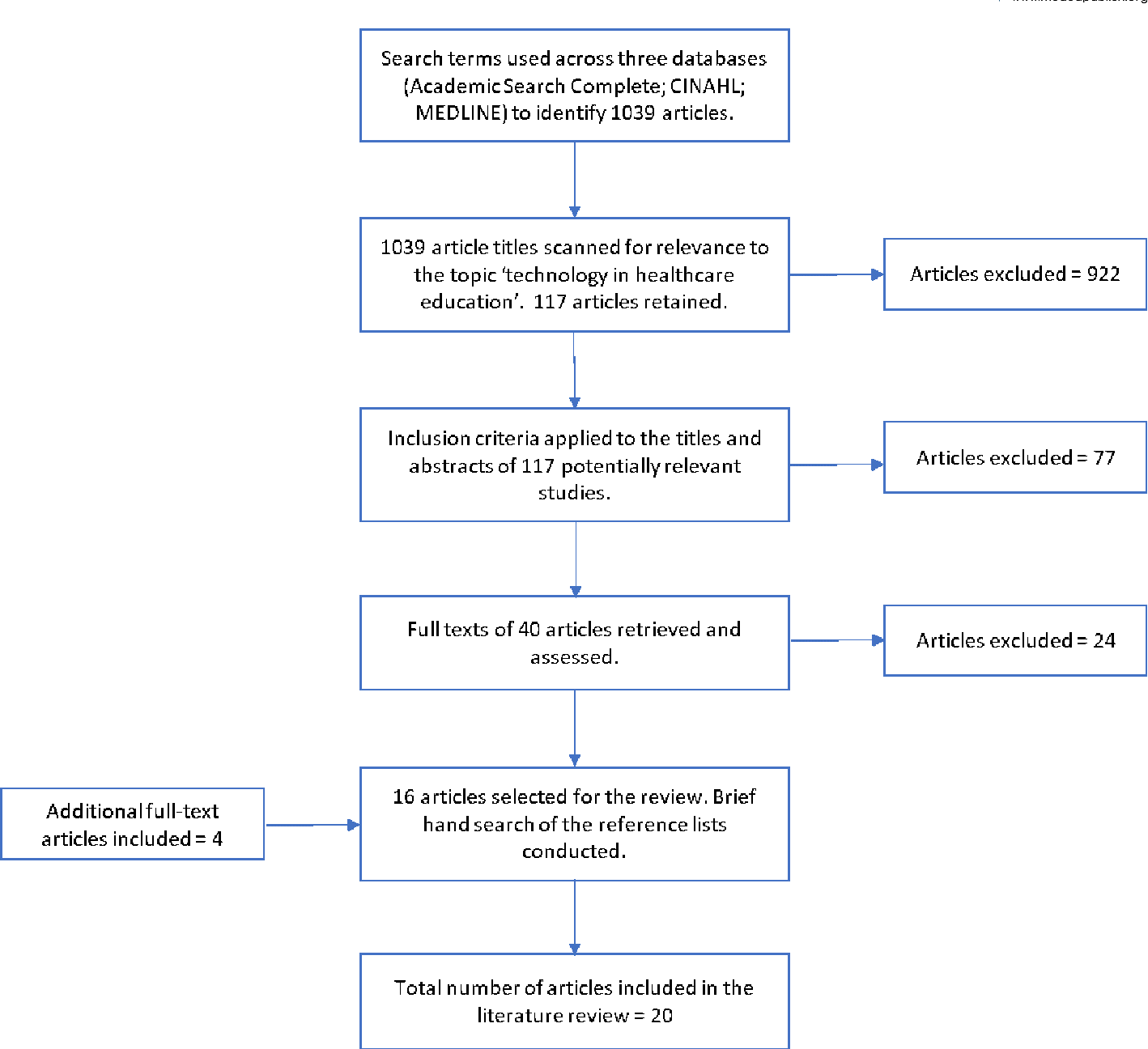
10 Citations
Proposing a framework guide for the integration of educational technologies and innovations into the teaching of anatomy and medical sciences: the asic framework, competencies and needs of nurse educators and clinical mentors for teaching in the digital age – a multi-institutional, cross-sectional study, technology skills in medical education during corona pandemic: special case study, a systematic review of digital innovations in technology-enhanced learning designs in higher education, efficacy of google form–based mcq tests for formative assessment in medical biochemistry education, association between clinical simulation design features and novice healthcare professionals’ cognitive load: a systematic review and meta-analysis, midwifery continuity of care in indonesia: initiation of mobile health development integrating midwives’ competency and service needs, the reality of e-learning in iraqi universities, amep_a_338262 1277..1282, considering and understanding developmental and deployment barriers for wearable technologies in neurosciences, 25 references, the role of blended learning in the clinical education of healthcare students: a systematic review, use of simulation in teaching and learning in health sciences: a systematic review..
- Highly Influential
The impact of clickers in nursing education: a review of literature.
Simulation education approaches to enhance collaborative healthcare: a best practices review, evaluation of technology-enhanced learning programs for health care professionals: systematic review, interprofessional online learning for primary healthcare: findings from a scoping review, technology in postgraduate medical education: a dynamic influence on learning, critical success factors for implementing healthcare e-learning, mobile technologies in medical education: amee guide no. 105.
- 13 Excerpts
Simulation in healthcare education: A best evidence practical guide. AMEE Guide No. 82
- 10 Excerpts
Related Papers
Showing 1 through 3 of 0 Related Papers
Technology Considerations in Health Professions and Clinical Education
- Living reference work entry
- First Online: 05 February 2020
- Cite this living reference work entry
- Christian Moro 5 ,
- Zane Stromberga 5 &
- James Birt 6
402 Accesses
14 Citations
105 Altmetric
Learning methods and pedagogy are shifting in clinical education. In particular, incorporating technology is increasingly important for training health professionals, where the necessary knowledge acquisition is typically much more experiential, self-directed, and hands-on than many other disciplines. Virtual or mixed reality interventions are becoming more accessible and can be delivered through various modes in a way that integrates directly into learning environments. However, there are often limits to the capacity of the technology, leaving educators unclear of which technologies are useful, and how they can be integrated into the learning environment. This chapter provides an overview of virtual and augmented reality; holograms and mixed reality; virtual dissection tables; social media; mobile applications and devices; 3D printing; online hosted video; simulation with technology enhanced learning; serious games; e-learning; and audience response software. We provide insight into the use of technology in health professions and clinical education by defining the types of technologies and exploring published use-cases across disciplines.
This is a preview of subscription content, log in via an institution to check access.
Access this chapter
Institutional subscriptions
Similar content being viewed by others
Immersive Technologies in ECMO Simulation
Virtual Reality, Haptic Simulators, and Virtual Environments
Effective Extended Reality: A Mixed-Reality Simulation Demonstration with Digitized and Holographic Tools and Intelligent Avatars
Abraham J, Wade DM, O’connell KA, Desharnais S, Jacoby R. The use of simulation training in teaching health care quality and safety: an annotated bibliography. Am J Med Qual. 2011;26:229–38.
Article Google Scholar
Adams SA. Blog-based applications and health information: two case studies that illustrate important questions for Consumer Health Informatics (CHI) research. Int J Med Inform. 2010;79:e89–96.
Ali AA, Miller ET. Effectiveness of video-assisted debriefing in health education: an integrative review. J Nurs Educ. 2018;57:14–20.
Anand M, Singel T. A comparative study of learning with “anatomage” virtual dissection table versus traditional dissection method in neuroanatomy. Indian J Clin Anat Physiol. 2017;4:177–80.
Google Scholar
Atlantis E, Cheema BS. Effect of audience response system technology on learning outcomes in health students and professionals: an updated systematic review. Int J Evid Based Healthc. 2015;13:3–8.
Azer SA, Eizenberg N. Do we need dissection in an integrated problem-based learning medical course? Perceptions of first- and second-year students. Surg Radiol Anat. 2007;29:173–80.
Birt J, Moore E, Cowling M. Improving paramedic distance education through mobile mixed reality simulation. Australas J Educ Technol. 2017;33:69.
Birt J, Stromberga Z, Cowling M, Moro C. Mobile mixed reality for experiential learning and simulation in medical and health sciences education. Information. 2018;9:31.
Boyle EA, Hainey T, Connolly TM, Gray G, Earp J, Ott M, Lim T, Ninaus M, Ribeiro C, Pereira J. An update to the systematic literature review of empirical evidence of the impacts and outcomes of computer games and serious games. Comput Educ. 2016;94:178–92.
Briz-Ponce L, García-Peñalvo FJ. An empirical assessment of a technology acceptance model for apps in medical education. J Med Syst. 2015;39:176.
Cai B, Rajendran K, Bay BH, Lee J, Yen CC. The effects of a functional three-dimensional (3D) printed knee joint simulator in improving anatomical spatial knowledge. Anat Sci Educ. 2018;12:610–18.
Cheston CC, Flickinger TE, Chisolm MS. Social media use in medical education: a systematic review. Acad Med. 2013;88:893–901.
Chugh R, Ruhi U. Social media in higher education: a literature review of Facebook. Educ Inf Technol. 2018;23:605–16.
Cook DA, Erwin PJ, Triola MM. Computerized virtual patients in health professions education: a systematic review and meta-analysis. Acad Med. 2010a;85:1589–602.
Cook DA, Garside S, Levinson AJ, Dupras DM, Montori VM. What do we mean by web-based learning? A systematic review of the variability of interventions. Med Educ. 2010b;44:765–74.
Cook DA, Hatala R, Brydges R, Zendejas B, Szostek JH, Wang AT, Erwin PJ, Hamstra SJ. Technology-enhanced simulation for health professions education: A systematic review and meta-analysis. JAMA. 2011;306:978–88.
Cowling M, Moro C. Holographic teachers were supposed to be part of our future. What happened? The Conversation. 2019. https://theconversation.com/holographic-teachers-were-supposed-to-be-part-of-our-future-what-happened-108500 .
Custer T, Michael K. The utilization of the anatomage virtual dissection table in the education of imaging science students. J Artic Radiat Sci Technol Educ. 2015;1:1–5.
Delello JA, Mcwhorter RR, Camp KM. Integrating augmented reality in higher education: a multidisciplinary study of student perceptions. J Educ Multimed Hypermed. 2015;24:209–33.
Dong C, Goh PS. Twelve tips for the effective use of videos in medical education. Med Teach. 2015;37:140–5.
Duncan I, Yarwood-Ross L, HAIGH C. YouTube as a source of clinical skills education. Nurse Educ Today. 2013;33:1576–80.
Gentry SV, Gauthier A, L’estrade Ehrstrom B, Wortley D, Lilienthal A, Tudor Car L, Dauwels-Okutsu S, Nikolaou CK, Zary N, Campbell J, Car J. Serious gaming and gamification education in health professions: systematic review. J Med Internet Res. 2019;21:e12994.
George PP, Papachristou N, Belisario JM, Wang W, Wark PA, Cotic Z, Rasmussen K, Sluiter R, Riboli-Sasco E, Tudor Car L, Musulanov EM, Molina JA, Heng BH, Zhang Y, Wheeler EL, AL Shorbaji N, Majeed A, Car J. Online eLearning for undergraduates in health professions: a systematic review of the impact on knowledge, skills, attitudes and satisfaction. J Glob Health. 2014;4:010406.
Girard C, Ecalle J, Magnan A. Serious games as new educational tools: how effective are they? A meta-analysis of recent studies. J Comput Assist Learn. 2013;29:207–19.
Gorbanev I, Agudelo-Londoño S, González RA, Cortes A, Pomares A, Delgadillo V, Yepes FJ, Muñoz Ó. A systematic review of serious games in medical education: quality of evidence and pedagogical strategy. Med Educ Online. 2018;23:1438718.
Gough S. Educating for professional practice through simulation. In: Delany C, Molloy E, editors. Learning and teaching in clinical contexts: a practical guide. Chatswood: Elsevier; 2018.
Gough S, Yohannes AM, Thomas C, Sixsmith J. Simulation-based education (SBE) within postgraduate emergency on-call physiotherapy in the United Kingdom. Nurse Educ Today. 2013;33:778–84.
Gough S, Yohannes AM, Murray J. Using video-reflexive ethnography and simulation-based education to explore patient management and error recognition by pre-registration physiotherapists. Adv Simul (Lond). 2016;1:9.
Hanson C, West J, Neiger B, Thackeray R, Barnes M, Mcintyre E. Use and acceptance of social media among health educators. Am J Health Educ. 2011;42:197–204.
Hosseini F, Oberoi V, Doroudi M, Vo L. A visual guide to foregut anatomy: using digital multimedia to enhance the learning of human gross anatomy. FASEB J. 2018;32:635.27.
Hunsu NJ, Adesope O, Bayly DJ. A meta-analysis of the effects of audience response systems (clicker-based technologies) on cognition and affect. Comput Educ. 2016;94:102–19.
Jayakumar N, Brunckhorst O, Dasgupta P, Khan MS, Ahmed K. e-Learning in surgical education: a systematic review. J Surg Educ. 2015;72:1145–57.
Jenson CE, Forsyth DM. Virtual reality simulation: using three-dimensional technology to teach nursing students. Comput Inform Nurs. 2012;30:312–8; quiz 319–20.
Jin W, Birckhead B, Perez B, Hoffe S. Augmented and virtual reality: exploring a future role in radiation oncology education and training. Appl Rad Oncol. 2017;6:13–20.
Jones DB, Sung R, Weinberg C, Korelitz T, Andrews R. Three-dimensional modeling may improve surgical education and clinical practice. Surg Innov. 2016;23:189–95.
Kilmon CA, Brown L, Ghosh S, Mikitiuk A. Immersive virtual reality simulations in nursing education. Nurs Educ Perspect. 2010;31:314–7.
Kim JH, Park H. Effects of smartphone-based mobile learning in nursing education: a systematic review and meta-analysis. Asian Nurs Res. 2019;13:20–9.
Klímová B. Mobile learning in medical education. J Med Syst. 2018;42:194.
Knight JF, Carley S, Tregunna B, Jarvis S, Smithies R, De Freitas S, Dunwell I, Mackway-Jones K. Serious gaming technology in major incident triage training: a pragmatic controlled trial. Resuscitation. 2010;81:1175–9.
Kuehn BM. Virtual and augmented reality put a twist on medical education. JAMA. 2018;319:756–8.
Lall P, Rees R, Law GCY, Dunleavy G, Cotič Ž, Car J. Influences on the implementation of mobile learning for medical and nursing education: qualitative systematic review by the Digital Health Education Collaboration. J Med Internet Res. 2019;21:e12895.
Lim KHA, Loo ZY, Goldie SJ, Adams JW, Mcmenamin PG. Use of 3D printed models in medical education: a randomized control trial comparing 3D prints versus cadaveric materials for learning external cardiac anatomy. Anat Sci Educ. 2016;9:213–21.
Liu C, Chen X, Liu S, Zhang X, Ding S, Long Y, Zhou D. The exploration on interacting teaching mode of augmented reality based on holoLens. In: Cheung SKS, Jiao J, Lee L-K, Zhang X, Li KC, Zhan Z, editors. Technology in education: pedagogical innovations. Singapore: Springer; 2019. p. 91–102.
Chapter Google Scholar
Maertens H, Madani A, Landry T, Vermassen F, Van Herzeele I, Aggarwal R. Systematic review of e-learning for surgical training. Br J Surg. 2016;103:1428–37.
Malik HH, Darwood ARJ, Shaunak S, Kulatilake P, El-Hilly AA, Mulki O, Baskaradas A. Three-dimensional printing in surgery: a review of current surgical applications. J Surg Res. 2015;199:512–22.
Mathiowetz V, Yu CH, Quake-Rapp C. Comparison of a gross anatomy laboratory to online anatomy software for teaching anatomy. Anat Sci Educ. 2016;9:52–9.
Mccallum S. Gamification and serious games for personalized health. Stud Health Technol Inform. 2012;177:85–96.
Mcgaghie WC, Issenberg SB, Petrusa ER, Scalese RJ. A critical review of simulation-based medical education research: 2003–2009. Med Educ. 2010;44:50–63.
Mcgaghie WC, Issenberg SB, Petrusa ER, Scalese RJ. Revisiting ‘A critical review of simulation-based medical education research: 2003–2009’. Med Educ. 2016;50:986–91.
Miller LM, Chang C-I, Wang S, Beier ME, Klisch Y. Learning and motivational impacts of a multimedia science game. Comput Educ. 2011;57:1425–33.
Moorhead SA, Hazlett DE, Harrison L, Carroll JK, Irwin A, Hoving C. A new dimension of health care: systematic review of the uses, benefits, and limitations of social media for health communication. J Med Internet Res. 2013;15:e85.
Moro C, Gregory S. Utilising anatomical and physiological visualisations to enhance the face-to-face student learning experience in biomedical sciences and medicine. Adv Exp Med Biol. 2019;1156:41–8.
Moro C, Hensen D. Engaging timid students: backchannel as a tool to provide opportunities for interactivity and engagement within the university classroom. Educ Technol Solut. 2017;81:38–41.
Moro C, Kinash S. Developing online worksheets that work. Educ Technol Solut. 2012;52:52–54.
Moro C, Mclean M. Supporting students’ transition to university and problem-based learning. Med Sci Educ. 2017;27:353–61.
Moro C, Periya S. Applied learning of anatomy and physiology: virtual dissection tables within medical and health sciences education. Bangkok Med J. 2019;15:121–7.
Moro C, Stromberga Z, Raikos A, Stirling A. The effectiveness of virtual and augmented reality in health sciences and medical anatomy. Anat Sci Educ. 2017a;10:549–59.
Moro C, Stromberga Z, Stirling A. Virtualisation devices for student learning: comparison between desktop-based (Oculus Rift) and mobile-based (Gear VR) virtual reality in medical and health science education. Australas J Educ Technol. 2017b;33:1.
Moro C, Spooner A, Mclean M. How prepared are students for the various transitions in their medical studies? An Australian university pilot study. MedEdPublish. 2019;8:25.
Narnaware Y, Neumeier M. Second-year nursing students’ retention of gross anatomical knowledge. Anat Sci Educ. 2019. https://doi.org/10.1002/ase.1906 .
Nelson C, Hartling L, Campbell S, Oswald AE. The effects of audience response systems on learning outcomes in health professions education. A BEME systematic review: BEME guide No. 21. Med Teach. 2012;34:e386–405.
O’connor S, Andrews T. Mobile technology and its use in clinical nursing education: a literature review. J Nurs Educ. 2015;54:137–44.
O’connor S, Jolliffe S, Stanmore E, Renwick L, Booth R. Social media in nursing and midwifery education: a mixed study systematic review. J Adv Nurs. 2018;74:2273–89.
Owen H. Simulation in healthcare education. An extensive history. Obstet Gynaecol. 2016;18:330.
Petit DIT Dariel OJ, Raby T, Ravaut F, Rothan-Tondeur M. Developing the serious games potential in nursing education. Nurse Educ Today. 2013;33:1569–75.
Pimmer C, Mateescu M, Gröhbiel U. Mobile and ubiquitous learning in higher education settings. A systematic review of empirical studies. Comput Hum Behav. 2016;63:490–501.
Ramsey-Stewart G, Burgess AW, Hill DA. Back to the future: teaching anatomy by whole-body dissection. Med J Aust. 2010;193:668–71.
Ricciardi F, De Paolis LT. A comprehensive review of serious games in health professions. Int J Comput Games Technol. 2014;2014:11.
Robert P, König A, Amieva H, Andrieu S, Bremond F, Bullock R, Ceccaldi M, Dubois B, Gauthier S, Kenigsberg P-A, Nave S, Orgogozo JM, Piano J, Benoit M, Touchon J, Vellas B, Yesavage J, Manera V. Recommendations for the use of serious games in people with Alzheimer’s disease, related disorders and frailty. Front Aging Neurosci. 2014;6:54.
Sterling M, Leung P, Wright D, Bishop TF. The use of social media in graduate medical education: a systematic review. Acad Med. 2017;92:1043–56.
Stirling A, Birt J. An enriched multimedia eBook application to facilitate learning of anatomy. Anat Sci Educ. 2014;7:19–27.
Su W, Xiao Y, He S, Huang P, Deng X. Three-dimensional printing models in congenital heart disease education for medical students: a controlled comparative study. BMC Med Educ. 2018;18:178.
Sural I. Augmented reality experience: initial perceptions of higher education students. Int J Instr. 2018;11:565–76.
Sutherland J, Belec J, Sheikh A, Chepelev L, Althobaity W, Chow BJW, Mitsouras D, Christensen A, Rybicki FJ, La Russa DJ. Applying modern virtual and augmented reality technologies to medical images and models. J Digit Imaging. 2019;32:38–53.
Tuong W, Larsen ER, Armstrong AW. Videos to influence: a systematic review of effectiveness of video-based education in modifying health behaviors. J Behav Med. 2014;37:218–33.
Wang R, Demaria S Jr, Goldberg A, Katz D. A systematic review of serious games in training health care professionals. Simul Healthc. 2016;11:41–51.
Winkelmann A. Anatomical dissection as a teaching method in medical school: a review of the evidence. Med Educ. 2007;41:15–22.
Wong G, Greenhalgh T, Pawson RJBME. Internet-based medical education: a realist review of what works, for whom and in what circumstances. BMC Med Educ. 2010;10:12.
Download references
Author information
Authors and affiliations.
Faculty of Health Sciences and Medicine, Bond University, Gold Coast, QLD, Australia
Christian Moro & Zane Stromberga
Faculty of Society and Design, Bond University, Gold Coast, QLD, Australia
You can also search for this author in PubMed Google Scholar
Corresponding author
Correspondence to Christian Moro .
Editor information
Editors and affiliations.
Monash University, Clayton, VIC, Australia
Debra Nestel
King's College London, London, UK
Gabriel Reedy
School of Nursing and Midwifery, La Trobe University, Bundoora, VIC, Australia
Lisa McKenna
Manchester Metropoli, Manchester, UK
Suzanne Gough
Rights and permissions
Reprints and permissions
Copyright information
© 2020 Springer Nature Singapore Pte Ltd.
About this entry
Cite this entry.
Moro, C., Stromberga, Z., Birt, J. (2020). Technology Considerations in Health Professions and Clinical Education. In: Nestel, D., Reedy, G., McKenna, L., Gough, S. (eds) Clinical Education for the Health Professions. Springer, Singapore. https://doi.org/10.1007/978-981-13-6106-7_118-1
Download citation
DOI : https://doi.org/10.1007/978-981-13-6106-7_118-1
Received : 20 September 2019
Accepted : 25 September 2019
Published : 05 February 2020
Publisher Name : Springer, Singapore
Print ISBN : 978-981-13-6106-7
Online ISBN : 978-981-13-6106-7
eBook Packages : Springer Reference Education Reference Module Humanities and Social Sciences Reference Module Education
- Publish with us
Policies and ethics
- Find a journal
- Track your research
Information
- Author Services
Initiatives
You are accessing a machine-readable page. In order to be human-readable, please install an RSS reader.
All articles published by MDPI are made immediately available worldwide under an open access license. No special permission is required to reuse all or part of the article published by MDPI, including figures and tables. For articles published under an open access Creative Common CC BY license, any part of the article may be reused without permission provided that the original article is clearly cited. For more information, please refer to https://www.mdpi.com/openaccess .
Feature papers represent the most advanced research with significant potential for high impact in the field. A Feature Paper should be a substantial original Article that involves several techniques or approaches, provides an outlook for future research directions and describes possible research applications.
Feature papers are submitted upon individual invitation or recommendation by the scientific editors and must receive positive feedback from the reviewers.
Editor’s Choice articles are based on recommendations by the scientific editors of MDPI journals from around the world. Editors select a small number of articles recently published in the journal that they believe will be particularly interesting to readers, or important in the respective research area. The aim is to provide a snapshot of some of the most exciting work published in the various research areas of the journal.
Original Submission Date Received: .
- Active Journals
- Find a Journal
- Proceedings Series
- For Authors
- For Reviewers
- For Editors
- For Librarians
- For Publishers
- For Societies
- For Conference Organizers
- Open Access Policy
- Institutional Open Access Program
- Special Issues Guidelines
- Editorial Process
- Research and Publication Ethics
- Article Processing Charges
- Testimonials
- Preprints.org
- SciProfiles
- Encyclopedia
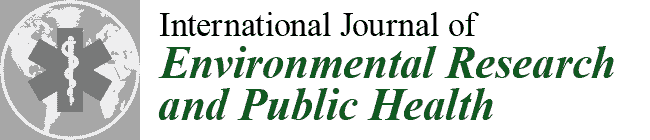
Article Menu
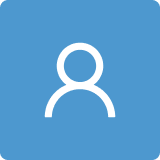
- Subscribe SciFeed
- Recommended Articles
- PubMed/Medline
- Google Scholar
- on Google Scholar
- Table of Contents
Find support for a specific problem in the support section of our website.
Please let us know what you think of our products and services.
Visit our dedicated information section to learn more about MDPI.
JSmol Viewer
Digital transformation in healthcare: technology acceptance and its applications.

1. Introduction
2. material and methods, 2.1. search strategy and bibliography reviews, 2.2. network analysis, initial search, 3.1. chronological development of the publications, 3.2. document type, 3.3. summary of the included articles, 4. concept matrix, 5. analysis of concepts, 5.1. information technology in health, 5.2. acceptance of e-health, 5.3. telemedicine, 5.4. security of ehealth, 5.5. education impact of e-health, 6. discussion, 7. conclusions, author contributions, institutional review board statement, informed consent statement, data availability statement, conflicts of interest.
- Iyawa, G.E.; Herselman, M.; Botha, A. Digital health innovation ecosystems: From systematic literature review to conceptual framework. Procedia Comput. Sci. 2016 , 100 , 244–252. [ Google Scholar ] [ CrossRef ] [ Green Version ]
- Gjellebæk, C.; Svensson, A.; Bjørkquist, C.; Fladeby, N.; Grundén, K. Management challenges for future digitalization of healthcare services. Futures 2020 , 124 , 102636. [ Google Scholar ] [ CrossRef ]
- Eden, R.; Burton-Jones, A.; Scott, I.; Staib, A.; Sullivan, C. Effects of eHealth on hospital practice: Synthesis of the current literature. Aust. Health Rev. 2018 , 42 , 568–578. [ Google Scholar ] [ CrossRef ] [ PubMed ] [ Green Version ]
- Eden, R.; Burton-Jones, A.; Grant, J.; Collins, R.; Staib, A.; Sullivan, C. Digitising an Australian university hospital: Qualitative analysis of staff-reported impacts. Aust. Health Rev. 2019 , 44 , 677–689. [ Google Scholar ] [ CrossRef ]
- Agarwal, R.; Gao, G.; DesRoches, C.; Jha, A.K. Research commentary—The digital transformation of healthcare: Current status and the road ahead. Inf. Syst. Res. 2010 , 21 , 796–809. [ Google Scholar ] [ CrossRef ] [ Green Version ]
- Gopal, G.; Suter-Crazzolara, C.; Toldo, L.; Eberhardt, W. Digital transformation in healthcare—Architectures of present and future information technologies. Clin. Chem. Lab. Med. CCLM 2019 , 57 , 328–335. [ Google Scholar ] [ CrossRef ] [ Green Version ]
- Webster, J.; Watson, R.T. Analyzing the past to prepare for the future: Writing a literature review. MIS Q. 2002 , 5 , xiii–xxiii. [ Google Scholar ]
- Marques, I.C.; Ferreira, J.J. Digital transformation in the area of health: Systematic review of 45 years of evolution. Health Technol. 2020 , 10 , 575–586. [ Google Scholar ] [ CrossRef ]
- Kraus, S.; Schiavone, F.; Pluzhnikova, A.; Invernizzi, A.C. Digital transformation in healthcare: Analyzing the current state-of-research. J. Bus. Res. 2021 , 123 , 557–567. [ Google Scholar ] [ CrossRef ]
- Kolasa, K.; Kozinski, G. How to Value Digital Health Interventions? A Systematic Literature Review. Int. J. Environ. Res. Public Health 2020 , 17 , 2119. [ Google Scholar ] [ CrossRef ] [ Green Version ]
- Hosseinzadeh, M.; Ahmed, O.H.; Ehsani, A.; Ahmed, A.M.; Hama, H.K.; Vo, B. The impact of knowledge on e-health: A systematic literature review of the advanced systems. Kybernetes 2021 , 50 , 1506–1520. [ Google Scholar ] [ CrossRef ]
- Nazir, S.; Ali, Y.; Ullah, N.; García-Magariño, I. Internet of Things for Healthcare Using Effects of Mobile Computing: A Systematic Literature Review. Wirel. Commun. Mob. Comput. 2019 , 2019 , 5931315. [ Google Scholar ] [ CrossRef ]
- Sanyal, C.; Stolee, P.; Juzwishin, D.; Husereau, D. Economic evaluations of eHealth technologies: A systematic review. PLoS ONE 2018 , 13 , e0198112. [ Google Scholar ] [ CrossRef ] [ Green Version ]
- Kampmeijer, R.; Pavlova, M.; Tambor, M.; Golinowska, S.; Groot, W. The use of e-health and m-health tools in health promotion and primary prevention among older adults: A systematic literature review. BMC Health Serv. Res. 2016 , 16 , 290. [ Google Scholar ] [ CrossRef ] [ Green Version ]
- Gagnon, M.-P.; Ngangue, P.; Payne-Gagnon, J.; Desmartis, M. m-Health adoption by healthcare professionals: A systematic review. J. Am. Med. Inform. Assoc. 2016 , 23 , 212–220. [ Google Scholar ] [ CrossRef ] [ Green Version ]
- Wilson, L.; Kim, A.; Szeto, D. The Evidence for the Economic Value of Ehealth in the United States Today: A Systematic Review. J. Int. Soc. Telemed. eHealth 2016 , 4 , e21. [ Google Scholar ]
- Hu, Y.; Bai, G. A Systematic Literature Review of Cloud Computing in Ehealth. Health Inform. Int. J. 2014 , 3 , 11–20. [ Google Scholar ] [ CrossRef ]
- Boonstra, A.; Versluis, A.; Vos, J.F.J. Implementing electronic health records in hospitals: A systematic literature review. BMC Health Serv. Res. 2014 , 14 , 370. [ Google Scholar ] [ CrossRef ] [ Green Version ]
- Pagliari, C.; Sloan, D.; Gregor, P.; Sullivan, F.; Detmer, D.; Kahan, J.P.; Oortwijn, W.; MacGillivray, S. What Is eHealth (4): A Scoping Exercise to Map the Field. J. Med. Internet Res. 2005 , 7 , e9. [ Google Scholar ] [ CrossRef ]
- Kesavadev, J.; Krishnan, G.; Mohan, V. Digital health and diabetes: Experience from India. Ther. Adv. Endocrinol. Metab. 2021 , 12 , 20420188211054676. [ Google Scholar ] [ CrossRef ]
- Attila, S.Z.; Miklos, S.; Tamas, P.; Viktoria, S.; Tamas, J. Global and national overview of the digital health ecosystem. Inf. Tarsad. 2021 , 21 , 47–66. [ Google Scholar ] [ CrossRef ]
- Malachynska, M.; Sheiko, V.; Polesova, T.; Samoylenko, I. Management of Healthcare Institutions in the Context of Changes and Reforms. AD ALTA-J. Interdiscip. Res. 2021 , 11 , 137–142. [ Google Scholar ]
- Lu, W.-C.; Tsai, I.C.; Wang, K.-C.; Tang, T.-A.; Li, K.-C.; Ke, Y.-C.; Chen, P.-T. Innovation Resistance and Resource Allocation Strategy of Medical Information Digitalization. Sustainability 2021 , 13 , 7888. [ Google Scholar ] [ CrossRef ]
- Burmann, A.; Tischler, M.; Faßbach, M.; Schneitler, S.; Meister, S. The Role of Physicians in Digitalizing Health Care Provision: Web-Based Survey Study. JMIR Med. Inform. 2021 , 9 , e31527. [ Google Scholar ] [ CrossRef ] [ PubMed ]
- Bogumil-Uçan, S.; Klenk, T. Varieties of health care digitalization: Comparing advocacy coalitions in Austria and Germany. Rev. Policy Res. 2021 , 38 , 478–503. [ Google Scholar ] [ CrossRef ]
- Zanutto, O. Digital transformation of care for older people. Int. J. Care Caring 2021 , 5 , 535–541. [ Google Scholar ] [ CrossRef ]
- Alauddin, M.S.; Baharuddin, A.S.; Mohd Ghazali, M.I. The Modern and Digital Transformation of Oral Health Care: A Mini Review. Healthcare 2021 , 9 , 118. [ Google Scholar ] [ CrossRef ]
- Alterazi, H.A. Towards Reaping the Promotions of Big Data in Healthcare Services. Int. Trans. J. Eng. Manag. Appl. Sci. Technol. 2021 , 12. [ Google Scholar ] [ CrossRef ]
- Schmidt-Kaehler, S.; Dadaczynski, K.; Gille, S.; Okan, O.; Schellinger, A.; Weigand, M.; Schaeffer, D. Health Literacy: Germany in the Digital Race to Catch Up Introduction of Technological Innovations is not Sufficient. Gesundheitswesen Bundesverb. Arzte Offentlichen Gesundheitsdienstes Ger. 2021 , 83 , 327–332. [ Google Scholar ] [ CrossRef ]
- Zhao, Y.; Canales, J.I. Never the twain shall meet? Knowledge strategies for digitalization in healthcare. Technol. Forecast. Soc. Chang. 2021 , 170 , 120923. [ Google Scholar ] [ CrossRef ]
- Roth, C.B.; Papassotiropoulos, A.; Brühl, A.B.; Lang, U.E.; Huber, C.G. Psychiatry in the Digital Age: A Blessing or a Curse? Int. J. Environ. Res. Public Health 2021 , 18 , 8302. [ Google Scholar ] [ CrossRef ]
- Ali, N.A.; NawazTahir, H.; Jabeen, R. Digitalisation provisions for controlling depression in developing countries: Short review. J. Pak. Med. Assoc. 2021 , 71 , 127–129. [ Google Scholar ] [ CrossRef ]
- Alimbaev, A.; Bitenova, B.; Bayandin, M. Information and Communication Technologies in the Healthcare System of the Republic of Kazakhstan: Economic Efficiency and Development Prospects. Montenegrin J. Econ. 2021 , 17 , 145–156. [ Google Scholar ] [ CrossRef ]
- Dick, H.; Doth, S.; Ernst, C.; Fischer, S.; Holderried, M. Current developments on digitalization Analysis of quality and economics in healthcare. Urologe 2021 , 60 , 1141–1149. [ Google Scholar ] [ CrossRef ]
- Alt, R.; Zimmermann, H.-D. The digital transformation of healthcare–An interview with Werner Dorfmeister. Electron. Mark. 2021 , 31 , 895–899. [ Google Scholar ] [ CrossRef ]
- Bartosiewicz, A.; Burzyńska, J.; Januszewicz, P. Polish Nurses’ Attitude to e-Health Solutions and Self-Assessment of Their IT Competence. J. Clin. Med. 2021 , 10 , 4799. [ Google Scholar ] [ CrossRef ]
- Mussener, U. Digital encounters: Human interactions in mHealth behavior change interventions. Digit Health 2021 , 7 , 20552076211029776. [ Google Scholar ] [ CrossRef ]
- Naumann, L.; Babitsch, B.; Hübner, U.H. eHealth policy processes from the stakeholders’ viewpoint: A qualitative comparison between Austria, Switzerland and Germany. Health Policy Technol. 2021 , 10 , 100505. [ Google Scholar ] [ CrossRef ]
- Saetra, H.S.; Fosch-Villaronga, E. Healthcare Digitalisation and the Changing Nature of Work and Society. Healthcare 2021 , 9 , 1007. [ Google Scholar ] [ CrossRef ]
- Zoltan, V.; Borbala, V.; Tamas, P.; Judit, L. Smart & Safe—Digitalisation strategy from a patient safety perspective. Orv. Hetil. 2021 , 162 , 1876–1884. [ Google Scholar ] [ CrossRef ]
- Hoch, P.; Arets, J. Video Consultation as a Model for the Acceptance of Digital Services in the Healthcare Sector. Beweg. Gesundh. 2021 , 37 , 151–156. [ Google Scholar ] [ CrossRef ]
- De Vos, J. A critique of digital mental health via assessing the psychodigitalisation of the COVID-19 crisis. Psychother. Polit. Int. 2021 , 19. [ Google Scholar ] [ CrossRef ]
- Beaulieu, M.; Bentahar, O. Digitalization of the healthcare supply chain: A roadmap to generate benefits and effectively support healthcare delivery. Technol. Forecast. Soc. Chang. 2021 , 167 , 120717. [ Google Scholar ] [ CrossRef ]
- Dang, T.H.; Nguyen, T.A.; Hoang Van, M.; Santin, O.; Tran, O.M.T.; Schofield, P. Patient-Centered Care: Transforming the Health Care System in Vietnam With Support of Digital Health Technology. J. Med. Internet Res. 2021 , 23 , e24601. [ Google Scholar ] [ CrossRef ]
- Gauthier, P.; Cardot, J.-M. Health care digitalization, the straightest pathway to personalization. Farmacia 2021 , 69 , 238–245. [ Google Scholar ] [ CrossRef ]
- Zhang, J.; Qi, L. Crisis Preparedness of Healthcare Manufacturing Firms during the COVID-19 Outbreak: Digitalization and Servitization. Int J Environ. Res Public Health 2021 , 18 , 5456. [ Google Scholar ] [ CrossRef ]
- Mallmann, C.A.; Domroese, C.M.; Schroeder, L.; Engelhardt, D.; Bach, F.; Rueckel, H.; Abramian, A.; Kaiser, C.; Mustea, A.; Faridi, A.; et al. Digital Technical and Informal Resources of Breast Cancer Patients from 2012 to 2020: Questionnaire-Based Longitudinal Trend Study. JMIR Cancer 2021 , 7 . [ Google Scholar ] [ CrossRef ]
- Quiros Fons, A. Limitations on conscientious objection in the area of healthcare in europe. Rev. Gen. Derecho Canonico Derecho Ecles. Estado 2021 . [ Google Scholar ]
- Kulkarni, P.; Chatterjee, S. Healthcare consumer behaviour: The impact of digital transformation of healthcare on consumer. Cardiometry 2021 , 135–144. [ Google Scholar ] [ CrossRef ]
- Wasmann, J.-W.A.; Lanting, C.P.; Huinck, W.J.; Mylanus, E.A.M.; van der Laak, J.W.M.; Govaerts, P.J.; Swanepoel, D.W.; Moore, D.R.; Barbour, D.L. Computational Audiology: New Approaches to Advance Hearing Health Care in the Digital Age. Ear Hear. 2021 , 42 , 1499. [ Google Scholar ] [ CrossRef ]
- Kanungo, R.P.; Gupta, S. Financial inclusion through digitalisation of services for well-being. Technol. Forecast. Soc. Change 2021 , 167 , 120721. [ Google Scholar ] [ CrossRef ]
- Fernandez-Luque, L.; Al Herbish, A.; Al Shammari, R.; Argente, J.; Bin-Abbas, B.; Deeb, A.; Dixon, D.; Zary, N.; Koledova, E.; Savage, M.O. Digital Health for Supporting Precision Medicine in Pediatric Endocrine Disorders: Opportunities for Improved Patient Care. Front. Pediatr. 2021 , 9 , 715705. [ Google Scholar ] [ CrossRef ]
- Wilson, A.; Saeed, H.; Pringle, C.; Eleftheriou, I.; Bromiley, P.A.; Brass, A. Artificial intelligence projects in healthcare: 10 practical tips for success in a clinical environment. BMJ Health CARE Inform. 2021 , 28 . [ Google Scholar ] [ CrossRef ]
- Ziadlou, D. Strategies during digital transformation to make progress in achievement of sustainable development by 2030. Leadersh. Health Serv. 2021 , 34 , 375–391. [ Google Scholar ] [ CrossRef ]
- Oh, S.S.; Kim, K.-A.; Kim, M.; Oh, J.; Chu, S.H.; Choi, J. Measurement of Digital Literacy Among Older Adults: Systematic Review. J. Med. Internet Res. 2021 , 23 , e26145. [ Google Scholar ] [ CrossRef ]
- Knitza, J.; Krusche, M.; Leipe, J. Digital diagnostic support in rheumatology. Z. Rheumatol. 2021 , 80 , 909–913. [ Google Scholar ] [ CrossRef ]
- Sergi, D.; Ucal Sari, I. Prioritization of public services for digitalization using fuzzy Z-AHP and fuzzy Z-WASPAS. Complex Intell. Syst. 2021 , 7 , 841–856. [ Google Scholar ] [ CrossRef ]
- Rosalia, R.A.; Wahba, K.; Milevska-Kostova, N. How digital transformation can help achieve value-based healthcare: Balkans as a case in point. Lancet Reg. Health Eur. 2021 , 4 , 100100. [ Google Scholar ] [ CrossRef ]
- New Study Smart Financing unlocks Billions in Funds for the digital Transformation of the Healthcare System. Gesundh. Qual. 2021 , 26 , 66–67. [ CrossRef ]
- Prisyazhnaya, N.V.; Pavlov, S.V. Sociohumanitarian knowledge and digitalization of medical education and healthcare. Sotsiologicheskie Issled 2021 , 1 , 146–148. [ Google Scholar ] [ CrossRef ]
- Odone, A.; Gianfredi, V.; Sorbello, S.; Capraro, M.; Frascella, B.; Vigezzi, G.P.; Signorelli, C. The Use of Digital Technologies to Support Vaccination Programmes in Europe: State of the Art and Best Practices from Experts’ Interviews. Vaccines 2021 , 9 , 1126. [ Google Scholar ] [ CrossRef ] [ PubMed ]
- Balta, M.; Valsecchi, R.; Papadopoulos, T.; Bourne, D.J. Digitalization and co-creation of healthcare value: A case study in Occupational Health. Technol. Forecast. Soc. Change 2021 , 168 , 120785. [ Google Scholar ] [ CrossRef ]
- Mues, S.; Surges, R. Telemedicine and mobile Healthcare Technologies Digitalization in Epilepsy Care—Needs and Challenges. Z. Epileptol. 2021 , 34 , 251–252. [ Google Scholar ] [ CrossRef ]
- Frick, N.R.J.; Möllmann, H.L.; Mirbabaie, M.; Stieglitz, S. Driving Digital Transformation during a Pandemic: Case Study of Virtual Collaboration in a German Hospital. JMIR Med. Inform. 2021 , 9 , e25183. [ Google Scholar ] [ CrossRef ] [ PubMed ]
- Dendere, R.; Janda, M.; Sullivan, C. Are we doing it right? We need to evaluate the current approaches for implementation of digital health systems. Aust. Health Rev. 2021 , 45 , 778–781. [ Google Scholar ] [ CrossRef ] [ PubMed ]
- Neumann, M.; Fehring, L.; Kinscher, K.; Truebel, H.; Dahlhausen, F.; Ehlers, J.P.; Mondritzki, T.; Boehme, P. Perspective of German medical faculties on digitization in the healthcare sector and its influence on the curriculum. GMS J. Med. Educ. 2021 , 38 . [ Google Scholar ] [ CrossRef ]
- Su, Y.; Hou, F.; Qi, M.; Li, W.; Ji, Y. A Data-Enabled Business Model for a Smart Healthcare Information Service Platform in the Era of Digital Transformation. J. Healthc. Eng. 2021 , 2021 , 5519891. [ Google Scholar ] [ CrossRef ]
- Masuda, Y.; Zimmermann, A.; Viswanathan, M.; Bass, M.; Nakamura, O.; Yamamoto, S. Adaptive enterprise architecture for the digital healthcare industry: A digital platform for drug development. Information 2021 , 12 , 67. [ Google Scholar ] [ CrossRef ]
- Frennert, S. Gender blindness: On health and welfare technology, AI and gender equality in community care. Nurs. Inq. 2021 , 28 , e12419. [ Google Scholar ] [ CrossRef ]
- Hasselgren, A.; Hanssen Rensaa, J.-A.; Kralevska, K.; Gligoroski, D.; Faxvaag, A. Blockchain for Increased Trust in Virtual Health Care: Proof-of-Concept Study. J. Med. Internet Res. 2021 , 23 , e28496. [ Google Scholar ] [ CrossRef ]
- Kim, H.K.; Lee, C.W. Relationships among Healthcare Digitalization, Social Capital, and Supply Chain Performance in the Healthcare Manufacturing Industry. Int. J. Environ. Res. Public Health 2021 , 18 , 1417. [ Google Scholar ] [ CrossRef ]
- Marchant, G.; Bonaiuto, F.; Bonaiuto, M.; Guillet Descas, E. Exercise and Physical Activity eHealth in COVID-19 Pandemic: A Cross-Sectional Study of Effects on Motivations, Behavior Change Mechanisms, and Behavior. Front. Psychol. 2021 , 12 , 618362. [ Google Scholar ] [ CrossRef ]
- Malfatti, G.; Racano, E.; Delle Site, R.; Gios, L.; Micocci, S.; Dianti, M.; Molini, P.B.; Allegrini, F.; Ravagni, M.; Moz, M. Enabling teleophthalmology during the COVID-19 pandemic in the Province of Trento, Italy: Design and implementation of a mHealth solution. PLoS ONE 2021 , 16 , e0257250. [ Google Scholar ] [ CrossRef ]
- Krasuska, M.; Williams, R.; Sheikh, A.; Franklin, B.; Hinder, S.; TheNguyen, H.; Lane, W.; Mozaffar, H.; Mason, K.; Eason, S.; et al. Driving digital health transformation in hospitals: A formative qualitative evaluation of the English Global Digital Exemplar programme. BMJ Health CARE Inform. 2021 , 28 . [ Google Scholar ] [ CrossRef ]
- Piccialli, F.; Giampaolo, F.; Prezioso, E.; Camacho, D.; Acampora, G. Artificial intelligence and healthcare: Forecasting of medical bookings through multi-source time-series fusion. Inf. Fusion 2021 , 74 , 1–16. [ Google Scholar ] [ CrossRef ]
- Kyllingstad, N.; Rypestøl, J.O.; Schulze-Krogh, A.C.; Tønnessen, M. Asset modification for regional industrial restructuring: Digitalization of the culture and experience industry and the healthcare sector. Reg. Stud. 2021 , 55 , 1764–1774. [ Google Scholar ] [ CrossRef ]
- Frasquilho, D.; Matias, R.; Grácio, J.; Sousa, B.; Luís-Ferreira, F.; Leal, J.; Cardoso, F.; Oliveira-Maia, A.J. Protocol for the Implementation and Assessment of “MoodUP”: A Stepped Care Model Assisted by a Digital Platform to Accelerate Access to Mental Health Care for Cancer Patients Amid the COVID-19 Pandemic. Int. J. Environ. Res. Public Health 2021 , 18 , 4629. [ Google Scholar ] [ CrossRef ]
- Leone, D.; Schiavone, F.; Appio, F.P.; Chiao, B. How does artificial intelligence enable and enhance value co-creation in industrial markets? An exploratory case study in the healthcare ecosystem. J. Bus. Res. 2021 , 129 , 849–859. [ Google Scholar ] [ CrossRef ]
- Kwon, I.-W.G.; Kim, S.-H.; Martin, D. Integrating Social Determinants of Health to Precision Medicine through Digital Transformation: An Exploratory Roadmap. Int. J. Environ. Res. Public Health 2021 , 18 , 5018. [ Google Scholar ] [ CrossRef ]
- Sim, S.S.; Yip, M.Y.T.; Wang, Z.; Tan, A.C.S.; Tan, G.S.W.; Cheung, C.M.G.; Chakravarthy, U.; Wong, T.Y.; Teo, K.Y.C.; Ting, D.S.W. Digital Technology for AMD Management in the Post-COVID-19 New Normal. Asia-Pac. J. Ophthalmol. 2021 , 10 , 39–48. [ Google Scholar ] [ CrossRef ]
- Christie, H.L.; Boots, L.M.M.; Hermans, I.; Govers, M.; Tange, H.J.; Verhey, F.R.J.; de Vugt, M. Business Models of eHealth Interventions to Support Informal Caregivers of People With Dementia in the Netherlands: Analysis of Case Studies. JMIR Aging 2021 , 4 , e24724. [ Google Scholar ] [ CrossRef ] [ PubMed ]
- Eberle, C.; Löhnert, M.; Stichling, S. Effectiveness of Disease-Specific mHealth Apps in Patients With Diabetes Mellitus: Scoping Review. JMIR Mhealth Uhealth 2021 , 9 , e23477. [ Google Scholar ] [ CrossRef ] [ PubMed ]
- Popkova, E.G.; Giyazov, A. Industrial and Manufacturing Engineering in Fight against the Virus Threat: Perspectives of Increasing Quality Based on Digitalization and Industry 4.0. Int. J. Qual. Res. 2021 , 15 , 291–308. [ Google Scholar ] [ CrossRef ]
- Reich, C.; Meder, B. Digital health highlights 2020 Digitalization in the year of the pandemic. Kardiologe 2021 , 15 , 153–159. [ Google Scholar ] [ CrossRef ]
- Hanrieder, T.; Maray, E.M. Digitalizing Community Health Work: A Struggle over the Values of Global Health Policy. Hist. Soc. Res.-Hist. Soz. 2021 , 46 , 136–159. [ Google Scholar ] [ CrossRef ]
- Aleksashina, A.A.; Bezuglov, S.V.; Fomicheva, L.M.; Grigoriev, A.V.; Katlishin, O.I.; Barinov, S. Innovations in the Management System of the Process of Taking Medicines by Patients Based on Digitalization. J. Pharm. Res. Int. 2021 , 33 , 216–222. [ Google Scholar ] [ CrossRef ]
- Haase, C.B.; Bearman, M.; Brodersen, J.; Hoeyer, K.; Risor, T. ‘You should see a doctor’, said the robot: Reflections on a digital diagnostic device in a pandemic age. Scand. J. Public Health 2021 , 49 , 33–36. [ Google Scholar ] [ CrossRef ]
- Mishra, A.; Gowrav, M.P.; Balamuralidhara, V.; Reddy, K.S. Health in Digital World: A Regulatory Overview in United States. J. Pharm. Res. Int. 2021 , 33 , 438–450. [ Google Scholar ] [ CrossRef ]
- Kokshagina, D.O. Managing shifts to value-based healthcare and value digitalization as a multi-level dynamic capability development process. Technol. Forecast. Soc. Change 2021 , 172 , 121072. [ Google Scholar ] [ CrossRef ]
- Loch, T.; Witzsch, U.; Reis, G. Digital transformation in urology-opportunity, risk or necessity? Urologe 2021 , 60 , 1125–1140. [ Google Scholar ] [ CrossRef ]
- Cajander, Å.; Hedström, G.; Leijon, S.; Larusdottir, M. Professional decision making with digitalisation of patient contacts in a medical advice setting: A qualitative study of a pilot project with a chat programme in Sweden. BMJ Open 2022 , 11 , e054103. [ Google Scholar ] [ CrossRef ]
- Botrugno, C. Information and Communication Technologies in Healthcare: A New Geography of Right to Health. Riv. Filos. Dirit.-J. Leg. Philos. 2021 , 10 , 163–187. [ Google Scholar ] [ CrossRef ]
- Jacquemard, T.; Doherty, C.P.; Fitzsimons, M.B. The anatomy of electronic patient record ethics: A framework to guide design, development, implementation, and use. BMC Med. Ethics 2021 , 22 , 9. [ Google Scholar ] [ CrossRef ]
- Behnke, M.; Valik, J.K.; Gubbels, S.; Teixeira, D.; Kristensen, B.; Abbas, M.; van Rooden, S.M.; Gastmeier, P.; van Mourik, M.S.M.; Network, P. Information technology aspects of large-scale implementation of automated surveillance of healthcare-associated infections. Clin. Microbiol. Infect. 2021 , 27 , S29–S39. [ Google Scholar ] [ CrossRef ]
- Peltoniemi, T.; Suomi, R.; Peura, S.; Lahteenoja, M.N.Y. Electronic prescription as a driver for digitalization in Finnish pharmacies. BMC Health Serv. Res. 2021 , 21 , 1–9. [ Google Scholar ] [ CrossRef ]
- Glock, H.; Nymberg, V.M.; Bolmsjö, B.B.; Holm, J.; Calling, S.; Wolff, M.; Pikkemaat, M. Attitudes, Barriers, and Concerns Regarding Telemedicine Among Swedish Primary Care Physicians: A Qualitative Study. Int. J. Gen. Med. 2021 , 14 , 9237. [ Google Scholar ] [ CrossRef ]
- Weitzel, E.C.; Quittschalle, J.; Welzel, F.D.; Loebner, M.; Hauth, I.; Riedel-Heller, S.G. E-Mental Health and healthcare apps in Germany. Nervenarzt 2021 , 92 , 1121–1129. [ Google Scholar ] [ CrossRef ]
- Sullivan, C.; Wong, I.; Adams, E.; Fahim, M.; Fraser, J.; Ranatunga, G.; Busato, M.; McNeil, K. Moving Faster than the COVID-19 Pandemic: The Rapid, Digital Transformation of a Public Health System. Appl. Clin. Inform. 2021 , 12 , 229–236. [ Google Scholar ] [ CrossRef ]
- Luca, M.M.; Mustea, L.; Taran, A.; Stefea, P.; Vatavu, S. Challenges on Radical Health Redesign to Reconfigure the Level of e-Health Adoption in EU Countries. Front. Public Health 2021 , 9 , 728287. [ Google Scholar ] [ CrossRef ]
- Negro-Calduch, E.; Azzopardi-Muscat, N.; Krishnamurthy, R.S.; Novillo-Ortiz, D. Technological progress in electronic health record system optimization: Systematic review of systematic literature reviews. Int. J. Med. Inf. 2021 , 152 , 104507. [ Google Scholar ] [ CrossRef ]
- Werutsky, G.; Barrios, C.H.; Cardona, A.F.; Albergaria, A.; Valencia, A.; Ferreira, C.G.; Rolfo, C.; de Azambuja, E.; Rabinovich, G.A.; Sposetti, G.; et al. Perspectives on emerging technologies, personalised medicine, and clinical research for cancer control in Latin America and the Caribbean. Lancet Oncol. 2021 , 22 , E488–E500. [ Google Scholar ] [ CrossRef ] [ PubMed ]
- Piasecki, J.; Walkiewicz-Zarek, E.; Figas-Skrzypulec, J.; Kordecka, A.; Dranseika, V. Ethical issues in biomedical research using electronic health records: A systematic review. Med. Health Care Philos. 2021 , 24 , 633–658. [ Google Scholar ] [ CrossRef ] [ PubMed ]
- Broenneke, J.B.; Hagen, J.; Kircher, P.; Matthies, H. Digitized healthcare in 2030-a possible scenario. Bundesgesundheitsblatt Gesundh. Gesundh. 2021 , 64 , 1285–1291. [ Google Scholar ] [ CrossRef ]
- Faure, S.; Napieralsk, J.; Cebelieu, T. The connected pharmacy, from today to tomorrow. Actual. Pharm. 2021 , 60 , 34–35. [ Google Scholar ] [ CrossRef ]
- Ghaleb, E.A.A.; Dominic, P.D.D.; Fati, S.M.; Muneer, A.; Ali, R.F. The Assessment of Big Data Adoption Readiness with a Technology–Organization–Environment Framework: A Perspective towards Healthcare Employees. Sustainability 2021 , 13 , 8379. [ Google Scholar ] [ CrossRef ]
- Verket, M.; Ickrath, M.; Haizmann, M.; Geldhauser, R.; Vite, S.; Bitzer, B.; Mueller-Wieland, D. Precision medicine using the electronic diabetes health record. Diabetologe 2021 , 17 , 807–812. [ Google Scholar ] [ CrossRef ]
- Lenz, S. “More like a support tool”: Ambivalences around digital health from medical developers’ perspective. Big Data Soc. 2021 , 8 , 2053951721996733. [ Google Scholar ] [ CrossRef ]
- De Sutter, E.; Coopmans, B.; Vanendert, F.; Dooms, M.; Allegaert, K.; Borry, P.; Huys, I. Clinical Research in Neonates: Redesigning the Informed Consent Process in the Digital Era. Front. Pediatr. 2021 , 9 , 724431. [ Google Scholar ] [ CrossRef ]
- Gevko, V.; Vivchar, O.; Sharko, V.; Radchenko, O.; Budiaiev, M.; Tarasenko, O. Cloud Technologies In Business Management. Financ. Credit Act.-Probl. Theory Pract. 2021 , 4 , 294–301. [ Google Scholar ] [ CrossRef ]
- El Majdoubi, D.; El Bakkali, H.; Sadki, S. SmartMedChain: A Blockchain-Based Privacy-Preserving Smart Healthcare Framework. J. Healthc. Eng. 2021 , 2021 , 4145512. [ Google Scholar ] [ CrossRef ]
- Thakur, A.; Soklaridis, S.; Crawford, A.; Mulsant, B.; Sockalingam, S. Using Rapid Design Thinking to Overcome COVID-19 Challenges in Medical Education. Acad. Med. 2021 , 96 . [ Google Scholar ] [ CrossRef ]
- Persson, J.; Rydenfält, C. Why Are Digital Health Care Systems Still Poorly Designed, and Why Is Health Care Practice Not Asking for More? Three Paths Toward a Sustainable Digital Work Environment. J. Med. Internet Res. 2021 , 23 , e26694. [ Google Scholar ] [ CrossRef ]
- Zippel-Schultz, B.; Palant, A.; Eurlings, C.; Ski, F.C.; Hill, L.; Thompson, D.R.; Fitzsimons, D.; Dixon, L.J.; Brandts, J.; Schuett, K.A.; et al. Determinants of acceptance of patients with heart failure and their informal caregivers regarding an interactive decision-making system: A qualitative study. BMJ Open 2021 , 11 , e046160. [ Google Scholar ] [ CrossRef ]
- Lam, K.; Purkayastha, S.; Kinross, J.M. The Ethical Digital Surgeon. J. Med. Internet Res. 2021 , 23 , e25849. [ Google Scholar ] [ CrossRef ]
- Manzeschke, A. Digitalization and organizational ethics. Scenarios from an ethical and philosophy of technology point of view. Ethik Med. 2021 , 33 , 219–232. [ Google Scholar ] [ CrossRef ]
- Dyda, A.; Fahim, M.; Fraser, J.; Kirrane, M.; Wong, I.; McNeil, K. Managing the Digital Disruption Associated with COVID-19-Driven Rapid Digital Transformation in Brisbane, Australia. Appl. Clin. Inform. 2021 , 12 , 1135–1143. [ Google Scholar ] [ CrossRef ]
- Beckmann, M.; Dittmer, K.; Jaschke, J.; Karbach, U.; Köberlein-Neu, J.; Nocon, M.; Rusniok, C.; Wurster, F.; Pfaff, H. Electronic patient record and its effects on social aspects of interprofessional collaboration and clinical workflows in hospitals (eCoCo): A mixed methods study protocol. BMC Health Serv. Res. 2021 , 21 , 377. [ Google Scholar ] [ CrossRef ]
- Numair, T.; Harrell, D.T.; Huy, N.T.; Nishimoto, F.; Muthiani, Y.; Nzou, S.M.; Lasaphonh, A.; Palama, K.; Pongvongsa, T.; Moji, K.; et al. Barriers to the Digitization of Health Information: A Qualitative and Quantitative Study in Kenya and Lao PDR Using a Cloud-Based Maternal and Child Registration System. Int. J. Environ. Res. Public Health 2021 , 18 , 6196. [ Google Scholar ] [ CrossRef ]
- Xiroudaki, S.; Schoubben, A.; Giovagnoli, S.; Rekkas, D.M. Dry Powder Inhalers in the Digitalization Era: Current Status and Future Perspectives. Pharmaceutics 2021 , 13 , 1455. [ Google Scholar ] [ CrossRef ]
- Droste, W.; Wieczorek, M. Advance of digitalization for specialized care? Working conditions of the care experts in ostomy, continence and wounds during the SARS-CoV-2 pandemic. Coloproctology 2021 , 43 , 223–228. [ Google Scholar ] [ CrossRef ]
- Lee, J.Y.; Irisboev, I.O.; Ryu, Y.-S. Literature Review on Digitalization in Facilities Management and Facilities Management Performance Measurement: Contribution of Industry 4.0 in the Global Era. Sustainability 2021 , 13 , 3432. [ Google Scholar ] [ CrossRef ]
- Giovagnoli, M.R.; Ciucciarelli, S.; Castrichella, L.; Giansanti, D. Artificial Intelligence in Digital Pathology: What Is the Future? Part 2: An Investigation on the Insiders. Healthcare 2021 , 9 , 1347. [ Google Scholar ] [ CrossRef ] [ PubMed ]
- Daguenet, E.; Magne, N. Use of telehealth services in the oncology setting: Daily routine and during sanitary crisis. Bull. Cancer 2021 , 108 , 627–634. [ Google Scholar ] [ CrossRef ] [ PubMed ]
- Hubmann, M.; Paetzmann-Sietas, B.; Morbach, H. Telemedicine and digital files-Where do we stand? Opportunities and challenges by the implementation into clinical and practice routines. Monatsschr. Kinderheilkd. 2021 , 169 , 711–716. [ Google Scholar ] [ CrossRef ]
- Vikhrov, I.; Abdurakhimov, Z.; Ashirbaev, S. The Use of Big Data in Healthcare: Lessons for Developing Countries from Uzbekistan. Health Probl. Civiliz. 2021 , 15 , 142–151. [ Google Scholar ] [ CrossRef ]
- Jahn, H.K.; Jahn, I.H.J.; Behringer, W.; Lyttle, M.D.; Roland, D.; On behalf of Paediatric Emergency Research United Kingdom and Ireland (PERUKI). Ireland A survey of mHealth use from a physician perspective in paediatric emergency care in the UK and Ireland. Eur. J. Pediatr. 2021 , 180 , 2409–2418. [ Google Scholar ] [ CrossRef ]
- Low, S.T.H.; Sakhardande, P.G.; Lai, Y.F.; Long, A.D.S.; Kaur-Gill, S. Attitudes and Perceptions toward Healthcare Technology Adoption among Older Adults in Singapore: A Qualitative Study. Front. Public Health 2021 , 9 , 588590. [ Google Scholar ] [ CrossRef ]
- Levasluoto, J.; Kohl, J.; Sigfrids, A.; Pihlajamaki, J.; Martikainen, J. Digitalization as an Engine for Change? Building a Vision Pathway towards a Sustainable Health Care System by Using the MLP and Health Economic Decision Modelling. Sustainability 2021 , 13 , 3007. [ Google Scholar ] [ CrossRef ]
- Verma, A.; Agarwal, G.; Gupta, A.K.; Sain, M. Novel Hybrid Intelligent Secure Cloud Internet of Things Based Disease Prediction and Diagnosis. Electronics 2021 , 10 , 3013. [ Google Scholar ] [ CrossRef ]
- Leung, P.P.L.; Wu, C.H.; Kwong, C.K.; Ip, W.H.; Ching, W.K. Digitalisation for optimising nursing staff demand modelling and scheduling in nursing homes. Technol. Forecast. Soc. Change 2021 , 164 , 120512. [ Google Scholar ] [ CrossRef ]
- Weber, S.; Heitmann, K.U. Interoperability in healthcare: Also prescribed for digital health applications (DiGA). Bundesgesundheitsblatt Gesundh. Gesundh. 2021 , 64 , 1262–1268. [ Google Scholar ] [ CrossRef ]
- Hogervorst, S.; Adriaanse, M.; Brandt, H.; Vervloet, M.; van Dijk, L.; Hugtenburg, J. Feasibility study of a digitalized nurse practitioner-led intervention to improve medication adherence in type 2 diabetes patients in Dutch primary care. Pilot Feasibility Stud. 2021 , 7 , 152. [ Google Scholar ] [ CrossRef ]
- Khan, I.H.; Javaid, M. Big Data Applications in Medical Field: A Literature Review. J. Ind. Integr. Manag. 2021 , 06 , 53–69. [ Google Scholar ] [ CrossRef ]
- Cherif, E.; Bezaz, N.; Mzoughi, M. Do personal health concerns and trust in healthcare providers mitigate privacy concerns? Effects on patients’ intention to share personal health data on electronic health records. Soc. Sci. Med. 2021 , 283 , 114146. [ Google Scholar ] [ CrossRef ]
- Bingham, G.; Tong, E.; Poole, S.; Ross, P.; Dooley, M. A longitudinal time and motion study quantifying how implementation of an electronic medical record influences hospital nurses’ care delivery. Int. J. Med. Inf. 2021 , 153 , 104537. [ Google Scholar ] [ CrossRef ]
- Broich, K.; Lobker, W.; Lauer, W. Digitization of the healthcare system: The BfArM’s contribution to the development of potential. Bundesgesundheitsblatt Gesundh. Gesundh. 2021 , 64 , 1292–1297. [ Google Scholar ] [ CrossRef ]
- Klemme, I.; Richter, B.; De Sabbata, K.; Wrede, B.; Vollmer, A.L. A Multi-Directional and Agile Academic Knowledge Transfer Strategy for Healthcare Technology. Front Robot AI 2021 , 8 , 789827. [ Google Scholar ] [ CrossRef ]
- Dillenseger, A.; Weidemann, M.L.; Trentzsch, K.; Inojosa, H.; Haase, R.; Schriefer, D.; Voigt, I.; Scholz, M.; Akgün, K.; Ziemssen, T. Digital Biomarkers in Multiple Sclerosis. Brain Sci. 2021 , 11 , 1519. [ Google Scholar ] [ CrossRef ]
- Wangler, J.; Jansky, M. The national health portal: Development opportunities and potential uses with special consideration of the general practitioner’s perspective. Bundesgesundheitsblatt Gesundh. Gesundh. 2021 , 64 , 360–367. [ Google Scholar ] [ CrossRef ]
- Kuhn, S.; Huettl, F.; Deutsch, K.; Kirchgaessner, E.; Huber, T.; Kneist, W. Surgical Education in the Digital Age—Virtual Reality, Augmented Reality and Robotics in the Medical School. Zentralbl. Chir. 2021 , 146 , 37–43. [ Google Scholar ] [ CrossRef ]
- Aldekhyyel, R.N.; Almulhem, J.A.; Binkheder, S. Usability of Telemedicine Mobile Applications during COVID-19 in Saudi Arabia: A Heuristic Evaluation of Patient User Interfaces. Healthcare 2021 , 9 , 1574. [ Google Scholar ] [ CrossRef ] [ PubMed ]
- Christlein, D.; Kast, J.; Baumhauer, M. Current developments in healthcare information technology Impact on structured reporting. Radiologe 2021 , 61 , 986–994. [ Google Scholar ] [ CrossRef ] [ PubMed ]
- Bergier, H.; Duron, L.; Sordet, C.; Kawka, L.; Schlencker, A.; Chasset, F.; Arnaud, L. Digital health, big data and smart technologies for the care of patients with systemic autoimmune diseases: Where do we stand? Autoimmun. Rev. 2021 , 20 , 102864. [ Google Scholar ] [ CrossRef ]
- Sitges-Maciá, E.; Bonete-López, B.; Sánchez-Cabaco, A.; Oltra-Cucarella, J. Effects of e-Health Training and Social Support Interventions for Informal Caregivers of People with Dementia—A Narrative Review. Int. J. Environ. Res. Public Health 2021 , 18 , 7728. [ Google Scholar ] [ CrossRef ] [ PubMed ]
- Rani, R.; Kumar, R.; Mishra, R.; Sharma, S.K. Digital health: A panacea in COVID-19 crisis. J. Fam. Med. Prim. CARE 2021 , 10 , 62–65. [ Google Scholar ] [ CrossRef ]
- Fredriksen, E.; Thygesen, E.; Moe, C.E.; Martinez, S. Digitalisation of municipal healthcare collaboration with volunteers: A case study applying normalization process theory. BMC Health Serv. Res. 2021 , 21 , 410. [ Google Scholar ] [ CrossRef ]
- Caixeta, M.C.B.F.; Fabricio, M.M. Physical-digital model for co-design in healthcare buildings. J. Build. Eng. 2021 , 34 , 101900. [ Google Scholar ] [ CrossRef ]
- Gupta, M.; Soeny, K. Algorithms for rapid digitalization of prescriptions. Vis. Inform. 2021 , 5 , 54–69. [ Google Scholar ] [ CrossRef ]
- Dobson, R.; Whittaker, R.; Wihongi, H.; Andrew, P.; Armstrong, D.; Bartholomew, K.; Sporle, A.; Wells, S. Patient perspectives on the use of health information. N. Z. Med. J. 2021 , 134 , 48–62. [ Google Scholar ]
- Choi, K.; Gitelman, Y.; Leri, D.; Deleener, M.E.; Hahn, L.; O’Malley, C.; Lang, E.; Patel, N.; Jones, T.; Emperado, K.; et al. Insourcing and scaling a telemedicine solution in under 2 weeks: Lessons for the digital transformation of health care. Healthc. J. Deliv. Sci. Innov. 2021 , 9 , 100568. [ Google Scholar ] [ CrossRef ]
- Müller-Wirtz, L.M.; Volk, T. Big Data in Studying Acute Pain and Regional Anesthesia. J. Clin. Med. 2021 , 10 , 1425. [ Google Scholar ] [ CrossRef ]
- Sembekov, A.; Tazhbayev, N.; Ulakov, N.; Tatiyeva, G.; Budeshov, Y. Digital modernization of Kazakhstan’s economy in the context of global trends. Econ. Ann.-XXI 2021 , 187 , 51–62. [ Google Scholar ] [ CrossRef ]
- Aulenkamp, J.; Mikuteit, M.; Löffler, T.; Schmidt, J. Overview of digital health teaching courses in medical education in Germany in 2020. GMS J. Med. Educ. 2021 , 38 . [ Google Scholar ] [ CrossRef ]
- Paul, K.T.; Janny, A.; Riesinger, K. Austria’s Digital Vaccination Registry: Stakeholder Views and Implications for Governance. Vaccines 2021 , 9 , 1495. [ Google Scholar ] [ CrossRef ]
- Lemmen, C.; Simic, D.; Stock, S. A Vision of Future Healthcare: Potential Opportunities and Risks of Systems Medicine from a Citizen and Patient Perspective—Results of a Qualitative Study. Int. J. Environ. Res. Public Health 2021 , 18 , 9879. [ Google Scholar ] [ CrossRef ]
- Golz, C.; Peter, K.A.; Zwakhalen, S.M.G.; Hahn, S. Technostress Among Health Professionals—A Multilevel Model and Group Comparisons between Settings and Professions. Inform. Health Soc. Care 2021 , 46 , 137–149. [ Google Scholar ] [ CrossRef ]
- Tarikere, S.; Donner, I.; Woods, D. Diagnosing a healthcare cybersecurity crisis: The impact of IoMT advancements and 5G. Bus. Horiz. 2021 , 64 , 799–807. [ Google Scholar ] [ CrossRef ]
- Li, T.; Zhao, Y.; Sun, L.; Feng, C. Artificial intelligence medical platform and clinical care of chronic obstructive pulmonary disease based on microprocessor. Microprocess. Microsyst. 2021 , 82 , 103879. [ Google Scholar ] [ CrossRef ]
- Rouge-Bugat, M.-E.; Beranger, J. Evolution and impact of digital technology in the general practitioner-patient relationship. Case of the cancer patient. Bull. Acad. Natl. Med. 2021 , 205 , 822–830. [ Google Scholar ] [ CrossRef ]
- Iodice, F.; Romoli, M.; Giometto, B.; Clerico, M.; Tedeschi, G.; Bonavita, S.; Leocani, L.; Lavorgna, L.; Digital Technologies, W.; Social Media Study Group of the Italian Society of, N. Stroke and digital technology: A wake-up call from COVID-19 pandemic. Neurol. Sci. 2021 , 42 , 805–809. [ Google Scholar ] [ CrossRef ]
- Kulzer, B. How do people with diabetes benefit from big data and artificial intelligence? Diabetologe 2021 , 17 , 799–806. [ Google Scholar ] [ CrossRef ]
- Khosla, S.; Tepie, M.F.; Nagy, M.J.; Kafatos, G.; Seewald, M.; Marchese, S.; Liwing, J. The Alignment of Real-World Evidence and Digital Health: Realising the Opportunity. Ther. Innov. Regul. Sci. 2021 , 55 , 889–898. [ Google Scholar ] [ CrossRef ] [ PubMed ]
- Dantas, E.; Nogaroli, R. The Rise of Robotics and Artificial in the Ligence in Healthcare: New Challenges for the Doctrine of Informed Consent. Med. LAW 2021 , 40 , 15–61. [ Google Scholar ]
- Gaur, L.; Afaq, A.; Singh, G.; Dwivedi, Y.K. Role of artificial intelligence and robotics to foster the touchless travel during a pandemic: A review and research agenda. Int. J. Contemp. Hosp. Manag. 2021 , 33 , 4079–4098. [ Google Scholar ] [ CrossRef ]
- Khodadad-Saryazdi, A. Exploring the telemedicine implementation challenges through the process innovation approach: A case study research in the French healthcare sector. Technovation 2021 , 107 , 102273. [ Google Scholar ] [ CrossRef ]
- Bellavista, P.; Torello, M.; Corradi, A.; Foschini, L. Smart Management of Healthcare Professionals Involved in COVID-19 Contrast with SWAPS. Front. Sustain. Cities 2021 , 3 . [ Google Scholar ] [ CrossRef ]
- Laukka, E.; Polkki, T.; Heponiemi, T.; Kaihlanen, A.-M.; Kanste, O. Leadership in Digital Health Services: Protocol for a Concept Analysis. JMIR Res. Protoc. 2021 , 10. [ Google Scholar ] [ CrossRef ]
- Singh, R.P.; Haleem, A.; Javaid, M.; Kataria, R.; Singhal, S. Cloud Computing in Solving Problems of COVID-19 Pandemic. J. Ind. Integr. Manag. 2021 , 6 , 209–219. [ Google Scholar ] [ CrossRef ]
- Patalano, R.; De Luca, V.; Vogt, J.; Birov, S.; Giovannelli, L.; Carruba, G.; Pivonello, C.; Stroetmann, V.; Triassi, M.; Colao, A.; et al. An Innovative Approach to Designing Digital Health Solutions Addressing the Unmet Needs of Obese Patients in Europe. Int. J. Environ. Res. Public. Health 2021 , 18 , 579. [ Google Scholar ] [ CrossRef ]
- Mantel-Teeuwisse, A.K.; Meilianti, S.; Khatri, B.; Yi, W.; Azzopardi, L.M.; Acosta Gómez, J.; Gülpınar, G.; Bennara, K.; Uzman, N. Digital Health in Pharmacy Education: Preparedness and Responsiveness of Pharmacy Programmes. Educ. Sci. 2021 , 11 , 296. [ Google Scholar ] [ CrossRef ]
- Mues, S.; Surges, R. Research and development of telemedical applications and mobile health technologies in epilepsy Survey in Germany, Austria and Switzerland. Z. Epileptol. 2021 , 34 , 253–256. [ Google Scholar ] [ CrossRef ]
- Bosch-Capblanch, X.; O’Donnell, D.; Krause, L.K.; Auer, C.; Oyo-Ita, A.; Samba, M.; Matsinhe, G.; Garba, A.B.; Rodríguez, D.; Zuske, M.; et al. Researching, co-creating and testing innovations in paper-based health information systems (PHISICC) to support health workers’ decision-making: Protocol of a multi-country, transdisciplinary, mixed-methods research programme in three sub-Saharan countries. Health Res. Policy Syst. 2021 , 19 , 112. [ Google Scholar ] [ CrossRef ]
- Jaboyedoff, M.; Rakic, M.; Bachmann, S.; Berger, C.; Diezi, M.; Fuchs, O.; Frey, U.; Gervaix, A.; Glucksberg, A.S.; Grotzer, M.; et al. SwissPedData: Standardising hospital records for the benefit of paediatric research. Swiss Med. Wkly. 2021 , 151 , w30069. [ Google Scholar ] [ CrossRef ]
- Nadhamuni, S.; John, O.; Kulkarni, M.; Nanda, E.; Venkatraman, S.; Varma, D.; Balsari, S.; Gudi, N.; Samantaray, S.; Reddy, H.; et al. Driving digital transformation of comprehensive primary health services at scale in India: An enterprise architecture framework. BMJ Glob. Health 2021 , 6 , e005242. [ Google Scholar ] [ CrossRef ]
- Hertling, S.; Loos, F.M.; Graul, I. Telemedicine as a Therapeutic Option in Sports Medicine: Results of a Nationwide Cross-Sectional Study among Physicians and Patients in Germany. Int. J. Environ. Res. Public. Health 2021 , 18 , 7110. [ Google Scholar ] [ CrossRef ]
- Khan, I.S.; Ahmad, M.O.; Majava, J. Industry 4.0 and sustainable development: A systematic mapping of triple bottom line, Circular Economy and Sustainable Business Models perspectives. J. Clean. Prod. 2021 , 297 , 126655. [ Google Scholar ] [ CrossRef ]
- Mun, S.K.; Wong, K.H.; Lo, S.-C.B.; Li, Y.; Bayarsaikhan, S. Artificial Intelligence for the Future Radiology Diagnostic Service. Front. Mol. Biosci. 2021 , 7 , 614258. [ Google Scholar ] [ CrossRef ]
- Xi, X.; Wei, S.; Lin, K.L.; Zhou, H.; Wang, K.; Zhou, H.; Li, Z.; Nan, N.; Qiu, L.; Hu, F.; et al. Digital Technology, Knowledge Level, and Food Safety Governance: Implications for National Healthcare System. Front. Public Health 2021 , 9 , 753950. [ Google Scholar ] [ CrossRef ]
- Weichert, J.; Welp, A.; Scharf, J.L.; Dracopoulos, C.; Becker, W.-H.; Gembicki, M. The Use of Artificial Intelligence in Automation in the Fields of Gynaecology and Obstetrics—An Assessment of the State of Play. Geburtshilfe Frauenheilkd. 2021 , 81 , 1203–1216. [ Google Scholar ] [ CrossRef ]
- Liang, J.; Li, Y.; Zhang, Z.; Shen, D.; Xu, J.; Zheng, X.; Wang, T.; Tang, B.; Lei, J.; Zhang, J. Adoption of Electronic Health Records (EHRs) in China during the Past 10 Years: Consecutive Survey Data Analysis and Comparison of Sino-American Challenges and Experiences. J. Med. Internet Res. 2021 , 23 , e24813. [ Google Scholar ] [ CrossRef ]
- Williams, R.; Sheikh, A.; Franklin, B.D.; Krasuska, M.; Nguyen, H.T.; Hinder, S.; Lane, W.; Mozaffar, H.; Mason, K.; Eason, S.; et al. Using Blueprints to promote interorganizational knowledge transfer in digital health initiatives-a qualitative exploration of a national change program in English hospitals. J. Am. Med. Inform. Assoc. 2021 , 28 , 1431–1439. [ Google Scholar ] [ CrossRef ] [ PubMed ]
- Feroz, A.S.; Khoja, A.; Saleem, S. Equipping community health workers with digital tools for pandemic response in LMICs. Arch. Public Health 2021 , 79 , 1. [ Google Scholar ] [ CrossRef ] [ PubMed ]
- Huser, D.; Bon, A.; Anifalaje, A. Uncovering generative mechanisms of information use for project monitoring in humanitarian health management information systems. Electron. J. Inf. Syst. Dev. Ctries. 2021 , 87 , e12184. [ Google Scholar ] [ CrossRef ]
- Apostolos, K. Electronic health records and personal data protection: The legal and sociological approach. Arch. Hell. Med. 2021 , 38 , 394–400. [ Google Scholar ]
- Simsek, P.; Gunduz, A.; Imamoglu, M. Technological Innovations in Emergency Department Services. Eurasian J. Emerg. Med. 2021 , 20 , 128–134. [ Google Scholar ] [ CrossRef ]
- Khamisy-Farah, R.; Furstenau, L.B.; Kong, J.D.; Wu, J.; Bragazzi, N.L. Gynecology Meets Big Data in the Disruptive Innovation Medical Era: State-of-Art and Future Prospects. Int. J. Environ. Res. Public Health 2021 , 18 , 5058. [ Google Scholar ] [ CrossRef ]
- Egarter, S.; Mutschler, A.; Brass, K. Impact of COVID-19 on digital medical education: Compatibility of digital teaching and examinations with integrity and ethical principles. Int. J. Educ. Integr. 2021 , 17 , 18. [ Google Scholar ] [ CrossRef ]
- Can, Y.S.; Ersoy, C. Privacy-preserving federated deep learning for wearable IoT-based biomedical monitoring. ACM Trans. Internet Technol. TOIT 2021 , 21 , 1–17. [ Google Scholar ] [ CrossRef ]
- Sung, S.; Hørthe, H.; Svendsen, Ø.V.; van Duinen, A.J.; Salvesen, Ø.; Vandi, A.; Bolkan, H.A. Early evaluation of the transition from an analog to an electronic surgical logbook system in Sierra Leone. BMC Med. Educ. 2021 , 21 , 578. [ Google Scholar ] [ CrossRef ]
- Zoellner, J.P.; Noda, A.H.; McCoy, J.; Roth, C.; Fischer, D.; Bollensen, E.; Henn, K.-H.; Willems, L.M.; Leyer, A.-C.; Schubert-Bast, S.; et al. Attitudes and barriers towards telemedicine in epilepsy care: A survey among neurological practices. Z. Epileptol. 2021 , 34 , 318–323. [ Google Scholar ] [ CrossRef ]
- Oliveira, J.; Azevedo, A.; Ferreira, J.J.; Gomes, S.; Lopes, J.M. An insight on B2B Firms in the Age of Digitalization and Paperless Processes. Sustainability 2021 , 13 , 1565. [ Google Scholar ] [ CrossRef ]
- Goudarzi, E.V.; Houshmand, M.; Valilai, O.F.; Ghezavati, V.; Bamdad, S. Equilibrial service composition model in Cloud manufacturing (ESCM) based on non-cooperative and cooperative game theory for healthcare service equipping. PeerJ Comput. Sci. 2021 , 7 , e410. [ Google Scholar ] [ CrossRef ]
- Li, J.-P.O.; Liu, H.; Ting, D.S.J.; Jeon, S.; Chan, R.V.P.; Kim, J.E.; Sim, D.A.; Thomas, P.B.M.; Lin, H.; Chen, Y.; et al. Digital technology, tele-medicine and artificial intelligence in ophthalmology: A global perspective. Prog. Retin. Eye Res. 2021 , 82 , 100900. [ Google Scholar ] [ CrossRef ]
- Klimanov, D.; Tretyak, O.; Goren, U.; White, T. Transformation of Value in Innovative Business Models: The Case of Pharmaceutical Market. Foresight Sti Gov. 2021 , 15 , 52–65. [ Google Scholar ] [ CrossRef ]
- Nadav, J.; Kaihlanen, A.-M.; Kujala, S.; Laukka, E.; Hilama, P.; Koivisto, J.; Keskimaki, I.; Heponiemi, T. How to Implement Digital Services in a Way That They Integrate into Routine Work: Qualitative Interview Study among Health and Social Care Professionals. J. Med. Internet Res. 2021 , 23 , e31668. [ Google Scholar ] [ CrossRef ]
- Spanakis, P.; Heron, P.; Walker, L.; Crosland, S.; Wadman, R.; Newbronner, E.; Johnston, G.; Gilbody, S.; Peckham, E. Use of the Internet and Digital Devices Among People With Severe Mental Ill Health During the COVID-19 Pandemic Restrictions. Front. Psychiatry 2021 , 12 , 732735. [ Google Scholar ] [ CrossRef ]
- Polyakov, M.; Kovshun, N. Diffusion of Innovations as a Key Driver of the Digital Economy Development. Balt. J. Econ. Stud. 2021 , 7 , 84–92. [ Google Scholar ] [ CrossRef ]
- Fristedt, S.; Smith, F.; Grynne, A.; Browall, M. Digi-Do: A digital information tool to support patients with breast cancer before, during, and after start of radiotherapy treatment: An RCT study protocol. BMC Med. Inform. Decis. Mak. 2021 , 21 , 76. [ Google Scholar ] [ CrossRef ]
- Mandal, M.; Dutta, R. Identity-based outsider anonymous cloud data outsourcing with simultaneous individual transmission for IoT environment. J. Inf. Secur. Appl. 2021 , 60 , 102870. [ Google Scholar ] [ CrossRef ]
- Ozdemir, V. Digital Is Political: Why We Need a Feminist Conceptual Lens on Determinants of Digital Health. OMICS- J. Integr. Biol. 2021 , 25 , 249–254. [ Google Scholar ] [ CrossRef ]
- Eberle, C.; Stichling, S. Clinical Improvements by Telemedicine Interventions Managing Type 1 and Type 2 Diabetes: Systematic Meta-review. J. Med. Internet Res. 2021 , 23 , e23244. [ Google Scholar ] [ CrossRef ] [ PubMed ]
- Iakovleva, T.; Oftedal, E.; Bessant, J. Changing Role of Users-Innovating Responsibly in Digital Health. Sustainability 2021 , 13 , 1616. [ Google Scholar ] [ CrossRef ]
- Von Solodkoff, M.; Strumann, C.; Steinhaeuser, J. Acceptance of Care Offers for exclusive Remote Treatment Illustrated by the Telemedical Model Project “docdirekt” with a Mixed-Methods Design. Gesundheitswesen 2021 , 83 , 186–194. [ Google Scholar ] [ CrossRef ] [ PubMed ]
- Khuntia, J.; Ning, X.; Stacey, R. Digital Orientation of Health Systems in the Post-COVID-19 “New Normal” in the United States: Cross-sectional Survey. J. Med. Internet Res. 2021 , 23 , e30453. [ Google Scholar ] [ CrossRef ]
- Ochoa, J.G.D.; Csiszár, O.; Schimper, T. Medical recommender systems based on continuous-valued logic and multi-criteria decision operators, using interpretable neural networks. BMC Med. Inform. Decis. Mak. 2021 , 21 , 186. [ Google Scholar ] [ CrossRef ]
- Masłoń-Oracz, A.; Ojiambo, J.; Kevin, O. Digital Economy as a Driver of Sustainable and Inclusive Growth in Africa—Case Study. In Research and Innovation Forum 2020: Disruptive Technologies in Times of Change ; Springer: Cham, Switzerland, 2020; pp. 605–616. [ Google Scholar ]
- Abrahams, M.; Matusheski, N.V. Personalised nutrition technologies: A new paradigm for dietetic practice and training in a digital transformation era. J. Hum. Nutr. Diet. 2020 , 33 , 295. [ Google Scholar ] [ CrossRef ] [ Green Version ]
- Agnihothri, S.; Cui, L.; Delasay, M.; Rajan, B. The value of mHealth for managing chronic conditions. Health Care Manag. Sci. 2020 , 23 , 185–202. [ Google Scholar ] [ CrossRef ]
- Bukowski, M.; Farkas, R.; Beyan, O.; Moll, L.; Hahn, H.; Kiessling, F.; Schmitz-Rode, T. Implementation of eHealth and AI integrated diagnostics with multidisciplinary digitized data: Are we ready from an international perspective? Eur. Radiol. 2020 , 30 , 5510–5524. [ Google Scholar ] [ CrossRef ]
- Chiang, D.-L.; Huang, Y.-T.; Chen, T.-S.; Lai, F.-P. Applying time-constraint access control of personal health record in cloud computing. Enterp. Inf. Syst. 2020 , 14 , 266–281. [ Google Scholar ] [ CrossRef ]
- Cobelli, N.; Chiarini, A. Improving customer satisfaction and loyalty through mHealth service digitalization: New challenges for Italian pharmacists. TQM J. 2020 , 32 , 1541–1560. [ Google Scholar ] [ CrossRef ]
- Crawford, A.; Serhal, E. Digital Health Equity and COVID-19: The Innovation Curve Cannot Reinforce the Social Gradient of Health. J. Med. Internet Res. 2020 , 22 , e19361. [ Google Scholar ] [ CrossRef ]
- Do Nascimento, M.G.; Iorio, G.; Thome, T.G.; Medeiros, A.A.M.; Mendonca, F.M.; Campos, F.A.; David, J.M.; Stroele, V.; Dantas, M.A.R. Covid-19: A Digital Transformation Approach to a Public Primary Healthcare Environment. In Proceedings of the 2020 IEEE Symposium on Computers and Communications (ISCC), Rennes, France, 6–9 June 2020; IEEE: Rennes, France; pp. 1–6. [ Google Scholar ]
- Geiger, M.F.; Wilhelmy, S.; Schmidt, M.; Firsching, R.; Groß, D.; Clusmann, H. Current Practice of Neurosurgical Teleconsultation in Germany. J. Neurol. Surg. Part Cent. Eur. Neurosurg. 2020 , 81 , 521–528. [ Google Scholar ] [ CrossRef ]
- Gochhait, S.; Singh, T.; Bende, A.; Thapliyal, M.; Vemulapalli, H.; Shukla, G.; Ghosh, D.; Chinta, D. Implementation of EHR using Digital Transformation: A study on Telemedicine. In Proceedings of the 2020 International Conference for Emerging Technology (INCET), Belgaum, India, 5–7 June 2020; pp. 1–4. [ Google Scholar ]
- Kernebeck, S.; Busse, T.S.; Böttcher, M.D.; Weitz, J.; Ehlers, J.; Bork, U. Impact of mobile health and medical applications on clinical practice in gastroenterology. World J. Gastroenterol. 2020 , 26 , 4182–4197. [ Google Scholar ] [ CrossRef ]
- Klinker, K.; Wiesche, M.; Krcmar, H. Digital Transformation in Health Care: Augmented Reality for Hands-Free Service Innovation. Inf. Syst. Front. 2020 , 22 , 1419–1431. [ Google Scholar ] [ CrossRef ] [ Green Version ]
- Krasuska, M.; Williams, R.; Sheikh, A.; Franklin, B.D.; Heeney, C.; Lane, W.; Mozaffar, H.; Mason, K.; Eason, S.; Hinder, S.; et al. Technological Capabilities to Assess Digital Excellence in Hospitals in High Performing Health Care Systems: International eDelphi Exercise. J. Med. Internet Res. 2020 , 22 , e17022. [ Google Scholar ] [ CrossRef ]
- Leigh, S.; Ashall-Payne, L.; Andrews, T. Barriers and Facilitators to the Adoption of Mobile Health Among Health Care Professionals From the United Kingdom: Discrete Choice Experiment. JMIR MHealth UHealth 2020 , 8 , e17704. [ Google Scholar ] [ CrossRef ]
- Minssen, T.; Mimler, M.; Mak, V. When Does Stand-Alone Software Qualify as a Medical Device in the European Union?—The Cjeu’s Decision in Snitem and What It Implies for the Next Generation of Medical Devices. Med. Law Rev. 2020 , 28 , 615–624. [ Google Scholar ] [ CrossRef ]
- Mueller, M.; Knop, M.; Niehaves, B.; Adarkwah, C.C. Investigating the Acceptance of Video Consultation by Patients in Rural Primary Care: Empirical Comparison of Preusers and Actual Users. JMIR Med. Inform. 2020 , 8 , e20813. [ Google Scholar ] [ CrossRef ]
- Nadarzynski, T.; Bayley, J.; Llewellyn, C.; Kidsley, S.; Graham, C.A. Acceptability of artificial intelligence (AI)-enabled chatbots, video consultations and live webchats as online platforms for sexual health advice. BMJ Sex. Reprod. Health 2020 , 46 , 210–217. [ Google Scholar ] [ CrossRef ]
- Pekkarinen, S.; Hasu, M.; Melkas, H.; Saari, E. Information ecology in digitalising welfare services: A multi-level analysis. Inf. Technol. People 2021 , 34 , 1697–1720. [ Google Scholar ] [ CrossRef ]
- SHAPES Secure Cloud Platform for HealthCare Solutions and Services. In Proceedings of the 19th European Conference on Cyber Warfare, Online, 25–26 June 2020.
- Salamah, Y.; Asyifa, R.D.; Afifah, T.Y.; Maulana, F.; Asfarian, A. Thymun: Smart mobile health platform for the autoimmune community to improve the health and well-being of autoimmune sufferers in Indonesia. In Proceedings of the 2020 8th International Conference on Information and Communication Technology (ICoICT), Yogyakarta, Indonesia, 24–26 June 2020; pp. 1–6. [ Google Scholar ]
- Stephanie, L.; Sharma, R.S. Digital health eco-systems: An epochal review of practice-oriented research. Int. J. Inf. Manag. 2020 , 53 , 102032. [ Google Scholar ] [ CrossRef ]
- Sultana, M.; Hossain, A.; Laila, F.; Taher, K.A.; Islam, M.N. Towards developing a secure medical image sharing system based on zero trust principles and blockchain technology. BMC Med. Inform. Decis. Mak. 2020 , 20 , 1–10. [ Google Scholar ] [ CrossRef ] [ PubMed ]
- Moro Visconti, R.; Morea, D. Healthcare digitalization and pay-for-performance incentives in smart hospital project financing. Int. J. Environ. Res. Public. Health 2020 , 17 , 2318. [ Google Scholar ] [ CrossRef ] [ PubMed ] [ Green Version ]
- Yousaf, K.; Mehmood, Z.; Awan, I.A.; Saba, T.; Alharbey, R.; Qadah, T.; Alrige, M.A. A comprehensive study of mobile-health based assistive technology for the healthcare of dementia and Alzheimer’s disease (AD). Health Care Manag. Sci. 2020 , 23 , 287–309. [ Google Scholar ] [ CrossRef ]
- Asthana, S.; Jones, R.; Sheaff, R. Why does the NHS struggle to adopt eHealth innovations? A review of macro, meso and micro factors. BMC Health Serv. Res. 2019 , 19 , 984. [ Google Scholar ] [ CrossRef ]
- Astruc, B. Challenges and perspectives in the private practice of psychiatry: The development of tele-psychiatry. Ann. Med. Psychol. 2019 , 177 , 67–70. [ Google Scholar ] [ CrossRef ]
- Baltaxe, E.; Czypionka, T.; Kraus, M.; Reiss, M.; Askildsen, J.E.; Grenkovic, R.; Lindén, T.S.; Pitter, J.G.; Rutten-van Molken, M.; Solans, O.; et al. Digital Health Transformation of Integrated Care in Europe: Overarching Analysis of 17 Integrated Care Programs. J. Med. Internet Res. 2019 , 21 , e14956. [ Google Scholar ] [ CrossRef ]
- Caumanns, J. For discussion: The state of digitization of the German healthcare system. Z. Evidenz Fortbild. Qual. Gesundh. 2019 , 143 , 22–29. [ Google Scholar ] [ CrossRef ]
- Diamantopoulos, S.; Karamitros, D.; Romano, L.; Coppolino, L.; Koutkias, V.; Votis, K.; Stan, O.; Campegiani, P.; Martinez, D.M.; Nalin, M. Secure Cross-Border Exchange of Health Related Data: The KONFIDO Approach ; Springer: Berlin/Heidelberg, Germany, 2019; pp. 318–327. [ Google Scholar ]
- Diviani, N.; Fredriksen, E.H.; Meppelink, C.S.; Mullan, J.; Rich, W.; Sudmann, T.T. Where else would I look for it? A five-country qualitative study on purposes, strategies, and consequences of online health information seeking. J. Public Health Res. 2019 , 8 , 1–17. [ Google Scholar ] [ CrossRef ] [ Green Version ]
- McBride, A. How Will Advances in Technology Put the Customer at the Center of Health Care? 2019. Available online: https://www.ey.com/en_gl/future-health/tech-enabled-change-health-care (accessed on 16 October 2022).
- Hatzivasilis, G.; Chatziadam, P.; Petroulakis, N.; Ioannidis, S.; Mangini, M.; Kloukinas, C.; Yautsiukhin, A.; Antoniou, M.; Katehakis, D.G.; Panayiotou, M. Cyber Insurance of Information Systems: Security and Privacy Cyber Insurance Contracts for ICT and Helathcare Organizations. In Proceedings of the 2019 IEEE 24th International Workshop on Computer Aided Modeling and Design of Communication Links and Networks (CAMAD), Limassol, Cyprus, 11–13 September 2019; IEEE: Limassol, Cyprus; pp. 1–6. [ Google Scholar ]
- Go Jefferies, J.; Bishop, S.; Hibbert, S. Customer boundary work to navigate institutional arrangements around service interactions: Exploring the case of telehealth. J. Bus. Res. 2019 , 105 , 420–433. [ Google Scholar ] [ CrossRef ]
- Kivimaa, P.; Boon, W.; Hyysalo, S.; Klerkx, L. Towards a typology of intermediaries in sustainability transitions: A systematic review and a research agenda. Res. Policy 2019 , 48 , 1062–1075. [ Google Scholar ] [ CrossRef ]
- Klocek, A.; Šmahelová, M.; Knapová, L.; Elavsky, S. GPs’ perspectives on eHealth use in the Czech Republic: A cross-sectional mixed-design survey study. BJGP Open 2019 , 3 , bjgpopen19X101655. [ Google Scholar ] [ CrossRef ]
- Kohl, S.; Schoenfelder, J.; Fügener, A.; Brunner, J.O. The use of Data Envelopment Analysis (DEA) in healthcare with a focus on hospitals. Health Care Manag. Sci. 2019 , 22 , 245–286. [ Google Scholar ] [ CrossRef ]
- Kouroubali, A.; Katehakis, D.G. The new European interoperability framework as a facilitator of digital transformation for citizen empowerment. J. Biomed. Inform. 2019 , 94 , 103166. [ Google Scholar ] [ CrossRef ]
- Manard, S.; Vergos, N.; Tamayo, S.; Fontane, F. Electronic health record in the era of industry 4.0: The French example. arXiv 2019 , arXiv:arXiv190710322. [ Google Scholar ]
- Mende, M. The innovation imperative in healthcare: An interview and commentary. AMS Rev. 2019 , 9 , 121–131. [ Google Scholar ] [ CrossRef ]
- Mishra, S.R.; Lygidakis, C.; Neupane, D.; Gyawali, B.; Uwizihiwe, J.P.; Virani, S.S.; Kallestrup, P.; Miranda, J.J. Combating non-communicable diseases: Potentials and challenges for community health workers in a digital age, a narrative review of the literature. Health Policy Plan. 2019 , 34 , 55–66. [ Google Scholar ] [ CrossRef ]
- Niemelä, R.; Pikkarainen, M.; Ervasti, M.; Reponen, J. The change of pediatric surgery practice due to the emergence of connected health technologies. Technol. Forecast. Soc. Change 2019 , 146 , 352–365. [ Google Scholar ] [ CrossRef ]
- Nittas, V.; Lun, P.; Ehrler, F.; Puhan, M.A.; Mütsch, M. Electronic Patient-Generated Health Data to Facilitate Disease Prevention and Health Promotion: Scoping Review. J. Med. Internet Res. 2019 , 21 , e13320. [ Google Scholar ] [ CrossRef ] [ Green Version ]
- Noor, A. Discovering Gaps in Saudi Education for Digital Health Transformation. Int. J. Adv. Comput. Sci. Appl. 2019 , 10 . [ Google Scholar ] [ CrossRef ] [ Green Version ]
- Pape, L.; Schneider, N.; Schleef, T.; Junius-Walker, U.; Haller, H.; Brunkhorst, R.; Hellrung, N.; Prokosch, H.U.; Haarbrandt, B.; Marschollek, M.; et al. The nephrology eHealth-system of the metropolitan region of Hannover for digitalization of care, establishment of decision support systems and analysis of health care quality. BMC Med. Inform. Decis. Mak. 2019 , 19 , 176. [ Google Scholar ] [ CrossRef ] [ PubMed ] [ Green Version ]
- Patrício, L.; Teixeira, J.G.; Vink, J. A service design approach to healthcare innovation: From decision-making to sense-making and institutional change. AMS Rev. 2019 , 9 , 115–120. [ Google Scholar ] [ CrossRef ]
- Russo Spena, T.; Cristina, M. Practising innovation in the healthcare ecosystem: The agency of third-party actors. J. Bus. Ind. Mark. 2019 , 35 , 390–403. [ Google Scholar ] [ CrossRef ]
- Rydenfält, C.; Persson, J.; Erlingsdottir, G.; Johansson, G. eHealth Services in the Near and Distant Future in Swedish Home Care Nursing. CIN Comput. Inform. Nurs. 2019 , 37 , 366–372. [ Google Scholar ] [ CrossRef ]
- Savikko, J.; Rauta, V. Implementing eHealth in Kidney Transplantation in Finland. Transplant. Proc. 2019 , 51 , 464–465. [ Google Scholar ] [ CrossRef ]
- Vial, G. Understanding digital transformation: A review and a research agenda. J. Strateg. Inf. Syst. 2019 , 28 , 118–144. [ Google Scholar ] [ CrossRef ]
- Wangdahl, J.M.; Dahlberg, K.; Jaensson, M.; Nilsson, U. Psychometric validation of Swedish and Arabic versions of two health literacy questionnaires, eHEALS and HLS-EU-Q16, for use in a Swedish context: A study protocol. BMJ Open 2019 , 9 , e029668. [ Google Scholar ] [ CrossRef ]
- Watson, H.A.; Tribe, R.M.; Shennan, A.H. The role of medical smartphone apps in clinical decision-support: A literature review. Artif. Intell. Med. 2019 , 100 , 101707. [ Google Scholar ] [ CrossRef ]
- Weigand, M.; Koester-Steinebach, I. For discussion: What potential does digitization hold for patient safety? Z. Evidenz Fortbild. Qual. Gesund. 2019 , 144 , 52–59. [ Google Scholar ] [ CrossRef ]
- Zanutto, A. ‘Two clicks and I’m in!’ Patients as co-actors in managing health data through a personal health record infrastructure. Health Inform. J. 2019 , 25 , 389–400. [ Google Scholar ] [ CrossRef ]
- Goh, W.; Jane, L.Y.W.; Aziz, A.F.A.; Phua, Y.X. CHARM: Adopting Digitalization in Community Health Assessment and Review on Mobile. In Proceedings of the 2018 Fourth International Conference on Advances in Computing, Communication & Automation (ICACCA), Subang Jaya, Malaysia, 26–28 October 2018; IEEE: Subang Jaya, Malaysia; pp. 1–6. [ Google Scholar ]
- Kayser, L.; Karnoe, A.; Furstrand, D.; Batterham, R.; Christensen, K.B.; Elsworth, G.; Osborne, R.H. A Multidimensional Tool Based on the eHealth Literacy Framework: Development and Initial Validity Testing of the eHealth Literacy Questionnaire (eHLQ). J. Med. Internet Res. 2018 , 20 , e36. [ Google Scholar ] [ CrossRef ] [ PubMed ] [ Green Version ]
- Poss-Doering, R.; Kunz, A.; Pohlmann, S.; Hofmann, H.; Kiel, M.; Winkler, E.C.; Ose, D.; Szecsenyi, J. Utilizing a Prototype Patient-Controlled Electronic Health Record in Germany: Qualitative Analysis of User-Reported Perceptions and Perspectives. JMIR Form. Res. 2018 , 2 , e10411. [ Google Scholar ] [ CrossRef ]
- Khatoon, A.; Umadevi, V. Integrating OAuth and Aadhaar with e-Health care System. In Proceedings of the 2018 3rd IEEE International Conference on Recent Trends in Electronics, Information & Communication Technology (RTEICT), Bangalore, India, 18–19 May 2018; IEEE: Bangalore, India; pp. 1681–1686. [ Google Scholar ]
- Melchiorre, M.G.; Papa, R.; Rijken, M.; van Ginneken, E.; Hujala, A.; Barbabella, F. eHealth in integrated care programs for people with multimorbidity in Europe: Insights from the ICARE4EU project. Health Policy 2018 , 122 , 53–63. [ Google Scholar ] [ CrossRef ]
- Ngwenyama, O.; Klein, S. Phronesis, argumentation and puzzle solving in IS research: Illustrating an approach to phronetic IS research practice. Eur. J. Inf. Syst. 2018 , 27 , 347–366. [ Google Scholar ] [ CrossRef ]
- Öberg, U.; Orre, C.J.; Isaksson, U.; Schimmer, R.; Larsson, H.; Hörnsten, Å. Swedish primary healthcare nurses’ perceptions of using digital eHealth services in support of patient self-management. Scand. J. Caring Sci. 2018 , 32 , 961–970. [ Google Scholar ] [ CrossRef ]
- Parkin, C.G.; Homberg, A.; Hinzmann, R. 10th Annual Symposium on Self-Monitoring of Blood Glucose, 27–29 April 2017, Warsaw, Poland. Diabetes Technol. Ther. 2018 , 20 , 68–89. [ Google Scholar ] [ CrossRef ] [ Green Version ]
- Tuzii, J. Healthcare information technology in Italy, critiques and suggestions for European digitalization. Pharm. Policy Law 2018 , 19 , 161–176. [ Google Scholar ] [ CrossRef ]
- Brockes, C.; Grischott, T.; Dutkiewicz, M.; Schmidt-Weitmann, S. Evaluation of the education “Clinical Telemedicine/e-Health” in the curriculum of medical students at the University of Zurich. Telemed. E-Health 2017 , 23 , 899–904. [ Google Scholar ] [ CrossRef ]
- Cavusoglu, L.; Demirbag-Kaplan, M. Health commodified, health communified: Navigating digital consumptionscapes of well-being. Eur. J. Mark. 2017 , 51 , 2054–2079. [ Google Scholar ] [ CrossRef ]
- Cerdan, J.; Catalan-Matamoros, D.; Berg, S.W. Online communication in a rehabilitation setting: Experiences of patients with chronic conditions using a web portal in Denmark. Patient Educ. Couns. 2017 , 100 , 2283–2289. [ Google Scholar ] [ CrossRef ]
- Coppolino, L.; D’Antonio, S.; Romano, L.; Sgaglione, L.; Staffa, M. Addressing Security Issues in the Eheatlh Domain Relying on SIEM Solutions. In Proceedings of the 2017 IEEE 41st Annual Computer Software and Applications Conference (COMPSAC), Turin, Italy, 4–8 July 2017; Volume 2, pp. 510–515. [ Google Scholar ]
- Geiger, S.; Gross, N. Does hype create irreversibilities? Affective circulation and market investments in digital health. Mark. Theory 2017 , 17 , 435–454. [ Google Scholar ] [ CrossRef ]
- Giacosa, E.; Ferraris, A.; Bresciani, S. Exploring voluntary external disclosure of intellectual capital in listed companies: An integrated intellectual capital disclosure conceptual model. J. Intellect. Cap. 2017 , 18 , 149–169. [ Google Scholar ] [ CrossRef ]
- Hong, G.E.; Lee, H.J.; Kim, J.A.; Yumnam, S.; Raha, S.; Saralamma, V.V.G.; Heo, J.D.; Lee, S.J.; Kim, E.H.; Won, C.K.; et al. Korean Byungkyul—Citrus platymamma Hort.et Tanaka flavonoids induces cell cycle arrest and apoptosis, regulating MMP protein expression in Hep3B hepatocellular carcinoma cells. Int. J. Oncol. 2017 , 50 , 575–586. [ Google Scholar ] [ CrossRef ] [ PubMed ] [ Green Version ]
- Huesers, J.; Huebner, U.; Esdar, M.; Ammenwerth, E.; Hackl, W.O.; Naumann, L.; Liebe, J.D. Innovative Power of Health Care Organisations Affects IT Adoption: A bi-National Health IT Benchmark Comparing Austria and Germany. J. Med. Syst. 2017 , 41. [ Google Scholar ] [ CrossRef ]
- Parviainen, P.; Tihinen, M. Tackling the digitalization challenge: How to benefit from digitalization in practice. IJISPM Int. J. Inf. Syst. Proj. Manag. 2017 , 63–77. [ Google Scholar ] [ CrossRef ]
- Paulin, A. Data Traffic Forecast in Health 4.0. In Health 4.0: How Virtualization and Big Data Are Revolutionizing Healthcare ; Thuemmler, C., Bai, C., Eds.; Springer International Publishing: Cham, Switzerland, 2017; pp. 39–60. [ Google Scholar ] [ CrossRef ]
- Schobel, J.; Pryss, R.; Schickler, M.; Reichert, M. Towards Patterns for Defining and Changing Data Collection Instruments in Mobile Healthcare Scenarios. In Proceedings of the 2017 IEEE 30th International Symposium on Computer-Based Medical Systems (CBMS), Thessaloniki, Greek, 24–27 June 2017; IEEE: Thessaloniki, Greek; pp. 101–102. [ Google Scholar ]
- Seddon, J.J.J.M.; Currie, W.L. Healthcare financialisation and the digital divide in the European Union: Narrative and numbers. Inf. Manag. 2017 , 54 , 1084–1096. [ Google Scholar ] [ CrossRef ]
- Thorseng, A.A.; Grisot, M. Digitalization as institutional work: A case of designing a tool for changing diabetes care. Inf. Technol. People 2017 , 30 , 227–243. [ Google Scholar ] [ CrossRef ]
- Amato, F.; Colace, F.; Greco, L.; Moscato, V.; Picariello, A. Semantic processing of multimedia data for e-government applications. J. Vis. Lang. Comput. 2016 , 32 , 35–41. [ Google Scholar ] [ CrossRef ]
- Bongaerts, R.; Henn, H.; Khare, A. The Pac-Man Principle in the Healthcare Market. In Phantom Ex Machina ; Khare, A., Stewart, B., Schatz, R., Eds.; Springer International Publishing: Cham, Swizerland, 2017; pp. 175–184. ISBN 978-3-319-44467-3. [ Google Scholar ]
- Cucciniello, M.; Lapsley, I.; Nasi, G. Managing health care in the digital world: A comparative analysis. Health Serv. Manag. Res. 2016 , 29 , 132–142. [ Google Scholar ] [ CrossRef ] [ Green Version ]
- Evans, R.S. Electronic Health Records: Then, Now, and in the Future. Yearb. Med. Inform. 2016 , 25 , S48–S61. [ Google Scholar ] [ CrossRef ]
- Faried, A.; Sutiono, A.B.; Djuwantono, T.; Arifin, M.Z.; Wirakusumah, F.F.; Yuniarto, S.A.; Hariyanto, H.; Jayadi, Y.T. Mother and children health reporting system: Innovative information system application in the rural West Bandung Area, Indonesia, by using multimodal communications systems. In Proceedings of the 2015 4th International Conference on Instrumentation, Communications, Information Technology, and Biomedical Engineering (ICICI-BME), Bandung, Indonesia, 2–3 November 2015; IEEE: Bandung, Indonesia; pp. 202–207. [ Google Scholar ]
- Harjumaa, M.; Saraniemi, S.; Pekkarinen, S.; Lappi, M.; Similä, H.; Isomursu, M. Feasibility of digital footprint data for health analytics and services: An explorative pilot study. BMC Med. Inform. Decis. Mak. 2016 , 16 , 139. [ Google Scholar ] [ CrossRef ] [ Green Version ]
- Mattsson, T. Quality Registries in Sweden, Healthcare Improvements and Elderly Persons with Cognitive Impairments. Eur. J. Health Law 2016 , 23 , 453–469. [ Google Scholar ] [ CrossRef ] [ Green Version ]
- Mazor, I.; Heart, T.; Even, A. Simulating the impact of an online digital dashboard in emergency departments on patients length of stay. J. Decis. Syst. 2016 , 25 , 343–353. [ Google Scholar ] [ CrossRef ] [ Green Version ]
- Anwar, M.; Joshi, J.; Tan, J. Anytime, anywhere access to secure, privacy-aware healthcare services: Issues, approaches and challenges. Health Policy Technol. 2015 , 4 , 299–311. [ Google Scholar ] [ CrossRef ]
- Kostkova, P. Grand Challenges in Digital Health. Front. Public Health 2015 , 3 , 134. [ Google Scholar ] [ CrossRef ] [ Green Version ]
- Laur, A. Fear of e-Health Records implementation? Med. Leg. J. 2015 , 83 , 34–39. [ Google Scholar ] [ CrossRef ]
- Sultan, N. Reflective thoughts on the potential and challenges of wearable technology for healthcare provision and medical education. Int. J. Inf. Manag. 2015 , 35 , 521–526. [ Google Scholar ] [ CrossRef ]
- Nudurupati, S.S.; Bhattacharya, A.; Lascelles, D.; Caton, N. Strategic sourcing with multi-stakeholders through value co-creation: An evidence from global health care company. Int. J. Prod. Econ. 2015 , 166 , 248–257. [ Google Scholar ] [ CrossRef ] [ Green Version ]
- Sanders, K.; Sánchez Valle, M.; Viñaras, M.; Llorente, C. Do we trust and are we empowered by “Dr. Google”? Older Spaniards’ uses and views of digital healthcare communication. Public Relat. Rev. 2015 , 41 , 794–800. [ Google Scholar ] [ CrossRef ]
- Cook, D.A.; West, C.P. Conducting systematic reviews in medical education: A stepwise approach. Med. Educ. 2012 , 46 , 943–952. [ Google Scholar ] [ CrossRef ]
- Khan, M.F.F.; Sakamura, K. Context-aware access control for clinical information systems. In Proceedings of the 2012 International Conference on Innovations in Information Technology (IIT), Abu Dhabi, United Arab Emirates, 18 March 2012; IEEE: Abu Dhabi, United Arab Emirates, 2012; pp. 123–128. [ Google Scholar ]
- Thomas, P. Bhoomi, Gyan Ganga, e-governance and the right to information: ICTs and development in India. Telemat. Inform. 2009 , 26 , 20–31. [ Google Scholar ] [ CrossRef ]
- Buccoliero, L.; Calciolari, S.; Marsilio, M. A methodological and operativeframework for the evaluation of ane-health project. Int. J. Health Plann. Manag. 2008 , 23 , 3–20. [ Google Scholar ] [ CrossRef ] [ PubMed ]
- Hikmet, N.; Bhattacherjee, A.; Menachemi, N.; Kayhan, V.O.; Brooks, R.G. The role of organizational factors in the adoption of healthcare information technology in Florida hospitals. Health Care Manag. Sci. 2008 , 11 , 1–9. [ Google Scholar ] [ CrossRef ] [ PubMed ]
- Zdravković, S. Telemedicine: Perspectives and expectations. Arch. Oncol. 2008 , 16 , 69–73. [ Google Scholar ] [ CrossRef ]
- Kitsios, F.; Kamariotou, M. Mapping new service development: A review and synthesis of literature. Serv. Ind. J. 2020 , 40 , 682–704. [ Google Scholar ] [ CrossRef ]
- Hsu, J.; Huang, J.; Kinsman, J.; Fireman, B.; Miller, R.; Selby, J.; Ortiz, E. Use of e-Health services between 1999 and 2002: A growing digital divide. J. Am. Med. Inform. Assoc. 2005 , 12 , 164–171. [ Google Scholar ] [ CrossRef ]
- Fang, Y. Visualizing the structure and the evolving of digital medicine: A scientometrics review. Scientometrics 2015 , 105 , 5–21. [ Google Scholar ] [ CrossRef ]
- Duplaga, M. The acceptance of e-health solutions among patients with chronic respiratory conditions. Telemed. E-Health 2013 , 19 , 683–691. [ Google Scholar ] [ CrossRef ] [ Green Version ]
- Chauhan, S.; Jaiswal, M. A meta-analysis of e-health applications acceptance. J. Enterp. Inf. Manag. 2017 , 30 , 295–319. [ Google Scholar ] [ CrossRef ]
- Holden, R.J.; Karsh, B.-T. The Technology Acceptance Model: Its past and its future in health care. J. Biomed. Inform. 2010 , 43 , 159–172. [ Google Scholar ] [ CrossRef ] [ Green Version ]
- WHO. A Health Telematics Policy in Support of WHO’s Health-For-All Strategy for Global Health Development, Report of the WHO Group Consultation on Health Telematics ; WHO: Geneva, Switzerland, 1997; pp. 11–16. [ Google Scholar ]
- Krupinski, E.A.; Antoniotti, N.; Bernard, J. Utilization of the American Telemedicine Association’s Clinical Practice Guidelines. Telemed. E-Health 2013 , 19 , 846–851. [ Google Scholar ] [ CrossRef ] [ Green Version ]
- Sachpazidis, I. Image and Medical Data Communication Protocols for Telemedicine and Teleradiology. Ph.D. Dissertation, Technische Universität, Berlin, Germany, 2008. [ Google Scholar ]
- Van’t Haaff, C. Virtually On-sight. Just Can. Dr. 2009 , 22 , 22. [ Google Scholar ]
- Moffatt, J.J.; Eley, D.S. Barriers to the up-take of telemedicine in Australia–a view from providers. Rural Remote Health 2011 , 11 , 116–121. [ Google Scholar ] [ CrossRef ]
- Craig, J.; Petterson, V. Introduction to the practice of telemedicine. J. Telemed. Telecare 2005 , 11 , 3–9. [ Google Scholar ] [ CrossRef ]
- Heinzelmann, P.J.; Lugn, N.E.; Kvedar, J.C. Telemedicine in the future. J. Telemed. Telecare 2005 , 11 , 384–390. [ Google Scholar ] [ CrossRef ]
- Wootton, R.; Geissbuhler, A.; Jethwani, K.; Kovarik, C.; Person, D.A.; Vladzymyrskyy, A.; Zanaboni, P.; Zolfo, M. Long-running telemedicine networks delivering humanitarian services: Experience, performance and scientific output. Bull. World Health Organ. 2012 , 90 , 341–347D. [ Google Scholar ] [ CrossRef ]
- Jennett, P.A.; Hall, L.A.; Hailey, D.; Ohinmaa, A.; Anderson, C.; Thomas, R.; Young, B.; Lorenzetti, D.; Scott, R.E. The socio-economic impact of telehealth: A systematic review. J. Telemed. Telecare 2003 , 9 , 311–320. [ Google Scholar ] [ CrossRef ]
- Wootton, R. Telemedicine support for the developing world. J. Telemed. Telecare 2008 , 14 , 109–114. [ Google Scholar ] [ CrossRef ]
- Qaddoumi, I.; Bouffet, E. Supplementation of a Successful Pediatric Neuro-Oncology Telemedicine-Based Twinning Program by E-Mails. Telemed. E-Health 2009 , 15 , 975–982. [ Google Scholar ] [ CrossRef ]
- Stanberry, B. Legal and ethical aspects of telemedicine. J. Telemed. Telecare 2006 , 12 , 166–175. [ Google Scholar ] [ CrossRef ]
Click here to enlarge figure
Reference | Keywords | Methodology | Results | |
---|---|---|---|---|
1. | Kraus, S., et al., Digital transformation in healthcare: Analyzing the current state-of-research [ ] | Digital* AND healthcare | 2 Databases (EBSCO)—130 articles (ELSEVIER Science Direct and Springer Link)—340 articles | The article assesses how multiple stakeholders implement digital technologies for management and business purposes. |
2. | Marques, Isabel C.P. and Ferreira, Joao J.M. Digital transformation in the area of health: a systematic review of 45 years of evolution. Health and Technology. 2020, 10, pp. 575–586. [ ] | Digital AND Health AND Information System AND Management AND Hospital | 1 Database (Scopus)—749 articles | Explore the potential of existing digital solutions to improve the quality and safety of healthcare and analyse the emerging trend of digital medicine. |
3. | Kolasa, K. and G. Kozinski, How to Value Digital Health Interventions? A Systematic Literature Review [ ] | Mhealth Mobile health Telemedicine Health app Wearables | 3 Databases (Pubmed, Scopus and Science Direct)—34 articles | It proposed five recommendations for the generation of evidence to be considered in developing digital health solutions and suggestions for adopting the methodological approach in DHIs’ pricing and reimbursement. |
4. | Mehdi Hosseinzadeh, Omed Hassan Ahmed, Ali Ehsani, Aram Mahmood Ahmed, Hawkar kamaran Hama. The impact of knowledge on e-health: a systematic literature review of the advanced systems [ ] | Knowledge health Knowledge e-health | 6 Databases (Google Scholar, Public Libraries, Science Direct, Springer Link, Web of Science and IEEE Xplore)—132 articles | Knowledge is considered one of the important research directions for many purposes in e-health. |
5. | Shah Nazir, Yasir Ali, Naeem Ullah and Ivan Garcia—Magarino. Internet of Things for Healthcare Using Effects of Mobile Computing: A Systematic Literature Review, Hindawi, Wireless Communications and Mobile Computing, Volume 2019. [ ] | (Internet of things OR IoT) AND (Smart hospitals) AND (Healthcare) AND (Mobile Computing) OR “Internet of things OR IoT” and “Smart hospitals” and “healthcare” and Mobile computing.” | 5 Databases (Science Direct, Springer, IEEE, Taylor and Francis, Hindawi)—116 articles | Mobile computing extends the functionality of IoT in the healthcare environment by bringing massive support in the form of mobile health (m-health). In this research, a systematic literature review protocol is proposed to study how mobile computing assists IoT applications in healthcare, contributes to the current and future research of IoT in the healthcare system, brings privacy and security to health IoT devices, and affects the IoT in the healthcare system. Furthermore, the paper intends to study the impacts of mobile computing on IoT in the healthcare environment or intelligent hospitals. |
6. | Chiranjeev Sanyal, Paul Stolee, Don Husereau. Economic evaluations of eHealth technologies: A systematic review, PLoS ONE [ ] | Assistive technology Socially assistive robots Mobile health Mobile robot Smart home system Telecare Telehealth Telemedicine Wander prevention systems Mobile locator devices Gps Location-based technology Mobile apps Mobile application Cell phone Web-based Internet M-health M-health eHealth e-health older adult elderly seniors older patient cost-effective cost-utility economic evaluation | 5 Databases (MEDLINE, EMBASE, CINAHL, NHS EED, and PsycINFO)—14 articles | E-health technologies can be used to provide resource-efficient patient-oriented care. This review identified the growing use of these technologies in managing chronic diseases in study populations, including older adults. |
7. | Kampmeijer, R., et al., The use of e-health and m-health tools in health promotion and primary prevention among older adults: a systematic literature review. [ ] | (“aged”[MeSH Terms] OR “aged”[All Fields] OR “elderly”[All Fields] OR “old”[All Fields] OR “senior”[All Fields] OR “seniors”[All Fields]) AND (“health promotion”[MeSH Terms] OR “health promotion”[All Fields] OR “promotion”[All Fields] OR “primary prevention”[MeSH Terms] OR “primary prevention”[All Fields] OR “prevention”[All Fields]) AND (“telemedicine”[MeSH Terms] OR “telemedicine”[All Fields] OR “telemedicine”[All Fields] OR “telehealth”[All Fields] OR “telehealth”[All Fields] OR “m-health”[All Fields] OR “m-health”[All Fields] OR “e-health”[All Fields] OR “e-health”[All Fields]) | 1 Database (PubMed)—45 articles | E-health and m-health tools are used by older adults in diverse health promotion programmes but also outside formal programmes to monitor and improve their health. |
8. | Iyawa, G.E., M. Herselman, and A. Botha, Digital health innovation ecosystems: From a systematic literature review to conceptual framework [ ] | Digital health Innovation Digital ecosystems | 4 Databases (ACM, Science Direct, IEEE Xplore and SpringerLink)—65 articles | The study identified components of digital health, components creation relevant to the healthcare domain, and components of digital ecosystems. |
9. | Gagnon, M.-P., et al., m-Health adoption by healthcare professionals: a systematic review. [ ] | m-Health healthcare professionals and adoption | 4 Databases (PubMed, Embase, Cinhal, PsychInfo)—33 articles | The Main perceived adoption factors to m-health at the individual, organisational, and contextual levels were the following: perceived usefulness and ease of use, design and technical concerns, cost, time, privacy and security issues, familiarity with the technology, risk-benefit assessment, and interaction with others (colleagues, patients, and management). |
10. | Leslie W., Kim, A. and D. Szeto, The evidence for the economic value of ehealth in the united states today: a systematic review. J Int Soc Telemed EHealth, 2016. [ ] | (telemedicine OR “Mobile Health” OR “Health, Mobile” OR mHealth OR mHealths OR Telehealth OR eHealth) AND (“Cost-Benefit Analysis” OR “Analyses, Cost-Benefit” OR “Analysis, Cost-Benefit” OR “Cost-Benefit Analyses” OR “Cost Benefit Analysis” OR “Analyses, Cost Benefit” OR “Analysis, Cost Benefit” OR “Cost Benefit Analyses” OR “Cost Effectiveness” OR “Effectiveness, Cost” OR “Cost-Benefit Data” OR “Cost Benefit Data” OR “Data, Cost-Benefit” OR “Cost-Utility Analysis” OR “Analyses, Cost-Utility” OR “Analysis, Cost-Utility” OR “Cost Utility Analysis” OR “Cost-Utility Analyses” OR “Economic Evaluation” OR “Economic Evaluations” OR “Evaluation, Economic” OR “Evaluations, Economic” OR “Marginal Analysis” OR “Analyses, Marginal” OR “Analysis, Marginal” OR “Marginal Analyses” OR “Cost Benefit” OR “Costs and Benefits” OR “Benefits and Costs” OR “CostEffectiveness Analysis” OR “Analysis, CostEffectiveness” OR “Cost-Effectiveness Analysis”) Virtual healthcare | 2 Databases (PubMed and The Cochrane Library) -20 articles | The goal of this study is to evaluate the published economic evidence for e-health in the United States, analyse how well it supports the growth of the current e-health environment, and suggest what evidence is needed. |
11. | Hu, Y. and G. Bai, A Systematic Literature Review of Cloud Computing in Ehealth. Health Informatics—[ ] | (Cloud) AND (eHealth OR “electronic health” OR e-health) | 5 Databases (ACM Digital Library, IEEE Xplore, Inspec, ISI Web of Science and Springer)—44 articles | With the unique superiority of the cloud in big data storage and processing ability, a hybrid cloud platform with mixed access control and security protection mechanisms will be the main research area for developing a citizen-centred home-based healthcare system. |
12. | Boonstra, A., A. Versluis, and J.F.J. Vos, Implementing electronic health records in hospitals: a systematic literature review. BMC Health Services Research, 2014. 14(1): p. 370. [ ] | “Electronic Health Record*” + implement* + hospital* “Electronic Health Record*” + implement* + “healthcare” “Electronic Health Record*” + implement* + clinic* “Electronic Patient Record*” + implment* + hospital* “Electronic Patient Record*” + implement* + “healthcare” “Electronic Patient Record*” + implement* + clinic* “Electronic Medical Record*” + implement* + hospital* “Electronic Medical Record*” + implement* + “healthcare” “Electronic Medical Record*” + implement* + clinic* “Computeri?ed Patient Record*” + implement* + hospital* “Computeri?ed Patient Record*” + implement* + “health care” “Computeri?ed Patient Record*” + implement* + clinic* “Electronic Health Care Record*” + implement* + hospital* “Electronic Health Care Record*” + implement* + “health care” “Electronic Health Care Record*” + implement* + clinic* “Computeri?ed Physician Order Entry” + implement* + hospital* “Computeri?ed Physician Order Entry” + implement* + “health care” “Computeri?ed Physician Order Entry” + implement* + clinic* | 3 Databases (Web of Knowledge, EBSCO and the Cochrane Library)—21 articles | Although EHR systems are anticipated to affect hospitals’ performance positively, their implementation is complex. |
13. | Pagliari, C., et al., What Is eHealth (4): A Scoping Exercise to Map the Field. J Med Internet Res, 2005. 7(1) [ ] | “Ehealth OR e-health OR e*health” | 8 Databases (Medline [PubMed], the Cumulative Index of Nursing and Allied Health Literature [CINAHL], the Science Citation Index [SCI], the Social Science Citation Index [SSCI], the Cochrane Library Database (including Dare, Central, NHS Economic Evaluation Database [NHS EED], Health Technology Assessment [HTA] database, NHS EED bibliographic) and Index to Scientific and Technical Proceedings (ISTP, now known as ISI Proceedings)—387 articles | Definitions of e-health vary concerning the functions, stakeholders, contexts, and theoretical issues targeted. |
Database | Search within | Keywords | No Sources | |
---|---|---|---|---|
1. | Scopus | Article title, Abstract, Keywords | (Digital transformation or digitalization) AND (Ehealth or e-health or mhealth or m-health or healthcare) AND (health economics) | 408 |
Article title, Abstract, Keywords | (Digital transformation) AND (health) | 1.152 | ||
2. | Science Direct | Article title | (Digital transformation) AND (health) | 2.142 |
3. | PubMed | Article title, Abstract | (Digital transformation or digitalization) AND (Ehealth or e-health or mhealth or m-health or healthcare) AND (health economics) | 978 |
Article title | (Digital transformation) AND (health) | 1.167 | ||
Total | 5.847 |
No. | Author | Year | Method | Sample | Data Analysis | Concepts | ||||
---|---|---|---|---|---|---|---|---|---|---|
Information Technology in Health | Education Impact of E-Health | Acceptance of E-Health | Telemedicine | Security of E-Health | ||||||
1 | Kesavadev, J, et al., [ ] | 2021 | Case Study | Χ | ||||||
2 | Attila, SZ et al., [ ] | 2021 | Survey | Χ | ||||||
3 | Malachynska, M et al., [ ] | 2021 | Case Study | Χ | ||||||
4 | Lu, WC et al., [ ] | 2021 | Survey | Χ | ||||||
5 | Burmann, A et al., [ ] | 2021 | Case Study | Χ | ||||||
6 | Bogumil-Ucan, S et al., [ ] | 2021 | Case Study | Χ | ||||||
7 | Zanutto, O [ ] | 2021 | Survey | Χ | ||||||
8 | Alauddin, MS; et al., [ ] | 2021 | Survey | Χ | ||||||
9 | Alterazi, HA [ ] | 2021 | Survey | Χ | ||||||
10 | Schmidt-Kaehler, S et al., [ ] | 2021 | Case Study | Χ | ||||||
11 | Zhao, Y et al., [ ] | 2021 | Case Study | Χ | Χ | |||||
12 | Roth, CB et al., [ ] | 2021 | Systematic Literature Review | Χ | Χ | |||||
13 | Ali, NA et al., [ ] | 2021 | Case Study | Χ | ||||||
14 | Alimbaev, A et al., [ ] | 2021 | Case Study | Χ | ||||||
15 | Dick, H et al., [ ] | 2021 | Systematic Literature Review | Χ | Χ | |||||
16 | Alt, R et al., [ ] | 2021 | Survey | a Vice President | - | Χ | ||||
17 | Bartosiewicz, A et al., [ ] | 2021 | Survey | Χ | Χ | |||||
18 | Mussener, U [ ] | 2021 | Survey | Χ | ||||||
19 | Naumann, L et al., [ ] | 2021 | Case Study | 59 qualitative telephone interviews | The findings hinted at five priorities of e-health policy making: strategy, consensus-building, decision-making, implementation and evaluation that emerged from the stakeholders’ perception of the e-health policy. | Χ | ||||
20 | Saetra, HS et al., [ ] | 2021 | Case Study | Χ | ||||||
21 | Zoltan, V et al., [ ] | 2021 | Survey | Χ | Χ | |||||
22 | Hoch, P et al., [ ] | 2021 | Survey | Χ | ||||||
23 | De Vos, J [ ] | 2021 | Survey | Χ | ||||||
24 | Beaulieu, M et al., [ ] | 2021 | Survey | Χ | ||||||
25 | Dang, TH et al., [ ] | 2021 | Survey | Χ | Χ | Χ | ||||
26 | Kraus, S et al., [ ] | 2021 | Systematic Literature Review | Χ | Χ | Χ | ||||
27 | Gauthier, P et al., [ ] | 2021 | Survey | Χ | ||||||
28 | Zhang, JS et al., [ ] | 2021 | Survey | Χ | ||||||
29 | Mallmann, CA et al., [ ] | 2021 | Survey | 513 breast cancer patients from 2012 to 2020 | Statistical analysis | Χ | ||||
30 | Fons, AQ [ ] | 2021 | Survey | Χ | ||||||
31 | Chatterjee, S et al., [ ] | 2021 | Survey | Consumers of different age groups & people working in the healthcare sector (including doctors) | Qualitative analysis | Χ | Χ | |||
32 | Wasmann, JWA et al., [ ] | 2021 | Survey | Χ | ||||||
33 | Kanungo, RP et al., [ ] | 2021 | Survey | Χ | ||||||
34 | Fernandez-Luque, L et al., [ ] | 2021 | Survey | Χ | ||||||
35 | Wilson, A et al., [ ] | 2021 | Survey | Χ | ||||||
36 | Ziadlou, D [ ] | 2021 | Survey | US health care leaders | Qualitative analysis | Χ | Χ | |||
37 | Oh, SS et al., [ ] | 2021 | Survey | Χ | Χ | |||||
38 | Knitza, J et al., [ ] | 2021 | Survey | Χ | ||||||
39 | Sergi, D et al., [ ] | 2021 | Survey | Χ | ||||||
40 | Rosalia, RA et al., [ ] | 2021 | Case Study | Χ | ||||||
41 | [Anonymous] [ ] | 2021 | Survey | Χ | ||||||
42 | Prisyazhnaya, NV et al., [ ] | 2021 | Survey | Χ | ||||||
43 | Odone, A et al. [ ] | 2021 | Case Study | Variety of participants | Qualitative and quantitative analysis | Χ | ||||
44 | Balta, M et al., [ ] | 2021 | Case Study | Χ | Χ | |||||
45 | Mues, S et al., [ ] | 2021 | Survey | Χ | ||||||
46 | Frick, NRJ et al., [ ] | 2021 | Case Study | Physicians (nine female and seven male experts) | Thematic analysis | Χ | ||||
47 | Dendere, R et al., [ ] | 2021 | Survey | Χ | ||||||
48 | Neumann, M et al., [ ] | 2021 | Survey | The dean or the most senior academic individual responsible for the medical curriculum development | Descriptive statistics in Microsoft Excel (Version 16.38) | Χ | ||||
49 | Su, Y et al., [ ] | 2021 | Case Study | Χ | ||||||
50 | Masuda, Y et al., [ ] | 2021 | Survey | Χ | ||||||
51 | Frennert, S [ ] | 2021 | Survey | Χ | Χ | |||||
52 | Hasselgren, A et al., [ ] | 2021 | Survey | Χ | Χ | |||||
53 | Kim, HK et al., [ ] | 2021 | Survey | Χ | Χ | |||||
54 | Marchant, G et al., [ ] | 2021 | Survey | 569 adults | Statistical analysis | Χ | ||||
55 | Malfatti, G et al., [ ] | 2021 | Survey | Χ | ||||||
56 | Krasuska, M et al., [ ] | 2021 | Case Study | 628 interviews, observed 190 meetings and analysed 499 documents | Thematical analysis | Χ | ||||
57 | Piccialli, F et al., [ ] | 2021 | Survey | Χ | ||||||
58 | Kyllingstad, N et al., [ ] | 2021 | Survey | Χ | ||||||
59 | Frasquilho, D et al., [ ] | 2021 | Case Study | Χ | ||||||
60 | Leone, D et al., [ ] | 2021 | Case Study | Χ | ||||||
61 | Kwon, IWG et al., [ ] | 2021 | Report | Χ | ||||||
62 | Sim, SS et al., [ ] | 2021 | Systematic Literature Review | Χ | ||||||
63 | Christie, HL et al., [ ] | 2021 | Case Study | Experts (n = 483) in the fields of e-health, dementia, and caregiving were contacted via email | Qualitative analysis | Χ | ||||
64 | Eberle, C et al., [ ] | 2021 | Survey | 2887 patients | Qualitative analysis | Χ | ||||
65 | Popkova, EG et al., [ ] | 2021 | Survey | Χ | ||||||
66 | Reich, C et al., [ ] | 2021 | Survey | Χ | ||||||
67 | Hanrieder, T et al., [ ] | 2021 | Survey | Χ | ||||||
68 | Aleksashina, AA et al., [ ] | 2021 | Survey | Χ | Χ | |||||
69 | Haase, CB et al., [ ] | 2021 | Survey | Χ | ||||||
70 | Mishra, A et al., [ ] | 2021 | Survey | Χ | ||||||
71 | Kokshagina, O [ ] | 2021 | Survey | Χ | ||||||
72 | Loch, T et al., [ ] | 2021 | Survey | Χ | ||||||
73 | Cajander, A et al., [ ] | 2021 | Survey | 17 interviews with nurses (n = 9) and physicians (n = 8) | Thematical analysis | Χ | Χ | |||
74 | Botrugno, C [ ] | 2021 | Survey | Χ | ||||||
75 | Jacquemard, T et al., [ ] | 2021 | Survey | Χ | ||||||
76 | Behnke, M et al., [ ] | 2021 | Survey | Χ | ||||||
77 | Peltoniemi, T et al., [ ] | 2021 | Case Study | Χ | ||||||
78 | Glock, H et al., [ ] | 2021 | Survey | Χ | ||||||
79 | Weitzel, EC et al., [ ] | 2021 | Survey | Χ | ||||||
80 | Sullivan, C et al., [ ] | 2021 | Case Study | Χ | ||||||
81 | Luca, MM et al., [ ] | 2021 | Survey | Χ | ||||||
82 | Negro-Calduch, E et al., [ ] | 2021 | Systematic Literature Review | Χ | ||||||
83 | Werutsky, G et al.,Denninghoff, V et al., [ ] | 2021 | Survey | Χ | ||||||
84 | Piasecki, J et al., [ ] | 2021 | Survey | Χ | Χ | |||||
85 | Broenneke, JB et al., [ ] | 2021 | Survey | Χ | ||||||
86 | Faure, S et al., [ ] | 2021 | Survey | Χ | ||||||
87 | Ghaleb, EAA et al., [ ] | 2021 | Survey | Χ | Χ | |||||
88 | Verket, M et al., [ ] | 2021 | Survey | Χ | ||||||
89 | Lenz, S [ ] | 2021 | Survey | 15 interviews with persons from different areas of digital health care | Theoretical sampling | Χ | ||||
90 | De Sutter, E et al., [ ] | 2021 | Survey | 31 healthcare professionals active | Qualitative analysis | Χ | ||||
91 | Gevko, V et al., [ ] | 2021 | Survey | Χ | ||||||
92 | El Majdoubi, D et al., [ ] | 2021 | Survey | Χ | ||||||
93 | Thakur, A et al., [ ] | 2021 | Case Study | Χ | ||||||
94 | Persson, J et al., [ ] | 2021 | Survey | Χ | ||||||
95 | Zippel-Schultz, B et al., [ ] | 2021 | Survey | 49 patients and 33 of their informal caregivers. | Qualitative analysis | Χ | ||||
96 | Lam, K et al., [ ] | 2021 | Survey | Χ | ||||||
97 | Manzeschke, A [ ] | 2021 | Survey | Χ | ||||||
98 | Dyda, A et al., [ ] | 2021 | Case Study | Χ | Χ | |||||
99 | Beckmann, M et al., [ ] | 2021 | Case Study | Variety of participants | Qualitative and quantitative analysis | Χ | ||||
100 | Numair, T et al., [ ] | 2021 | Survey | Kenya: Interviewees included nurses, community health workers, and operators hired exclusively for data entry in the WIRE system. Laos: As no operators were hired in Lao PDR, interviewees included nurses, doctors, and midwives who used the WIRE system daily. (20 healthcare workers in Kenya & Laos PDR) | Qualitative and quantitative analysis | Χ | ||||
101 | Xiroudaki, S et al., [ ] | 2021 | Case Study | Χ | ||||||
102 | Droste, W et al., [ ] | 2021 | Survey | Χ | ||||||
103 | Lee, JY et al., [ ] | 2021 | Systematic Literature Review | Χ | ||||||
104 | Giovagnoli, et al., [ ] | 2021 | Survey | Χ | ||||||
105 | Daguenet, et al., [ ] | 2021 | Survey | Χ | ||||||
106 | Hubmann, et al., [ ] | 2021 | Survey | Χ | ||||||
107 | Vikhrov, et al., [ ] | 2021 | Survey | Χ | ||||||
108 | Jahn, HK et al., [ ] | 2021 | Survey | 198 complete and 45 incomplete survey responses from physicians | Statistical analysis | Χ | ||||
109 | Low et al., [ ] | 2021 | Survey | Χ | ||||||
110 | Levasluoto, et al., [ ] | 2021 | Case Study | 23 interviews | Thematical analysis | Χ | ||||
111 | Verma, et al., [ ] | 2021 | Survey | Χ | ||||||
112 | Leung, PPL et al., [ ] | 2021 | Case Study | Χ | ||||||
113 | Weber, S et al., [ ] | 2021 | Survey | Χ | ||||||
114 | Hogervorst, S et al., [ ] | 2021 | Survey | Patients (11), group HCPs (5 + 6), interviews HCPs (4) | Thematical analysis | Χ | ||||
115 | Khan, ich et al., [ ] | 2021 | Systematic Literature Review | Χ | ||||||
116 | Cherif, et al., [ ] | 2021 | Survey | Χ | ||||||
117 | Bingham, et al., [ ] | 2021 | Survey | 19 registered nurses | Descriptive statistics | Χ | ||||
118 | Broich, et al., [ ] | 2021 | Survey | Χ | ||||||
119 | Klemme, et al., [ ] | 2021 | Survey | The study consisted of 15 semi-structured interviews with academic staff (n = 7 professors and postdoctoral researchers, three female, four male) in the field of intelligent systems and technology in healthcare and staff at practice partners (n = 8 heads of department, two female, six male) in healthcare technology and economy (a hospital, a digital innovation and engineering company and a manufacturer of household appliances) and social institutions (foundations and aid organisations for people with disabilities). | Qualitative analysis | Χ | Χ | |||
120 | Dillenseger, et al., [ ] | 2021 | Survey | Χ | ||||||
121 | Wangler, et al., [ ] | 2021 | Survey | Χ | ||||||
122 | Kuhn, et al., [ ] | 2021 | Survey | Students (35) | Qualitative analysis | Χ | ||||
123 | Aldekhyyel, et al., [ ] | 2021 | Survey | Χ | ||||||
124 | Christlein, et al., [ ] | 2021 | Survey | Χ | ||||||
125 | Bergier, et al., [ ] | 2021 | Survey | Χ | ||||||
126 | Sitges-Macia, et al., [ ] | 2021 | Survey | Χ | ||||||
127 | Rani, et al., [ ] | 2021 | Survey | Χ | ||||||
128 | Fredriksen, et al., [ ] | 2021 | Case Study | Healthcare employees from a volunteer centre and from municipality healthcare units in three municipalities | Qualitative analysis | Χ | ||||
129 | Caixeta, et al., [ ] | 2021 | Survey | Χ | ||||||
130 | Gupta, et al., [ ] | 2021 | Survey | Χ | ||||||
131 | Dobson, et al., [ ] | 2021 | Survey | Χ | ||||||
132 | Choi, K et al., [ ] | 2021 | Survey | Χ | ||||||
133 | Muller-Wirtz, et al., [ ] | 2021 | Case Study | Χ | ||||||
134 | Sembekov, et al., [ ] | 2021 | Survey | Χ | ||||||
135 | Aulenkamp, et al., [ ] | 2021 | Survey | Χ | Χ | |||||
136 | Paul, et al., [ ] | 2021 | Survey | 16 key stakeholders | Thematical analysis | Χ | ||||
137 | Lemmen, et al., [ ] | 2021 | Survey | 62 citizens and 13 patients | Qualitative analysis | Χ | ||||
138 | Golz, et al., [ ] | 2021 | Survey | Χ | ||||||
139 | Tarikere, et al., [ ] | 2021 | Survey | Χ | ||||||
140 | Li, et al., [ ] | 2021 | Case Study | Χ | ||||||
141 | Rouge-Bugat, et al., [ ] | 2021 | Case Study | Χ | ||||||
142 | Iodice, et al., [ ] | 2021 | Survey | Χ | ||||||
143 | Kulzer, B [ ] | 2021 | Survey | Χ | ||||||
144 | Khosla, et al., [ ] | 2021 | Survey | Χ | ||||||
145 | Dantas, et al., [ ] | 2021 | Survey | Χ | ||||||
146 | Gaur, et al., [ ] | 2021 | Survey | Χ | ||||||
147 | Khodadad-Saryazdi, A [ ] | 2021 | Case Study | Χ | Χ | Χ | ||||
148 | Bellavista, et al., [ ] | 2021 | Case Study | Χ | ||||||
149 | Laukka, et al., [ ] | 2021 | Case Study | Χ | Χ | |||||
150 | Singh, et al., [ ] | 2021 | Survey | Χ | ||||||
151 | Patalano, et al., [ ] | 2021 | Survey | Χ | ||||||
152 | Mantel-Teeuwisse, et al., [ ] | 2021 | Survey | Χ | ||||||
153 | Mues, et al., [ ] | 2021 | Survey | Χ | ||||||
154 | Bosch-Capblanch, et al., [ ] | 2021 | Survey | Χ | ||||||
155 | Jaboyedoff, et al., [ ] | 2021 | Survey | 336 common data elements (CDEs) | Qualitative analysis | Χ | ||||
156 | Nadhamuni, et al., [ ] | 2021 | Survey | Χ | ||||||
157 | Hertling, et al., [ ] | 2021 | Survey | Χ | ||||||
158 | Khan, et al., [ ] | 2021 | Survey | Χ | ||||||
159 | Mun, et al., [ ] | 2021 | Survey | Χ | Χ | |||||
160 | Xi, et al., [ ] | 2021 | Survey | Χ | ||||||
161 | Weichert, et al., M [ ] | 2021 | Survey | Χ | ||||||
162 | Liang, et al., [ ] | 2021 | Survey | Χ | ||||||
163 | Williams, et al., [ ] | 2021 | Survey | 508 interviews, 163 observed meetings, and analysis of 325 documents. | Qualitative analysis—Sociotechnical principles, combining deductive and inductive methods | Χ | ||||
164 | Feroz, et al., [ ] | 2021 | Case Study | Χ | ||||||
165 | Huser, et al., [ ] | 2021 | Case Study | Χ | ||||||
166 | Apostolos, K [ ] | 2021 | Survey | Χ | ||||||
167 | Simsek, et al., [ ] | 2021 | Survey | Χ | Χ | |||||
168 | Khamisy-Farah, et al., [ ] | 2021 | Survey | Χ | ||||||
169 | Egarter, et al., [ ] | 2021 | Case Study | Χ | ||||||
170 | Can, et al., [ ] | 2021 | Survey | Χ | ||||||
171 | Sung, et al., [ ] | 2021 | Survey | 278 e-logbook database entries and 379 procedures in the hospital records from 14 users were analysed. Interviews with 12 e-logbook users found overall satisfaction. | Statistical analysis | Χ | Χ | |||
172 | Zoellner, et al., [ ] | 2021 | Survey | Χ | ||||||
173 | Oliveira, et al., [ ] | 2021 | Case Study | Recipients numbering 151 (21% of the universe) completed the questionnaire: trade (49), industry (41), services (28), health (15), and education (18). | Quantitative analysis | Χ | ||||
174 | Goudarzi, et al., [ ] | 2021 | Survey | Χ | ||||||
175 | Li, et al., [ ] | 2021 | Survey | Χ | Χ | |||||
176 | Klimanov, et al., [ ] | 2021 | Case Study | Χ | ||||||
177 | Nadav, et al., [ ] | 2021 | Survey | Eight focus group interviews were conducted with 30 health and social care professionals | Qualitative analysis | Χ | ||||
178 | Spanakis, et al., [ ] | 2021 | Survey | Χ | ||||||
179 | Polyakov, et al., [ ] | 2021 | Survey | Χ | ||||||
180 | Fristedt, et al., [ ] | 2021 | Survey | Intervention group (n = 80) & control group (n = 80) | Data will be coded and manually entered in SPSS | Χ | ||||
181 | Mandal, et al., [ ] | 2021 | Survey | Χ | ||||||
182 | Ozdemir, V [ ] | 2021 | Survey | Χ | ||||||
183 | Eberle, et al., [ ] | 2021 | Survey | Χ | ||||||
184 | Iakovleva, et al., [ ] | 2021 | Case Study | Χ | ||||||
185 | von Solodkoff, et al., [ ] | 2021 | Survey | In the questionnaire, the participants (n = 217). A total of 27 subjects (mean age 51 years, min: 23 years, max: 86 years) participated in the interviews. | Statistical analysis | Χ | ||||
186 | Khuntia, et al., [ ] | 2021 | Survey | Χ | Χ | |||||
187 | Ochoa, et al., [ ] | 2021 | Survey | Χ | ||||||
188 | Masłoń-Oracz, et al., [ ] | 2021 | Case Study | X | X | |||||
189 | Abrahams, et al., [ ] | 2020 | Survey | X | X | |||||
190 | Agnihothri, et al., [ ] | 2020 | Survey | X | ||||||
191 | Bukowski, et al., [ ] | 2020 | Survey | X | X | |||||
192 | Chiang, et al., [ ] | 2020 | Survey | X | X | |||||
193 | Cobelli, et al., [ ] | 2020 | Survey | Pharmacists (82) | Qualitative content analysis | X | ||||
194 | Crawford, et al., [ ] | 2020 | Survey | X | X | |||||
195 | Gjellebæk, et al., [ ] | 2020 | Case Study | Employees and middle managers | Thematic analysis | X | ||||
196 | Nascimento, et al., [ ] | 2020 | Case Study | X | ||||||
197 | Geiger, et al., [ ] | 2020 | Case Study | Specialist in neurosurery & resident (296) | Statistical Analysis | X | X | |||
198 | Eden, et al., [ ] | 2020 | Survey | Medical, nursing, allied health, administrative and executive roles (92) | Analysis of Cohen’s kappa (k) | X | X | |||
199 | Gochhait, et al., [ ] | 2020 | Case Study | X | X | |||||
200 | Kernebeck, et al., [ ] | 2020 | Case Study | X | ||||||
201 | Klinker, et al., [ ] | 2020 | Survey | Staff of health care facilities (14) | Microsoft HoloLens, Vuzix m100 | X | ||||
202 | Krasuska, et al., M.; Williams, R.; Sheikh, A.; Franklin, B. D.; Heeney, C.; Lane, W.; Mozaffar, H.; Mason, K.; Eason, et al., [ ] | 2020 | Survey | Staff of health care facilities (113) | Qualitative analysis | X | ||||
203 | Leigh, et al., [ ] | 2020 | Survey | X | ||||||
204 | Minssen, et al., [ ] | 2020 | Survey | X | ||||||
205 | Mueller, et al., [ ] | 2020 | Case Study | Staff of health care facilities (20) | Qualitative analysis | X | X | |||
206 | Nadarzynski, et al., [ ] | 2020 | Case Study | Patients (257) | Statistical analysis | X | X | |||
207 | Pekkarinen, et al., [ ] | 2020 | Case Study | Variety of participants (24) | The analytical framework is based on Nardi and O’Day’s five components of information ecology: system, diversity, co-evolution, keystone species, and locality. | X | ||||
208 | Rajamäki, et al., [ ] | 2020 | Survey | X | ||||||
209 | Salamah, et al., [ ] | 2020 | Case Study | X | ||||||
210 | Stephanie, et al., [ ] | 2020 | Survey | X | ||||||
211 | Sultana, et al., [ ] | 2020 | Survey | X | X | |||||
212 | Visconti, et al., [ ] | 2020 | Case Study | X | ||||||
213 | Yousaf et al., [ ] | 2020 | Case Study | X | ||||||
214 | Asthana, et al., [ ] | 2019 | Survey | X | ||||||
215 | Astruc, B. [ ] | 2019 | Case Study | X | X | |||||
216 | Baltaxe, et al., [ ] | 2019 | Report | X | ||||||
217 | Caumanns, J. [ ] | 2019 | Case Study | X | ||||||
218 | Diamantopoulos, et al., [ ] | 2019 | Case Study | X | X | |||||
219 | Diviani, et al., [ ] | 2019 | Survey | Variety of participants (165) | Qualitative analysis | X | ||||
220 | EYGM [ ] | 2019 | Survey | X | ||||||
221 | Hatzivasilis, et al., [ ] | 2019 | Survey | X | ||||||
222 | Go Jefferies, et al., [ ] | 2019 | Case Study | X | X | |||||
223 | Kivimaa, P., et al., [ ] | 2019 | Systematic Literature Review | X | ||||||
224 | Klocek, A., et al., [ ] | 2019 | Case Study | Variety of people (153) | Statistical analysis | X | ||||
225 | Kohl, S., et al., [ ] | 2019 | Survey | X | ||||||
226 | Kouroubali, et al., [ ] | 2019 | Case Study | X | X | |||||
227 | Manard, et al., [ ] | 2019 | Case Study | X | ||||||
228 | Mende M. [ ] | 2019 | Survey | X | ||||||
229 | Mishra et al., [ ] | 2019 | Systematic Literature Review | X | X | X | ||||
230 | Niemelä, et al., [ ] | 2019 | Survey | Health professionals, child patients’ parents, and the healthcare industry | Systematically analysed according to the process structure (pre-, intra-, post-surgery, and home care). | X | ||||
231 | Nittas, V., et al. [ ] | 2019 | Survey | X | ||||||
232 | Noor, A. [ ] | 2019 | Case Study | Students and Staff in colleges and universities | Qualitative analysis | X | ||||
233 | Pape, L., et al. [ ] | 2019 | Case Study | X | ||||||
234 | Patrício, et al., [ ] | 2019 | Survey | X | ||||||
235 | Russo Spena, T., Cristina, M. [ ] | 2019 | Survey | X | ||||||
236 | Rydenfält, C., et al., [ ] | 2019 | Case Study | Variety of people (264) | NVivo 10 (QSR International, Melbourne, Australia) | X | ||||
237 | Savikko, et al., [ ] | 2019 | Case Study | X | ||||||
238 | Vial, G [ ] | 2019 | Systematic Literature Review | X | ||||||
239 | Wangdahl, J.M., et al., [ ] | 2019 | Case Study | Variety of people (600) | Binary logistic regression analysis | X | ||||
240 | Watson, et al., [ ] | 2019 | Systematic Literature Review | X | ||||||
241 | Weigand, et al., [ ] | 2019 | Survey | X | ||||||
242 | Zanutto, A. [ ] | 2019 | Survey | Staff of health care facilities (6836) | Qualitative analysis | X | ||||
243 | Eden, et al., [ ] | 2018 | Systematic Literature Review | X | ||||||
244 | Goh, W., et al. [ ] | 2018 | Survey | X | ||||||
245 | Kayser, L., et al., [ ] | 2018 | Survey | X | ||||||
246 | Poss-Doering, R. et al., [ ] | 2018 | Case Study | Patients (11) & Doctors (3) | Statistical analysis | X | X | X | ||
247 | Khatoon, et al., [ ] | 2018 | Survey | X | X | |||||
248 | Melchiorre, M.G., et al., [ ] | 2018 | Case Study | X | ||||||
249 | Ngwenyama, et al., [ ] | 2018 | Survey | X | ||||||
250 | Öberg, U.A.-O., et al., [ ] | 2018 | Survey | Primary healthcare nurses (20) | Qualitative analysis | X | ||||
251 | Parkin, et al., [ ] | 2018 | Report | X | ||||||
252 | Tuzii, J., [ ] | 2018 | Case Study | X | ||||||
253 | Brockes, C., et al., [ ] | 2017 | Survey | Students (28) | Mann–Whitney U-Test | X | X | |||
254 | Cavusoglu, et al., [ ] | 2017 | Survey | X | ||||||
255 | Cerdan, et al., [ ] | 2017 | Case Study | Patients (29) | Qualitative analysis | X | ||||
256 | Coppolino, et al., [ ] | 2017 | Survey | X | ||||||
257 | Geiger, et al., [ ] | 2017 | Survey | X | ||||||
258 | Giacosa, et al., [ ] | 2017 | Survey | X | ||||||
259 | Hong, et al., [ ] | 2017 | Survey | X | ||||||
260 | Hüsers, J., et al., [ ] | 2017 | Case Study | Nurses (534) | All data were analysed using R (Version 3.2.1) | X | ||||
261 | Parviainen, et al., [ ] | 2017 | Survey | X | ||||||
262 | Paulin, A. [ ] | 2017 | Survey | X | ||||||
263 | Schobel, J., et al. [ ] | 2017 | Survey | X | ||||||
264 | Seddon, et al., [ ] | 2017 | Survey | X | ||||||
265 | Thorseng, et al., [ ] | 2017 | Survey | Variety of participants | Qualitative analysis | X | ||||
266 | Tuzii, J. [ ] | 2017 | Case Study | X | ||||||
267 | Amato, F., et al., [ ] | 2016 | Survey | X | ||||||
268 | Bongaerts, et al., [ ] | 2016 | Survey | X | ||||||
269 | Cucciniello, et al., [ ] | 2016 | Survey | X | ||||||
270 | Evans, R.S. [ ] | 2016 | Survey | X | ||||||
271 | Faried, et al., [ ] | 2016 | Report | X | ||||||
272 | Harjumaa, M., et al., [ ] | 2016 | Survey | Various organisations (12) | Interview data was then analysed thematically. | X | ||||
273 | Mattsson, T., [ ] | 2016 | Case Study | X | ||||||
274 | Mazor, et al., [ ] | 2016 | Survey | X | ||||||
275 | Anwar, et al., [ ] | 2015 | Survey | X | X | |||||
276 | Kostkova, P., [ ] | 2015 | Survey | X | ||||||
277 | Laur, A., [ ] | 2015 | Survey | X | ||||||
278 | Sultan, N., [ ] | 2015 | Survey | X | X | |||||
279 | Nudurupati, et al., [ ] | 2015 | Survey | X | ||||||
280 | Sanders, K., et al., [ ] | 2015 | Survey | Healthcare professionals (17) | Qualitative analysis | X | ||||
281 | Cook, et al., [ ] | 2012 | A Systematic Literature Review | X | ||||||
282 | Khan, et al., [ ] | 2012 | Survey | X | ||||||
283 | Agarwal, R., et al., [ ] | 2010 | Survey | X | ||||||
284 | Thomas, et al., [ ] | 2009 | Case Study | X | ||||||
285 | Buccoliero, et al., [ ] | 2008 | Survey | X | ||||||
286 | Hikmet, et al., [ ] | 2008 | Case Study | Variety of participants | Quantitive analysis | X | ||||
287 | Zdravković, S. [ ] | 2008 | Survey | Χ | X |
The statements, opinions and data contained in all publications are solely those of the individual author(s) and contributor(s) and not of MDPI and/or the editor(s). MDPI and/or the editor(s) disclaim responsibility for any injury to people or property resulting from any ideas, methods, instructions or products referred to in the content. |
Share and Cite
Stoumpos, A.I.; Kitsios, F.; Talias, M.A. Digital Transformation in Healthcare: Technology Acceptance and Its Applications. Int. J. Environ. Res. Public Health 2023 , 20 , 3407. https://doi.org/10.3390/ijerph20043407
Stoumpos AI, Kitsios F, Talias MA. Digital Transformation in Healthcare: Technology Acceptance and Its Applications. International Journal of Environmental Research and Public Health . 2023; 20(4):3407. https://doi.org/10.3390/ijerph20043407
Stoumpos, Angelos I., Fotis Kitsios, and Michael A. Talias. 2023. "Digital Transformation in Healthcare: Technology Acceptance and Its Applications" International Journal of Environmental Research and Public Health 20, no. 4: 3407. https://doi.org/10.3390/ijerph20043407
Article Metrics
Article access statistics, further information, mdpi initiatives, follow mdpi.
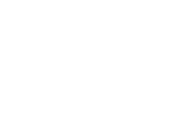
Subscribe to receive issue release notifications and newsletters from MDPI journals

Health Care Comes Home: The Human Factors (2011)
Chapter: 7 conclusions and recommendations.
7 Conclusions and Recommendations
Health care is moving into the home increasingly often and involving a mixture of people, a variety of tasks, and a broad diversity of devices and technologies; it is also occurring in a range of residential environments. The factors driving this migration include the rising costs of providing health care; the growing numbers of older adults; the increasing prevalence of chronic disease; improved survival rates of various diseases, injuries, and other conditions (including those of fragile newborns); large numbers of veterans returning from war with serious injuries; and a wide range of technological innovations. The health care that results varies considerably in its safety, effectiveness, and efficiency, as well as its quality and cost.
The committee was charged with examining this major trend in health care delivery and resulting challenges from only one of many perspectives: the study of human factors. From the outset it was clear that the dramatic and evolving change in health care practice and policies presents a broad array of opportunities and problems. Consequently the committee endeavored to maintain focus specifically on how using the human factors approach can provide solutions that support maximizing the safety and quality of health care delivered in the home while empowering both care recipients and caregivers in the effort.
The conclusions and recommendations presented below reflect the most critical steps that the committee thinks should be taken to improve the state of health care in the home, based on the literature reviewed in this report examined through a human factors lens. They are organized into four areas: (1) health care technologies, including medical devices and health information technologies involved in health care in the home; (2)
caregivers and care recipients; (3) residential environments for health care; and (4) knowledge gaps that require additional research and development. Although many issues related to home health care could not be addressed, applications of human factors principles, knowledge, and research methods in these areas could make home health care safer and more effective and also contribute to reducing costs. The committee chose not to prioritize the recommendations, as they focus on various aspects of health care in the home and are of comparable importance to the different constituencies affected.
HEALTH CARE TECHNOLOGIES
Health care technologies include medical devices that are used in the home as well as information technologies related to home-based health care. The four recommendations in this area concern (1) regulating technologies for health care consumers, (2) developing guidance on the structure and usability of health information technologies, (3) developing guidance and standards for medical device labeling, and (4) improving adverse event reporting systems for medical devices. The adoption of these recommendations would improve the usability and effectiveness of technology systems and devices, support users in understanding and learning to use them, and improve feedback to government and industry that could be used to further improve technology for home care.
Ensuring the safety of emerging technologies is a challenge, in part because it is not always clear which federal agency has regulatory authority and what regulations must be met. Currently, the U.S. Food and Drug Administration (FDA) has responsibility for devices, and the Office of the National Coordinator for Health Information Technology (ONC) has similar authority with respect to health information technology. However, the dividing line between medical devices and health information technology is blurring, and many new systems and applications are being developed that are a combination of the two, although regulatory oversight has remained divided. Because regulatory responsibility for them is unclear, these products may fall into the gap.
The committee did not find a preponderance of evidence that knowledge is lacking for the design of safe and effective devices and technologies for use in the home. Rather than discovering an inadequate evidence base, we were troubled by the insufficient attention directed at the development of devices that account, necessarily and properly, for users who are inadequately trained or not trained at all. Yet these new users often must
rely on equipment without ready knowledge about limitations, maintenance requirements, and problems with adaptation to their particular home settings.
The increased prominence of the use of technology in the health care arena poses predictable challenges for many lay users, especially people with low health literacy, cognitive impairment, or limited technology experience. For example, remote health care management may be more effective when it is supported by technology, and various electronic health care (“e-health”) applications have been developed for this purpose. With the spectrum of caregivers ranging from individuals caring for themselves or other family members to highly experienced professional caregivers, computer-based care management systems could offer varying levels of guidance, reminding, and alerting, depending on the sophistication of the operator and the criticality of the message. However, if these technologies or applications are difficult to understand or use, they may be ignored or misused, with potentially deleterious effects on care recipient health and safety. Applying existing accessibility and usability guidelines and employing user-centered design and validation methods in the development of health technology products designed for use in the home would help ensure that they are safe and effective for their targeted user populations. In this effort, it is important to recognize how the line between medical devices and health information technologies has become blurred while regulatory oversight has remained distinct, and it is not always clear into which domain a product falls.
Recommendation 1. The U.S. Food and Drug Administration and the Office of the National Coordinator for Health Information Technology should collaborate to regulate, certify, and monitor health care applications and systems that integrate medical devices and health information technologies. As part of the certification process, the agencies should require evidence that manufacturers have followed existing accessibility and usability guidelines and have applied user-centered design and validation methods during development of the product.
Guidance and Standards
Developers of information technologies related to home-based health care, as yet, have inadequate or incomplete guidance regarding product content, structure, accessibility, and usability to inform innovation or evolution of personal health records or of care recipient access to information in electronic health records.
The ONC, in the initial announcement of its health information technology certification program, stated that requirements would be forthcom-
ing with respect both to personal health records and to care recipient access to information in electronic health records (e.g., patient portals). Despite the importance of these requirements, there is still no guidance on the content of information that should be provided to patients or minimum standards for accessibility, functionality, and usability of that information in electronic or nonelectronic formats.
Consequently, some portals have been constructed based on the continuity of care record. However, recent research has shown that records and portals based on this model are neither understandable nor interpretable by laypersons, even by those with a college education. The lack of guidance in this area makes it difficult for developers of personal health records and patient portals to design systems that fully address the needs of consumers.
Recommendation 2. The Office of the National Coordinator for Health Information Technology, in collaboration with the National Institute of Standards and Technology and the Agency for Healthcare Research and Quality, should establish design guidelines and standards, based on existing accessibility and usability guidelines, for content, accessibility, functionality, and usability of consumer health information technologies related to home-based health care.
The committee found a serious lack of adequate standards and guidance for the labeling of medical devices. Furthermore, we found that the approval processes of the FDA for changing these materials are burdensome and inflexible.
Just as many medical devices currently in use by laypersons in the home were originally designed and approved for use only by professionals in formal health care facilities, the instructions for use and training materials were not designed for lay users, either. The committee recognizes that lack of instructional materials for lay users adds to the level of risk involved when devices are used by populations for whom they were not intended.
Ironically, the FDA’s current premarket review and approval processes inadvertently discourage manufacturers from selectively revising or developing supplemental instructional and training materials, when they become aware that instructional and training materials need to be developed or revised for lay users of devices already approved and marketed. Changing the instructions for use (which were approved with the device) requires manufacturers to submit the device along with revised instructions to the FDA for another 510(k) premarket notification review. Since manufacturers can find these reviews complicated, time-consuming, and expensive, this requirement serves as a disincentive to appropriate revisions of instructional or training materials.
Furthermore, little guidance is currently available on design of user
training methods and materials for medical devices. Even the recently released human factors standard on medical device design (Association for the Advancement of Medical Instrumentation, 2009), while reasonably comprehensive, does not cover the topic of training or training materials. Both FDA guidance and existing standards that do specifically address the design of labeling and ensuing instructions for use fail to account for up-to-date findings from research on instructional systems design. In addition, despite recognition that requirements for user training, training materials, and instructions for use are different for lay and professional users of medical equipment, these differences are not reflected in current standards.
Recommendation 3. The U.S. Food and Drug Administration (FDA) should promote development (by standards development organizations, such as the International Electrotechnical Commission, the International Organization for Standardization, the American National Standards Institute, and the Association for the Advancement of Medical Instrumentation) of new standards based on the most recent human factors research for the labeling of and ensuing instructional materials for medical devices designed for home use by lay users. The FDA should also tailor and streamline its approval processes to facilitate and encourage regular improvements of these materials by manufacturers.
Adverse Event Reporting Systems
The committee notes that the FDA’s adverse event reporting systems, used to report problems with medical devices, are not user-friendly, especially for lay users, who generally are not aware of the systems, unaware that they can use them to report problems, and uneducated about how to do so. In order to promote safe use of medical devices in the home and rectify design problems that put care recipients at risk, it is necessary that the FDA conduct more effective postmarket surveillance of medical devices to complement its premarket approval process. The most important elements of their primarily passive surveillance system are the current adverse event reporting mechanisms, including Maude and MedSun. Entry of incident data by health care providers and consumers is not straightforward, and the system does not elicit data that could be useful to designers as they develop updated versions of products or new ones that are similar to existing devices. The reporting systems and their importance need to be widely promoted to a broad range of users, especially lay users.
Recommendation 4. The U.S. Food and Drug Administration should improve its adverse event reporting systems to be easier to use, to collect data that are more useful for identifying the root causes of events
related to interactions with the device operator, and to develop and promote a more convenient way for lay users as well as professionals to report problems with medical devices.
CAREGIVERS IN THE HOME
Health care is provided in the home by formal caregivers (health care professionals), informal caregivers (family and friends), and individuals who self-administer care; each type of caregiver faces unique issues. Properly preparing individuals to provide care at home depends on targeting efforts appropriately to the background, experience, and knowledge of the caregivers. To date, however, home health care services suffer from being organized primarily around regulations and payments designed for inpatient or outpatient acute care settings. Little attention has been given to how different the roles are for formal caregivers when delivering services in the home or to the specific types of training necessary for appropriate, high-quality practice in this environment.
Health care administration in the home commonly involves interaction among formal caregivers and informal caregivers who share daily responsibility for a person receiving care. But few formal caregivers are given adequate training on how to work with informal caregivers and involve them effectively in health decision making, use of medical or adaptive technologies, or best practices to be used for evaluating and supporting the needs of caregivers.
It is also important to recognize that the majority of long-term care provided to older adults and individuals with disabilities relies on family members, friends, or the individual alone. Many informal caregivers take on these responsibilities without necessary education or support. These individuals may be poorly prepared and emotionally overwhelmed and, as a result, experience stress and burden that can lead to their own morbidity. The committee is aware that informational and training materials and tested programs already exist to assist informal caregivers in understanding the many details of providing health care in the home and to ease their burden and enhance the quality of life of both caregiver and care recipient. However, tested materials and education, support, and skill enhancement programs have not been adequately disseminated or integrated into standard care practices.
Recommendation 5. Relevant professional practice and advocacy groups should develop appropriate certification, credentialing, and/or training standards that will prepare formal caregivers to provide care in the home, develop appropriate informational and training materials
for informal caregivers, and provide guidance for all caregivers to work effectively with other people involved.
RESIDENTIAL ENVIRONMENTS FOR HEALTH CARE
Health care is administered in a variety of nonclinical environments, but the most common one, particularly for individuals who need the greatest level and intensity of health care services, is the home. The two recommendations in this area encourage (1) modifications to existing housing and (2) accessible and universal design of new housing. The implementation of these recommendations would be a good start on an effort to improve the safety and ease of practicing health care in the home. It could improve the health and safety of many care recipients and their caregivers and could facilitate adherence to good health maintenance and treatment practices. Ideally, improvements to housing design would take place in the context of communities that provide transportation, social networking and exercise opportunities, and access to health care and other services.
Safety and Modification of Existing Housing
The committee found poor appreciation of the importance of modifying homes to remove health hazards and barriers to self-management and health care practice and, furthermore, that financial support from federal assistance agencies for home modifications is very limited. The general connection between housing characteristics and health is well established. For example, improving housing conditions to enhance basic sanitation has long been part of a public health response to acute illness. But the characteristics of the home can present significant barriers to autonomy or self-care management and present risk factors for poor health, injury, compromised well-being, and greater dependence on others. Conversely, physical characteristics of homes can enhance resident safety and ability to participate in daily self-care and to utilize effectively health care technologies that are designed to enhance health and well-being.
Home modifications based on professional home assessments can increase functioning, contribute to reducing accidents such as falls, assist caregivers, and enable chronically ill persons and people with disabilities to stay in the community. Such changes are also associated with facilitating hospital discharges, decreasing readmissions, reducing hazards in the home, and improving care coordination. Familiar modifications include installation of such items as grab bars, handrails, stair lifts, increased lighting, and health monitoring equipment as well as reduction of such hazards as broken fixtures and others caused by insufficient home maintenance.
Deciding on which home modifications have highest priority in a given
setting depends on an appropriate assessment of circumstances and the environment. A number of home assessment instruments and programs have been validated and proven to be effective to meet this need. But even if needed modifications are properly identified and prioritized, inadequate funding, gaps in services, and lack of coordination between the health and housing service sectors have resulted in a poorly integrated system that is difficult to access. Even when accessed, progress in making home modifications available has been hampered by this lack of coordination and inadequate reimbursement or financial mechanisms, especially for those who cannot afford them.
Recommendation 6. Federal agencies, including the U.S. Department of Health and Human Services and the Centers for Medicare & Medicaid Services, along with the U.S. Department of Housing and Urban Development and the U.S. Department of Energy, should collaborate to facilitate adequate and appropriate access to health- and safety-related home modifications, especially for those who cannot afford them. The goal should be to enable persons whose homes contain obstacles, hazards, or features that pose a home safety concern, limit self-care management, or hinder the delivery of needed services to obtain home assessments, home modifications, and training in their use.
Accessibility and Universal Design of New Housing
Almost all existing housing in the United States presents problems for conducting health-related activities because physical features limit independent functioning, impede caregiving, and contribute to such accidents as falls. In spite of the fact that a large and growing number of persons, including children, adults, veterans, and older adults, have disabilities and chronic conditions, new housing continues to be built that does not account for their needs (current or future). Although existing homes can be modified to some extent to address some of the limitations, a proactive, preventive, and effective approach would be to plan to address potential problems in the design phase of new and renovated housing, before construction.
Some housing is already required to be built with basic accessibility features that facilitate practice of health care in the home as a result of the Fair Housing Act Amendments of 1998. And 17 states and 30 cities have passed what are called “visitability” codes, which currently apply to 30,000 homes. Some localities offer tax credits, such as Pittsburgh through an ordinance, to encourage installing visitability features in new and renovated housing. The policy in Pittsburgh was impetus for the Pennsylvania Residential VisitAbility Design Tax Credit Act signed into law on October 28, 2006, which offers property owners a tax credit for new construction
and rehabilitation. The Act paves the way for municipalities to provide tax credits to citizens by requiring that such governing bodies administer the tax credit (Self-Determination Housing Project of Pennsylvania, Inc., n.d.).
Visitability, rather than full accessibility, is characterized by such limited features as an accessible entry into the home, appropriately wide doorways and one accessible bathroom. Both the International Code Council, which focuses on building codes, and the American National Standards Institute, which establishes technical standards, including ones associated with accessibility, have endorsed voluntary accessibility standards. These standards facilitate more jurisdictions to pass such visitability codes and encourage legislative consistency throughout the country. To date, however, the federal government has not taken leadership to promote compliance with such standards in housing construction, even for housing for which it provides financial support.
Universal design, a broader and more comprehensive approach than visitability, is intended to suit the needs of persons of all ages, sizes, and abilities, including individuals with a wide range of health conditions and activity limitations. Steps toward universal design in renovation could include such features as anti-scald faucet valve devices, nonslip flooring, lever handles on doors, and a bedroom on the main floor. Such features can help persons and their caregivers carry out everyday tasks and reduce the incidence of serious and costly accidents (e.g., falls, burns). In the long run, implementing universal design in more homes will result in housing that suits the long-term needs of more residents, provides more housing choices for persons with chronic conditions and disabilities, and causes less forced relocation of residents to more costly settings, such as nursing homes.
Issues related to housing accessibility have been acknowledged at the federal level. For example, visitability and universal design are in accord with the objectives of the Safety of Seniors Act (Public Law No. 110-202, passed in 2008). In addition, implementation of the Olmstead decision (in which the U.S. Supreme Court ruled that the Americans with Disabilities Act may require states to provide community-based services rather than institutional placements for individuals with disabilities) requires affordable and accessible housing in the community.
Visitability, accessibility, and universal design of housing all are important to support the practice of health care in the home, but they are not broadly implemented and incentives for doing so are few.
Recommendation 7. Federal agencies, such as the U.S. Department of Housing and Urban Development, the U.S. Department of Veterans Affairs, and the Federal Housing Administration, should take a lead role, along with states and local municipalities, to develop strategies that promote and facilitate increased housing visitability, accessibil-
ity, and universal design in all segments of the market. This might include tax and other financial incentives, local zoning ordinances, model building codes, new products and designs, and related policies that are developed as appropriate with standards-setting organizations (e.g., the International Code Council, the International Electrotechnical Commission, the International Organization for Standardization, and the American National Standards Institute).
RESEARCH AND DEVELOPMENT
In our review of the research literature, the committee learned that there is ample foundational knowledge to apply a human factors lens to home health care, particularly as improvements are considered to make health care safe and effective in the home. However, much of what is known is not being translated effectively into practice, neither in design of equipment and information technology or in the effective targeting and provision of services to all those in need. Consequently, the four recommendations that follow support research and development to address knowledge and communication gaps and facilitate provision of high-quality health care in the home. Specifically, the committee recommends (1) research to enhance coordination among all the people who play a role in health care practice in the home, (2) development of a database of medical devices in order to facilitate device prescription, (3) improved surveys of the people involved in health care in the home and their residential environments, and (4) development of tools for assessing the tasks associated with home-based health care.
Health Care Teamwork and Coordination
Frail elders, adults with disabilities, disabled veterans, and children with special health care needs all require coordination of the care services that they receive in the home. Home-based health care often involves a large number of elements, including multiple care providers, support services, agencies, and complex and dynamic benefit regulations, which are rarely coordinated. However, coordinating those elements has a positive effect on care recipient outcomes and costs of care. When successful, care coordination connects caregivers, improves communication among caregivers and care recipients and ensures that receivers of care obtain appropriate services and resources.
To ensure safe, effective, and efficient care, everyone involved must collaborate as a team with shared objectives. Well-trained primary health care teams that execute customized plans of care are a key element of coordinated care; teamwork and communication among all actors are also
essential to successful care coordination and the delivery of high-quality care. Key factors that influence the smooth functioning of a team include a shared understanding of goals, common information (such as a shared medication list), knowledge of available resources, and allocation and coordination of tasks conducted by each team member.
Barriers to coordination include insufficient resources available to (a) help people who need health care at home to identify and establish connections to appropriate sources of care, (b) facilitate communication and coordination among caregivers involved in home-based health care, and (c) facilitate communication among the people receiving and the people providing health care in the home.
The application of systems analysis techniques, such as task analysis, can help identify problems in care coordination systems and identify potential intervention strategies. Human factors research in the areas of communication, cognitive aiding and decision support, high-fidelity simulation training techniques, and the integration of telehealth technologies could also inform improvements in care coordination.
Recommendation 8 . The Agency for Healthcare Research and Quality should support human factors–based research on the identified barriers to coordination of health care services delivered in the home and support user-centered development and evaluation of programs that may overcome these barriers.
Medical Device Database
It is the responsibility of physicians to prescribe medical devices, but in many cases little information is readily available to guide them in determining the best match between the devices available and a particular care recipient. No resource exists for medical devices, in contrast to the analogous situation in the area of assistive and rehabilitation technologies, for which annotated databases (such as AbleData) are available to assist the provider in determining the most appropriate one of several candidate devices for a given care recipient. Although specialists are apt to receive information about devices specific to the area of their practice, this is much less likely in the case of family and general practitioners, who often are responsible for selecting, recommending, or prescribing the most appropriate device for use at home.
Recommendation 9. The U.S. Food and Drug Administration, in collaboration with device manufacturers, should establish a medical device database for physicians and other providers, including pharmacists, to use when selecting appropriate devices to prescribe or recommend
for people receiving or self-administering health care in the home. Using task analysis and other human factors approaches to populate the medical device database will ensure that it contains information on characteristics of the devices and implications for appropriate care recipient and device operator populations.
Characterizing Caregivers, Care Recipients, and Home Environments
As delivery of health care in the home becomes more common, more coherent strategies and effective policies are needed to support the workforce of individuals who provide this care. Developing these will require a comprehensive understanding of the number and attributes of individuals engaged in health care in the home as well as the context in which care is delivered. Data and data analysis are lacking to accomplish this objective.
National data regarding the numbers of individuals engaged in health care delivery in the home—that is, both formal and informal caregivers—are sparse, and the estimates that do exist vary widely. Although the Bureau of Labor Statistics publishes estimates of the number of workers employed in the home setting for some health care classifications, they do not include all relevant health care workers. For example, data on workers employed directly by care recipients and their families are notably absent. Likewise, national estimates of the number of informal caregivers are obtained from surveys that use different methodological approaches and return significantly different results.
Although numerous national surveys have been designed to answer a broad range of questions regarding health care delivery in the home, with rare exceptions such surveys reflect the relatively limited perspective of the sponsoring agency. For example,
- The Medicare Current Beneficiary Survey (administered by the Centers for Medicare & Medicaid Services) and the Health and Retirement Survey (administered by the National Institute on Aging) are primarily geared toward understanding the health, health services use, and/or economic well-being of older adults and provide no information regarding working-age adults or children or information about home or neighborhood environments.
- The Behavioral Risk Factors Surveillance Survey (administered by the Centers for Disease Control and Prevention, CDC), the National Health Interview Survey (administered by the CDC), and the National Children’s Study (administered by the U.S. Department of Health and Human Services and the U.S. Environmental Protection Agency) all collect information on health characteristics, with limited or no information about the housing context.
- The American Housing Survey (administered by the U.S. Department of Housing and Urban Development) collects detailed information regarding housing, but it does not include questions regarding the health status of residents and does not collect adequate information about home modifications and features on an ongoing basis.
Consequently, although multiple federal agencies collect data on the sociodemographic and health characteristics of populations and on the nation’s housing stock, none of these surveys collects data necessary to link the home, its residents, and the presence of any caregivers, thus limiting understanding of health care delivered in the home. Furthermore, information is altogether lacking about health and functioning of populations linked to the physical, social, and cultural environments in which they live. Finally, in regard to individuals providing care, information is lacking regarding their education, training, competencies, and credentialing, as well as appropriate knowledge about their working conditions in the home.
Better coordination across government agencies that sponsor such surveys and more attention to information about health care that occurs in the home could greatly improve the utility of survey findings for understanding the prevalence and nature of health care delivery in the home.
Recommendation 10. Federal health agencies should coordinate data collection efforts to capture comprehensive information on elements relevant to health care in the home, either in a single survey or through effective use of common elements across surveys. The surveys should collect data on the sociodemographic and health characteristics of individuals receiving care in the home, the sociodemographic attributes of formal and informal caregivers and the nature of the caregiving they provide, and the attributes of the residential settings in which the care recipients live.
Tools for Assessing Home Health Care Tasks and Operators
Persons caring for themselves or others at home as well as formal caregivers vary considerably in their skills, abilities, attitudes, experience, and other characteristics, such as age, culture/ethnicity, and health literacy. In turn, designers of health-related devices and technology systems used in the home are often naïve about the diversity of the user population. They need high-quality information and guidance to better understand user capabilities relative to the task demands of the health-related device or technology that they are developing.
In this environment, valid and reliable tools are needed to match users with tasks and technologies. At this time, health care providers lack the
tools needed to assess whether particular individuals would be able to perform specific health care tasks at home, and medical device and system designers lack information on the demands associated with health-related tasks performed at home and the human capabilities needed to perform them successfully.
Whether used to assess the characteristics of formal or informal caregivers or persons engaged in self-care, task analysis can be used to develop point-of-care tools for use by consumers and caregivers alike in locations where such tasks are encouraged or prescribed. The tools could facilitate identification of potential mismatches between the characteristics, abilities, experiences, and attitudes that an individual brings to a task and the demands associated with the task. Used in ambulatory care settings, at hospital discharge or other transitions of care, and in the home by caregivers or individuals and family members themselves, these tools could enable assessment of prospective task performer’s capabilities in relation to the demands of the task. The tools might range in complexity from brief screening checklists for clinicians to comprehensive assessment batteries that permit nuanced study and tracking of home-based health care tasks by administrators and researchers. The results are likely to help identify types of needed interventions and support aids that would enhance the abilities of individuals to perform health care tasks in home settings safely, effectively, and efficiently.
Recommendation 11. The Agency for Healthcare Research and Quality should collaborate, as necessary, with the National Institute for Disability and Rehabilitation Research, the National Institutes of Health, the U.S. Department of Veterans Affairs, the National Science Foundation, the U.S. Department of Defense, and the Centers for Medicare & Medicaid Services to support development of assessment tools customized for home-based health care, designed to analyze the demands of tasks associated with home-based health care, the operator capabilities required to carry them out, and the relevant capabilities of specific individuals.
Association for the Advancement of Medical Instrumentation. (2009). ANSI/AAMI HE75:2009: Human factors engineering: Design of medical devices. Available: http://www.aami.org/publications/standards/HE75_Ch16_Access_Board.pdf [April 2011].
Self-Determination Housing Project of Pennsylvania, Inc. (n.d.) Promoting visitability in Pennsylvania. Available: http://www.sdhp.org/promoting_visitability_in_pennsy.htm [March 30, 2011].
In the United States, health care devices, technologies, and practices are rapidly moving into the home. The factors driving this migration include the costs of health care, the growing numbers of older adults, the increasing prevalence of chronic conditions and diseases and improved survival rates for people with those conditions and diseases, and a wide range of technological innovations. The health care that results varies considerably in its safety, effectiveness, and efficiency, as well as in its quality and cost.
Health Care Comes Home reviews the state of current knowledge and practice about many aspects of health care in residential settings and explores the short- and long-term effects of emerging trends and technologies. By evaluating existing systems, the book identifies design problems and imbalances between technological system demands and the capabilities of users. Health Care Comes Home recommends critical steps to improve health care in the home. The book's recommendations cover the regulation of health care technologies, proper training and preparation for people who provide in-home care, and how existing housing can be modified and new accessible housing can be better designed for residential health care. The book also identifies knowledge gaps in the field and how these can be addressed through research and development initiatives.
Health Care Comes Home lays the foundation for the integration of human health factors with the design and implementation of home health care devices, technologies, and practices. The book describes ways in which the Agency for Healthcare Research and Quality (AHRQ), the U.S. Food and Drug Administration (FDA), and federal housing agencies can collaborate to improve the quality of health care at home. It is also a valuable resource for residential health care providers and caregivers.
READ FREE ONLINE
Welcome to OpenBook!
You're looking at OpenBook, NAP.edu's online reading room since 1999. Based on feedback from you, our users, we've made some improvements that make it easier than ever to read thousands of publications on our website.
Do you want to take a quick tour of the OpenBook's features?
Show this book's table of contents , where you can jump to any chapter by name.
...or use these buttons to go back to the previous chapter or skip to the next one.
Jump up to the previous page or down to the next one. Also, you can type in a page number and press Enter to go directly to that page in the book.
Switch between the Original Pages , where you can read the report as it appeared in print, and Text Pages for the web version, where you can highlight and search the text.
To search the entire text of this book, type in your search term here and press Enter .
Share a link to this book page on your preferred social network or via email.
View our suggested citation for this chapter.
Ready to take your reading offline? Click here to buy this book in print or download it as a free PDF, if available.
Get Email Updates
Do you enjoy reading reports from the Academies online for free ? Sign up for email notifications and we'll let you know about new publications in your areas of interest when they're released.
Thank you for visiting nature.com. You are using a browser version with limited support for CSS. To obtain the best experience, we recommend you use a more up to date browser (or turn off compatibility mode in Internet Explorer). In the meantime, to ensure continued support, we are displaying the site without styles and JavaScript.
- View all journals
- Explore content
- About the journal
- Publish with us
- Sign up for alerts
- Open access
- Published: 26 May 2023
Artificial intelligence in healthcare and education
- Manas Dave 1 &
- Neil Patel 2
British Dental Journal volume 234 , pages 761–764 ( 2023 ) Cite this article
17k Accesses
26 Citations
12 Altmetric
Metrics details
Artificial intelligence (AI) is rapidly transforming the healthcare and medical and dental education sectors. With advancements in AI technology and its integration into routine tasks, the field of healthcare and education is rapidly evolving. This article aims to provide an in-depth analysis of the impact of AI in these sectors and to discuss the advantages and disadvantages of its integration. The article will begin by examining the use of AI in healthcare, including its impact on patient care, diagnosis and treatment, and the benefits it brings to medical professionals and patients alike. The article will then delve into the use of AI in medical and dental education, exploring its impact on student learning and teaching practices, and the benefits and challenges it presents for educators and students. Additionally, this article will also cover the impact of AI on the publishing of scientific articles in journals. With the increasing volume of submissions and the need for more efficient management, AI is being utilised to streamline the peer-review process and improve the quality of peer-review. The article will also delve into the possibility of AI enabling new forms of publication and supporting reproducibility, helping to improve the overall quality of scientific publications. Furthermore, the authors of this article have written it using AI, making it a landmark paper that showcases the true technological power of AI in the field of writing.
This article has been entirely written by the artificial intelligence (AI) software ChatGPT.
This article seeks to describe ways in which AI can be implemented in different fields of healthcare and medical and dental education.
The use of AI in publishing is discussed.
The authors comment on the newly emerging field of AI and discusses its limitations, risks and benefits.
Similar content being viewed by others
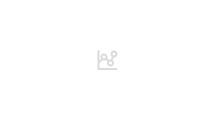

A Multimodal Generative AI Copilot for Human Pathology
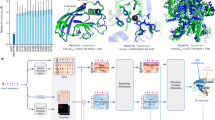
Highly accurate protein structure prediction with AlphaFold
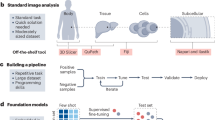
A guide to artificial intelligence for cancer researchers
Introduction.
Artificial Intelligence (AI): AI refers to the development of computer systems that can perform tasks that typically require human intelligence, such as perception, reasoning, and decision-making. In healthcare, AI is used to analyse large amounts of patient data, such as medical records, imaging studies, and laboratory results, to support clinical decision-making and improve patient outcomes. Machine Learning (ML): ML is a subfield of AI that involves the development of algorithms and models that can learn from data, without being explicitly programmed. In healthcare, ML algorithms can be trained on large datasets to identify patterns, predict outcomes, and make diagnoses. This helps doctors to make informed decisions and improve the accuracy of their diagnoses. Cloud Networks: a cloud network is a network of remote servers hosted on the internet that are used to store, process, and manage data. In healthcare, cloud networks can be used to store and access patient data, as well as to run AI and ML algorithms. This provides doctors with secure, remote access to patient information, enabling them to provide better care for their patients.
AI in healthcare
AI has the potential to bring about positive changes in healthcare and to empower patients by providing them with more control over their health. In recent years, AI has been used to improve the delivery of healthcare in a variety of ways, from providing personalized health information to enabling virtual consultations and remote monitoring.
One of the key benefits of AI in healthcare is the ability to provide personalized health information. By analysing patient data, such as medical histories and lifestyle factors, AI algorithms can provide patients with tailored recommendations for maintaining good health. This information can help patients better understand their health and make informed decisions about their care. Another important application of AI in healthcare is remote monitoring. With AI-powered remote monitoring systems, patients can have their vital signs tracked and monitored, alerting healthcare providers to any potential issues. This can lead to earlier intervention and improved patient outcomes, as well as reducing the need for in-person visits to healthcare facilities. Virtual consultations are another way in which AI is being used to improve the delivery of healthcare. By providing remote medical care, patients can receive medical treatment without having to travel to a healthcare facility. This can be especially beneficial for those who live in remote areas or who have mobility issues. Medication management is another area where AI can play an important role in empowering patients. By analysing patient data, such as prescription histories and vital signs, AI algorithms can help healthcare providers improve medication management and reduce the risk of adverse drug events. This can improve patient safety and lead to better health outcomes. Finally, AI can increase transparency in healthcare by providing patients with more information about their health and the treatments they are receiving. This can empower patients to make informed decisions about their care and help to build trust between patients and healthcare providers.
The integration of Artificial Intelligence (AI) in medical radiology has the potential to bring about a significant improvement in patient outcomes and the accuracy of diagnoses. Medical radiology plays a crucial role in the diagnosis and treatment of various medical conditions, and the use of AI has the potential to enhance this important field in a number of ways. One of the key ways that AI is being used in medical radiology is through the analysis of medical images, such as X-rays and CT scans. AI algorithms are able to analyse these images, identify abnormalities, and assist in the diagnosis of various medical conditions. This has the potential to significantly improve the speed and accuracy of diagnoses, and ultimately lead to better patient outcomes. In addition, AI algorithms can also be used to automatically detect lesions in medical images. This has the potential to significantly reduce the risk of missed diagnoses and improve patient outcomes. AI algorithms can also analyse medical images and patient data to predict the progression of diseases, such as cancer, and help develop personalized treatment plans.
Another key benefit of the use of AI in medical radiology is in the area of quality control. AI algorithms can be used to evaluate the quality of medical images and improve the accuracy of diagnoses. This has the potential to help ensure that medical images are of the highest quality, and that diagnoses are made with the utmost accuracy.
Finally, the use of AI in medical radiology also has the potential to reduce radiation exposure to patients. AI algorithms can be used to optimize imaging protocols and minimize the amount of radiation exposure that patients receive during medical imaging procedures. This has the potential to significantly improve patient safety and reduce the risk of harm.
The integration of Artificial Intelligence (AI) in diagnostic histopathology has the potential to revolutionize the medical field. The application of AI in this area has the potential to bring about significant advancements in the accuracy of diagnoses, speed up the diagnostic process, and enhance the overall patient experience.
One of the key ways in which AI is being used in diagnostic histopathology is through image analysis. AI algorithms can be utilized to analyse microscopic images of tissue samples, which can then be used to identify abnormalities and assist in the diagnosis of various medical conditions. This has the potential to greatly improve the accuracy of diagnoses and help ensure that patients receive the most effective and appropriate treatment.
Another area in which AI is being utilized in diagnostic histopathology is through automated tissue segmentation. This process involves the use of AI algorithms to automatically segment tissue samples into individual cells and structures, thereby reducing the risk of human error and improving the accuracy of diagnoses. Predictive analysis is another key area in which AI is being used in diagnostic histopathology. AI algorithms can analyse tissue samples to predict the progression of diseases such as cancer and help develop personalized treatment plans.
Quality control is also a crucial area in which AI is being utilized in diagnostic histopathology. AI algorithms can be used to evaluate the quality of tissue samples and improve the accuracy of diagnoses. This is particularly important for ensuring that patients receive the correct diagnosis and the most appropriate treatment.
Finally, AI algorithms can also be utilized to increase efficiency in diagnostic histopathology. Automating routine tasks in this area can free up pathologists to focus on complex cases and speed up the diagnostic process. This has the potential to greatly enhance the overall patient experience, ensuring that patients receive the care they need as quickly and efficiently as possible.
The application of Artificial Intelligence (AI) in the management of patient complaints has the potential to greatly enhance the hospital experience. One of the ways AI can aid in this process is through the automation of complaint management. By utilizing AI algorithms, the process of registering, categorizing, and resolving patient complaints can be streamlined, reducing the administrative burden on hospital staff and improving the overall efficiency of complaint management. Another way in which AI can help manage patient complaints is through the analysis of patient feedback data. By analysing the data, trends and patterns can be identified, allowing hospitals to pinpoint areas that require improvement and make informed decisions on how to address patient concerns. This can also contribute to an improvement in patient satisfaction by predicting which patients are most likely to make a complaint and proactively addressing their concerns. Additionally, AI can be used for predictive maintenance of medical equipment. By using AI algorithms to predict when equipment is likely to fail, hospitals can schedule maintenance in advance, reducing the number of equipment failures that lead to patient complaints and thus improving patient satisfaction.
Artificial Intelligence (AI) has the potential to play a significant role in enhancing the quality of medical care and helping doctors to reflect and learn from their mistakes. There are several ways in which AI can be utilized for this purpose.
One of the key ways that AI can help is by detecting and preventing errors in medical care. AI algorithms can be trained to analyse medical records, identifying errors or potential risks such as misdiagnoses, incorrect treatments, or adverse events. This information can be used to help doctors prevent similar errors from happening in the future. Another way AI can be used is through clinical decision support. AI algorithms can be designed to provide doctors with real-time guidance and recommendations based on patient data, helping them to make informed decisions and reducing the risk of errors. This kind of technology can greatly benefit doctors who are facing complex cases and require quick access to relevant information.
Continuous medical education is another area where AI can be of great benefit to doctors. AI algorithms can analyse medical literature and provide doctors with updates and recommendations for best practices in their field. This can help doctors stay up to date with the latest advancements in their field and continuously improve their skills. Finally, AI can play a role in quality improvement. AI algorithms can monitor and analyse the performance of healthcare providers, providing feedback and recommendations for improvement. This information can be used by doctors to reflect on their practices and identify areas for growth. In conclusion, the use of AI in medical care has the potential to enhance the quality of care, improve the learning process of doctors, and promote continuous improvement in the field.
AI in medical and dental education
Artificial Intelligence (AI) has been increasingly integrated into medical and dental education, offering numerous benefits to both students and instructors. One of the main applications of AI in this field is virtual simulation and training, allowing students to practice complex procedures on virtual patients without risking harm to real patients. This type of hands-on training is also customizable, enabling students to work at their own pace and repeat procedures until they have mastered them.
In addition to its use in simulation and training, AI also has the potential to assist medical professionals in the diagnosis and treatment of patients. AI algorithms can analyse vast amounts of medical data to identify patterns and make more accurate diagnoses. They can also assist in developing personalized treatment plans based on a patient's individual medical history and needs. AI is also being utilized in university teaching, with the potential to greatly enhance the learning experience for students and improve educational outcomes. AI algorithms can analyse student data to provide personalized learning experiences and can be used to grade assignments and create intelligent tutoring systems. AI can also be used to create virtual reality and simulation experiences, allowing students to gain hands-on experience in a controlled environment.
However, the integration of AI into medical and dental education is not without its challenges. There may be concerns about the loss of human touch and empathy in medical diagnoses and treatments, and there is a risk that students may become overly reliant on AI and neglect to develop critical thinking and problem-solving skills. Additionally, there may be challenges in ensuring the accuracy and bias-free operation of AI algorithms, which could lead to incorrect diagnoses or treatment plans.
The integration of Artificial Intelligence (AI) into dental education has shown promising results in enhancing the learning experience and improving patient care. Dental students can benefit from virtual simulations, where they can practice complex procedures such as fillings and root canals without putting real patients at risk. AI algorithms can analyse dental images and patient records to provide more accurate diagnoses and personalized treatment plans. The use of AI in dental education also includes educational games and quizzes to test students' knowledge and improve information retention. AI-powered devices can monitor oral hygiene and provide personalized recommendations for maintaining good oral health. In addition, AI-powered virtual consultations can provide remote dental care, making it easier for patients to receive treatment.
However, the integration of AI into education presents new challenges, including the potential for cheating. Students may use AI to gain an unfair advantage over their peers, undermining the credibility of the education system. Automated essay generators and online cheating tools provide students with the means to submit work they have not completed, while gaming the grading system can allow students to artificially inflate their grades. It is crucial for educational institutions to implement measures to prevent such occurrences and maintain the integrity of the educational process.
The integration of Artificial Intelligence (AI) in university medical education presents both advantages and disadvantages. In the context of exam preparation and evaluation, AI has the potential to bring objectivity, adaptability, efficiency, and reduced cost to the process. However, there are also concerns regarding the quality of AI-generated questions, unpredictability, lack of creativity, and ethical considerations. The utilization of AI algorithms in question generation can ensure fair, unbiased, and consistent evaluation of medical students' knowledge and skills. AI algorithms can also personalize exams by analysing student performance data and generating questions that focus on areas of weakness, thereby improving student learning. Additionally, AI algorithms can automate many manual processes involved in exam preparation and grading, reducing time, effort, and cost.
However, there are also concerns regarding the quality of AI-generated questions compared to those created by human examiners with years of experience and knowledge. AI algorithms may also generate questions that are too easy, too difficult, or not relevant to the course material. The lack of creativity in AI-generated questions can also result in exams that are less engaging for students.
Moreover, there are ethical considerations regarding the use of AI in exams, such as potential algorithmic bias, privacy issues, and the impact on human jobs. To address these issues, universities must carefully consider the benefits and drawbacks of AI integration and implement strict policies to ensure fair and ethical evaluation of medical students. It is also important for universities to educate students on the importance of academic integrity and ethical considerations related to AI use.
AI in publishing
Artificial Intelligence (AI) has the potential to revolutionize the publishing of scientific articles in journals. The advancements in AI technology are likely to have a significant impact on the publishing process, offering new and improved ways to manage the peer-review process, enhance the quality of peer review, and enable new forms of publication. One way in which AI is expected to affect the publishing process is by streamlining the peer-review process. With the use of AI algorithms, the publishing process can become more efficient by automating the peer-review process, thereby reducing the workload on human reviewers. This can lead to faster publication times and an improved efficiency in the publishing process. Moreover, AI has the potential to enhance the quality of peer review. AI algorithms can be employed to analyse large amounts of data and identify patterns that may be missed by human reviewers. This could result in more thorough and accurate peer review and help to identify potential biases in the review process. This is crucial in ensuring that scientific information is accurate, valid, and reliable. AI can also enable new forms of publication, such as interactive articles that incorporate multimedia and allow for more immersive experiences for readers. This provides a more engaging and accessible way for readers to consume scientific information and can help to improve the overall impact of scientific publications.
Finally, AI algorithms can play a crucial role in supporting reproducibility in scientific research. AI can be utilized to analyse and validate scientific data, helping to support the reproducibility of research. This can help to improve the overall quality of scientific publications and reduce the number of retractions due to errors or inaccuracies, thereby enhancing the credibility and reliability of scientific information.
In conclusion, the advancements in AI technology are poised to have a significant impact on the publishing of scientific articles in journals. By streamlining the peer-review process, enhancing the quality of peer review, enabling new forms of publication, and supporting reproducibility, AI has the potential to revolutionize the publishing process and improve the overall quality of scientific information.
In conclusion, the integration of Artificial Intelligence (AI) in medical and dental education has the potential to revolutionize the way in which healthcare professionals are trained. From AI-powered virtual patients for hands-on training, to AI-generated exam questions for objective assessment, the applications of AI in healthcare education are numerous and exciting. However, as with any new technology, there is a need for ongoing research and regulation to ensure that the benefits of AI are maximized, and the potential risks are minimized. One of the biggest challenges facing the use of AI in healthcare education is the need for high-quality data to train AI algorithms. As healthcare data is often complex, confidential, and subject to strict privacy regulations, it is essential that researchers and educators work closely with medical professionals, data scientists, and regulatory bodies to ensure that the data used to train AI algorithms is both accurate and ethically obtained.
As AI continues to evolve, it is likely that we will see even more exciting changes in the way in which medical and dental students are trained. With the right research and regulation, AI has the potential to help to improve the quality and efficiency of healthcare education, and to better equip future healthcare professionals with the knowledge and skills they need to provide the best possible care to their patients.
As you read through this article, it may appear as a rather a matter-of-fact series of text, devoid of creative references, metaphors or human conversation, and this is because the entire article, including the abstract, has been written by ChatGPT. 1
ChatGPT (by OpenAI in San Francisco, California) is a large language model trained by accessing a substantial number of words in a database through which it was taught the complex relationship between words to construct language. The model was trained using human AI trainers that provided the input as both the user and the AI assistant, with a reward model created for reinforcing learning. OpenAI acknowledges the limitations of ChatGPT, some of which include: the output sounding plausible but incorrect; sensitivity to changes in phrasing of input (ChatGPT may inform that it does not know the answer, but when the same question is phrased in a different way, it can answer correctly); and overuse of certain phrases (which you may have noticed in this article), among others. 2
ChatGPT is just one example of AI software that is publicly available to use for free (at the time of writing) and represents a change in access to resources we have not seen before. For the first time, you can try and explain a complex question through a conversational input and AI will be able to articulate the context and better understand what you are trying to say.
Nature reported ChatGPT gaining formal authorship on four published papers and preprints in January 2023; 3 however, it is important to remember that authors are responsible for the integrity of the content and to hold accountability for what they submit to a journal, something which AI is unable to do. ChatGPT should not be listed as an author; however, its use should be appropriately documented in the publication.
There are concerns with the use of OpenAI and cheating in exams, especially for open-book or coursework assessments. Educators need to adapt the way they assess their learners: essays that assess critical thinking (for example, student insight into management of a patient through radiographs and clinical photos) is a method ChatGPT will not be able to overcome. However, online, short-answer questions that test memory can be easily answered by ChatGPT. While the use of ChatGPT is technically use of an available online resource (a resource where its main function is not to help students cheat, such as essay mills), the concern would be in students over-relying on ChatGPT or other AI software and not developing their information-searching skills and ability to understand concepts. Finding the right answer is important, but the process of getting there is where the learning is done.
As AI will extract information from publicly available sources, it becomes difficult to reference this and there may be a risk of plagiarism. We tried asking ChatGPT to provide references to a question on gastrointestinal physiology and it returned a series of old (pre-2000) publications that were not publicly accessible - the DOIs provided were broken links. Furthermore, we have found coding in R to have some limitations, with the AI unable to bypass certain errors and mistakes when asked to construct multiple-choice questions. Fact-checking is required to ensure validity of the output. Like all systems, there are limitations; however, there are many advantages of having access to such a resource.
ChatGPT was released in November 2022 and to the current date of writing this article (March 2023), numerous scientific publications have been identified. An electronic search of online databases (Embase, Medline, Education Resources Information Center and the Cochrane Database of Systematic Reviews) identified 70 publications (correct of 11 March 2023) that included the key word 'ChatGPT'. Additionally, 25 manuscripts were found in a pre-print server. 4 With such global popularity, stakeholders across all fields will be trialling how AI can help within their own respective industries. With Microsoft and Google announcing their own AI releases in the coming future, 5 , 6 many more publications highlighting the advantages and limitations will come to light.
OpenAI. ChatGPT. Available at https://chat.openai.com/ (accessed January 2023).
OpenAI. Introducing ChatGPT. Available at https://openai.com/blog/chatgpt (accessed March 2023).
Stokel-Walker C. ChatGPT listed as author on research papers: many scientists disapprove. Nature 2023; 613: 620-621.
medRxiv. ChatGPT Search Results. Available at https://www.medrxiv.org/search/ChatGPT (accessed March 2023).
Pichai S. An important next step on our AI journey. 2023. Available at https://blog.google/technology/ai/bard-google-ai-search-updates/ (accessed March 2023).
Mehdi Y. Reinventing search with a new AI-powered Microsoft Bing and Edge, your copilot for the web. 2023. Available at https://blogs.microsoft.com/blog/2023/02/07/reinventing-search-with-a-new-ai-powered-microsoft-bing-and-edge-your-copilot-for-the-web/ (accessed March 2023).
Download references
Acknowledgements
The authors acknowledge the use of ChatGPT in this article. The main text of this article has not been copyedited to ensure authenticity of AI-generated content.
Author information
Authors and affiliations.
Lecturer in Dental Education, University of Manchester, Manchester, United Kingdom
Consultant and Senior Lecturer in Oral Surgery, University of Manchester, Manchester, United Kingdom
You can also search for this author in PubMed Google Scholar
Contributions
Manas Dave: conceptualisation, methodology, writing - original draft preparation and writing - reviewing and editing. Neil Patel: conceptualisation, methodology, writing - original draft preparation, writing - reviewing and editing and supervision.
Corresponding author
Correspondence to Manas Dave .
Ethics declarations
The authors declare no conflicts of interest.
Rights and permissions
Open Access. This article is licensed under a Creative Commons Attribution 4.0 International License, which permits use, sharing, adaptation, distribution and reproduction in any medium or format, as long as you give appropriate credit to the original author(s) and the source, provide a link to the Creative Commons licence, and indicate if changes were made. The images or other third party material in this article are included in the article's Creative Commons licence, unless indicated otherwise in a credit line to the material. If material is not included in the article's Creative Commons licence and your intended use is not permitted by statutory regulation or exceeds the permitted use, you will need to obtain permission directly from the copyright holder. To view a copy of this licence, visit http://creativecommons.org/licenses/by/4.0 . © The Author(s) 2023
Reprints and permissions
About this article
Cite this article.
Dave, M., Patel, N. Artificial intelligence in healthcare and education. Br Dent J 234 , 761–764 (2023). https://doi.org/10.1038/s41415-023-5845-2
Download citation
Received : 31 January 2023
Revised : 12 March 2023
Accepted : 04 April 2023
Published : 26 May 2023
Issue Date : 26 May 2023
DOI : https://doi.org/10.1038/s41415-023-5845-2
Share this article
Anyone you share the following link with will be able to read this content:
Sorry, a shareable link is not currently available for this article.
Provided by the Springer Nature SharedIt content-sharing initiative
This article is cited by
Perceptions of undergraduate medical students on artificial intelligence in medicine: mixed-methods survey study from palestine.
- Kamel Jebreen
- Eqbal Radwan
- Mohammed Alajez
BMC Medical Education (2024)
In-depth analysis of ChatGPT’s performance based on specific signaling words and phrases in the question stem of 2377 USMLE step 1 style questions
- Leonard Knoedler
- Samuel Knoedler
- Michael Alfertshofer
Scientific Reports (2024)
Crystal ball gazing: What does the future have in store for four elements of the profession?
- David Westgarth
BDJ In Practice (2024)
A Beginner’s Guide to Artificial Intelligence for Ophthalmologists
- Daohuan Kang
- Hongkang Wu
- Andrzej Grzybowski
Ophthalmology and Therapy (2024)
- Stephen Hancocks OBE
British Dental Journal (2023)
Quick links
- Explore articles by subject
- Guide to authors
- Editorial policies

Essay on the Impact of Technology on Health Care
Technology has grown to become an integral part of health. Healthcare organizations in different parts of the world are using technology to monitor their patients’ progress while others are using technology to store patients’ data (Bonato 37). Patient outcomes have improved due to technology, and health organizations that sought profits have significantly increased their income because of technology. It is no doubt that technology has influenced medical services in varied ways. Therefore, it would be fair to conclude that technology has positively affected healthcare.
First, technology has improved access to medical information and data (Mettler 33). One of the most significant advantages triggered by technology is the ability to store and access patient data. Medical professionals can now track patients’ progress by retrieving data from anywhere. At the same time, the internet has allowed doctors to share medical information rapidly amongst themselves, an instance that leads to more efficient patient care.
Second, technology has allowed clinicians to gather big data in a limited time (Chen et al. 72). Digital technology allows instant data collection for professionals engaged in epidemiological studies, clinical trials, and those in research. The collection of data, in this case, allows for meta-analysis and permits healthcare organizations to stay on top of cutting edge technological trends.
In addition to allowing quick access to medical data and big data technology has improved medical communication (Free et al. 54). Communication is a critical part of healthcare; nurses and doctors must communicate in real-time, and technology allows this instance to happen. Also, healthcare professionals can today make their videos, webinars and use online platforms to communicate with other professionals in different parts of the globe.
Technology has revolutionized how health care services are rendered. But apart from improving healthcare, critics argue that technology has increased or added extra jobs for medical professionals (de Belvis et al. 11). Physicians need to have excellent clinical skills and knowledge of the human body. Today, they are forced to have knowledge of both the human body and technology, which makes it challenging for others. Technology has also improved access to data, and this has allowed physicians to study and understand patients’ medical history. Nevertheless, these instances have opened doors to unethical activities such as computer hacking (de Belvis et al. 13). Today patients risk losing their medical information, including their social security numbers, address and other critical information.
Despite the improvements that have come with adopting technology, there is always the possibility that digital technological gadgets might fail. If makers of a given technology do not have a sustainable business process or a good track record, their technologies might fail. Many people, including patients and doctors who solely rely on technology, might be affected when it does. Apart from equipment failure, technology has created the space for laziness within hospitals.
Doctors and patients heavily rely on medical technology for problem-solving. In like manner, medical technologies that use machine learning have removed decision-making in different hospitals; today, medical tools are solving people’s problems. Technology has been great for our hospitals, but the speed at which different hospitals are adapting to technological processes is alarming. Technology often fails, and when it does, health care may be significantly affected. Doctors and patients who use technology may be forced to go back to traditional methods of health care services.
Bonato, P. “Advances in Wearable Technology and Its Medical Applications.” 2010 Annual International Conference of The IEEE Engineering in Medicine and Biology , 2010, pp. 33-45.
Chen, Min et al. “Disease Prediction by Machine Learning Over Big Data from Healthcare Communities.” IEEE Access , vol. 5, 2017, pp. 69-79.
De Belvis, Antonio Giulio et al. “The Financial Crisis in Italy: Implications for The Healthcare Sector.” Health Policy , vol. 106, no. 1, 2012, pp. 10-16.
Free, Caroline et al. “The Effectiveness of M-Health Technologies for Improving Health and Health Services: A Systematic Review Protocol.” BMC Research Notes , vol. 3, no. 1, 2010, pp. 42-78.
Mettler, Matthias. “Blockchain Technology in Healthcare: The Revolution Starts Here.” 2016 IEEE 18Th International Conference On E-Health Networking, Applications and Services (Healthcom) , 2016, pp. 23-78.
Cite this page
Similar essay samples.
- Essay on Private Enterprises in Regulated Markets and Free Markets
- Essay on How International Law and Diplomacy Boosts Economic Developme...
- Sign language communication across a volatile network.
- Art Analysis
- INCIDENCE RESPONSE NOVEL APPROACH TO SECURITY
- Essay on Tuan’s Artifact
Impact of Technology on Healthcare Services Analytical Essay
- To find inspiration for your paper and overcome writer’s block
- As a source of information (ensure proper referencing)
- As a template for you assignment
Introduction
Technology and the cost of health care, growth and development in the health care sector, sustainability of health technology, counter arguments, reference list.
This is a position paper based on the given topic. It contains the author’s opinion on the impact of technology on healthcare services. The paper also discusses how technology has changed the education and labor requirements for the healthcare sector.
Health care is among the sectors that lack sufficiency pertaining to adoption of technology and its utility. The turbulent healthcare market has been inundated by increased patient demands, declining margins, constant revisions of the current laws and restrictive laws.
The healthcare sector is increasingly viewing IT as a basic asset in the provision of decision support and health related services, as well as managing the increasing costs of health care. Advancement in technology has influenced the manufacture, distribution, and even administration of healthcare equipments and medicine.
The medical world is now full of machines and bioengineered equipments that require a certain degree of technological proficiency to operate. Moreover, technology has also empowered customers to seek and access their healthcare rights and information. Customers can now use current technology to assess the credentials of medical practitioners.
Negligence and errors in medical treatment that were witnessed before the inception of modern technology have reduced. With this hint in mind, there is a need for research on the impact that technological advancement has had on healthcare. Besides, there is a call for further research on how such technological changes have altered healthcare education and labor requirements in the health sector.
The paper therefore provides a framework/plan for a case study final paper on this subject with four chapters each providing a detailed scrutiny of the issue under study. Primary sources such as statistical findings on the subject will be used in the research. Archival documents such as reports, government publications, responses, and reflections on the subject from different stakeholders will also be used.
Technology has enabled healthcare professionals to treat critical diseases but at the same time the cost of such treatments has increased, making it out of reach for most of the patients. The concerned departments do provide subsidy for the costly technology but that too is for a limited period and only for select technologies.
The first chapter will involve a literature search on the impact of technological changes on the cost of health care. Owing to the adoption of new technological methods of treatment, the cost of healthcare has also risen in the past few years. In this chapter, the impact of technology on the healthcare financing will be evaluated using relevant literature, detailing the same.
Medical technology is expensive and hence today the cost of medicine and medical services has risen. Modern technology has also been credited with the current increased level of accuracy in medical treatment. Cannon (2000), in his literary work, provides details of the various ways that technology has aided in the compliance to medication for mental health patients.
Nagykaldi and Mold (2007) evaluated the role of health information technology on the translation of research into practice, and managed to capture some of the limitations, with cost being a major factor. Teich et al. (2000) also duplicated the findings, with their study showing that cost limits the adoption of basic medical technologies. According to Galas and Hood (2009), medical schools and other health institutions also find it expensive to adapt to new technologies.
This chapter will highlight some of the important milestones in the health sector in relation to health technology. Cannon and Allen (2000) state its usefulness in the medication compliance. However, with the increased medical technology development, the government has to do higher spending in the health sector (Bardhan & Thouin, 2012, 443).
The benefits of the spending will be weighed against the efficacy of the technology in this chapter. Chaudhry et al. (2006) evaluated the benefits over a ten-year period, with benefits being weighed against the costs of technology in healthcare.
The growth in the industry will be evaluated in terms of mechanization and in the solid facilities housing the machines. According to Haddad (2012), these have also seen significant developments. The study by Nagykaldi and Mold (2007) will be important in this chapter to highlight technological developments.
Impacts of the current technology on health education and labor requirements
Information technology can have a great impact on the variety of jobs and their necessity. As is understood, new technology needs appropriate knowledge. The healthcare employees who can adapt to the change will continue and those who can’t will be eliminated. New technology has even changed the approach of the patients. Patients now prefer to contact the doctors via emails.
This has reduced the regular visits by patients to healthcare centers. Obviously, this aspect has reduced the need of more healthcare employees. Cannon and Allen (2000) detail some of the changes in the industry, especially in the dispensing of drugs.
This chapter will deal with the relevance of technological advances in the health sector to the labor requirements and in health education. Modern technology and medical informatics are quickly taking over some of the traditional roles that were played by medical practitioners (Korzep, 2010, p. 354).
Despite the developments in the health technology, the medical practitioners remain the primary healthcare providers. Machines will not replace them any time soon. However, mechanization has led to the reduction in the number of personnel required to perform certain procedures as evidenced in some of the literary works to be reviewed (Nagykaldi & Mold, 2007; Haddad, 2012).
This will be discussed in this chapter in relation to the labor laws. Well-documented health records on computers are quickly replacing the period of bad handwriting of doctors (Dhillon, 2011, 397).
There have been ongoing discussions on whether the investment done by healthcare centers on new technology is necessary and whether the new technology will be able to sustain itself. It is understood that initially, such new technologies might receive subsidies from charity organizations or the government. Sustenance in healthcare means that with time any particular machinery becomes self sufficient (doesn’t need any external aid or grant).
In this chapter, an evaluation of the sustainability of the existing health technology will be evaluated. Over time, smaller, better, and more sophisticated machines have always replaced most of the technological innovations in different fields. The health industry is no exception. Researchers are always looking for better interventions (Chaudhry et al., 2006).
In this section, a review of the likely changes will be done. Some of these are available in the recommendations of the researches that will be reviewed (Cannon and Allen, 2000; Haddad, 2012). In their research, Nagykaldi and Mold (2007) state that technology is dynamic and will change in line with the prevailing innovations. The sustainability of such changes and the existing technological milestones will therefore be reviewed in this section.
It may be argued that while adoption of new technology has improved medical treatment, it has also increased the cost. Another drawback of new technology is the reduction in the job opportunities. The new technology also needs adequate training that again involves cost and time. Specialized personnel are required to handle such technology. A small mistake in handling such technology might be disastrous. So there are both pros and cons of technology.
Bardhan, Indranil, and Mark Thouin.”Health information technology and its impact on the quality and cost of healthcare delivery.” Decision Support Systems 55, no. 2 (May 2013): 438-449.
Cannon, Dale and Allen Steveb. “Comparison of the effects of computer and manual reminders on compliance with a mental health clinical practice guideline.” Journal of the American Medical Informatics Association 7, no. 2 (May 2000):196-203.
Chaudhry, Basit, Wang Jerome, Wu Shinyi, Maglione Margaret, Mojica Walter, Roth Elizabeth, Morton Sally, and Shekelle Paul. “Systematic review: impact of health information technology on quality, efficiency and cost of medical care.” Annals of Internal Medicine 12, no.144 (June 2006): 742-753.
Dhillon, Sigh. “Medical Equipment Reliability: a review, analysis methods and improvement strategies.” International Journal of Reliability, Quality & Safety Engineering 18, no. 4 (June 2011): 391-403.
Galas, David, and Leroy Hood. “Systems Biology and Emerging Technologies Will Catalyze the Transition from Reactive Medicine to Predictive, Personalized, Preventive and Participatory (P4) Medicine.” Interdisciplinary Bio Central 1, no. 1 (March 2009): 1-4.
Haddad, Tamer. “The Applicability of Total Productive Maintenance for Healthcare Facilities: an Implementation Methodology.” International Journal of Business, Humanities and Technology 2, no. 2 (March 2012): 148.
Korzep, Karen. “The future of technology and the effect it may have on replacing human jobs.” Technology & Health Care 18, no. 4/5 (August 2010): 353-358.
Nagykaldi, Zsolt, and Mold James. “The role of health information technology in the translation of research into practice: An Oklahoma Physicians Resource/Research Network (OKPRN) study.” Journal of the American Board of Family Medicine 2, no. 2 (June 2007): 188-195.
Teich, Jonathan, Merchia Pankaj, Schmiz Jennifer, Kuperman Gilad, Spurr Cynthia, and Bates, David. “Effect of computerized physician order entry on prescribing practices.” Archives of Internal Medicine 1, no. 160 (June 2000): 2741-2747.
- Advancement in Health Information Technology
- Marketing Plan: Johnson & Johnson Inc
- Cancel Culture Meaning and Role in Modern Society
- Technological Development in the Healthcare Industry
- Implementing Telemedicine Solutions: Grand Hospital
- Wireless Technology in Health Monitoring
- Investigative Report on Health IT
- How Technology Is Changing the Health Care Field
- Chicago (A-D)
- Chicago (N-B)
IvyPanda. (2019, July 9). Impact of Technology on Healthcare Services. https://ivypanda.com/essays/impact-of-technology-on-healthcare-services/
"Impact of Technology on Healthcare Services." IvyPanda , 9 July 2019, ivypanda.com/essays/impact-of-technology-on-healthcare-services/.
IvyPanda . (2019) 'Impact of Technology on Healthcare Services'. 9 July.
IvyPanda . 2019. "Impact of Technology on Healthcare Services." July 9, 2019. https://ivypanda.com/essays/impact-of-technology-on-healthcare-services/.
1. IvyPanda . "Impact of Technology on Healthcare Services." July 9, 2019. https://ivypanda.com/essays/impact-of-technology-on-healthcare-services/.
Bibliography
IvyPanda . "Impact of Technology on Healthcare Services." July 9, 2019. https://ivypanda.com/essays/impact-of-technology-on-healthcare-services/.

An official website of the United States government
The .gov means it’s official. Federal government websites often end in .gov or .mil. Before sharing sensitive information, make sure you’re on a federal government site.
The site is secure. The https:// ensures that you are connecting to the official website and that any information you provide is encrypted and transmitted securely.
- Publications
- Account settings
Preview improvements coming to the PMC website in October 2024. Learn More or Try it out now .
- Advanced Search
- Journal List
- Trans Am Clin Climatol Assoc
- v.126; 2015
Using Technology to Meet the Challenges of Medical Education
Medical education is rapidly changing, influenced by many factors including the changing health care environment, the changing role of the physician, altered societal expectations, rapidly changing medical science, and the diversity of pedagogical techniques. Changes in societal expectations put patient safety in the forefront, and raises the ethical issues of learning interactions and procedures on live patients, with the long-standing teaching method of “see one, do one, teach one” no longer acceptable. The educational goals of using technology in medical education include facilitating basic knowledge acquisition, improving decision making, enhancement of perceptual variation, improving skill coordination, practicing for rare or critical events, learning team training, and improving psychomotor skills. Different technologies can address these goals.
Technologies such as podcasts and videos with flipped classrooms, mobile devices with apps, video games, simulations (part-time trainers, integrated simulators, virtual reality), and wearable devices (google glass) are some of the techniques available to address the changing educational environment. This article presents how the use of technologies can provide the infrastructure and basis for addressing many of the challenges in providing medical education for the future.
INTRODUCTION
The use of technology in medical education has been developing over many years. The trend in the use of technology has primarily developed in response to the challenges facing medical education. These challenges to medical education are numerous ( Table 1 ). The changing healthcare environment, with the movement of medical care from the traditional hospital setting to ambulatory medicine, has necessitated the ability to provide care in a much shorter period of time and requires changes in documentation with all information, including both health knowledge and medical records, becoming digital. Emphasis on cost-containment and evidence-based use of resources is a national imperative. There are changes in societal expectations so that patient safety is a focus at all levels of medical education. This has also raised the ethical issues of learning interactions and procedures on live patients, with the long-standing teaching method of “see one, do one, teach one” no longer being acceptable.
Challenges of Medical Education
Changing healthcare environment |
Changing societal expectations |
Patient safety |
Ethics — “see one, do one, teach one” |
Changing curricular emphasis — competencies and milestones |
Explosion of medical knowledge |
Need for life-long learning |
New generation of learners |
Rapidly changing technology |
There is also the change in curricular emphasis, both in undergraduate and post-graduate training, from simple knowledge acquisition to the need to demonstrate competencies in the learner ( 1 ). The explosion of medical knowledge no longer allows physicians to keep in their mind all knowledge that is necessary to provide quality patient care. It is estimated that more than 600,000 articles are published in biomedical literature every year. If a student attempted to keep up with the literature by reading 2 articles per day, in 1 year this conscientious individual would be more than 800 years behind ( 2 ). Although the profession has long held that physicians need to be life-long learners, this concept is now an imperative. There is also a new generation of learners; “digital natives,” a phrase termed by Prensky ( 3 ). These are young people born into the digital world who speak the language of technology fluently. They expect their education to reflect their expertise in different levels of technology integration and are accustomed to technology-enhanced learning environments. Finally, medicine is experiencing a rapidly changing use of technology in the delivery of care.
The educational goals of using technology in medical education include facilitating basic knowledge acquisition, improving decision making, enhancement of perceptual variation, improving skill coordination, practicing for rare or critical events, learning team training, and improving psychomotor skills. Different technologies can address these goals. The task of medical educators is to use these new technologies effectively to transform learning into a more collaborative, personalized, and empowering experience. Bonk captures the essence of this new age of technology tools for education by stating “Anyone can learn anything from anyone at any time” ( 4 ).
TECHNOLOGY AND MEDICAL EDUCATION
There are many technologies currently being used in medical education. Although the following attempts to present these as individual approaches, the applications overlap in terms of technological components and instructional possibilities.
Computer-assisted Learning
Education of undergraduate medical students can be enhanced through the use of computer-assisted learning. One example is the use of “flipped classrooms” in which students review an online lecture before the lecture session, and come to the classroom to have an interactive session with the teacher. This time can now be spent on further exploring complex issues or discussing and solving questions in a more personalized guidance and interaction with students, instead of lecturing. Research in this area has not been extensive. Although randomized trials in education suffer due to difficulty with standardization, contamination between two arms, inability to blind the participants, and difficulty measuring outcomes, a few randomized trials have been conducted asking outcome questions about flipped classrooms with some success ( 5 , 6 ). These studies showed a positive effect in the areas of student involvement, satisfaction, and knowledge acquisition. Bridge et al conducted a 5-year retrospective study of streaming video use at Wayne State University School of Medicine and found the student response to be overwhelmingly positive, with just a small percentage of students reporting that they rarely or never used streaming video of lectures ( 6 ).
Mobile Devices
Personal digital assistants (PDAs) are routinely used by students for medical questions, patient management, and treatment decisions. Medical apps for iPhones and Android devices are numerous. Although many focus on anatomy and physiology, some address medical problem solving, diagnosis, and treatment. The website iMedicalApps.com ( 7 ) provides recommendations for the best apps for students and residents and links to online app stores for purchases. Stanford University, as one example, has a “Student App” webpage and Stanford apps that can be obtained from the Apple store. Many medical apps are also available to be used on tablets as well as phones.
Digital Games
The application of digital games for training medical professionals is on the rise. The so-called “serious” games provide training tools that provide challenging stimulating environments, and are often used for training for future surgeons ( 8 , 9 , 10 ). Use of serious games for surgical training improves eye-hand coordination and reflex times ( 10 ). At Florida State University College of Medicine, students in geriatric clerkships play ElderQuest, a role playing game in which players work to locate the Gray Sage, a powerful wizard in poor health that each player must nurse back to health ( 11 ). One published assessment of this tool was used to teach geriatric house calls to medical students. The investigators found that this method provided medical students with a fun and structured experience that had an effect not only on their learning, but also on their understanding of the particular needs of the elderly population ( 12 ).
The aim of simulation is to imitate real patients, anatomic regions, or clinical tasks, and/or mirror the real-life circumstances in which medical services are rendered. Simulations can fulfill a number of educational goals ( Table 2 ). A qualitative, systematic review by Issenberg et al, spanning 34 years and 670 peer-reviewed journal articles, found that the weight of the best available evidence suggests that high-fidelity medical simulations facilitate learning under the right conditions ( 13 ). The learning characteristics identified included providing feedback, repetitive practice, curriculum integrations, range of difficulty levels, multiple learning strategies, capture of clinical variation, individual learning, and the ability to define outcomes or benchmarks. Issenberg et al concluded that although research in this field needs improvement in terms of rigor and quality, high-fidelity medical simulations are educationally effective and simulation-based education complements medical education in patient care settings ( 13 ). Bradley has published a review on the history of simulation and Lane et al, a comprehensive review of simulation in medical education ( 14 , 15 ).
Education Goals for Simulation
Provides effective feedback |
Repetitive practice |
Range of difficulties |
Multiple learning strategies |
Capture clinical variation |
Controlled learning environment |
Individualized learning/mastery and team training |
Defined outcomes and benchmarks |
Effective method for team training |
Simulator validity |
The use of simulation spans a spectrum of sophistication, from the simple reproduction of isolated body parts through to complex human interactions portrayed by simulated patients or high-fidelity human patient simulators replicating whole body appearance and variable physiological parameters ( 14 , 15 ). One of the earliest simulators, a mannequin named Rescusi Anne, was developed 35 years ago when mouth-to-mouth resuscitation protocols were introduced ( 16 ). About the same time, Harvey, a simulator to teach cardiac examination skills, was developed and is still used worldwide in medical schools and hospitals ( 17 ).
Part-task trainers consist of 3-D representations of body parts/regions with functional anatomy for teaching and evaluating procedural or psychomotor skills, such as plastic arms for venipuncture or suturing. Palp-Sim ( 18 ) is an example of a program that uses a haptic system which provides simulation for placing a cannula in the femoral artery. Haptic systems refer to those simulators that replicate the kinesthetic and tactile perception and produce a feeling of resistance when using instruments within a simulated environment ( 14 , 19 , 20 ).
Integrated simulators combine a mannequin (usually a whole body) with sophisticated computer controls that can be manipulated to provide various physiological parameter outputs that can be physical (such as a pulse rate or respiratory movements) or electrical (presented as monitor readouts). These simulators are often used as the core platforms of simulation centers. Simulation centers attempt to replicate fully functioning operating rooms, intensive care units, emergency departments, or patient rooms ( 15 ). A well-structured case in the simulation center can teach and assess many, if not all, of the patient and process-centered skills, as well as team involvement and management.
Virtual Reality (VR) simulation refers to the recreation of environments or objects as a complex, computer-generated image. In VR simulations, the computer display simulates the physical world and user interactions are with the computer within that simulated (virtual) world. There are a number of VR programs used in medical education. One example, MIST VR (Minimally Invasive Surgery Trainer–Virtual Reality), has been specifically designed to provide trainees with a realistic and assessable environment for developing skills, particularly in the area of laparoscopy ( 21 , 22 ).
The LINDSAY Virtual Human Project, a computer-generated 3-D anatomy and physiology model, permits the user to visualize anatomy and other human components in a 3-D simulation using 2-D computer interfaces, including mobile devices, and provides an immersive approach to anatomy and physiology ( 23 , 24 ). Use of anatomy simulation models have not been well studied although computer-generated 3-D models to teach anatomy have proliferated. One randomized study by Nicholson did show that the 3-D computer-based anatomical model enhanced students' learning of anatomy of the ear ( 25 ).
Second Life is an online virtual world, developed by Linden Lab (a company based in San Francisco, CA) and launched on June 23, 2003, as of 2014 has approximately 1 million regular users. Within any Second Life simulated environment, users exist through avatars which interact realistically with other avatars online. Islands or areas of learning can be established where avatars can visit, interact with other avatars, and also interact with information provided by institutions such as the CDC, NLM, PubMed, and medical schools to mention a few ( 26 ). Second Life currently features a number of medical and health education projects ( 27 , 28 ) and educators are in the process of evaluating the value of Second Life in different aspects of medical education ( 26 , 29 , 30 ).
Wearable Technologies
Google Glass is being tested as a new layer of technology that makes education more realistic and potentially more effective. At the University of California, San Francisco (UCSF) School of Medicine, a cardiothoracic surgeon, Pierre Theodore, MD, has used Glass in more than 20 surgeries. He uses Google Glass to project radiologic images (CTs, MRIs, etc) into the field of vision as he operates to assist in cases where he can use additional clinical data to help guide activity ( 31 ).
The role of Google Glass and other devices will become commonplace across the healthcare continuum and provide an essential clinical tool, from use by the paramedic on location to advanced care and consultations ( 32 ). The University of California, Irvine School of Medicine may be the first to integrate Google Glass into the curriculum ( 33 ). Educators believe that students will benefit from Glass's unique ability to display information in a smartphone-like, hands-free format, being able to communicate with the internet via voice commands and being able to securely broadcast and record patient care and student training activities using proprietary software compliant with the 1996 federal Health Insurance Portability & Accountability Act ( 31 ).
Medical education is rapidly changing, influenced by many factors including the changing healthcare environment, the changing role of the physician, altered societal expectations, rapidly changing medical science, and the diversity of pedagogical techniques. Societal influences and the changing healthcare environment are influenced by the internet, globalization, cost containment, aging of society, increasing public accountability, a medically informed public, demands of personalized care, population diversity, expansion of healthcare delivery by non-physicians, and changing boundaries between health and healthcare. Physicians now work in teams, are salaried, part of a complex organization, and must be highly accountable. Challenges of preparing the future doctor involve emphasis and standardization of competencies and learning outcomes, integration of formal knowledge and clinical experience, patient-centered care, population health, cost-conscious−high value care, and understanding the organization of health services.
Use of technologies for undergraduate, postgraduate, and continuing medical education has become increasingly prevalent. There are a number of educational advantages that are listed in Table 3 . These modalities facilitate knowledge acquisition, improve decision making, enhance perceptual variation, improve skill coordination, and provide an educational environment that engages the learner and allows learning that does not endanger the patient. Use of computer technologies has the additional benefit of being able to assess competencies and milestones, and provide the student, at any level, with the tools to continue to access the medical knowledge necessary to deliver quality care and be a life-long learner.
Educational Advantages of Technology
Safe, controlled environments that eliminate risk to patients |
Enhanced, realistic visualization |
Authentic contexts for learning and assessment |
Documentation of learner behavior and outcomes |
Instruction tailored to individual or group needs |
Learner control of the educational experience |
Repetition and deliberate practice |
Enhance perceptual variation and improve skill coordination |
Standardization of instruction and assessment |
The use of technology in medical education should be to support learning; it should not be a replacement for face-to-face learning. Educators must still focus on the principals of teaching, not on the specific technologies. Technologies are just one tool in the educational toolbox. The task of medical educators is to use these new technologies effectively to transform learning into a more collaborative, personalized, and empowering experience. To paraphrase Confucius: “Tell me and I will forget, show me and I may remember, involve me and I will understand” ( 34 ).
Potential Conflicts of Interest: None disclosed.
Weinberger, Philadelphia: One of the things with education is the whole regulatory environment surrounding it; the LCME for medical schools, ACGME for graduate medical education, and so forth. Have they taken a stand in terms of promoting or restricting — obviously you are talking about changing a lot in the educational environment — and I am wondering what the regulatory bodies are doing in terms of incorporating this new type of technology?
Guze, Riverside: The accrediting bodies are very conservative. However, what they overwhelmingly use as outcomes are usually things like test exams. They also use student satisfaction, because they use student surveys. So far their silence has been basically that if you get to the end and can demonstrate competence in whatever you do then they have been accepting of it.
Zeidel, Boston: We have actually developed at our place simulations for physiology which make the physiology dynamic rather than static. Of course, this seems like a great idea. I would urge that as we apply these technologies that we actually develop the ability to test whether they work. There is an awful lot that has gone on in education since Flexner, actually where we change the way we educate, and we don't measure whether it makes any difference. All of us who have lived in suburbs have recognized that every 5 years there is a new math curriculum. We have no idea whether it is any better than the old math curriculum, but everyone has to learn it. I would urge that we try with the developing field of measuring efficacy in educational interventions to measure whether these things improve things or not, so that we can try to be a bit rigorous about what we are doing. Of course, all the medical schools in the country are probably going to flip their curriculum in the next several years, and probably I fear there will be no effort to figure out if it is any better than what we were doing before.
Guze, Riverside: It's a very good point. Fortunately or unfortunately, in this area the major research is really qualitative research and not quantitative research. There have been a few studies that have looked at exam rates and shown that students who are more actively involved, through using some simulation technologies, have done better.
Katz, Boston: My comment has to do with ultimately the binary characteristics of technology, which is, there is a right answer and there is a wrong answer in general. Just this week, I heard that there were complaints about our otherwise very good co-training simulation session. That there was sort of always a right answer, but they rarely got beyond that. They very rarely got into the variability of the real situations. It gets to this issue of sort of ambiguity; the value of being able to hold multiple right answers at the same time without an exact correct answer. That's one of my concerns about some of this technology. Although I readily acknowledge the many, many advantages. I was wondering if you could talk about that. How does this affect the learners ability to be open minded in a situation where there is often time a right or wrong answer?
Guze, Riverside: I don't think this is a substitute for a certain amount of face-to-face interaction that has to go on. There is the art of medicine that we can't ever, from my perspective, leave behind. These are tools that actually get a student, I believe, more actively involved. But without the modeling I think by the physicians who apply the art and are able to say, “This may be right in this individual but may not be right in others,” we then would be missing an important component of education. I think what the technology does is take advantage of the fact that as adults we do much better if we are actively involved in doing something.
Bradsher, Little Rock: When my son graduated from medical school a few years ago, he was distraught that his intern coat wouldn't fit his IPad, so my communal wife, who is home economics major, took one of my coats and harvested a pocket and put inside his coat an iPad pocket. He showed it to his intern mates who said, “You are such a nerd.” Then about 20 minutes later they said, “Can we get one of those?”

- Advanced Search
Browse Theses
January 2012
- State University of New York at Buffalo
- Computer Science Department 226 Bell Hall Buffalo, NY
- United States

Information technology has been transforming health care industry. This dissertation investigates the use of health information technology by health care providers and patients as well as its outcomes. This dissertation consists of three essays studying workflow optimization in hospital emergency departments, people's search for online health information, and the relationships between EMR (Electronic Medical Records) usage and health care outcomes, respectively.
Hospital emergency departments' capacities to deal with a patient surge play an important role in preparedness for natural or man-made disasters. The first essay examines how emergency departments could optimize workflows during extreme events when there is a patient surge. This essay proposes a framework to reconfigure workflows while maintaining treatment quality. Our results show that reorganizing lower-priority processes and relocating the resources associated with those processes can shorten total waiting time in emergency departments, allowing better management of patient flows.
People are increasingly using the Internet to access health information and the information obtained has an impact on their health care outcomes. The second essay examines the impacts of IT enablers and health motivators on people's online health information search behaviors. We characterize users' online health information search behaviors along three dimensions: the frequency of online health information search, the diversity of online health information usage, and the preference of the Internet for initial search. Using the 2003 Health Information National Trends Survey (HINTS) data on cancers, we find that ease of access to Internet and trust in online health information could affect all three dimensions of search behaviors. While perceived quality of communication with doctors has an impact on diversity of search and preference of search, we surprisingly do not find an impact on the frequency of search for online health information. In addition, our results find that perceived health status could affect both frequency and diversity of search for online health information. But we do not find evidence that perceived health status could lead to a preference for using the Internet as a source for health information.
The US government has initiated incentive programs to encourage the adoption of Electronic Medical Records (EMR). To qualify for the incentive payment, health care providers need to demonstrate "meaningful use" of EMR systems, which requires the use of certified EMRs and the implementation of a set of standard functionalities. In the third essay, we examine how the meaningful use of EMRs would affect health care outcomes in outpatient settings. Our results show that the use of core functionalities required by "meaningful use" criteria and the use of certified EMRs have a positive impact on the quality and efficiency of care. In addition, we find the relationship between the "meaningful use" and quality of care is moderated by the length of EMR use.
Save to Binder
- Publication Years 1990 - 2023
- Publication counts 121
- Citation count 2,894
- Available for Download 36
- Downloads (cumulative) 86,668
- Downloads (12 months) 5,786
- Downloads (6 weeks) 922
- Average Downloads per Article 2,407
- Average Citation per Article 24
- Publication Years 1998 - 2024
- Publication counts 44
- Citation count 466
- Available for Download 7
- Downloads (cumulative) 13,190
- Downloads (12 months) 619
- Downloads (6 weeks) 110
- Average Downloads per Article 1,884
- Average Citation per Article 11
- Publication Years 2007 - 2015
- Publication counts 8
- Citation count 54
- Available for Download 2
- Downloads (cumulative) 1,158
- Downloads (12 months) 29
- Downloads (6 weeks) 1
- Average Downloads per Article 579
- Average Citation per Article 7
Recommendations
Obesity in health care: perceptions of health care providers and patients at an academic medical center, essays in health economics, investigating primary health care nurses' intention to use information technology: an empirical study in taiwan.
Information technology (IT) has become a significant part of providing consistent care quality. The applications of the primary health information system (PHIS) in primary health care have rapidly influenced the care service delivery. Improved ...
Export Citations
- Please download or close your previous search result export first before starting a new bulk export. Preview is not available. By clicking download, a status dialog will open to start the export process. The process may take a few minutes but once it finishes a file will be downloadable from your browser. You may continue to browse the DL while the export process is in progress. Download
- Download citation
- Copy citation
We are preparing your search results for download ...
We will inform you here when the file is ready.
Your file of search results citations is now ready.
Your search export query has expired. Please try again.

IMAGES
VIDEO
COMMENTS
Abstract. The COVID-19 pandemic has sparked radical shifts in the ways that both health care and health professions education are delivered. Before the pandemic, some degree programs were offered fully online or in a hybrid format, but in-person learning was considered essential to the education and training of health professionals.
Selected technologies: benefits and challenges. The nursing literature contains many analyses of digital technologies used to support or extend the profession, including practice (eg, hospital information systems, electronic health records, monitoring systems, decision support, telehealth); education (eg, e-Learning, virtual reality, serious games); and, rehabilitative and personalized ...
With advancements in AI technology and its integration into routine tasks, the field of healthcare and education is rapidly evolving. ... undermining the credibility of the education system. Automated essay generators and online cheating tools provide students with the means to submit work they have not completed, while gaming the grading ...
Technology in Society, Healthcare and Education Essay. Our lives depend on technology. In recent years, technology became introduced to almost every part of our society. The proliferation of personal computers, electronic systems, and smartphones changed the way people interact with the world and led to profound changes in human behavior.
According to the Higher Education Funding Council for England (HEFCE, 2009), the benefits. of using technology in education can be identified at three levels - efficiency, enhancement and ...
The theme of the series aligns with one of the medical education priorities of Jessica Illuzzi, MD, MS, deputy dean for education and Harold W. Jockers Professor of Medical Education—to develop innovative approaches to medical education that are irrevocably engaging and compelling, enhanced by increased use of simulation and evolving ...
It is demonstrated that a wide range of technologies are now used within healthcare education, and this requires educators to adapt their practice and develop their technical skills to be competent users. This paper presents a literature review into the use of technology in healthcare education. A search of three electronic databases resulted in 20 articles for inclusion in the review.
We share published use-cases, research manuscripts, and reviews to highlight the breadth and depth of technology used in clinical health education. A literature search of different electronic databases was conducted using the following terms: (education OR training OR skills) AND (clinical OR health OR medical) AND (technology OR computer OR ...
The future of healthcare is technology, whether you're a seasoned professional or a first-year student. With the move to online education, technology has also become a bridge between schools, instructors, and students. In addition to virtual lectures and online exams, you have patient simulations, augmented reality, and other training ...
How Has Technology Changed the Health Education Essay. Technology influenced the process of study drastically, particularly in the medical field. Students now have an opportunity to access a more significant amount of information and research than ever, which can be extremely valuable for the educational process.
Abstract. Technology has revolutionized the healthcare industry, impacting various aspects of patient care, clinical practice, and healthcare systems. This paper provides a comprehensive review of ...
Health professions education has been slow in adopting new technology, but that pace, too, has now accelerated. 24 There are now many technologies embedded in our educational system that have improved efficiency and pedagogy and have helped to accomplish other educational goals. Simulation has provided safe and controllable settings for skill ...
This literature review sought to explore the types of technologies used within healthcare education, the modes of delivery and successful outcomes, and what measures are used to assess and evaluate the use of technology. Three databases were used to conduct a systematic search for literature: Academic Search Complete, CINAHL and MEDLINE.
This essay examines the state of Artificial Intelligence (AI) based technology applications in healthcare and the impact they have on the industry. This study comprised a detailed review of the ...
The search was made during August 2022 and identified 5847 papers, of which 321 fulfilled the inclusion criteria for further process. Finally, by removing and adding additional studies, we ended with 287 articles grouped into five themes: information technology in health, the educational impact of e-health, the acceptance of e-health ...
The World Health Organization (WHO) defines health technology as the application of organised knowledge and skills in the form of medicines, medical devices, vaccines, procedures and systems developed to solve a health problem and improve quality of life (World Health Organization, Health technology assessment).The Organisation for Economic Co-operation and Development (OECD) defines health ...
The increased prominence of the use of technology in the health care arena poses predictable challenges for many lay users, especially people with low health literacy, cognitive impairment, or limited technology experience. For example, remote health care management may be more effective when it is supported by technology, and various ...
British Dental Journal (2023) Artificial intelligence (AI) is rapidly transforming the healthcare and medical and dental education sectors. With advancements in AI technology and its integration ...
Therefore, it would be fair to conclude that technology has positively affected healthcare. First, technology has improved access to medical information and data (Mettler 33). One of the most significant advantages triggered by technology is the ability to store and access patient data. Medical professionals can now track patients' progress ...
This is a position paper based on the given topic. It contains the author's opinion on the impact of technology on healthcare services. The paper also discusses how technology has changed the education and labor requirements for the healthcare sector. Get a custom Critical Writing on Impact of Technology on Healthcare Services. 809 writers ...
The use of technology in medical education has been developing over many years. The trend in the use of technology has primarily developed in response to the challenges facing medical education. These challenges to medical education are numerous ( Table 1 ). The changing healthcare environment, with the movement of medical care from the ...
This dissertation investigates the use of health information technology by health care providers and patients as well as its outcomes. This dissertation consists of three essays studying workflow optimization in hospital emergency departments, people's search for online health information, and the relationships between EMR (Electronic Medical ...
500 Words Essay on Technology In Healthcare. Impact Of Technology In Healthcare Technology plays a massive role on almost all procedures and practices performed by healthcare professionals. From dominating small devices used by people on a daily basis to the complex gigantic equipment that rules the entire manufacturing processes of the ...