- Meet the Community
- Submit Content
- Become a Partner
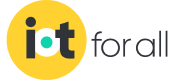
- About IoT For All

SUBMIT CONTENT
- White Papers

What is Smart Farming? It's The Future of Agriculture

The Internet of Things (IoT) has provided ways to improve nearly every industry imaginable. In agriculture, IoT has not only provided solutions to often time-consuming and tedious tasks but is totally changing the way we think about agriculture. What exactly is a smart farm, though? Here is a rundown of what smart farming is and how it’s changing agriculture.
What is a Smart Farm?
Smart farming refers to managing farms using modern Information and communication technologies to increase the quantity and quality of products while optimizing the human labor required.
Among the technologies available for present-day farmers are:
- Sensors : soil, water, light, humidity, temperature management
- Software : specialized software solutions that target specific farm types or applications agnostic IoT platforms
- Connectivity : cellular , LoRa
- Location : GPS, Satellite
- Robotics : Autonomous tractors, processing facilities
- Data analytics : standalone analytics solutions, data pipelines for downstream solutions
Armed with such tools, farmers can monitor field conditions and make strategic decisions for the whole farm or a single plant without even needing to step foot in the field.
The driving force of smart farming is IoT — connecting machines and sensors integrated on farms to make farming processes data-driven and automated.
The IoT-Based Smart Farming Cycle
The core of IoT is the data you can draw from things and transmit over the internet. To optimize the farming process, IoT devices installed on a farm should collect and process data in a repetitive cycle that enables farmers to react quickly to emerging issues and changes in ambient conditions. Smart farming follows a cycle similar to this one:
1. Observation . Sensors record observational data from the crops, livestock, soil, or atmosphere.
2. Diagnostics. The sensor values are fed to a cloud-hosted IoT platform with predefined decision rules and models—also called “business logic”—that ascertain the condition of the examined object and identify any deficiencies or needs.
3. Decisions . The user and/or the machine learning-driven components of the IoT platform assess the revealed issues to decide if location-specific treatment is necessary.
4. Action . After end-user evaluation and action, the cycle repeats from the beginning.
Smart Solutions to Agriculture’s Problems
Many believe that IoT can add value to all areas of farming, from growing crops to forestry. While there are several ways that IoT can improve farming, two of the major ways IoT can revolutionize agriculture are precision farming and farming automation.
Precision Farming
Precision farming, or precision agriculture, is an umbrella concept for IoT-based approaches that make farming more controlled and accurate. In simple words, plants and cattle get precisely the treatment they need, determined by machines with superhuman accuracy. Precision farming enables decision-making on a per square meter or even per plant/animal basis, contrasting with the classical approach which makes decisions at the field level.
By precisely measuring variations within a field, farmers can boost the effectiveness of pesticides and fertilizers, or use them selectively.
Precision Livestock Farming
As is the case of precision agriculture, smart farming techniques enable farmers better to monitor the needs of individual animals and adjust their nutrition accordingly, thereby preventing disease and enhancing herd health.
Large farm owners can use wireless IoT applications to monitor the location, well-being, and health of their cattle. With this information, they can identify sick animals, so that they can be separated from the herd to prevent the spread of disease.
Automation in Smart Greenhouses
Traditional greenhouses control the environmental parameters through manual intervention or a proportional control mechanism, which often results in production loss, energy loss, and increased labor costs.
IoT-driven smart greenhouses can intelligently monitor as well as control the climate, eliminating the need for manual intervention. Various sensors are deployed to measure the environmental parameters according to the specific requirements of the crop. That data is stored in a cloud-based platform for further processing and control with minimal manual intervention.
Agricultural Drones
Agriculture is one of the major verticals to incorporate ground-based and aerial drones for crop health assessment, irrigation, crop monitoring, and other spheres.
Since drones collect multispectral, thermal, and visual imagery while flying, the data they gather provide farmers with insights into a whole array of metrics: plant health indices, plant counting and yield prediction, and so on.
Importantly, IoT-based smart farming doesn’t only target large-scale farming operations. It can add value to emerging trends in agriculture like organic farming, family farming, including breeding particular cattle and/or growing specific cultures, preservation of particular or high-quality varieties, and enhance highly transparent farming to consumers, society and market consciousness.
Third Green Revolution
Smart farming and IoT-driven agriculture are paving the way for what can be called a Third Green Revolution.
Following the plant breeding and genetics revolutions, the Third Green Revolution is taking over agriculture. That revolution draws upon the combined application of data-driven analytics technologies, such as precision farming equipment, IoT, big data analytics, robotics, etc.
In the future, this smart farming revolution depicts, pesticide and fertilizer use will drop while overall efficiency will rise. IoT technologies will enable better food traceability, which in turn will lead to increased food safety. It will also be beneficial for the environment, through, for example, more efficient use of water, or optimization of treatments and inputs.
Therefore, smart farming has a real potential to deliver a more productive and sustainable form of agricultural production, based on a more precise and resource-efficient approach. New farms will finally realize the eternal dream of mankind. It’ll feed our population, which may explode to 9.8 billion by 2050 .
This article was originally published on June 22, 2020 and updated November 11, 2023 .
- Agriculture
- Data Analytics
More Like This

Smart Cities and EU Compliance: Data Governance and Sustainability

Revolutionizing Waste Management on Construction Sites with IoT and AI

NDT and IoT: Improving Safety in Industrial Applications

How Are AI and IoT Changing the Landscape?
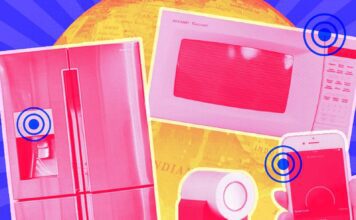
Why Sub-GHz Continues to be a Critical Piece of the Smart Home Puzzle
Trending now.

AI Ethics and the Human Problem
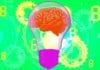
8 Helpful Everyday Examples of Artificial Intelligence
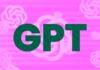
What Does GPT Stand For?

Cat 1bis, 5G RedCap & Satellite NTN: Which Should You Choose?

Best Practices for IoT Security: 7 Strategies for IoT Vendors
More articles.

Latest IoT News

Zendata raises $2M to redefine AI governance and data privacy with no-code platform
The digicert 2024 state of digital trust report, edf boosts private lte at french nuclear plants with ericsson, thales, fact of the day – 5/31/2024, the pivotal role of sensory intelligence in modern manufacturing, google introduces generative ai for mobile and web applications with firebase genkit, intelligent edge: the future of iot, semtech, itron, druid, nokia bring support for 1.8 ghz ‘utility’ band in canada, benefits of robotic process automation for fully automated companies, a review of top 10 generative ai market size reports 2024-2030 in uk, europe, asia, and usa for market growth, segments and forecast, mckinsey: gen ai adoption rockets, generates value for enterprises, anthropic’s claude ai now autonomously interacts with external data and tools, which country is leading in iot, state-directed, mega-sized, business-changing – five key private 5g deployments in china, fact of the day – 5/30/2024, edge as a service for factory modernization, blecon unveils bluetooth le-based iot connectivity solution, blecon debuts iot connectivity solution using bluetooth le, atlas copco installs ericsson private 5g at new uk smart factory, fact of the day – 5/29/2024, quectel unveils latest iot innovations at communicasia 2024, kpn launches private 5g product for dutch enterprises, the rise of saas, ai, and what comes next, structured roadmap: mastering digital transformation in manufacturing.

Search IoT For All

An official website of the United States government
The .gov means it’s official. Federal government websites often end in .gov or .mil. Before sharing sensitive information, make sure you’re on a federal government site.
The site is secure. The https:// ensures that you are connecting to the official website and that any information you provide is encrypted and transmitted securely.
- Publications
- Account settings
Preview improvements coming to the PMC website in October 2024. Learn More or Try it out now .
- Advanced Search
- Journal List
- Sensors (Basel)

A Systematic Review of IoT Solutions for Smart Farming
Emerson navarro.
1 School of Technology and Management, Computer Science and Communication Research Centre, Polytechnic Institute of Leiria, Campus 2, Morro do Lena—Alto do Vieiro, Apartado 4163, 2411-901 Leiria, Portugal; tp.airielpi.ym@3320812 (E.N.); [email protected] (N.C.)
António Pereira
2 INOV INESC Inovação, Institute of New Technologies, Leiria Office, Campus 2, Morro do Lena—Alto do Vieiro, Apartado 4163, 2411-901 Leiria, Portugal
The world population growth is increasing the demand for food production. Furthermore, the reduction of the workforce in rural areas and the increase in production costs are challenges for food production nowadays. Smart farming is a farm management concept that may use Internet of Things (IoT) to overcome the current challenges of food production. This work uses the preferred reporting items for systematic reviews (PRISMA) methodology to systematically review the existing literature on smart farming with IoT. The review aims to identify the main devices, platforms, network protocols, processing data technologies and the applicability of smart farming with IoT to agriculture. The review shows an evolution in the way data is processed in recent years. Traditional approaches mostly used data in a reactive manner. In more recent approaches, however, new technological developments allowed the use of data to prevent crop problems and to improve the accuracy of crop diagnosis.
1. Introduction
The challenge of food production in the 21st century is an increasingly relevant theme as population growth increases year after year. It is estimated that by 2050 the world will have between 9.4 and 10.1 billion people who depend on the world’s biodiversity to live, increasing the demand for dedicated food production areas—specifically for planting and livestock [ 1 ]. Environmental changes caused by human beings could potentially cause conditions in which the development of new crops is not possible. Likewise, the growing urbanization decreases labor in areas typically involved in food production, increases costs and reduces the productive capacity of the sector [ 2 ]. In view of this, smart farming is a new farm management concept that employs techniques and technologies at various levels and scales of agricultural production, enabling to overcome the challenges in food production demands and reduction in the workforce [ 3 , 4 ]. For example smart farming may use different types of sensors to collect data (e.g., temperature, humidity, light, pressure, presence, etc.), communication networks to send and receive data which is then managed and analyzed by management information systems and data analysis solutions [ 5 ]. This system of interconnected devices is commonly referred to as internet of things (IoT) [ 6 ]. The use of the data provided by smart farming helps boosting productivity and minimizing waste by allowing necessary actions to be carried out at the right time, quantity and place [ 7 ].
Moreover, recent technological developments in areas relevant to IoT facilitates an easier adoption and use of smart farming with IoT [ 8 ]. Such technological developments include, for example, network communications, reduction of hardware size, optimization of power consumption and devices cheapening. Furthermore, the World’s largest agricultural producers are promoting the usage of IoT in smart farming by creating incentive programs and public policies to fund research and training [ 9 , 10 ].
Several reviews have been published on IoT solutions for smart agriculture in recent years which denotes that this research field is being constantly receiving new contributions and constant improvement. Existing reviews usually focus on topics like network technologies, embedded system platforms, unmanned aerial vehicles (UAV) devices, network protocols and topologies and enabling cloud platforms. For instance [ 11 ] focuses on arable farming from year 2008 to 2018 and surveys communication technologies and protocols, the generation and analysis of data, IoT architectures and applications and highlights the challenges and future directions related with the application of IoT technologies on arable farming. Review [ 12 ] presents technologies used for communication and data collection within IoT solutions for smart farming as well as several cloud-based IoT platforms used for IoT solutions for smart farming. Additionally, authors present several use cases for the identified applications of IoT for smart farming. Review [ 13 ] presents a systematic review of papers published between 2006 and 2016 and classifies these papers in application domains, such as monitoring, controlling, logistic and prediction. Within these domains, authors also identified the data visualization strategies and the technologies used for communication and edge computing. Review [ 14 ] presents a review of papers published between 2010 and 2016. The authors rely on an IoT architecture with three layers (perception, network, application) to analyze the reviewed papers in terms of perception devices, network technologies and applications. With this, they identify embedded platforms and communication technologies used in IoT solutions as well as the application of such IoT solutions. Finally, [ 15 ] reviewed papers published between 2010 and 2015 and presents a state-of-the-art of IoT solutions for smart farming and smart agriculture. Authors relied on an IoT architecture with three layers (perception, network and application) to analyze the application of sensor and actuator devices and communication technologies within several farming domains, such as agriculture, food consumption, livestock farming, among others.
This study aims to identify how IoT is used with smart farming by (i) presenting a systematic review of the state of the art of the IoT adoption in smart agriculture and (ii) identifying the most commonly used hardware, platforms, network protocols and technologies and their applicability to the proposed solutions. To give a more up-to-date view to the reader about the smart agriculture research field, this review article surveys literature until year 2020 and evaluates the acceptance of (new) IT technologies, such as big data, computer vision, artificial intelligence, blockchain and fuzzy logic in the smart agriculture field. This review complements the analysis already carried out by the academy by performing a comprehensive review of the state-of-the-art of IoT in smart farming. To do so, we rely on an IoT architecture with 4 layers (perception, network, processing and application) to identify the technologies that enable IoT in smart farming. From this approach, the present work identifies an increasing use of modern techniques and technologies for processing the collected data in recent years, such as big data, machine learning, computer vision and blockchain. Moreover, this review contributes to the existent literature by reporting a change in the treatment of data in recent works: while previous work showed that the majority of decision support systems used simple processing mechanisms to handle data collected in real-time, more recent work showed an increasing number of management systems that use complementary technologies that rely on cloud and big data computing for processing large amounts of data. In terms of research domain, this article addresses the agriculture economic sector, including indoor and outdoor agriculture (greenhouse, hydroponics, crop beds, pots, orchards, permanent crops, and arable lands). However, the livestock farming (both indoors and outdoors) was left out because, due to its specificities like impact on nature, associated mobility, different species (mammals, birds, fishing) deserves a separated and specific review.
For this purpose, the remaining work is organized as follows: Section 2 presents the introductory concepts discussed in this study, Section 3 presents the methodology followed for the systematic review, Section 4 details the results obtained, Section 5 presents the considerations and Section 6 presents the conclusions.
2. Concepts
To better understand the questions related to the use of IoT in agriculture during the 21st century, it is important go deeper in some fundamental concepts.
2.1. Smart Farming
Smart farming can be defined as the application of supplementary technologies to agricultural production techniques to help minimize waste and boost productivity.
For such, intelligent farms use technological resources that help in various stages of the production process, such as monitoring of plantations, soil management, irrigation, pest control, delivery tracking, etc. [ 16 ]. Such resources include, among others, temperature, luminosity, humidity, pressure, ground chemical concentration, unmanned flying equipment, video cameras, agricultural information management systems, global positioning systems (GPS) and communication networks [ 17 ].
The integration of technological resources into the agricultural production process is a relevant issue. From an economic point of view, the precision agriculture market is expected to have a revenue of US$10 billion in 2023 [ 18 ] with opportunities for technology providers, agricultural equipment and machinery providers, producers and others involved in this business. In addition, smart farms are expected to be able to optimize food production by improving the application of nutrients to the soil, reducing the amount of pesticides and water consumption in irrigation [ 19 ].
2.2. Internet of Things
IoT can be understood as a network of interconnected intelligent devices capable of communicating with each other, generating relevant data about the environment in which they operate. Thus, virtually any device capable of establishing a connection to the Internet can be considered a “thing” within the context of IoT, such as household appliances, electronics, furniture, agricultural or industrial machinery and even people [ 6 ].
Although the idea of IoT is not new, its adoption has increased in recent years, mainly thanks to the development of technologies that support it, among which the improvement of hardware—with the consequent reduction in size and power consumption—improvements in connectivity with the Internet and between devices via wireless connection, cloud computing, artificial intelligence and big data. All these technological components help build a network of devices capable of sharing data and information, as well as acting actively based on network inputs [ 20 ].
According to [ 21 ], the architecture of IoT systems is similar to the architecture of other computer systems but it must take into account the particularities of this paradigm, such as the limited computing capabilities of the devices, identification, detection and control of remote objects.
2.3. Intelligent Agriculture
The IoT architecture proposed in [ 22 ] and [ 23 ], and shown in Figure 1 , presents four layers, considering the main components of an IoT solution: devices, network, services and application.

IoT solution architecture that includes 4 layers: perception, transport, processing and application, based on [ 22 , 23 ].
The perception layer relates to the physical devices in the solution and how they interact with each other and with the transport layer. These devices are responsible for collecting data, enabling the communication of the so-called “things”. This can be done by using commercial solutions—such as UAV devices [ 24 ], sensor nodes [ 25 ]—or new devices, developed with components like sensors and single-board computers (SBC)—such as Arduino or Raspberry Pi—to build sensor nodes and communication gateways. Sensor nodes, for example, are used to monitor plant diseases [ 26 ], control environmental variables in greenhouses [ 27 ] and external crops [ 28 , 29 , 30 ], among others. The interaction between the devices that belong to the perception layer and the services that belongs to the processing layer is intermediated by the transport layer and might occur in several ways, such as through the direct communication between sensor nodes and a data processing platform (such as FIWARE [ 31 ], SmartFarmNet [ 32 ] and Thinger.io [ 33 ]) or through a gateway that, besides intermediating the communication between sensor nodes and the internet, acts as a data hub and enables the communication between network protocols that are originally incompatible, such as ZigBee and the Internet [ 28 ].
The transport layer refers to the network and transport capabilities such as network and application protocols [ 22 ]. IoT solutions use network protocols to enable communication between the perception layer and the processing layer. These protocols are used to create the so-called wireless sensor networks (WSN), that allows wireless communication between sensor nodes and applications. Each protocol has important characteristics, such as the data exchange rate, range and power consumption. Based on these characteristics such protocols can be classified in short-range, cellular networks and long-range [ 34 ]. Protocols for short-range networks (e.g., Bluetooth, ZigBee and Wi-Fi) enable communication in short distances. According to [ 34 ], usually such protocols have a high data transmission rate and low power consumption. Therefore, they are used for the communication between devices that are near each other. Protocols for cellular networks (e.g., GPRS, 3G) enable communication in long distances and with a high data transmission rate. However, they have a high power consumption [ 35 ] and costs for licensing [ 34 ]. Protocols for long-range networks (e.g., LoRaWAN and Sigfox) enable communication in very long distances [ 34 ]. These protocols are used to establish the low power wide area networks (LPWAN) due to the fact that they have a low power consumption [ 36 ]. However, the data transmission rate of these protocols is low. Therefore, these protocols are appropriate for use when the solution needs to transmit a few amounts of data in very long distances. Table 1 presents the characteristics of some network technologies used for IoT.
Examples of network technologies used in IoT [ 34 ].
As shown in Table 1 there is a trade-off between coverage, data rate and energy consumption. Considering the technologies for star networks presented in Table 1 , it is possible to notice that energy consumption is higher in technologies with a high data rate and short coverage. On the other hand, LoRa has a small data rate but a large coverage and low power consumption. These questions are especially relevant when considering agriculture because agricultural scenarios often have limited or no energy supply and obstacles for wireless communication.
Different topologies can be used for implementing networks, such as tree, star and mesh. Star networks have a central node and several peripheral nodes. The communication in such topology occurs as follows: peripheral nodes send data directly to the central node. The central node can implement capabilities for routing messages and communicating through multiple network protocols [ 23 ]. Tree networks are composed of router nodes and leaf nodes. Such networks can be understood as a cluster of star networks. Within each cluster, leaf nodes send messages their father node. In mesh networks, in theory, each node can be a router with rerouting capability. Thus, messages in mesh networks are routed hop by hop until reaching the final destination [ 37 ].
Data is sent to the destination through application protocols such as the message queueing telemetry transport (MQTT) [ 38 ] or the constrained application protocol (CoAP) [ 39 ]. MQTT is an open-source messaging protocol that enables communication between constrained devices and in unreliable networks [ 40 ]. MQTT runs over TPC/IP or similar protocols (e.g., Bluetooth) [ 41 ], which makes the use of MQTT appropriate for different IoT solutions. The MQTT protocol, which is based on the publish/subscribe architecture, allows communication between devices to take place in the following way. First, devices publish messages that are structured in topics on a message broker. Then, other devices read these messages by subscribing to relevant topics on the message broker. These topics allow the organization of messages based on categories, subjects, etc. [ 42 ]. The use of MQTT for communication between device allows low coupling between the device that publishes the message and the devices that listen to the messages, the so-called “one-to-many” communication [ 38 ]. Like MQTT, CoAP is a communication protocol optimized for constrained devices and unreliable networks. However, CoAP messages are interchanged using User Datagram Protocol (UDP) and the CoAP protocol is based on the client/server architecture. This architecture requires that a connection is established between devices before any messages are transmitted [ 38 ]. For this reason, communication using CoAP works in the following way. First, the device that sends messages needs to know the address of each device that is expected to receive messages. Then, messages are sent over UDP to the specified address. Due to the use of UDP, CoAP messages are classified accordingly to the required status of confirmation of receival, for example, confirmable or non-confirmable [ 39 ]. The CoAP protocol does not implement a structure of topics for messages. However, a similar approach can be implemented using application programming interface (API). Nonetheless, the use of CoAP creates a high coupling between the device that sends messages and the device that is expected to receive messages, as the communication is “one-to-one” [ 39 ].
The processing layer comprises data storage, visualization and processing resources. In this context, big data allows distributed storage and parallel data processing, enabling the extraction of information in the shortest possible time [ 43 ]. Such information are used as models by artificial intelligence (AI) systems—which, according to [ 44 ], can be understood as the ability of a system to operate as if it had the thinking capacity of a human being—and machine learning—that, according to [ 45 ] is a data processing technique to detect patterns and correlation among complex and unrelated data—for the development of decision support systems and automation of irrigation control systems [ 46 ], monitoring [ 47 ] and diseases detection in crops [ 48 ], for example.
Finally, the application layer comprises IoT applications that, supported by the other mentioned layers, provide management information to farmers, being able to manage the entire production process in the plantations.
Several related works are being developed in recent years. This rich literature has already been analyzed by the academia from multiple perspectives with objective of determining the state of the smart farming development. Thus [ 49 ] presented a systematic review of precision livestock farming in the poultry sector and [ 50 ] made a review of state of the art of technologies used in precision agriculture, focusing in the innovations, measured parameters, technologies and application areas. On the other hand [ 4 ] has focused on the use of big data as a tool to support agriculture, pointing out the main opportunities and challenges of using this technology. Finally, [ 51 ] presented a quantitative literature review on smart farming related papers, helping to outline an overview of academic production related to the subject. In this way, the present work aims to complement such analyses by making a systematic review of IoT solutions applied to smart farming.
To reach the proposed objectives, this study has used the Preferred Reporting Items for Systematic Reviews (PRISMA) methodology, which is a framework developed to support reports and systematic reviews of literature [ 52 ].
As a research strategy, in October 2019 a search was made in the Scopus database through the search tool available on the website. In addition, in June 2020 a new search was made in the same database to include papers published in 2020. The choice of this database took into consideration its scope and relevance in the academia, since this database indexes several journals and catalogues, such as IEEE, ACM and Elsevier, besides being widely used in similar bibliographic reviews, as in [ 4 ] and [ 51 ]. In addition, in February 2020 a new search was performed in the same database. The strategy adopted for the work research in this database looked for terms used to refer to the application of technology in the area of agriculture, such as “Precision Agriculture”, “Precision Farming”, “Smart Farming” and “Smart Agriculture” in association with “IoT” and synonyms terms. The publication date of the articles was not a criterion for ignoring them. The scope of the research was limited to documents such as journal and conference articles, published in English, Portuguese or Spanish, and whose access was fully available. Thus, the resulting search instruction for the database was as follows:
(“Smart Farming” OR “Smart Agriculture” OR “Precision Farming” OR “Precision Agriculture”) AND (“IoT” OR “Internet of Things” OR “internet-of-things”) AND (LIMIT-TO(ACCESSTYPE(OA)))
It should be noted that the quotation marks have the function of ensuring that terms composed of multiple words were searched together, thus preventing words from being considered individually.
After extracting the articles that resulted from the search, they were manually reviewed through the analysis of the title, keywords, abstract and text. Initially, based on this review, the works identified in the researched database were consolidated, thus eliminating duplicate articles.
Subsequently, the articles were validated as to their framing in the objectives proposed for this study and considered valid when: (i) they were not a review or bibliographical research (ii) they were related to theme (iii) they presented a technology or solution based on IoT to solve problems related to agriculture (iv) they were published in English, Portuguese or Spanish. Furthermore, works were also excluded when they were related to livestock activities instead of agriculture.
The process of searching and selecting papers for this study followed the workflow summarized in Figure 2 , where it can be observed that the initial search resulted in a total of 463 articles, which were analyzed, filtered and classified in a narrowing process that culminated in the selection of 159 articles.

PRISMA flowchart of the systematic review on state-of-the-art IoT solutions in smart farming.
In the identification phase 463 articles were selected with the search tool.
During the screening phase, a manual review of the articles was carried out to identify in the titles, abstract and key words the papers adherent to the objectives proposed for this study, following the criteria mentioned in this section. Among these, 257 were considered invalid and discarded. About 62% of the discarded items did not consider smart farming to be the focus of the work, although some presented improvements for IoT that could benefit smart farming indirectly. Additionally, almost 31% of the discarded papers were studies or literature reviews related to smart farming and the use of various technologies. A smaller number of papers related to smart farming but not addressing IoT (about 5%) and papers where the abstract or text were not available (about 2%) were also discarded.
During the eligibility phase, the content of the 206 resulting articles were reviewed and the papers were classified using the same criteria used in the previous step. In this phase 47 articles were discarded. Among the discarded articles 29% were not related to IoT and 30% were not related to smart farming. The other 41% of the discarded papers were paper reviews or papers without content available. This analysis resulted in 159 articles considered eligible which were included as a sample for this study.
4. Discussion
Based on the results obtained in the analysis of the articles considered for this study, it was possible to observe a growth trend in the number of publications related to IoT and smart farming since 2011, with special emphasis from 2016 onwards, as shown in Figure 3 .

Classification of reviewed papers according to the year of publication.
It is possible to observe an expressive increase of 278% in the number of published papers in 2017/2018. It’s also possible to observe a very similar number of published papers in 2019/2020, until the first semester of 2020. The amount of published papers in recent years evidences the increasing in discussion and the relevancy of the topic IoT applied to smart farming.
Within the reviewed papers it was identified the main scenarios and environments of agriculture. As shown in Figure 4 , such scenarios can be divided into indoor and outdoor. Environments for indoor scenario are protected from climatic impacts, such as solar radiation, rain and wind. Examples of environments for indoor scenarios include greenhouse, hydroponics, crop beds, pots, etc. In contrast, environments for outdoor scenario are more susceptible to climatic impacts. Examples of environments for outdoor scenario are arable lands, orchards and generic outdoor plantation.

Typical scenarios in agriculture can be divided in indoor and outdoor. Indoor agriculture includes environments such as greenhouse, hydroponics and crop beds. Outdoor agriculture includes environments such as orchards and arable lands. IoT solutions that may be applied to multiple environments are referred to as “Generic”.
4.1. Application
Within the reviewed papers it was also identified that the most common applications of IoT solutions for smart farming are:
- Chemical control (e.g., pesticides and fertilizers).
- Crop monitoring.
- Disease prevention.
- Irrigation control.
- Soil management.
- Supply chain traceability
- Vehicles and machinery control.
Table 2 presents the reviewed papers, grouped by agricultural environment and application of the IoT solution. It is worth mentioning that several IoT solutions presented on the reviewed papers could be applied to multiple environments ( Figure 4 ). Thus, such IoT solutions are classified as “Generic”. Additionally, the “Others” column in Table 2 includes papers whose IoT solutions were developed for agricultural environments that were less mentioned, such as pots, crop beds, etc. It is possible noting a predominance in projects where the application is for crop monitoring, irrigation management, and disease prevention.
Smart farming, application and environments.
As shown in Table 2 , the most common application of IoT solutions for smart farming is crop monitoring. Moreover, as shown in Table 2 , these solutions have been developed for multiple agricultural environments, such as arable lands, orchards, greenhouses, etc. The fact that this type of application is so common in agriculture can be justified by the relevance that crop monitoring has for farmers. IoT solutions developed for monitoring crops focused on collecting environmental data of plantations (such as temperature, humidity, luminosity, etc.). Farmers can use these data to obtain a better insight of the plantations. For example, such data was used to determine the vigor of rice [ 47 , 58 ], alfalfa [ 30 ] and maize [ 57 ] crops and to control the environmental conditions of greenhouses [ 99 , 100 , 102 , 104 ]. Similarly, IoT solutions for irrigation control has also been developed for multiple agricultural environments, as demonstrated in Table 2 . Such IoT solutions aimed to optimize the use of water resources in agriculture in different ways, such as by simply using sensors for measuring the soil moisture and using these data for controlling the irrigation source [ 34 , 139 ] or in a more sophisticated way, by combining humidity data with datasets of weather to determine the amount of water required during the irrigation [ 140 ]. IoT solutions for disease prevention aimed to identify and prevent diseases on plantations. For this purpose, these IoT solutions collected multiple environmental and plantation data, such as images of plants [ 129 , 132 , 134 ], sounds [ 135 ], temperature, humidity, etc. [ 131 , 137 ]. These data were processed with different approaches, such as image processing [ 129 , 134 ] or artificial intelligence [ 132 , 137 ]. For example, the IoT solution developed in [ 129 ] processes images collected from a sugarcane crop and identifies diseases on the leaves of plants. In addition, [ 135 ] developed an IoT-enabled device that captures sounds produced by larvae inside trees. IoT solutions for chemical control presented in Table 2 aimed to optimize the application of fertilizers and pesticides on plantations. For this purpose, these IoT solutions collect data (such as nitrogen, salinity or PH) from the crops. Based on the collected data, such IoT solutions can identify crop areas that may require the application of fertilizers or pesticides. For example, in [ 54 ] aerial images of crops are processed to determine the nitrogen concentration in a large plantation. These images are useful to determine the specific region that requires fertilizer. In addition, [ 55 ] developed an automated robot that optimizes the application of pesticides in greenhouse cultivations. IoT solutions for soil management aimed to identify different soil attributes used for planting. For example, such IoT solutions are used to measure the soil moisture [ 163 ], to identify the water consumption pattern [ 159 , 161 ] and to identify the nutrients of the soil [ 158 ]. IoT solutions for vehicles and machinery control focused on collecting data of and managing agricultural equipment and machinery such as tractors, harvesters and trucks. For this purpose, IoT solutions had to deal with the characteristics inherent to agricultural equipment, such as mobility. Data from the equipment itself, such as implement status, engine performance, or speed are collected using sensors [ 41 ] to optimize their maintenance cycle. Additionally, due to the mobility of agricultural equipment, opportunistic computing was used to collect data from remote crop areas by using sensors coupled to tractors [ 174 ].
Each agricultural environment presented in Table 2 brings its own challenges for the projects, which includes the environment impact on the communication between sensors, either by the distance between the sensor nodes [ 25 , 105 , 180 ], by the lack of communication in the croplands [ 98 , 174 ] or even by the impact of vegetation in the signal propagation [ 70 , 175 ]. Furthermore, as indicated in [ 28 ], climatic elements—such as rain, snow or solar radiation—have influence on both the planting and the sensor nodes.
To cover these scenarios commercial electronic sensors are used by 96% of the reviewed papers. This expressive usage can be justified by the fact that such sensors are affordable, certified, ready-to-market and meet the main monitoring needs in IoT solutions for smart farming. Such sensors are used for collecting real-time data about multiple agricultural parameters, such as climatic data, substrate information, luminosity, CO2 concentration and images through cameras and multispectral sensors, as shown in Table 3 . Moreover, several papers (4%) focused on developing custom-made sensors for monitoring specific agricultural aspects, such as soil nutrients (e.g., nitrate [ 158 ]) and leaf evapotranspiration for measuring the hydric stress in tobacco crops [ 81 ].
Types of physical sensors and use in smart farming.
Different types of sensors are used in agriculture for collecting data from different aspects of agriculture such as crop monitoring, substrate monitoring and environment monitoring.
As presented in Table 3 , different types of sensors were used in IoT solutions for smart agriculture to collect data from multiple aspects of agriculture, such as the crop, substrate, environment and other. For this purpose, as shown in Table 3 , for environment monitoring electronic sensors were used in IoT solutions to collect environmental data, such as temperature, humidity and luminosity [ 104 , 109 , 114 ]. In addition, for substrate monitoring electronic sensors were used to collect data from the substrate (e.g., soil and water), such as temperature, moisture and nitrogen. Likewise, pH sensors were used for measuring the acidity or the alkalinity of the water in hydroponics cultivations. For crop monitoring, cameras and multispectral sensors were used to collect images of crops. These sensors can be installed on an UAV to obtain aerial images of large plantations [ 47 , 57 , 58 ] or used in robots to retrieve a detailed image of the leaf of a plant [ 111 ].
4.2. Perception
The choice of hardware is a very important aspect of the IoT project development because it impacts the costs and the technologies that can be used. 60% of the reviewed papers mentioned the hardware used to support the IoT solution. Furthermore, SBCs were mentioned by 40% of the reviewed papers. The use of SBCs can be justified by the fact that these devices are affordable and versatile [ 38 ], enabling the development of custom-made IoT devices. For example, some SBCs such as Arduino has an integrated development environment (IDE). This IDE enables the development of custom programs to be installed as firmware on the Arduino boards [ 184 ]. Similarly, Raspberry Pi is compatible with several operating systems, such as Raspbian, Ubuntu Core or Mozilla Web Things [ 185 ]. Some of these operating systems are open-source, which allow for the customization of its source-code. Besides, these operating systems support applications developed with programming languages such as Python [ 26 ]. Furthermore, the capabilities of SBCs can be extended by associating them with other hardware components, such as sensors or transceivers. This characteristic makes SBCs able to work as gateways or sensor nodes in IoT solutions. Among the papers that mentioned SBCs, 82% mentioned the use of Arduino, Raspberry Pi and ESP boards (such as ESP8266, ESP12 and ESP32). Table 4 presents the application of embedded system platforms and UAV devices in smart farming.
Embedded system platforms and UAV devices in smart farming.
As shown in Table 4 , IoT-enabling devices are used for multiple applications on IoT solutions for smart farming. SBCs were used both as sensor nodes and gateways. Table 4 reveals that Arduino was the most commonly used embedded system platform among the reviewed papers. The extensive use of Arduino can be justified by the fact that Arduino is open-source hardware that enables the development of different devices through the use of boards that extend their native functionality. Table 4 also shows that embedded system platforms have been more widely used in IoT solutions for crop monitoring. As sensor nodes, for example, in [ 124 ] sensors for collecting environmental data such as soil humidity, solar radiation and rain are connected to an Arduino Uno. The Arduino is, then, used to monitor the health of a vineyard. Likewise, in [ 117 ] a Raspberry Pi is used to manage the temperature and air humidity of a greenhouse. IoT devices are also used as gateways to connect short-range WSN with the internet by using long-range communication protocols. For example, in [ 127 ] a gateway is used to connect WSNs using 3 different protocols (ZigBee, Bluetooth and Wi-Fi) with a remote server by using 3G. In [ 84 ] a LoRaWAN gateway obtains data from sensor nodes using LoRa and retransmits this data to a cloud-hosted platform by using 4G. 3G and 4G are cellular network technologies that, as discussed in Section 2.3 , enable communication in long distances and with a high data transmission rate. These technologies will be discussed with more details in Section 4.3 .
In addition, Table 4 also reveals that UAV is widely used by IoT solutions for monitoring crops, disease prevention and chemical control. The use of UAV for crop monitoring is due to the fact that UAV has the potential to accelerate and reduce the cost of monitoring extensive crops. For this purpose, cameras and multispectral sensors are attached to UAV devices that are used to obtain aerial images from large crops. Such images are processed by the IoT solution to calculate agricultural parameters, such as the leaf area index (LAI). The LAI is a parameter used to determine the vegetation coverage within a specific area. LAI, combined with other parameters, can be used to evaluate the amount of nitrogen in rice crops [ 58 ], determine the vigor of rice and maize [ 47 , 57 ] crops and detect diseases in sugarcane crops [ 129 ]. Moreover, UAV devices are used in [ 46 ] to optimize the application of pesticides and fertilizers in arable lands.
4.3. Network
Data obtained with sensor nodes are usually sent to the destination (e.g., database, server, IoT platform) through a wired or wireless network. Within the reviewed papers, 60% have mentioned the network protocol used in the IoT solution. Among the mentioned network protocols, CAN and Ethernet were the most used ones for wired networks. Likewise, LoRaWAN and protocols for cellular network (e.g., GPRS, 3G, etc.) were the most used protocols for long-range wireless networks. Analogously, ZigBee, Wi-Fi and Bluetooth were the most used protocols for short and mid-range wireless networks. Table 5 shows network protocols used for the IoT solutions within the reviewed articles.
Use of network protocols in smart farming for different farming scenarios.
As shown in Table 5 , several network protocols are used in different environments of agriculture (e.g., arable land, greenhouse, orchard) to enable communication between IoT solution devices, such as sensor nodes and gateways. Such network protocols enable the creation of short or long-range networks. Table 5 reveals that for short and middle-range communication, IoT solutions of the reviewed papers used different technologies, such as Wi-Fi, ZigBee and Bluetooth. Moreover, it is possible to observe in Table 5 that Wi-Fi is the most common network technology for communication within the analyzed articles. This extensive use of Wi-Fi can be justified by the fact that Wi-Fi is a ubiquitous technology and, therefore, easy to implement. However, due to the higher energy consumption of Wi-Fi, low-energy consumption technologies, such as ZigBee or Bluetooth, are also extensively used. For example, [ 62 ] used ZigBee to send images from a plantation to a remote server and [ 188 ] developed a sensor node that uses Bluetooth to deliver monitoring information from the farm directly to an application installed on a smartphone. Table 5 also demonstrates that IoT solutions of the reviewed papers used cellular networks, Sigfox, or LoRaWAN for long-range networks. Cellular networks are prevalent in IoT solutions for Smart Farming. This can be justified by the fact that cellular networks allow the communication of IoT devices in long distances and with a high data rate. For example, [ 141 ] uses cellular network to send data collected from humidity sensors to a cloud-based platform and to control an irrigation system. Similarly, Sigfox and LoRaWAN enable communication in very long distances while requiring low energy to operate. Based on these characteristics, Sigfox and LoRaWAN were used for long-range communication, as an alternative to cellular networks or in regions where there was no cellular network coverage. Sigfox is used in [ 34 ] as the network protocol of an IoT solution used to control the irrigation of a plantation. Likewise, in [ 137 ] the LoRaWAN is used to send data from multiple sensors installed in a greenhouse to a remote platform.
Besides the distance between sensor nodes, gateways, and other network elements, the vegetation itself can be an obstacle for sensor communication, as demonstrated by [ 175 ] and [ 70 ] who analyzed the impacts on signal propagation on 433 MHz and 2.4 GHz frequencies in rice plantations and an orchard. An additional challenge for greenhouses arises from the high density of sensors, which can lead to interference in the wireless signal due to proximity [ 105 , 112 , 180 ]. To mitigate this problem wired networks, such as CAN [ 100 ] or Ethernet [ 122 ], can be used. As shown in Table 5 , these technologies have been more used in greenhouses, because usually this type of agricultural environment is more appropriated for implementing wired networks. Moreover, [ 112 ] investigated the path loss on wireless signals and concluded that the proper positioning of directional antennas can optimize the number of sensory nodes required for monitoring a greenhouse.
Network topology is another important aspect of an IoT solution. According to [ 61 ] the topology of sensor networks can be star, tree (or cluster) or mesh. The network topology impacts the distance between the sensor nodes and the destination and, consequently, the number of sensor nodes in the WSN [ 190 ]. For example, star networks are composed of a central node (coordinator) and several peripheral nodes. In such topology, peripheral nodes send data to the central node [ 93 ]. Therefore, the maximum distance between the peripheral nodes and the central node is limited by the maximum distance allowed by the physical layer communication standard. On the other hand, as discussed in Section 2.3 , in mesh networks each node has routing capability, hence extending the network coverage by allowing multi-hop communications [ 191 ]. Based on the architecture of the IoT solution and on the project description it was possible to identify the topology adopted by 61% of the reviewed papers. For example, a star topology is used in [ 34 ] for connecting sensor nodes to a central node using the LoRa protocol. This central node acts as a gateway and retransmits messages to a cloud-based application that controls an irrigation system using Sigfox. Also, in [ 107 ] the star topology is used to connect multiple sensors within a greenhouse. Such sensors use the ZigBee protocol to send messages to a central node, which acts as the network gateway. Mesh networks are considered more complex to be implemented but also more reliable due to the redundancy of communication between the sensor nodes [ 105 ]. Such topology is used in [ 105 , 107 ] for monitoring a greenhouse. Tree (or cluster) networks combine multiple star networks. Both [ 61 ] and [ 178 ] implement a cluster network for monitoring crops. In [ 61 ] sensor nodes collect information from a crop and send messages to a router node. This router node acts as the gateway of the cluster and retransmits the message to the main router node of the network. In [ 178 ] several router nodes are deployed in the crop area in order to optimize the energy consumption of sensor nodes.
Furthermore, embedded system platforms have been used to support network topologies. The chart in Figure 5 presents the distribution of embedded system platforms by network topology or device connection type. It is worth mentioning that although point to point is not a network topology, this type of device connection was used in several IoT solutions within the review articles. As shown in Figure 5 , Raspberry Pi is often used in IoT solutions implementing the star network topology. Arduino is the embedded system platform used in multiple types of network topology or device connections. Additionally, Arduino is the most frequently used embedded system platform to support star network topology and point-to-point communication. Finally, ESP-based devices include devices that use system-on-a-chip (SoC) modules such as ESP-32 and ESP8266 (Espressif Systems, Shanghai, China). ESP-based devices are often used in IoT solutions that implement star network topology or point-to-point communication.

Distribution of IoT-enabling devices by network topology or device connection type within the reviewed papers.
As mentioned in Section 4.2 , embedded system platforms can be used to build gateways or sensor nodes. As shown in Figure 5 the use of Raspberry Pi, Arduino and ESP stand out, probably because such embedded system platforms are cost-effective [ 38 ] and enable different network protocols (e.g., ZigBee, Wi-Fi and Bluetooth) with the use of transceivers. This characteristic allows such embedded system platforms to act as sub-nodes and central nodes in a star network [ 27 , 30 , 34 ] or as router nodes in mesh and cluster networks [ 106 , 178 ].
IoT devices transmit information to cloud-based platforms or applications through application protocols [ 109 ]. Such protocols can follow the publisher/subscriber architecture which, as mentioned in Section 2 , are appropriate for devices with limited computing resources. Among the application protocols used in the reviewed papers HTTP, MQTT and CoAP stand out. Such application protocols are useful to enable compatibility between non-standardized IoT devices and IoT platforms. For example, SmarFarmNet developed in [ 32 ] adopts the “bring your own IoT device” concept by implementing loosely coupled application protocols such as MQTT and CoAP. Furthermore, although HTTP is not a specific protocol for machine-to-machine (M2M) communication, its use associated with REST APIs enables low coupling between IoT devices and applications, analogous to MQTT, for example. However, as [ 109 ] concludes, the MQTT protocol is preferable for smart farming applications due to its resiliency, interoperability across different network protocols and transmission rate.
Finally, although the power consumption is not an exclusive topic within the transport layer, according to [ 178 ] the highest power consumption for IoT devices within a WSN occur during the transmission of data. This review identified several approaches for optimizing the power consumption in IoT solutions for smart farming. Among the identified solutions are the use of low energy protocols (e.g., BLE, ZigBee, Sigfox), reduction of data transmission in sensor nodes by an optimized duty cycle [ 177 , 178 , 192 ] and the use of message routing approaches that are more energy-efficient [ 72 , 193 ].
4.4. Processing
Among the analyzed papers it was possible to observe that initially, the main objective of IoT solutions was to collect and store data from sensor nodes. However, in more recent years, it is possible to observe an increasing number of IoT solutions that used supplementary techniques and technologies to treat the collected data, such as cloud computing and big data. Likewise, it is possible to observe an increasing number of works that used simultaneously two or more techniques or technologies for processing data. As shown in Figure 6 , the most cited technologies within the reviewed papers are cloud computing (34%), machine learning (15%), big data (13%), and artificial intelligence (9%).

Techniques and technologies for data-processing in smart farming identified within the reviewed papers. In recent years, the use of modern processing techniques, such as artificial intelligence and big data became more common. IoT applications relies on cloud computing for storing and processing the big data of agricultural information collected by IoT devices.
Table 6 presents IoT solutions that relied on cloud-based platforms for processing data and highlights the main data processing techniques (e.g., Artificial Intelligence, Big Data, etc.). The column “Other/Not identified” comprehends IoT solutions that have used cloud-based platforms but have either (i) used any of the data processing technologies identified by other columns on Table 6 or (ii) not explicitly mentioned the type of data processing technology that was adopted.
IoT-enabling platforms and data processing technologies used in smart farming within the reviewed papers.
Table 6 reveals that the most found cloud-based platforms in the reviewed papers are ThinkgSpeak, FIWARE, Ubidots, SmartFarmNet, AWS IoT and Thinger.io. In particular ThingSpeak is the most used cloud-based platform across all the reviewed papers, due to the fact that this platform is open-source with low infrastructure requirements [ 34 ]. In addition, Table 6 shows that AWS IoT was used with a higher number of data processing techniques. Not all cloud-based platforms offer the same set of functionalities, but in general, they have capabilities for data storage [ 30 , 94 , 102 , 122 , 132 ], processing [ 194 ] and visualization [ 102 ] and action control on farms [ 34 ]. Furthermore, Table 6 also reveals that, even though there are multiple cloud-based platforms, several reviewed papers developed their own cloud-based platform for the IoT solution.
Cloud-based platforms provide scalability for IoT solutions by relying on cloud computing to process and data. For instance, some platforms shown in Table 6 , such as Thinger.io [ 33 ], are built entirely on top of infrastructure services provided by cloud providers (e.g., Amazon AWS and Microsoft Azure). Also supported by such services, the platforms make available data analysis modules with graphics and panels that allow real-time monitoring of the information obtained or the creation of customized panels from the integration of multiple data [ 33 ].
Due to the scalability provided by these platforms, the large amount of data generated by the sensors is stored in databases to form the so-called big data, an unstructured set of information that is used to generate information about crops. According to [ 197 ] big data demands the use of technologies to optimize the processing time due to the large volume of information. For example, Hadoop—a parallel database for big data applications—proved to be efficient when analyzing the rainfall index data from several meteorological stations [ 197 ].
IoT solutions use different types of techniques and technologies for processing the collected data. Table 7 presents commonly used technologies per applications as identified in the reviewed papers. Column “Other Technologies” encompasses all the technologies that are not identified by any of the other columns in Table 7 .
Technologies and application in smart farming.
Table 7 reveals that the most commonly used technologies to support data processing are artificial intelligence, machine learning, and big data. The use of these technologies is related to their ability to process large amounts of information in a short time. In addition, Table 7 also shows that crop monitoring is the most common type of application for IoT solutions that have used data processing technologies. Moreover, crop monitoring is also the type of application that used the most different technologies for data processing. This can be understood by the fact that usually IoT solutions for monitoring crops collect a bunch of data and rely on machine learning and big data to process such data.
As demonstrated in Table 7 , bigdata was used for different applications in IoT solutions, such as crop monitoring, soil management and irrigation control. For example, supported by big data, in [ 142 , 161 , 194 ] the soil moisture data gathered by physical sensors were related to data made available in datasets, such as the NASA Prediction of Worldwide Energy Resources (POWER) [ 198 ]—which contains meteorological data—purchase and sale values of crops, information from the user and government agencies to optimize the amount of water in irrigation cycles, support the farmer in the acquisition of agricultural inputs—such as seeds and fertilizers—and generate information and perspectives about other activities related to agriculture. Big data was also used by [ 56 ] in the development of a decision support system to provide irrigation and monitoring advice to farmers from a knowledge base created with data obtained by physical sensors (e.g., temperature, soil moisture) and virtual sensors (e.g., soil type, season). Virtual sensor is a type of software that, given the available information, processes what a physical sensor otherwise would [ 199 ].
In addition, automatic management with IoT depends on the manipulation of multiple variables. Initially, the simple observation of soil humidity and temperature can be used to trigger irrigation or cooling systems, as proposed by [ 187 ]. Nevertheless, greenhouse management can be more complex. As shown in [ 104 , 107 , 120 ], greenhouse parameters like temperature and humidity are closely tied and changing one of them can affect several others.
Fuzzy logic, as indicated in Table 7 , was used in IoT solutions applications that need to handle multiple variables, such as irrigation control and monitoring crops. For this purpose, [ 120 ] uses fuzzy logic to handle multiple variables of temperature and humidity into a greenhouse and determine when a cooling system and an irrigation system should be started. Similarly, [ 73 ] uses fuzzy logic to optimize the number of sensors for monitoring soil temperature and moisture. Machine learning was also used in data processing by [ 46 ] to predict environmental conditions based on the forecast values of weather, humidity, temperature and water level and thus to control an irrigation system, by [ 47 ] to combine multiple parameters obtained from images, such as color and texture indices and by [ 48 ] to identify marks on the plants and, thus, to identify possible diseases. Similarly, in [ 58 , 125 ] it was used to detect diseases, identify growth stages and the health of plantations.
Similarly, as shown in Table 7 , IoT solutions used computer vision for applications that need to deal with image processing, such as crop monitoring and diseases prevention. It was also possible to observe in the reviewed papers the use of computer vision to identify and classify elements in images obtained by cameras, enabling the identification of fruit in an orchard [ 200 ] or the existence of diseases and pests in plantations [ 48 , 129 , 133 ]. Additionally, in [ 133 ] computer vision was used as a monitoring tool to detect the presence of insects that can cause diseases in olive groves and in [ 48 ] the same technique was employed to analyze diseases that cause morphological deformations in plants. Additionally, computer vision was used in crop management systems, for example in [ 134 ] where it was implanted in a robot equipped with a camera and other sensors, being able to obtain images of vegetation and, through computer vision, detect weeds in plantations and eliminate them. Similarly, in [ 111 ] a robot can identify a plant and interact with the environment to irrigate it, if necessary.
Finally, blockchain proved to be an opportune technology for systems that need to implement traceability of the supply chain, as shown in Table 7 . According to [ 171 ] blockchain is a global public distributed ledger that records all transactions between users. In fact, this type of control is relevant for agriculture in several aspects, such as food safety, guarantee of origin or cost reduction. To ensure information security, this technology was proposed by [ 30 , 167 , 171 ] for agricultural product traceability. For example, in [ 171 ] an IoT solution uses blockchain to record information regarding the tea production based on 5 business processes: production plan, quality inspection, sales processing, product quality inspection and order delivery. In [ 167 ] a production tracking system for agricultural cooperatives have been developed. In [ 30 ] a similar system is being proposed but still in development stage.
5. Considerations
IoT solutions for smart farming take advantage of the scalability provided by platforms and cloud computing to store large amounts of data obtained by sensors. These big data of specific information may be processed with artificial intelligence techniques—such as machine learning—to improve the management of smart farming. For example, the processing of big data may be used to obtain crop insights, optimize water resources and increase the crop quality by preventing disease and reducing the amount of chemical products employed. Crop monitoring solutions use SBC (e.g., Arduino and Raspberry Pi) or UAV (e.g., drones) together with sensors (e.g., humidity, temperature, CO 2 or image) to collect data in indoor or outdoor environments.
Different types of network connections are used for communication between IoT devices, such as wired and wireless connections. The review shows that wired networks, such as CAN and Ethernet, are used for indoor agriculture (e.g., greenhouses). The use of wired network on indoor agriculture may be justified by the fact that in this scenario the physical components of the network are less susceptible to climatic agents impacts. Likewise, generally distance between sensor nodes in indoor agriculture enables this type of connection. Wireless connection, on the other hand, is used both in indoor and outdoor agriculture. Wi-Fi is the most mentioned protocol within the analyzed projects, due to its ubiquitous utilization in the daily life. However, power consumption and signal range characteristics may limit use of Wi-Fi in larger projects or in projects with power restrictions. To overcome the power consumption issue, energy-efficient protocols such as ZigBee, BLE or LoRa are used for communication in wireless networks.
Furthermore, it is worth mentioning that this review investigated papers where the IoT solution for smart farming was applied to agriculture only. However, the use of IoT for smart farming can also be applied to other activities related to farming, such as livestock [ 201 ]. Moreover, despite the fact that power-supply in IoT solutions for smart farming does not represent a specific layer of an IoT solution architecture [ 17 , 18 ], this topic has been covered in some of the reviewed papers. For example, [ 72 , 177 ] proposed improvements in algorithms for message routing and in duty cycles in sensor nodes. These approaches contribute to the reduction of power consumption by IoT devices. Similarly, a mission-based approach was used in [ 53 ] to optimize the power consumption in UAV. This approach was used to identify the most efficient path for a set of drones. Likewise, [ 177 ] proposed an intelligent activity cycle to improve the performance of data aggregators in terms of energy efficiency on cloudy days.
6. Conclusions
This work presented a systematic review of the state-of-the-art of IoT adoption in smart agriculture and identified the main components and applicability of IoT solutions. This review reported a change in the treatment of data in recent works: while previous work showed that the majority of decision support systems used simple processing mechanisms to handle data collected in real-time, more recent work showed an increasing number of management systems that use complementary technologies that rely on cloud and big data computing for processing large amounts of data. Furthermore, it was observed in this review that in recent work the use of artificial intelligence and image processing techniques has become more common to improve the management of smart farming. From the identified applications of IoT for smart farming it was observed that the most common application is the monitoring of crops. This review also showed that different network protocols may be simultaneously used in IoT solutions for smart farming. In addition, the comparison of types of network connections used in IoT solutions for smart farming revealed that wired networks are used in indoor scenarios (e.g., greenhouse) while wireless networks are used both in indoor and outdoor scenarios (e.g., arable lands, orchards). Moreover, the review discussed in this work suggests the increasing relevance of IoT solutions for smart farming. Future work may extend this review by including other relevant articles and complementary analysis of project costs, usability and regional challenges intrinsic to IoT applications. Another important future research direction could be the analysis of the edge and fog computing usage in smart agriculture as a way to deal with challenges associated with traditional centralized cloud solutions such as high communication latencies, lack of support for real-time reaction to detected events, large bandwidths, etc.
Acknowledgments
The authors acknowledge to the Mobile Computing Laboratory of the School of Technology and Management of the Polytechnic Institute of Leiria and to the Computer Science and Communication Research Center for the facilities granted in the implementation of part of this work, in the context of the Smart IoT Ecosystems research line.
Author Contributions
All authors contributed equally to this work. Conceptualization, E.N., N.C., A.P.; data curation, E.N., N.C., A.P.; formal analysis, N.C., A.P.; funding acquisition, A.P.; investigation, E.N., N.C., A.P.; methodology, N.C., A.P.; resources, A.P.; supervision, A.P.; validation, N.C.; writing—original draft, E.N., N.C.; writing—review & editing, E.N., N.C, A.P. All authors have read and agreed to the published version of the manuscript.
This work was partially supported by Portuguese Fundação para a Ciência e a Tecnologia–FCT, I.P., under the project UIDB/04524/2020” and by Portuguese National funds through FITEC–Programa Interface, with reference CIT “INOV–INESC Inovação–Financiamento Base”.
Conflicts of Interest
The authors declare no conflict of interest.
Agriculture’s connected future: How technology can yield new growth
The agriculture industry has radically transformed over the past 50 years. Advances in machinery have expanded the scale, speed, and productivity of farm equipment, leading to more efficient cultivation of more land. Seed, irrigation, and fertilizers also have vastly improved, helping farmers increase yields. Now, agriculture is in the early days of yet another revolution, at the heart of which lie data and connectivity. Artificial intelligence, analytics, connected sensors, and other emerging technologies could further increase yields, improve the efficiency of water and other inputs, and build sustainability and resilience across crop cultivation and animal husbandry.
The future of connectivity
As the world experiences a quantum leap in the speed and scope of digital connections, industries are gaining new and enhanced tools to boost productivity and spur innovation. Over the next decade, existing technologies like fiber, low-power wide-area networks (LPWAN), Wi-Fi 6, low- to mid-band 5G, and short-range connections like radio-frequency identification (RFID) will expand their reach as networks are built out and adoption grows. At the same time, new generations of these technologies will appear, with upgraded standards. In addition, new types of more revolutionary—and more capital-intensive—frontier connectivity, like high-band 5G and low-Earth-orbit (LEO) satellites, will begin to come online.
Together, these technological developments will unlock powerful new capabilities across industries. Near-global coverage will allow the expansion of use cases even to remote areas and will enable constant connectivity universally. Massive use of Internet of Things (IoT) applications and use cases will be enabled as new technologies allow very high device densities. And mission-critical services will take advantage of ultralow-latency, high-reliability, and high-security connections.
Without a solid connectivity infrastructure, however, none of this is possible. If connectivity is implemented successfully in agriculture, the industry could tack on $500 billion in additional value to the global gross domestic product by 2030, according to our research. This would amount to a 7 to 9 percent improvement from its expected total and would alleviate much of the present pressure on farmers. It is one of just seven sectors that, fueled by advanced connectivity, will contribute $2 trillion to $3 trillion in additional value to global GDP over the next decade, according to research by the McKinsey Center for Advanced Connectivity and the McKinsey Global Institute (MGI) (see sidebar “The future of connectivity”).
Demand for food is growing at the same time the supply side faces constraints in land and farming inputs. The world’s population is on track to reach 9.7 billion by 2050, 1 The World Population Prospects: 2015 Revision, United Nations, Department of Economic and Social Affairs, Population Division, 2015. requiring a corresponding 70 percent increase in calories available for consumption, even as the cost of the inputs needed to generate those calories is rising. 2 World Resources Report: Creating a Sustainable Food Future, United Nations, World Resources Institute, and the World Bank, 2013. By 2030, the water supply will fall 40 percent short of meeting global water needs, 3 World Could Face Water Availability Shortfall by 2030 if Current Trends Continue, Secretary-General Warns at Meeting of High-Level Panel, United Nations, 2016. and rising energy, labor, and nutrient costs are already pressuring profit margins. About one-quarter of arable land is degraded and needs significant restoration before it can again sustain crops at scale. 4 The State of the World’s Land and Water Resources for Food and Agriculture: Managing systems at risk, Food and Agriculture Organization of the United Nations and Earthscan, 2011. And then there are increasing environmental pressures, such as climate change and the economic impact of catastrophic weather events, and social pressures, including the push for more ethical and sustainable farm practices, such as higher standards for farm-animal welfare and reduced use of chemicals and water.
To address these forces poised to further roil the industry, agriculture must embrace a digital transformation enabled by connectivity. Yet agriculture remains less digitized compared with many other industries globally. Past advances were mostly mechanical, in the form of more powerful and efficient machinery, and genetic, in the form of more productive seed and fertilizers. Now much more sophisticated, digital tools are needed to deliver the next productivity leap. Some already exist to help farmers more efficiently and sustainably use resources, while more advanced ones are in development. These new technologies can upgrade decision making, allowing better risk and variability management to optimize yields and improve economics. Deployed in animal husbandry, they can enhance the well-being of livestock, addressing the growing concerns over animal welfare.
Demand for food is growing at the same time the supply side faces constraints in land and farming inputs.
But the industry confronts two significant obstacles. Some regions lack the necessary connectivity infrastructure, making development of it paramount. In regions that already have a connectivity infrastructure, farms have been slow to deploy digital tools because their impact has not been sufficiently proven.
The COVID-19 crisis has further intensified other challenges agriculture faces in five areas: efficiency, resilience, digitization, agility, and sustainability. Lower sales volumes have pressured margins, exacerbating the need for farmers to contain costs further. Gridlocked global supply chains have highlighted the importance of having more local providers, which could increase the resilience of smaller farms. In this global pandemic, heavy reliance on manual labor has further affected farms whose workforces face mobility restrictions. Additionally, significant environmental benefits from decreased travel and consumption during the crisis are likely to drive a desire for more local, sustainable sourcing, requiring producers to adjust long-standing practices. In short, the crisis has accentuated the necessity of more widespread digitization and automation, while suddenly shifting demand and sales channels have underscored the value of agile adaptation.
Current connectivity in agriculture
In recent years, many farmers have begun to consult data about essential variables like soil, crops, livestock, and weather. Yet few if any have had access to advanced digital tools that would help to turn these data into valuable, actionable insights. In less-developed regions, almost all farmwork is manual, involving little or no advanced connectivity or equipment.
Even in the United States, a pioneer country in connectivity, only about one-quarter of farms currently use any connected equipment or devices to access data, and that technology isn’t exactly state-of-the-art, running on 2G or 3G networks that telcos plan to dismantle or on very low-band IoT networks that are complicated and expensive to set up. In either case, those networks can support only a limited number of devices and lack the performance for real-time data transfer, which is essential to unlock the value of more advanced and complex use cases.
Nonetheless, current IoT technologies running on 3G and 4G cellular networks are in many cases sufficient to enable simpler use cases, such as advanced monitoring of crops and livestock. In the past, however, the cost of hardware was high, so the business case for implementing IoT in farming did not hold up. Today, device and hardware costs are dropping rapidly, and several providers now offer solutions at a price we believe will deliver a return in the first year of investment.
These simpler tools are not enough, though, to unlock all the potential value that connectivity holds for agriculture. To attain that, the industry must make full use of digital applications and analytics, which will require low latency, high bandwidth, high resiliency, and support for a density of devices offered by advanced and frontier connectivity technologies like LPWAN, 5G, and LEO satellites (Exhibit 1).
The challenge the industry is facing is thus twofold: infrastructure must be developed to enable the use of connectivity in farming, and where connectivity already exists, strong business cases must be made in order for solutions to be adopted. The good news is that connectivity coverage is increasing almost everywhere. By 2030, we expect advanced connectivity infrastructure of some type to cover roughly 80 percent of the world’s rural areas; the notable exception is Africa, where only a quarter of its area will be covered. The key, then, is to develop more—and more effective—digital tools for the industry and to foster widespread adoption of them.
As connectivity increasingly takes hold, these tools will enable new capabilities in agriculture:
- Massive Internet of Things. Low-power networks and cheaper sensors will set the stage for the IoT to scale up, enabling such use cases as precision irrigation of field crops, monitoring of large herds of livestock, and tracking of the use and performance of remote buildings and large fleets of machinery.
- Mission-critical services. Ultralow latency and improved stability of connections will foster confidence to run applications that demand absolute reliability and responsiveness, such as operating autonomous machinery and drones.
- Near-global coverage. If LEO satellites attain their potential, they will enable even the most remote rural areas of the world to use extensive digitization, which will enhance global farming productivity.
Connectivity’s potential for value creation
By the end of the decade, enhanced connectivity in agriculture could add more than $500 billion to global gross domestic product, a critical productivity improvement of 7 to 9 percent for the industry. 5 This represents our estimate of the total potential for value added in agricultural production; it is not an estimate of the agritech and precision-agriculture market size. Much of that value, however, will require investments in connectivity that today are largely absent from agriculture. Other industries already use technologies like LPWAN, cloud computing, and cheaper, better sensors requiring minimal hardware, which can significantly reduce the necessary investment. We have analyzed five use cases—crop monitoring, livestock monitoring, building and equipment management, drone farming, and autonomous farming machinery—where enhanced connectivity is already in the early stages of being used and is most likely to deliver the higher yields, lower costs, and greater resilience and sustainability that the industry needs to thrive in the 21st century (Exhibit 2).
It’s important to note that use cases do not apply equally across regions. For example, in North America, where yields are already fairly optimized, monitoring solutions do not have the same potential for value creation as in Asia or Africa, where there is much more room to improve productivity. Drones and autonomous machinery will deliver more impact to advanced markets, as technology will likely be more readily available there (Exhibit 3).
About the use-case research
The value of our agriculture-connectivity use cases resides primarily in labor efficiencies, input optimization, yield increases, reduced overhead, and improvements in operation and maintenance of machinery. Each use case enables a series of improvement levers in those areas that promise to enhance the productivity of farming (exhibit).
We applied those levers to the profitability drivers of agricultural production to derive an economic potential for the industry as a whole. For example, a use case might enable a 5 to 10 percent reduction in fertilizer usage, saving costs for the farmer, or enable 3 percent higher yields, leading to greater revenues for the farmer. In fact, higher yields represent the largest opportunity, with advanced connectivity potentially adding some $350 billion of value to global food production without additional inputs or labor costs.
Potential value initially will accrue to large farms that have more investing power and better incentives to digitize. Connectivity promises easier surveying of large tracts, and the fixed costs of developing IoT solutions are more easily offset in large production facilities than on small family farms. Crops like cereals, grains, fruits, and vegetables will generate most of the value we identified, for similar reasons. Connectivity enables more use cases in these sectors than in meat and dairy, because of the large average size of farms, relatively higher player consolidation, and better applicability of connected technologies, as IoT networks are especially adapted to static monitoring of many variables. It’s also interesting to note that Asia should garner about 60 percent of the total value simply because it produces the biggest volume of crops (see sidebar “About the use-case research”).
Use case 1: Crop monitoring
Connectivity offers a variety of ways to improve the observation and care of crops. Integrating weather data, irrigation, nutrient, and other systems could improve resource use and boost yields by more accurately identifying and predicting deficiencies. For instance, sensors deployed to monitor soil conditions could communicate via LPWAN, directing sprinklers to adjust water and nutrient application. Sensors could also deliver imagery from remote corners of fields to assist farmers in making more informed and timely decisions and getting early warnings of problems like disease or pests.
Smart monitoring could also help farmers optimize the harvesting window. Monitoring crops for quality characteristics—say, sugar content and fruit color—could help farmers maximize the revenue from their crops.
Most IoT networks today cannot support imagery transfer between devices, let alone autonomous imagery analysis, nor can they support high enough device numbers and density to monitor large fields accurately. Narrowband Internet of Things (NB-IoT) and 5G promise to solve these bandwidth and connection-density issues. The use of more and smoother connections between soil, farm equipment, and farm managers could unlock $130 billion to $175 billion in value by 2030.

Use case 2: Livestock monitoring
Preventing disease outbreaks and spotting animals in distress are critical in large-scale livestock management, where most animals are raised in close quarters on a regimen that ensures they move easily through a highly automated processing system. Chips and body sensors that measure temperature, pulse, and blood pressure, among other indicators, could detect illnesses early, preventing herd infection and improving food quality. Farmers are already using ear-tag technology from providers such as Smartbow (part of Zoetis) to monitor cows’ heat, health, and location, or technology from companies such as Allflex to implement comprehensive electronic tracing in case of disease outbreaks.
Similarly, environmental sensors could trigger automatic adjustments in ventilation or heating in barns, lessening distress and improving living conditions that increasingly concern consumers. Better monitoring of animal health and growth conditions could produce $70 billion to $90 billion in value by 2030.
Would you like to learn more about the McKinsey Center for Advanced Connectivity ?
Use case 3: building and equipment management.
Chips and sensors to monitor and measure levels of silos and warehouses could trigger automated reordering, reducing inventory costs for farmers, many of whom are already using such systems from companies like Blue Level Technologies. Similar tools could also improve shelf life of inputs and reduce post-harvest losses by monitoring and automatically optimizing storage conditions. Monitoring conditions and usage of buildings and equipment also has the potential to reduce energy consumption. Computer vision and sensors attached to equipment and connected to predictive-maintenance systems could decrease repair costs and extend machinery and equipment life.
Such solutions could achieve $40 billion to $60 billion in cost savings by 2030.
Use case 4: Farming by drone
Agriculture has been using drones for some two decades, with farmers around the world relying on pioneers like Yamaha’s RMAX remote-controlled helicopter to help with crop spraying. Now the next generation of drones is starting to impact the sector, with the ability to survey crops and herds over vast areas quickly and efficiently or as a relay system for ferrying real-time data to other connected equipment and installations. Drones also could use computer vision to analyze field conditions and deliver precise interventions like fertilizers, nutrients, and pesticides where crops most need them. Or they could plant seed in remote locations, lowering equipment and workforce costs. By reducing costs and improving yields, the use of drones could generate between $85 billion and $115 billion in value.
Use case 5: Autonomous farming machinery
More precise GPS controls paired with computer vision and sensors could advance the deployment of smart and autonomous farm machinery. Farmers could operate a variety of equipment on their field simultaneously and without human intervention, freeing up time and other resources. Autonomous machines are also more efficient and precise at working a field than human-operated ones, which could generate fuel savings and higher yields. Increasing the autonomy of machinery through better connectivity could create $50 billion to $60 billion of additional value by 2030.
Additional sources of value
Connected technologies offer an additional, indirect benefit, the value of which is not included in the estimates given in these use cases. The global farming industry is highly fragmented, with most labor done by individual farm owners. Particularly in Asia and Africa, few farms employ outside workers. On such farms, the adoption of connectivity solutions should free significant time for farmers, which they can use to farm additional land for pay or to pursue work outside the industry.
We find the value of deploying advanced connectivity on these farms to achieve such labor efficiencies represents almost $120 billion, bringing the total value of enhanced connectivity from direct and indirect outcomes to more than $620 billion by 2030. The extent to which this value will be captured, however, relies largely on advanced connectivity coverage, which is expected to be fairly low, around 25 percent, in Africa and poorer parts of Asia and Latin America. Achieving the critical mass of adopters needed to make a business case for deploying advanced connectivity also will be more difficult in those regions, where farming is more fragmented than in North America and Europe.
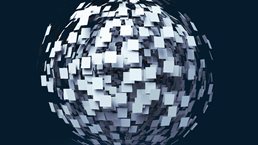
Connected world: An evolution in connectivity beyond the 5G revolution
Implications for the agricultural ecosystem.
As the agriculture industry digitizes, new pockets of value will likely be unlocked. To date, input providers selling seed, nutrients, pesticides, and equipment have played a critical role in the data ecosystem because of their close ties with farmers, their own knowledge of agronomy, and their track record of innovation. For example, one of the world’s largest fertilizer distributors now offers both fertilizing agents and software that analyzes field data to help farmers determine where to apply their fertilizers and in what quantity. Similarly, a large-equipment manufacturer is developing precision controls that make use of satellite imagery and vehicle-to-vehicle connections to improve the efficiency of field equipment.
Advanced connectivity does, however, give new players an opportunity to enter the space. For one thing, telcos and LPWAN providers have an essential role to play in installing the connectivity infrastructure needed to enable digital applications on farms. They could partner with public authorities and other agriculture players to develop public or private rural networks, capturing some of the new value in the process.
Agritech companies are another example of the new players coming into the agriculture sphere. They specialize in offering farmers innovative products that make use of technology and data to improve decision making and thereby increase yields and profits. Such agritech enterprises could proffer solutions and pricing models that reduce perceived risk for farmers—with, for example, subscription models that remove the initial investment burden and allow farmers to opt out at any time—likely leading to faster adoption of their products. An Italian agritech is doing this by offering to monitor irrigation and crop protection for wineries at a seasonal, per-acre fee inclusive of hardware installation, data collection and analysis, and decision support. Agritech also could partner with agribusinesses to develop solutions.
Still, much of this cannot happen until many rural areas get access to a high-speed broadband network. We envision three principal ways the necessary investment could take place to make this a reality:
- Telco-driven deployment. Though the economics of high-bandwidth rural networks have generally been poor, telcos could benefit from a sharp increase in rural demand for their bandwidth as farmers embrace advanced applications and integrated solutions.
- Provider-driven deployment. Input providers, with their existing industry knowledge and relationships, are probably best positioned to take the lead in connectivity-related investment. They could partner with telcos or LPWAN businesses to develop rural connectivity networks and then offer farmers business models integrating connected technology and product and decision support.
- Farmer-driven deployment. Farm owners, alone or in tandem with LPWAN groups or telcos, could also drive investment. This would require farmers to develop the knowledge and skills to gather and analyze data locally, rather than through third parties, which is no small hurdle. But farmers would retain more control over data.
How to do it
Regardless of which group drives the necessary investment for connectivity in agriculture, no single entity will be able to go it alone. All of these advances will require the industry’s main actors to embrace collaboration as an essential aspect of doing business. Going forward, winners in delivering connectivity to agriculture will need deep capabilities across various domains, ranging from knowledge of farm operations to advanced data analytics and the ability to offer solutions that integrate easily and smoothly with other platforms and adjacent industries. For example, data gathered by autonomous tractors should seamlessly flow to the computer controlling irrigation devices, which in turn should be able to use weather-station data to optimize irrigation plans.
Connectivity pioneers in the industry, however, have already started developing these new capabilities internally. Organizations prefer keeping proprietary data on operations internal for confidentiality and competitive reasons. This level of control also makes the data easier to analyze and helps the organization be more responsive to evolving client needs.
But developing new capabilities is not the end game. Agriculture players able to develop partnerships with telcos or LPWAN players will gain significant leverage in the new connected-agriculture ecosystem. Not only will they be able to procure connectivity hardware more easily and affordably through those partnerships, they will also be better positioned to develop close relationships with farmers as connectivity becomes a strategic issue. Input providers or distributors could thus find themselves in a connectivity race. If input providers manage to develop such partnerships, they could connect directly with farmers and cut out distributors entirely. If distributors win that race, they will consolidate their position in the value chain by remaining an essential intermediary, closer to the needs of farmers.
The public sector also could play a role by improving the economics of developing broadband networks, particularly in rural areas. For example, the German and Korean governments have played a major role in making network development more attractive by heavily subsidizing spectrum or providing tax breaks to telcos. 6 “Das Breitbandförderprogramm des Bundes” [in German], Bundesministerium für Verkehr und digitale Infrastruktur, 2020, bmvi.de; 5G in Korea: Volume 1: Get a taste of the future, Samsung Electronics, 2019, samsungnetworks.com. Other regions could replicate this model, accelerating development of connective products by cost-effectively giving input providers and agritech companies assurance of a backbone over which they could deliver services. Eventual deployment of LEO satellite constellations would likely have a similar impact.
Agriculture, one of the world’s oldest industries, finds itself at a technological crossroads. To handle increasing demand and several disruptive trends successfully, the industry will need to overcome the challenges to deploying advanced connectivity. This will require significant investment in infrastructure and a realignment of traditional roles. It is a huge but critical undertaking, with more than $500 billion in value at stake. The success and sustainability of one of the planet’s oldest industries may well depend on this technology transformation, and those that embrace it at the outset may be best positioned to thrive in agriculture’s connectivity-driven future.
Lutz Goedde is a senior partner and global leader of McKinsey’s Agriculture Practice in the Denver office; Joshua Katz is a partner in the Stamford office; and Alexandre Menard is a senior partner in the Paris office, where Julien Revellat is an associate partner.
The authors wish to thank Nicolas D. Estais, Claus Gerckens, Vincent Tourangeau, and the McKinsey Center for Advanced Connectivity for their contributions to the article.
This article was edited by Daniel Eisenberg, a senior editor in the New York office.
Explore a career with us
Related articles.

Agriculture supply-chain optimization and value creation
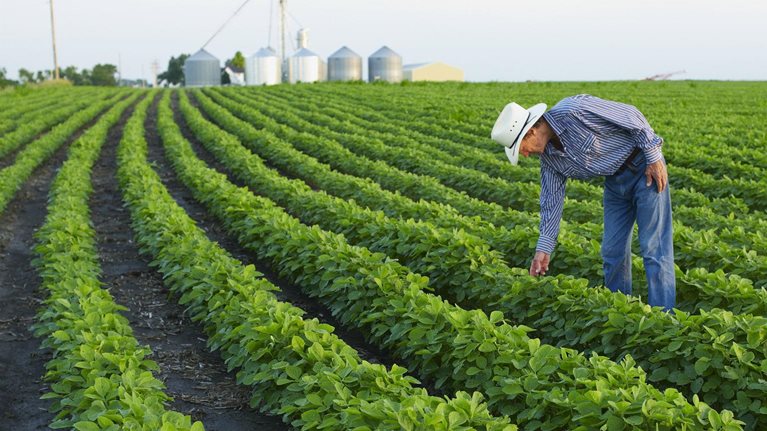
How to incentivize food systems to meet the realities of the 21st century
Accessibility Links
- Skip to content
- Skip to search IOPscience
- Skip to Journals list
- Accessibility help
- Accessibility Help
Click here to close this panel.
Purpose-led Publishing is a coalition of three not-for-profit publishers in the field of physical sciences: AIP Publishing, the American Physical Society and IOP Publishing.
Together, as publishers that will always put purpose above profit, we have defined a set of industry standards that underpin high-quality, ethical scholarly communications.
We are proudly declaring that science is our only shareholder.
Smart Agriculture and Smart Farming using IoT Technology
V Dankan Gowda 1 , M Sandeep Prabhu 2 , M Ramesha 3 , Jayashree M Kudari 4 and Ansuman Samal 5
Published under licence by IOP Publishing Ltd Journal of Physics: Conference Series , Volume 2089 , 1st International Conference on Applied Mathematics, Modeling and Simulation in Engineering (AMSE) 2021 15-16 September 2021, India (Virtual) Citation V Dankan Gowda et al 2021 J. Phys.: Conf. Ser. 2089 012038 DOI 10.1088/1742-6596/2089/1/012038
Article metrics
5916 Total downloads
Share this article
Author e-mails.
Author affiliations
1 Department of Electronics and Communication Engineering, B.M.S Institute of Technology and Management, Bangalore, Karnataka, India
2 Department of Electronics and Communication Engineering, Canara Engineering College, Benjanapadavu, Bantwal, Mangalore, Karnataka, India
3 Department of Electronics and Communication Engineering, GITAM School of Technology GITAM(Deemed to be University), Bangalore, Karnataka, India
4 Department of Computer Science and Information Technology, JAIN Deemed to be University, Bangalore, Karnataka, India
5 Faculty of Hospitality and Tourism Management, Siksha O Anusandhan Deemed to be University, Bhubaneshwar, Odisha, India
Buy this article in print
It has become easier to access agriculture data in recent years as a result of a decline in digital breaches between agricultural producers and IoT technologies. These future technologies can be used to boost productivity by cultivating food more sustainably while also preserving the environment, thanks to improved water use and input and treatment optimization. The Internet of Things (IoT) enables the production of agricultural process-supporting systems. Referred to as remote monitoring systems, decision support tools, automated irrigation systems, frost protection systems, and fertilisation systems, respectively. Farmers and researchers must be provided with a detailed understanding of IoT applications in agriculture as a result of the knowledge described above. This study is about using Internet of Things (IoT) technologies and techniques to enhance agriculture. This article is meant to serve as an introduction to IoT-based applications in agriculture by identifying need for such tools and explaining how they support agriculture.
Export citation and abstract BibTeX RIS
Content from this work may be used under the terms of the Creative Commons Attribution 3.0 licence . Any further distribution of this work must maintain attribution to the author(s) and the title of the work, journal citation and DOI.
- Understanding Poverty
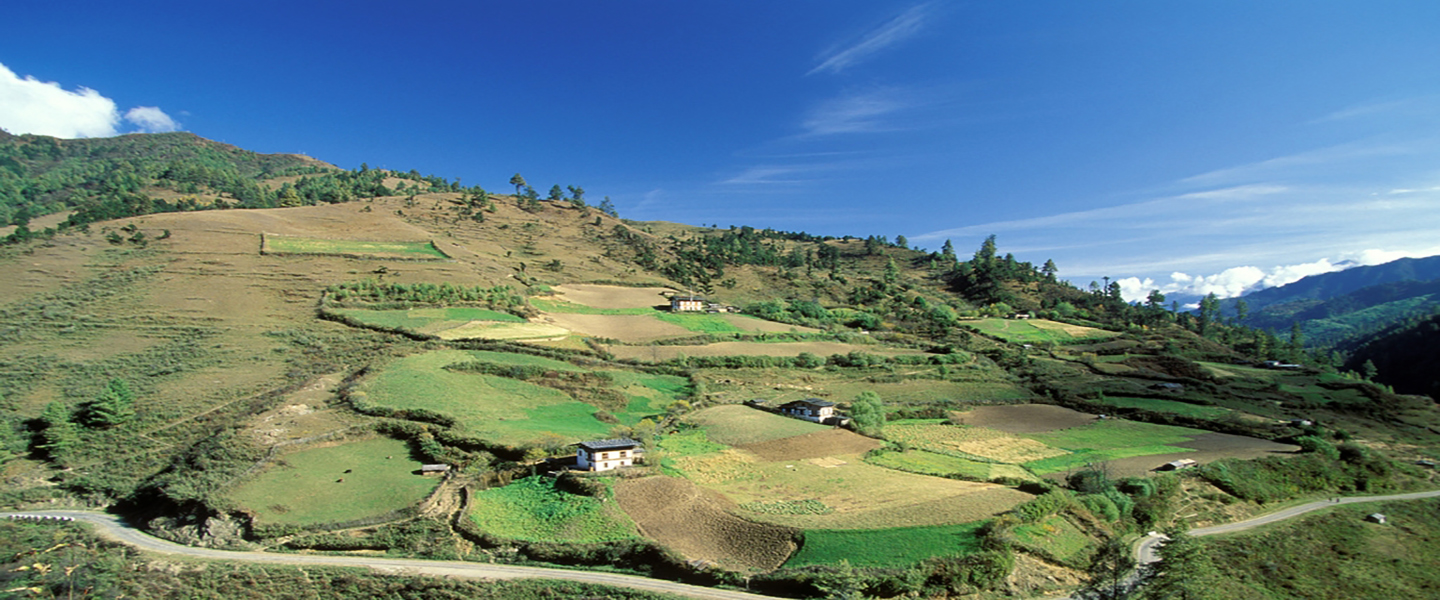
Climate-smart agriculture
Climate-smart agriculture (csa) is an integrated approach to managing landscapes—cropland, livestock, forests and fisheries--that address the interlinked challenges of food security and climate change..
Climate change and food and nutrition insecurity pose two of the greatest development challenges of our time. Yet a more sustainable food system can not only heal the planet, but ensure food security for all.
Today, the global agrifood system emits one-third of all emissions. Global food demand is estimated to increase to feed a projected global population of 9.7 billion people by 2050. Traditionally, the increase in food production has been linked to agricultural expansion, and unsustainable use of land and resources. This creates a vicious circle, leading to an increase in emissions.
Food systems are the leading source of methane emissions and biodiversity loss, and they use around 70% of fresh water. If food waste were a country, it would be the third highest emitter in the world. Meanwhile, emissions from agriculture are increasing in developing countries – a worrying trend which must be reversed.
Without significant climate mitigation action in the agri-food sector, the Paris Agreement goals cannot be reached. Agriculture is the primary cause of deforestation, threatening pristine ecosystems such as the Amazon and the Congo Basin. Without action, emissions from food systems will rise even further, with increasing food production.
Achieving the Triple Win of CSA
The global agrifood system must therefore deliver on multiple fronts. It must feed the world, adapt to climate change, and drastically reduce its greenhouse gas emissions. In response to these challenges, the concept of Climate-smart Agriculture (CSA) has emerged as a holistic approach to end food security and promote sustainable development while addressing climate change issues.
CSA is a set of agricultural practices and technologies which simultaneously boost productivity, enhance resilience and reduce GHG emissions. Although it is built on existing agricultural knowledge, technologies, and sustainability principles, CSA is distinct in several ways. First, it has an explicit focus on addressing climate change in the agrifood system. Second, CSA systematically considers the synergies and tradeoffs that exist between productivity, adaptation, and mitigation. And third, CSA encompasses a range of practices and technologies that are tailored to specific agro-ecological conditions and socio-economic contexts including the adoption of climate-resilient crop varieties, conservation agriculture techniques, agroforestry, precision farming, water management strategies, and improved livestock management. By implementing these practices, triple win results can be achieved:
1. Increased productivity: Produce more and higher quality food without putting an additional strain on natural resources, to improve nutrition security and boost incomes, especially for 75 percent of the world’s poor who live in rural areas and mainly rely on agriculture for their livelihoods.
2. Enhanced resilience: Reduce vulnerability to droughts, pests, diseases and other climate-related risks and shocks; and improve the capacity to adapt and grow in the face of longer-term stresses like increased seasonal variability and more erratic weather patterns.
3. Reduced emissions: Reduce greenhouse gas emissions of the food system, avoid deforestation due to cropland expansion, and increase the carbon sequestration of plants and soils.
Finally, funding for CSA needs to be increased to align available finance with the relevance of the sector. Despite causing one third of global greenhouse gas emissions, agrifood systems receive 4% of climate finance, with only a fifth of this going to smallholders. Current financial flows need to be realigned in order to support a sustainable agrifood system transformation.
Climate-Smart Agriculture and the World Bank Group
The World Bank has significantly scaled up its engagement and investment in climate-smart agriculture (CSA). In its Climate Change Action Plan (2021- 2025), the World Bank has identified Agriculture, Food, Water and Land as one of the five key transitions needed to tackle the Paris Agreement. Since the adoption of the Paris Agreement, the World Bank has increased financing for CSA by eight times, to almost $3 billion annually.
As of July 2023, all new World Bank operations must be aligned with the goals of the Paris Agreement , meaning that CSA is at the core of all the World Bank’s new agriculture and food operations. To this end, the World Bank has prepared a Sector Note of Paris Alignment of its Agriculture and Food operations. Furthermore, all projects are screened for climate and disaster risks. Climate change indicators are used to measure outputs and outcomes, and greenhouse gas accounting of projects is conducted prior to approval . These actions will help client countries implement their Nationally Determined Contributions (NDCs) in the agriculture sector, and will contribute to progress on the Sustainable Development Goals (SDGs) for climate action, poverty, and the eradication of hunger.
The World Bank engages strategically with countries, supporting them to enhance productivity, improve resilience and reduce greenhouse gas emissions. The World Bank uses the following tools, diagnostics and other analytics to help countries in the transition towards sustainable agriculture.
- Country Climate and Development Reports (CCDRs), new core diagnostics, help countries prioritize the most impactful actions that can reduce greenhouse gas emissions and boost adaptation, while delivering on broader development goals. CCDRs identify climate impacts on countries’ agrifood systems, such as reduced yields and increased food prices, and present a variety of country-specific technology options as well as policy reforms under the umbrella of CSA.
- Climate-Smart Agriculture (CSA) Country Profiles developed by the World Bank and partners, give an overview of the agricultural challenges in countries around the world, and how CSA can help them adapt to and mitigate climate change. They bridge knowledge gaps by providing clarity on CSA terminology, components, relevant issues, and how to contextualize them under different country conditions.
- Climate-Smart Agriculture Investment Plans (CSAIPs) developed for a subset of client countries aim to mainstream CSA into national agricultural policies and to identify investment opportunities in CSA. The World Bank provides technical assistance and financial support to help countries develop and implement CSAIPs. These plans prioritize investments in climate-resilient infrastructure, capacity building, and knowledge sharing to promote sustainable agricultural practices. CSAIPs are available, or currently under preparation, for Bangladesh , Belize, Burkina Faso, Cote D’Ivoire , Cameroon, the Republic of Congo, Ethiopia, Ghana , Iraq, Jordan, Kenya, Lesotho , Madagascar, Mali , Morocco , Nepal , Senegal, Zambia , and Zimbabwe .
- The World Bank also supports research programs such as with the CGIAR , which develops and supports climate-smart technologies and management methods, early warning systems, risk insurance, and other innovations that promote resilience and combat climate change.”
Working Toward Resilience and Food and Nutrition Security, while Curbing GHG Emissions
The Bank’s support of CSA is making a difference across the globe, for example:
- A new US$345 million loan for the China Green Agricultural and Rural Revitalization Program for Results will support China’s global public goods agenda by promoting the greening of agriculture and rural development in Hubei and Hunan provinces in central China. The program will reduce greenhouse gas (GHG) emissions from crop and livestock farming, increase carbon sequestration in farmlands, and improve biodiversity protection and restoration in agricultural ecosystems, while strengthening the institutional capacity of local governments to integrate environmental and decarbonization objectives in government rural revitalization plans and investments. World Bank financing will complement a US$4.1 billion commitment by the Government of China (GoC).
- The US$621 million Food Systems Resilience Program for Eastern and Southern Africa (Phase 3) FSRP Project in Kenya, Comoros, Malawi, Somalia aims to increase the resilience of food systems and the recipients’ preparedness for food insecurity. The project has six components, including building resilient agricultural production capacity to strengthen the productivity and resilience of domestic food production to shocks and stressors, by supporting the development and adoption of improved agricultural inputs and services and climate-smart and gender-sensitive farming technologies in the crops, livestock, and fisheries sectors.
- A US$200 million credit for the Punjab Resilient and Inclusive Agriculture Transformation Project (PRIAT) will help Pakistan enhance access to, and productivity of, agricultural water, and improve incomes of farmers supported by the project. PRIAT will notably reduce the differences in water availability among head, middle, and tail end users of watercourses, increase agricultural output per unit of water used at farm level for selected crops, increase the share of area under high-value crops cultivation, and increase agriculture incomes of households participating in project activities, yielding important climate change adaptation and mitigation co-benefits.
- The US$125 million Agriculture Resilience, Value Chain Development and Innovation (ARDI) program will play a pivotal role in strengthening the transition Jordan’s agri-food sector. It supports Jordan's National Sustainable Agriculture Plan and aims to enhance climate resilience, competitiveness, and inclusivity of the agri-food sector. Over the next five years, it will support 30,000 farming household with the adoption of climate-smart and water-efficient agricultural practices, provide needs-based training, create about 12,000 employment opportunities, and promote value chain and export promotion through advanced market diagnostics. A particular focus will be on strengthening the participation of women, youth and refugees.
Last Updated: Feb 26, 2024
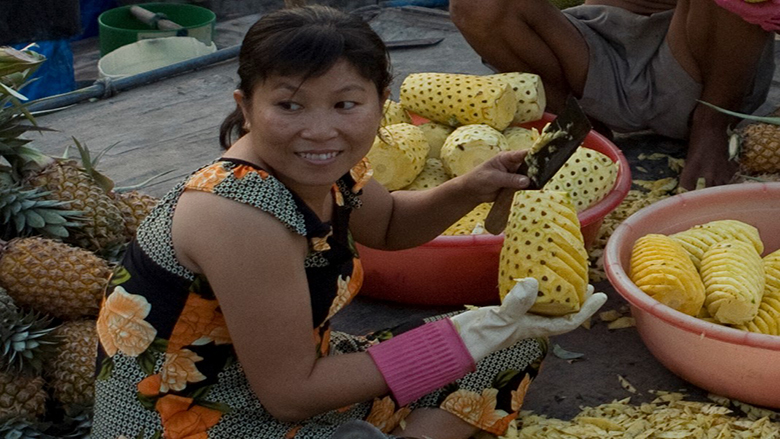
Agriculture and Food
Go back to main topic.
- 'Recipe for a Livable Planet' report launch event, May 22 - Watch live stream
- Recipe for a Livable Planet: Achieving Net Zero Emissions in the Agrifood System
- Climate-Smart Agriculture Country Profiles
- Climate-Smart Agriculture Investment Plans
- Future of Food: Shaping a Climate-Smart Global Food System
- Podcast: Can the World Feed Itself as the Climate Changes?
- Brief: Livestock and Sustainability at the World Bank
- Bringing the Concept of Climate-Smart Agriculture to Life
A Better Food System for Healthier People, Planet and Economy
Advertisement
Smart greenhouses as the path towards precision agriculture in the food-energy and water nexus: case study of Qatar
- Open access
- Published: 02 June 2022
- Volume 42 , pages 521–546, ( 2022 )
Cite this article
You have full access to this open access article
- Theodora Karanisa 1 ,
- Yasmine Achour 2 ,
- Ahmed Ouammi 1 &
- Sami Sayadi 1
15k Accesses
24 Citations
4 Altmetric
Explore all metrics
Greenhouse farming is essential in increasing domestic crop production in countries with limited resources and a harsh climate like Qatar. Smart greenhouse development is even more important to overcome these limitations and achieve high levels of food security. While the main aim of greenhouses is to offer an appropriate environment for high-yield production while protecting crops from adverse climate conditions, smart greenhouses provide precise regulation and control of the microclimate variables by utilizing the latest control techniques, advanced metering and communication infrastructures, and smart management systems thus providing the optimal environment for crop development. However, due to the development of information technology, greenhouses are undergoing a big transformation. In fact, the new generation of greenhouses has gone from simple constructions to sophisticated factories that drive agricultural production at the minimum possible cost. The main objective of this paper is to present a comprehensive understanding framework of the actual greenhouse development in Qatar, so as to be able to support the transition to sustainable precision agriculture. Qatar’s greenhouse market is a dynamic sector, and it is expected to mark double-digit growth by 2025. Thus, this study may offer effective supporting information to decision and policy makers, professionals, and end-users in introducing new technologies and taking advantage of monitoring techniques, artificial intelligence, and communication infrastructure in the agriculture sector by adopting smart greenhouses, consequently enhancing the Food-Energy-Water Nexus resilience and sustainable development. Furthermore, an analysis of the actual agriculture situation in Qatar is provided by examining its potential development regarding the existing drivers and barriers. Finally, the study presents the policy measures already implemented in Qatar and analyses the future development of the local greenhouse sector in terms of sustainability and resource-saving perspective and its penetration into Qatar’s economy.
Similar content being viewed by others
Smart Greenhouse Development: A Case Study in West Java, Indonesia
Energy-Efficient Wireless Sensor Networks for Greenhouse Management
Vertical Greenhouses in the Arctic
Avoid common mistakes on your manuscript.
1 Introduction
Food security is a complex multidimensional phenomenon encompassed by the key dimensions of food availability, access, utilization, and stability of food supply for all people (FAO 2010 ). A country’s food security status is the sum of its citizens’ food security situation. Thus, food security is a global concern facing nowadays many challenges such as climate change, population growth, economic development, and the change in diet which consequently are putting pressure on resource availability (FAO 2020a ). Climate change resulting in different weather patterns can threaten food production as both plant and animal productivity are affected. Crop yields are reduced, infectious diseases and pests are prevailing, frequent occurrence of environmental contaminants and chemical residues are observed along the food chain hampering food safety and security (FAO 2020b ). As stated by the United Nations, the world’s population is expected to increase by 2 billion people in the next decades, from 7.7 billion in 2020 to 9.7 billion in 2050 (United Nations Homepage 2020a ) with a subsequent required increase of 60% in food production by then (FAO 2020a ). However, an estimated 821 million people worldwide suffered from hunger in 2018, making hunger and malnutrition among the biggest risks to health. From a wider perspective, it is assessed that more than 2 billion people do not have consistent access to safe, nutritious, and sufficient food, including not only those suffering from hunger but also those who are affected by moderate levels of food insecurity (United Nations Homepage 2020b ). Agricultural food production is a resource-intensive activity accounting for 70% of global water withdrawal including the need for irrigation, livestock, and aquaculture (FAO Aquastat Homepage 2020 ). Besides, approximately 75% of all industrial water withdrawals were used for energy production in 2014 (United Nations Homepage 2020c ) and 25% of the energy used worldwide is utilized for food production and supply (FAO 2020a ). Therefore, it is evident that traditional agricultural systems are insufficient to meet the increasing food demand. Consequently, there is an urgent need for a transition to sustainable and precision agriculture taking advantage of the technological progress to increase crop productivity while preserving the existing resources. Controlled Environment Agriculture (CEA) and smart greenhouses are key solutions to overcome these challenges as they can optimize crop production by manipulating the indoor climate while mitigating the climate change effect (Ouammi et al. 2020a ). Moreover, they offer effective energy and water management solutions optimizing resource input while there have been recently developed innovative systems reaching nearly zero energy consumption and pesticide use with minimum water consumption (Bersani et al. 2020 ). Finally, the smart grid can play a significant role in enhancing the sustainable energy supply (Ouammi et al. 2020b ). The term Controlled Environment Agriculture (CEA) refers to the growth and development of plants in a fully controlled environment with the use of modern horticultural techniques and technological advances resulting in achieving higher yields and improving product quality (Gómez et al. 2019 ). On the other hand, greenhouses are structures with transparent materials in which the microclimatic parameters are also modified to enhance plant growth and productivity and ensure all-year-round production (Shrawan Singh et al. 2008 ). CEA is a broader term that includes greenhouses, rooftop greenhouses, growth chambers, plant factories as well as vertical farms. This paper focuses on the technological advances in the greenhouse sector worldwide as well as in Qatar, as food production in the country is mainly realized in open fields and greenhouses.
Qatar is a small country in terms of land area located in the Arabian Gulf with an arid desert climate. The available land and resources are limited; therefore, the country was importing 90% of its food basket products until 2017 (Miniaoui et al. 2018 ). Agricultural food production in Qatar faces many challenges such as scarce freshwater, limited arable land and a climate that is not favoring agriculture as the summers are long, hot, and humid with low annual rainfall and often dust storms. Nonetheless, food security is a national priority for the State of Qatar and the government has launched many initiatives to boost local agricultural production while reducing its import dependency. Therefore, in the last decade between 2010 and 2019, the greenhouse and open field production of the most consumed vegetables in the country has increased from 31.573 tons to 66.500 tons, recording an approximate 110% increase (Planning and Statistics Authority in Qatar 2020a , 2014 ). Moreover, the Qatar greenhouse market is a developing sector, expected to mark double-digit growth during the near future and reach a value of approximately 259 million US$ by 2025 (Expert market research Homepage 2020 ).
The research method of this study includes a thorough review of the scholarly literature as well as data from gray literature (e.g., reports, discussion articles, policy documents) and databases (e.g., FAOSTAT, World Bank) from Qatar and other countries. The search included all the documents that were indexed up to the year 2015, so only recent studies were considered on the development of greenhouses and technologies worldwide as well as for the greenhouse sector development in Qatar.
Hence, the present paper provides an overview of the current status of greenhouse technology, aiming to offer effective supporting information to decision and policymakers as well as overall professionals and end-users in introducing new technologies and benefiting from the advances in monitoring techniques, artificial intelligence and communication infrastructure in the agricultural sector. Then, it focuses on Qatar, with the main objective to present a comprehensive understanding framework of the actual greenhouse development in the country, able to support the transition to sustainable precision agriculture meeting the country’s food security and sustainability goals.
Specifically, this review paper:
analyzes the actual development of greenhouses and technologies worldwide (Sect. 2 );
elaborates on smart greenhouses in the food-energy-water nexus focusing on the energy and water resources (Sect. 3 );
gives an overview of the greenhouse sector development in Qatar by analyzing the actual agriculture situation and examining its potential development regarding the existing drivers and barriers (Sect. 4 ); and
presents the policy measures already implemented in Qatar while analyzing the future development of the local greenhouse sector in terms of sustainability and resources saving perspective as well as its penetration into Qatar’s economy (Sect. 5 ).
2 Actual development of greenhouses and technologies
Global challenges linked to population growth, urbanization, and climate change have led to bringing innovative features to conventional greenhouse cultivation techniques (Specht et al. 2019 ). In fact, over the past few years, the greenhouse industry has been transformed to include modern technologies allowing farmers to grow a consistent product all year round using far fewer resources than conventional production (Tuomisto 2019 ). The main part of this transformation is the introduction of vertical systems that have been proposed as a concept to address the issue of sustainability (Graamans et al. 2018 ) and as a solution to improving food production involving land and water use optimization (Kalantari et al. 2020 ; Benke and Tomkins 2017 ; Bao et al. 2018 ; Yeşil and Tatar 2020 ). Vertical systems consist of growing crops in vertically stacked layers under a protected environment to limit the use of the agricultural land and therefore the ecological footprint of agriculture (Anda and Shear 2017 ). This concept, in addition to other major advances, has not only transformed the greenhouse industry but also led to new farming methods such as hydroponics (Al-Kodmany 2018 ), as well as the introduction and widespread adoption of full automation technologies and advanced lighting techniques.
2.1 Full automation technologies
Currently, greenhouse crop production is facing increased demand for automation and robotics. In fact, although the initial reason for using greenhouses was to grow crops in controlled environments, today the integration of smart systems can reduce reliance on labor and increase profitability while ensuring efficiency and sustainability. In this context, enormous progress has been made in the automation of greenhouses, mainly based on environmental sensors that play a key role in the programmed operation. Indeed, smart greenhouse solutions incorporating advanced sensor technologies have become widely used for crop growth environment monitoring. For example, different sensors of various environmental parameters have been used to support a greenhouse monitoring system consisting of a Programmable Logic Controller (PLC) and a Supervisory Control and Data Acquisition (SCADA) system which are different layers of automation in a system known as the Automation Pyramid (AP) (Ding et al. 2018 ). The different sensors and machines can communicate with each other by exchanging data, the so-called Cyber-Physical Production Systems (CPPS). These systems can fit into the existing farm decision-making tools and particular in the production processes as the database and information they offer is precise and constantly updated thus creating transparency in multifactorial decisions. A camera has been also used as part of the imaging platform of a greenhouse powdery mildew detection machine vision system (Wspanialy and Moussa 2016 ). Furthermore, huge steps forward have been also made in the field of Wireless Sensor Networks (WSN) as an effective solution to many problems associated with the conventional cabled sensors (Oliveira et al. 2016 ; Cenedese et al. 2012 ). Moreover, WSN has been developed under the Internet of Things (IoT) paradigm, by integrating web-based technologies, providing thus an interface to view the greenhouse status and remotely control the environmental parameters (Li et al. 2017a ; Akkaş and Sokullu 2017 ; Danita et al. 2018 ). As an illustration, different sensors ranging from simple (temperature, humidity, pH, illumination, pressure, UV, CO 2 , wind speed, solar radiation (Jahnavi and Ahamed 2015 ; Thirukkuralkani et al. 2018 ; Jiang et al. 2016 ; Erazo-Rodas et al. 2018 ) to complex [cameras (Hwang and Yoe 2016 ), mid-infrared spectroscopy (Wang et al. 2016 )] have been used, and their measurements were communicated wirelessly to the host system using several technologies such as Zigbee (Chen et al. 2016a , 2017 ; Xing et al. 2017 ; Raheemah et al. 2016 ; Pascual et al. 2015 ; Luo et al. 2016 ; Liu and Bi 2017 ; Aiello et al. 2018 ; Rao et al. 2016 ; Ismail et al. 2016 ; Ibayashi et al. 2016 ; Erazo, et al. 2015 ; Çaylı et al. 2017 ), radio (Liang-Ying and Zhao-Wei, 2015 ; Achour et al. 2018 ; Mahbub 2020 ), GSM/GPRS (Liang-Ying and Zhao-Wei, 2015 ; Liu and Zhang 2017 ; Navarro-Hellín et al. 2015 ; Mat et al. 2016 ), 3G/4G (Zhou and Duan 2016 ; Chung et al. 2015 ; Zhang et al. 2015 ), Wi-Fi (Aiello et al. 2018 ; Chung et al. 2015 ; Mohapatra and Lenka 2016 ; Liang et al. 2018a ) and Bluetooth (Hong and Hsieh 2016 ; Taşkın et al. 2018 ). However, to keep the greenhouse automation trouble-free, a secure protocol for wireless sensor networks has been proposed in the automated agricultural environment and has allowed more reliability (Sivamani et al. 2018 ).
The WSN’s sensed data are processed and then used as multi-inputs to drive advanced control algorithms-based artificial intelligence to manage intelligently the greenhouse internal microclimate and to optimize the use of water and energy. These controllers can also vary from simple, such as feedback controllers (Mekki et al. 2015 ; Maher et al. 2016 ; Pawlowski et al. 2017 ), to modern ones such as fuzzy logic controllers (Rahmawati et al. 2018 ; Alpay and Erdem 2018 ; Xu et al. 2016 ; Pahuja et al. 2015 ; Márquez-Vera et al. 2016 ; Chen et al. 2016b ; Wang and Zhang 2018 ; Li et al. 2017b , 2018 ; Ben Ali et al. 2018 ; Faouzi et al. 2017 ; Carrasquilla-Batista and Chacon-Rodrıguez 2017 ), artificial neural networks (Nicolosi et al. 2017 ; Huang et al. 2018 ; Francik and Kurpaska 2020 ; Singh 2017 ; Hongkang et al. 2018 ; Moon et al. 2018 ; Taki et al. 2016 ), genetic algorithms (Wang et al. 2017a ; Mahdavian and Wattanapongsakorn 2017 ), model predictive controllers (Ouammi et al. 2020b ; Liang et al. 2018b ; Hamza and Ramdani 2020 ), sliding controllers (Khelifa et al. 2020 ; Oubehar, et al. 2016 ), adaptative controllers (Essahafi and Lafkih 2018 ), frequency controllers (Bagheri Sanjareh et al. 2021 ), and receding horizon controllers based on prioritized multi-operational ranges (Singhal et al. 2020 ).
Nevertheless, although WSN has been widely implemented as an automation technology offering numerous benefits, the research on applied robotics has also grown recently attaching great importance to mobile sensing as a sustainable solution for cost improvement (Li et al. 2019a ; Roldán et al. 2016 ). On this basis, an autonomous mobile robot was developed to map the greenhouse and collect humidity, temperature, and light data (Durmus et al. 2016 ). Another improved robot, comprising ground and aerial vehicles, has been proposed to measure the temperature, humidity, luminosity, and carbon dioxide concentration in the ground and at different heights of the greenhouse (Roldán et al. 2016 ). However, robotic systems have not been used only for environmental monitoring, but also to perform greenhouse unsafe tasks like the UV-C treatment against powdery mildew (Mazar et al. 2018 ). In fact, robotic systems have been designed to help labor tasks such as pesticide spraying with path-planning and traveling-control abilities (Nakao et al. 2017 ; Mahmud et al. 2019 ), transplanting (Han et al. 2018 ), fertilizing (Yuan et al. 2016 ), phenotyping (Atefi et al. 2019 ) and harvesting (Wang et al. 2017b ; Arad et al. 2020 ; Woo et al. 2020 ). In addition, they have been tightly related to image-based detection algorithms and near-infrared spectroscopy, and therefore, to crop disease detection (Rizk and Habib 2018 ; Schor et al. 2016 , 2017 ), classification and picking (Zhao et al. 2016 ; Feng et al. 2015 ). But despite the fast development of these greenhouse robotic systems, there are several research challenges concerning the supervision, control and optimization of robots that must be addressed to ensure the competitiveness of this sector. Several steps have been made towards optimizing robots’ efficacy, for example, heuristic and genetic algorithms have been implemented to reduce the duration of type-c ultraviolet radiation treatment performed by a battery-powered robot (Mazar et al. 2020 ). Moreover, a green pepper recognition method based on the least-squares support vector machine optimized by the improved particle swarm optimization (IPSO-LSSVM) has been proposed (Ji et al. 2019 ). And finally, a greenhouse layout optimization system that enables rapid and safe robot navigation has been developed (Uyeh et al. 2019 ).
2.2 Hydroponics
The introduction of hydroponics may be taken as a significant advance in modern greenhouses, where crops are grown in a soil-free environment, using only mineral nutrient solutions in water. The hydroponic techniques used to this end are mainly the deep flow technique (DFT) and nutrient film technique (NFT) (Son et al. 2020 ). Regarding the first one, panels are floating in a container filled with nutrient solution (10–20 cm deep), while for the second, a nutrient solution flows from the upper end to the lower end and passes through troughs of about 10 cm placed on trays at a slope of 0.5–2%. Generally, the choice of the DFT or NFT hydroponic system does not lead to important changes under greenhouse conditions (Santos et al. 2019 ). Nevertheless, DFT allowed an increase of 2.6 g in fresh weight of fresh basil compared to plants grown in NFT systems (Walters and Currey 2015 ).
Overall, compared to conventional greenhouses, hydroponics offers several advantages such as: improving the quality and nutrient content, increasing yield and conserving water. It has been documented in the literature that various plant species have been found to have better characteristics when grown hydroponically. For example, hydroponic strawberries were higher in terms of fruit yield and plant survival rate compared to systems traditionally grown in soil (Treftz and Omaye 2016 ). In addition, for hydroponic lettuce, the technique has offered 11 ± 1.7 times higher yields and 13 ± 2.7 times less water, but required 82 ± 11 times more energy compared to conventionally produced lettuce (Barbosa et al. 2015 ). However, to deal with the high-energy consumption issue, an innovative hydroponic greenhouse and a new solar air heater with latent storage energy have been assessed and have allowed an annual energy production reduction of over 4600 kWh. The innovation of this work is to guarantee an enhanced hydroponic greenhouse microclimate through a pilot unit with latent thermal storage using exclusively solar energy (Baddadi et al. 2019 ).
2.3 Advanced lighting techniques
The adoption of supplemental artificial lighting systems is a key solution providing important progress in modern greenhouses promoting agricultural production. Commonly, high-pressure sodium (HPS) lamps have been widely used as the main supplemental light source in greenhouses, because of their high electrical efficiency and high photosynthetically active radiation emission (Viršilė et al. 2017 ). However, recent developments have given rise to light-emitting diode (LED) due to their technological advantages, especially in terms of spectral output, light distribution, heat emission, lifespan, and control capabilities (Iersel 2017 ; Alsanius et al. 2017 ). In the literature, numerous comparative studies have been carried out to assess the effectiveness of each technology. For instance, three are different types of lighting, namely HPS standard lighting, HPS top lighting and LED inter-lighting, and 100% LED lighting have been tested in a lettuce greenhouse, and the results indicated that the efficiency of light use was the highest in the 100% LED combination and the lowest in HPS (Kowalczyk et al. 2020 ). Also, a comparison of 1000 W HPS lighting system versus 650 W LED lighting system has shown that LED lighting has 38%-47% less environmental impact in terms of global warming, ozone depletion, and acidification than HPS lighting system (Zhang et al. 2017 ). In fact, supplemental lighting by light-emitting diodes (LED) has shown further benefits in other studies, such as improving water use efficiency of greenhouse crops (Li et al. 2019b ), increasing fruit weight and size (Paucek et al. 2020 ), enhancing lettuce growth and yield (Zhang et al. 2019 ) and reducing energy consumption (Wang and Wei 1956 ). In addition, extra studies have been conducted to determine the effects of LED colors on crop production, such as for lettuce where supplemental lighting with 80% red and 20% blue light has proven to be advantageous (Długosz-Grochowska et al. 2017 ), as well as for the artichoke where seedlings under red light condition were much better (Rabara et al. 2017 ). Furthermore, another important step consistent with the research is the design of an intelligent hybrid system for greenhouse plants by 3D supplementary lighting, which can be auto switched to sunlight or LED depending on weather conditions. This last has shown the ability to accelerate vegetables and fruits’ growth rate (Tang et al. 2019 ).
3 Smart greenhouses in the food-energy-water nexus
Due to the intensive exploitation of energy, water, and food resources, induced by population growth and rapid urbanization, increasing interest has been given to the preservation of these resources and leading to the introduction of the food-energy-water nexus (FEW) as an approach to the sustainable development of agriculture among other practices (Li et al. 2019c ). The FEW nexus is a holistic approach to the resources of food, energy and water examining their interrelatedness and interdependencies rather than considering each resource individually (United Nations University 2022 ). Hence, sustainable FEW systems can be defined as systems that can meet their food, energy, and water demands with sustainable sources rather than using non-renewable inputs. They aim at effective management of natural resources, optimization of potential conflicts and reinforcement of multisectoral integration (Lahlou et al. 2020 ; El-gafy 2017 ). In this context, agricultural technologies like smart greenhouses have been increasingly encouraged as means to achieve regional food security and reduce the stress on water and energy resources. They can be considered as FEW systems able to yield food while minimizing energy and water consumption and maximizing economic productivity (Karan et al. 2018 ).
As part of this paradigm, a decision-making framework based on the approach of the FEW nexus for the development of new greenhouses has been introduced and has led to a reduction in CO 2 emissions and an economy of water resources (Al-Ansari et al. 2018 ). In addition, a novel decision-making methodology in greenhouse cultivation has been proposed using the FEW nexus approaches, which assesses the investment and operating costs and the potential for global warming, for food production based on economic and environmental performance (Namany et al. 2019 ). Moreover, an approach based on both GIS, analytical hierarchy process and resource assessment has been proposed to improve water and energy efficiencies (Haji et al. 2020 ). However, the performance of the FEW nexus has been conventionally analyzed through energy and water indicators.
3.1 Energy considerations in smart greenhouses
From the energy viewpoint, smart greenhouses focus consideration on integrating different types of renewable energy to support sustainable growth. Solar energy remains the primary renewable source used to generate electricity in greenhouses to economically meet the electrical load of any actuating machine. Solar technologies used for this purpose can be divided into three categories: photovoltaic systems, solar thermal collectors, and hybrid photovoltaic/thermal (PV/T) modules. Classically, solar thermal collectors have been adopted in greenhouses to accumulate the maximum amount of solar energy (Patil and Gawande 2016 ; Awani et al. 2015 ), especially for drying purposes, where their use has allowed a remarkable reduction in drying hours from 24 to 17 h for red pepper compared to open sun drying (ELkhadraoui et al. 2015 ). As for photovoltaic systems, they have also been highly integrated into greenhouse applications to cater in a clean way to energy-consuming activity (Trypanagnostopoulos et al. 2017 ; Marucci and Cappuccini 2016 ; Fatnassi et al. 2015 ; Marucci et al. 2018 ; Gao et al. 2019 ). Moreover, by combining these two technologies, photovoltaic/thermal (PV/T) systems have gained popularity by increasing the use of solar energy (Good 2016 ; Achour and Zejli 2018 ; Kim et al. 2016 ). They have been used for several greenhouse applications such as crop drying (Tiwari and Tiwari 2016a , b ; Shyam et al. 2015 ; Tiwari et al. 2018 , 2016a ) and biogas heating (Tiwari and Tiwari 2017 ; Tiwari et al. 2016b ). Differently, another example of renewable energy implemented in greenhouses is geothermal energy. It consists of circulating fluid in the depths of the earth to use its underground temperature as a source of energy. Its employment in greenhouses includes space heating and cooling, agricultural drying, and ground-source heating pumps (GSHP) (Ha et al. 2015 ; Roumi et al. 2017 ; Ozturk 2017 ; Andritsos et al. 2015 ; Papachristou et al. 2016 ; Urbancl et al. 2016 ).
In addition, the implementation of energy supply optimization strategies is another aspect of efficiently improving the water, energy, and food security in greenhouses. The optimization strategies have varied from feedback conventional controllers to advanced ones based on artificial intelligence such as: fuzzy logic controllers (Su et al. 2017 ), hybrid particle swarm optimization and genetic algorithms (Chen et al. 2015 ), model predictive controllers (Ouammi et al. 2020a , b ; Lin et al. 2020 ) and optimal controllers (Beveren et al. 2015 , Beveren et al. 2020 ; Singh et al. 2015 ). Their common goal is to maximize production yield by tracking the desired trajectories of the controlled variables while minimizing the use of available water and energy resources.
In another way, the optimization of energy consumption can be done by implementing high-tech solutions that facilitate the development of smart greenhouses. In this context, new innovative hardware technologies have been studied to ensure the safe and sustainable use of resources such as LED and novel covering materials. In fact, recent developments have opened a new perspective for LEDs as a light source technology having more technical advantages for greenhouse cultivation over traditional light sources, in terms of high-energy efficiency, low maintenance cost and longevity (Singh et al. 2015 ). In addition, innovative covering materials have gained a significant amount of attention in the research community as promising technologies. By way of illustration, a material based on TiO 2 mixed with low-density polyethylene in the form of heterogeneous granules, developed as an alternative material to face up to the high heating and cooling energy requirements showed improved thermal insulation characteristics (Kavga et al. 2018 ). In addition, a feasibility study of many combinations of different types of glass with different types of F-Clean plastic films showed that the double covering material has high insulation levels, saving 50% energy compared to single glass (Kempkes et al. 2017 ).
While considering the energy needs of a greenhouse it is important to include the energy demands for the desalination of water for irrigation purposes. The salinization of water resources is a progressive phenomenon mostly affecting coastal and delta areas, as well as arid and semi-arid regions. This is due to natural and anthropogenic sources such as climate change and overexploitation of groundwater resources (Mastrocicco and Colombani 2021 ). Especially in the case of hydroponics, the regulation of salinity levels is imperative as it can detrimentally affect plant growth and development. Although desalination increases water resources and improves water quality, the general problem with this process is the relatively high-energy requirement and the associated CO 2 emissions in the case of conventional energy supply (Hussein and Lambert 2020 ). Specifically, the energy required to desalinate seawater could be between 2.5 and 4 kW-h/m 3 (Pearce 2008 ) depending on salinity levels and other factors, such as operating conditions, prevailing temperature, recovery, etc.
3.2 Water considerations in smart greenhouses
From a water-saving point of view, although there is consensus that smart greenhouses are sustainable in terms of their water use, they remain undoubtedly the sector of activity where the consumption of irrigation water is very intensive. For that, smart greenhouses are challenged to save more water without compromising their economic viability (Warner et al. 2020 ), by adopting effective management strategies aiming at conserving water while maintaining satisfactory production and water use efficiency levels.
Smart greenhouse water management techniques include water conservation practices (Warner et al. 2018 ), scheduling strategies (Nikolaou et al. 2019 ) and desalination processes (Kabeel and El-Said 2015 ; Martínez-Alvarez et al. 2016 ). Regarding water conservation practices, drip irrigation was the method justifying the most efficient use of water and has been widely used for many species, like tomato (HongJun et al. 2015 ), muskmelon (Li et al. 2016 ), pepper (Sharma et al. 2017 ; Rameshwaran et al. 2016 ), sugar beet (Kiymaz and Ertek 2015 ) and cotton (He et al. 2018 ). In fact, dispensing small amounts of water at high frequencies has shown a significant increase in water use efficiency (Yao et al. 2019 ), but greater efficiency has been achieved with water pillow irrigation. The latter is a new irrigation method incorporating mulching techniques to drip irrigation through flexible plastic pipes (Fang et al. 2018 ). In this context, many studies have been carried out to assess the superiorities of water pillow irrigation over the drip irrigation method under greenhouse conditions. For example, for the tomato crop, the exploitation of water pillows has proven to have more efficient water use by saving 52% of water compared to drip irrigation (Gerçek et al. 2017 ; Altunlu et al. 2017 ). As well as for eggplant, the use of colored water pillows and mulching treatments has demonstrated a decrease in the irrigation water amounts of 22% and 16%, respectively (Gerçek and Demirkaya 2020 ). For lettuce also, the results suggested that using plastic film mulching was interesting for increasing the biological water use efficiency (Chen, et al. 2019 ). Overall, the benefits of the mulching effect in maintaining the water amount in the root area made this technique yield better outcomes than drip irrigation regarding crop water consumption and irrigation water use efficiency.
Always within the framework of water conservation practices, an alternative water source for greenhouse crop production is the use of rainwater harvesting systems that are demonstrated to reduce the consumption from the water supply network by 80–90% in the case of a rooftop greenhouse. Otherwise, for a standard greenhouse, the application of drainage water blended with water of low electrical conductivity has allowed savings of 17% of the water in comparison to the standard nutrient solution treatment (García-Caparrós et al. 2018a , b ).
Also, deficit irrigation, defined as an optimization strategy consisting of irrigating the root zone with water below the evapotranspiration requirements (Aly et al. 2015 ), has proved to be a success in water saving for a wide range of crops. Indeed, many studies have been carried out to investigate the effects of applying different amounts of water on crop yield and water use efficiency under greenhouse conditions. A summary of the most recent studies, since 2015, is presented in Table 1 .
However, to further improve water conservation techniques, greenhouse irrigation scheduling has been widely promoted as an effective tool in attempting to prevent water wastage. The main technique used for this purpose is the use of soil and plant sensors (Çakir et al. 2017 ). Indeed, although several technological innovations based mainly on thermal infrared imaging systems have been introduced in humidity sensors (Wang et al. 2020 ), the tensiometer remains the most common sensor due to its low cost, simplicity of use, high accuracy, and direct measurement of matric potential (Garcia-Caparros et al. 2107 ; Montesano et al. 2015 ; Contreras et al. 2017 ). It offers a better use of the water resource without being influenced by the temperature and the osmotic potential of the soil (Buttaro et al. 2015 ). The main interest of this functionality is to provide the opportunity to integrate tensiometer based sensor networks in the classic irrigation systems, like what was reported in numerous researches carried out on wireless sensor networks, proving this to be an alternative way to solve the water resources optimization and to support the decision making (Hamouda and Elhabil 2017 ; Harun et al. 2015 ; Montesano et al. 2018 ; Nawandar and Satpute 2019 ; Lea-Cox et al. 2017 ; Khan et al. 2018 ; Ferentinos et al. 2017 ; Silva et al. 2018 ). Besides, the tensiometer demonstrated the ability to assist the development of smart autonomous precise irrigation control systems that can lead considerably surpass the conventional approach in terms of water consumption (Angelopoulos et al. 2020 ; Kim et al. 2015 ).
A resource-saving method as it maximizes overall output and minimizes the use of space, soil, water, and other resources is hydroponics, a soilless cultivation technique. Hydroponic crop production apart from the low land and chemical fertilizers use, requires less water as the nutrient solution can be recirculated. Hence, the hydroponic production of lettuce can result in 64% water savings than the soil cultivation (Majid et al. 2021 ) or even higher, as it needs 13 ± 2.7 times less water compared to conventionally produced lettuce (Barbosa et al. 2015 ). Fully automated hydroponic systems for smart farming (Shetty et al. 2021 ) and the use of internet of thing (IoT) technology (Aliac and Maravillas 2018 ), results in even less amount of water resource consumption when compared to traditional methods. Further water use optimization in hydroponics can be realized by the implementation of the circular economy concept. A two-level cascade cultivation system using the drainage solution of a primary crop to irrigate a secondary crop significantly improved water use efficiency as it reduced by approximately 30% the freshwater consumption of the second crop plants in comparison to plants irrigated with freshwater (Elvanidi et al. 2020 ).
4 Overview of greenhouses development in Qatar
4.1 market growth.
Qatar is a small peninsula with a set of islands located in the Arabian Gulf with a total area of approximately 11.628 km 2 including the islands (Planning and Statistics Authority in Qatar 2013a ). Food production faces many challenges in hot arid countries like Qatar, which is marked by sparse rainfall with an annual average of 83.4 mm in 2019, high temperatures in summer (> 45°C) starting from June till the middle of September, high humidity and strong winds as reported in Figs. 1 and 2 (Homepage 2020 ). The harsh climate, the limited freshwater availability with the dependency on the desalination treatment as well as the limited available arable land, with 65.000 ha or 5.6% of the total area being arable (Al Yousef et al. 2000 ) are reflected in the limited agricultural sector in the country, which provided 0.2% of Qatar’s GDP in 2019 (Planning and Statistics Authority in Qatar 2018 ).
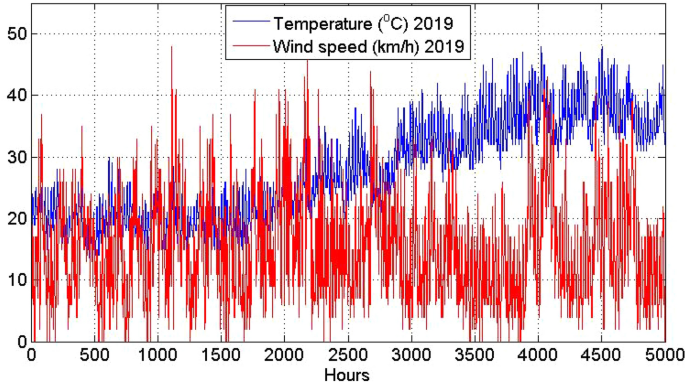
Hourly temperature (°C) and wind speed (km/h) in Qatar in 2019 (Homepage 2020 )
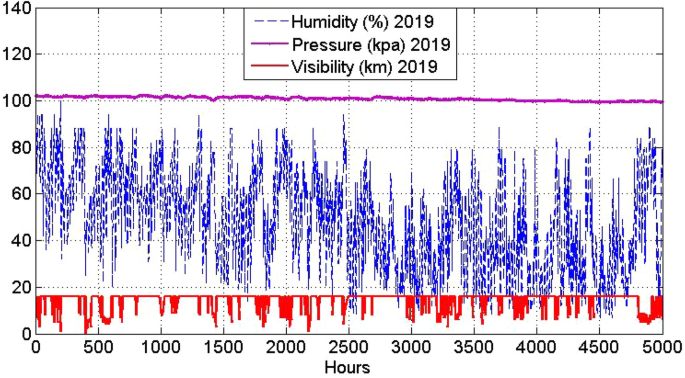
Hourly humidity (%), pressure (kPa) and visibility (km) in Qatar in 2019 (Homepage 2020 )
The main component driving the progress of the greenhouse market in Qatar is the capability to maximize crop production contrasted to the traditional cultivation practices. To expand the self-sufficiency of agricultural production, the need for greenhouses has been increasing in Qatar. As Qatar has a harsh climate throughout the year, consequently, new technologies, methods and practices are introduced to ensure the quality the continuous crop production as well as increase their conservation periods. Furthermore, the Ministry of Municipality and Environment (MME) declared 34 new strategic investment projects-based greenhouses technology in 2018 (Gulf Times Homepage 2020 ). Additionally, the adoption of hydroponic greenhouse and the collaborations with Spanish companies in Qatar have offered a variety of valuable businesses in the country (Businesswire Homepage 2020 ). On the other side, many factors like an elevated investment and import-dependent agriculture in Qatar favour the expansion of the market. Therefore, the Qatar greenhouse market reached a value of US$ 97.9 million in 2018, a value of 112 million US$ in 2019, and it is anticipated to mark a value of US$ 216 million by 2024 (IMARC Group Homepage 2020 ) as stated in Fig. 3 .
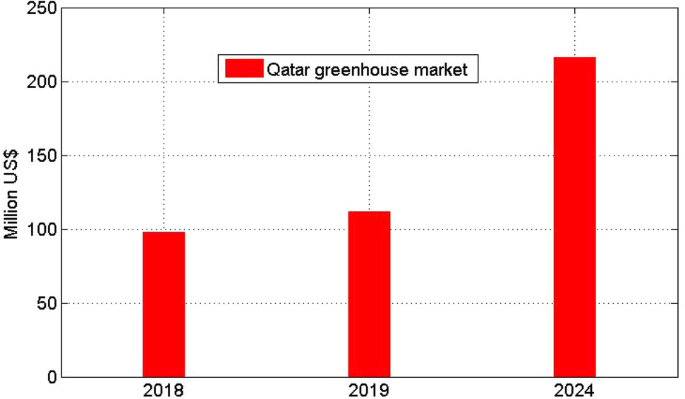
Greenhouse market evolution (million US$) from 2018 to 2024 in Qatar (IMARC Group Homepage 2020 )
More specifically, the cooled greenhouse area increased from 49 ha in 2017 to 73 ha in 2018, with a subsequent increase in vegetable production from 4.814 tons to 6.500 tons in the same years (Table 2 ). Similarly, the no-cooled greenhouse area increased from 200 to 220 ha from 2017 to 2018 with an increase in vegetable production from 17 thousand tons to 20 thousand tons during the same period. The same trend is recorded for the open field vegetable cultivation where the cropped and production area increased from 1,910 ha to 2,484 ha and from 33,000 tons to 36,000 tons, respectively. Therefore, according to Table 2 , the total vegetable production in Qatar has been significantly increased within one year (2017–2018) from 54,814 tons to 62,500 tons and it is noteworthy that the land-use efficiency, in terms of land resource input and production output varies among the three crop production systems. In the case of vegetable greenhouse production, the land-use efficiency is much higher when compared to open field cultivation. However, there are small differences between the cooled and the non-cooled greenhouse production.
According to market experts, the Qatar greenhouse market is a dynamic sector, and it is expected to mark double-digit growth during the next five years and reach a value of almost 259 million US$ by 2025 (Expert market research Homepage 2020 ). This trend is reflected in the increased domestic production of vegetables in the country, as shown in Fig. 4 . During the last decade, the greenhouse and open field production of the most consumed vegetables, like tomatoes, cucumber, marrows, eggplants, lettuce, and green pepper, has increased from 31,573 tons in 2010 to 75,063 tons in 2020, marking an approximate 138% increase. It should be highlighted that after 2017, domestic vegetable production has more than doubled, reaching a more than 100% increase within three years, from 2017 to 2020 (Planning and Statistics Authority in Qatar 2020a , 2014 ). The main driver behind this remarkable increase in local food production was a 3.5-year land, air and sea blockade imposed on Qatar by neighboring countries and allies in the region between June 2017 and January 2021, making the issue of food security a fundamental national security concern. Till then, the state of Qatar imported approximately 90% of its foodstuffs from other countries while 27.4% of the imports came from the blockade imposing countries such as Saudi Arabia and the UAE (Miniaoui et al. 2018 ). After the blockade, Qatar was forced to develop new suppliers for food products and begin to implement local production of perishable goods such as vegetables and milk while nearly tripling local production (Collins 2018 ; Hassen and Bilali 2019 ). Self-sufficiency in many goods like meat, dairy products and vegetables has increased in the country since 2017 and although the blockade has ended, the government of Qatar together with the Ministry of Municipality and Environment has set solid targets to increase up to 70% self-sufficiency on greenhouse vegetables and reach the size of 110 hectares with high-tech greenhouses by 2023 (Ministry of Municipalities and Environment 2020 ).
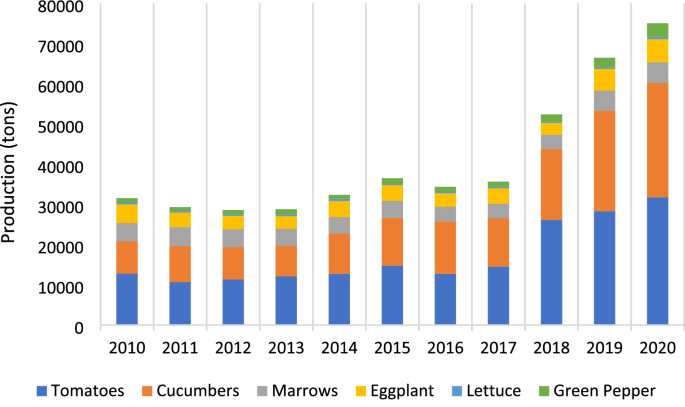
Production (tons) of main vegetables (greenhouse and open field cultivation) in Qatar over the period 2010–2020 (Planning and Statistics Authority in Qatar 2020a , 2014 )
The main companies and competitors in the Qatari greenhouse market are the NAAAS Agricultural Consulting & Greenhouse (Naaas Group), the Arab Qatari Agricultural Production Company (QTFA), the Qatari Agricultural Development Company (Agrico), the Al Rayyan Agricultural and final the Pergola Contracting & Green Houses company.
4.2 Technologies implemented
Greenhouse design in hot arid climates should tackle challenging climatic conditions such as the high temperature and humidity levels and the abundant sunshine. The types of greenhouses found in Qatar can be segmented into categories by the type, the used material and technology. Amongst free-standing and gutter-connected greenhouses, the latter represents the leading type, holding the largest share (IMARC Group Homepage 2020 ). As Qatar has mild winter, the most important function is cooling than heating and consequently, most greenhouses have cooling systems with the evaporative cooling system with pads and fans being the most frequently used. Moreover, the most common greenhouses in the country have plastic or net as covering material, instead of glass. This is because glass is mostly used in countries with colder climates since the temperature inside is higher than the ambient. The following three types of greenhouses could be distinguished according to the used technology:
Low-tech: uncooled and unheated plastic houses or net houses.
Mid-tech: greenhouses with evaporative cooling and with/without air-conditioning for night cooling.
High-tech: computer-controlled greenhouse microclimate i.e., temperature, humidity, CO 2 concentration and lighting.
For Qatar, the most frequent types are the low-tech uncooled, reaching 220 ha total area, followed by the cooled greenhouses with 73 ha area, as shown in Table 2 . The number of high-tech greenhouses is gradually increasing with technologies adapted to the country’s specific geo-climatic conditions (Businesswire Homepage 2020 ). Thus, CO 2 enrichment of the greenhouse environment and artificial light are not common practices in Qatar, as most greenhouses are not fully closed, for ventilation purposes and sunshine is abundant. The methods of natural and forced ventilation and external shading are effective and low-cost ways to control the greenhouse climate in hot areas, with the most optimal method being the evaporative cooling pads (Ghani et al. 2019 ). However, the evaporating cooling method, has its limitations in the summer months, as the high levels of humidity are reducing its effectiveness (Hassabou and Khan 2019 ). Besides, this type of cooling requires a significant amount of water which in most cases exceeds the amount of water needed for irrigation purposes, especially during the hot season from April to June.
According to the collaborative research report of important agricultural companies in the field of greenhouse crop production, fertilizer and plant products in Qatar, Agrico, Qatar Fertilizer Company (QAFCO) and Yara International ASA conducted in 2019 (Enikeeva and Khadra 2019 ), the low-tech net houses have low cost and a 10-month growing period per year for winter crops. However, the growing season can be extended in summer for crops like okra and melon. The mid-tech greenhouses have a growing period of 10 months per year; nonetheless, the air-conditioning night cooling function can extend the summer growing season. The most productive system is the multi-span plastic greenhouse equipped with evaporative cooling and air-conditioning for night cooling, which can reach a tomato yield of 33.2 kg/m 2 , followed by the same type of greenhouse without night air-conditioning with a tomato yield of 29 kg/m 2 . Nevertheless, the first greenhouse type has a higher capital expenditure (CAPEX) compared to the latter, as shown in Table 3 .
Greenhouse cultivation includes soil and soilless cultivation systems, like hydroponics and recently in 2021, aquaponics. Soilless cultivation methods are more suitable for arid climates with limited arable land, like Qatar, as they overcome the limitations of soil and water availability. As agriculture is one of the main water consumers in Qatar, consuming almost 1/2 of the total gross water supplied by the water supply industry which is 310 out 671 million m 3 per year in 2019 (Planning and Statistics Authority in Qatar 2019 ), water-saving hydroponic and aquaponic systems are a possible solution reducing agricultural water use (Barbosa et al. 2015 ). There are many pilot projects in Qatar initiated mainly by the Ministry of Municipality and Environment aiming to improve yields and consequently increase self-sufficiency in various crops (Qatar International Islamic Bank 2019 ). According to researchers, aquaponics is indeed a viable solution to address both food security and sustainability issues in the country but as is an emerging innovative system, it needs more research and development (Abusin and Mandikiana 2020 ). Nonetheless, it is a promising sector and the first aquaponic farm in Qatar by Agrico Agricultural Development started producing tilapia and lettuce in 2021 (Doha News Homepage 2022 ).
4.3 Capacity and potential development
Greenhouse cultivation is an effective way of getting over the limitations in agricultural production in Qatar, which are the harsh climate and the resources scarcity of water, land, and soil. Therefore, the greenhouse sector has significant potential for development. This rise of course is following the country’s efforts to increase food and nutrition security by reducing at the same time import-dependent agriculture. Other factors that seem to favor the sector’s rise are economic welfare and population growth with a consequent increase in food demand. Qatar is among the richest countries in the world with a 66.622 US$ GDP per capita and a 0.1% unemployment rate for 2019 (Focus Economics Homepage 2020 ). The country has witnessed unceasing variations in the population size and its growth rates as reported in Fig. 5 , showing the estimation of the yearly population growth (Planning and Statistics Authority of Qatar Homepage). According to the Planning and Statistics Authority of Qatar, the population has augmented to reach 2.733.624 in September 2020; 1.969.032 of them being males and 754.592 females (Planning and Statistics Authority of Qatar Homepage).
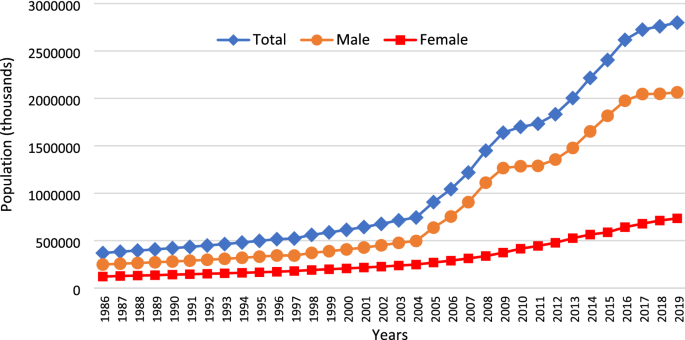
Recorded population growth (thousands) from 1986 to 2019 in Qatar (Planning and Statistics Authority of Qatar Homepage)
The greenhouse industry could be further developed with the help of knowledge and innovation and the introduction of new techniques for modernizing the agricultural sector across the value chain. Greenhouse production can become smarter and less resource-intensive while integrating dynamic technologies such as hydroponics, aeroponics, and aquaponics and adapting advanced cooling technologies to increase crops’ shelf-life (Embassy of the Kingdom of the Netherlands in Doha 2019 ; Karanisa et al. 2021 ). The government’s attitude can enable the greenhouse sector to advance even further, in cooperation with educational institutes, researchers and the private sector on a national and international level. Thus, in summary, the following research areas could boost the agricultural sector and domestic production (GDA 2017 ) and subsequently achieve food security up to a certain level:
Greenhouse research on hydroponics, aquaponics and vertical farming.
Smart agriculture focused on increasing agricultural productivity.
Resources management research on soil, water, and energy to optimize their use avoiding depletion while enhancing conservation.
Marine, fisheries, and aquaculture research to develop innovative technologies to produce fishes, shrimps, seaweeds, etc.
New crop varieties that are heat and humidity-resistant and less water-intensive.
Post-harvest technology to increase crops’ shelf-life.
4.4 Policies and regulations
Food security and sustainable development are high priorities in the Qatar National Vision 2030 (General Secretariat for Development Planning 2008 ), thus the Qatari government has launched different initiatives and strategies for boosting the local food production, as the further development of Qatar’s agricultural industry is fundamental to ensuring food security in the country. Consequently, some of these initiatives are focusing on the greenhouse market as it represents the second major agricultural production method in the country (Qatar International Islamic Bank 2019 ). Additionally, the government’s attention is engaged in resource security by improving their use efficiency and ensuring their conservation. Therefore, special attention is given to the use of groundwater, as without appropriate water resource management, the aquifers will be depleted while the salinity levels of groundwater water will be increased.
According to the Ministry of Municipality and Environment (MME), Qatar is aiming to double the number of farms in the near future to cover 8% of Qatar’s surface area compared to 4% in 2019 (Qatar International Islamic Bank 2019 ). These farms will include animal and livestock farmlands along with vegetable production. That is in alliance with the Qatar National Food Security Strategy (QNFSS) 2018–2023, which has set a target to increase up to 70% domestic self-sufficiency in greenhouse vegetables and reach the size of 110 hectares with high-tech greenhouses by 2023. This strategical and policy guidance aims to achieve a high level of food security while regulating local production, improving crop yield, and protecting the country’s food supply from external shocks. Moreover, special attention is given to environmental protection by preserving water resources and reducing food waste. These targets will be achieved by finalizing greenhouse cluster infrastructure plans and by developing bid guidelines and subsidy programs (Ministry of Municipalities and Environment 2020 ). Besides, the local government had launched agricultural strategic investment projects in 2018 and had been jointly working with companies from Spain to install state-of-the-art greenhouse facilities spread across 100 hectares of land, which required an initial investment outlay of about 430 million QR (117.6 million US$) (Businesswire Homepage 2020 ; Alpen Capital 2019 ). Moreover, 350 greenhouses, cooled and non-cooled, were installed on 85 local farms in 2019 by the Department of Agricultural Affairs of MME with all the necessary equipment and agricultural supplies to enhance agricultural production (Qatar Tribune Front 2020 ). In addition, Qatar Development Bank launched a ‘’Greenhouse financing program’’ in 2019. Urban scale greenhouses can be financed by 100% of the value with a maximum of 70.000 QR, developing the culture of agriculture and enhancing self-sufficiency among the local community (Qatar Development Bank Homepage 2020 ). Although there have been considerable steps enhancing the agricultural and greenhouse sector, Qatar should strengthen even more synergies among government, industry, academic circles, and community. The lack of agricultural legislation in the State of Qatar is a big challenge for the local farmers and agricultural companies because the legislation would support marketing and monitor production more effectively.
5 Food security-greenhouses interaction in Qatar
5.1 climatic and socioeconomic barriers to sustainable ghs development in qatar.
The barriers to sustainable greenhouse development in Qatar, in terms of continuation and technological advancement, are geo-climatic and socioeconomic. Qatar’s climate is characterized by a hot summer starting from May till October with recorded temperatures as high as 34–37.2°C between 2010 to 2020, whereas winter starts from December till the end of February, and it is generally mild (Fig. 6 ). Rainfall is low and humidity is high; 71.4 mm recorded average rainfall between 2010 and 2020 and 51.4% average annual relative humidity during the same period, as shown in Fig. 7 .
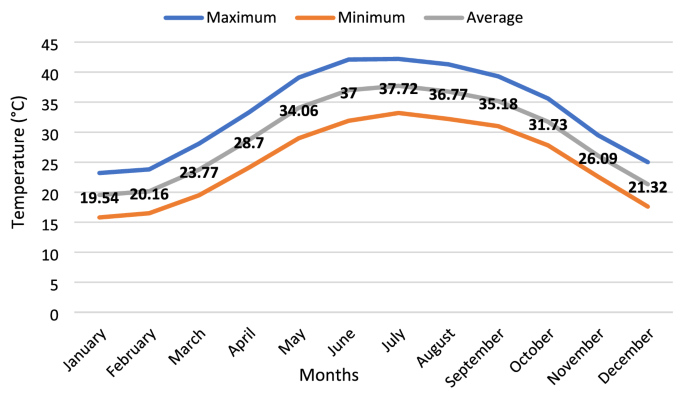
Temperature (°C) per month in Qatar from 2010 to 2020 (Planning and Statistics Authority in Qatar 2013a , 2015a , 2020b )
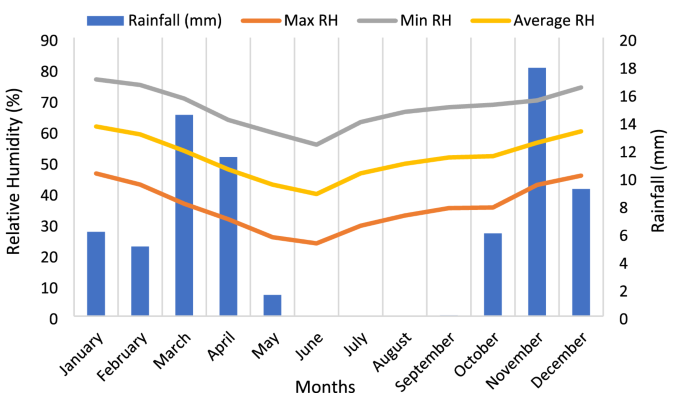
Relative Humidity (%) and rainfall (mm) per month in Qatar from 2010 to 2020 (Planning and Statistics Authority in Qatar 2013a , 2015a , 2020b )
Qatar’s total arable land is a stable area of 65.000 ha and represents only 5.6% of the total country’s area (Planning and Statistics Authority in Qatar 2013a ). In the years between 2010 and 2020, the cultivated land was between 14 to 21% of the total arable land while most of the land is used for green fodder production followed by date palm and vegetable production (Fig. 8 ).
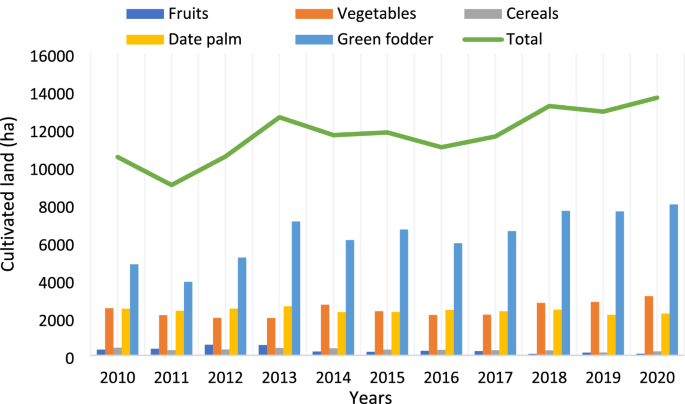
Land utilization of crops (ha) in Qatar from 2010 to 2020 (Planning and Statistics Authority in Qatar 2014 , 2020a )
These factors have hindered the development of the greenhouse and the agricultural sector in general in the past and might prevail the further development of the sector, as they limit the crop production period and the land availability. Greenhouse cooling systems like the pad and fan system, which are widely used in Qatar, are not operating effectively in the summertime due to high humidity levels, causing big stress in plants thus having a negative yield impact.
The socio-economic barriers to the future development of the greenhouse sector are mainly the acceptability and the adaptability of the existing high technology by the farmers and the agricultural professionals, followed by the initial high greenhouse capital investment, the lack of awareness and the need for the expertise in labor force.
It is very often that agricultural professionals are reluctant to adopt new methods and technologies as opposed to the traditional ones, with the main barriers being the risk and the cost they might face. The research on the greenhouse sector has been making great advances as proved by the number of published research articles on agriculture, which has an increased average annual rate of 0.4% between 1999 and 2018, while the greenhouse technology articles have increased by 1.1%, which highlights the advancement of the sector (Aznar-Sánchez et al. 2020 ). Therefore, it is evident that the research institutions have resources and facilities to support the farmers in adopting new technologies, however, there is a gap between scientific knowledge and its implementation. On one hand, adoption of new technologies by the farmers is mostly influenced by their personal characteristics such as their age, educational level, income, their general attitude towards technology which is influenced by the level of accessibility they have (Li et al. 2020 ), and on the other hand, it is also often negatively influenced by perceptions of being a costly and risky investment. Thus, it is recommended that the economic-financial feasibility of the novel applications should be addressed together upon introduction for their successful adaptation by the end-users (Aznar-Sánchez et al. 2020 ).
Moreover, farmers should be well trained and experienced enough to manage efficiently the agricultural farms, thus achieving the highest possible yields. The highly skilled farmers are even more important in the case of the hydroponic cultivation system, which requires specialized knowledge. Additionally, hydroponics has expensive infrastructure costs related mainly to irrigation. As most of the water used in agriculture in Qatar is abstracted fresh groundwater (Planning and Statistics Authority in Qatar 2017a ), it should be desalinated before use in the hydroponic cultivation system, because of elevated levels of salinity, ranging between 6 to 10 g/l. The high salinity levels in the groundwater are caused by overexploitation; the excessed extraction of aquifers causes seawater intrusion (Ahmad and Al-Ghouti 2020 ). Thus, a desalination system investment including reverse osmosis water filtration system and water reservoirs is crucial, and these high costs are contemplated only in large-scale investments.
5.2 Energy and water savings potential in Qatar
Greenhouse crop production consumes large amounts of energy and water resources. In countries with a similar climate as Qatar, most of this energy is required for cooling and ventilation while the agricultural sector is the first biggest water consumer using 92% of the total abstracted groundwater (Ahmad and Al-Ghouti 2020 ). Besides, a considerable amount of water is needed for cooling purposes apart from irrigation as the evaporative cooling system, which is the most prevalent cooling system in Qatar, is significantly water intensive. Notably, the groundwater abstraction rates in Qatar substantially exceed the rate of rainfall-induced recharge, leading to decreasing aquifers levels and deterioration of groundwater quality. Indeed, the groundwater salinity levels have been increased due to over pumping and subsequent seawater intrusion affecting negatively not only the crop yield but also the used equipment such as water pipelines and irrigation drippers. Consequently, the increasing groundwater salinity levels will make groundwater not suitable for direct irrigation use without pre-treatment in desalination systems. In turn, this will increase the total energy needs as desalination is a process with relatively high-energy requirements and there are associated CO 2 emissions in the case of conventional energy supply (Hussein and Lambert 2020 ).
For energy needs, the greenhouse sector in Qatar depends mostly on fossil fuels, which have a big environmental impact on the country. Fossil fuels emit high CO 2 e (carbon dioxide equivalent) gases, making Qatar amongst the top countries with high carbon emissions per capita, recording 35.640 metric tons in 2020 (Knoema. 2022 ). The biggest share of emissions in 2019 came from the natural gas sector with 42.7%, followed by the manufacturing sector with a 21.7% share while the domestic power sector contributed 16% of total net emissions (Doha News 2016 ). Although only 0.1% of the total GHG emissions were from the agricultural sector in 2015 in Qatar (Sayeed 2016 ), nonetheless, this number is expected to have increased with the subsequent growth of the sector despite the lack of more recent data. Besides, the whole food chain including transportation and processing is energy-intensive, along with production itself.
The greenhouse sector could be more sustainable if alternative sources were used for water consumption and energy production, shifting away from fossil fuel use such as petroleum and carbon energy and natural groundwater resources. Namely, the use of Treated Sewage Effluent (TSE) and recycled water for irrigation purposes as well as the use of renewable energies like solar, wind, hydro and geothermal, respectively, in combination with innovative resource-saving technologies and greenhouse designs adapted to the local conditions, could contribute to lower carbon emissions and save the groundwater resources of Qatar. Practices and standards from other regions such as Europe and America cannot always be applied in hot arid countries like Qatar due to climatic differences which affect energy efficiency and consequently the operation cost (Alkhalidi et al. 2020 ). Pakari and Ghani (Pakari and Ghani 2019 ) have proposed a new greenhouse design that limits the available solar radiation thus reducing the high cooling demand during the hot season in subtropical countries like Qatar. The innovative thermally insulated greenhouse can reduce approximately 80% of the cooling load in comparison with a conventional greenhouse. Other researchers (Hassabou and Khan 2019 ) have developed a Passive Solar greenhouse in Qatar with roof PV panels, which can have a grid zero carbon footprint. The energy and ventilation needs are covered with solar cooling, achieving more than a 77% reduction in annual energy consumption, in comparison with a conventional greenhouse design.
The use of TSE for irrigation purposes in agriculture and landscaping in Qatar had already started back in 2004 with 25 million m 3 (5%) and increased by about 3 times from 32.69 m 3 in 2006 to 86.0629 million m 3 in 2019, as shown in Fig. 9 . There have been considerable efforts to increase the use of TSE in agriculture and landscaping from different sectors, thus in 2017, 30% of the TSE was used for agriculture irrigation by the agricultural sector and 27% was used for green space irrigation by the government sector (Planning and Statistics Authority in Qatar 2017a ).
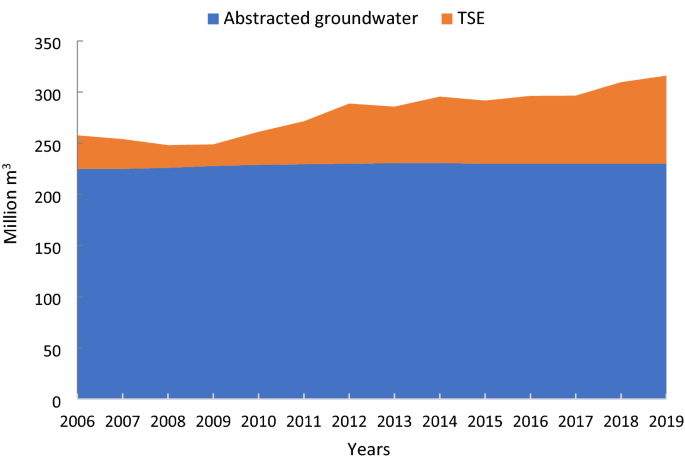
Agricultural water sources for Qatar (million m 3 ) from 2006 to 2019. TSE: Treated Sewage Effluent (Planning and Statistics Authority in Qatar 2019 , 2017a )
5.3 Impacts of greenhouses penetration on the national economy
Open field farming in Qatar is suitable only for a limited type of crops while the growing season is limited to certain months per year, from October to June approximately. Therefore, during the hot summer season, the country is relying exclusively on food imports to cover the local market needs. Additionally, the environmental impact of this type of farming is high, as it consumes large amounts of resources such as water, pesticides, and fertilizers with substantial losses, compared to greenhouse farming (Marucci et al. 2011 ; Bartzas et al. 2015 ; Planning and Statistics Authority in Qatar 2017b ).
Greenhouse production could lead Qatar in achieving higher levels of food security since it can overcome all the limitations that hindered the development of agriculture in the past, such as water scarcity, limited land availability, and the harsh climate. Additionally, it can achieve higher yields than open-field farming with significant product quality increase, while offering the possibility of year-round production, therefore changing the balance between exports and imports of foodstuff in the country. In the last decade, from 2010 to 2020, Qatar’s imports in basic agricultural plant products for human consumption such as cereals, fruits, dates, and vegetables were 10,909,591 tons whereas the exports and re-exports were 132,396 tons. Simultaneously, the local production of the same products reached only 994.932 tons, as shown in Fig. 10 . Vegetable production under greenhouse cultivation can boost domestic production while reducing the reliance on exports and increase Qatar’s resilience to supply shocks and disruptions in the future.
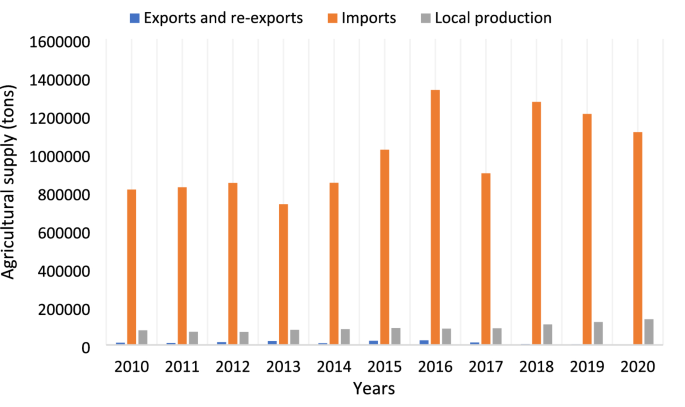
Agricultural supply (tons) of cereals, fruits, dates, and vegetables in Qatar from 2010 to 2020 (Planning and Statistics Authority in Qatar 2012 , 2013b , 2014 , 2015b , 2017b , 2020a )
6 Conclusion and policy implications
Considering climate change, shortage of resources, population growth, and harsh climate, the agricultural sector in Qatar is encountering several challenges in ensuring its growing food needs. Advanced technological solutions such as active smart greenhouses, IoT and precision agriculture, as well as alternative sources for water and energy, can support the state of Qatar to achieve its National Vision 2030, particularly the food security, environmental and sustainability challenges.
This paper aims to generate the knowledge, innovation, technologies, and practices needed to boost the local food production as well as increase the self-reliance of communities in four aspects:
lead to a state-of-art deep understanding of the actual situation of agriculture in Qatar as well as introducing smart greenhouses as a key solution to overcome agriculture challenges at the national level;
develop win–win business opportunities for massive portfolios assets that are beneficial for consumers, farmers, and industrials;
contribute to the modernization and transition to precision agriculture through adoption of smart greenhouses, integrating effective energy us, and water into agri-technology, as well as promoting the incorporation of pertinent technologies in the agricultural sector and
may offer researchers and scientists with knowledge, which encourage them to develop advanced solutions and practices to answer the challenges linked to the transition to sustainable and precise agriculture.
Therefore, the areas of greenhouse development that could potentially lead to higher food security levels while meeting the sustainability goals of Qatar are:
innovative greenhouse designs adopted to the Qatari climate characterized by high temperatures, humidity and solar radiation;
new technological systems for food production like hydroponics, aquaponics and vertical farming;
innovative cooling systems allowing all-year-round crop production especially during summer months while being less water intensive than e.g., the evaporative cooling system;
advanced technological solutions such as the Internet of Things (IoT), metering and communication infrastructure, artificial intelligence, advanced monitoring and control techniques and smart greenhouses targeting ways to optimize resource use, maximize agricultural productivity, adaptation and mitigation against climate change;
use of alternative energy sources like solar, wind, geothermal, etc. to cover the needs of irrigation, cooling and desalination while reducing the associated carbon emissions of the use of fossil fuels and
use of alternative water sources other than fresh groundwater like TSE or recycled water in agriculture.
These greenhouse advancements will generate local socio-economic and environmental advantages. Besides, they will provide an attractive occasion for fostering sustainable development, guaranteeing the high-performance abilities and thus promoting the renovation of the agricultural sector. This is of incredible significance for the efficient design and expansion of the next-generation agricultural sector in terms of self-monitoring, self-control, and optimal operation.
Data availability
The authors confirm that the data supporting the findings of this study are available within the article.
Code availability
Not applicable.
Abusin SAA, Mandikiana BW (2020) Towards sustainable food production systems in Qatar: assessment of the viability of aquaponics. Glob Food Sec. https://doi.org/10.1016/j.gfs.2020.100349
Article Google Scholar
Achour Y, Zejli D (2018) A thermo-economic analysis of solar energy-based heating systems for greenhouse application. In: 2018 6th International Renewable and Sustainable Energy Conference (IRSEC). pp 1‑6. https://doi.org/10.1109/IRSEC.2018.8702897
Achour Y, El Mernissi N, Zejli D (2018) Design and implementation of greenhouse remote monitor system. In: IEEE 5th International Congress on Information Science and Technology (CiSt). pp 1‑6. https://doi.org/10.1109/CIST.2018.8596660
Ahmad AY, Al-Ghouti MA (2020) Approaches to achieve sustainable use and management of groundwater resources in Qatar: a review. Groundw Sustain Dev. https://doi.org/10.1016/j.gsd.2020.100367
Aiello G, Giovino I, Vallone M, Catania P, Argento A (2018) A decision support system based on multisensor data fusion for sustainable greenhouse management. J Clean Prod 172:4057–4065. https://doi.org/10.1016/j.jclepro.2017.02.197
Akkaş MA, Sokullu R (2017) An IoT-based greenhouse monitoring system with Micaz motes. Procedia Comput Sci 113:603–608. https://doi.org/10.1016/j.procs.2017.08.300
Al Yousef AH, El Sharief Abdalla OA, Akbar MA (2000) Progress report for the national report of the state of Qatar on the UNCCD implementation; State of Qatar. Ministry of Municipal Affairs & Agriculture. Dept. Of Agricultural & Water Research, Soil Research Section
Al-Ansari T, Govindan R, Korre A, Nie Z, Shah N (2018) An energy, water and food nexus approach aiming to enhance food production systems through CO 2 fertilization. Comput Aided Chem Eng 43:1487–1492
Alaoui SM, Salghi R, Abouatallah A, Ayoub M (2015) Impact of drip irrigation scheduling on fruit quality parameters and water use efficiency on tomato plant ( Lycopersicon esculentum Mill.) under unheated greenhouse. J Mater Environ Sci 6(2):315–321
CAS Google Scholar
Alenazi M, Abdel-Razzak H, Ibrahim A, Wahb-Allah M, Alsadon A (2015) Response of muskmelon cultivars to plastic mulch and irrigation regimes under greenhouse conditions. J Anim Plant Sci 25(5):1398–1410
Aliac CJG, Maravillas E (2018) IoT hydroponics management system. In: 2018 IEEE 10th International Conference on Humanoid, Nanotechnology, Information Technology, Communication and Control, Environment and Management (HNICEM). pp 1–5. https://doi.org/10.1109/HNICEM.2018.8666372
Alkhalidi A, Khawaja MK, Abusubaih D (2020) Energy efficient cooling and heating of aquaponics facilities based on regional climate. Int J Low-Carbon Technol 15:287–298. https://doi.org/10.1093/ijlct/ctz053
Article CAS Google Scholar
Al-Kodmany K (2018) The vertical farm: a review of developments and implications for the vertical city. Buildings 8(2):24. https://doi.org/10.3390/buildings8020024
Alpay O, Erdem E (2018) Climate Control of a Smart Greenhouse based on Android. In: International Conference on Artificial Intelligence and Data Processing (IDAP). pp 1‑5
Alpen Capital (2019). GCC Food Industry; Alpen Capital: Abu Dhabi, UAE
Alsanius BW et al (2017) Ornamental flowers in new light: artificial lighting shapes the microbial phyllosphere community structure of greenhouse grown sunflowers ( Helianthus annuus L.). Sci Hortic 216:234–247. https://doi.org/10.1016/j.scienta.2017.01.022
Altunlu H, Ayranci Y, Gercek S, Ayranci Y, Gercek S (2017) Comparison of water pillow and drip irrigation systems for tomato ( Lycopersicon esculentum mill.). Production under greenhouse conditions in the mediterranean region of Turkey. Bulg J Agric Sci 23(1):76–82
Google Scholar
Aly AA, Al-Omran AM, Khasha AA (2015) Water management for cucumber: greenhouse experiment in Saudi Arabia and modeling study using SALTMED model. J Soil Water Conserv 70(1):1–11. https://doi.org/10.2489/jswc.70.1.1
Andritsos N, Dalambakis P, Arvanitis A, Papachristou M, Fytikas M (2015) Geothermal Developments in Greece—Country update 2010–2014. Proceedings of 2015 World Geothermal Congress. pp 11
Angelopoulos CM, Filios G, Nikoletseas S, Raptis TP (2020) Keeping data at the edge of smart irrigation networks: a case study in strawberry greenhouses. Comput Netw 167:107039. https://doi.org/10.1016/j.comnet.2019.107039
Arad B et al (2020) Development of a sweet pepper harvesting robot. J Field Robot 37(6):1027–1039. https://doi.org/10.1002/rob.21937
Atefi A, Ge Y, Pitla S, Schnable J (2019) In vivo human-like robotic phenotyping of leaf traits in maize and sorghum in greenhouse. Comput Electron Agric 163:104854. https://doi.org/10.1016/j.compag.2019.104854
Awani S, Chargui R, Kooli S, Farhat A, Guizani A (2015) Performance of the coupling of the flat plate collector and a heat pump system associated with a vertical heat exchanger for heating of the two types of greenhouses system. Energy Convers Manage 103:266–275. https://doi.org/10.1016/j.enconman.2015.06.032
Aznar-Sánchez JA, Velasco-Muñoz JF, López-Felices B, Román-Sánchez IM (2020) An analysis of global research trends on greenhouse technology: towards a sustainable agriculture. Int J Environ Res Public Health 17:664. https://doi.org/10.3390/ijerph17020664
Baddadi S, Bouadila S, Ghorbel W, Guizani A (2019) Autonomous greenhouse microclimate through hydroponic design and refurbished thermal energy by phase change material. J Clean Prod 211:360–379. https://doi.org/10.1016/j.jclepro.2018.11.192
Bagheri Sanjareh M, Nazari MH, Hosseinian SH (2021) A novel strategy for frequency control of islanded greenhouse with cooperative usage of BESS and LED lighting loads. Electr Eng 103:265–277. https://doi.org/10.1007/s00202-020-01077-5
Bao J, Lu W-H, Zhao J, Bi XT (2018) Greenhouses for CO 2 sequestration from atmosphere. Carbon Res Conv 1(2):183–190. https://doi.org/10.1016/j.crcon.2018.08.002
BaoZhong Y, QingYa Z, ZhiLong B (2015) Effects of regulated deficit irrigation on growth of drip-irrigated muskmelon in greenhouse. J Drain Irrig Machin Eng 33(7):611–617
Barbosa G et al (2015) Comparison of land, water, and energy requirements of lettuce grown using hydroponic vs. conventional. Agric Methods IJERPH 12(6):6879–6891. https://doi.org/10.3390/ijerph120606879
Bartzas G, Zaharaki D, Komnitsas K (2015) Life cycle assessment of open field and greenhouse cultivation of lettuce and barley. Info Processing Agric 2(3–4):191–207. https://doi.org/10.1016/j.inpa.2015.10.001
Ben Ali R, Bouadila S, Mami A (2018) Development of a fuzzy logic controller applied to an agricultural greenhouse experimentally validated. Appl Therm Eng 141:798–810. https://doi.org/10.1016/j.applthermaleng.2018.06.014
Benke K, Tomkins B (2017) Future food-production systems: vertical farming and controlled-environment agriculture. Sustainability 13(1):13–26. https://doi.org/10.1080/15487733.2017.1394054
Bersani C, Ouammi A, Sacile R, Zero E (2020) Model predictive control of smart greenhouses as the path towards near zero energy consumption. Energies 13:3647. https://doi.org/10.3390/en13143647
Businesswire Homepage (2020) https://www.businesswire.com/news/home/20190903005749/en/Qatar-Greenhouse-Market-Report-2019-Industry-Trends-Share-Size-Growth-Opportunity-and-Forecasts-2011-2018-2019-2024---ResearchAndMarkets.com#:~:text=The%20Qatar%20greenhouse%20market%20reached,14.36%25%20during%202019%2D2024 . Accessed 27 Dec 2020
Buttaro D et al (2015) Irrigation management of greenhouse tomato and cucumber using tensiometer: effects on yield, quality and water use». Agric Agric Sci Procedia 4:440–444. https://doi.org/10.1016/j.aaspro.2015.03.050
Çakir R, Kanburoglu-Çebi U, Altintas S, Ozdemir A (2017) Irrigation scheduling and water use efficiency of cucumber grown as a spring-summer cycle crop in solar greenhouse. Agric Water Manag 180:78–87. https://doi.org/10.1016/j.agwat.2016.10.023
Cantore V et al (2016) Combined effect of deficit irrigation and strobilurin application on yield, fruit quality and water use efficiency of “cherry” tomato ( Solanum lycopersicum L.). Agric Water Manag 167:53–61. https://doi.org/10.1016/j.agwat.2015.12.024
Carrasquilla-Batista A, Chacon-Rodrıguez A (2017) Proposal of a fuzzy logic controller for the improvement of irrigation scheduling decision-making in greenhouse horticulture. In: 2017 1st Conference on PhD Research in Microelectronics and Electronics Latin America (PRIME-LA). pp 1‑4. https://doi.org/10.1109/PRIME-LA.2017.7899172
Çaylı A, Akyüz A, Baytorun AN, Boyacı S, Üstün S, Kozak FB (2017) Control of greenhouse environmental conditions with IOT based monitoring and analysis system. Turkish J Agric Food Sci Technol 5(11):1279–1289. https://doi.org/10.24925/turjaf.v5i11.1279-1289.1282
Cenedese A, Schenato L, Vitturi S (2012) Wireless sensor/actor networks for real–time climate control and monitoring of greenhouses. OAI. http://paduaresearch.cab.unipd.it/1045/1/serra_sv_v11.pdf
Chen Z et al (2019) Assessing the performance of different irrigation systems on lettuce ( Lactuca sativa L.) in the greenhouse. PLoS ONE 14(2):e0209329. https://doi.org/10.1371/journal.pone.0209329
Chen J, Zhao J, Xu F, Hu, Ai Q, Yang J (2015) Modeling of Energy Demand in the Greenhouse Using PSO-GA Hybrid H Algorithms. Mathematical problems in engineering. https://www.hindawi.com/journals/mpe/2015/871075/ . Accessed 29 Mar 2020
Chen Y et al (2016a) Connectivity of wireless sensor networks for plant growth in greenhouse Citation. Int J Agric Biol Eng 9(1):89. https://doi.org/10.3965/j.ijabe.201606901.1314
Chen L, Zhang B, Yao F, Cui L (2016b) Modeling and simulation of a solar greenhouse with natural ventilation based on error optimization using fuzzy controller. In: 2016 35th Chinese Control Conference (CCC). pp 2097‑2102
Chen F, Qin L, Li X, Wu G, Shi C (2017) Design and implementation of ZigBee wireless sensor and control network system in greenhouse. In: 36th Chinese Control Conference (CCC). pp 8982‑8986. https://doi.org/10.23919/ChiCC.2017.8028786 .
Chung SO, Kang SW, Bae KS, Ryu MJ, Kim YJ (2015) The potential of remote monitoring and control of protected crop production environment using mobile phone under 3G and Wi-Fi communication conditions. Eng Agric Environ Food 8(4):251–256. https://doi.org/10.1016/j.eaef.2015.04.007
Collins G (2018) Anti-Qatar Embargo Grinds Toward Strategic Failure. Issue brief no. 01.22.18. Rice University’s Baker Institute for Public Policy, Houston, Texas
Contreras JI, Alonso F, Cánovas G, Baeza R (2017) Irrigation management of greenhouse zucchini with different soil matric potential level. Agronomic and environmental effects. Agric Water Manag 183:26–34. https://doi.org/10.1016/j.agwat.2016.09.025
Coyago-Cruz E et al (2019) Yield response to regulated deficit irrigation of greenhouse cherry tomatoes. Agric Water Manag 213:212–221. https://doi.org/10.1016/j.agwat.2018.10.020
da Silva LM, Junior EH, Carneiro KJP, de Matos JM, Anacilia AP, de Vieira MC, da Barreto R (2018) Tellus—Greenhouse irrigation automation system. In: 2018 IEEE Symposium on Computers and Communications (ISCC). pp 01239‑01242. https://doi.org/10.1109/ISCC.2018.8538494
Danita M, Mathew B, Shereen N, Sharon, Paul JJ (2018) IoT Based automated greenhouse monitoring system. In: Second International N Conference on Intelligent Computing and Control Systems (ICICCS). pp 1933‑1937. https://doi.org/10.1109/ICCONS.2018.8662911 .
de Anda J, Shear H (2017) Potential of vertical hydroponic agriculture in Mexico. Sustainability 9:140. https://doi.org/10.3390/su9010140
de Lima RSN et al (2015) Partial rootzone drying (PRD) and regulated deficit irrigation (RDI) effects on stomatal conductance, growth, photosynthetic capacity, and water-use efficiency of papaya. Sci Hortic 183:13–22. https://doi.org/10.1016/j.scienta.2014.12.005
Ding JT, Tu HY, Zang ZL, Huang M, Zhou SJ (2018) Precise control and prediction of the greenhouse growth environment of Dendrobium candidum . Comput Electron Agric 151:453–459. https://doi.org/10.1016/j.compag.2018.06.037
Djurović N, Ćosić M, Stričević R, Savić S, Domazet M (2016) Effect of irrigation regime and application of kaolin on yield, quality and water use efficiency of tomato. Sci Hortic 201:271–278. https://doi.org/10.1016/j.scienta.2016.02.017
Długosz-Grochowska O, Wojciechowska R, Kruczek M, Habela A (2017) Supplemental lighting with LEDs improves the biochemical composition of two Valerianella locusta (L.) cultivars. Hortic Environ Biotechnol 58(5):441–449. https://doi.org/10.1007/s13580-017-0300-4
Doha news (2022) Qatar records 3.9% drop in total greenhouse gas emissions since 2016: report. https://www.dohanews.co/qatar-records-3-9-drop-in-total-greenhouse-gas-emissions-since-2016-report/#:~:text=Emissions%20from%20the%20manufacturing%20sector,%2C%20emissions%20increased%20by%20289%25.&text=The%20domestic%20power%20sector%20contributed,412%25%20between%20the%20same%20period.&text=and%202019.,-With%20a%20total . Accessed 26 Jan 2022
Doha News Homepage (2022) Qatar biggest aquaculture farming project begins producing vegetables. https://www.dohanews.co/qatar-biggest-aquaculture-farming-project-begins-producing-vegetables/ . Accessed 2 Feb 2022
dos Santos JF, Coelho Filho MA, Cruz JL, Soares TM, Cruz AML (2019) Growth, water consumption and basil production in the hydroponic system under salinity. Rev Ceres 66(1):45–53. https://doi.org/10.1590/0034-737x201966010007
Du Y, Cao H, Liu S, Gu X, Cao Y (2017) Response of yield, quality, water and nitrogen use efficiency of tomato to different levels of water and nitrogen under drip irrigation in Northwestern China. J Integr Agric 16(5):1153–1161. https://doi.org/10.1016/S2095-3119(16)61371-0
Durmus H, Gunes E, Kirci M (2016) Data acquisition from greenhouses by using autonomous mobile robot. In: 2016 Fifth International Conference on Agro-Geoinformatics (Agro-Geoinformatics). pp 1‑5. https://doi.org/10.1109/Agro-Geoinformatics.2016.7577696
El-gafy I (2017) Water–food–energy nexus index: analysis of water–energy–food nexus of crop’s production system applying the indicators approach. Appl Water Sci 7:2857–2868. https://doi.org/10.1007/s13201-017-0551-3
ELkhadraoui A, Kooli S, Hamdi I, Farhat A (2015) Experimental investigation and economic evaluation of a new mixed-mode solar greenhouse dryer for drying of red pepper and grape. Renew Energy 77:1–8. https://doi.org/10.1016/j.renene.2014.11.090
Elvanidi A, Benitez Reascos CM, Gourzoulidou E, Kunze A, Max JFJ, Katsoulas N (2020) Implementation of the circular economy concept in greenhouse hydroponics for ultimate use of water and nutrients. Horticulturae 6(4):83. https://doi.org/10.3390/horticulturae6040083
Embassy of the Kingdom of the Netherlands in Doha (2019) Water-Energy-Food Nexus in Qatar. Doha, Qatar
Enikeeva K, Khadra C (2019) Agrico Qafco Yara Trial and Demonstration Center. Report on trials conducted in December 2019–December 2020; Yara International. Doha, Qatar. https://www.qafco.qa/sites/default/files/Report%20on%20Agrico%20Qafco%20Yara%20trial%20station.pdf . Accessed 21 Dec 2020
Erazo M et al (2015) Design and implementation of a wireless sensor network for rose greenhouses monitoring. In: 6th International Conference on Automation, Robotics and Applications (ICARA). pp 256‑261. https://doi.org/10.1109/ICARA.2015.7081156 .
Erazo-Rodas M et al (2018) Multiparametric monitoring in equatorian tomato greenhouses (I): wireless sensor network benchmarking. Sensors 18(8):2555. https://doi.org/10.3390/s18082555
Essahafi M, Lafkih MA (2018) Microclimate control of a greenhouse by adaptive generalized linear quadratic strategy. Indo J Elec Eng Comput Sci 11:377–385. https://doi.org/10.11591/ijeecs.v11.i1.pp377-385
Expert market research Homepage (2020) https://www.expertmarketresearch.com/reports/qatar-greenhouse-market . Accessed 13 Oct 2020
Fang Q, Zhang X, Shao L, Chen S, Sun H (2018) Assessing the performance of different irrigation systems on winter wheat under limited water supply. Agric Water Manag 196:133–143. https://doi.org/10.1016/j.agwat.2017.11.005
FAO (2010) Food security definitions and measures. Towards a Food Insecurity. Multidimensional Index (FIMI). Master’s in Human Development and Food Security. Marion Napoli (2010/2011). http://www.fao.org/fileadmin/templates/ERP/uni/FIMI.pdf . Accessed 29 Dec 2020
FAO (2020a) Land and water. http://www.fao.org/land-water/world-water-day-2021/watergovernance/waterfoodenergynexus/en/ . Accessed 29 Dec 2020
FAO (2020b) Climate change: implications for food safety http://www.fao.org/3/i0195e/i0195e00.pdf . Accessed 29 Dec 2020
FAO AQUASTAT Homepage (2020) http://www.fao.org/aquastat/en/overview/methodology/water-use . Accessed 26 Dec 2020
Faouzi D, Bibi-Triki N, Draoui B, Abene A (2017) The optimal management of the micro climate of the agricultural greenhouse through the modeling of a fuzzy logic controller. J Global Agric Ecol 7(1):1–15
Fatnassi H, Poncet C, Bazzano MM, Brun R, Bertin N (2015) A numerical simulation of the photovoltaic greenhouse microclimate. Sol Energy 120:575–584. https://doi.org/10.1016/j.solener.2015.07.019
Feng Q, Wang X, Wang G, Li Z (2015) Design and test of tomatoes harvesting robot. In: 2015 IEEE International Conference on Information and Automation. pp 949‑952. https://doi.org/10.1109/ICInfA.2015.7279423
Ferentinos KP, Katsoulas N, Tzounis A, Bartzanas T, Kittas C (2017) Wireless sensor networks for greenhouse climate and plant condition assessment. Biosys Eng 153:70–81. https://doi.org/10.1016/j.biosystemseng.2016.11.005
Focus Economics Homepage (2020) https://www.focus-economics.com/country-indicator/qatar/population . Accessed 8 Dec 2020
Francik S, Kurpaska S (2020) The use of artificial neural networks for forecasting of air temperature inside a heated foil tunnel. Sensors. https://doi.org/10.3390/s20030652
Gao Y et al (2019) Modeling and analyses of energy performances of photovoltaic greenhouses with sun-tracking functionality. Appl Energy 233–234:424–442. https://doi.org/10.1016/j.apenergy.2018.10.019
Garcia-Caparros P, Contreras J, Baeza R, Segura M, Lao M (2017) Integral management of irrigation water in intensive horticultural systems of Almería. Sustainability 9(12):2271. https://doi.org/10.3390/su9122271
García-Caparrós P, Llanderal A, El-Tarawy A, Maksimovic I, Lao M (2018a) Crop and irrigation management systems under greenhouse conditions. Water 10(1):62. https://doi.org/10.3390/w10010062
García-Caparrós P, Llanderal A, Maksimovic I, Lao M (2018b) Cascade cropping system with horticultural and ornamental plants under greenhouse conditions. Water 10(2):125. https://doi.org/10.3390/w10020125
Global Dryland Alliance (GDA) (2017) Food security in Drylands; Final Status of Food Security Report
General Secretariat for Development Planning (2008) Qatar National Vision 2030; General Secretariat for Development Planning: Doha, Qatar
Gerçek S, Demirkaya M (2020) Effects of coloured water pillows on yield and water productivity of eggplant. Irrig and Drain 69(4):658–667. https://doi.org/10.1002/ird.2463
Gerçek S, Demirkaya M, Işik D (2017) Water pillow irrigation versus drip irrigation with regard to growth and yield of tomato grown under greenhouse conditions in a semi-arid region. Agric Water Manag 180:172–177. https://doi.org/10.1016/j.agwat.2016.11.012
Ghani S, Bakochristou F, ElBialy EMAA, Gamaledin SMA, Rashwan MM, Abdelhalim AM, Ismail SM (2019) Design challenges of agricultural greenhouses in hot and arid environments—a review. Eng Agric Environ Food 12(1):48–70. https://doi.org/10.1016/j.eaef.2018.09.004
Gómez C, Currey CJ, Dickson RW, Kim HJ, Hernández R, Sabeh NC, Raudales RE, Brumfield RG, Laury-Shaw A, Wilke AK et al (2019) Controlled environment food production for urban agriculture. HortScience 54:1448–1458
Good C (2016) Environmental impact assessments of hybrid photovoltaic–thermal (PV/T) systems—a review. Renew Sustain Energy Rev 55:234–239. https://doi.org/10.1016/j.rser.2015.10.156
Graamans L, Baeza E, van den Dobbelsteen A, Tsafaras I, Stanghellini C (2018) Plant factories versus greenhouses: comparison of resource use efficiency. Agric Syst 160:31–43. https://doi.org/10.1016/j.agsy.2017.11.003
Gulf Times Homepage (2020) https://www.gulf-times.com/story/613702/MME-announces-investment-projects-to-produce-vegetables-in-greenhouses . Accessed 13 Oct 2020
Ha T, Lee I, Kwon K, Hong S-W (2015) Computation and field experiment validation of greenhouse energy load using building energy simulation model. Int J Agric Biol Eng 8(6):116–127. https://doi.org/10.25165/ijabe.v8i6.2037
Haji M, Govindan R, Al-Ansari T (2020) Novel approaches for geospatial risk analytics in the energy–water–food nexus using an EWF nexus node. Comput Chem Eng 140:106936. https://doi.org/10.1016/j.compchemeng.2020.106936
Hamouda YEM, Elhabil BHY (2017) Precision agriculture for greenhouses using a wireless sensor network. 2017 Palestinian International Conference on Information and Communication Technology (PICICT), p. 78‑83. https://doi.org/10.1109/PICICT.2017.20
Hamza A, Ramdani M (2020) Non-PDC interval type-2 fuzzy model predictive microclimate control of a greenhouse. J Control Autom Electr Syst 31(1):62–72. https://doi.org/10.1007/s40313-019-00532-4
Han L, Mao H, Kumi F, Hu J (2018) Development of a multi-task robotic transplanting workcell for greenhouse seedlings. Appl Eng Agric 34(2):335–342. https://doi.org/10.13031/aea.12462
Harun AN, Kassim MRM, Mat I, Ramli SS (2015) Precision irrigation using Wireless Sensor Network. In: 2015 International Conference on Smart Sensors and Application (ICSSA). pp 71‑75
Hassabou AM, Khan MA (2019) Towards autonomous solar driven agricultural greenhouses in Qatar—integration with solar cooling. In: 7th International Renewable and Sustainable Energy Conference (IRSEC). pp 1–8
Hassen TB, El Bilali H (2019) Food security in the gulf cooperation council countries: challenges and prospects. J Food Security 7:159–169
He J, Zhou L, Yao Q, Liu B, Xu H, Huang J (2018) Greenhouse and field-based studies on the distribution of dimethoate in cotton and its effect on Tetranychus urticae by drip irrigation: dissipation and control efficacy of dimethoate on T. urticae by drip irrigation. Pest Manag Sci 74(1):225–233. https://doi.org/10.1002/ps.4704
Hong G-Z, Hsieh C-L (2016) Application of integrated control strategy and bluetooth for irrigating romaine lettuce in greenhouse. IFAC-PapersOnLine 49(16):381–386. https://doi.org/10.1016/j.ifacol.2016.10.070
Hong Jun L, Ming Z, Zhen Hua Z, Xin L, Jian Xin X, Hong Wei P (2015) Growth and quality of greenhouse tomato under cycle aerated subsurface drip irrigation. J Drain Irrig Mach Eng 33(3):253–259
Hongkang W, Li L, Yong W, Fanjia M, Haihua W, Sigrimis NA (2018) Recurrent neural network model for prediction of microclimate in solar greenhouse. IFAC-PapersOnLine 51(17):790–795. https://doi.org/10.1016/j.ifacol.2018.08.099
Hooshmand M, Albaji M, Boroomand nasab S, Alam zadeh Ansari N (2019) The effect of deficit irrigation on yield and yield components of greenhouse tomato ( Solanum lycopersicum ) in hydroponic culture in Ahvaz region, Iran. Sci Horticul 254:84–90. https://doi.org/10.1016/j.scienta.2019.04.084
Huang L, Chen L, Wang Q, Yan S, Gao X, Luan J (2018) Regional Short-term Micro-climate Air Temperature Prediction with CBPNN. In: E3S Web Conf 53:03009. https://doi.org/10.1051/e3sconf/20185303009
Hussein H, Lambert LAA (2020) Rentier state under blockade: Qatar’s water-energy-food predicament from energy abundance and food insecurity to a silent water crisis. Water 12:1051
Hwang J-H, Yoe H (2016) Design of wireless sensor network based smart greenhouse system. In: Int'l Conf. Wireless Networks (ICWN'16).pp 43–48
Ibayashi H, Kaneda Y, Imahara J, Oishi N, Kuroda M, Mineno H (2016) A reliable wireless control system for tomato hydroponics. Sensors 16(5):644. https://doi.org/10.3390/s16050644
IMARC Group Homepage (2020) https://www.imarcgroup.com/qatar-greenhouse-market . Accessed 13 Oct 2020
Ismail MT, Ismail MN, Sameon SS, Zin ZM, Mohd N (2016) Wireless Sensor Network: Smart greenhouse prototype with smart design. In: 2nd International Symposium on Agent, Multi-Agent Systems and Robotics (ISAMSR). pp 57‑62. https://doi.org/10.1109/ISAMSR.2016.7810003 .
Jahnavi VS, Ahamed SF (2015) Smart wireless sensor network for automated greenhouse. IETE J Res 61(2):180–185. https://doi.org/10.1080/03772063.2014.999834
Ji W, Chen G, Xu B, Meng X, Zhao D (2019) Recognition method of green pepper in greenhouse based on least-squares support vector machine optimized by the improved particle swarm optimization. IEEE Access 7:119742–119754. https://doi.org/10.1109/ACCESS.2019.2937326
Jiang JA et al (2016) A wireless sensor network-based monitoring system with dynamic convergecast tree algorithm for precision cultivation management in orchid greenhouses. Precision Agric 17(6):766–785. https://doi.org/10.1007/s11119-016-9448-7
Kabeel AE, El-Said EMS (2015) Water production for irrigation and drinking needs in remote arid communities using closed-system greenhouse: a review. Eng Sci Technol Int J 18(2):294–301. https://doi.org/10.1016/j.jestch.2014.12.003
Kalantari F, Nochian A, Darkhani F, Asif N (2020) The significance of vertical farming concept in ensuring food security for high-density urban areas. Jurnal Kejuruteraan 32(1):105–111. https://doi.org/10.17576/jkukm-2020-32(1)-13
Karan E, Asadi S, Mohtar R, Baawain M (2018) Towards the optimization of sustainable food-energy-water systems: a stochastic approach. J Clean Prod 171:662–674. https://doi.org/10.1016/j.jclepro.2017.10.051
Karanisa T, Amato A, Richer R, Abdul Majid S, Skelhorn C, Sayadi S (2021) Agricultural production in Qatar’s hot arid climate. Sustainability 13:4059. https://doi.org/10.3390/su13074059
Kavga A, Souliotis M, Koumoulos EP, Fokaides PA, Charitidis CA (2018) Environmental and nanomechanical testing of an alternative polymer nanocomposite greenhouse covering material. Sol Energy 159:1–9. https://doi.org/10.1016/j.solener.2017.10.073
Kempkes FLK, Janse J, Hemming S (2017) Greenhouse concept with high insulating cover by combination of glass and film: design and first experimental results. Acta Hortic 1170:469–486. https://doi.org/10.17660/ActaHortic.2017.1170.58
Khan R, Ali I, Zakarya M, Ahmad M, Imran M, Shoaib M (2018) Technology-assisted decision support system for efficient water utilization: a real-time testbed for irrigation using wireless sensor networks. IEEE Access 6:25686–25697. https://doi.org/10.1109/ACCESS.2018.2836185
Khelifa L, Bounaama F, Ouradj B, Belkacem D (2020) Constrained ga pi sliding mode control of indoor climate coupled mimo greenhouse model. J Therm Eng. https://doi.org/10.18186/therma.711554
Kim HJ et al (2015) Design and testing of an autonomous irrigation controller for precision water management of greenhouse crops. Eng Agric Environ Food 8(4):228–234. https://doi.org/10.1016/j.eaef.2015.03.001
Kim JH, Ahn JG, Kim JT (2016) Demonstration of the performance of an air-type photovoltaic thermal (PVT) system coupled with a heat-recovery ventilator. Energies. https://doi.org/10.3390/en9090728
Kiymaz S, Ertek A (2015) Yield and quality of sugar beet ( Beta vulgaris L.) at different water and nitrogen levels under the climatic conditions of Kırsehir, Turkey. Agric Water Manag. https://doi.org/10.1016/j.agwat.2015.05.004
Knoema. World Data Atlas, Qatar, Environment. Qatar CO 2 emissions per capita. https://knoema.com/atlas/Qatar/CO2-emissions-per-capita . Accessed 26 Jan 2022
Kowalczyk K, Olewnicki D, Mirgos M, Gajc-Wolska J (2020) Comparison of selected costs in greenhouse cucumber production with LED and HPS supplemental assimilation lighting. Agronomy 10(9):1342. https://doi.org/10.3390/agronomy10091342
Lahlou F, Mackey HR, McKay G, Onwusogh U, Al-Ansari T (2020) Water planning framework for alfalfa fields using treated wastewater fertigation in Qatar: an energy-water-food nexus approach. Comput Chem Eng 141:106999. https://doi.org/10.1016/j.compchemeng.2020.106999
Lea-Cox JD et al (2017) Demonstrated benefits of using sensor networks for automated irrigation control in nursery and greenhouse production systems. Acta Hortic 1150:507–514. https://doi.org/10.17660/ActaHortic.2017.1150.70
Li Y, Niu W, Xu J, Wang J, Zhang M, Lv W (2016) Root morphology of greenhouse produced muskmelon under sub-surface drip irrigation with supplemental soil aeration. Sci Hortic 201:287–294. https://doi.org/10.1016/j.scienta.2016.02.018
Li Z, Wang J, Higgs R, Zhou L, Yuan W (2017a) Design of an Intelligent Management System for Agricultural Greenhouses Based on the Internet of Things. IEEE Int Conf Comput Sci Eng IEEE Int Conf Embedded Ubiquitous Comput 2:154–160. https://doi.org/10.1109/CSE-EUC.2017.212
Li Q, Zhang D, Ji J, Sun Z, Wang Y (2018) Modeling of natural ventilation using a hierarchical fuzzy control system for a new energy-saving solar greenhouse. Appl Eng Agric 34(6):953–962. https://doi.org/10.13031/aea.12757
Li X, Ma Z, Chu X, Liu Y (2019a) A cloud-assisted region monitoring strategy of mobile robot in smart greenhouse. Mob Inf Syst 2019:1–10. https://doi.org/10.1155/2019/5846232
Li Q et al (2019b) Effect of supplemental lighting on water transport, photosynthetic carbon gain and water use efficiency in greenhouse tomato. Sci Hortic 256:108630. https://doi.org/10.1016/j.scienta.2019.108630
Li M, Fu Q, Singh VP, Liu D, Li T (2019c) Stochastic multi-objective modeling for optimization of water-food-energy nexus of irrigated agriculture. Adv Water Resour 127:209–224. https://doi.org/10.1016/j.advwatres.2019.03.015
Li H, Huang D, Qi Ma, Qi W, Li H (2020) Factors influencing the technology adoption behaviours of litchi farmers in China. Sustainability 12:271. https://doi.org/10.3390/su12010271
Li L, Cheng KWE, Pan JF (2017b) Design and application of intelligent control system for greenhouse environment. In: 7th International Conference on Power Electronics Systems and Applications—Smart Mobility, Power Transfer Security (PESA). pp 1‑5
Liang MH, He YF, Chen LJ, Du SF (2018a) Greenhouse environment dynamic monitoring system based on WIFI. IFAC-PapersOnLine 51(17):736–740. https://doi.org/10.1016/j.ifacol.2018.08.108
Liang MH, Chen LJ, He YF, Du SF (2018b) Greenhouse temperature predictive control for energy saving using switch actuators. IFAC-PapersOnLine 51(17):747–751. https://doi.org/10.1016/j.ifacol.2018.08.106
Liang-Ying, Yun-feng G, Zhao-Wei (2015) Greenhouse environment monitoring system design based on WSN and GPRS networks. In: 2015 IEEE International Conference on Cyber Technology in Automation, Control, and Intelligent Systems (CYBER). pp 795‑798. https://doi.org/10.1109/CYBER.2015.7288044 .
Lin D, Zhang L, Xia X (2020) Hierarchical model predictive control of Venlo-type greenhouse climate for improving energy efficiency and reducing operating cost. J Clean Prod 264:121513. https://doi.org/10.1016/j.jclepro.2020.121513
Liu H et al (2019) Optimizing irrigation frequency and amount to balance yield, fruit quality and water use efficiency of greenhouse tomato. Agric Water Manag 226:105787. https://doi.org/10.1016/j.agwat.2019.105787
Liu Y, Bi C (2017) The design of greenhouse monitoring system based on ZigBee WSNs. In: IEEE International Conference on Computational Science and Engineering (CSE) and IEEE International Conference on Embedded and Ubiquitous Computing (EUC) 2:430‑433. https://doi.org/10.1109/CSE-EUC.2017.268 .
Liu L, Zhang Y (2017) Design of greenhouse environment monitoring system based on Wireless Sensor Network. In: 3rd International Conference on Control, Automation and Robotics (ICCAR). pp 463‑466. https://doi.org/10.1109/ICCAR.2017.7942739 .
Lovelli S, Potenza G, Castronuovo D, Perniola M, Candido V (2016) Yield, quality and water use efficiency of processing tomatoes produced under different irrigation regimes in Mediterranean environment. Ital J Agron. https://doi.org/10.4081/ija.2016.795
Luo Q, Qin L, Li X, G Wu (2016) The implementation of wireless sensor and control system in greenhouse based on ZigBee. In: 2016 35th Chinese Control Conference (CCC). pp 8474‑8478. https://doi.org/10.1109/ChiCC.2016.7554709 .
Mahbub M (2020) A smart farming concept based on smart embedded electronics, internet of things and wireless sensor network. Internet of Things 9:100161. https://doi.org/10.1016/j.iot.2020.100161
Mahdavian M, Wattanapongsakorn N (2017) Optimizing greenhouse lighting for advanced agriculture based on real time electricity market price. Math Probl Eng. https://doi.org/10.1155/2017/6862038
Maher A, Kamel E, Enrico F, Atif I, Abdelkader M (2016) An intelligent system for the climate control and energy savings in agricultural greenhouses. Energ Effi 9(6):1241–1255. https://doi.org/10.1007/s12053-015-9421-8
Mahmud MSA, Abidin MSZ, Mohamed Z, Rahman MKIA, Iida M (2019) Multi-objective path planner for an agricultural mobile robot in a virtual greenhouse environment. Comput Electron Agric 157:488–499. https://doi.org/10.1016/j.compag.2019.01.016
Majid M, Khan JN, Shah QMA, Masoodi KZ, Afroza B, Parvaze S (2021) Evaluation of hydroponic systems for the cultivation of Lettuce ( Lactuca sativa L., var. Longifolia) and comparison with protected soil-based cultivation. Agric Water Manage. https://doi.org/10.1016/j.agwat.2020.106572
Márquez-Vera MA, Ramos-Fernández JC, Cerecero-Natale LF, Lafont F, Balmat JF, Esparza-Villanueva JI (2016) Temperature control in a MISO greenhouse by inverting its fuzzy model. Comput Electron Agric 124:168–174. https://doi.org/10.1016/j.compag.2016.04.005
Martínez-Alvarez V, Martin-Gorriz B, Soto-García M (2016) Seawater desalination for crop irrigation—a review of current experiences and revealed key issues. Desalination 381:58–70. https://doi.org/10.1016/j.desal.2015.11.032
Marucci A, Cappuccini A (2016) Dynamic photovoltaic greenhouse: energy balance in completely clear sky condition during the hot period. Energy 102:302–312. https://doi.org/10.1016/j.energy.2016.02.053
Marucci A, Campiglia E, Colla G, Pagniello B (2011) Environmental impact of fertilization and pesticide application in vegetable cropping systems under greenhouse and open field conditions. J Food Agric Environ 9(34):840–846
Marucci A, Zambon I, Colantoni A, Monarca D (2018) A combination of agricultural and energy purposes: evaluation of a prototype of photovoltaic greenhouse tunnel. Renew Sustain Energy Rev 82:1178–1186. https://doi.org/10.1016/j.rser.2017.09.029
Mastrocicco M, Colombani N (2021) The issue of groundwater salinization in coastal areas of the mediterranean region: a review. Water. https://doi.org/10.3390/w13010090
Mat I, Mohd Kassim MR, Harun AN, Mat Yusoff I (2016) IoT in Precision Agriculture applications using Wireless Moisture Sensor Network. In: IEEE Conference on Open Systems (ICOS). pp 24‑29. https://doi.org/10.1109/ICOS.2016.7881983 .
Mazar M, Sahnoun M, Bettayeb B, Klement N, Louis A (2020) Simulation and optimization of robotic tasks for UV treatment of diseases in horticulture. Oper Res Int J. https://doi.org/10.1007/s12351-019-00541-w
Mazar M, Sahnoun M, Bettayeb B, Klement N (2018) Optimization of Robotized Tasks for the UV-C Treatment of Diseases in Horticulture. In: 2018 International Conference of the African Federation of Operational Research Societies (AFROS 2018). https://hal.archives-ouvertes.fr/hal-02053335 . Accessed 27 Nov 2020
Mekki M, Abdallah O, Amin MBM, Eltayeb M, Abdalfatah T, Babiker A (2015) Greenhouse monitoring and control system based on wireless Sensor Network. In: International Conference on Computing, Control, Networking, Electronics and Embedded Systems Engineering (ICCNEEE). pp 384–387. https://doi.org/10.1109/ICCNEEE.2015.7381396 .
Miniaoui H, Irungu P, Kaitibie S (2018) Contemporary issues in Qatar’s food security. Middle East Insights No. 185; Middle East Institute: Singapore
Ministry of Municipalities and Environment (2020) Qatar National Food Security Strategy 2018–2023; Ministry of Municipalities and Environment, Food Security Department. Doha, Qatar
Mohapatra AG, Lenka SK (2016) Neural network pattern classification and weather dependent fuzzy logic model for irrigation control in wsn based precision agriculture. Procedia Comput Sci 78:499–506. https://doi.org/10.1016/j.procs.2016.02.094
Montesano FF, Serio F, Mininni C, Signore A, Parente A, Santamaria P (2015) Tensiometer-based irrigation management of subirrigated soilless tomato: effects of substrate matric potential control on crop performance. Front Plant Sci. https://doi.org/10.3389/fpls.2015.01150
Montesano FF, van Iersel MW, Boari F, Cantore V, D’Amato G, Parente A (2018) Sensor-based irrigation management of soilless basil using a new smart irrigation system: effects of set-point on plant physiological responses and crop performance. Agric Water Manag 203:20–29. https://doi.org/10.1016/j.agwat.2018.02.019
Moon TW, Jung DH, Chang SH, Son JE (2018) Estimation of greenhouse CO 2 concentration via an artificial neural network that uses environmental factors. Hortic Environ Biotechnol 59(1):45–50. https://doi.org/10.1007/s13580-018-0015-1
Nakao N, Suzuki H, Kitajima T, Kuwahara A, Yasuno T (2017) Path planning and traveling control for pesticide-spraying robot in greenhouse. J Signal Process 21(4):175–178. https://doi.org/10.2299/jsp.21.175
Namany S, Al-Ansari T, Govindan R (2019) Sustainable energy, water and food nexus systems: a focused review of decision-making tools for efficient resource management and governance. J Clean Prod 225:610–626. https://doi.org/10.1016/j.jclepro.2019.03.304
Navarro-Hellín H, Torres-Sánchez R, Soto-Valles F, Albaladejo-Pérez C, López-Riquelme JA, Domingo-Miguel R (2015) A wireless sensors architecture for efficient irrigation water management. Agric Water Manag 151:64–74. https://doi.org/10.1016/j.agwat.2014.10.022
Nawandar NK, Satpute VR (2019) IoT based low cost and intelligent module for smart irrigation system. Comput Electron Agric 162:979–990. https://doi.org/10.1016/j.compag.2019.05.027
Nicolosi G, Volpe R, Messineo A (2017) An Innovative adaptive control system to regulate microclimatic conditions in a greenhouse. Energies 10(5):722. https://doi.org/10.3390/en10050722
Nikolaou G, Neocleous D, Katsoulas N, Kittas C (2019) Irrigation of greenhouse crops. Horticulturae 5(1):7. https://doi.org/10.3390/horticulturae5010007
Oliveira J, Boaventura-Cunha J, Oliveira PM (2016) Automation and control in greenhouses: state-of-the-art and future trends. In: Garrido P, Soares F, PauloMoreira A (eds) CONTROLO 2016, vol 402. Springer, Cham, pp 597–606
Chapter Google Scholar
Ouammi A, Achour Y, Zejli D, Dagdougui H (2020a) Supervisory model predictive control for optimal energy management of networked smart greenhouses integrated microgrid. IEEE Trans Autom Sci Eng 17(1):117–128. https://doi.org/10.1109/TASE.2019.2910756
Ouammi A, Achour Y, Dagdougui H, Zejli D (2020b) Optimal operation scheduling for a smart greenhouse integrated microgrid. Energy Sustain Dev 58:129–137. https://doi.org/10.1016/j.esd.2020.08.001
Oubehar H et al (2016) High-order sliding mode control of greenhouse temperature. Indo J Elec Eng Comput Sci. https://doi.org/10.11591/ijeecs.v4.i3.pp548-554
Ozturk HH (2017) Present status and future prospects of geothermal energy use for greenhouse heating in Turkey. Sci Papers Hortic 61:441–450
Pahuja R, Verma HK, Uddin M (2015) Implementation of greenhouse climate control simulator based on dynamic model and vapor pressure deficit controller. Eng Agric Environ Food 8(4):273–288. https://doi.org/10.1016/j.eaef.2015.04.009
Pakari A, Ghani S (2019) Evaluation of a novel greenhouse design for reduced cooling loads during the hot season in subtropical regions. Sol Energy 181:234–242. https://doi.org/10.1016/j.solener.2019.02.006
Papachristou M, Mendrinos D, Dalampakis P, Arvanitis A, Karytsas C, Andritsos N (2016) Geothermal Energy Use, Country Update for Greece. In: 2016 European Geothermal Congress (EGC), Strasbourg, France
Pascual RL, Sanchez DMR, Naces DLE, Nuñez WA (2015) A wireless sensor network using XBee for precision agriculture of sweet potatoes ( Ipomoea batatas ). In: International Conference on Humanoid, Nanotechnology, Information Technology, Communication and Control, Environment and Management (HNICEM). pp 1‑4. https://doi.org/10.1109/HNICEM.2015.7393212 .
Patil R, Gawande R (2016) A review on solar tunnel greenhouse drying system. Renew Sustain Energy Rev 56:196–214. https://doi.org/10.1016/j.rser.2015.11.057
Paucek I et al (2020) Supplementary LED interlighting improves yield and precocity of greenhouse tomatoes in the mediterranean. Agronomy 10(7):1002. https://doi.org/10.3390/agronomy10071002
Pawlowski A, Sánchez-Molina JA, Guzmán JL, Rodríguez F, Dormido S (2017) Evaluation of event-based irrigation system control scheme for tomato crops in greenhouses. Agric Water Manag 183:16–25. https://doi.org/10.1016/j.agwat.2016.08.008
Pazzagli PT, Weiner J, Liu F (2016) Effects of CO 2 elevation and irrigation regimes on leaf gas exchange, plant water relations, and water use efficiency of two tomato cultivars. Agric Water Manag 169:26–33. https://doi.org/10.1016/j.agwat.2016.02.015
Pearce GK (2008) UF/MF pre-treatment to RO in seawater and wastewater reuse applications: a comparison of energy costs. Desalination 222:66–73
Planning and Statistics Authority in Qatar (2012) Economic and agricultural statistics 2012; Planning and statistics authority: Doha, Qatar
Planning and Statistics Authority in Qatar (2013a) Physical and climate features Statistics 2013; Planning and Statistics Authority: Doha, Qatar
Planning and Statistics Authority in Qatar (2013b) Economic and Agricultural statistics 2013 Planning and statistics authority: Doha, Qatar
Planning and Statistics Authority in Qatar (2014) Economic and agricultural statistics 2014; Planning and Statistics Authority: Doha, Qatar
Planning and Statistics Authority in Qatar (2015a) Physical and climate features Statistics 2015; Planning and Statistics Authority: Doha, Qatar
Planning and Statistics Authority in Qatar (2015b) Economic and agricultural statistics 2015; Planning and statistics authority: Doha, Qatar
Planning and Statistics Authority in Qatar (2017a) Water statistics in the state of Qatar 2017; Planning and statistics authority: Doha, Qatar
Planning and Statistics Authority in Qatar (2017b) Economic and agricultural statistics 2017; Planning and statistics authority: Doha, Qatar
Planning and Statistics Authority in Qatar (2018) Qatar in competitiveness yearbook 2018; Planning and Statistics Authority: Doha, Qatar
Planning and Statistics Authority in Qatar (2019) Infographic of Water Statistic in Qatar 2019; Planning and Statistics Authority: Doha, Qatar
Planning and Statistics Authority in Qatar (2020a) Economic and Agricultural Statistics 2020; Planning and Statistics Authority: Doha, Qatar
Planning and Statistics Authority in Qatar (2020b) Physical and climate features Statistics 2020; Planning and Statistics Authority: Doha, Qatar
Planning and Statistics Authority of Qatar Homepage. https://www.psa.gov.qa/en/Pages/default.aspx
Qatar Development Bank Homepage (2020). https://www.qdb.qa/en/Pages/Green-House-Financing.aspx . Accessed 8 Oct 2020
Qatar International Islamic Bank (2019) Annual Report 2018; QIIB: Doha, Qatar
Qatar Tribune Front (2020) https://www.qatar-tribune.com/news-details/id/164815 . Accessed 28 Sept 2020
Rabara RC, Behrman G, Timbol T, Rushton PJ (2017) Effect of spectral quality of monochromatic LED lights on the growth of artichoke seedlings. Front Plant Sci. https://doi.org/10.3389/fpls.2017.00190
Raheemah A, Sabri N, Salim MS, Ehkan P, Ahmad RB (2016) New empirical path loss model for wireless sensor networks in mango greenhouses. Comput Electron Agric 127:553–560. https://doi.org/10.1016/j.compag.2016.07.011
Rahil MH, Qanadillo A (2015) Effects of different irrigation regimes on yield and water use efficiency of cucumber crop. Agric Water Manag 148:10–15. https://doi.org/10.1016/j.agwat.2014.09.005
Rahmawati D, Ulum M, Setiawan H (2018) Design of android base fuzzy wireles sensor network for mini smart green house. J Phys Conf Ser 1028:012051. https://doi.org/10.1088/1742-6596/1028/1/012051
Rameshwaran P, Tepe A, Yazar A, Ragab R (2016) Effects of drip-irrigation regimes with saline water on pepper productivity and soil salinity under greenhouse conditions. Sci Hortic 199:114–123. https://doi.org/10.1016/j.scienta.2015.12.007
Rao Y, Jiang Z, Lazarovitch N (2016) Investigating signal propagation and strength distribution characteristics of wireless sensor networks in date palm orchards. Comput Electron Agric 124:107–120. https://doi.org/10.1016/j.compag.2016.03.023
Rizk H, Habib MK (2018) Robotized early plant health monitoring system. In: IECON 2018—44th Annual Conference of the IEEE Industrial Electronics Society. pp 3795‑3800. https://doi.org/10.1109/IECON.2018.8592833
Roldán J, Garcia-Aunon P, Garzón M, de León J, del Cerro J, Barrientos A (2016) Heterogeneous multi-robot system for mapping environmental variables of greenhouses. Sensors 16(7):1018. https://doi.org/10.3390/s16071018
Roumi S, Yousefi H, Noorollahi Y, Bekhrad K, Zarrouk SJ (2017) Direct applications of geothermal energy; economic and environmental assessment: Meshkin-Shahr, Iran. Proceedings of 42nd Workshop on Geothermal Reservoir Engineering Stanford University. pp 13
Sayeed M (2016) Qatar’s national emission inventory report. figshare. https://doi.org/10.6084/m9.figshare.4154238.v12016
Schiattone MI et al (2018) Impact of irrigation regime and nitrogen rate on yield, quality and water use efficiency of wild rocket under greenhouse conditions. Sci Hortic 229:182–192. https://doi.org/10.1016/j.scienta.2017.10.036
Schor N, Berman S, Dombrovsky A, Elad Y, Ignat T, Bechar A (2017) Development of a robotic detection system for greenhouse pepper plant diseases. Precision Agric 18(3):394–409. https://doi.org/10.1007/s11119-017-9503-z
Schor N, Bechar A, Ignat T, Dombrovsky A, Elad Y, Berman S (2016) Robotic disease detection in greenhouses: combined detection of powdery mildew and tomato spotted wilt virus. In: IEEE Robot. Autom. Lett 1(1):354‑360. https://doi.org/10.1109/LRA.2016.2518214
Sharma H, Shukla MK, Bosland PW, Steiner R (2017) Soil moisture sensor calibration, actual evapotranspiration, and crop coefficients for drip irrigated greenhouse chile peppers. Agric Water Manag 179:81–91. https://doi.org/10.1016/j.agwat.2016.07.001
Shetty HM, Pai K, Mallya N, Pratheeksha (2021) Fully automated hydroponics system for smart farming. I J Eng Manufac 4:33–41. https://doi.org/10.5815/ijem.2021.04.04
Shyam IM, Al-Helal AK, Singh GN, Tiwari S (2015) Performance evaluation of photovoltaic thermal greenhouse dryer and development of characteristic curve. J Renew Sustain Energy 7(3):033109. https://doi.org/10.1063/1.4921408
Singh VK (2017) Prediction of greenhouse micro-climate using artificial neural network. Appl Ecol Env Res 15(1):767–778. https://doi.org/10.15666/aeer/1501_767778
Singh S, Singh DR, Velmurugan A, Jaisankar I, Swarnam TP (2008) Chapter 23—coping with climatic uncertainties through improved production technologies in tropical island conditions. In: Sivaperuman C, Velmurugan A, Singh AK, Jaisankar I (eds) Biodiversity and climate change adaptation in tropical islands. Academic Press, London, pp 623–666
Singh D, Basu C, Meinhardt-Wollweber M, Roth B (2015) LEDs for energy efficient greenhouse lighting. Renew Sustain Energy Rev 49:139–147. https://doi.org/10.1016/j.rser.2015.04.117
Singhal R, Kumar R, Neeli S (2020) Receding horizon control based on prioritised multi-operational ranges for greenhouse environment regulation. Comput Electron Agric 180:105840. https://doi.org/10.1016/j.compag.2020.105840
Sivamani S, Choi J, Bae K, Ko H, Cho Y (2018) A smart service model in greenhouse environment using event-based security based on wireless sensor network: a smart service model in greenhouse environment using event-based security based on wireless sensor network. Concurrency Computat 30(2):e4240. https://doi.org/10.1002/cpe.4240
Son JE, Kim HJ, Ahn TI (2020) Hydroponic systems. In: Kozai T, Niu G, Takagaki M (eds) Plant factory: an indoor vertical farming system for efficient quality food production, 2nd edn. Academic Press, Cambridge, pp 273–283
Specht K, Zoll F, Schümann H, Bela J, Kachel J, Robischon M (2019) How Will we eat and produce in the cities of the future? From edible insects to vertical farming—a study on the perception and acceptability of new approaches. Sustainability. https://doi.org/10.3390/su11164315
Su Y, Xu L, Goodman ED (2017) Greenhouse climate fuzzy adaptive control considering energy saving. Int J Control Autom Syst 15(4):1936–1948. https://doi.org/10.1007/s12555-016-0220-6
Taki M, Ajabshirchi Y, Ranjbar SF, Matloobi M (2016) Application of Neural Networks and multiple regression models in greenhouse climate estimation. Agric Eng Int 18(3):29
Tang Y et al (2019) 3D intelligent supplement light illumination using hybrid sunlight and LED for greenhouse plants. Optik 183:367–374. https://doi.org/10.1016/j.ijleo.2019.02.002
Taşkın D, Taşkin C, Yazar S (2018) Developing a bluetooth low energy sensor node for greenhouse in precision agriculture as internet of things Application. Adv Sci Technol Res J. http://yadda.icm.edu.pl/baztech/element/bwmeta1.element.baztech-c7f9f014-2d25-4d60-a757-e55688ca379a . Accessed 26 Jan 2020
Thirukkuralkani KN, Kaushik S, Ninan CJ (2018) LabVIEW based greenhouse automation system through wireless protocol. In: 2nd International Conference on Inventive Systems and Control (ICISC). pp 1427‑1430. https://doi.org/10.1109/ICISC.2018.8399082 .
Timeanddate Homepage (2020) https://www.timeanddate.com/weather/qatar/doha . Accessed 27 Dec 2020
Tiwari S, Tiwari GN (2016a) Exergoeconomic analysis of photovoltaic-thermal (PVT) mixed mode greenhouse solar dryer. Energy 114:155–164. https://doi.org/10.1016/j.energy.2016.07.132
Tiwari S, Tiwari GN (2016b) Thermal analysis of photovoltaic-thermal (PVT) single slope roof integrated greenhouse solar dryer. Sol Energy 138:128–136. https://doi.org/10.1016/j.solener.2016.09.014
Tiwari S, Tiwari GN (2017) Thermal analysis of photovoltaic thermal integrated greenhouse system (PVTIGS) for heating of slurry in potable biogas plant: an experimental study. Sol Energy 155:203–211. https://doi.org/10.1016/j.solener.2017.06.021
Tiwari S, Bhatti J, Tiwari GN, Al-Helal IM (2016b) Thermal modelling of photovoltaic thermal (PVT) integrated greenhouse system for biogas heating. Sol Energy 136:639–649. https://doi.org/10.1016/j.solener.2016.07.048
Tiwari S, Agrawal S, Tiwari GN (2018) PVT air collector integrated greenhouse dryers. Renew Sustain Energy Rev 90:142–159. https://doi.org/10.1016/j.rser.2018.03.043
Tiwari S, Tripathi R, Tiwari GN (2016a) Effect of packing factor of photovoltaic module on performance of photovoltaic-thermal (PVT) greenhouse solar dryer. In: 2016 International Conference on Emerging Trends in Electrical Electronics Sustainable Energy Systems (ICETEESES). pp 52‑55
Treftz C, Omaye ST (2016) Comparision between hydroponic and soil systems for growing strawberries in a greenhouse. Int J Agric Extension 3(3):195–200
Trypanagnostopoulos G, Kavga A, Souliotis Μ, Tripanagnostopoulos Y (2017) Greenhouse performance results for roof installed photovoltaics. Renew Energy 111:724–731. https://doi.org/10.1016/j.renene.2017.04.066
Tuomisto HL (2019) Vertical farming and cultured meat: immature technologies for urgent problems. One Earth 1(3):275–277. https://doi.org/10.1016/j.oneear.2019.10.024
Ullah I, Hanping M, Chuan Z, Javed Q, Azeem A (2017) Optimization of irrigation and nutrient concentration based on economic returns, substrate salt accumulation and water use efficiency for tomato in greenhouse. Arch Agronomy Soil Sci 63(12):1748–1762. https://doi.org/10.1080/03650340.2017.1306641
United Nations Homepage (2020a) https://www.un.org/en/sections/issues-depth/population/ . Accessed 26 Dec 2020
United Nations Homepage (2020b) https://www.un.org/en/sections/issues-depth/food/index.html . Accessed 26 Dec 2020
United Nations Homepage (2020c) https://www.un.org/en/sections/issues-depth/water/index.html . Accessed 26 Dec 2020
United Nations University (2022) The Nexus Approach to Environmental Resource Management. https://flores.unu.edu/en/research/nexus#overview . Accessed 1 Feb 2022
Urbancl D, Trop P, Goričanec D (2016) Geothermal heat potential—the source for heating greenhouses in Southestern Europe. Therm Sci 20(4):1061–1071
Uyeh DD et al (2019) Evolutionary greenhouse layout optimization for rapid and safe robot navigation. IEEE Access 7:88472–88480. https://doi.org/10.1109/ACCESS.2019.2926566
van Iersel MW (2017) Optimizing LED lighting in controlled environment agriculture. In: Dutta Gupta S (ed) Light emitting diodes for agriculture. Singapore, Springer Singapore, pp 59–80
van Beveren PJM, Bontsema J, van Straten G, van Henten EJ (2015) Optimal control of greenhouse climate using minimal energy and grower defined bounds. Appl Energy 159:509–519. https://doi.org/10.1016/j.apenergy.2015.09.012
van Beveren PJM, Bontsema J, van Ooster A, van Straten G, van Henten EJ (2020) Optimal utilization of energy equipment in a semi-closed greenhouse. Comput Electron Agric 179:105800. https://doi.org/10.1016/j.compag.2020.105800
Viršilė A, Olle M, Duchovskis P (2017) LED lighting in horticulture. In: DuttaGupta S (ed) Light emitting diodes for agriculture. Singapore, Springer Singapore, pp 113–147
Walters KJ, Currey CJ (2015) Hydroponic greenhouse basil production: comparing systems and cultivars. HortTechnology 25(5):645–650. https://doi.org/10.21273/HORTTECH.25.5.645
Wang L, Zhang H (2018) An adaptive fuzzy hierarchical control for maintaining solar greenhouse temperature. Comput Electron Agric 155:251–256. https://doi.org/10.1016/j.compag.2018.10.023
Wang Y, Wei R, Xu L (1956) Dynamic control of supplemental lighting for greenhouse. AIP Conf Proc 1:020050. https://doi.org/10.1063/1.5034302
Wang J, Niu X, Zheng L, Zheng C, Wang Y (2016) Wireless mid-infrared spectroscopy sensor network for automatic carbon dioxide fertilization in a greenhouse environment. Sensors 16(11):1941. https://doi.org/10.3390/s16111941
Wang H, Sánchez-Molina JA, Li M, Berenguel M, Yang XT, Bienvenido JF (2017a) Leaf area index estimation for a greenhouse transpiration model using external climate conditions based on genetics algorithms, back-propagation neural networks and nonlinear autoregressive exogenous models. Agric Water Manag 183:107–115. https://doi.org/10.1016/j.agwat.2016.11.021
Wang LL et al (2017b) Development of a tomato harvesting robot used in greenhouse. Int J Agric Biol Eng 10:140–149. https://doi.org/10.25165/j.ijabe.20171004.3204
Wang H et al (2019) Optimal drip fertigation management improves yield, quality, water and nitrogen use efficiency of greenhouse cucumber. Sci Hortic 243:357–366. https://doi.org/10.1016/j.scienta.2018.08.050
Wang X et al (2020) Effects of schedules of subsurface drip irrigation with air injection on water consumption, yield components and water use efficiency of tomato in a greenhouse in the North China Plain. Sci Hortic 269:109396. https://doi.org/10.1016/j.scienta.2020.109396
Warner LA, Lamm AJ, Beattie P, White SA, Fisher PR (2018) Identifying opportunities to promote water conservation practices among nursery and greenhouse growers. Horts 53(7):958–962. https://doi.org/10.21273/HORTSCI12906-18
Warner LA, Lamm AJ, White SA, Fisher PR, Beattie PN (2020) A new perspective on adoption: delivering water conservation extension programming to nursery and greenhouse growers. J Agric Educ 61(1):172–189
Woo S et al (2020) Analyses of work efficiency of a strawberry-harvesting robot in an automated greenhouse. Agronomy 10(11):1751. https://doi.org/10.3390/agronomy10111751
Wspanialy P, Moussa M (2016) Early powdery mildew detection system for application in greenhouse automation. Comput Electron Agric 127:487–494. https://doi.org/10.1016/j.compag.2016.06.027
Xing X, Song J, Lin L, Tian M, Lei Z (2017) Development of intelligent information monitoring system in greenhouse based on wireless sensor network. In: 4th International Conference on Information Science and Control Engineering (ICISCE). pp 970‑974. https://doi.org/10.1109/ICISCE.2017.205 .
Xiukang W, Yingying X (2016) Evaluation of the effect of irrigation and fertilization by drip fertigation on tomato yield and water use efficiency in greenhouse. Int J Agronomy. https://doi.org/10.1155/2016/3961903
Xu Y-H, Wu W-L, Xu Y, Tham M-L, Ramli N (2016) A framework of fuzzy control-based intelligent control system for greenhouse. AIR 6(1):1. https://doi.org/10.5430/air.v6n1p1
Yang H et al (2017) Improved water use efficiency and fruit quality of greenhouse crops under regulated deficit irrigation in northwest China. Agric Water Manag 179:193–204. https://doi.org/10.1016/j.agwat.2016.05.029
Yao Z, Yan G, Wang R, Zheng X, Liu C, Butterbach-Bahl K (2019) Drip irrigation or reduced N-fertilizer rate can mitigate the high annual N 2 O+NO fluxes from Chinese intensive greenhouse vegetable systems. Atmos Environ 212:183–193. https://doi.org/10.1016/j.atmosenv.2019.05.056
Yeşil V, Tatar Ö (2020) An innovative approach to produce forage crops: barley fodder in vertical farming system. Sci Papers Ser A 63(1):723–728
Yuan T, Zhang S, Sheng X, Wang D, Gong Y, Li W (2016) An autonomous pollination robot for hormone treatment of tomato flower in greenhouse. In: 3rd International Conference on Systems and Informatics (ICSAI). pp 108‑113. https://doi.org/10.1109/ICSAI.2016.7810939
Zhang L, Li C, Jia Y, Xiao Z (2015) Design of greenhouse environment remote monitoring system based on android platform. Chem Eng Trans 46:739–744
Zhang H, Burr J, Zhao F (2017) A comparative life cycle assessment (LCA) of lighting technologies for greenhouse crop production. J Clean Prod 140:705–713. https://doi.org/10.1016/j.jclepro.2016.01.014
Zhang M, Whitman CM, Runkle ES (2019) Manipulating growth, color, and taste attributes of fresh cut lettuce by greenhouse supplemental lighting. Sci Hortic 252:274–282. https://doi.org/10.1016/j.scienta.2019.03.051
Zhao Y, Gong L, Zhou B, Huang Y, Liu C (2016) Detecting tomatoes in greenhouse scenes by combining AdaBoost classifier and colour analysis. Biosys Eng 148:127–137. https://doi.org/10.1016/j.biosystemseng.2016.05.001
Zhou YH, Duan JG (2016) Design and simulation of a wireless sensor network greenhouse-monitoring system based on 3G network communication. Int J Online Biomed Eng (iJOE) 12(5):48–52
Download references
Acknowledgements
The authors are grateful to Qatar National Research Fund (QNRF) for funding and supporting the M-NEX Project (Grant No. BFSUGI01-1120-170005) in Qatar. The M-NEX is a project of the Collaborative Research Area Belmont Forum (Grant No. 11314551).
Open Access funding provided by the Qatar National Library.
Author information
Authors and affiliations.
Biotechnology Program, Center for Sustainable Development, College of Arts and Sciences, Qatar University, 2713, Doha, Qatar
Theodora Karanisa, Ahmed Ouammi & Sami Sayadi
Electrical Systems and Telecommunications Engineering Laboratory, The National School of Applied Sciences of Kenitra, 14000, Kenitra, Morocco
Yasmine Achour
You can also search for this author in PubMed Google Scholar
Corresponding author
Correspondence to Theodora Karanisa .
Ethics declarations
Conflict of interest.
The authors declare no conflict of interest.
Rights and permissions
Open Access This article is licensed under a Creative Commons Attribution 4.0 International License, which permits use, sharing, adaptation, distribution and reproduction in any medium or format, as long as you give appropriate credit to the original author(s) and the source, provide a link to the Creative Commons licence, and indicate if changes were made. The images or other third party material in this article are included in the article's Creative Commons licence, unless indicated otherwise in a credit line to the material. If material is not included in the article's Creative Commons licence and your intended use is not permitted by statutory regulation or exceeds the permitted use, you will need to obtain permission directly from the copyright holder. To view a copy of this licence, visit http://creativecommons.org/licenses/by/4.0/ .
Reprints and permissions
About this article
Karanisa, T., Achour, Y., Ouammi, A. et al. Smart greenhouses as the path towards precision agriculture in the food-energy and water nexus: case study of Qatar. Environ Syst Decis 42 , 521–546 (2022). https://doi.org/10.1007/s10669-022-09862-2
Download citation
Accepted : 11 May 2022
Published : 02 June 2022
Issue Date : December 2022
DOI : https://doi.org/10.1007/s10669-022-09862-2
Share this article
Anyone you share the following link with will be able to read this content:
Sorry, a shareable link is not currently available for this article.
Provided by the Springer Nature SharedIt content-sharing initiative
- Greenhouse technology
- controlled environment agriculture (CEA)
- Precision agriculture
- Sustainable development
- Food security
- Find a journal
- Publish with us
- Track your research

IMAGES
VIDEO
COMMENTS
Abstract. The drive toward efficient yet sustainable agriculture in the contemporary world necessitates the adoption of smart farming. Smart farming refers to a set of solutions that increase agricultural efficiency, mainly through the use of information and communication technologies. We will write a custom essay on your topic.
Smart farming reduces the ecological footprint of farming. Minimized or site-specific application of inputs, such as fertilizers and pesticides, in precision agriculture systems will mitigate leaching problems as well as the emission of greenhouse gases ( 6 ). With current ICT, it is possible to create a sensor network allowing for almost ...
Therefore, smart farming has a real potential to deliver a more productive and sustainable form of agricultural production, based on a more precise and resource-efficient approach. New farms will finally realize the eternal dream of mankind. It'll feed our population, which may explode to 9.8 billion by 2050.
Smart agriculture plays a crucial role in enhancing environmental sustainability through improved resource utilisation efficiency. PA technologies, like crop editing and variable-rate technologies, contribute to resource efficiency (Clapp and Ruder, 2020). This leads to increased productivity, reduces the necessity of land clearing and helps ...
The smart farming is a subset of precision agriculture, which is designed to control the suitable environments. for crops inside a greenhouse by using the least resources. It combines modern ...
Smart farming offers more sustainable methods to conserve resources, minimize chemicals, and shorten the time to market, so everyone can reach cleaner, better-quality, and more affordable food with more sustainable, fresher options from growers, greenhouses, and farmers. In addition, the plant crop is produced in a healthier way by managing it ...
The applications of Smart Farming can contribute to enhancing sustainable development [197] [198] [199]. Smart Farming can provide numerous advantages to the agricultural industry, including ...
Smart agriculture technology based on the Internet of Things (IoT) technologies has many advantages related to all agricultural processes and practices in real-time, which include irrigation and plant protection, improving product quality, fertilization process control, and disease prediction, etc.(Adamides et al., 2020).The advantages of smart agriculture can be summarized as follows: 1 ...
Smart farming reformed the agriculture scenario, and it offers a path towards sustainable agriculture through versatile technologies. In this paper, various smart farming practices in the agriculture environment are discussed. The possibilities of these smart practices might lead to improved productivity in the farms.
The forecasted market value of smart farming worldwide is 23.1 billion dollars (including precision farming). The following chapters in Part V underlines the importance of smart farming in terms of agricultural productivity, environmental impact, food security, and sustainability, with applications in the areas of crops, soil, biodiversity ...
Given the importance of technologies in transforming agricultural practices, smart technologies are the premise under which this book was developed. Fig. 1.1 illustrates the relationships among the three CSA pillars and the chapters of this book that address the same pillars. Download : Download full-size image.
The review paper [12] examines the application of smart agriculture technologies in crop production, animal husbandry and harvesting, and describes the advantages and challenges of implementation ...
Smart farming is a farm management concept that may use Internet of Things (IoT) to overcome the current challenges of food production. This work uses the preferred reporting items for systematic reviews (PRISMA) methodology to systematically review the existing literature on smart farming with IoT. The review aims to identify the main devices ...
The future of connectivity. Without a solid connectivity infrastructure, however, none of this is possible. If connectivity is implemented successfully in agriculture, the industry could tack on $500 billion in additional value to the global gross domestic product by 2030, according to our research. This would amount to a 7 to 9 percent ...
Smart farming is not such an established term yet as precision agriculture, but it where precision agriculture is mainly taking in-field variability into account, smart farming goes beyond that by basing management tasks not only on location but also on data, enhanced by context- and situation awareness, triggered by real-time events (Citation ...
The information that crops offer is turned into profitable decisions only when efficiently managed. Current advances in data management are making Smart Farming grow exponentially as data have become the key element in modern agriculture to help producers with critical decision-making. Valuable advantages appear with objective information acquired through sensors with the aim of maximizing ...
This study is about using Internet of Things (IoT) technologies and techniques to enhance agriculture. This article is meant to serve as an introduction to IoT-based applications in agriculture by identifying need for such tools and explaining how they support agriculture. Export citation and abstract BibTeX RIS. Previous article in issue.
As agriculture gets increasingly complex, smart farming has emerged as a tool for dealing with current challenges. However, fast decision-making is still a challenging task for decision-makers at the regional and national levels. Furthermore, smart agriculture is the cutting edge technology in the current scenario.
In response to these challenges, the concept of Climate-smart Agriculture (CSA) has emerged as a holistic approach to end food security and promote sustainable development while addressing climate change issues. CSA is a set of agricultural practices and technologies which simultaneously boost productivity, enhance resilience and reduce GHG ...
Digital agriculture, sometimes known as smart farming or e-agriculture, is tools that digitally collect, store, analyze, and share electronic data and/or information in agriculture.The Food and Agriculture Organization of the United Nations has described the digitalization process of agriculture as the digital agricultural revolution. Other definitions, such as those from the United Nations ...
Greenhouse farming is essential in increasing domestic crop production in countries with limited resources and a harsh climate like Qatar. Smart greenhouse development is even more important to overcome these limitations and achieve high levels of food security. While the main aim of greenhouses is to offer an appropriate environment for high-yield production while protecting crops from ...
Farmers need help to develop climate-smart agricultural practices that can adapt to and mitigate the impacts of climate change, but also have the potential to increase food production. Natural resources, such as land, water, soil and genetic resources, must be better managed so that more productive and resilient agriculture can be achieved.
This paper aims to analyze the huge opportunities of smart farming by utilizing the potential of millennial farmers as actors and analyzing various government policies to support smart farming 4.0.