- Original article
- Open access
- Published: 09 April 2020

Why does peer instruction benefit student learning?
- Jonathan G. Tullis 1 &
- Robert L. Goldstone 2
Cognitive Research: Principles and Implications volume 5 , Article number: 15 ( 2020 ) Cite this article
84k Accesses
46 Citations
52 Altmetric
Metrics details
In peer instruction, instructors pose a challenging question to students, students answer the question individually, students work with a partner in the class to discuss their answers, and finally students answer the question again. A large body of evidence shows that peer instruction benefits student learning. To determine the mechanism for these benefits, we collected semester-long data from six classes, involving a total of 208 undergraduate students being asked a total of 86 different questions related to their course content. For each question, students chose their answer individually, reported their confidence, discussed their answers with their partner, and then indicated their possibly revised answer and confidence again. Overall, students were more accurate and confident after discussion than before. Initially correct students were more likely to keep their answers than initially incorrect students, and this tendency was partially but not completely attributable to differences in confidence. We discuss the benefits of peer instruction in terms of differences in the coherence of explanations, social learning, and the contextual factors that influence confidence and accuracy.
Significance
Peer instruction is widely used in physics instruction across many universities. Here, we examine how peer instruction, or discussing one’s answer with a peer, affects students’ decisions about a class assignment. Across six different university classes, students answered a question, discussed their answer with a peer, and finally answered the question again. Students’ accuracy consistently improved through discussion with a peer. Our peer instruction data show that students were hesitant to switch away from their initial answer and that students did consider both their own confidence and their partner’s confidence when making their final decision, in accord with basic research about confidence in decision making. More broadly, the data reveal that peer discussion helped students select the correct answer by prompting them to create new knowledge. The benefit to student accuracy that arises when students discuss their answers with a partner is a “process gain”, in which working in a group yields better performance than can be predicted from individuals’ performance alone.
Peer instruction is specific evidence-based instructional strategy that is well-known and widely used, particularly in physics (Henderson & Dancy, 2009 ). In fact, peer instruction has been advocated as a part of best methods in science classrooms (Beatty, Gerace, Leonard, & Dufresne, 2006 ; Caldwell, 2007 ; Crouch & Mazur, 2001 ; Newbury & Heiner, 2012 ; Wieman et al., 2009 ) and over a quarter of university physics professors report using peer instruction (Henderson & Dancy, 2009 ). In peer instruction, instructors pose a challenging question to students, students answer the question individually, students discuss their answers with a peer in the class, and finally students answer the question again. There are variations of peer instruction in which instructors show the class’s distribution of answers before discussion (Nielsen, Hansen-Nygård, & Stav, 2012 ; Perez et al., 2010 ), in which students’ answers are graded for participation or for correctness (James, 2006 ), and in which instructors’ norms affect whether peer instruction offers opportunities for answer-seeking or for sense-making (Turpen & Finkelstein, 2007 ).
Despite wide variations in its implementation, peer instruction consistently benefits student learning. Switching classroom structure from didactic lectures to one centered around peer instruction improves learners’ conceptual understanding (Duncan, 2005 ; Mazur, 1997 ), reduces student attrition in difficult courses (Lasry, Mazur, & Watkins, 2008 ), decreases failure rates (Porter, Bailey-Lee, & Simon, 2013 ), improves student attendance (Deslauriers, Schelew, & Wieman, 2011 ), and bolsters student engagement (Lucas, 2009 ) and attitudes to their course (Beekes, 2006 ). Benefits of peer instruction have been found across many fields, including physics (Mazur, 1997 ; Pollock, Chasteen, Dubson, & Perkins, 2010 ), biology (Knight, Wise, & Southard, 2013 ; Smith, Wood, Krauter, & Knight, 2011 ), chemistry (Brooks & Koretsky, 2011 ), physiology (Cortright, Collins, & DiCarlo, 2005 ; Rao & DiCarlo, 2000 ), calculus (Lucas, 2009 ; Miller, Santana-Vega, & Terrell, 2007 ), computer science (Porter et al., 2013 ), entomology (Jones, Antonenko, & Greenwood, 2012 ), and even philosophy (Butchart, Handfield, & Restall, 2009 ). Additionally, benefits of peer instruction have been found at prestigious private universities, two-year community colleges (Lasry et al., 2008 ), and even high schools (Cummings & Roberts, 2008 ). Peer instruction benefits not just the specific questions posed during discussion, but also improves accuracy on later similar problems (e.g., Smith et al., 2009 ).
One of the consistent empirical hallmarks of peer instruction is that students’ answers are more frequently correct following discussion than preceding it. For example, in introductory computer science courses, post-discussion performance was higher on 70 out of 71 questions throughout the semester (Simon, Kohanfars, Lee, Tamayo, & Cutts, 2010 ). Further, gains in performance from discussion are found on many different types of questions, including recall, application, and synthesis questions (Rao & DiCarlo, 2000 ). Performance improvements are found because students are more likely to switch from an incorrect answer to the correct answer than from the correct answer to an incorrect answer. In physics, 59% of incorrect answers switched to correct following discussion, but only 13% of correct answers switched to incorrect (Crouch & Mazur, 2001 ). Other research on peer instruction shows the same patterns: 41% of incorrect answers are switched to correct ones, while only 18% of correct answers are switched to incorrect (Morgan & Wakefield, 2012 ). On qualitative problem-solving questions in physiology, 57% of incorrect answers switched to correct after discussion, and only 7% of correct answers to incorrect (Giuliodori, Lujan, & DiCarlo, 2006 ).
There are two explanations for improvements in pre-discussion to post-discussion accuracy. First, switches from incorrect to correct answers may be driven by selecting the answer from the peer who is more confident. When students discuss answers that disagree, they may choose whichever answer belongs to the more confident peer. Evidence about decision-making and advice-taking substantiates this account. First, confidence is correlated with correctness across many settings and procedures (Finley, Tullis, & Benjamin, 2010 ). Students who are more confident in their answers are typically more likely to be correct. Second, research examining decision-making and advice-taking indicates that (1) the less confident you are, the more you value others’ opinions (Granovskiy, Gold, Sumpter, & Goldstone, 2015 ; Harvey & Fischer, 1997 ; Yaniv, 2004a , 2004b ; Yaniv & Choshen-Hillel, 2012 ) and (2) the more confident the advisor is, the more strongly they influence your decision (Kuhn & Sniezek, 1996 ; Price & Stone, 2004 ; Sah, Moore, & MacCoun, 2013 ; Sniezek & Buckley, 1995 ; Van Swol & Sniezek, 2005 ; Yaniv, 2004b ). Consequently, if students simply choose their final answer based upon whoever is more confident, accuracy should increase from pre-discussion to post-discussion. This explanation suggests that switches in answers should be driven entirely by a combination of one’s own initial confidence and one’s partner’s confidence. In accord with this confidence view, Koriat ( 2015 ) shows that an individual’s confidence typically reflects the group’s most typically given answer. When the answer most often given by group members is incorrect, peer interactions amplify the selection of and confidence in incorrect answers. Correct answers have no special draw. Rather, peer instruction merely amplifies the dominant view through differences in the individual’s confidence.
In a second explanation, working with others may prompt students to verbalize explanations and verbalizations may generate new knowledge. More specifically, as students discuss the questions, they need to create a common representation of the problem and answer. Generating a common representation may compel students to identify gaps in their existing knowledge and construct new knowledge (Schwartz, 1995 ). Further, peer discussion may promote students’ metacognitive processes of detecting and correcting errors in their mental models. Students create more new knowledge and better diagnostic tests of answers together than alone. Ultimately, then, the new knowledge and improved metacognition may make the correct answer appear more compelling or coherent than incorrect options. Peer discussion would draw attention to coherent or compelling answers, more so than students’ initial confidence alone and the coherence of the correct answer would prompt students to switch away from incorrect answers. Similarly, Trouche, Sander, and Mercier ( 2014 ) argue that interactions in a group prompt argumentation and discussion of reasoning. Good arguments and reasoning should be more compelling to change individuals’ answers than confidence alone. Indeed, in a reasoning task known to benefit from careful deliberation, good arguments and the correctness of the answers change partners’ minds more than confidence in one’s answer (Trouche et al., 2014 ). This explanation predicts several distinct patterns of data. First, as seen in prior research, more students should switch from incorrect answers to correct than vice versa. Second, the intrinsic coherence of the correct answer should attract students, so the likelihood of switching answers would be predicted by the correctness of an answer above and beyond differences in initial confidence. Third, initial confidence in an answer should not be as tightly related to initial accuracy as final confidence is to final accuracy because peer discussion should provide a strong test of the coherence of students’ answers. Fourth, because the coherence of an answer is revealed through peer discussion, student confidence should increase more from pre-discussion to post-discussion when they agree on the correct answers compared to agreeing on incorrect answers.
Here, we examined the predictions of these two explanations of peer instruction across six different classes. We specifically examined whether changes in answers are driven exclusively through the confidence of the peers during discussion or whether the coherence of an answer is better constructed and revealed through peer instruction than on one’s own. We are interested in analyzing cognitive processes at work in a specific, but common, implementation of classroom-based peer instruction; we do not intend to make general claims about all kinds of peer instruction or to evaluate the long-term effectiveness of peer instruction. This research is the first to analyze how confidence in one’s answer relates to answer-switching during peer instruction and tests the impact of peer instruction in new domains (i.e., psychology and educational psychology classes).
Participants
Students in six different classes participated as part of their normal class procedures. More details about these classes are presented in Table 1 . The authors served as instructors for these classes. Across the six classes, 208 students contributed a total of 1657 full responses to 86 different questions.
The instructors of the courses developed multiple-choice questions related to the ongoing course content. Questions were aimed at testing students’ conceptual understanding, rather than factual knowledge. Consequently, questions often tested whether students could apply ideas to new settings or contexts. An example of a cognitive psychology question used is: Which is a fixed action pattern (not a reflex)?
Knee jerks up when patella is hit
Male bowerbirds building elaborate nests [correct]
Eye blinks when air is blown on it
Can play well learned song on guitar even when in conversation
The procedures for peer instruction across the six different classes followed similar patterns. Students were presented with a multiple-choice question. First, students read the question on their own, chose their answer, and reported their confidence in their answer on a scale of 1 “Not at all confident” to 10 “Highly confident”. Students then paired up with a neighbor in their class and discussed the question with their peer. After discussion, students answered the question and reported the confidence for a second time. The course instructor indicated the correct answer and discussed the reasoning for the answer after all final answers had been submitted. Instruction was paced based upon how quickly students read and answered questions. Most student responses counted towards their participation grade, regardless of the correctness of their answer (the last question in each of the cognitive psychology classes was graded for correctness).
There were small differences in procedures between classes. Students in the cognitive psychology classes input their responses using classroom clickers, but those in other classes wrote their responses on paper. Further, students in the cognitive psychology classes explicitly reported their partner’s answer and confidence, while students in other classes only reported the name of their partner (the partners’ data were aligned during data recording). The cognitive psychology students then were required to mention their own answer and their confidence to their partner during peer instruction; students in other classes were not required to tell their answer or their confidence to their peer. Finally, the questions appeared at any point during the class period for the cognitive psychology classes, while the questions typically happened at the beginning of each class for the other classes.
Analytic strategy
Data are available on the OpenScienceFramework: https://mfr.osf.io/render?url=https://osf.io/5qc46/?action=download%26mode=render .
For most of our analyses we used linear mixed-effects models (Baayen, Davidson, & Bates, 2008 ; Murayama, Sakaki, Yan, & Smith, 2014 ). The unit of analysis in a mixed-effect model is the outcome of a single trial (e.g., whether or not a particular question was answered correctly by a particular participant). We modeled these individual trial-level outcomes as a function of multiple fixed effects - those of theoretical interest - and multiple random effects - effects for which the observed levels are sampled out of a larger population (e.g., questions, students, and classes sampled out of a population of potential questions, students, and classes).
Linear mixed-effects models solve four statistical problems involved with the data of peer instruction. First, there is large variability in students’ performance and the difficulty of questions across students and classes. Mixed-effect models simultaneously account for random variation both across participants and across items (Baayen et al., 2008 ; Murayama et al., 2014 ). Second, students may miss individual classes and therefore may not provide data across every item. Similarly, classes varied in how many peer instruction questions were posed throughout the semester and the number of students enrolled. Mixed-effects models weight each response equally when drawing conclusions (rather than weighting each student or question equally) and can easily accommodate missing data. Third, we were interested in how several different characteristics influenced students’ performance. Mixed effects models can include multiple predictors simultaneously, which allows us to test the effect of one predictor while controlling for others. Finally, mixed effects models can predict the log odds (or logit) of a correct answer, which is needed when examining binary outcomes (i.e., correct or incorrect; Jaeger, 2008 ).
We fit all models in R using the lmer() function of the lme4 package (Bates, Maechler, Bolker, & Walker, 2015 ). For each mixed-effect model, we included random intercepts that capture baseline differences in difficulty of questions, in classes, and in students, in addition to multiple fixed effects of theoretical interest. In mixed-effect models with hundreds of observations, the t distribution effectively converges to the normal, so we compared the t statistic to the normal distribution for analyses involving continuous outcomes (i.e., confidence; Baayen, 2008 ). P values can be directly obtained from Wald z statistics for models with binary outcomes (i.e., correctness).
Does accuracy change through discussion?
First, we examined how correctness changed across peer discussion. A logit model predicting correctness from time point (pre-discussion to post-discussion) revealed that the odds of correctness increased by 1.57 times (95% confidence interval (conf) 1.31–1.87) from pre-discussion to post-discussion, as shown in Table 2 . In fact, 88% of students showed an increase or no change in accuracy from pre-discussion to post-discussion. Pre-discussion to post-discussion performance for each class is shown in Table 3 . We further examined how accuracy changed from pre-discussion to post-discussion for each question and the results are plotted in Fig. 1 . The data show a consistent improvement in accuracy from pre-discussion to post-discussion across all levels of initial difficulty.
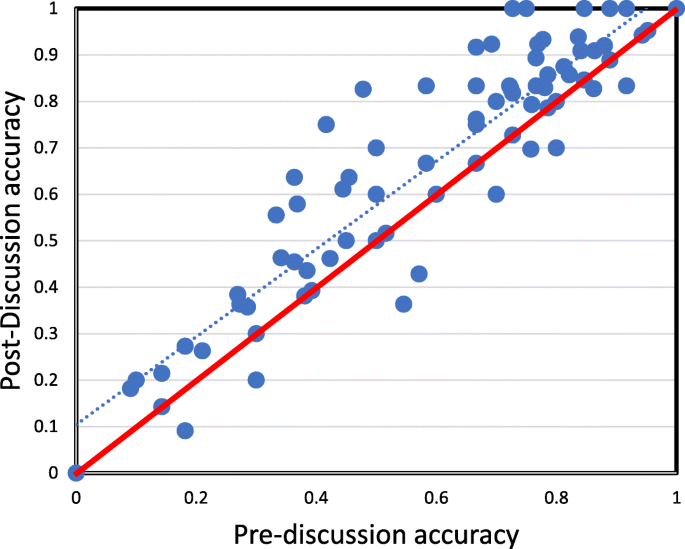
The relationship between pre-discussion accuracy (x axis) and post-discussion accuracy (y axis). Each point represents a single question. The solid diagonal line represents equal pre-discussion and post-discussion accuracy; points above the line indicate improvements in accuracy and points below represent decrements in accuracy. The dashed line indicates the line of best fit for the observed data
We examined how performance increased from pre-discussion to post-discussion by tracing the correctness of answers through the discussion. Figure 2 tracks the percent (and number of items) correct from pre-discussion to post-discussion. The top row shows whether students were initially correct or incorrect in their answer; the middle row shows whether students agreed or disagreed with their partner; the last row show whether students were correct or incorrect after discussion. Additionally, Fig. 2 shows the confidence associated with each pathway. The bottow line of each entry shows the students’ average confidence; in the middle white row, the confidence reported is the average of the peer’s confidence.
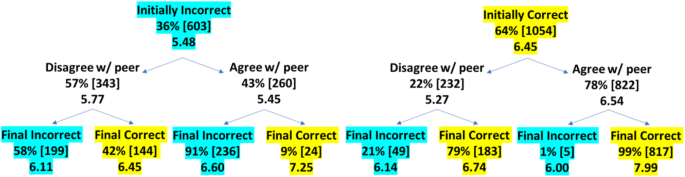
The pathways of answers from pre-discussion (top row) to post-discussion (bottom row). Percentages indicate the portion of items from the category immediately above in that category, the numbers in brackets indicate the raw numbers of items, and the numbers at the bottom of each entry indicate the confidence associated with those items. In the middle, white row, confidence values show the peer’s confidence. Turquoise indicates incorrect answers and yellow indicates correct answers
Broadly, only 5% of correct answers were switched to incorrect, while 28% of incorrect answers were switched to correct following discussion. Even for the items in which students were initially correct but disagreed with their partner, only 21% of answers were changed to incorrect answers after discussion. However, out of the items where students were initially incorrect and disagreed with their partner, 42% were changed to the correct answer.
Does confidence predict switching?
Differences in the amount of switching to correct or incorrect answers could be driven solely by differences in confidence, as described in our first theory mentioned earlier. For this theory to hold, answers with greater confidence must have a greater likelihood of being correct. To examine whether initial confidence is associated with initial correctness, we calculated the gamma correlation between correctness and confidence in the answer before discussion, as shown in the first column of Table 4 . The average gamma correlation between initial confidence and initial correctness (mean (M) = 0.40) was greater than zero, t (160) = 8.59, p < 0.001, d = 0.68, indicating that greater confidence was associated with being correct.
Changing from an incorrect to a correct answer, then, may be driven entirely by selecting the answer from the peer with the greater confidence during discussion, even though most of the students in our sample were not required to explicitly disclose their confidence to their partner during discussion. We examined how frequently students choose the more confident answer when peers disagree. When peers disagreed, students’ final answers aligned with the more confident peer only 58% of the time. Similarly, we tested what the performance would be if peers always picked the answer of the more confident peer. If peers always chose the more confident answer during discussion, the final accuracy would be 69%, which is significantly lower than actual final accuracy (M = 72%, t (207) = 2.59, p = 0.01, d = 0.18). While initial confidence is related to accuracy, these results show that confidence is not the only predictor of switching answers.
Does correctness predict switching beyond confidence?
Discussion may reveal information about the correctness of answers by generating new knowledge and testing the coherence of each possible answer. To test whether the correctness of an answer added predictive power beyond the confidence of the peers involved in discussion, we analyzed situations in which students disagreed with their partner. Out of the instances when partners initially disagreed, we predicted the likelihood of keeping one’s answer based upon one’s own confidence, the partner’s confidence, and whether one’s answer was initially correct. The results of a model predicting whether students keep their answers is shown in Table 5 . For each increase in a point of one’s own confidence, the odds of keeping one’s answer increases 1.25 times (95% conf 1.13–1.38). For each decrease in a point of the partner’s confidence, the odds of keeping one’s answer increased 1.19 times (1.08–1.32). The beta weight for one’s confidence did not differ from the beta weight of the partner’s confidence, χ 2 = 0.49, p = 0.48. Finally, if one’s own answer was correct, the odds of keeping one’s answer increased 4.48 times (2.92–6.89). In other words, the more confident students were, the more likely they were to keep their answer; the more confident their peer was, the more likely they were to change their answer; and finally, if a student was correct, they were more likely to keep their answer.
To illustrate this relationship, we plotted the probability of keeping one’s own answer as a function of the difference between one’s own and their partner’s confidence for initially correct and incorrect answers. As shown in Fig. 3 , at every confidence level, being correct led to equal or more frequently keeping one’s answer than being incorrect.
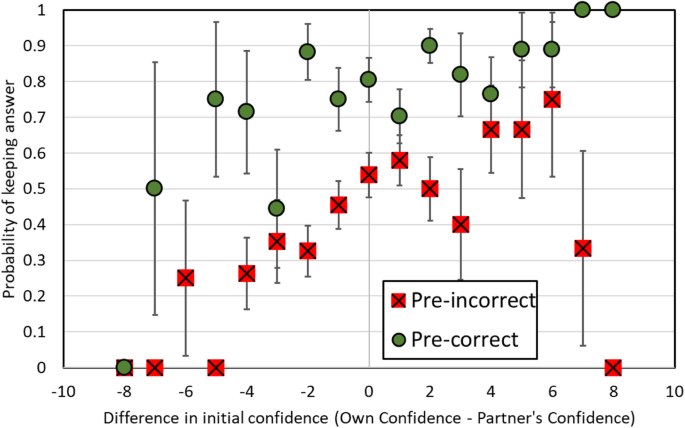
The probability of keeping one’s answer in situations where one’s partner initially disagreed as a function of the difference between partners’ levels of confidence. Error bars indicate the standard error of the proportion and are not shown when the data are based upon a single data point
As another measure of whether discussion allows learners to test the coherence of the correct answer, we analyzed how discussion impacted confidence when partners’ answers agreed. We predicted confidence in answers by the interaction of time point (i.e., pre-discussion versus post-discussion) and being initially correct for situations in which peers initially agreed on their answer. The results, displayed in Table 6 , show that confidence increased from pre-discussion to post-discussion by 1.08 points and that confidence was greater for initially correct answers (than incorrect answers) by 0.78 points. As the interaction between time point and initial correctness shows, confidence increased more from pre-discussion to post-discussion when students were initially correct (as compared to initially incorrect). To illustrate this relationship, we plotted pre-confidence against post-confidence for initially correct and initially incorrect answers when peers agreed (Fig. 4 ). Each plotted point represents a student; the diagonal blue line indicates no change between pre-confidence and post-confidence. The graph reflects that confidence increases more from pre-discussion to post-discussion for correct answers than for incorrect answers, even when we only consider cases where peers agreed.
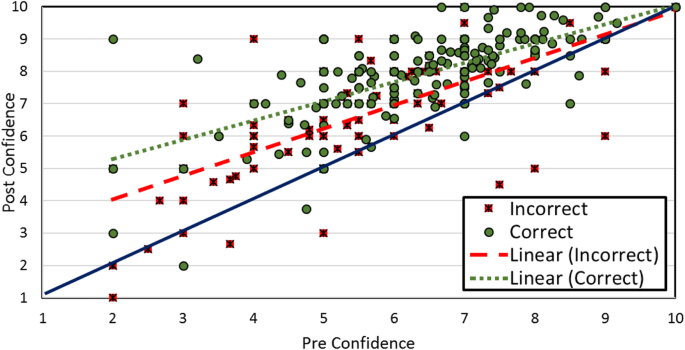
The relationship between pre-discussion and post-discussion confidence as a function of the accuracy of an answer when partners agreed. Each dot represents a student
If students engage in more comprehensive answer testing during discussion than before, the relationship between confidence in their answer and the accuracy of their answer should be stronger following discussion than it is before. We examined whether confidence accurately reflected correctness before and after discussion. To do so, we calculated the gamma correlation between confidence and accuracy, as is typically reported in the literature on metacognitive monitoring (e.g., Son & Metcalfe, 2000 ; Tullis & Fraundorf, 2017 ). Across all students, the resolution of metacognitive monitoring increases from pre-discussion to post-discussion ( t (139) = 2.98, p = 0.003, d = 0.24; for a breakdown of gamma calculations for each class, see Table 4 ). Confidence was more accurately aligned with accuracy following discussion than preceding it. The resolution between student confidence and correctness increases through discussion, suggesting that discussion offers better coherence testing than answering alone.
To examine why peer instruction benefits student learning, we analyzed student answers and confidence before and after discussion across six psychology classes. Discussing a question with a partner improved accuracy across classes and grade levels with small to medium-sized effects. Questions of all difficulty levels benefited from peer discussion; even questions where less than half of students originally answered correctly saw improvements from discussion. Benefits across the spectrum of question difficulty align with prior research showing improvements when even very few students initially know the correct answer (Smith et al., 2009 ). More students switched from incorrect answers to correct answers than vice versa, leading to an improvement in accuracy following discussion. Answer switching was driven by a student’s own confidence in their answer and their partner’s confidence. Greater confidence in one’s answer indicated a greater likelihood of keeping the answer; a partner’s greater confidence increased the likelihood of changing to their answer.
Switching answers depended on more than just confidence: even when accounting for students’ confidence levels, the correctness of the answer impacted switching behavior. Across several measures, our data showed that the correctness of an answer carried weight beyond confidence. For example, the correctness of the answer predicted whether students switched their initial answer during peer disagreements, even after taking the confidence of both partners into account. Further, students’ confidence increased more when partners agreed on the correct answer compared to when they agreed on an incorrect answer. Finally, although confidence increased from pre-discussion to post-discussion when students changed their answers from incorrect to the correct ones, confidence decreased when students changed their answer away from the correct one. A plausible interpretation of this difference is that when students switch from a correct answer to an incorrect one, their decrease in confidence reflects the poor coherence of their final incorrect selection.
Whether peer instruction resulted in optimal switching behaviors is debatable. While accuracy improved through discussion, final accuracy was worse than if students had optimally switched their answers during discussion. If students had chosen the correct answer whenever one of the partners initially chose it, the final accuracy would have been significantly higher (M = 0.80 (SD = 0.19)) than in our data (M = 0.72 (SD = 0.24), t (207) = 6.49, p < 0.001, d = 0.45). While this might be interpreted as “process loss” (Steiner, 1972 ; Weldon & Bellinger, 1997 ), that would assume that there is sufficient information contained within the dyad to ascertain the correct answer. One individual selecting the correct answer is inadequate for this claim because they may not have a compelling justification for their answer. When we account for differences in initial confidence, students’ final accuracy was better than expected. Students’ final accuracy was better than that predicted from a model in which students always choose the answer of the more confident peer. This over-performance, often called “process gain”, can sometimes emerge when individuals collaborate to create or generate new knowledge (Laughlin, Bonner, & Miner, 2002 ; Michaelsen, Watson, & Black, 1989 ; Sniezek & Henry, 1989 ; Tindale & Sheffey, 2002 ). Final accuracy reveals that students did not simply choose the answer of the more confident student during discussion; instead, students more thoroughly probed the coherence of answers and mental models during discussion than they could do alone.
Students’ final accuracy emerges from the interaction between the pairs of students, rather than solely from individuals’ sequestered knowledge prior to discussion (e.g. Wegner, Giuliano, & Hertel, 1985 ). Schwartz ( 1995 ) details four specific cognitive products that can emerge through working in dyads. Specifically, dyads force verbalization of ideas through discussion, and this verbalization facilitates generating new knowledge. Students may not create a coherent explanation of their answer until they engage in discussion with a peer. When students create a verbal explanation of their answer to discuss with a peer, they can identify knowledge gaps and construct new knowledge to fill those gaps. Prior research examining the content of peer interactions during argumentation in upper-level biology classes has shown that these kinds of co-construction happen frequently; over three quarters of statements during discussion involve an exchange of claims and reasoning to support those claims (Knight et al., 2013 ). Second, dyads have more information processing resources than individuals, so they can solve more complex problems. Third, dyads may foster greater motivation than individuals. Finally, dyads may stimulate the creation of new, abstract representations of knowledge, above and beyond what one would expect from the level of abstraction created by individuals. Students need to communicate with their partner; to create common ground and facilitate discourse, dyads negotiate common representations to coordinate different perspectives. The common representations bridge multiple perspectives, so they lose idiosyncratic surface features of individuals’ representation. Working in pairs generates new knowledge and tests of answers that could not be predicted from individuals’ performance alone.
More broadly, teachers often put students in groups so that they can learn from each other by giving and receiving help, recognizing contradictions between their own and others’ perspectives, and constructing new understandings from divergent ideas (Bearison, Magzamen, & Filardo, 1986 ; Bossert, 1988-1989 ; Brown & Palincsar, 1989 ; Webb & Palincsar, 1996 ). Giving explanations to a peer may encourage explainers to clarify or reorganize information, recognize and rectify gaps in understandings, and build more elaborate interpretations of knowledge than they would have alone (Bargh & Schul, 1980 ; Benware & Deci, 1984 ; King, 1992 ; Yackel, Cobb, & Wood, 1991 ). Prompting students to explain why and how problems are solved facilitates conceptual learning more than reading the problem solutions twice without self-explanations (Chi, de Leeuw, Chiu, & LaVancher, 1994 ; Rittle-Johnson, 2006 ; Wong, Lawson, & Keeves, 2002 ). Self-explanations can prompt students to retrieve, integrate, and modify their knowledge with new knowledge; self-explanations can also help students identify gaps in their knowledge (Bielaczyc, Pirolli, & Brown, 1995 ; Chi & Bassock, 1989 ; Chi, Bassock, Lewis, Reimann, & Glaser, 1989 ; Renkl, Stark, Gruber, & Mandl, 1998 ; VanLehn, Jones, & Chi, 1992 ; Wong et al., 2002 ), detect and correct errors, and facilitate deeper understanding of conceptual knowledge (Aleven & Koedinger, 2002 ; Atkinson, Renkl, & Merrill, 2003 ; Chi & VanLehn, 2010 ; Graesser, McNamara, & VanLehn, 2005 ). Peer instruction, while leveraging these benefits of self-explanation, also goes beyond them by involving what might be called “other-explanation” processes - processes recruited not just when explaining a situation to oneself but to others. Mercier and Sperber ( 2019 ) argue that much of human reason is the result of generating explanations that will be convincing to other members of one’s community, thereby compelling others to act in the way that one wants.
Conversely, students receiving explanations can fill in gaps in their own understanding, correct misconceptions, and construct new, lasting knowledge. Fellow students may be particularly effective explainers because they can better take the perspective of their peer than the teacher (Priniski & Horne, 2019 ; Ryskin, Benjamin, Tullis, & Brown-Schmidt, 2015 ; Tullis, 2018 ). Peers may be better able than expert teachers to explain concepts in familiar terms and direct peers’ attention to the relevant features of questions that they do not understand (Brown & Palincsar, 1989 ; Noddings, 1985 ; Vedder, 1985 ; Vygotsky, 1981 ).
Peer instruction may benefit from the generation of explanations, but social influences may compound those benefits. Social interactions may help students monitor and regulate their cognition better than self-explanations alone (e.g., Jarvela et al., 2015 ; Kirschner, Kreijns, Phielix, & Fransen, 2015 ; Kreijns, Kirschner, & Vermeulen, 2013 ; Phielix, Prins, & Kirschner, 2010 ; Phielix, Prins, Kirschner, Erkens, & Jaspers, 2011 ). Peers may be able to judge the quality of the explanation better than the explainer. In fact, recent research suggests that peer instruction facilitates learning even more than self-explanations (Versteeg, van Blankenstein, Putter, & Steendijk, 2019 ).
Not only does peer instruction generate new knowledge, but it may also improve students’ metacognition. Our data show that peer discussion prompted more thorough testing of the coherence of the answers. Specifically, students’ confidences were better aligned with accuracy following discussion than before. Improvements in metacognitive resolution indicate that discussion provides more thorough testing of answers and ideas than does answering questions on one’s own. Discussion facilitates the metacognitive processes of detecting errors and assessing the coherence of an answer.
Agreement among peers has important consequences for final behavior. For example, when peers agreed, students very rarely changed their answer (less than 3% of the time). Further, large increases in confidence occurred when students agreed (as compared to when they disagreed). Alternatively, disagreements likely engaged different discussion processes and prompted students to combine different answers. Whether students weighed their initial answer more than their partner’s initial answer remains debatable. When students disagreed with their partner, they were more likely to stick with their own answer than switch; they kept their own answer 66% of the time. Even when their partner was more confident, students only switched to their partner’s answer 50% of the time. The low rate of switching during disagreements suggests that students weighed their own answer more heavily than their partner’s answer. In fact, across prior research, deciders typically weigh their own thoughts more than the thoughts of an advisor (Harvey, Harries, & Fischer, 2000 ; Yaniv & Kleinberger, 2000 ).
Interestingly, peers agreed more frequently than expected by chance. When students were initially correct (64% of the time), 78% of peers agreed. When students were initially incorrect (36% of the time), peers agreed 43% of the time. Pairs of students, then, agree more than expected by a random distribution of answers throughout the classroom. These data suggest that students group themselves into pairs based upon likelihood of sharing the same answer. Further, these data suggest that student understanding is not randomly distributed throughout the physical space of the classroom. Across all classes, students were instructed to work with a neighbor to discuss their answer. Given that neighbors agreed more than predicted by chance, students seem to tend to sit near and pair with peers that share their same levels of understanding. Our results from peer instruction reveal that students physically locate themselves near students of similar abilities. Peer instruction could potentially benefit from randomly pairing students together (i.e. not with a physically close neighbor) to generate the most disagreements and generative activity during discussion.
Learning through peer instruction may involve deep processing as peers actively challenge each other, and this deep processing may effectively support long-term retention. Future research can examine the persistence of gains in accuracy from peer instruction. For example, whether errors that are corrected during peer instruction stay corrected on later retests of the material remains an open question. High and low-confidence errors that are corrected during peer instruction may result in different long-term retention of the correct answer; more specifically, the hypercorrection effect suggests that errors committed with high confidence are more likely to be corrected on subsequent tests than errors with low confidence (e.g., Butler, Fazio, & Marsh, 2011 ; Butterfield & Metcalfe, 2001 ; Metcalfe, 2017 ). Whether hypercorrection holds for corrections from classmates during peer instruction (rather than from an absolute authority) could be examined in the future.
The influence of partner interaction on accuracy may depend upon the domain and kind of question posed to learners. For simple factual or perceptual questions, partner interaction may not consistently benefit learning. More specifically, partner interaction may amplify and bolster wrong answers when factual or perceptual questions lead most students to answer incorrectly (Koriat, 2015 ). However, for more “intellective tasks,” interactions and arguments between partners can produce gains in knowledge (Trouche et al., 2014 ). For example, groups typically outperform individuals for reasoning tasks (Laughlin, 2011 ; Moshman & Geil, 1998 ), math problems (Laughlin & Ellis, 1986 ), and logic problems (Doise & Mugny, 1984; Perret-Clermont, 1980 ). Peer instruction questions that allow for student argumentation and reasoning, therefore, may have the best benefits in student learning.
The underlying benefits of peer instruction extend beyond the improvements in accuracy seen from pre-discussion to post-discussion. Peer instruction prompts students to retrieve information from long-term memory, and these practice tests improve long-term retention of information (Roediger III & Karpicke, 2006 ; Tullis, Fiechter, & Benjamin, 2018 ). Further, feedback provided by instructors following peer instruction may guide students to improve their performance and correct misconceptions, which should benefit student learning (Bangert-Drowns, Kulik, & Kulik, 1991 ; Thurlings, Vermeulen, Bastiaens, & Stijnen, 2013 ). Learners who engage in peer discussion can use their new knowledge to solve new, but similar problems on their own (Smith et al., 2009 ). Generating new knowledge and revealing gaps in knowledge through peer instruction, then, effectively supports students’ ability to solve novel problems. Peer instruction can be an effective tool to generate new knowledge through discussion between peers and improve student understanding and metacognition.
Availability of data and materials
As described below, data and materials are available on the OpenScienceFramework: https://mfr.osf.io/render?url=https://osf.io/5qc46/?action=download%26mode=render .
Aleven, V., & Koedinger, K. R. (2002). An effective metacognitive strategy: Learning by doing and explaining with a computer based cognitive tutor. Cognitive Science , 26 , 147–179.
Article Google Scholar
Atkinson, R. K., Renkl, A., & Merrill, M. M. (2003). Transitioning from studying examples to solving problems: Effects of self-explanation prompts and fading worked-out steps. Journal of Educational Psychology , 95 , 774–783.
Baayen, R. H. (2008). Analyzing linguistic data: A practical introduction to statistics . Cambridge: Cambridge University Press.
Baayen, R. H., Davidson, D. J., & Bates, D. M. (2008). Mixed-effects modeling with crossed random effects for subjects and items. Journal of Memory and Language , 59 , 390–412.
Bangert-Drowns, R. L., Kulik, J. A., & Kulik, C.-L. C. (1991). Effects of frequent classroom testing. Journal of Educational Research , 85 , 89–99.
Bargh, J. A., & Schul, Y. (1980). On the cognitive benefit of teaching. Journal of Educational Psychology , 72 , 593–604.
Bates, D., Maechler, M., Bolker, B., & Walker, S. (2015). Fitting linear mixed-effects models using lme4. Journal of Statistical Software , 67 , 1–48.
Bearison, D. J., Magzamen, S., & Filardo, E. K. (1986). Sociocognitive conflict and cognitive growth in young children. Merrill-Palmer Quarterly , 32 (1), 51–72.
Google Scholar
Beatty, I. D., Gerace, W. J., Leonard, W. J., & Dufresne, R. J. (2006). Designing effective questions for classroom response system teaching. American Journal of Physics , 74 (1), 31e39.
Beekes, W. (2006). The “millionaire” method for encouraging participation. Active Learning in Higher Education , 7 , 25–36.
Benware, C. A., & Deci, E. L. (1984). Quality of learning with an active versus passive motivational set. American Educational Research Journal , 21 , 755–765.
Bielaczyc, K., Pirolli, P., & Brown, A. L. (1995). Training in self-explanation and self regulation strategies: Investigating the effects of knowledge acquisition activities on problem solving. Cognition and Instruction , 13 , 221–251.
Bossert, S. T. (1988-1989). Cooperative activities in the classroom. Review of Research in Education , 15 , 225–252.
Brooks, B. J., & Koretsky, M. D. (2011). The influence of group discussion on students’ responses and confidence during peer instruction. Journal of Chemistry Education , 88 , 1477–1484.
Brown, A. L., & Palincsar, A. S. (1989). Guided, cooperative learning and individual knowledge acquisition. In L. B. Resnick (Ed.), Knowing, learning, and instruction: essays in honor of Robert Glaser , (pp. 393–451). Hillsdale: Erlbaum.
Butchart, S., Handfield, T., & Restall, G. (2009). Using peer instruction to teach philosophy, logic and critical thinking. Teaching Philosophy , 32 , 1–40.
Butler, A. C., Fazio, L. K., & Marsh, E. J. (2011). The hypercorrection effect persists over a week, but high-confidence errors return. Psychonomic Bulletin & Review , 18 (6), 1238–1244.
Butterfield, B., & Metcalfe, J. (2001). Errors committed with high confidence are hypercorrected. Journal of Experimental Psychology: Learning, Memory, and Cognition , 27 (6), 1491.
PubMed Google Scholar
Caldwell, J. E. (2007). Clickers in the large classroom: current research and best-practice tips. CBE-Life Sciences Education , 6 (1), 9–20.
Article PubMed PubMed Central Google Scholar
Chi, M., & VanLehn, K. A. (2010). Meta-cognitive strategy instruction in intelligent tutoring systems: How, when and why. Journal of Educational Technology and Society , 13 , 25–39.
Chi, M. T. H., & Bassock, M. (1989). Learning from examples via self-explanations. In L. B. Resnick (Ed.), Knowing, learning, and instruction: Essays in honor of Robert Glaser , (pp. 251–282). Hillsdale: Erlbaum.
Chi, M. T. H., Bassock, M., Lewis, M., Reimann, P., & Glaser, R. (1989). Self-explanations: How students study and use examples in learning to solve problems. Cognitive Science , 13 , 145–182.
Chi, M. T. H., de Leeuw, N., Chiu, M. H., & LaVancher, C. (1994). Eliciting self-explanations improves understanding. Cognitive Science , 18 , 439–477.
Cortright, R. N., Collins, H. L., & DiCarlo, S. E. (2005). Peer instruction enhanced meaningful learning: Ability to solve novel problems. Advances in Physiology Education , 29 , 107–111.
Article PubMed Google Scholar
Crouch, C. H., & Mazur, E. (2001). Peer instruction: Ten years of experience and results. American Journal of Physics , 69 , 970–977.
Cummings, K., & Roberts, S. (2008). A study of peer instruction methods with school physics students. In C. Henderson, M. Sabella, & L. Hsu (Eds.), Physics education research conference , (pp. 103–106). College Park: American Institute of Physics.
Deslauriers, L., Schelew, E., & Wieman, C. (2011). Improved learning in a large-enrollment physics class. Science , 332 , 862–864.
Duncan, D. (2005). Clickers in the classroom: How to enhance science teaching using classroom response systems . San Francisco: Pearson/Addison-Wesley.
Finley, J. R., Tullis, J. G., & Benjamin, A. S. (2010). Metacognitive control of learning and remembering. In M. S. Khine, & I. M. Saleh (Eds.), New science of learning: Cognition, computers and collaborators in education . New York: Springer Science & Business Media, LLC.
Giuliodori, M. J., Lujan, H. L., & DiCarlo, S. E. (2006). Peer instruction enhanced student performance on qualitative problem solving questions. Advances in Physiology Education , 30 , 168–173.
Graesser, A. C., McNamara, D., & VanLehn, K. (2005). Scaffolding deep comprehension strategies through AutoTutor and iSTART. Educational Psychologist , 40 , 225–234.
Granovskiy, B., Gold, J. M., Sumpter, D., & Goldstone, R. L. (2015). Integration of social information by human groups. Topics in Cognitive Science , 7 , 469–493.
Harvey, N., & Fischer, I. (1997). Taking advice: Accepting help, improving judgment, and sharing responsibility. Organizational Behavior and Human Decision Processes , 70 , 117–133.
Harvey, N., Harries, C., & Fischer, I. (2000). Using advice and assessing its quality. Organizational Behavior and Human Decision Processes , 81 , 252–273.
Henderson, C., & Dancy, M. H. (2009). The impact of physics education research on the teaching of introductory quantitative physics in the United States. Physical Review Special Topics: Physics Education Research , 5 (2), 020107.
Jaeger, T. F. (2008). Categorical data analysis: away from ANOVAs (transformation or not) and towards logit mixed models. Journal of Memory and Language , 59 , 434–446.
James, M. C. (2006). The effect of grading incentive on student discourse in peer instruction. American Journal of Physics , 74 (8), 689–691.
Jarvela, S., Kirschner, P., Panadero, E., Malmberg, J., Phielix, C., Jaspers, J., … Jarvenoja, H. (2015). Enhancing socially shared regulation in collaborative learning groups: Designing for CSCL regulation tools. Educational Technology Research and Development , 63 (1), 125e142.
Jones, M. E., Antonenko, P. D., & Greenwood, C. M. (2012). The impact of collaborative and individualized student response system strategies on learner motivation, metacognition, and knowledge transfer. Journal of Computer Assisted Learning , 28 (5), 477–487.
King, A. (1992). Facilitating elaborative learning through guided student-generated questioning. Educational Psychologist , 27 , 111–126.
Kirschner, P. A., Kreijns, K., Phielix, C., & Fransen, J. (2015). Awareness of cognitive and social behavior in a CSCL environment. Journal of Computer Assisted Learning , 31 (1), 59–77.
Knight, J. K., Wise, S. B., & Southard, K. M. (2013). Understanding clicker discussions: student reasoning and the impact of instructional cues. CBE-Life Sciences Education , 12 , 645–654.
Koriat, A. (2015). When two heads are better than one and when they can be worse: The amplification hypothesis. Journal of Experimental Psychology: General , 144 , 934–950. https://doi.org/10.1037/xge0000092 .
Kreijns, K., Kirschner, P. A., & Vermeulen, M. (2013). Social aspects of CSCL environments: A research framework. Educational Psychologist , 48 (4), 229e242.
Kuhn, L. M., & Sniezek, J. A. (1996). Confidence and uncertainty in judgmental forecasting: Differential effects of scenario presentation. Journal of Behavioral Decision Making , 9 , 231–247.
Lasry, N., Mazur, E., & Watkins, J. (2008). Peer instruction: From Harvard to the two-year college. American Journal of Physics , 76 (11), 1066–1069.
Laughlin, P. R. (2011). Group problem solving. Princeton: Princeton University Press.
Book Google Scholar
Laughlin, P. R., Bonner, B. L., & Miner, A. G. (2002). Groups perform better than individuals on letters-to-numbers problems. Organisational Behaviour and Human Decision Processes , 88 , 605–620.
Laughlin, P. R., & Ellis, A. L. (1986). Demonstrability and social combination processes on mathematical intellective tasks. Journal of Experimental Social Psychology, 22, 177–189.
Lucas, A. (2009). Using peer instruction and i-clickers to enhance student participation in calculus. Primus , 19 (3), 219–231.
Mazur, E. (1997). Peer instruction: A user’s manual . Upper Saddle River: Prentice Hall.
Mercier, H., & Sperber, D. (2019). The enigma of reason . Cambridge: Harvard University Press.
Metcalfe, J. (2017). Learning from errors. Annual Review of Psychology , 68 , 465–489.
Michaelsen, L. K., Watson, W. E., & Black, R. H. (1989). Realistic test of individual versus group decision making. Journal of Applied Psychology , 64 , 834–839.
Miller, R. L., Santana-Vega, E., & Terrell, M. S. (2007). Can good questions and peer discussion improve calculus instruction? Primus , 16 (3), 193–203.
Morgan, J. T., & Wakefield, C. (2012). Who benefits from peer conversation? Examining correlations of clicker question correctness and course performance. Journal of College Science Teaching , 41 (5), 51–56.
Moshman, D., & Geil, M. (1998). Collaborative reasoning: Evidence for collective rationality. Thinking and Reasoning, 4, 231–248.
Murayama, K., Sakaki, M., Yan, V. X., & Smith, G. M. (2014). Type I error inflation in the traditional by-participant analysis to metamemory accuracy: A generalized mixed-effects model perspective. Journal of Experimental Psychology: Learning, Memory, and Cognition , 40 , 1287–1306.
Newbury, P., & Heiner, C. (2012). Ready, set, react! getting the most out of peer instruction using clickers. Retrieved October 28, 2015, from http://www.cwsei.ubc.ca/Files/ReadySetReact_3fold.pdf .
Nielsen, K. L., Hansen-Nygård, G., & Stav, J. B. (2012). Investigating peer instruction: how the initial voting session affects students’ experiences of group discussion. ISRN Education , 2012 , article 290157.
Noddings, N. (1985). Small groups as a setting for research on mathematical problem solving. In E. A. Silver (Ed.), Teaching and learning mathematical problem solving , (pp. 345–360). Hillsdale: Erlbaum.
Perret-Clermont, A. N. (1980). Social Interaction and Cognitive Development in Children. London: Academic Press.
Perez, K. E., Strauss, E. A., Downey, N., Galbraith, A., Jeanne, R., Cooper, S., & Madison, W. (2010). Does displaying the class results affect student discussion during peer instruction? CBE Life Sciences Education , 9 , 133–140.
Phielix, C., Prins, F. J., & Kirschner, P. A. (2010). Awareness of group performance in a CSCL-environment: Effects of peer feedback and reflection. Computers in Human Behavior , 26 (2), 151–161.
Phielix, C., Prins, F. J., Kirschner, P. A., Erkens, G., & Jaspers, J. (2011). Group awareness of social and cognitive performance in a CSCL environment: Effects of a peer feedback and reflection tool. Computers in Human Behavior , 27 (3), 1087–1102.
Pollock, S. J., Chasteen, S. V., Dubson, M., & Perkins, K. K. (2010). The use of concept tests and peer instruction in upper-division physics. In M. Sabella, C. Singh, & S. Rebello (Eds.), AIP conference proceedings , (vol. 1289, p. 261). New York: AIP Press.
Porter, L., Bailey-Lee, C., & Simon, B. (2013). Halving fail rates using peer instruction: A study of four computer science courses. In SIGCSE ‘13: Proceedings of the 44th ACM technical symposium on computer science education , (pp. 177–182). New York: ACM Press.
Price, P. C., & Stone, E. R. (2004). Intuitive evaluation of likelihood judgment producers. Journal of Behavioral Decision Making , 17 , 39–57.
Priniski, J. H., & Horne, Z. (2019). Crowdsourcing effective educational interventions. In A. K. Goel, C. Seifert, & C. Freska (Eds.), Proceedings of the 41st annual conference of the cognitive science society . Austin: Cognitive Science Society.
Rao, S. P., & DiCarlo, S. E. (2000). Peer instruction improves performance on quizzes. Advances in Physiological Education , 24 , 51–55.
Renkl, A., Stark, R., Gruber, H., & Mandl, H. (1998). Learning from worked-out examples: The effects of example variability and elicited self-explanations. Contemporary Educational Psychology , 23 , 90–108.
Rittle-Johnson, B. (2006). Promoting transfer: Effects of self-explanation and direct instruction. Child Development , 77 , 1–15.
Roediger III, H. L., & Karpicke, J. D. (2006). Test-enhanced learning: Taking memory tests improves long-term retention. Psychological Science , 17 , 249–255.
Ryskin, R., Benjamin, A. S., Tullis, J. G., & Brown-Schmidt, S. (2015). Perspective-taking in comprehension, production, and memory: An individual differences approach. Journal of Experimental Psychology: General , 144 , 898–915.
Sah, S., Moore, D. A., & MacCoun, R. J. (2013). Cheap talk and credibility: The consequences of confidence and accuracy on advisor credibility and persuasiveness. Organizational Behavior and Human Decision Processes , 121 , 246–255.
Schwartz, D. L. (1995). The emergence of abstract representations in dyad problem solving. The Journal of the Learning Sciences , 4 , 321–354.
Simon, B., Kohanfars, M., Lee, J., Tamayo, K., & Cutts, Q. (2010). Experience report: peer instruction in introductory computing. In Proceedings of the 41st SIGCSE technical symposium on computer science education .
Smith, M. K., Wood, W. B., Adams, W. K., Wieman, C., Knight, J. K., Guild, N., & Su, T. T. (2009). Why peer discussion improves student performance on in-class concept questions. Science , 323 , 122–124.
Smith, M. K., Wood, W. B., Krauter, K., & Knight, J. K. (2011). Combining peer discussion with instructor explanation increases student learning from in-class concept questions. CBE-Life Sciences Education , 10 , 55–63.
Sniezek, J. A., & Buckley, T. (1995). Cueing and cognitive conflict in judge–Advisor decision making. Organizational Behavior and Human Decision Processes , 62 , 159–174.
Sniezek, J. A., & Henry, R. A. (1989). Accuracy and confidence in group judgment. Organizational Behavior and Human Decision Processes , 43 , 1–28.
Son, L. K., & Metcalfe, J. (2000). Metacognitive and control strategies in study-time allocation. Journal of Experimental Psychology: Learning, Memory, and Cognition , 26 , 204–221.
Steiner, I. D. (1972). Group processes and productivity . New York: Academic Press.
Thurlings, M., Vermeulen, M., Bastiaens, T., & Stijnen, S. (2013). Understanding feedback: A learning theory perspective. Educational Research Review , 9 , 1–15.
Tindale, R. S., & Sheffey, S. (2002). Shared information, cognitive load, and group memory. Group Processes & Intergroup Relations , 5 (1), 5–18.
Trouche, E., Sander, E., & Mercier, H. (2014). Arguments, more than confidence, explain the good performance of reasoning groups. Journal of Experimental Psychology: General , 143 , 1958–1971.
Tullis, J. G. (2018). Predicting others’ knowledge: Knowledge estimation as cue-utilization. Memory & Cognition , 46 , 1360–1375.
Tullis, J. G., Fiechter, J. L., & Benjamin, A. S. (2018). The efficacy of learners’ testing choices. Journal of Experimental Psychology: Learning, Memory, and Cognition , 44 , 540–552.
Tullis, J. G., & Fraundorf, S. H. (2017). Predicting others’ memory performance: The accuracy and bases of social metacognition. Journal of Memory and Language , 95 , 124–137.
Turpen, C., & Finkelstein, N. (2007). Understanding how physics faculty use peer instruction. In L. Hsu, C. Henderson, & L. McCullough (Eds.), Physics education research conference , (pp. 204–209). College Park: American Institute of Physics.
Van Swol, L. M., & Sniezek, J. A. (2005). Factors affecting the acceptance of expert advice. British Journal of Social Psychology , 44 , 443–461.
VanLehn, K., Jones, R. M., & Chi, M. T. H. (1992). A model of the self-explanation effect. Journal of the Learning Sciences , 2 (1), 1–59.
Vedder, P. (1985). Cooperative learning: A study on processes and effects of cooperation between primary school children . Westerhaven: Rijkuniversiteit Groningen.
Versteeg, M., van Blankenstein, F. M., Putter, H., & Steendijk, P. (2019). Peer instruction improves comprehension and transfer of physiological concepts: A randomized comparison with self-explanation. Advances in Health Sciences Education , 24 , 151–165.
Vygotsky, L. S. (1981). The genesis of higher mental functioning. In J. V. Wertsch (Ed.), The concept of activity in Soviet psychology , (pp. 144–188). Armonk: Sharpe.
Webb, N. M., & Palincsar, A. S. (1996). Group processes in the classroom. In D. C. Berliner, & R. C. Calfee (Eds.), Handbook of educational psychology , (pp. 841–873). New York: Macmillan Library Reference USA: London: Prentice Hall International.
Wegner, D. M., Giuliano, T., & Hertel, P. (1985). Cognitive interdependence in close relationships. In W. J. Ickes (Ed.), Compatible and incompatible relationships , (pp. 253–276). New York: Springer-Verlag.
Chapter Google Scholar
Weldon, M. S., & Bellinger, K. D. (1997). Collective memory: Collaborative and individual processes in remembering. Journal of Experimental Psychology: Learning, Memory, and Cognition , 23 , 1160–1175.
Wieman, C., Perkins, K., Gilbert, S., Benay, F., Kennedy, S., Semsar, K., et al. (2009). Clicker resource guide: An instructor’s guide to the effective use of personalresponse systems (clickers) in teaching . Vancouver: University of British Columbia Available from http://www.cwsei.ubc.ca/resources/files/Clicker_guide_CWSEI_CU-SEI.pdf .
Wong, R. M. F., Lawson, M. J., & Keeves, J. (2002). The effects of self-explanation training on students’ problem solving in high school mathematics. Learning and Instruction , 12 , 23.
Yackel, E., Cobb, P., & Wood, T. (1991). Small-group interactions as a source of learning opportunities in second-grade mathematics. Journal for Research in Mathematics Education , 22 , 390–408.
Yaniv, I. (2004a). The benefit of additional opinions. Current Directions in Psychological Science , 13 , 75–78.
Yaniv, I. (2004b). Receiving other people’s advice: Influence and benefit. Organizational Behavior and Human Decision Processes , 93 , 1–13.
Yaniv, I., & Choshen-Hillel, S. (2012). Exploiting the wisdom of others to make better decisions: Suspending judgment reduces egocentrism and increases accuracy. Journal of Behavioral Decision Making , 25 , 427–434.
Yaniv, I., & Kleinberger, E. (2000). Advice taking in decision making: Egocentric discounting and reputation formation. Organizational Behavior and Human Decision Processes , 83 , 260–281.
Download references
Acknowledgements
Not applicable.
No funding supported this manuscript.
Author information
Authors and affiliations.
Department of Educational Psychology, University of Arizona, 1430 E. Second St., Tucson, AZ, 85721, USA
Jonathan G. Tullis
Department of Psychology, Indiana University, Bloomington, IN, USA
Robert L. Goldstone
You can also search for this author in PubMed Google Scholar
Contributions
JGT collected some data, analyzed the data, and wrote the first draft of the paper. RLG collected some data, contributed significantly to the framing of the paper, and edited the paper. The authors read and approved the final manuscript.
Authors’ information
JGT: Assistant Professor in Educational Psychology at University of Arizona. RLG: Chancellor’s Professor in Psychology at Indiana University.
Corresponding author
Correspondence to Jonathan G. Tullis .
Ethics declarations
Ethics approval and consent to participate.
The ethics approval was waived by the Indiana University Institutional Review Board (IRB) and the University of Arizona IRB, given that these data are collected as part of normal educational settings and processes.
Consent for publication
No individual data are presented in the manuscript.
Competing interests
The authors declare that they have no competing interests.
Additional information
Publisher’s note.
Springer Nature remains neutral with regard to jurisdictional claims in published maps and institutional affiliations.
Rights and permissions
Open Access This article is licensed under a Creative Commons Attribution 4.0 International License, which permits use, sharing, adaptation, distribution and reproduction in any medium or format, as long as you give appropriate credit to the original author(s) and the source, provide a link to the Creative Commons licence, and indicate if changes were made. The images or other third party material in this article are included in the article's Creative Commons licence, unless indicated otherwise in a credit line to the material. If material is not included in the article's Creative Commons licence and your intended use is not permitted by statutory regulation or exceeds the permitted use, you will need to obtain permission directly from the copyright holder. To view a copy of this licence, visit http://creativecommons.org/licenses/by/4.0/ .
Reprints and permissions
About this article
Cite this article.
Tullis, J.G., Goldstone, R.L. Why does peer instruction benefit student learning?. Cogn. Research 5 , 15 (2020). https://doi.org/10.1186/s41235-020-00218-5
Download citation
Received : 08 October 2019
Accepted : 25 February 2020
Published : 09 April 2020
DOI : https://doi.org/10.1186/s41235-020-00218-5
Share this article
Anyone you share the following link with will be able to read this content:
Sorry, a shareable link is not currently available for this article.
Provided by the Springer Nature SharedIt content-sharing initiative
- Group decisions
- Peer instruction
- Metacognition
- Decision making
You are using an outdated browser. Please upgrade your browser to improve your experience.

Health & Nursing
Courses and certificates.
- Bachelor's Degrees
- View all Business Bachelor's Degrees
- Business Management – B.S. Business Administration
- Healthcare Administration – B.S.
- Human Resource Management – B.S. Business Administration
- Information Technology Management – B.S. Business Administration
- Marketing – B.S. Business Administration
- Accounting – B.S. Business Administration
- Finance – B.S.
- Supply Chain and Operations Management – B.S.
- Accelerated Information Technology Bachelor's and Master's Degree (from the School of Technology)
- Health Information Management – B.S. (from the Leavitt School of Health)
Master's Degrees
- View all Business Master's Degrees
- Master of Business Administration (MBA)
- MBA Information Technology Management
- MBA Healthcare Management
- Management and Leadership – M.S.
- Accounting – M.S.
- Marketing – M.S.
- Human Resource Management – M.S.
- Master of Healthcare Administration (from the Leavitt School of Health)
- Data Analytics – M.S. (from the School of Technology)
- Information Technology Management – M.S. (from the School of Technology)
- Education Technology and Instructional Design – M.Ed. (from the School of Education)
Certificates
- Supply Chain
- Accounting Fundamentals
- View all Business Degrees
Bachelor's Preparing For Licensure
- View all Education Bachelor's Degrees
- Elementary Education – B.A.
- Special Education and Elementary Education (Dual Licensure) – B.A.
- Special Education (Mild-to-Moderate) – B.A.
- Mathematics Education (Middle Grades) – B.S.
- Mathematics Education (Secondary)– B.S.
- Science Education (Middle Grades) – B.S.
- Science Education (Secondary Chemistry) – B.S.
- Science Education (Secondary Physics) – B.S.
- Science Education (Secondary Biological Sciences) – B.S.
- Science Education (Secondary Earth Science)– B.S.
- View all Education Degrees
Bachelor of Arts in Education Degrees
- Educational Studies – B.A.
Master of Science in Education Degrees
- View all Education Master's Degrees
- Curriculum and Instruction – M.S.
- Educational Leadership – M.S.
- Education Technology and Instructional Design – M.Ed.
Master's Preparing for Licensure
- Teaching, Elementary Education – M.A.
- Teaching, English Education (Secondary) – M.A.
- Teaching, Mathematics Education (Middle Grades) – M.A.
- Teaching, Mathematics Education (Secondary) – M.A.
- Teaching, Science Education (Secondary) – M.A.
- Teaching, Special Education (K-12) – M.A.
Licensure Information
- State Teaching Licensure Information
Master's Degrees for Teachers
- Mathematics Education (K-6) – M.A.
- Mathematics Education (Middle Grade) – M.A.
- Mathematics Education (Secondary) – M.A.
- English Language Learning (PreK-12) – M.A.
- Endorsement Preparation Program, English Language Learning (PreK-12)
- Science Education (Middle Grades) – M.A.
- Science Education (Secondary Chemistry) – M.A.
- Science Education (Secondary Physics) – M.A.
- Science Education (Secondary Biological Sciences) – M.A.
- Science Education (Secondary Earth Science)– M.A.
- View all Technology Bachelor's Degrees
- Cloud Computing – B.S.
- Computer Science – B.S.
- Cybersecurity and Information Assurance – B.S.
- Data Analytics – B.S.
- Information Technology – B.S.
- Network Engineering and Security – B.S.
- Software Engineering – B.S.
- Accelerated Information Technology Bachelor's and Master's Degree
- Information Technology Management – B.S. Business Administration (from the School of Business)
- View all Technology Master's Degrees
- Cybersecurity and Information Assurance – M.S.
- Data Analytics – M.S.
- Information Technology Management – M.S.
- MBA Information Technology Management (from the School of Business)
- Full Stack Engineering
- Web Application Deployment and Support
- Front End Web Development
- Back End Web Development
3rd Party Certifications
- IT Certifications Included in WGU Degrees
- View all Technology Degrees
- View all Health & Nursing Bachelor's Degrees
- Nursing (RN-to-BSN online) – B.S.
- Nursing (Prelicensure) – B.S. (Available in select states)
- Health Information Management – B.S.
- Health and Human Services – B.S.
- Psychology – B.S.
- Health Science – B.S.
- Healthcare Administration – B.S. (from the School of Business)
- View all Nursing Post-Master's Certificates
- Nursing Education—Post-Master's Certificate
- Nursing Leadership and Management—Post-Master's Certificate
- Family Nurse Practitioner—Post-Master's Certificate
- Psychiatric Mental Health Nurse Practitioner —Post-Master's Certificate
- View all Health & Nursing Degrees
- View all Nursing & Health Master's Degrees
- Nursing – Education (BSN-to-MSN Program) – M.S.
- Nursing – Leadership and Management (BSN-to-MSN Program) – M.S.
- Nursing – Nursing Informatics (BSN-to-MSN Program) – M.S.
- Nursing – Family Nurse Practitioner (BSN-to-MSN Program) – M.S. (Available in select states)
- Nursing – Psychiatric Mental Health Nurse Practitioner (BSN-to-MSN Program) – M.S. (Available in select states)
- Nursing – Education (RN-to-MSN Program) – M.S.
- Nursing – Leadership and Management (RN-to-MSN Program) – M.S.
- Nursing – Nursing Informatics (RN-to-MSN Program) – M.S.
- Master of Healthcare Administration
- MBA Healthcare Management (from the School of Business)
- Business Leadership (with the School of Business)
- Supply Chain (with the School of Business)
- Accounting Fundamentals (with the School of Business)
- Back End Web Development (with the School of Technology)
- Front End Web Development (with the School of Technology)
- Web Application Deployment and Support (with the School of Technology)
- Full Stack Engineering (with the School of Technology)
- Single Courses
- Course Bundles
Apply for Admission
Admission requirements.
- New Students
- WGU Returning Graduates
- WGU Readmission
- Enrollment Checklist
- Accessibility
- Accommodation Request
- School of Education Admission Requirements
- School of Business Admission Requirements
- School of Technology Admission Requirements
- Leavitt School of Health Admission Requirements
Additional Requirements
- Computer Requirements
- No Standardized Testing
- Clinical and Student Teaching Information
Transferring
- FAQs about Transferring
- Transfer to WGU
- Transferrable Certifications
- Request WGU Transcripts
- International Transfer Credit
- Tuition and Fees
- Financial Aid
- Scholarships
Other Ways to Pay for School
- Tuition—School of Business
- Tuition—School of Education
- Tuition—School of Technology
- Tuition—Leavitt School of Health
- Your Financial Obligations
- Tuition Comparison
- Applying for Financial Aid
- State Grants
- Consumer Information Guide
- Responsible Borrowing Initiative
- Higher Education Relief Fund
FAFSA Support
- Net Price Calculator
- FAFSA Simplification
- See All Scholarships
- Military Scholarships
- State Scholarships
- Scholarship FAQs
Payment Options
- Payment Plans
- Corporate Reimbursement
- Current Student Hardship Assistance
- Military Tuition Assistance
WGU Experience
- How You'll Learn
- Scheduling/Assessments
- Accreditation
- Student Support/Faculty
- Military Students
- Part-Time Options
- Virtual Military Education Resource Center
- Student Outcomes
- Return on Investment
- Students and Gradutes
- Career Growth
- Student Resources
- Communities
- Testimonials
- Career Guides
- Skills Guides
- Online Degrees
- All Degrees
- Explore Your Options
Admissions & Transfers
- Admissions Overview
Tuition & Financial Aid
Student Success
- Prospective Students
- Current Students
- Military and Veterans
- Commencement
- Careers at WGU
- Advancement & Giving
- Partnering with WGU
Peer Learning: Overview, Benefits, and Models
- Classroom Strategies
- See More Tags
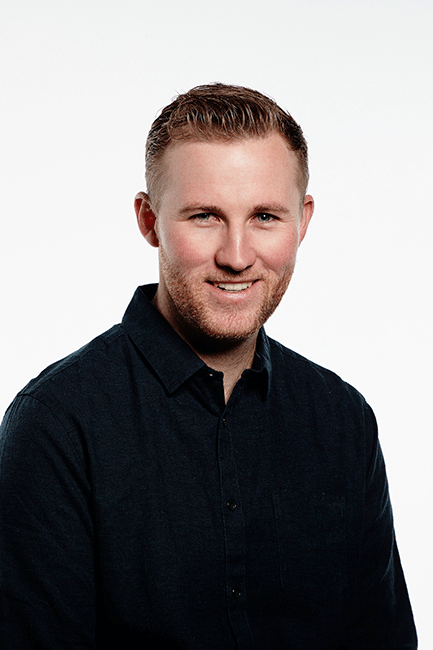
How do K-12 teachers facilitate effective learning? The best teachers do more than just read from a textbook. They understand that there are many different techniques, theories, and teaching models that can give students a well-rounded education that’s foundational to a lifetime of success and continual improvement.
Effective learning happens in many ways. Some students learn well directly from a teacher. Others are skilled independent learners. Yet, one of the most effective active learning techniques is that of peer learning. Put simply, peer learning is when students teach each other. This type of learning aids retention and encourages communication and collaboration.
Learn more about peer learning and how a teaching degree from WGU can prepare you to make a difference in the classroom.
What Is Peer Learning?
Peer learning is an education method that helps students solidify their knowledge by teaching each other. One student tutoring another in a supervised environment can result in better learning and retention. Why? Because to teach another, one must first fully understand a concept themselves. Verbalizing a concept and sharing the information with a peer serves to reinforce the knowledge gained.
Peer learning is best supported by other learning strategies, including the Constructivism Learning Theory and the Connectivism Learning Theory .
Constructivist learning suggests that knowledge is constructed by each individual student. The new concepts they learn are built upon their existing knowledge and beliefs. Constructivism also proposes that learning is an active process and a social activity. These concepts tie in well with peer learning.
Next, there’s Connectivism. Introduced in 2005 by George Siemens, the Connectivism Learning Theory focuses on technology as a critical component of connected learning. Today’s social networks allow rapid information transfer, but not every piece of information is equally helpful or enriching. Siemens suggests that being able to distinguish between important and unimportant information is vital. Even young students today are connected to the world and to each other through online means. An understanding of connectivism is especially helpful for K-12 teachers in the digital age.
Why Is Peer Learning Important?
To thrive in school, in the workplace, and in society, individuals must be able to learn from others and work with them to achieve mutual success. Below are even more reasons why peer learning is important.
Teamwork: Peer learning fosters teamwork, cooperation, patience, and better social skills. In a cooperative peer learning environment, each student’s strengths can serve to complement the group and enhance learning. Becoming skilled at working with and learning from one's peers can start at a young age in the classroom.
Better Feedback : Often, students are not able to recognize the gaps in their own knowledge. But when they learn with their peers, they can see new processes for answering questions and come up with creative, collaborative solutions. Importantly, they will carry these new perspectives, as well as a willingness to seek and accept feedback, with them as they progress in their education.
Supports Diversity: Peer learning fosters diversity and depth in a student’s knowledge and opinions. Learning from peers of different backgrounds, views, and ethnicities fosters an environment of mutual respect, gratitude, and progress. It’s the differences between students that add a richness to the learning environment. Supporting diversity through peer learning is part of culturally responsive teaching .
What Are the Benefits of Peer Learning?
It’s hard to number all the benefits of peer learning, but some of them include new perspectives, more social interaction, and deepened personal learning. See more information on these specific areas below.
New Perspectives for Students: If a student learns exclusively from the teacher, they may only gain one new perspective. Learning from their peers can add numerous helpful perspectives, nuances, and layers to a student’s knowledge.
Social Interaction Makes Studying Fun: By nature, humans are social beings. We long to make connections and be part of a group. The added element of social interaction in peer learning can be exciting and enriching. Students who may be hesitant to interact with the teacher may be more willing to open up to their peers.
Teaching Others Helps Students Learn: Nothing requires you to feel confident in your own knowledge quite like teaching what you know to someone else. As mentioned, peer learning can help students learn and solidify their own knowledge. Effective teaching requires a deeper level of knowledge on a subject.
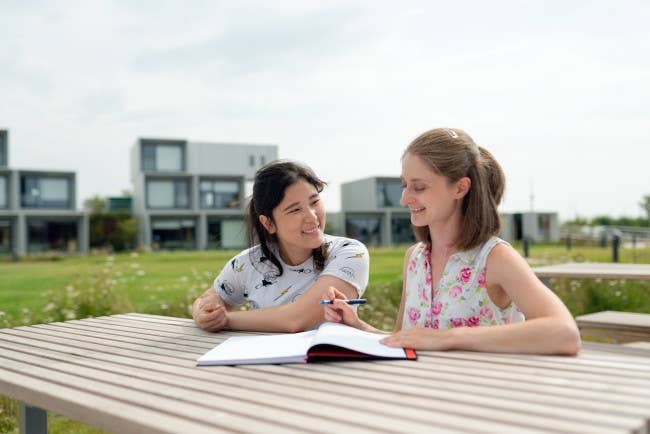
Peer Learning Drawbacks
While there are many benefits to peer learning, there are also some drawbacks, including distraction and lack of respect for feedback.
Working in Groups Can Be Distracting: Learning from your peers can be exciting. However, especially for younger students, that excitement can lead to distraction. When working with their friends, some students can easily get off track, misbehave, and focus on anything but learning.
Students Might Not Respect the Feedback of Their Peers: If a teacher gives feedback, the student is more likely to listen carefully. After all, the teacher is the authority in the classroom and the resident expert on the subject being taught. On the other hand, if one’s peer gives them feedback, it’s easier to disregard it.
Peer Learning Models
Effective peer learning can take place through many different models and strategies. See some of the tried-and-true ways to encourage peer learning.
Proctor Model: In the proctor model, an older or more experienced student teaches a younger or less experienced peer. In an elementary school, this might mean that students from a higher grade level come and teach kindergarteners. It could also entail having a more skilled student within the class teach their classmate.
Discussion Seminars: Discussion seminars are more common at the university level. They’re often held after students learn the material through a lecture or a weekly reading. Through these discussions, students deepen their knowledge and gain additional perspectives.
Peer Support Groups: Sometimes referred to as private study groups, peer support groups are student-led gatherings that are generally held outside of class without teacher support. Peers might meet up to study for a test together or complete a group project.
Peer Assessment Schemes: Peer assessment schemes can be common in writing courses. For instance, an AP English Language teacher might have students read one another’s essays to provide informal feedback.
Collaborative Projects: Assigning students to work on collaborative projects can serve them well for their future endeavors in the workplace and society. These projects teach collaboration, the importance of combining skills, and the need to meet deadlines.
Cascading Groups: Cascading groups is a learning method by which students are split into groups that get either progressively larger or smaller. For instance, students might be encouraged to learn about a distinct topic on their own and then share it with a partner. That partnership would then share their knowledge with another partnership and so forth.
Mentoring: A mentor is someone who has experience in a certain area. They guide a student, training them and teaching them the lessons they once had to learn. Peer tutoring is a form of mentoring. Sometimes students who require extra support are assigned a personal peer mentor who works one-on-one with them to help them succeed.
Reciprocal Teaching: In reciprocal teaching, students must develop the skills of questioning, predicting, summarizing, and clarifying. They teach one another using these techniques. They serve to form a sort of scaffolding for peer-led learning.
Jigsaw Method: In the jigsaw method of peer learning, students are split into groups, with each group given a different topic to study. Then, one student from each group is taken to form a collaborative group where multiple concepts are discussed. If there are eight jigsaw groups, then eight topics will ultimately be discussed in one group.
Discover More Learning Models with WGU
Peer learning is an effective way to facilitate deep learning. It also lends itself to many different approaches. The power of a classroom where students come together is that of collaborative learning. Teachers who implement peer learning strategies in their classroom may see higher levels of student performance, satisfaction, and overall engagement.
If you’re ready to learn new teaching methods and prepare to make a difference in the classroom, check out the WGU School of Education . The programs help teachers learn up-to-date teaching methods for the modern learning environment.
Ready to Start Your Journey?
HEALTH & NURSING
Recommended Articles
Take a look at other articles from WGU. Our articles feature information on a wide variety of subjects, written with the help of subject matter experts and researchers who are well-versed in their industries. This allows us to provide articles with interesting, relevant, and accurate information.
{{item.date}}
{{item.preTitleTag}}
{{item.title}}
The university, for students.
- Student Portal
- Alumni Services
Most Visited Links
- Business Programs
- Student Experience
- Diversity, Equity, and Inclusion
- Student Communities
Change Password
Your password must have 8 characters or more and contain 3 of the following:.
- a lower case character,
- an upper case character,
- a special character
Password Changed Successfully
Your password has been changed
- Sign in / Register
Request Username
Can't sign in? Forgot your username?
Enter your email address below and we will send you your username
If the address matches an existing account you will receive an email with instructions to retrieve your username
Peer Instruction
- Jennifer K. Knight
- Cynthia J. Brame
Department of Molecular, Cellular, and Developmental Biology, University of Colorado, Boulder, CO 80309
Search for more papers by this author
*Address correspondence to: Cynthia J. Brame ( E-mail Address: [email protected] ).
Center for Teaching and Department of Biological Sciences, Vanderbilt University, Nashville, TN 37203
Peer instruction, a form of active learning, is generally defined as an opportunity for peers to discuss ideas or to share answers to questions in an in-class environment, where they also have opportunities for further interactions with their instructor. When implementing peer instruction, instructors have many choices to make about group design, assignment format, and grading, among others. Ideally, these choices can be informed by research about the impact of these components of peer instruction on student learning. This essay describes an online, evidence-based teaching guide published by CBE—Life Sciences Education at http://lse.ascb.org/evidence-based-teaching-guides/peer-instruction . The guide provides condensed summaries of key research findings organized by teaching choices, summaries of and links to research articles and other resources, and actionable advice in the form of a checklist for instructors. In addition to describing key features of the guide, this essay also identifies areas in which further empirical studies are warranted.
INTRODUCTION
Peer instruction is a well-researched active-learning technique that has been widely adopted in college science classes. In peer instruction, the instructor poses a question with discrete options and gives students the chance to consider and record their answers individually, often by voting using clickers. Students then discuss their answers with neighbors, explaining their reasoning, before being given a chance to vote again. Finally, the instructor discusses the answer to the question, often soliciting input from the class. While instructors vary the exact implementation of this process—sometimes eliminating the individual voting process, sometimes using colored cards or a show of hands instead of clickers—the general process is an adaptation of the think–pair–share technique ( Crouch and Mazur, 2001 ).
Peer instruction can improve students’ conceptual understanding and problem-solving skills, an effect that has been observed in multiple disciplines, in courses at different levels, and with different instructors (for a review, see Vickrey et al. , 2015 ). Student response to peer instruction is generally positive; students report that the technique helps them learn course material and that the immediate feedback it provides is valuable.
Peer instruction’s value as a teaching approach is unsurprising, as it incorporates many elements known to promote learning. It is a form of cooperative learning, which has been shown to increase student achievement, persistence, and attitudes toward science (e.g., Johnson and Johnson, 2009 ). The peer instruction cycle provides opportunities for all the elements that social interdependence theory identify as necessary for cooperative learning: individual action; positive interdependence, wherein individual success is enhanced by the success of other group members; promotive interaction, or actions by individuals to help other group members’ efforts; and group processing ( Johnson and Johnson, 2009 ). It explicitly incorporates opportunities for students to explain their reasoning and engage in argumentation, practices that help students integrate new information with existing knowledge and revise their mental models (e.g., Chi et al. , 1994 ). In addition, as with many types of informal cooperative learning, peer instruction provides opportunities for formative assessment with immediate feedback and thus incorporates opportunities for students to be metacognitive, monitoring their understanding and reflecting on misunderstanding ( McDonnell and Mullally, 2016 ).
In implementing peer instruction, instructors have many choices to make that can impact students’ experience. In this article, we describe an evidence-based teaching guide that condenses, summarizes, and provides actionable advice from research findings (including many articles from CBE—Life Sciences Education ). It can be accessed at http://lse.ascb.org/evidence-based-teaching-guides/peer-instruction . The guide has several features intended to help instructors: a landing page that indicates starting points for instructors ( Figure 1 ), syntheses of observations from the literature, summaries of and links to selected papers ( Figure 2 ), and an instructor checklist that details recommendations and points to consider. The guide is meant to aid instructors as they implement peer instruction and may also benefit researchers new to this area. Some of the questions that serve to organize the guide are highlighted below.
FIGURE 1. Screenshot of the landing page of the guide, which provides readers with an overview of choice points.
FIGURE 2. Screenshot showing a summary of research findings and representative article summaries for one element of peer instruction.
WHAT TYPES OF QUESTIONS SHOULD BE USED?
There are a few clear recommendations about the types of questions that are particularly beneficial in peer instruction. First, questions should be challenging enough to provoke interest and discussion, and the greatest gains are seen with the most difficult questions ( Knight et al. , 2013 ; Zingaro and Porter, 2014 ). Importantly, question difficulty is not necessarily defined by the level of cognitive activity a student engages in to answer the question (e.g., Bloom’s application vs. evaluation levels). Questions that require lower-order cognitive skills can promote as robust peer discussion as those that require higher-order skills, with discussions on both potentially leading to conceptual change ( Knight et al. , 2013 ; Lemons and Lemons, 2013 ). Further, questions that uncover misconceptions can have particular benefits ( Modell et al. , 2005 ), in that they expose students to a commonly held incorrect idea and then give them opportunity to discover why that idea is incorrect.
Are there question types or formats that are particularly effective at helping students meet particular types of outcomes? For example, do questions that ask students to illustrate their ideas, or constructively build theoretical models, impact student learning?
What combinations of question cognitive level (e.g., Bloom’s level) and difficulty help promote self-efficacy, conceptual change, and conceptual understanding? Do different “levels” of questions promote some of these outcomes over others?
WHAT INSTRUCTIONAL PRACTICES PROMOTE PRODUCTIVE PEER INTERACTIONS?
Incentives for students to participate in peer instruction increase student engagement. Low-stakes grading incentives, in which correct and incorrect answers receive equal or very similar credit, result in more robust exchanges of reasoning and more equitable contribution of all group members to the discussion, whereas high-stakes grading incentives tend to lead to dominance of the discussion by a single group member (e.g., James, 2006 , and others within the Accountability section of the guide). Social incentives can also impact peer discussion. For example, randomly calling on groups to explain reasoning for an answer rather than asking for volunteers increases exchanges of reasoning during peer discussion ( Knight et al. , 2016 ).
Instructor cues that encourage students to explain their reasoning influence both student behavior and the classroom norms that students perceive. Thus, these cues can have a large impact on the nature of peer discussion ( Turpen and Finkelstein, 2010 , and others in the Instructional Cues section of the guide). Specifically, instructor language that encourages students to explain their reasoning can lead to higher-quality peer discussion and greater use of scientific argumentation moves ( Knight et al. , 2013 ). Further, instructor-led discussion of the answer after peer discussion provides clear benefits, particularly for weaker students and on more difficult questions ( Smith et al. , 2009 , 2011 ; Zingaro and Porter, 2014 ).
One common practice may have unintended negative consequences. Traditional implementation of peer instruction involves displaying the histogram of student responses after students answer individually but before peer discussion. Several lines of work suggest that this practice may bias students toward the most common answer and reduce the value of peer discussion ( Perez et al. , 2010 ). Thus, instructors may choose to prompt peer discussion that focuses on reasoning before showing the response histogram, and only use the histogram as a summary of student choices after students have shared their reasoning.
One of the steps that is most commonly omitted during peer instruction is the individual response ( Turpen and Finkelstein, 2009 ). Students have been reported to prefer the inclusion of individual thinking time, and it appears to increase discussion time ( Nicol and Boyle, 2003 ; Nielsen et al. , 2014 ). What is the role of this step in promoting productive peer discussion? Can objective measures of student learning be applied to determine its efficacy? ( Vickrey et al. , 2015 ).
Several studies indicate that students prefer to use personal response devices during peer instruction but that their use does not appear to impact students’ learning when compared with other reporting methods (such as a show of hands or colored cards). The role of anonymity and its potential relationship to stereotype threat has not been investigated, however. Can peer instruction induce stereotype threat, and if so, can the effect be mitigated by an anonymous reporting device or by other instructor interventions?
Further, stereotype threat is most relevant when people are working at the edge of their ability ( O’Brien and Crandall 2003 ), and it therefore seems more likely to be a factor for more difficult peer instruction questions. While active-learning approaches have generally been shown to be particularly effective for students from underrepresented groups (e.g., Eddy and Hogan, 2014 ), investigating the nuanced effects within particular groups of students can help instructors make effective choices ( Eddy et al. , 2015 ). Can personal response devices, which afford anonymity, have particular value for more difficult questions?
WHAT CHALLENGES ARE ASSOCIATED WITH PEER INSTRUCTION?
Finally, it is important to note that there can be challenges to implementing peer instruction. As noted earlier, instructors implement peer instruction differently, leading to classroom norms that can work to enhance or detract from student learning and affect student perceptions. Further, students have many different kinds of discussions during peer instruction, not all focused on the topic and not all centered around the concepts instructors intend. By its very nature, peer instruction allows exposure to others’ ideas, which can lead to better understanding but also potentially to shared misconceptions, an effect that may be enhanced among students who feel less confident in the classroom. Thus, the peer discussion part of each clicker question cycle is truly the key to successful peer instruction. Perhaps due to the reasons cited above, peer instruction does not uniformly improve students’ course grades. However, it clearly improves students’ use of reasoning and argumentation skills ( Knight et al. , 2013 , 2016 ), which may contribute to student learning in nonobvious ways. Avoiding the pitfalls discussed in this article and maximizing the benefits of peer instruction require that instructors carefully construct challenging questions and intentionally promote classroom norms that value reasoning and argumentation.
ACKNOWLEDGMENTS
We acknowledge and thank Adele Wolfson and Kristy Wilson for their thoughtful review. We also thank William Pierce and Thea Clarke for their efforts in producing the Evidence-Based Teaching Guide website.
- Chi, M. T. H., de Leeuw, N., Chiu, M-H., & Lavancher, C. ( 1994 ). Eliciting self-explanations improves understanding . Cognitive Science , 18 , 439–477. Google Scholar
- Crouch, C. H., & Mazur, E. ( 2001 ). Peer instruction: Ten years of experience and results . American Journal of Physics , 69 , 970. 10.1119/1.1374249 Google Scholar
- Eddy, S. L., Brownell, S. E., Thummaphan, P., Lan, M-C., & Wenderoth, M. P. ( 2015 ). Caution, student experience may vary: Social identities impact a student’s experience in peer discussions . CBE—Life Sciences Education , 14 , ar45. Link , Google Scholar
- Eddy, S. L., & Hogan, K. A. ( 2014 ). Getting under the hood: How and for whom does increasing course structure work . CBE—Life Sciences Education , 13 , 453–468. Link , Google Scholar
- James, M. C. ( 2006 ). The effect of grading incentive on student discourse in peer instruction . American Journal of Physics , 74 , 689. Google Scholar
- Johnson, D. W., & Johnson, R. T. ( 2009 ). An educational psychology success story: Social interdependence theory and cooperative learning . Educational Research , 38 , 365–379. Google Scholar
- Knight, J. K., Wise, S. B., & Sieke, S. ( 2016 ). Group random call can positively affect student in-class clicker discussions . CBE—Life Sciences Education , 15 (4), ar56. Link , Google Scholar
- Knight, J. K., Wise, S. B., & Southard, K. M. ( 2013 ). Understanding clicker discussions: Student reasoning and the impact of instructional cues . CBE—Life Sciences Education , 12 , 645–654. Link , Google Scholar
- Lemons, P. P., & Lemons, J. D. ( 2013 ). Questions for assessing higher-order cognitive skills: It’s not just Bloom’s . CBE—Life Sciences Education , 12 , 47–58. Link , Google Scholar
- McDonnell, L., & Mullally, M. ( 2016 ). Research and teaching: Teaching students how to check their work while solving problems in genetics . Journal of College Science Teaching , 46 , 68–75. Google Scholar
- Modell, H., Michael, J., & Wenderoth, M. P. ( 2005 ). Helping the learner to learn: The role of uncovering misconceptions . The American Biology Teacher , 67 , 20. Google Scholar
- Nicol, D. J., & Boyle, J. T. ( 2003 ). Peer instruction versus class-wide discussion in large classes: A comparison of two interaction methods in the wired classroom . Studies in Higher Education , 28 , 458–473. Google Scholar
- Nielsen, K. L., Hansen, G., & Stav, J. B. ( 2014 ). How the initial thinking period affects student argumentation during peer instruction: Students’ experiences versus observations . Studies in Higher Education , 3 , 1–15. Google Scholar
- O’Brien, L. T., & Crandall, C. S. ( 2003 ). Stereotype threat and arousal: Effects on women’s math performance . Personality and Social Psychology Bulletin , 29 , 782–789. Medline , Google Scholar
- Perez, K. E., Strauss, E. A., Downey, N., Galbraith, A., Jeanne, R., & Cooper, S. ( 2010 ). Does displaying the class results affect student discussion during peer instruction . CBE—Life Sciences Education , 9 , 133–140. Link , Google Scholar
- Smith, M. K., Wood, W. B., Adams, W. K., Wieman, C., Knight, J. K., Guild, N., & Su, T. T. ( 2009 ). Why peer discussion improves student performance on in-class concept questions . Science , 323 , 122–124. Medline , Google Scholar
- Smith, M. K., Wood, W. B., Krauter, K., & Knight, J. K. ( 2011 ). Combining peer discussion with instructor explanation increases student learning from in-class concept questions . CBE—Life Sciences Education , 10 , 55. Link , Google Scholar
- Turpen, C., & Finkelstein, N. D. ( 2009 ). Not all interactive engagement is the same: Variations in physics professors’ implementation of peer instruction . Physical Review Special Topics–Physics Education Research , 5 , 020101. Google Scholar
- Turpen, C., & Finkelstein, N. D. ( 2010 ). The construction of different classroom norms during peer instruction: Students perceive differences . Physical Review Special Topics–Physics Education Research , 6 , 020123. Google Scholar
- Vickrey, T., Rosploch, K., Rahmanian, R., Pilarz, M., & Stains, M. ( 2015 ). Research-based implementation of peer instruction: A literature review . CBE—Life Sciences Education , 14 , es3. Link , Google Scholar
- Zingaro, D., & Porter, L. ( 2014 ). Peer instruction in computing: The value of instructor intervention . Computers & Education , 71 , 87–96. Google Scholar
- Tab-Meta Key: A Model for Exam Review 30 January 2024 | Journal of College Science Teaching, Vol. 53, No. 1
- Differentiated Instruction as a Viable Framework for Meeting the Needs of Diverse Adult Learners in Health Professions Education 28 June 2023 | Medical Science Educator, Vol. 33, No. 4
- Incorporating Technologies to Achieve Active Learning in Large Engineering Classrooms 19 May 2023
- Simplifying Texts for Easier Comprehension in an Introductory Computer Science Course: An Evaluation of Rewordify 28 July 2023
- The Future of Higher Education: Identifying Current Educational Problems and Proposed Solutions 3 December 2022 | Education Sciences, Vol. 12, No. 12
- Student Response Systems in Online Nursing Education 1 Dec 2022 | Nursing Clinics of North America, Vol. 57, No. 4
- Reactions of Computer Science Students to Peer Instruction Activities in Class 1 Dec 2022
- Implementing Design Thinking and Peer Instruction in an Online Environment to Develop Tourism Undergraduates’ English Language Skills 1 March 2023 | Journal of Language and Cultural Education, Vol. 10, No. 2
- Review of Trends in Peer Instruction: Bibliometric Mapping Analysis and Systematic Review 13 January 2022 | Journal of Learning and Teaching in Digital Age, Vol. 7, No. 1
- 2022 | Education and Information Technologies, Vol. 27, No. 4
- 2022 | Academic Psychiatry, Vol. 46, No. 4
- Applying Peer Instruction to Computer Science Students Using Non-native Language: A Study with Undergraduate Students 5 Dec 2021
- Connecting Activity Implementation Characteristics to Student Buy-In Toward and Utilization of Formative Assessments Within Undergraduate Biology Courses 8 June 2021 | Journal for STEM Education Research, Vol. 4, No. 3
- Group Size and Peer Learning: Peer Discussions in Different Group Size Influence Learning in a Biology Exercise Performed on a Tablet With Stylus 10 November 2021 | Frontiers in Education, Vol. 6
- An agile approach to teach introductory programming in the hybrid classroom 1 Jul 2021
- Learning by Creating: Making Games in a Political Science Course 10 December 2020 | PS: Political Science & Politics, Vol. 54, No. 2
- The Impact of Peer Instruction on Ninth Grade Students’ Trigonometry Knowledge 1 January 2021 | Bolema: Boletim de Educação Matemática, Vol. 35, No. 69
- Guiding the use of collective feedback displayed on heatmaps to reduce group conformity and improve learning in Peer Instruction 19 June 2020 | Journal of Computer Assisted Learning, Vol. 36, No. 6
- Meeting the Needs of A Changing Landscape: Advances and Challenges in Undergraduate Biology Education 13 May 2020 | Bulletin of Mathematical Biology, Vol. 82, No. 5
- Peer Interaction in Active Learning Biology 24 February 2020
- 2020 | Informação & Informação, Vol. 25, No. 3
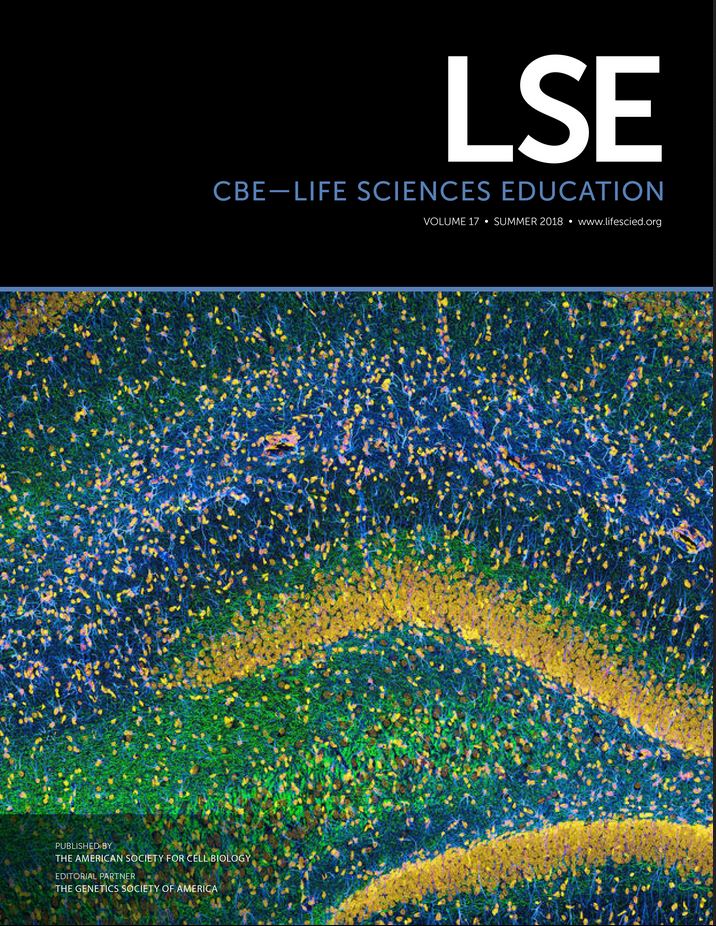
© 2018 J. K. Knight and C. J. Brame. CBE—Life Sciences Education © 2018 The American Society for Cell Biology. This article is distributed by The American Society for Cell Biology under license from the author(s). It is available to the public under an Attribution–Noncommercial–Share Alike 3.0 Unported Creative Commons License (http://creativecommons.org/licenses/by-nc-sa/3.0).

An official website of the United States government
The .gov means it’s official. Federal government websites often end in .gov or .mil. Before sharing sensitive information, make sure you’re on a federal government site.
The site is secure. The https:// ensures that you are connecting to the official website and that any information you provide is encrypted and transmitted securely.
- Publications
- Account settings
Preview improvements coming to the PMC website in October 2024. Learn More or Try it out now .
- Advanced Search
- Journal List
- Iran J Public Health
- v.42(11); 2013 Nov
The Peer Education Approach in Adolescents- Narrative Review Article
Fatemeh abdi.
1. Students Research Committee, Shahid Beheshti University of Medical Sciences, Tehran, Iran
Masoumeh Simbar
2. Dept. of Reproductive Health, Faculty of Nursing and Midwifery, Shahid Beheshti University of Medical Sciences, Tehran, Iran
Adolescence is an important stage of human life span, which crucial developmental processes occur. Since peers play a critical role in the psychosocial development of most adolescents, peer education is currently considered as a health promotion strategy in adolescents. Peer education is defined as a system of delivering knowledge that improves social learning and provides psychosocial support. As identifying the outcomes of different educational approaches will be beneficial in choosing the most effective programs for training adolescents, the present article reviewed the impact of the peer education approach on adolescents. In this review, databases such as PubMed, EMBASE, ISI, and Iranian databases, from 1999 to 2013, were searched using a number of keywords. Peer education is an effective tool for promoting healthy behaviors among adolescents. The development of this social process depends on the settings, context, and the values and expectations of the participants. Therefore, designing such programs requires proper preparation, training, supervision, and evaluation.
Introduction
Adolescence, an important stage of human life ( 1 ), involves crucial developmental processes ( 2 ) through which a person goes over to adulthood from childhood ( 3 ). These changes may potentially pose pressure on adolescents ( 4 ) and cause multidimensional problems necessitating a holistic approach. The majority of adolescents experience some level of emotional, behavioral, and social difficulties ( 2 , 5 ). On the other hand, adolescents naturally tend to resist any dominant source of authority such as parents and prefer to socialize more with their peers than with their families ( 4 , 6 ). Research suggests that adolescents are more likely to modify their behaviors and attitudes if they receive health messages from peers who face similar concerns and pressures ( 7 ).
A peer is a person whose has equal standing with another as in age, background, social status, and interests. Peers play a critical role in the psychosocial development of most adolescents. They, in fact, provide opportunities for personal relationships, social behaviors, and a sense of belonging. Therefore, peer education is considered as a health promotion strategy in adolescents ( 8 , 9 ).
Adolescents comprise 20% of the world population and live mostly (85%) in developing communities ( 10 ). Moreover, about a quarter (25.1%) of Iran’s population belongs to the age group of 11-14 years old. Unfortunately, more than half of this huge population does not develop healthy life skills. Since peers can effect on each other’s feelings of health, habits, and behaviors ( 11 , 12 ), various studies have indicated peer education to be more effective than traditional methods (e.g. training provision by teachers) when sensitive subjects like sexual relationships and substance abuse are concerned ( 12 ). Studies have also evaluated peer education as a mechanism to promote behavior and attitude modification ( 13 ). Peer education has been shown beneficial in improving knowledge and the intention to change behavior in human immunodeficiency virus infection/acquired immunodeficiency syndrome (HIV/AIDS) prevention programs among high school students ( 14 ). It is, hence, a system of delivering knowledge that promotes social skills ( 15 ).
As the important role of peers in quality of life of adolescents warrants further research on peer education, the present study reviewed the peer education approach in adolescents. Knowing the outcomes of different educational approaches will help choose the most effective programs in training adolescents.
Searching Method
In this narrative literature review, databases of PubMed, EMBASE, ISI, and Iranian databases including IranMedex and SID were searched to review the relevant literature. A comprehensive search was performed through PubMed and Google scholar using the combinations of the following keywords: adolescent, peer, peer group, peer education, peer intervention, peer educator. All published data from 1999 to 2013 were then included in this review.
Results and Discussion
Peer education (pe).
Peer education is known as sharing of information and experiences among individuals with something in common ( 16 , 17 ). It aims to assist young people in developing the knowledge, attitudes, and skills that are necessary for positive behavior modification through the establishment of accessible and inexpensive preventive and psychosocial support. Peer education programs mainly focus on harm reduction information, prevention, and early intervention. The youth have accepted peer education as a preferred strategy to reach unreachable populations such as sex workers and to approach and discuss topics that are insufficiently addressed or considered taboo within other contexts ( 17 – 19 ). Sexual health peer education has been found to significantly increase the use of modern contraceptives and methods to prevent sexually transmitted infections (STIs) ( 20 ). A systematic review of interventions to prevent the spread of STIs among young people indicated that peer-led interventions were more accepted, and thus more successful in improving sexual knowledge, than teacher-led interventions ( 21 ).
Different methods of peer education have been proposed. The audience can be reached through a variety of interactive strategies such as small group presentations, role plays, or games ( 15 ). Formal delivery of peer education in highly structured settings such as class teaching in schools is also possible. Other methods may include informal tutoring in unstructured settings during the course of everyday interactions or individual discussions and counseling. Various methods are adopted based on the intended outcomes of the project (e.g. communicating information, behavior modifi-cation, or development of skills) ( 22 ).
Peer educator
A peer educator is a member of a peer group that receives special training and information and tries to sustain positive behavior change among the group members ( 18 , 23 ). The levels of trust and comfort between the peer educator and his/her peer group will facilitate more open discussions on sensitive topics ( 24 ). Peer educators can in fact act as role models of attitude and behavior for their peers ( 25 ).
Peer educators should receive adequate training enabling them to understand the purpose of the program, be good listeners, provide encouragement, motivation, and support healthy decisions and behaviors. They should also know other sources of information and counseling so as to refer other peers to appropriate help ( 5 ).
More attention to the specific personal characteristics, for instance leadership skills of peer educators is important ( 26 ). Identification and selection of peer educators with sufficient confidence, technical competency, compassion, and communication skills who are accepted by other peers are crucial aspects of program success ( 27 ). Borgia et al. stated that peer educator selection is a crucial and delicate point in the efficacy of peer education interventions ( 28 ).
Peer educators should allow that emotions, feelings, attitudes, and beliefs to be expressed and discussed openly ( 29 ). They should also be aware of the usefulness of jokes and humor in establishing relationships with the target group ( 23 ). Moreover, initiation of trainings at early ages of adolescence will maintain and consolidate a healthy function. Nevertheless, educational outcomes will widely depend on the relationship with peers ( 29 ). Sharing socioeconomic conditions with program participants, peer educators are able to make educational material accessible and credible to participants and hence increase the efficacy of a peer education program ( 15 ). A variety of financial, intellectual, and emotional reasons leads to the attractiveness of youth peer education. In addition, the participation of unpaid volunteers makes peer education inexpensive ( 30 ).
Theories of Peer Education
As a broadly accepted effective behavioral change strategy, peer education relies on several well-known behavioral theories:
The social learning theory asserts that some individuals function as role models of human behavior due to their aptitude for stimulating behavior changes in other individuals ( 31 ).
The theory of reasoned action states that a person’s perception of social norms or beliefs about what people, who are important to the individual, do or think about a particular behavior can affect behavior change ( 32 ). In other words, people’s attitudes toward changing a behavior is strongly influenced by their view of its positive or negative consequences and what their peer educators would think about it ( 7 ).
The diffusion of innovation theory considers an innovation as new information, an attitude, a belief, or a practice that is perceived as novel by an individual and that can be diffused to a particular group. This theory employs ‘opinion leaders’ to propagate information, influence group norms, and finally act as change agents within the population they belong to ( 27 ).
The theory of participatory education has also played a key role in the development of peer education. According to participatory or empo-werment models of education, powerlessness at the community or group level along with socioeconomic conditions caused by the lack of power are major risk factors for poor health ( 7 ).
The social inoculation theory postulates that people may adopt unhealthy behaviors under social pressures ( 33 ).
Other available theories (the role theory, health belief model, and transtheoretical model) imply partnership, ownership, empowerment, and reinforcement as the critical principles of peer education.
Peer education program
Peer education programs have been used as public health strategies to promote various positive health behaviors such as smoking cessation and vio-lence, substance abuse, and HIV/AIDS prevention. Since such programs seek to produce behavior change in a peer group (the unit of change) by the help of a peer educator or facilitator (the agent of change) ( 34 ), they may simultaneously empower the educator and the target group by creating a sense of collective action. In non-hierarchical structure, the management structure of peer education comprises two distinct parallel roles ( 15 ), i.e. peer educators and adult support workers. While the first group are the “bosses” and control the direction of the program, the second group (also known as program facilitators) guide and support the peer educators throughout the process ( 35 , 36 ) ( Fig. 1 ). Peer education programs require careful planning ( 37 ), identification and training of peer educators, and follow-up evaluations.

Management model of peer education program
Peer educator training, as the most important component of a peer education program, involves:
- An introductory meeting to familiarize the peer educators with the concept of peer education and the training needs;
- Training the educators with communication, facilitation, research, and evaluation skills;
- Providing opportunities for personal development;
- Providing access to formal knowledge ( 13 ).
The period between the training and the delivery of knowledge to the target group should not be longer than a few weeks ( 23 ). After the initial training, peer educators will undoubtedly require continuous supervision and opportunities to give feedback about the program ( 38 ).
Peer education strategies engage all five senses and can also improve the participants’ power of thinking and innovation. In fact, the participants will take part in all stages of the program including planning, implementation, and evaluation ( 12 ). Studies with more rigorous designs reported peer education programs to increase knowledge and help-seeking about STIs and condom use to prevent HIV infection and to delay first sexual experience ( 39 ). Youth peer education programs, whose numbers are growing throughout the world, are extensively used to promote reproductive health. These programs require appropriate technical frameworks, particularly training and supervision, to satisfy the needs of the young and adolescent volunteers ( 30 ).
The general approach to peer observation was first described in Bell’s model ( Fig. 2 ) which involved pre-observation meeting, observation, post-observation feedback, and reflection ( 40 ).

Peer observation process (Bell’s model)
Peer education intervention
Peer education interventions are commonly employed to prevent HIV and other STI ( 41 ). By selecting and training peer educators, peer education interventions try to increase the peer group’s knowledge and stimulate behavior change among them. More cost-effective than programs that incorporate highly trained professionals; have been applied in various target populations including the youth, commercial sex workers, and injection drug abusers in developing countries ( 42 , 43 ). A study in 10 African, Asian, and Latin American countries indicated that peer education interventions can be effective strategies in prevention of risky behaviors and increasing self-esteem and psychosocial aspects ( 12 ). According to Merakou and Kourea-Kremastinou, peer education interventions can affect the youth’s behavior about self-protection from HIV infection ( 25 ). Similarly, a systematic review suggested peer learning as an efficient method in improving the standing of health science students in clinical placements ( 44 ).
Peer education interventions can be used in multiple domains including physical activity, mental health, nutrition, HIV/AIDS and STIs, tobacco and alcohol use, and drug abuse. Visser believed that peer education can postpone the onset of sexual activity and hence play a critical role in the prevention of HIV/AIDS among adolescents ( 45 ). Besides, other researchers have identified school-based HIV education as the basis of youth-focused HIV prevention interventions ( 46 ). Studies have found the mean score of knowledge regarding breast self-examination to increase in students who receive peer education about breast cancer prevention through the learning of self-examination ( 29 , 47 ). Rhee et al. showed that a peer-led asthma self-management program can be successfully implemented and absorbed by adolescent learners ( 48 ). In addition, the peer education program designed by Karayurt et al. could increase knowledge about breast cancer, enhance the performance of breast self-examination, and improve perceived health beliefs ( 49 ). Peer mentorship has also been broadly and successfully used to treat alcohol and substance abuse disorders ( 50 ). Finally, some researchers believe that although school-based behavioral interventions which teach sexual health skills can improve the youth’s levels of knowledge and self-efficacy, they may not have great impacts on sexual behavior ( 51 , 52 ).
We briefly reviewed the impacts of the peer education approach on adolescents. Peer education, which is considered as an effective tool in promoting healthy behaviors among adolescents ( 53 ), is a social process affected by the settings, organizational context, key personnel, and the values and expectations of the participants. It requires proper preparation, training, supervision, and evaluation. We found various studies suggesting the success of different peer education programs. We hope that this paper will serve as a starting point in the application of this method in health promotion.
Ethical Considerations
Ethical issues including plagiarism, data falsification, double publication or submission have been completely observed by the authors.
Acknowledgements
We would like to express our appreciation to everyone involved in this project. The authors declare that there is no conflict of interest.
- Shahhosseini Z, Simbar M, Ramezankhani A, Majd HA (2012). An inventory for assessment of the health needs of Iranian female adolescents . East Mediterr Health J , 18 ( 8 ):850–856. [ PubMed ] [ Google Scholar ]
- Anisi J, Salimi H, Mirzamani M, Reisi F, Niknam m (2007). A Survey Study on Behavioral Problems in Adolescence . Journal of Behavioral Sciences , 1 ( 2 ):163–170.[Persian] [ Google Scholar ]
- Alavi Manizheh PK, Khosravi A (2009). Puberty health: knowledge, attitude and practice of the adolescent girls in Tehran, Iran. Payesh , 8 ( 1 ( 29 ):59–65. [Persian] [ Google Scholar ]
- Golchin NA, Hamzehgardeshi Z, Fakhri M, Hamzehgardeshi L (2012). The experience of puberty in Iranian adolescent girls: a qualitative content analysis . BMC Public Health , 12 :698. [ PMC free article ] [ PubMed ] [ Google Scholar ]
- World Health Organization (2005). Adolescent peer education in formal and non-formal setting . p:1–37. [ Google Scholar ]
- Akers AY, Gold MA, Bost JE, Adimora AA, Orr DP, Fortenberry JD (2011). Variation in sexual behaviors in a cohort of adolescent females: the role of personal, perceived peer, and perceived family attitudes. J Adolesc Health- , 48 ( 1 ):87–93. [ PMC free article ] [ PubMed ] [ Google Scholar ]
- Wye SQ, Madden A, Poeder F, McGuckin S, Shying K (2006). A framework for peer education by drug-user organizations. Australia, pp:5–39. [ Google Scholar ]
- Peykari N, Tehrani FR, Malekafzali H, Hashemi Z, Djalalinia Sh (2011). An Experience of Peer Education Model among Medical Science University Students in Iran . Iran J Publ Health , 40 ( 1 ):57–62. [ PMC free article ] [ PubMed ] [ Google Scholar ]
- Ochieng BM(2001). Health promotion strategy for adolescents’ sexual behaviour . J Child Health Care , 5 ( 2 ):77–81. [ PubMed ] [ Google Scholar ]
- Shahhosseini Z, Simbar M, Ramezankhani A, Alavi Majd H, Moslemizadeh N (2013). The Challenges of Female Adolescents’ Health Needs . Community Ment Health J , May 16. [Epub ahead of print]. [ PubMed ] [ Google Scholar ]
- Parvizi S, Ahmadi F (2007). Adolescence health and friendships, a Qualitative study. Feyz ,- 10 ( 4 ):46–51. [Persian] [ Google Scholar ]
- Noori Sistani M, Merghati K (2010). The impact of peer-based educational approaches on girls’ physicalpractice of pubertal health . Arak Medical University Journal , 12 ( 4 ):129–135.[Persian] [ Google Scholar ]
- Campbell C, MacPhail C (2002). Peer education, gender and the development of critical consciousness: participatory HIV prevention by southern African youth. Social Science & Medicine , 55 ( 2 ), 331–345. [ PubMed ] [ Google Scholar ]
- Cai Y, Hong H, Shi R, Ye X, Xu G, Li S, Shen L(2008). Long-term follow-up study on peer-led school-based HIV/AIDS prevention among youths in Shanghai. Int J STD AIDS , 19 ( 12 ):848–850. [ PubMed ] [ Google Scholar ]
- Evans DL, Tripp JH (2006). Sex education: the case for primary prevention and peer education . Current Paediatrics , 16 ( 2 ), 95–99. [ Google Scholar ]
- Sandhu S, Veinot P, Embuldeniya G, Brooks S, Sale J (2013). Peer-to-peer mentoring for individuals with early inflammatory arthri-tis:feasibility pilot . BMJ Open , 3 ( 3 ). pii: e002267. [ PMC free article ] [ PubMed ] [ Google Scholar ]
- Peel NM, Warburton J (2009). Using senior volunteers as peer educators: What is the evidence of effectiveness in falls prevention? Australas J Ageing , 28 ( 1 ):7–11. [ PubMed ] [ Google Scholar ]
- Mason-Jones AJ, Flisher AJ, Mathews C(2011). Who are the peer educators? HIV prevention in South African schools. Health Educ Res , 26 ( 3 ):563–571. [ PubMed ] [ Google Scholar ]
- Swartz S, Deutsch C, Makoae M, Michel B, Harding JH, Garzouzie G (2012). Measuring change in vulnerable adolescents: findings from a peer education evaluation in South Africa . SAHARA J , 9 ( 4 ):242–254. [ PubMed ] [ Google Scholar ]
- Speizer IS, Tambashe BO, Tegang SP (2001). An evaluation of the “Entre Nous Jeunes” peereducator program for adolescents in Cameroon . Stud Fam Plann , 32 ( 4 ):339–351. [ PubMed ] [ Google Scholar ]
- Lazarus JV, Sihvonen-Riemenschneider H, Laukamm-Josten U, Wong F, Liljestrand J(2010). Systematic review of interventions to prevent the spread of sexually transmitted infections, including HIV, among young people in Europe. Croat Med J , 51 ( 1 ):74–84. [ PMC free article ] [ PubMed ] [ Google Scholar ]
- Turner G, Shepherd J (1999). A method in search of a theory: peer education and health promotion . Health Educ Res , 14 ( 2 ):235–247. [ PubMed ] [ Google Scholar ]
- Strange V, Forrest S, Oakley A (2002). Peer-led sex education--characteristics of peer educators and their perceptions of the impact on them of participation in a peer education programme . Health Educ Res , 17 ( 3 ):327–337. [ PubMed ] [ Google Scholar ]
- Medley A, Kennedy C, O’Reilly K, Sweat M (2009). Effectiveness of peer education interventions for HIV prevention in developing countries: a systematic review and meta-analysis . AIDS Educ Prev , 21 ( 3 ):181–206. [ PMC free article ] [ PubMed ] [ Google Scholar ]
- Merakou K, Kourea-Kremastinou J (2006). Peer education in HIV prevention: an evaluation in schools . Eur J Public Health , 16 ( 2 ):128–132. [ PubMed ] [ Google Scholar ]
- Shepherd J, Kavanagh J, Picot J, Cooper K, Harden A, Barnett-Page E(2010). The effecti-veness and cost-effectiveness of behavioral interventions for the prevention of sexually transmitted infections in young people aged 13–19: a systematic review and economic evaluation. Health Technol Assess , 14 ( 7 ):1–206, iii–iv. [ PubMed ] [ Google Scholar ]
- Al-Iryani B, Basaleem H, Al-Sakkaf K, Kok G, van den Borne B(2013). Process evaluation of school-based peer education for HIV prevention among Yemeni adolescents . SAHARA J , 10 ( 1 ):55–64. [ PMC free article ] [ PubMed ] [ Google Scholar ]
- Borgia P, Marinacci C, Schifano P, Perucci CA(2005). Is peer education the best approach for HIV prevention in schools? Findings from a randomized controlled trial. J Adolesc Health , 36 ( 6 ):508–516. [ PubMed ] [ Google Scholar ]
- Zangiabadizade M (2012). Comparing The Effect of Peer Education To Health Care Personnel’s on Knowledge of Breast Self-Examination and The Obstacles among Undergraduate Students of Shiraz University of Medical Sciences . Iranian Journal of Medical Education , 12 ( 8 ):607–615. [Persian] [ Google Scholar ]
- Svenson G, Burke H (2005). Formative Research on Youth Peer Education Program Productivity and Sustainability. Family health international. FHI Working Paper Series No. WP05–04 . [ Google Scholar ]
- Burke H, Mancuso L(2012). Social cognitive theory, metacognition, and simulation learning in nursing education. J Nurs Educ , 51 ( 10 ):543–548. [ PubMed ] [ Google Scholar ]
- Orr MG, Thrush R, Plaut DC(2013). The theory of reasoned action as parallel constraint satisfaction: towards a dynamic computational model of health behavior . PLoS One , 8 ( 5 ):e62490. [ PMC free article ] [ PubMed ] [ Google Scholar ]
- Banas John A. Rains SA (2010). A Meta-Analysis of Research on Inoculation Theory . Communication Monographs , 77 ( 3 ):281–311. [ Google Scholar ]
- Chandan U, Cambanis E, Bhana A, Boyce G, Makoae M, Mukoma W (2008). Evaluation of My Future Is My Choice (MFMC) Peer Education Life Skills Programme in Namibia: Identifying Strengths, Weaknesses, and Areas of Improvement. Windhoek, UNICEF Namibia. [ Google Scholar ]
- Backett-Milburn K, Wilson S (2000). Understanding peer education: insights from a process evaluation . Health Educ Res , 15 ( 1 ):85–96. [ PubMed ] [ Google Scholar ]
- Mellanby AR, Rees JB, Tripp JH (2000). Peer-led and adult-led school health education: a critical review of available comparative research . Health Educ Res , 15 ( 5 ):533–545. [ PubMed ] [ Google Scholar ]
- Rodgers J(2006). Guidance on delivering effective group education . Br J Community Nurs ,- 11 ( 11 ):476–482. [ PubMed ] [ Google Scholar ]
- Seymour JE, Almack K, Kennedy S, Froggatt K (2013). Peer education for advance care planning: volunteers’ perspectives on training and community engagement activities . Health Expect , 16 ( 1 ):43–55. [ PMC free article ] [ PubMed ] [ Google Scholar ]
- Mash R. Mash RJ (2012). A quasi-experimental evaluation of an HIV prevention programme by peer education in the Anglican Church of the Western Cape, South Africa. BMJ Open , 2 ( 2 ), 1–9. [ PMC free article ] [ PubMed ] [ Google Scholar ]
- Sullivan PB, Buckle A, Nicky G, Atkinson SH(2012). Peer observation of teaching as a faculty development tool . BMC Med Educ , 12 : 26 . [ PMC free article ] [ PubMed ] [ Google Scholar ]
- Tolli MV(2012). Effectiveness of peer education interventions for HIV prevention, adolescentpregnancy prevention and sexual health promotion for young people: a systematic review of European studies. Health Educ Res , 27 ( 5 ):904–913. [ PubMed ] [ Google Scholar ]
- Kim CR, Free C (2008). Recent evaluations of the peer-led approach in adolescent sexual health education: a systematic review . Perspect Sex Reprod Health , 40 ( 3 ):144–151. [ PubMed ] [ Google Scholar ]
- Harden A, Oakley A, Oliver S (2001). Peer delivered health promotion for young people: A systematic review of different study designs . Health Education Journal , 60 : 339–353. [ Google Scholar ]
- Secomb J(2008). A systematic review of peer teaching and learning in clinical education . J Clin Nurs , 17 ( 6 ):703–716. [ PubMed ] [ Google Scholar ]
- Visser MJ(2007). HIV/AIDS prevention through peer education and support in secondary schools in South Africa. SAHARA J , 4 ( 3 ):678–694. [ PMC free article ] [ PubMed ] [ Google Scholar ]
- Denison JA, Tsui S, Bratt J, Torpey K, Weaver MA, Kabaso M(2012). Do peer educators make a difference? An evaluation of a youth-led HIV prevention model in Zambian Schools. Health Educ Res , 27 ( 2 ):237–247. [ PubMed ] [ Google Scholar ]
- Malak AT, Dicle A(2007). Assessing the efficacy of a peer education model in teaching breast self-examination to university students . Asian Pac J Cancer Prev , 8 ( 4 ):481–484. [ PubMed ] [ Google Scholar ]
- Rhee H, McQuillan BE, Belyea MJ (2012). Evaluation of a peer-led asthma self-management program and benefits of the program for adolescent peer leaders . Respir Care , 57 ( 12 ):2082–2089. [ PMC free article ] [ PubMed ] [ Google Scholar ]
- Karayurt O, Dicle A, Malak AT (2009). Effects of peer and group education on knowledge, beliefsand breast self-examination practice amonguniversity students in turkey. Turk J Med Sc ., 39 ( 1 ):59–66. [ Google Scholar ]
- Tracy K, Burton M, Miescher A(2012)-. Mentorship for Alcohol Problems (MAP): a peer to peer modular intervention for outpatients. Alcohol , 47 ( 1 ):42–47. [ PMC free article ] [ PubMed ] [ Google Scholar ]
- Cooper K, Shepherd J, Picot J, Jones J, Kavanagh J, Harden A(2012). An economic model of school-based behavioral interventions to prevent sexually transmitted infections . Int J Technol Assess Health Care , 28 ( 4 ):407–414. [ PMC free article ] [ PubMed ] [ Google Scholar ]
- Shahhosseini Z, Simbar M, Ramezankhani A, Majd HA(2011). Iranian female adolescents reproductive health services needs: A qualitative study . World Applied Sciences Journal , 13 ( 7 ):1580–1585. [ Google Scholar ]
- Simbar M, Tehrani F. R, Hashemi Z (2005). Reproductive health knowledge, attitudes and practices of Iranian college students. East Mediterr Health J , 11 ( 5 / 6 ):199–208. [ PubMed ] [ Google Scholar ]
- Open access
- Published: 02 December 2022
School-based peer education interventions to improve health: a global systematic review of effectiveness
- Steven Dodd 1 na1 ,
- Emily Widnall 2 na1 ,
- Abigail Emma Russell 3 ,
- Esther Louise Curtin 4 ,
- Ruth Simmonds 5 ,
- Mark Limmer 1 &
- Judi Kidger 2
BMC Public Health volume 22 , Article number: 2247 ( 2022 ) Cite this article
13k Accesses
21 Citations
9 Altmetric
Metrics details
Introduction
Peer education, whereby peers (‘peer educators’) teach their other peers (‘peer learners’) about aspects of health is an approach growing in popularity across school contexts, possibly due to adolescents preferring to seek help for health-related concerns from their peers rather than adults or professionals. Peer education interventions cover a wide range of health areas but their overall effectiveness remains unclear. This review aims to summarise the effectiveness of existing peer-led health interventions implemented in schools worldwide.
Five electronic databases were searched for eligible studies in October 2020. To be included, studies must have evaluated a school-based peer education intervention designed to address the health of students aged 11–18-years-old and include quantitative outcome data to examine effectiveness. The number of interventions were summarised and the impact on improved health knowledge and reductions in health problems or risk-taking behaviours were investigated for each health area separately, the Mixed Methods Appraisal Tool was used to assess quality.
A total of 2125 studies were identified after the initial search and 73 articles were included in the review. The majority of papers evaluated interventions focused on sex education/HIV prevention ( n = 23), promoting healthy lifestyles ( n = 17) and alcohol, smoking and substance use ( n = 16). Papers mainly reported peer learner outcomes (67/73, 91.8%), with only six papers (8.2%) focussing solely on peer educator outcomes and five papers (6.8%) examining both peer learner and peer educator outcomes. Of the 67 papers reporting peer learner outcomes, 35/67 (52.2%) showed evidence of effectiveness, 8/67 (11.9%) showed mixed findings and 24/67 (35.8%) found limited or no evidence of effectiveness. Of the 11 papers reporting peer educator outcomes, 4/11 (36.4%) showed evidence of effectiveness, 2/11 (18.2%) showed mixed findings and 5/11 (45.5%) showed limited or no evidence of effectiveness. Study quality varied greatly with many studies rated as poor quality, mainly due to unrepresentative samples and incomplete data.
School-based peer education interventions are implemented worldwide and span a wide range of health areas. A number of interventions appear to demonstrate evidence for effectiveness, suggesting peer education may be a promising strategy for health improvement in schools. Improvement in health-related knowledge was most common with less evidence for positive health behaviour change. In order to quantitatively synthesise the evidence and make more confident conclusions, there is a need for more robust, high-quality evaluations of peer-led interventions using standardised health knowledge and behaviour measures.
Peer Review reports
Ensuring good health and wellbeing amongst school-aged children is a global public health priority and the contribution schools can make to this goal is increasingly recognised [ 1 ]. Worldwide, we have seen a rise in peer education interventions over recent decades [ 2 ]. For example, a survey in England revealed that 62% of primary and secondary schools had offered a peer-led intervention in 2009 [ 3 ]. Peer-led interventions within school settings are popular for many reasons, including the important role peers play within the lives of young people, a perception that this approach involves relatively few resources, and the more even balance of authority than in teacher-led lessons [ 4 ]. The use of peer educators for health improvement has also been linked with the importance of peer influence in adolescence [ 5 ]. This is a time of increased social development and peer attachments are central to young people’s development, particularly during adolescence [ 5 , 6 ]. Further, there is evidence that young people are more likely to seek help from informal sources of support such as friends in comparison to adults [ 7 ], and of older students being perceived as role models by their younger peers [ 8 ]. Benefits are also likely to exist for peer educators themselves, including opportunities to develop confidence and leadership skills, as well as many schools rewarding peer educators with a qualification or endorsement for their participation [ 9 ].
Existing peer education interventions cover a wide range of health areas, including mental health, physical health, sexual health, and a general promotion of healthy lifestyles including eating habits and smoking prevention [ 10 , 11 , 12 , 13 ]. There is also variation in the format or delivery of peer-led interventions including 1:1 peer mentoring, peer buddy initiatives, peer counselling, and peer education [ 14 , 15 , 16 , 17 ]. This review focuses specifically on peer education, which typically involves the selection and training of ‘peer educators’ or ‘leaders’, who subsequently relay health related information or skills to younger or similar aged students in their school, known as ‘peer learners’ or ‘recipients’.
Summary of related reviews
The current literature on peer education indicates a mixed evidence base regarding its effectiveness.
Ten previous reviews were found concerning health-related peer education among young people [ 10 , 12 , 18 , 19 , 20 , 21 , 22 , 23 , 24 ]. Of these, six concerned sexual health/HIV prevention, two concerned health promotion/education more broadly, one focused on substance abuse and one focused on mental health.
Kim and Free’s review concerning sexual health [ 21 ] found no overall effect of peer education on condom use, mixed findings on sexually transmitted infection (STI) prevention, and positive findings regarding improvements in knowledge, attitudes and intentions. Siddiqui et al. [ 20 ] reviewed peer education programmes for promoting the sexual and reproductive health of young people in India, revealing large variations in the way peer education is implemented as well as mixed effectiveness findings and limited effects of behaviour relative to knowledge. Maticka-Tyndale and Barnet [ 22 ] compiled a review into peer-led interventions to reduce HIV risk among youth using a narrative synthesis, and found that peer interventions led to positive change in knowledge and condom use, and had some success in changing community attitudes and norms, but no significant findings for effects on other sexual behaviours and STI rates. By comparison, Tolli’s review [ 12 ] regarding the effectiveness of peer education interventions for HIV prevention found no clear evidence of peer education effectiveness for HIV prevention, adolescent pregnancy prevention or sexual health promotion in young people of member countries of the European Union.
Mellanby et al. [ 23 ] reviewed the literature comparing peer-led and adult-led school health education and identified eleven studies. Seven of these studies found peer-led to be more effective for health behaviour change than adult-led and three of these studies found peer-led to me more effective for change in knowledge and attitudes. Harden et al. [ 24 ] identified 64 peer-delivered health interventions for young people aged 11 to 24 in any setting (i.e. not restricted to school settings), with only 12 evaluations judged to be methodologically sound. Of these 12, 7 studies (58%) showed a positive effect on at least one behavioural outcome. This review concluded an unclear evidence base for peer-delivered health promotion for young people.
MacArthur et al’s [ 19 ] investigation of peer-led interventions to prevent tobacco, alcohol and/or drug use among young people aged 11–21, comprised a meta-analysis, pooling 10 studies on tobacco use, and found lower prevalence of smoking among those receiving the peer-led interventions compared with controls. The authors also found that peer-led interventions were associated with benefit in relation to alcohol use, and three studies suggested an association with lower odds of cannabis use.
A recent systematic review by King and Fazel of 11 school-based peer-led mental health interventions studies revealed mixed effectiveness [ 10 ]. Some studies showed significant improvements in peer educator self-esteem and social stress [ 25 ], but one study showed an increase in guilt in peer educators [ 26 ]. Two studies also found improvements in self-confidence [ 27 ], and quality of life in peer learners [ 28 ], but one study found an increase in learning stress and decrease in overall mental health scores [ 26 ]. The review concluded there is better evidence if benefits for peer educators compared to peer learners. The summary above of previous systematic assessments of the peer education approach reveals a limited evidence base for school-based peer education interventions. Only two reviews were included regarding school-based peer education, one of which occurred over 20 years ago [ 23 ], while the other [ 10 ] was more narrowly concerned with mental health outcomes.
Despite the widespread use of peer-led interventions, the evidence base across all health areas still remains limited and little is known regarding their overall effectiveness in terms of changing behaviours or increasing health-related knowledge and/or attitudes. Due to the limited evidence base of peer education interventions, this review is broad in scope and will cover global peer education interventions covering all health areas. Although some peer education interventions are targeted towards specific populations, this review focuses on universal interventions available to an entire cohort of students (for example whole class or whole year group). The review aims to summarise the effectiveness of existing peer-led health interventions in schools. This is a review of quantitative data; the qualitative peer education literature will be published in a separate review.
We followed the PICO (Population, Intervention, Comparator and Outcome) format to develop our research question. We completed the systematic review in accordance with the 2009 PRISMA statement [ 29 ] and registered it with PROSPERO (CRD42021229192).
Search strategy and selection criteria
Five electronic databases were searched for eligible studies: CINAHL, Embase, ERIC, MEDLINE and PsycINFO. The list of search terms (see Supplementary Materials ) were developed after scanning relevant literature for key terms. Searches took place during October 2020.
Once the search terms had been agreed amongst the study team, pilot searches were run to check that key texts were appearing. Search terms were subsequently refined and this process was repeated until all key texts appeared. Search strategies such as truncations were used to maximise results. No restrictions were placed on publication date, country or language.
Inclusion/exclusion criteria
To be included studies had to be concerned with school-based peer education interventions designed to address aspects of the health of pupils aged 11–18 years old. We are interested in this age group in particular as it is a period when peers take on a particularly important role in young people’s lives. Peer education interventions concerned with health are defined here as interventions in which school-aged children deliver the education of other pupils for the purposes of improving health outcomes or awareness/literacy relating to health, including knowledge, behaviours and attitudes. Interventions must have taken place within a school, during school hours and must be universal, i.e. not targeted towards a specific sub-group of students or students with a particular health condition.
Where comparators/controls existed, they had to include non-exposure to the interventions concerned, exposure to a differing version of the same intervention, or exposure to the intervention within a substantially differing context.
Papers were excluded from data synthesis if they satisfied any of the following criteria:
Peer education interventions only concerned academic outcomes (e.g., reading and writing achievement).
Interventions concerning anger management, behavioural problems, or social skills.
Interventions concerning traffic safety, health and safety, avoidance of injuries, or first aid.
Interventions concerning cultural, social or political awareness (e.g., media literacy).
Interventions in which health outcomes are secondary to other outcomes (e.g., interventions focused on reading that indirectly improve self-esteem).
One-to-one mentoring interventions.
Conference abstracts, research briefings, commentaries, editorials, study protocol papers and pre-prints.
Primary outcome(s)
Improvements in health, including health awareness and understanding as indicated by responses to questionnaires.
Reductions in health problems or risk-taking behaviours.
These outcomes may concern the peer educators and/or peer learners.
Data extraction, selection and coding
Two reviewers independently screened all papers according to the inclusion criteria above using the Rayyan online review platform. In cases where the reviewers were uncertain, or where the decision was disputed, the decision was discussed and agreed among the wider research team. Two reviewers (SD and EW) then divided the papers between them and independently extracted the data, discussing and queries that arose with each other and the wider team.
Data extraction included the following:
Bibliographic details – authors, year of publication, nation in which intervention was carried out
Aims of the study
Description of study design
Sample size and demographic characteristics.
Context into which the intervention is introduced (characteristics of the school involved, the area in which the school is located, characterisations of the student body, relevant policy considerations).
Description of intervention (including duration of intervention).
Outcome measures (measurement tools, time points of data collection).
Data concerning improvements in health.
Quality appraisal
We used the Mixed Methods Appraisal Tool (MMAT) to assess quality of reporting procedures. This tool consists of five specific quality rating items depending on study design (qualitative, quantitative randomized, quantitative non-randomized, quantitative descriptive and quantitative mixed methods). There are 5 quality questions specific to each study design, so all papers are rated between 0 to 5. The following ratings were used to summarise study quality; 0–1 indicating poor quality, 2–3 indicating average quality and 4–5 indicating high quality. Two reviewers (SD and EW) completed quality ratings on each paper and discussed any discrepancies between them.
Examples of randomized design quality questions included items such as: “ Is randomization appropriately performed ? And “ Are the groups comparable at baseline ?” Examples of non-randomized design quality questions included items such as: “ Are the participants representative of the target population?” and “Are there complete outcome data?”
Effectiveness summary
EW and SD completed data synthesis. Due to the volume of studies, and the large number and heterogeneity of outcome measures, in order to summarise effectiveness, we created the following scoring system to indicate effectiveness:
Significant effects are effects where there was an improvement in health-related outcomes either after the peer education intervention, or when compared to a control group, with a p value of <0.05. Due to the volume of studies and varied follow-up periods, we looked at effectiveness at first follow-up, which in the majority of papers was immediately post-intervention.
A total of 2125 articles were identified after the initial search and 73 articles were eligible for inclusion (see Fig. 1 for a flow diagram of the search). Study designs of the 73 articles were as follows: 23 were controlled trial designs (15 cluster or group randomised, 6 randomised controlled and 2 non-randomised). 15 used randomisation methods but were not controlled trials and the remaining 35 studies used uncontrolled non-randomised methods comparing intervention with a comparison group or using a pre-post survey.
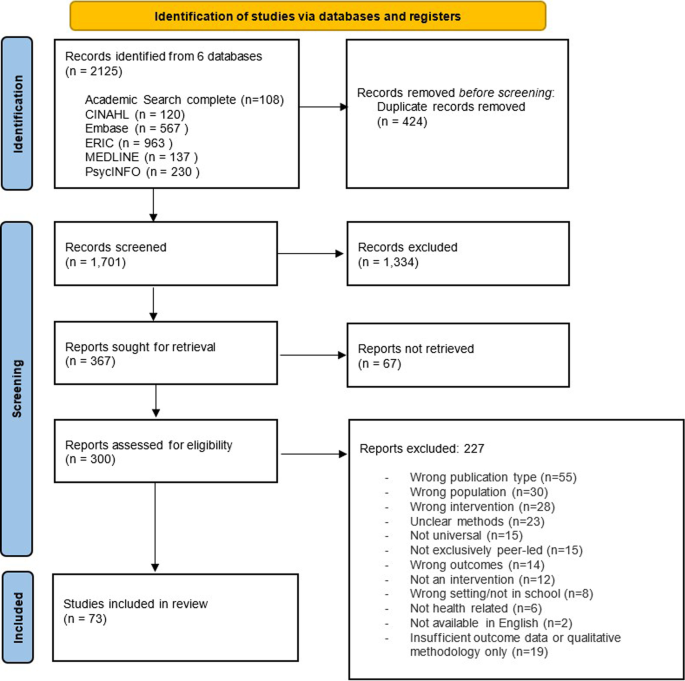
Prisma flow diagram of included studies
Health and geographical areas
The 73 quantitative papers included in this review demonstrated a wide range of health areas. The majority of papers evaluated interventions aimed at sex education/HIV prevention ( n = 23), promoting healthy lifestyles ( n = 17) and reducing alcohol, smoking and substance use ( n = 16). Fig. 2 illustrates number of papers per health area by peer learner or peer educator outcome focus and Table 2 illustrates a summary of proportion of health areas, overall effectiveness and quality ratings.
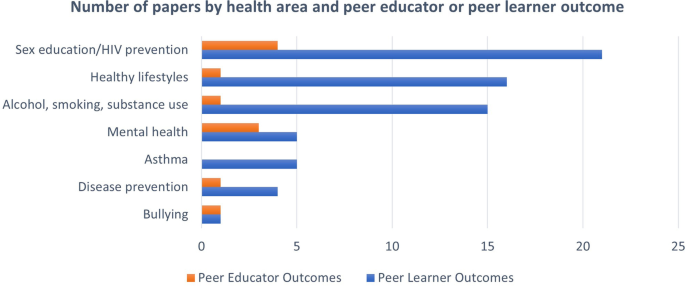
Number of papers by health area. NB See Supplementary Materials for full description of study designs and outcomes
Papers mainly focussed on peer learner outcomes (67/73, 91.8%), with only six papers (8.2%) focussing solely on peer educator outcomes and only five papers (6.8%) reporting on both peer learner and peer educator outcomes. The majority of papers that focussed on peer educator outcomes were those concerned with sex education (n = 4) and mental health (n = 3).
Papers typically reported knowledge, attitude and/or behavioural outcomes. Of the 73 papers, 42/73 (57.5%) reported knowledge outcomes, 43/73 (58.9%) reported attitude outcomes, 35/73 (47.9%) reported behavioural outcomes and 13/73 (17.8%) reported behavioural intentions.
As well as a broad range of health areas, the papers included in the review also spanned several different countries (Fig. 3 ).
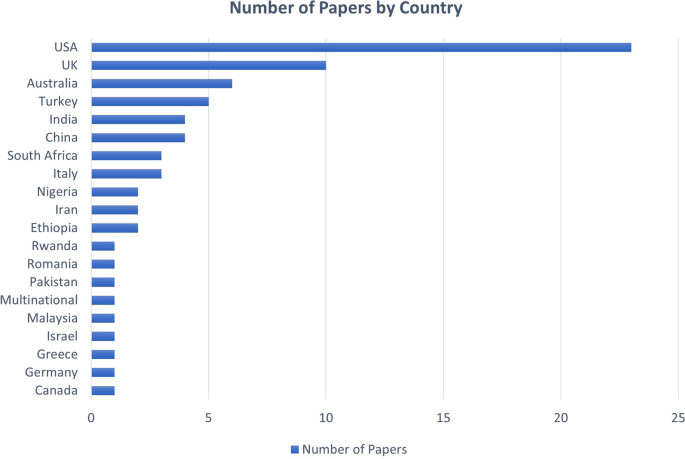
Summary of number of papers by country
We have summarised the results first by student type and then by health area.
Results by student type
Summary of peer learner outcomes.
Of the 67 papers reporting peer learner health outcomes, 35/67 (52.2%) showed evidence of effectiveness (as per our thresholds shown in Table 1 ), 8/67 (11.9%) showed mixed findings and 24/67 (35.8%) found limited or no evidence of effectiveness.
Of the 35 papers that demonstrated effectiveness, 9/35 studies (25.7%) were rated as high quality. Therefore only 9/67 (13.4%) of the total papers showed evidence of effectiveness and were rated as high quality.
Twenty-one papers (31.3%) reported controlled trial designs (including 14 cluster or group randomised, and 5 randomised controlled and 2 non-randomised). Thirteen papers used randomisation methods but were not controlled trials and the remaining 33 papers used uncontrolled non-randomised methods comparing intervention with a comparison group or using a pre-post survey design.
Summary of peer educator outcomes
Of the 11 papers reporting on peer educator health outcomes, 4/11 (36.4%) showed evidence of effectiveness, 2/11 (18.1%) showed mixed findings and 5/11 (45.5%) showed limited or no evidence of effectiveness. Of the 4 papers showing evidence for effectiveness, 2 studies (50%) were rated as high quality.
Four papers had a randomised design comparing intervention vs. control or ‘peer educators vs. classmates’ one of which was a cluster randomised controlled trial. The remaining 7 papers used non-randomised intervention vs. control ( n = 2) or pre-post survey designs ( n = 5).
A full table of included studies, outcomes and effectiveness and quality ratings can be found in Supplementary Material 1 .

Results by health area
Sex education/hiv prevention.
Twenty-three studies concerned sex education/HIV prevention [ 30 , 31 , 32 , 33 , 34 , 35 , 36 , 37 , 38 , 39 , 40 , 41 , 42 , 43 , 44 , 45 , 46 , 47 , 48 , 49 , 50 , 51 , 52 ]. 9/23 studies had a randomised design with the 8 studies comparing peer-led to teacher-led or ‘lessons as usual’ and one study comparing peer-led with nurse-led. 14/23 involved non-randomised designs comparing intervention vs. control or a pre-post survey design. Studies covered a wide geographical range, among which there were 7 US studies, but also studies from Canada, UK, Africa, South Africa, Turkey and Greece.
Of the twenty-three papers, 21 reported peer learner outcomes, 4 papers reported peer educator outcomes, with 2 papers reporting on both peer educator and peer learner outcomes. The mean number of participants across the studies was 2033 (range: n = 106–9000).
8/23 (34.8%) of studies showed evidence of effectiveness, and all studies demonstrating effectiveness consisted of knowledge and attitude outcomes rather than behavioural change.
Only 4/23 studies were rated high in quality (two of which showed evidence of effectiveness), whilst the majority of studies were rated medium quality (15/23) and 4/23 rated as low quality.
Healthy lifestyles (exercise, nutrition, oral health, health information)
Seventeen studies reported interventions addressing healthy lifestyles [ 53 , 54 , 55 , 56 , 57 , 58 , 59 , 60 , 61 , 62 , 63 , 64 , 65 , 66 , 67 , 68 , 69 ]. Of these papers, ten used a randomised controlled trial design primarily comparing peer-led vs. teacher-led or ‘lessons as usual’, but two oral health papers also used a dentist-led condition. Seven papers used non-randomised research designs comparing intervention vs. control or a pre-post survey design.
The most common focus was nutrition and exercise, but interventions also covered oral health, accessing health information online and interventions taking a more general approach to health improvement. Regarding geographical spread, 5/17 papers reported interventions carried out in the USA, with Australia, China, India and UK represented by two papers per country.
Sixteen of the seventeen papers reported peer learner outcomes, and only one reported peer educator outcomes. The mean number of participants per intervention was 1245 (range: n = 76–4576).
7/17 papers in this health area were shown to be effective, 8/17 were found to be ineffective, and 2/17 showed mixed results. In other words, less than half (41.1%) showed evidence of effectiveness. Of the studies demonstrating effectiveness, the outcomes largely centred around knowledge and attitudes, but one study did demonstrate positive behaviour change [ 62 ].
Over half of the studies (9/17) were rated as high quality, 4/17 were rated medium quality and 4/17 low quality. Of the studies showing evidence for effectiveness, 4/7 (57.1%) were rated as high quality.
Alcohol, smoking, substance use
Sixteen papers were classified within the category of alcohol, smoking and substance use [ 70 , 71 , 72 , 73 , 74 , 75 , 76 , 77 , 78 , 79 , 80 , 81 , 82 , 83 , 84 , 85 ]. Ten of these papers had a randomised design (including 3 cluster randomised controlled trials) comparing peer-led (intervention) vs. teacher-led (control). Six papers were non-randomised and used either a pre-post survey design or intervention vs. control. The 16 papers varied in quality with six rated ‘high quality’, seven rated ‘medium quality’, and three rated ‘low quality’. Studies took place across more than 10 countries with one study being conducted internationally. The mean number of participants across all studies was 2165 (range: n = 105–10,730).
Fifteen papers evaluated the effect of the intervention on peer learner outcomes and only one paper evaluated the effect of the intervention on peer educator outcomes. 8/16 (50%) papers showed evidence of effectiveness. 2/16 (12.5%) papers showed mixed findings and 6/16 (37.5%) showed little to no evidence for effectiveness, including the peer educator outcome paper. Of the eight papers demonstrating evidence for effectiveness, only four (50%) were rated as high quality.
Of the studies demonstrating effectiveness, there was a combination of knowledge, attitude and behavioural outcomes, but more evidence for positive changes in knowledge and attitude.
Mental health and well-being
Six studies assessed mental health and well-being [ 27 , 86 , 87 , 88 , 89 , 90 ]. This category was inclusive of common mental health problems, self-harm and suicide prevention as well as broader topics such as self-esteem and social connectedness. Four of the six studies used non-randomised pre-post survey designs and two studies used randomised design, one of which was a cluster randomised controlled trial.
Of the six studies, 5/6 explored peer learner outcomes, 3/6 explored peer educator outcomes, 2 of which explored both peer learner and peer educator outcomes. The average sample size across the seven mental health studies was 1118 (range: n = 50–4128).
Study quality was mixed, with two studies rated as high quality, three medium quality and one low quality. Outcome measures largely consisted of knowledge and attitude questionnaires, help-seeking behaviour and help-seeking confidence as well as condition-specific measures including body satisfaction and self-report of emotional and behavioural difficulties.
The majority of mental health studies (5/6) were rated as showing evidence for effectiveness and one study was rated ineffective. Of the studies demonstrating effectiveness, only one reported positive behaviour change (help-seeking behaviours) and this behaviour changed was observed in peer educators as opposed to peer learners [ 86 ].
Disease prevention
Four studies assessed outcomes relating to disease prevention [ 91 , 92 , 93 , 94 ] which included hepatitis, tuberculosis, cervical cancer and blood borne diseases. All four studies focused on peer learner outcomes and one study also included peer educator outcomes. Three of the four studies were non-randomised pre-post survey designs and one study was randomised. The average sample size across the four studies was 2116 (range: 1265–2930).
Three out of the four studies (75%) showed evidence for effectiveness and one study showed mixed results. No studies were rated as high quality, three were rated medium and one was rated low.
Outcomes were largely knowledge or intention based. Studies showing effectiveness mostly related to knowledge, intentions and attitudes and one study did find a positive change in behaviour [ 93 ].
Five included studies assessed asthma interventions [ 95 , 96 , 97 , 98 , 99 ]. 4/5 of these were randomised trials and one study used a non-randomised pre-post survey design. Average sample size across all studies was 427 (range: n = 203–935). Three studies took place in Australia and two in the US. All papers evaluated the impact of the intervention on peer learner outcomes with none focussing on peer educator outcomes.
4/5 studies showed evidence for effectiveness with only one study showing no evidence for effectiveness. All studies were rated as medium quality. Measures ranged from asthma knowledge, quality of life, school absenteeism, asthma attacks at school and asthma tests. Effectiveness was largely observed for knowledge outcomes, there was less evidence for asthma attacks or symptoms.
Two studies conducted in Italy assessed bullying by evaluating the ‘NoTrap!’ anti-bullying intervention [ 100 , 101 ]. The first study rated as high quality, evaluated two independent trials and focussed on peer learner outcomes ( n = 622; n = 461). This study found significant reductions in victimization, bullying, cybervictimization and cyberbullying and was rated as high quality. The second study, rated as medium quality, focussed on peer educator outcomes ( n = 524) and used a non-randomised, pre-post survey design but overall, only showed some evidence of effectiveness amongst males in terms of reduced victimization and increased prosocial behaviour and social support. No evidence was found for effectiveness among females.
Peer education interventions to improve student health cover a wide variety of topics and are used globally. This review aimed to summarise the results from peer education health interventions in secondary school students (aged 11–18-years-old), which were universal (rather than targeted interventions of sub-groups of students) and carried out at school.
Due to the heterogeneity of findings, range of health areas, types of studies and diversity of outcome measurements used, it was not possible to perform a meta-analysis or formal data synthesis to assess effectiveness. However, some broad conclusions can be made. A number of interventions appear to demonstrate evidence for effectiveness which indicates that peer education interventions can be an important school-based intervention for health improvement. Asthma interventions appeared to be particularly effective. In terms of outcome measures, the strongest evidence was for a positive change in knowledge and attitude measures, but there was less evidence overall for health behaviour outcomes which supports previous findings [ 20 , 22 ].
Although many studies did demonstrate positive results, findings overall were very mixed and several studies were of poor quality. In addition to the shortcomings picked up on by our quality appraisal, many papers lacked methodological detail and clarity regarding the intervention procedure, particularly in regard to how peer educators were selected and trained, which seems to be an important factor in those studies that found positive results and was also emphasised in a previous review [ 10 ]. Further, there were widespread problems of data reporting including noting ‘significant’ results without providing any measure of effect size or between-study variability. Other problems included selective reporting of results, such as selective emphasis on anomalous positive results, or only revealing measures of statistical significance in the case of positive effects. Interestingly, there did not appear to be a relationship between study quality and findings, given that several studies rated as effective were rated both high and low quality with a similar picture for studies showing mixed effectiveness and ineffectiveness.
In terms of frequency of health areas covered, our findings are similar to a recent ‘review of reviews’ of peer education for health and wellbeing which found that the majority of reviews focused on sexual health and HIV/AIDS interventions [ 13 ]. This previous review focused on both children and adults, however, in line with our findings, it found mixed effectiveness and considerable diversity in methods, findings and rigour of evaluation. It was particularly noted that details of peer educator training were rarely provided in HIV/AIDS interventions which supports our findings. Notably, however, the quality of studies was actually highest for peer education programs in HIV/AIDS, which differed to our review which found few studies rated as high quality. This discrepancy may be due to the different measures used to assess quality. Like our study, this review concluded that each health area showed some promising results, but also pointed to a need for higher levels of quality and rigour in future evaluations.
Despite the rising prevalence in mental health difficulties, there were relatively few studies focused on mental health outcomes, particularly more general preventative approaches to mental health and well-being, with many of the included studies focusing on suicide prevention, self-harm or specific disorders. However, many of mental health studies included in this review showed evidence for effectiveness, suggesting peer education approaches for mental health should be further studied and evaluated.
Another key finding of our review is that papers tended to focus more on peer learner outcomes and therefore impacts of peer-led interventions on peer educators themselves appear to be under-explored. This has been reported by previous reviews [ 10 ] and highlights the importance of examining and comparing both peer educators’ and learners’ outcomes within studies. In this context, we found more evidence of peer learners benefitting from the interventions, with 55.2% of studies showing a positive effect, versus only 36.4% for peer educators. This contrasted with a previous review of mental health interventions that concluded peer educators seemed to yield more benefits from participating in the interventions, possibly due to the attention they are given during training and throughout the programmes [ 10 ].
Although common measures existed across studies, including health knowledge, health intentions, and health behaviours, many studies used novel or unvalidated measurements, indicating a need for more standardised health literacy measures and a need for future validation work in this area. This supports two systematic reviews carried out in 2015, firstly a review of health literacy measures which found a lack of comprehensive instruments to measure health literacy and suggested the need for the development of new instruments [ 102 ], and secondly a review of mental health literacy measures which found a number of unvalidated measures and lack of measures that measured all components of mental health literacy concurrently [ 103 ].
Although there are a number of existing reviews summarising the extent to which peer education may improve young peoples health, the literature is still lacking on why peer education is effective within the quantitative literature. It remains unclear which mechanisms involved in peer education lead to its effectiveness (or ineffectiveness). Although many peer education studies are grounded in theory such as Diffusion of Innovation Theory [ 104 ] and Bandura’s Social Cognitive/Social Learning Theory [ 105 , 106 ], the literature is lacking a more nuanced analysis of the mechanisms through which peer education improve young people’s health. This is therefore a key area for future research.
A recent review of peer education and peer counselling for health and well-being highlights how peer education interventions are inherently difficult to quality control and evaluate [ 13 ], partly due to what makes peer education attractive; peer education defies the conventions of traditional formal education and allows young people to learn by more unstructured means, in more ‘real world’ ways, benefiting from meaningful examples and conversations with their peers. Although there are an increasing number of well-designed peer education studies [ 13 ], new evaluation methods may be needed given the complexity and multi-component nature of peer-education approaches (i.e., training, more informal teaching approaches and informal diffusion of knowledge).
Limitations
Despite our review being comprehensive, we acknowledge certain limitations. ‘Peer education’ is a complex and widely contested term and therefore how studies described their approach varied substantially. This may have meant some relevant studies were not picked up from our initial search. A previous review [ 10 ] also noted this potential limitation, with unclear and heterogeneous methods precluding meta-analysis. Therefore, a consensus on how to define ‘peer education’ and using standardised measures to assess effectiveness would facilitate more definitive synthesis of the evidence. Another potential limitation of our approach is that we only searched scientific databases, and therefore could have missed important evidence in the grey literature as we retrieved a relatively small number of initial records ( n = 2125). Despite this, given the wide variety of study type, age range, health area and country reviewed, this suggests our search strategy was fairly robust, and yielded results that were representative of the breadth in the current literature base.
This review focussed on universal peer education interventions delivered within the secondary school setting during school hours. Further research could explore the effectiveness of varying forms of peer education including 1:1 mentoring, more targeted (not universal) interventions, as well as peer education interventions in other settings including youth clubs or community and local organisations.
Due to the breadth of this review, we did not conduct a detailed comparison between knowledge, attitude and behavioural outcomes, however the studies demonstrating effectiveness tended to show positive change on knowledge and attitude outcomes, but less evidence was seen for positive behavioural change. This is in line with previous reviews which have suggested that peer education better improves health knowledge but often does not lead to behavioural gains [ 13 , 107 ]. To this vein, it remains unclear the differential impact on behavioural intention and actual performance of behaviour, and therefore we urge future researchers to measure outcomes relating to knowledge and attitude, intentions, and actual behaviour in order to synthesise the evidence in a more standardised way. Although the literature is heterogeneous, there is available data to conduct distinct analysis on different outcome measures (knowledge, attitude and behaviour) to create a more nuanced understanding of each health area.
Given the large number of studies and variation in outcome measures (behaviour, knowledge, attitude), this review focussed on findings at first follow-up (usually immediately after intervention) and therefore the effectiveness findings are not likely to represent longer-term effects of peer education interventions, which would require further research. In addition, due to the low number of optimally designed randomised-controlled trials identified, our review could not meaningfully compare results between randomised and non-randomised studies. However, as more high quality trials continue to be published in this growing area of research, a future review could be conducted that looks into the effect of randomisation on young people’s outcomes. Our results also focused on p-values rather than effect sizes due to the large variability in how and what studies measures, future researchers should aim to agree on more standardises ways of measuring outcomes to enable better synthesis.
To conclude, school-based peer education interventions occur worldwide and span a number of health areas. A number of interventions appear to demonstrate evidence for effectiveness, suggesting peer education may be a promising strategy for health improvement in schools. However overall evidence for effectiveness and study quality are mixed. Improvement in health-related knowledge was most common with less evidence for positive health behaviour change. In order to synthesise the evidence and make more confident conclusions, it is imperative that more robust, high-quality evaluations of peer-led interventions are conducted and that studies follow reporting guidelines to describe their methods and results in sufficient detail so that meta-analyses can be conducted. In addition, further research is needed to develop understanding of the intervention mechanisms that lead to health improvement in peer education approaches as well as more focussed work on standardising and validating health literacy and behaviour measurement tools.
Pre-registration
This review was pre-registered on PROSPERO: CRD42021229192. One deviation was made from the original protocol which was the use of a different quality appraisal tool. Initially we had planned to use the Canadian Effective Public Health Project Practice (EPHPP) Quality Assessment Tool for Quantitative Studies and the Critical Appraisals Skills Programme (CASP) checklist for qualitative studies. The authors instead used a combined mixed methods tool (the Mixed Methods Appraisal Tool; MMAT) for both quantitative and qualitative studies. This was due to the large volume and variation of studies which meant there were benefits to using a single brief quality check tool across all included studies, allowing us to standardise scores across study types. The qualitative studies will be discussed in a separate realist review on key mechanisms of peer education interventions.
Availability of data and materials
All data generated or analysed during this study are included in this published article and its supplementary information files.
Fazel M, Hoagwood K, Stephan S, Ford T. Mental health interventions in schools 1: Mental health interventions in schools in high-income countries. Lancet Psychiatry. 2014;1(5):377–87.
Article PubMed PubMed Central Google Scholar
McKeganey SP, Neil. The rise and rise of peer education approaches. Drugs. 2000;7(3):293–310.
Google Scholar
Houlston C, Smith PK, Jessel J. Investigating the extent and use of peer support initiatives in English schools. Educ Psychol. 2009;29(3):325–44.
Article Google Scholar
Winterton CI, Dunk RD, Wiles JR. Peer-led team learning for introductory biology: relationships between peer-leader relatability, perceived role model status, and the potential influences of these variables on student learning gains. Discipli Interdisciplin Sci Educ Res. 2020;2(1):1–9.
Blakemore SJ, Robbins TW. Decision-making in the adolescent brain. Nat Neurosci. 2012;15(9):1184–91.
Article CAS PubMed Google Scholar
Lam CB, McHale SM, Crouter AC. Time with peers from middle childhood to late adolescence: developmental course and adjustment correlates. Child Dev. 2014;85(4):1677–93.
NHS Digital. Mental Health of Children and Young People in England, 2020: Wave 1 follow up to the 2017 Survey. England: Health and Social Care Information Centre; 2020. Available online: https://digital.nhs.uk/data-and-information/publications/statistical/mental-health-of-children-and-young-people-in-england/2020-wave-1-follow-up# . Accessed 01 Aug 2022.
Johnson EC, Robbins BA, Loui M. What do students experience as peer leaders of learning teams? What Do Students Experience as Peer Leaders of Learning Teams? 2015.
Morgan D, Robbins J, Tripp J. Celebrating the Achievements of Sex and Relationship Peer Educators: The Development of an Assessment Process. Sex Educ. 2004;4(2):167–83.
King T, Fazel M. Examining the mental health outcomes of school-based peer-led interventions on young people: A scoping review of range and a systematic review of effectiveness. PLoS One. 2021;16(4):e0249553.
Article CAS PubMed PubMed Central Google Scholar
Boyle J, Mattern CO, Lassiter JW, Ritzler JA. Peer 2 peer: Efficacy of a course-based peer education intervention to increase physical activity among college students. J Am Coll Heal. 2011;59(6):519–29.
Tolli MV. Effectiveness of peer education interventions for HIV prevention, adolescent pregnancy prevention and sexual health promotion for young people: a systematic review of European studies. Health Educ Res. 2012;27(5):904–13.
Topping KJ. Peer Education and Peer Counselling for Health and Well-Being: A Review of Reviews. Int J Environ Res Public Health. 2022;19(10):6064.
Dennison S. Peer mentoring: Untapped potential. J Nurs Educ. 2010;49(6):340–2.
Article PubMed Google Scholar
Thalluri J, O'Flaherty JA, Shepherd PL. Classmate peer-coaching:" A Study Buddy Support scheme". J Peer Learn. 2014;7(1):92–104.
Boulton MJ. School peer counselling for bullying services as a source of social support: a study with secondary school pupils. Bri J Guidance Counsel. 2005;33(4):485–94.
Abdi F, Simbar M. The peer education approach in adolescents-narrative review article. Iran J Public Health. 2013;42(11):1200.
PubMed PubMed Central Google Scholar
Mahat G, Scoloveno MA. Effectiveness of adolescent peer education programs on reducing HIV/STI risk: an integrated review. Res Theory Nurs Pract. 2018;32(2):168–98.
MacArthur GJ, Harrison S, Caldwell DM, Hickman M, Campbell R. Peer-led interventions to prevent tobacco, alcohol and/or drug use among young people aged 11–21 years: a systematic review and meta-analysis. Addiction. 2016;111(3):391–407.
Siddiqui M, Kataria I, Watson K, Chandra-Mouli V. A systematic review of the evidence on peer education programmes for promoting the sexual and reproductive health of young people in India. Sex Reprod Health Matters. 2020;28(1):1741494.
Kim CR, Free C. Recent evaluations of the peer-led approach in adolescent sexual health education: A systematic review. Perspect Sex Reprod Health. 2008;40(3):144–51.
Maticka-Tyndale E, Barnett JP. Peer-led interventions to reduce HIV risk of youth: a review. Eval Program Plann. 2010;33(2):98–112.
Mellanby AR, Rees JB, Tripp JH. Peer-led and adult-led school health education: a critical review of available comparative research. Health Educ Res. 2000;15(5):533–45.
Harden A, Oakley A, Oliver S. Peer-delivered health promotion for young people: a systematic review of different study designs. Health Educ J. 2001;60(4):339–53.
Yogev A, Ronen R. Cross-age tutoring: Effects on tutors’ attributes. J Educ Res. 1982;75(5):261–8.
Song Y, Loewenstein G, Shi Y. Heterogeneous effects of peer tutoring: Evidence from rural Chinese middle schools. Res Econ. 2018;72(1):33–48.
Ellis LA, Marsh HW, Craven RG. Addressing the challenges faced by early adolescents: a mixed-method evaluation of the benefits of peer support. Am J Community Psychol. 2009;44(1–2):54–75.
Shah S, McCallum GB, Wilson C, Saunders J, Chang AB. Feasibility of a peer-led asthma and smoking prevention program (ASPP) in australian schools with high indigenous youth. Respirology. 2017;22:35.
Moher D, Shamseer L, Clarke M, Ghersi D, Liberati A, Petticrew M, et al. Preferred reporting items for systematic review and meta-analysis protocols (PRISMA-P) 2015 statement. Syst Rev. 2015;4(1):1.
Parwej S, Kumar R, Walia I, Aggarwal AK. Reproductive health education intervention trial. Indian J Pediatr. 2005;72(4):287–91.
Rotz D, Goesling B, Manlove J, Welti K, Trenholm C. Impacts of a School-Wide, Peer-Led Approach to Sexuality Education: A Matched Comparison Group Design. J Sch Health. 2018;88(8):549–59.
Mellanby AR, Newcombe RG, Rees J, Tripp JH. A comparative study of peer-led and adult-led school sex education. Health Educ Res. 2001;16(4):481–92.
Timol F, Vawda MY, Bhana A, Moolman B, Makoae M, Swartz S. Addressing adolescents' risk and protective factors related to risky behaviours: Findings from a school-based peer-education evaluation in the Western Cape. SAHARA J. 2016;13(1):197–207.
Aten MJ, Siegel DM, Enaharo M, Auinger P. Keeping middle school students abstinent: outcomes of a primary prevention intervention. J Adolesc Health. 2002;31(1):70–8.
Caron F, Godin G, Otis J, Lambert LD. Evaluation of a theoretically based AIDS/STD peer education program on postponing sexual intercourse and on condom use among adolescents attending high school. Health Educ Res. 2004;19(2):185–97.
Ebreo A, Feist-Price S, Siewe Y, Zimmerman RS. Effects of peer education on the peer educators in a school-based HIV prevention program: where should peer education research go from here?...including commentary by Main DS. Health Educ Behav. 2002;29(4):411–24.
Mason-Jones AJ, Flisher AJ, Mathews C. Who are the peer educators? HIV prevention in South African schools. Health Educ Res. 2011;26(3):563–71.
Menna T, Ali A, Worku A. Effects of peer education intervention on HIV/AIDS related sexual behaviors of secondary school students in Addis Ababa, Ethiopia: a quasi-experimental study. Reprod Health. 2015;12(1):84.
Siegel DM, Aten MJ, Roghmann KJ, Enaharo M. Early effects of a school-based human immunodeficiency virus infection and sexual risk prevention intervention. Arch Pediatr Adolesc Med. 1998;152(10):961–70.
Siegel DM, Aten MJ, Enaharo M. Long-term effects of a middle school- and high school-based human immunodeficiency virus sexual risk prevention intervention. Arch Pediatr Adolesc Med. 2001;155(10):1117–26.
Stephenson JM, Strange V, Forrest S, Oakley A, Copas A, Allen E, et al. Pupil-led sex education in England (RIPPLE study): cluster-randomised intervention trial. Lancet. 2004;364(9431):338–46.
Stephenson J, Strange V, Allen E, Copas A, Johnson A, Bonell C, et al. The long-term effects of a peer-led sex education programme (RIPPLE): a cluster randomised trial in schools in England. PLoS Med. 2008;5(11):224 discussion e.
Strange V, Forrest S, Oakley A. What influences peer-led sex education in the classroom? A view from the peer educators. Health Educ Res. 2002;17(3):339–49.
Borgia P, Marinacci C, Schifano P, Perucci CA. Is peer education the best approach for HIV prevention in schools? Findings from a randomized controlled trial. J Adolesc Health. 2005;36(6):508–16.
Fisher JD, Fisher WA, Bryan AD, Misovich SJ. Information-motivation-behavioral skills model-based HIV risk behavior change intervention for inner-city high school youth. Health Psychol. 2002;21(2):177–86.
Huang H, Ye X, Cai Y, Shen L, Xu G, Shi R, et al. Study on peer-led school-based HIV/AIDS prevention among youths in a medium-sized city in China. Int J STD AIDS. 2008;19(5):342–6.
Mahat G, Scoloveno MA. HIV peer education: Relationships between adolescents' HIV/AIDS knowledge and self-efficacy. J HIV AIDS Soc Serv. 2010;9(4):371–84.
Merakou K, Kourea-Kremastinou J. Peer education in HIV prevention: an evaluation in schools. Eur J Pub Health. 2006;16(2):128–32.
Michielsen K, Beauclair R, Delva W, Roelens K, Van Rossem R, Temmerman M. Effectiveness of a peer-led HIV prevention intervention in secondary schools in Rwanda: results from a non-randomized controlled trial. BMC Public Health. 2012;12(1):729.
Ozcebe H, Akin L, Aslan D. A peer education example on HIV/AIDS at a high school in Ankara. Turk J Pediatr. 2004;46(1):54–9.
PubMed Google Scholar
Visser MJ. HIV/AIDS prevention through peer education and support in secondary schools in South Africa. Sahara J. 2007;4(3):678–94.
Jennings JM, Howard S, Perotte CL. Effects of a school-based sexuality education program on peer educators: the Teen PEP model. Health Educ Res. 2014;29(2):319–29.
Cohen RY, Felix MR, Brownell KD. The role of parents and older peers in school-based cardiovascular prevention programs: implications for program development. Health Educ Q. 1989;16(2):245–53.
Lytle LA, Murray DM, Perry CL, Story M, Birnbaum AS, Kubik MY, et al. School-based approaches to affect adolescents' diets: results from the TEENS study. Health Educ Behav. 2004;31(2):270–87.
Shankar P, Sievers D, Sharma R. Evaluating the Impact of a School-Based Youth-Led Health Education Program for Adolescent Females in Mumbai, India. Ann Glob Health. 2020;86(1):57.
Forneris T, Fries E, Meyer A, Buzzard M, Uguy S, Ramakrishnan R, et al. Results of a rural school-based peer-led intervention for youth: goals for health. J Sch Health. 2010;80(2):57–65.
Foley BC, Shrewsbury VA, Hardy LL, Flood VM, Byth K, Shah S. Evaluation of a peer education program on student leaders' energy balance-related behaviors. BMC Public Health. 2017;17(1):695.
Cui Z, Shah S, Yan L, Pan Y, Gao A, Shi X, et al. Effect of a school-based peer education intervention on physical activity and sedentary behaviour in Chinese adolescents: a pilot study. BMJ Open. 2012;2(3):e000721.
Ishak SIZS, Siew CY, Mohd Shariff Z, Mun CY, Moh TN. Effectiveness of a school-based, peer-led intervention program on the adolescents' body composition, eating behaviors and health-related quality of life. Ann Nutr Metab. 2019;75(3):359.
Tamiru D, Argaw A, Gerbaba M, Ayana G, Nigussie A, Jisha H, et al. Enhancing Personal Hygiene Behavior and Competency of Elementary School Adolescents through Peer-Led Approach and School-Friendly: A Quasi-Experimental Study. Ethiop J Health Sci. 2017;27(3):245–54.
Bogart LM, Elliott MN, Cowgill BO, Klein DJ, Hawes-Dawson J, Uyeda K, et al. Two-Year BMI Outcomes From a School-Based Intervention for Nutrition and Exercise: A Randomized Trial. Pediatrics. 2016;137(5):e20152493.
Shrewsbury VA, Venchiarutti RL, Hardy LL, Foley BC, Bonnefin A, Byth K, et al. Impact and cost of the peer-led Students As LifeStyle Activists programme in high schools. Health Educ J. 2020;79(1):3–20.
Bell SL, Audrey S, Cooper AR, Noble S, Campbell R. Lessons from a peer-led obesity prevention programme in English schools. Health Promot Int. 2017;32(2):250–9.
Bogart LM, Cowgill BO, Elliott MN, Klein DJ, Hawes-Dawson J, Uyeda K, et al. A randomized controlled trial of students for nutrition and eXercise: a community-based participatory research study. J Adolesc Health. 2014;55(3):415–22.
Ajuwon GA, Ajuwon AJ. Teaching high school students to use online consumer health resources on mobile phones: outcome of a pilot project in Oyo State, Nigeria. J Med Lib Assoc. 2019;107(2):194–202.
Haleem A, Siddiqui MI, Khan AA. School-based strategies for oral health education of adolescents--a cluster randomized controlled trial. BMC Oral Health. 2012;12:54.
Vangipuram S, Jha A, Raju R, Bashyam M. Effectiveness of peer group and conventional method (Dentist) of oral health education programme among 12-15 year old school children - A randomized controlled trial. J Clin Diagn Res. 2016;10(5):ZC125–ZC9.
Sebire SJ, Jago R, Banfield K, Edwards MJ, Campbell R, Kipping R, et al. Results of a feasibility cluster randomised controlled trial of a peer-led school-based intervention to increase the physical activity of adolescent girls (PLAN-A). Int J Behav Nutr Phys Act. 2018;15(1):50.
Ping HU, Lingli HAN, Manoj S, Huan Z, Yong Z, Hui LI, et al. Evaluation of Cognitive and Behavioral Effects of Peer Education Model-Based Intervention to Sun Safe in Children. Iran J Public Health. 2014;43(3):300–9.
Perry CL, Grant M, Ernberg G, Florenzano RU, Langdon MC, Myeni AD, et al. WHO Collaborative Study on Alcohol Education and Young People: outcomes of a four-country pilot study. Int J Addict. 1989;24(12):1145–71.
Weichold K, Silbereisen RK. Peers and teachers as facilitators of the life skills program IPSY - Results from a pilot study. Sucht. 2012;58(4):247–58.
Lachausse RG. The effectiveness of a multimedia program to prevent fetal alcohol syndrome. Health Promot Pract. 2008;9(3):289–93.
Erhard R. Peer-led and adult-led programs--student perceptions. J Drug Educ. 1999;29(4):295–308.
Audrey S, Holliday J, Campbell R. It's good to talk: adolescent perspectives of an informal, peer-led intervention to reduce smoking. Soc Sci Med. 2006;63(2):320–34.
Bloor M, Frankland J, Langdon NP, Robinson M, Allerston S, Catherine A, et al. A controlled evaluation of an intensive, peer-led, schools-based, anti-smoking programme. Health Educ J. 1999;58(1):17–25.
Campbell R, Starkey F, Holliday J, Audrey S, Bloor M, Parry-Langdon N, et al. An informal school-based peer-led intervention for smoking prevention in adolescence (ASSIST): a cluster randomised trial. Lancet. 2008;371(9624):1595–602.
Lotrean LM, Dijk F, Mesters I, Ionut C, De Vries H. Evaluation of a peer-led smoking prevention programme for Romanian adolescents. Health Educ Res. 2010;25(5):803–14.
Mall ASK, Bhagyalaxmi A. An Informal School-based, Peer-led Intervention for Prevention of Tobacco Consumption in Adolescence: A Cluster Randomized Trial in Rural Gandhinagar. Indian J Community Med. 2017;42(3):143–6.
Mohammadi M, Ghaleiha A, Rahnama R. Effectiveness of a peer-led behavioral intervention program on tobacco use-related knowledge, attitude, normative beliefs, and intention to smoke among adolescents at Iranian Public High Schools. Int J Prev Med. 2019;10(1):260245.
Murray DM, Richards PS, Luepker RV, Johnson CA. The prevention of cigarette smoking in children: two- and three-year follow-up comparisons of four prevention strategies. J Behav Med. 1987;10(6):595–611.
Perry CL, et al. Peer Teaching and Smoking Prevention among Junior High Students. Adolescence. 1980;15(58):277–82.
CAS PubMed Google Scholar
Botvin GJ, Baker E, Filazzola AD, Botvin EM. A cognitive-behavioral approach to substance abuse prevention: one-year follow-up. Addict Behav. 1990;15(1):47–63.
Demirezen D, Karaca A, Konuk Sener D, Ankarali H. Agents of change: the role of the peer education program in preventing adolescent substance abuse. J Child Adolesc Subst Abuse. 2019;28(5):376–87.
Severson HH, Glasgow R, Wirt R, Brozovsky P, Zoref L, Black C, et al. Preventing the use of smokeless tobacco and cigarettes by teens: results of a classroom intervention. Health Educ Res. 1991;6(1):109–20.
Aslan D, Sahin A. Adolescent peers and anti-smoking activities. Promot Educ. 2007;14(1):36–40.
Wyman PA, Brown CH, LoMurray M, Schmeelk-Cone K, Petrova M, Yu Q, et al. An outcome evaluation of the Sources of Strength suicide prevention program delivered by adolescent peer leaders in high schools. Am J Public Health. 2010;100(9):1653–61.
Ciao AC, Latner JD, Brown KE, Ebneter DS, Becker CB. Effectiveness of a peer-delivered dissonance-based program in reducing eating disorder risk factors in high school girls. Int J Eat Disord. 2015;48(6):779–84.
Eisenstein C, Zamperoni V, Humphrey N, Deighton J, Wolpert M, Rosan C, et al. Evaluating the peer education project in secondary schools. J Public Ment Health. 2019;18(2):58–65.
Kaveh MH, Hesampour M, Ghahremani L, Tabatabaee HR. The effects of a peer-led training program on female students' self-esteem in public secondary schools in Shiraz. J Adv Med Educ Prof. 2014;2(2):63–70.
Parikh SV, Taubman DS, Antoun C, Cranford J, Foster CE, Grambeau M, et al. The Michigan Peer-to-Peer Depression Awareness Program: School-Based Prevention to Address Depression Among Teens. Psychiatr Serv. 2018;69(4):487–91.
Isik M, Set T, Khan AS, Avsar UZ, Cansever Z, Acemoglu H. Prevalence of Blood Brotherhood among High School Students in Erzurum and the Effect of Peer-led Education on this Practice. Eurasian J Med. 2013;45(2):83–7.
Sadoh AE, Okonkwo C, Nwaneri DU, Ogboghodo BC, Eregie C, Oviawe O, et al. Effect of peer education on knowledge of human papilloma virus and cervical cancer among female adolescent students in Benin city, Nigeria. Ann Global Health. 2018;84(1):121–8.
Acemoglu H, Palanci Y, Set T, Vancelik S, Isik M, Polat H. An intervention study for viral hepatitis: Peer-led health education among high school students. Saudi Med J. 2011;32(2):183–7.
Liu Q, Liu L, Vu H, Liu X, Tang S, Wang H. Comparison between peer-led and teacher-led education in tuberculosis prevention in rural middle schools in Chongqing, China. Asia Pac J Public Health. 2015;27(2):NP2101–NP11.
Al-sheyab N, Gallagher R, Crisp J, Shah S. Peer-led education for adolescents with asthma in Jordan: a cluster-randomized controlled trial. Pediatrics. 2012;129(1):e106–12.
Al-sheyab NA, Alomari MA, Shah S, Gallagher R. "Class smoke-free" pledge impacts on nicotine dependence in male adolescents: A cluster randomized controlled trial. J Subst Abus. 2016;21(6):566–74.
Gibson PG, Shah S, Mamoon HA. Peer-led asthma education for adolescents: impact evaluation. J Adolesc Health. 1998;22(1):66–72.
McCallum GB, Chang AB, Wilson CA, Petsky HL, Saunders J, Pizzutto SJ, et al. Feasibility of a Peer-Led Asthma and Smoking Prevention Project in Australian Schools with High Indigenous Youth. Front Pediatr. 2017;5:33.
Shah S, Peat JK, Mazurski EJ, Wang H, Sindhusake D, Bruce C, et al. Effect of peer led programme for asthma education in adolescents: cluster randomised controlled trial. BMJ. 2001;322(7286):583–5.
Palladino BE, Nocentini A, Menesini E. Evidence-based intervention against bullying and cyberbullying: Evaluation of the NoTrap! program in two independent trials. Aggress Behav. 2016;42(2):194–206.
Zambuto V, Palladino BE, Nocentini A, Menesini E. Why do some students want to be actively involved as peer educators, while others do not? Findings from NoTrap! Anti-bullying and anti-cyberbullying program. Eur J Dev Psychol. 2019;16(4):373–86.
Tavousi M, Ebadi M, Fattahi E, Jahangiry L, Hashemi A, Hashemiparast M, et al. Health literacy measures: A systematic review of the literature. 2015.
Wei Y, McGrath PJ, Hayden J, Kutcher S. Mental health literacy measures evaluating knowledge, attitudes and help-seeking: a scoping review. BMC Psychiatry. 2015;15(1):291.
Kaminski J. Diffusion of innovation theory. Can J Nurs Inform. 2011;6(2):1–6.
Bandura A, Walters RH. Social learning theory: Englewood cliffs Prentice Hall; 1977.
Bandura A. The evolution of social cognitive theory. In: Smith KG, Hitt MA, editors. Great Minds in Management. Oxford: Oxford University Press; 2019. p. 9–35.
Milburn K. A critical review of peer education with young people with special reference to sexual health. Health Educ Res. 1995;10(4):407–20.
Download references
Acknowledgements
Not applicable.
This research study is funded by the National Institute for Health and Care Research (NIHR) School for Public Health Research (project number SPHR PHPES025). The views and opinions expressed in the paper are those of the authors and do not necessarily reflect those of the NIHR. The funding body played no role in the design, analysis, interpretation or writing of the manuscript.
Author information
Steven Dodd and Emily Widnall are joint first authors.
Authors and Affiliations
Faculty of Health and Medicine, Lancaster University, Lancaster, UK
Steven Dodd & Mark Limmer
Population Health Sciences, University of Bristol, Bristol, UK
Emily Widnall & Judi Kidger
College of Medicine and Health, University of Exeter, Exeter, UK
Abigail Emma Russell
Faculty of Epidemiology and Population Health, London School of Hygiene and Tropical Medicine, London, UK
Esther Louise Curtin
Mental Health Foundation, London, UK
Ruth Simmonds
You can also search for this author in PubMed Google Scholar
Contributions
All authors contributed to the design of the systematic review. SD led on designing the search strategy with input from all co-authors. SD carried out the initial searches across four databases. SD and EW led on retrieving papers and screening abstracts and full papers. EW and SD led on data extraction with support from AR. SD and EW drafted the initial manuscript. All co-authors reviewed the manuscript and approved the final version.
Corresponding author
Correspondence to Emily Widnall .
Ethics declarations
Ethics approval and consent to participate, consent for publication, competing interests.
None to declare.
Additional information
Publisher’s note.
Springer Nature remains neutral with regard to jurisdictional claims in published maps and institutional affiliations.
Supplementary Information
Additional file 1., rights and permissions.
Open Access This article is licensed under a Creative Commons Attribution 4.0 International License, which permits use, sharing, adaptation, distribution and reproduction in any medium or format, as long as you give appropriate credit to the original author(s) and the source, provide a link to the Creative Commons licence, and indicate if changes were made. The images or other third party material in this article are included in the article's Creative Commons licence, unless indicated otherwise in a credit line to the material. If material is not included in the article's Creative Commons licence and your intended use is not permitted by statutory regulation or exceeds the permitted use, you will need to obtain permission directly from the copyright holder. To view a copy of this licence, visit http://creativecommons.org/licenses/by/4.0/ . The Creative Commons Public Domain Dedication waiver ( http://creativecommons.org/publicdomain/zero/1.0/ ) applies to the data made available in this article, unless otherwise stated in a credit line to the data.
Reprints and permissions
About this article
Cite this article.
Dodd, S., Widnall, E., Russell, A.E. et al. School-based peer education interventions to improve health: a global systematic review of effectiveness. BMC Public Health 22 , 2247 (2022). https://doi.org/10.1186/s12889-022-14688-3
Download citation
Received : 09 August 2022
Accepted : 21 November 2022
Published : 02 December 2022
DOI : https://doi.org/10.1186/s12889-022-14688-3
Share this article
Anyone you share the following link with will be able to read this content:
Sorry, a shareable link is not currently available for this article.
Provided by the Springer Nature SharedIt content-sharing initiative
- Peer education
- School-based interventions
- Systematic review
- School health
- Adolescents
BMC Public Health
ISSN: 1471-2458
- General enquiries: [email protected]
- Open access
- Published: 23 May 2024
Interpretation of course conceptual structure and student self-efficacy: an integrated strategy of knowledge graphs with item response modeling
- Zhen-Yu Cao 1 ,
- Feng Lin 2 &
- Chun Feng 3 , 4
BMC Medical Education volume 24 , Article number: 563 ( 2024 ) Cite this article
111 Accesses
Metrics details
There is a scarcity of studies that quantitatively assess the difficulty and importance of knowledge points (KPs) depending on students’ self-efficacy for learning (SEL). This study aims to validate the practical application of psychological measurement tools in physical therapy education by analyzing student SEL and course conceptual structure.
From the “Therapeutic Exercise” course curriculum, we extracted 100 KPs and administered a difficulty rating questionnaire to 218 students post-final exam. The pipeline of the non-parametric Item Response Theory (IRT) and parametric IRT modeling was employed to estimate student SEL and describe the hierarchy of KPs in terms of item difficulty. Additionally, Gaussian Graphical Models with Non-Convex Penalties were deployed to create a Knowledge Graph (KG) and identify the main components. A visual analytics approach was then proposed to understand the correlation and difficulty level of KPs.
We identified 50 KPs to create the Mokken scale, which exhibited high reliability (Cronbach’s alpha = 0.9675) with no gender bias at the overall or at each item level ( p > 0.05 ). The three-parameter logistic model (3PLM) demonstrated good fitness with questionnaire data, whose Root Mean Square Error Approximation was < 0.05. Also, item-model fitness unveiled good fitness, as indicated by each item with non-significant p-values for chi-square tests. The Wright map revealed item difficulty relative to SEL levels. SEL estimated by the 3PLM correlated significantly with the high-ability range of average Grade-Point Average ( p < 0.05 ). The KG backbone structure consisted of 58 KPs, with 29 KPs overlapping with the Mokken scale. Visual analysis of the KG backbone structure revealed that the difficulty level of KPs in the IRT could not replace their position parameters in the KG.
The IRT and KG methods utilized in this study offer distinct perspectives for visualizing hierarchical relationships and correlations among the KPs. Based on real-world teaching empirical data, this study helps to provide a research foundation for updating course contents and customizing learning objectives.
Trial registration
Not applicable.
Peer Review reports
Introduction
Knowledge points (KPs) serve as fundamental units within the realm of learning content, encompassing theories, ideas, thoughts, etc [ 1 ]. Determining the importance and difficulty of KPs is crucial for effective curriculum development [ 2 ]. Experts typically identify key KPs and peripheral KPs aligned with learning objectives [ 3 ]. Key KPs, or important points, are the core concepts of course content. Additionally, KPs can be classified as either complex or simple, considering their respective levels of teaching difficulty. Complex KPs, or difficult points, are challenging for students to master and require more education time [ 4 ]. Apart from teaching proficiency, KPs are considered a relative concept contingent upon student abilities [ 5 ]. Students with advanced abilities may identify certain KPs as relatively easy, while those with weaker abilities might find them comparatively challenging [ 6 ]. As a psychological attribute, the student’s ability is considered a “latent trait”, and is generally an inherent and intricate individual characteristic that cannot be directly measured by instruments or equipment.
In the context of learning theory, latent traits can usually be divided into two types, namely learning capacity and self-efficacy. Learning capacity specifies the capacity that one will produce positive learning outcomes, which can be manifested by the Grade-Point Average (GPA) [ 7 ]. Self-efficacy measured by psychometric questionnaires like the Learning Self-Efficacy Scale for Clinical Skills (L-SES) [ 8 ], reflects the belief in one’s ability to learn effectively [ 9 ].
Recent research has underscored the connection between high self-efficacy for learning (SEL) and successful academic performance [ 9 , 10 , 11 , 12 ]. However, there was still a knowledge gap regarding the varying degrees of difficulty that each student may experience when dealing with specific KPs. The existing tools, like the L-SES with its 12-item scale [ 8 ], primarily assess SEL but do not concurrently measure the difficulty of KPs. This limitation hinders the understanding of students’ learning experiences, as it overlooks the varying degrees of difficulty associated with specific KPs.
To address this gap, this study applied the Item Response Theory (IRT), a theoretical framework for considering person ability, and item difficulty on the same scale (in units of logit). The corresponding test analysis method is item response modeling (IRM), which can quantify how individuals with different levels of the latent trait are likely to respond to specific items. IRT can be broadly categorized into two main types, namely, non-parametric IRT (npIRT) and parametric IRT (pIRT) [ 13 ]. Compared to pIRT with explicit assumptions, the npIRT is more flexible in handling data and makes fewer assumptions about the underlying structure of the item responses. The npIRT may focus on ranking items based on their discriminatory power without assuming specific functional forms. The analytical pipeline for the npIRT and the pIRT modeling has been previously validated [ 14 , 15 , 16 , 17 ] as a sufficient and reliable scaling method [ 18 ], which offers a promising approach to measuring both SEL and the difficulty of KPs.
In addition to investigating the difficulty of KPs in alignment with diverse student abilities, the main purpose of educational activities is to facilitate the construction of knowledge schemas. The construction process of knowledge schemas involves connecting new KPs with existing knowledge [ 19 ]. To effectively assimilate new knowledge, one prerequisite is the acquisition of enough foundational knowledge [ 20 ]. During the dynamic process of expanding and shaping knowledge schemas, certain KPs play a pivotal role by introducing other KPs connected to the overarching schema, which are referred to as necessary points [ 21 ]. Accordingly, KPs should be sorted sequentially to determine the priority of teaching content. The utilization of a knowledge graph (KG) [ 22 ] provides an opportunity for representing KPs as nodes and their relationships as connections. The knowledge graph model (KGM) is the corresponding technical approach to exploring knowledge schemas, enabling the quantitative calculation of the weight of KPs [ 23 ].
This study attempts to offer innovative teaching application methods and explore research directions by incorporating student self-evaluation difficulties of KPs, along with IRT and KGM techniques. Pinpointing the difficult points by the IRT addresses the concern of “which KPs demand additional teaching resources for enhanced comprehension” [ 5 ]. Determining the important points in the KG tackles the query of “which knowledge points are the indispensable core of this course” [ 2 ]. Uncovering the necessary points by the KGM resolves the issue of “which KPs need to be taught first” [ 24 ]. The ultimate goal is to customize teaching plans based on the implication of the difficulty and importance of KPs.
Data collection
This study was approved by the Committee for Ethics in Human Research at the Nanjing University of Chinese Medicine (NJUCM), with the issued number as No. 2021-LL-13(L).
A collaborative process involved a three-person voting method facilitated by three rehabilitation professors. These three rehabilitation professors jointly assessed and selected 100 KPs from the curriculum. Following the completion of the final exam, physical therapy students were engaged in the online questionnaire regarding the difficulty rating of KPs. This survey assessed the perceived difficulty of the 100 KPs based on a five-point Likert scale, where students indicated their perception on a scale ranging from 0 (very easy) to 4 (very difficult).
Statistical tools and methods
The data analysis process, as depicted in Fig. 1 , involved several R packages within the R software (Version 4.2.0) [ 25 ] to facilitate key steps. We used the mokken [ 26 ] and mirt [ 27 ] packages [ 25 ] to construct the IRM and parameter estimation. The ggstatsplot package [ 28 ] was operated for correlation analysis and visualization. The robustbase package [ 29 ] was employed to analyze the upper and lower bounds of skewed distributions. The GGMncv package [ 30 ] was conducted for network modeling, while the backbone package [ 31 ] interpreted the network skeleton structure. The igraph package [ 32 ] was exploited for network visualization and parameter analysis.
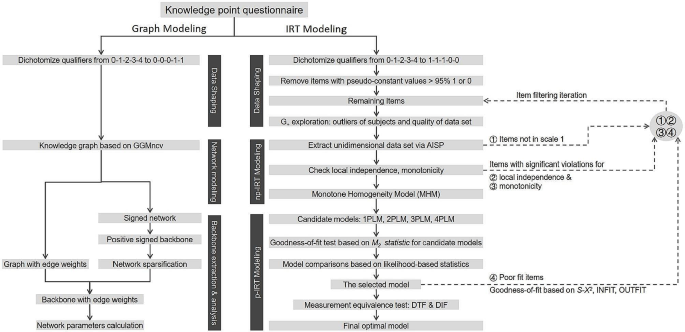
Data processing pipeline AISP: automatic item selection procedure; IRT: item response theory; np-IRT: non-parametric IRT; p-IRT: parametric IRT; 1PLM, 2PLM, 3PLM, and 4PLM: logistic item response model with 1 parameter, and 2, 3, and 4 parameters; INFIT: inlier-sensitive fit/information-weighted fit; OUTFIT: outlier-sensitive fit; S-X2: signed chi-squared test statistic; DTF: differential test functioning; DIF: differential item functioning
IRT modelling
Data transformation.
The difficulty rating scores for KPs were binarized from 0-1-2-3-4 to 1-1-1-0-0. Our study employed the “ascending assignment principle” or assigning by confidence. Under this scoring system, 0 indicated students had self-perceived difficulty mastering certain KPs, whereas 1 indicated students were confident that the knowledge point was easy to learn. A higher questionnaire score (total score of all items) corresponded to greater SEL.
To avoid ceiling and floor effects, the mastery ratio (proportion of KPs with a binary value of 1) and the unfamiliarity ratio (proportion of KPs with a binary value of 0) were calculated for each knowledge point. If KPs with a mastery rate or unfamiliarity rate greater than 95%, they are considered pseudo-constant.
Guttman errors were calculated after removing pseudo-constant KPs. The upper fence of the Guttman error distribution was calculated using the corrected box plot [ 29 ]. If Guttman errors exceed the upper fence, it is considered as extreme response bias and would be eliminated. The IRT modeling analysis was conducted as follows. The SEL of students estimated by IRM was defined by the “person ability”, or “θ” value of latent trait. Item difficulty computed by IRM was expressed as the same logit as the person ability. The “outcome” or “learning capacity” implied the academic level measured by exam scores.
Mokken scale analysis
Mokken Scale Analysis (MSA) is a npIRT model, which can extract a parsimonious subset of items from the original questionnaire items. The total score of one or more subsets of items informs the ordering of the latent traits. We adopted the monotone homogeneity model (MHM) as one of Mokken models, which relies on three assumptions to order persons using the sum score on a set of items [ 18 ]: ① Unidimensionality: The scale measures the single latent trait, equivalent to one factor in the scale; ② Local independence: The associations between scores of two items are solely explained by the θ, where the individual item score is conditionally independent given the latent trait; ③ Monotonicity: Monotonicity is depicted as the item characteristic curve (ICC) that increases or remains constant, but cannot decrease as the θ increases. The ICC is plotted to speculate the relation between the θ and the probability of obtaining item scores, which is typically an S-shaped curve.
The homogeneity coefficients, also known as scalability coefficients, are key indicators of MSA. Considering the sample size and number of questionnaire items, the threshold for the global homogeneity coefficient of all items (denoted as H) was set at 0.42 [ 33 ]. According to this boundary value, the automatic item selection procedure (AISP) was exerted to obtain a set of items that meet the unidimensionality [ 34 ]. The inter-item homogeneity coefficient (H ij ) was then calculated, where H ij < 0 violates the MHM assumption.
The conditional association proposed by Straat et al. [ 35 ] was also utilized to compute three W indices to identify the local dependence. The W 1 index detects the positive local dependence (cov(i, j|θ) > 0). The W 2 index determines the likelihood of each item being in a positive local dependence. The W 3 index explores negative local dependence (cov(i, j|θ) < 0). The upper limit of the Tukey threshold regarding each W index distribution is the criteria to screen the extreme W values. If W values are larger than the upper limit, it means the violation of local independence. Additionally, we employed the ICC visualization analysis and counted the number of violations to test for monotonicity in MHM.
Logistic model analysis
Although the MSA can extract a set of items that meets three assumptions of the MHM, it employs face values rather than parameters to characterize person abilities and item difficulties. On the other hand, the stricter pIRT models have been designed to compare individual abilities and item difficulties on the same scale, which also needs to satisfy unidimensionality, local independence, and monotonicity assumptions. Thus, constructing the MHM and extracting candidate items derived from the MSA are more effective in conducting the pIRT modeling [ 15 , 16 , 18 , 35 , 36 , 37 ].
One common unidimensional pIRT model is the logistic item response model. These models implement log odds (Logit) as the unit of measurement for person abilities (θ, i.e., latent traits) and item parameters. Within the logistic model, four key item parameters are illustrated in the fICC [ 38 ]: ① Discrimination (a): This parameter corresponds to the maximum slope value at the inflection point on the ICC. It quantifies how effectively items can differentiate between individuals with high and low abilities. ② Difficulty (b): The θ value corresponds to the inflection point on the ICC. As the b value increases, the ICC shifts to the right, indicating an increase in item difficulties, resulting in a decreased scoring rate for test items, even when person abilities remain unchanged. Conversely, a decrease in the b value shifts the ICC to the left, signifying a decrease in item difficulties. ③ Guessing (g): The lower asymptote of the ICC. If g is greater than zero, it indicates that individuals with low ability have a certain probability of obtaining scores due to guessing. ④ Carelessness (u): The upper asymptote of the ICC. If u is less than 1, it suggests that individuals with high ability may lose points due to carelessness.
These parameters are instrumental in constructing four different logistic models. The 1-parameter logistic model (1PLM) estimates the b value, assuming default values of a = 1 (consistent discrimination for all items), g = 0 (no guessing), and u = 1 (no carelessness). The 2-parameter logistic model (2PLM) estimates a and b, with default values of g = 0 and u = 1. The 3-parameter logistic model (3PLM) estimates a, b, and g, while assuming a default value of u = 1. The 4-parameter logistic model (4PLM) estimates all four parameters. The estimations for the four alternative models are conventionally set in the range of -6 to 6 Logit for θ values, and the parameter estimation usually adopts the expectation-maximization (EM) algorithm. The calculation precision (i.e., EM convergence threshold) default is set as 10 − 5 . The assessment of these models was carried out by two-step tests.
First step assessed the goodness of fit (GOF) of the model and the questionnaire data, including p -value based upon M 2 statistic, root mean square error approximation (RMSEA), Tucker-Lewis index (TLI), and comparative fit index (CFI). This study determines the model fit following the criteria: p > 0.05 [ 39 ], RMSEA < 0.05 [ 40 ], TLI > 0.95, and CFI > 0.95 [ 41 ].
Second, when multiple models display good fitness, it’s essential to conduct pairwise comparisons using likelihood ratio tests. If the p -value < 0.05 signifies a significant difference between the two models, the model with lower Akaike information criterion (AIC) and Bayesian information criterion (BIC) values is preferred. The p -value is great than 0.05, which indicates no significant difference between the models. Even though the model with a smaller AIC and BIC might be a reasonable choice in this scenario, it’s important to contemplate the inclusion of g and u parameters. A significant positive correlation between total scores and Gutmann errors indicates that individuals with higher scores tend to make more Guttman errors, likely due to carelessness. In this case, including the u parameter is recommended, leaning towards the 4PLM. Conversely, if there’s a significant negative correlation, it suggests that individuals with lower scores are prone to more Guttman errors, possibly resulting from excessive guessing. Here, the g parameter should be integrated, pointing to a preference for the 3PLM.
Analysis of the final model
Four key indicators were employed to evaluate the final model’s internal consistency, including Cronbach’s alpha, Guttman’s lambda-2, Molenaar-Sijtsma statistic, and latent class reliability coefficient (LCRC). As per van der Ark et al. [ 42 ], the LCRC stands out as a superior measure of reliability compared to the other three indicators. A value exceeding 0.9 is deemed indicative of high reliability.
Also, we developed the self-report-based knowledge point learning IRT model. The estimated θ values stand for individuals’ SEL in mastering the course material. The correlation analysis was also performed between the θ values and students’ final exam scores in the “Therapeutic Exercise” course (course learning outcomes), as well as their average GPA (comprehensive learning capacity). The aim was to explore the relationship between SEL and both course learning outcomes and comprehensive learning capacity.
The examination of measurement equivalence, also known as measurement invariance, was conducted to revolve around the principle that individuals with the same θ value exhibit score differences attributable to factors other than θ [ 43 , 44 , 45 ]. These extraneous factor-related differences can be classified into two categories: differential item functioning (DIF) at the item level, and differential test functioning (DTF) at the overall test level. The exclusion of DIF and DTF can ensure unbiased assessment results across different populations by eliminating potential biases introduced by specific items or scales. DIF analysis is built on the concept of anchor items, which are items exhibiting no significant between-group differences in their parameters. The DIF analysis comprises two steps, each step involving an internal iterative process [ 45 ], that is, exploratory DIF analysis and confirmatory DIF analysis.
The initial step involved a stepwise iterative approach, where the assumption of “all other items as anchors (AOAA)” was applied. Each item was sequentially selected to gauge any discernible between-group differences in its parameters (a, b, g, u) using likelihood ratio tests. Any item with a p -value > 0.05 was designated as an anchor item, while items with p- values < 0.05 were categorized as suspected DIF items. These suspected DIF items were methodically removed one by one until every item had undergone inspection. This process yielded two lists: one consisting of anchor items and another comprising suspected DIF items.
The second step was a systematic iterative process, where the assumption of “the suspected DIF item as the anchor item” was derived from the previously identified anchor items. Each item with suspected differential item functioning (DIF) was incorporated into the model one at a time. We then conducted a likelihood ratio test to evaluate any between-group differences in the item parameters. If an item exhibited a p -value < 0.05 and a substantial effect size, it was categorized as a DIF item. Items with p -values < 0.05 but with a small effect size, in accordance with the criteria outlined by Meade [ 44 ], as well as items with p -values exceeding 0.05, were classified as non-DIF items. Upon completing this second-step iteration, the non-DIF items identified during this phase, along with the anchor items from the initial step, were merged to create a conclusive list of non-DIF items. To visualize the results of the second-step analysis, an expected score distribution plot was presented.
In this study, the focus group was the male group, with the female group serving as the reference group. Using the θ values of the focus group as the reference point, we employed the item parameters specific to each group to compute the expected item scores and overall test scores. These calculations enabled the creation of an expected score distribution plot, revealing the comparative performance of both groups.
KG modeling
Data shaping.
The knowledge point rating scores were transformed from 0-1-2-3-4 to 0-0-0-1-1. A dichotomization strategy was employed, assigning a score of 1 to KPs categorized as “difficult” or “very difficult”, and a score of 0 to those deemed “easy”, “relatively easy”, or “slightly difficult”.
Network preparation
Gaussian Graphical Models with Non-Convex Penalties (GGMncv) were used to compute the partial correlation coefficients between KPs [ 30 ]. KPs were considered as network nodes. The partial correlation relationships constituted connections, and the magnitude of the partial correlation coefficients manifested the strength of these connections. This methodology facilitated the construction of a KG rooted in network theory. There was a total of 100 nodes and 1197 connections in our KG. All nodes were interconnected in a singular network structure without any isolated or separate subnetworks.
Skeleton extraction
A three-step process was adopted to extract the skeleton structure of the KG [ 31 ]. The first step extracted a positive signed backbone through the disparity filter method [ 46 ]. The disparity filter determined the significance of the connection values, retaining only those connections that had a significant difference at a significance level of 0.05, with Bonferroni correction for multiple testing. This step led to a 50.7% reduction in connections, transforming the previous network into a signed network, where positive and negative connections were respectively represented as + 1 and − 1.
This yielded a positive signed backbone comprising 104 connections. The positive signed backbone specifically elucidated the positive correlation relationships between KPs, where mastering the knowledge point i aided in comprehending the knowledge point j. 461 negative connections were ruled out due to lack of practical significance, as they meant mastering the knowledge point i would make it more difficult to understand the knowledge point j.
The second step involved network sparsification. The most important connections of each node were extracted from the labeled skeleton with the L-Spar model, as introduced by Satuluri et al. (2011) [ 47 ]. The threshold of the L-Spar model was set to 0, which enabled the preservation of the single most crucial connection for each node. This step led to a further reduction of 2.9% in connections. Finally, a sparse positive signed backbone structure emerged, encapsulating 101 connections.
The third step restored the actual connection values. According to the positive signed backbone structure, the corresponding structure containing the actual connection value was extracted from the original network, thus obtaining the positive backbone of the KG. The positive signed backbone structure only included connections with a value of 1, which resulted in a skeleton structure of the positive correlation relationships in the KG. The positive backbone illustrates the most important positive correlation relationship structure in the KG, which could be further utilized to examine the weight of each knowledge point.
Network analysis
The term “ego” denoted a specific knowledge point that was selected for examining its weight. ① Degree (DEG) and weighted degree (wDEG): DEG measures the number of connections a given ego node has, while wDEG considers the cumulative strength or weight value of those connections. A higher value indicates that the ego has a greater local impact on the network. ② Betweenness (BET): BET of the ego quantifies the information flow. The range of values is standardized to 0–1. A higher value indicates that the ego serves as a bottleneck, meaning other nodes rely on it to connect. ③ Hub score (HUB): HUB exhibits the centrality of the ego as an information hub within the network. It considers not only the number and strength of connections held by the ego but also the connections of the ego’s neighboring nodes. The range of values is standardized to 0–1, with a higher value signifying a more central position for the ego in the network. ④ Laplacian centrality (LAP): LAP measures the extent of disruption to the overall network structure if the ego is removed. The extent of damage will involve the overall network connectivity and structure if the ego is removed. A higher value indicates that the ego is more indispensable to the network.
Demographic
218 students (62 male and 156 female) majoring in Rehabilitation Therapy at the NJUCM were enrolled in this study. 10 excluded students took ≤ 100s to complete the questionnaire, and were therefore excluded from this study, as their responses were considered too hasty. The results of the remaining 208 students (56 males and 152 females) were specified for further analysis. The average time to complete the questionnaire was 205.00 s (s) [95% CI: 106.35s, 660.32s]. When stratified by gender, female and male students completed the questionnaire in 212.50s [95% CI: 110.65s, 680.87s] and 189.50s [95% CI: 104.88s, 455.00s] respectively, with no significant gender differences (Kruskal-Wallis χ 2 = 3.585, df = 1, p = 0.0583, η 2 = 0.0173). Average final exam scores were 86.00 [95% CI: 63.00, 95.00] for females, and 82.00 [95% CI: 62.38, 95.88] for males, without significant gender differences (Kruskal -Wallis χ 2 = 3.4216, df = 1, p = 0.0644, η 2 = 0.0165). The final exam GPA was 3.35 [95% CI: 2.43, 3.98], of which 3.42 [95% CI: 2.46, 3.97] GPA for females and 3.20 [95% CI: 2.41, 4.00] GPA for males. Although there was a significant difference in GPA across gender (Kruskal-Wallis χ 2 = 9.4402, df = 1, p = 0.0021), the effect was small ( η 2 = 0.0456) and considered negligible.
IRT modeling results
Data preparation.
The difficulty rating of 100 KPs was binarized, which did not exhibit constant values. All of them entered the following IRM. There were no pseudo-constant KPs after dichotomization. As also shown in Figure S1 , no students were excluded due to exceeding the criterion of the upper limit of Guttman errors, thereby allowing integration of the data collected from 208 students into the subsequent IRM phase.
Non-parametric IRT: mokken scale analysis
Aisp analysis (table s1 ).
The threshold of 0.42 was set for the H, which led to the removal of 33 items that did not align with any specific dimension. There were 67 remaining items divided into 5 dimensions (scales), of which 50 items were in dimension 1. Elevating the H threshold did not increase in the number of items allocated to any dimension. Following the methodology recommended by Straat et al. [ 33 ], this study adopted the H threshold of 0.42. Consequently, 50 items from dimension 1 (scale 1) were chosen for further detailed analysis (Table S2 ).
Unidimensionality analysis
The 50-item scale exhibited an H of 0.4756 and a standard error (SE) of 0.0355. In accordance with the criteria established by Sijtsma and van der Ark [ 26 ], the homogeneity of our scale was determined to be at a medium level. Given that the extracted items were situated within dimension 1, there was no need for additional assessments of unidimensionality.
Local independence analysis
According to Sijtsma and van der Ark [ 26 ], if the relationship between any two items i and j violates the local independence given H ij < 0, the MHM was not satisfied. The minimum value of H ij for the 50-item scale was 0.2063, confirming that none of the H ij values violated the prerequisites of the MHM. Moreover, the W 2 index also affirmed the absence of any items engaged in locally positive dependent relationships [ 35 ].
Monotonicity analysis
In the monotonicity test, if the diagnostic critical value (Crit) is ≥ 80, it can be considered a significant violation [ 48 ]. There were no obvious violations in 50 KPs (Table S3), which conformed to monotonicity. The monotonicity can also be further confirmed by the ICC shape of the model built in the pIRT stage. The monotonicity was achieved when the ICC of each item increased with θ but did not decrease (Figure S2).
Parameter IRT: logistic regression model analysis
Model-data fit analysis.
As shown in Table S4, the p values resulted from the M 2 test for all models yielding a value of 0, but the RMSEA of the 3PLM fell below 0.05. The TLI and CFI for all models exceeded 0.95. Therefore, all models were assigned for pairwise comparisons.
Model-model fit comparison
In Table S5, a significant difference (χ 2 (49) = 77.2966, p = 0.0061) was observed between the 1PLM and 2PLM (χ 2 (49) = 77.2966, p = 0.0061), with 1PLM exhibiting a lower BIC (ΔBIC = -184.2427), suggesting potential superiority to 2PLM. However, there was no significant difference when comparing the 1PLM to either the 3PLM (χ 2 (99) = 88.6090, p = 0.7637), or the 4PLM (χ 2 (149) = 121.8965, p = 0.9492).
As illustrated in Figure S3, the total scores of the 50-item scale for each student were negatively correlated with the number of Guttman errors ( p = 1.51 × 10 − 22 ). The correlation coefficient ( \( {\widehat{\rho }}_{Spearman}\) = -0.61) fell within the range of 0.4–0.7, indicating a moderate correlation according to Akoglu’s standards [ 49 ]. This correlation revealed that students with lower scores tended to demonstrate more Guttman errors, implying that they were more likely to guess correctly on items with higher difficulty. This observation underscored the importance of considering guessing behavior in the analysis. Additionally, there was no significant positive correlation, suggesting higher-scoring students did not have more Guttman errors, i.e., they did not lose scores on items with lower difficulty, and thus, the carelessness parameter did not need to be considered.
The 3PLM was ultimately chosen, which did not have a significant difference with 1PLM and additionally incorporated a guessing parameter compared to the 1PLM. Guessing was the lower bound of the ICC, as shown in Figure S2, where a number of KPs (such as kp.75, kp.55, kp.31, and kp.14) had non-zero guessing values. To ensure the property of the 3PLM result, considering the small sample size, we also performed Monte Carlo simulation that generated 500 models with each simulating 1000 response patterns [ 50 ].
Reliability analysis
Cronbach’s α = 0.9684, Guttman’s λ2 = 0.9689, Molenaar Sijtsma Statistic = 0.9708, LCRC = 0.9776. All four coefficients were > 0.95, indicating good internal consistency for the 50-item scale.
Grade-related analysis
As shown in Fig. 2 , the estimated SEL was not significantly correlated with exam scores ( p = 0.81) or GPA ( p = 0.81). However, within the range of θ > 0, a weak positive correlation was observed between GPA and θ ( p = 0.02, r = 0.22).
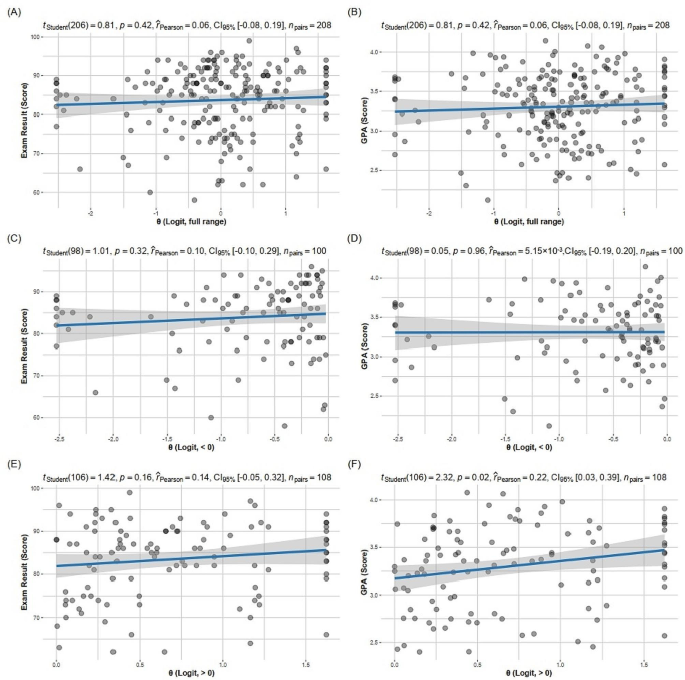
Correlation of exam or GPA scores with full range, < 0, or > 0 values of θ
Gender bias analysis
The distribution of expected item scores (Figure S4) and expected test scores (Figure S5) suggested that the 50-item scale did not elucidate significant gender bias.
Model parameter analysis
Table 1 displayed the three parameters of the 3PLM arranged in descending order of difficulty. The majority of items illustrated a guessing parameter of either 0 or very close to zero (< 0.1). The item with the highest guessing parameter was kp.31 (g = 0.2803).
Table 1 also displayed the model fit test results for each item. Only kp.14 showed significant differences in the S-X 2 test ( p = 0.0466), but its RMSEA (0.0575) reached a publishable level, as suggested by Xia and Yang [ 41 ]. Furthermore, the OUTFIT (1.0073) and INFIT (0.9765) for kp.14 both fell within the recommended range of 0.7 to 1.3 according to the thumb rule for item fit [ 51 ]. The z-OUTFIT (0.1431) and z-INFIT (-0.131) also fell within the acceptable range of z values (-2.0 to 2.0). Therefore, we concluded that kp.14 fit well in the model.
Total score conversion
There was a significant positive correlation between the total score (TTS) on the 50-item scale and the model-estimated θ value ( p = 1.96 × 10 − 235 , \( {\widehat{\rho }}_{Spearman}\) = 1.0) (Figure S6). The binomial function to facilitate conversion between these two variables can be applied as follows: \( \widehat{\theta }= - 2.09+0.0322\times TTS+0.000622\times TT{S}^{2}\) .
Wright map analysis
Figure 3 showed the student W.,T.’s SEL (-0.4165 Logit). For the student W.,T., the items with the lowest difficulty within his learning competency area were identified as kp.14 (Muscular endurance) and kp.78 (Different balance forms). The student W.,T. should prioritize to grasp these KPs.
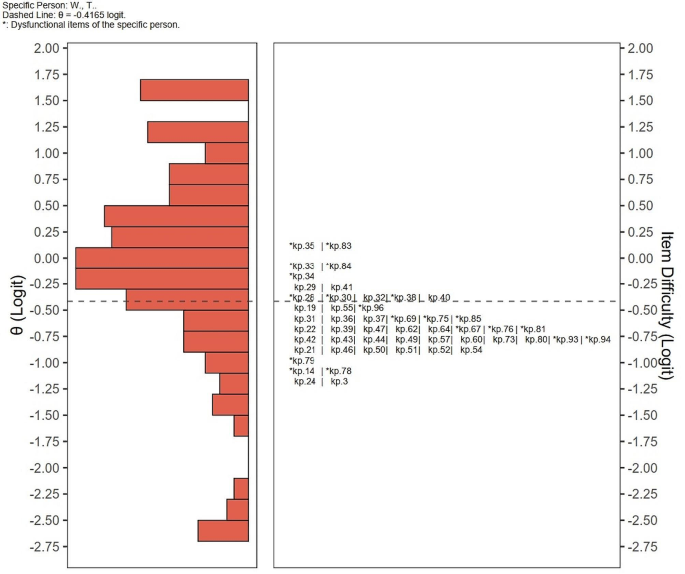
Wright map denoting self-efficacy of student W.,T. The * symbol indicated W.,T received 0 on items in the difficulty rating questionnaire after binarization, which are the items the student perceived as difficult. Knowledge points below the ability line are relatively easy to master, while those above the ability line are relatively difficult. Therefore, the area below the ability line represents competency, while the area above represents challenging points
Knowledge graph analysis
Network parameters for knowledge points.
We investigated a KGM composed of 100 KPs, revealing a backbone structure with 68 connections. The connection values were indicated by partial correlation coefficients, ranging from a minimum of 0.106 (weak correlation) to a maximum of 0.464 (moderate correlation) [ 49 ].
The analysis of network parameters of KPs within the backbone structure (Table S6) identified 39 isolated points with a degree of 0. The top three KPs in terms of hub score were kp.46 (1.00, Indications for joint mobility techniques), kp.13 (0.8480, Muscle strength), and kp.90 (0.8204, Contraindications for PNF technique). These three points also held the top three in terms of the BET.
The top three KPs considering Laplacian centrality were kp.46 (Indications for joint mobility techniques), kp.13 (Muscle strength), and kp.63 (Indications for joint mobilization). These three KPs also ranked among the top three in terms of weighted degrees. Overall, kp.46 not only featured prominently in the IRM, but also occupied the most critical position within the backbone structure, underscoring its significance in the knowledge structure of the course “Therapeutic Exercise”. According to Table 1 , kp.46 had a relatively low difficulty (-0.8479), which fell below the mean difficulty of 50 items (-0.6346). Moreover, it exhibited moderate discrimination (2.5353), closely aligning with the mean discrimination of 50 items (2.42046).
Visualization of the main component in the backbone structure
The primary subnetwork within the backbone structure, identified as the main component, comprised 58 nodes, with 29 KPs incorporated into the final IRM. This main component captured 66 out of the 68 connections in the backbone structure. Figure 4 showed the visualization of this main component. The layout was improved by adopting the Sugiyama method for unveiling its hierarchical structure [ 52 , 53 ].
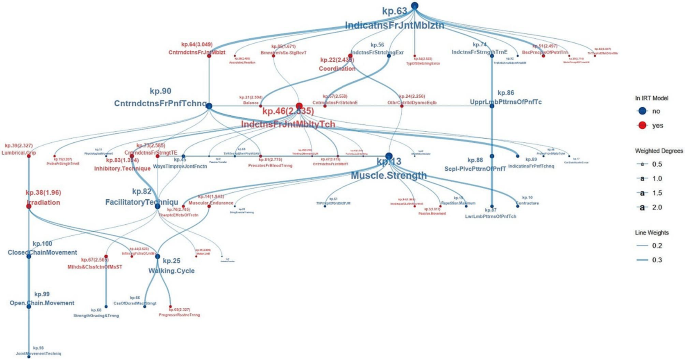
Main component in backbone structure of knowledge graph for Physical Therapy The knowledge points in IRT model (red) were tagged with discrimination parameter
Adapting teaching strategies stemming from the difficulty of KPs is essential for ensuring quality management in curriculum development. However, there is a lack of current reports that quantitatively assess the self-perceived learning difficulty of KPs in medical education. Course difficulty can be categorized into teaching difficulty and learning difficulty from the perspectives of teachers and students, respectively. To effectively evaluate the learning difficulty of KPs, it is necessary to consider students’ learning capacities.
Clinical education applies a wide range of assessment formats, and structured exams are not consistently employed. This diversity poses a substantial challenge when weighing the difficulty of KPs. Meanwhile, the difficulty of KPs can be intertwined with students’ personal traits. Consequently, integrating comprehensive methods to discern students’ personal traits, evaluate the difficulty of KPs, and understand the correlations between different KPs, is a critical process in achieving pedagogical excellence.
The study extracted 100 KPs from the course “Therapeutic Exercise” to investigate students’ perceived difficulty in comprehending KPs. The npIRT and pIRT modeling were sequentially conducted to obtain a parsimonious item set that could be sufficient to distinguish the personal trait levels of the participants without gender bias. IRM was employed to estimate students’ SEL and item difficulty. Students’ SEL was referred to person ability or θ in the IRM. It should be noted that the interpretation of item difficulties is determined by the binarization strategy, indicating the difficulty of attaining scores. In this study, we assigned value 1 as self-confidence in the questionnaire. Therefore, the practical meaning of item difficulty was the difficulty of being self-confident about mastering certain KPs.
Furthermore, graph modeling techniques were also applied to construct a KG based on the conditional association of difficulty correlations among each knowledge point. Although the KG established in this research might not exactly mirror the knowledge schema formed by students through course learning, it can be used to analyze the knowledge schema affected by personal traits. In other words, it can be regarded as the correlation structure of KPs’ difficulty under the influence of SEL.
Implication of the IRM-derived student ability
Our result did not find a significant correlation between one-time exam scores and θ values. However, a significant correlation between the GAP and the estimated θ values, particularly within the spectrum of positive θ values. This correlation supports the reliability of the model which contains 50 KPs, in evaluating students’ learning abilities.
Our findings are in line with the research conducted by Richardson et al. [ 7 ]. They propose that GPA cannot be solely explained by exam scores, for example, the Scholastic Aptitude Test. They indicate that exam scores are a one-time assessment of course-specific learning effectiveness, while GPA can reveal broader academic performance. GPA is a comprehensive indicator of students’ academic performance, reflecting not only learning abilities but also potential career prospects. They conducted correlation analyses involving GPA during undergraduate university with various traits of students. Their research unveiled a medium-to-large correlation between students’ SEL and GPA, with academic self-efficacy (ASE) exhibiting a medium correlation and performance self-efficacy (PSE) showing a strong correlation. Among the 50 factors they examined, PSE showed the strongest correlation with GPA.
Self-efficacy was first introduced by Bandura to manifest individuals feeling of confidence in their capabilities necessary to reach specific goals [ 9 ]. Richardson further defines the self-efficacy into the ASE and PSE. ASE means students’ general perception of their academic competence, which is specifically described as “I have a great deal of control over my academic performance in my courses”. PSE encompasses students’ perceptions of their academic performance capability, as articulated by “What is the highest GPA that you feel completely certain you can attain”. ASE predominantly focuses on self-ability level, while PSE is oriented towards evaluating the anticipated outcomes of the learning process. Our study adopted the difficulty of the KPs scale that was analogous to the concept of PSE as described by Richardson et al. [ 7 ], as both aim to gauge the extent of knowledge mastery with complete certainty.
The results of the correlational analysis within this study suggested that SEL, derived from a questionnaire on KPs’ difficulty, can be categorized into two distinct types: positive SEL and negative SEL. For students displaying positive SEL, there was a weak positive correlation between GPA and SEL ( p = 0.02, r = 0.22). It indicated that higher SEL corresponded to higher GPAs, which aligns with self-efficacy theory [ 9 ].
On the contrary, this study also identified negative SEL that failed to predict the one-time course exam scores or correlate the comprehensive learning ability measured by GPA. Therefore, it further suggests that SEL based on psychological questionnaires and learning outcomes based on exams should be treated differently. When evaluating students’ learning abilities, reliance on one-time assessment results alone is insufficient.
Furthermore, how to foster positive SEL in students to enhance their overall learning capabilities is also crucial. Encouraging individuals to set realistic and attainable goals can build confidence and contribute to a positive self-perception [ 54 ]. The following might offer a practical example to assist students in establishing personal goals regarding their person ability as well as importance and difficulty of KPs.
Practical example based on the wright map and the knowledge graph
The wright map displays both persons (in terms of their ability) and items (in terms of their difficulty) on the same scale. It was plotted according to individual θ values to assess individual competency, delineating areas of competence (below the θ value) and incompetence (above the θ value). The analysis of a student’s θ was instrumental in pinpointing specific KPs that warrant focused review. Table 1 , as provided by 3PLM, offered insights to educators to identify KPs demanding increased attention during future teaching endeavors. KPs characterized by higher difficulty should be allocated more teaching time and resources. Furthermore, KPs with higher discrimination, exemplified by kp.31 (motor unit, with the highest discrimination), in this example, should be subjected to more in-class assessments and feedback. Proficiency in these highly discriminative KPs plays a pivotal role in refining the individual ability.
We selected 100 KPs that were considered important points, while 50-item 3PLM could distinguish the difficulty levels of KPs, so as to figure out the relative difficult points. The excluded items were also important for the course, but they were not “simplified enough to distinguish student abilities in IRT model”. Since the items aside from the model did not have difficulty parameters, how to assess the difficulty of these items was another puzzle that needed to be addressed.
In order to solve the above issue, this study also applied the Gaussian random graph model leveraging conditional correlations to calculate partial correlations between different KPs. The correlation between two KPs within the graph model displayed their “difficulty correlation”. In other words, how likely it was that when one knowledge point was difficult or very difficult, the other knowledge point exhibited a similar difficulty level. Therefore, the KG portrayed the relationships based on difficulty correlations.
An examination of the skeleton structure of the KG, as depicted in Fig. 4 , revealed that kp.63 (indications for joint mobilization) occupied the highest position within the hierarchical structure. Notably, it was observed that KPs capable of distinguishing individual traits did not necessarily hold prominent positions within the network. Regarding the principle of constructing graph models based on risk correlation relationships, if important positions are not thoroughly mastered, it would increase the risk of not comprehending other associated items. Therefore, the item kp.46, as a necessary point, which occupied a crucial position and had a certain level of discrimination, should be prioritized for the student to master.
Limitations and future directions
This study was not without its limitations, which were rooted in the constraints imposed by real-world teaching conditions. These limitations provide opportunities for further improvement.
Firstly, the sample size in our study remained relatively small, and the research was confined to specific courses and KPs. We also observed from the Wright map that a majority of KPs within a similar difficulty range, posing a challenge in distinguishing between individuals with high and low abilities. To enhance the robustness of the findings, we would progressively increase the sample size in each cohort of students in future research. There is also a need to continuously broaden the curriculum by incorporating new KPs and domains, extending the generalizability of our findings. Integrating E-learning platforms with the capability to customize and adapt teaching plans through expert-selected, student-rated questionnaires assessing the difficulty of KPs, holds promise for enhancing the educational experience.
Secondly, our study relied on cross-sectional data, and the difficulty questionnaire was administered only once. While GPAs provide a more comprehensive manifestation of the PSE compared to one-time exams, there is still a requirement for quantitative evidence to support long-term effect of SEL on academic performance. Thus, a promising avenue for future research involves undertaking longitudinal studies to explore the impact of adjusted SEL on long-term academic performance. Our approach could potentially provide a measurement tool for assessing the effectiveness of different interventions aimed at improving SEL over an extended period in future studies.
Thirdly, the validity indicators of the model were singular. Future research should consider supplementing exam scores with other learning ability assessment scales, as well as novel measures like brain-computer interfaces and online learning behavior records. These additions will provide valuable multimodal data to evaluate knowledge point significance and candidate abilities more comprehensively. Despite these limitations, this study introduced an innovative and up-to-date quantitative analysis approach, and its results serve as a foundation for ongoing improvement.
Fourthly, the KGM in this study involved a narrow concept network model which requires to integration of various elements of multiple types such as courses, personnel, and locations, as well as multiple relationship structures. This will enable the incorporation of person abilities and item difficulties calculated by the IRM as indicators for related elements, resulting in a more holistic KG for a comprehensive evaluation of the teaching process [ 23 ].
Lastly, the questionnaire was based on students’ self-assessment of the difficulty of KPs, which could reflect the students’ SEL as θ values. Although the IRM defines the θ as personal ability, it might not be directly equated to students’ learning abilities. Nevertheless, the correlation between SEL and GPA provided partial evidence that the questionnaire could also be a useful tool for evaluating learning abilities. Research into the relationships between psychological traits and learning ability traits could become a promising long-term avenue for investigation, and this study contributes practical evidence and tools to this evolving field.
This study employs a self-assessment questionnaire to achieve students’ perceptions of the difficulty of KPs. It integrates the IRM and KGM to quantitatively assess parameters like students’ SEL, the difficulty level of being self-confident about mastering certain KPs, and importance of KPs. The results affirm that IRM and KGM offer quantitative metrics rooted in empirical data. These metrics are instrumental in identifying and categorizing important, difficult, and necessary points within the curriculum. Furthermore, our study serves as a valuable tool for establishing an evidence-based and refined teaching management approach, thereby enhancing the overall quality of education.
Data availability
The datasets used and/or analyzed during the current study are available from the corresponding author on reasonable request.
Bossé É, Barès M. Knowledge and its dimensions. In: Barès M, Bossé É, editors. Relational Calculus for actionable knowledge. Cham: Springer International Publishing; 2022. pp. 45–115.
Chapter Google Scholar
Whetten DA. Principles of effective Course Design: what I wish I had known about learning-centered teaching 30 years ago. J Manag Educ. 2007;31:339–57.
Article Google Scholar
Li W, Li X, Wu W. Knowledge Innovation mechanism based on linkages between Core Knowledge and Periphery Knowledge: the Case of R&D Cooperation between latecomers and forerunners. Complexity. 2022;2022:e8417784.
Google Scholar
Shou Z, Lai J-L, Wen H, Liu J-H, Zhang H. Difficulty-based Knowledge Point Clustering Algorithm using students’ multi-interactive behaviors in Online Learning. Math Probl Eng. 2022;2022:e9648534.
Guo H, Yu X, Wang X, Guo L, Xu L, Lu R. Discovering knowledge-point importance from the learning-evaluation data. Int J Distance Educ Technol. 2022;20:1–20.
Chen SY, Wang J-H. Individual Differences and Personalized Learning: a Review and Appraisal. Univers Access Inf Soc. 2021;20:833–49.
Richardson M, Abraham C, Bond R. Psychological correlates of university students’ academic performance: a systematic review and meta-analysis. Psychol Bull. 2012;138:353–87.
Kang Y-N, Chang C-H, Kao C-C, Chen C-Y, Wu C-C. Development of a short and universal learning self-efficacy scale for clinical skills. PLoS ONE. 2019;14:e0209155.
Bandura A. Self-efficacy: toward a unifying theory of behavioral change. Psychol Rev. 1977;84:191–215.
Collard A, Gelaes S, Vanbelle S, Bredart S, Defraigne J-O, Boniver J, et al. Reasoning versus knowledge retention and ascertainment throughout a problem-based learning curriculum. Med Educ. 2009;43:854–65.
Honicke T, Broadbent J, Fuller-Tyszkiewicz M. The self-efficacy and academic performance reciprocal relationship: the influence of Task Difficulty and Baseline Achievement on Learner Trajectory. High Educ Res Dev. 2023;42:1936–53.
Honicke T, Broadbent J. The influence of academic self-efficacy on academic performance: a systematic review. Educ Res Rev. 2016;17:63–84.
Sijtsma K, Meijer RR. 22 nonparametric item response theory and special topics. In: Rao CR, Sinharay S, editors. Handbook of statistics. Elsevier; 2006. pp. 719–46.
Feng C, Jiang Z-L, Sun M-X, Lin F. Simplified post-stroke Functioning Assessment based on ICF via Dichotomous Mokken Scale Analysis and Rasch Modeling. Front Neurol. 2022;13:827247.
Jiang Y-E, Zhang D-M, Jiang Z-L, Tao X-J, Dai M-J, Lin F. ICF-Based simple scale for children with cerebral palsy: application of Mokken scale analysis and Rasch modeling. Dev Neurorehabilitation. 2023;:1–18.
Feng C, Geng B-F, Liu S-G, Jiang Z-L, Lin F. Activity and participation in haemophiliacs: item response modelling based on international classification of functioning, disability and health. Haemophilia. 2022. https://doi.org/10.1111/hae.14702 .
CHEN J-J, ZHU Z-Y, BIAN J-J, LIN F. Nutrition-associated health levels in persons with cancer: item response modelling based on the International Classification of Functioning, disability and health. Eur J Phys Rehabil Med. 2023;59:593–604.
Stochl J, Jones PB, Croudace TJ. Mokken scale analysis of mental health and well-being questionnaire item responses: a non-parametric IRT method in empirical research for applied health researchers. BMC Med Res Methodol. 2012;12:74.
van Kesteren MTR, Meeter M. How to optimize knowledge construction in the brain. Npj Sci Learn. 2020;5:1–7.
Brod G. Toward an understanding of when prior knowledge helps or hinders learning. Npj Sci Learn. 2021;6:1–3.
Leake DB, Maguitman AG, Reichherzer T. Understanding Knowledge Models: Modeling Assessment of Concept Importance in Concept Maps. 2004.
Chaudhri VK, Baru C, Chittar N, Dong XL, Genesereth M, Hendler J, et al. Knowledge graphs: introduction, history, and perspectives. AI Mag. 2022;43:17–29.
Aliyu I, Department of Computer Science ABUZ, Aliyu DKAF, Department of Computer Science ABUZ. Development of Knowledge Graph for University Courses Management. Int J Educ Manag Eng. 2020;10:1.
Moro C, Douglas T, Phillips R, Towstoless M, Hayes A, Hryciw DH, et al. Unpacking and validating the integration core concept of physiology by an Australian team. Adv Physiol Educ. 2023;47:436–42.
R Core Team. A language and environment for statistical computing. 2022.
Sijtsma K, van der Ark LA. A tutorial on how to do a Mokken Scale Analysis on your test and Questionnaire Data. Br J Math Stat Psychol. 2017;70:137–58.
Chalmers RP. Mirt: a Multidimensional Item Response Theory Package for the R environment. J Stat Softw. 2012;48:1–29.
Patil I. Visualizations with statistical details: the ggstatsplot approach. J Open Source Softw. 2021;6:3167.
Hubert M, Vandervieren E. An adjusted boxplot for skewed distributions. Comput Stat Data Anal. 2008;52:5186–201.
Williams DR. Beyond Lasso: A Survey of Nonconvex Regularization in Gaussian Graphical Model. 2020.
Neal ZP. Backbone: an R package to extract network backbones. PLoS ONE. 2022;17:e0269137.
Csardi G, Nepusz T. The igraph software package for complex network research. Interjournal Complex Syst. 2006;1695.
Straat JH, van der Ark LA, Sijtsma K. Minimum sample size requirements for Mokken Scale Analysis. Educ Psychol Meas. 2014;74:809–22.
Straat JH, van der Ark LA, Sijtsma K. Comparing optimization algorithms for Item Selection in Mokken Scale Analysis. J Classif. 2013;30:75–99.
Straat JH, van der Ark LA, Sijtsma K. Using Conditional Association To Identify Locally Independent Item Sets. Methodology. 2016;12:117–23.
Feng C, Lai Q-L, Ferland A, Lin F. Mandarin Stroke Social Network Scale and Item Response Theory. Front Stroke. 2022;1.
Koopman L, Zijlstra BJH, van der Ark LA. A two-step, test-guided mokken scale analysis, for nonclustered and clustered data. Qual Life Res. 2021. https://doi.org/10.1007/s11136-021-02840-2 .
Brzezińska J. Item response theory models in the measurement theory. Commun Stat - Simul Comput. 2020;49:3299–313.
Xu J, Paek I, Xia Y. Investigating the behaviors of M2 and RMSEA2 in fitting a Unidimensional Model to Multidimensional Data. Appl Psychol Meas. 2017;41:632–44.
Maydeu-Olivares A, Joe H. Assessing approximate fit in categorical data analysis. Multivar Behav Res. 2014;49:305–28.
Xia Y, Yang Y, RMSEA, CFI. Structural equation modeling with ordered categorical data: the Story they tell depends on the estimation methods. Behav Res Methods. 2019;51:409–28.
van der Ark LA, van der Palm DW, Sijtsma K. A latent Class Approach to estimating test-score reliability. Appl Psychol Meas. 2011;35:380–92.
Liu X, Jane Rogers H. Treatments of Differential Item Functioning: a comparison of four methods. Educ Psychol Meas. 2022;82:225–53.
Meade AW. A taxonomy of effect size measures for the differential functioning of items and scales. J Appl Psychol. 2010;95:728–43.
Nugent WR, Understanding DIF. Description, methods, and Implications for Social Work Research. J Soc Soc Work Res. 2017;8:305–34.
Serrano MA, Boguñá M, Vespignani A. Extracting the multiscale backbone of complex weighted networks. Proc Natl Acad Sci U S A. 2009;106:6483–8.
Satuluri V, Parthasarathy S, Ruan Y. Local graph sparsification for scalable clustering. In: Proceedings of the 2011 ACM SIGMOD International Conference on Management of data (SIGMOD'11). New York, NY, USA: Association for Computing Machinery;2011. pp. 721–732. https://doi.org/10.1145/1989323.1989399 .
van der Ark LA. New Developments in Mokken Scale Analysis in R. J Stat Softw. 2012;48:1–27.
Akoglu H. User’s guide to correlation coefficients. Turk J Emerg Med. 2018;18:91–3.
Harwell M, Stone CA, Hsu T-C, Kirisci L. Monte Carlo Studies in Item Response Theory. Appl Psychol Meas. 1996;20:101–25.
Hodge KJ, Morgan GB. Stability of INFIT and OUTFIT compared to simulated estimates in Applied setting. J Appl Meas. 2017;18:383–92.
Nikolov NS. Sugiyama Algorithm. In: Kao M-Y, editor. Encyclopedia of algorithms. New York, NY: Springer; 2016. pp. 2162–6.
Sugiyama K, Tagawa S, Toda M. Methods for Visual understanding of Hierarchical System structures. IEEE Trans Syst Man Cybern. 1981;11:109–25.
Bandura A. Self-efficacy: the exercise of control. New York, NY, US: W H Freeman/Times Books/ Henry Holt & Co; 1997.
Download references
Acknowledgements
We extend our gratitude to Amanda Ferland for her meticulous proofreading, addressing grammar errors, and refining the expression of our work.
This study was supported by the following teaching fundings.
(1) National Higher Education of Traditional Chinese Medicine “14th Five-Year Plan” 2023 Educational Research Project (YB-23-21): Research on the Reform of Fine Teaching Management of Traditional Chinese Medicine Colleges and Universities Driven by Digital Educational Measurement Technology - Taking the Therapeutic Exercise Course as an Example. (2) 2021 Jiangsu Province Higher Education Teaching Reform Research Project(2021JSJG295): Exploration of the Teaching Content System of Rehabilitation Therapy with Chinese Medicine Characteristics Based on the Standard of International Classification of Functioning, Disability and Health (ICF). (3) Shanghai Rising-Star Program & Shanghai Sailing Program (23YF1433700).
Author information
Authors and affiliations.
Department of Rehabilitation Medicine, School of Acupuncture-Moxibustion and Tuina, School of Health Preservation and Rehabilitation, Nanjing University of Chinese Medicine, 210023, Nanjing, China
Zhen-Yu Cao
School of Rehabilitation Medicine, Nanjing Medical University, 211100, Nanjing, China
School of Medicine, Tongji University, 200331, Shanghai, China
The Center of Rehabilitation Therapy, The First Rehabilitation Hospital of Shanghai, Rehabilitation Hospital Affiliated to Tongji University, 200090, Shanghai, China
You can also search for this author in PubMed Google Scholar
Contributions
FL, ZY.C, and CF contributed to the research concept, supervised the entire study; ZY.C collected data. FL performed the analysis, generated the images, and wrote the manuscript with ZY.C and CF; All authors contributed to the article and approved the submitted version.
Corresponding author
Correspondence to Chun Feng .
Ethics declarations
Ethics approval and consent to participate.
This study was approved by the Institutional Ethics Committee of Nanjing University of Chinese Medicine (No. NJUCM 2021-LL-13(L)). The research was conducted ethically, with all study procedures being performed in accordance with the requirements of the World Medical Association’s Declaration of Helsinki. Written informed consent was obtained from each participant/patient for study participation and data publication.
Conflict of interest
The authors declare no conflict of interest.
Additional information
Publisher’s note.
Springer Nature remains neutral with regard to jurisdictional claims in published maps and institutional affiliations.
Electronic supplementary material
Below is the link to the electronic supplementary material.
Supplementary Material 1
Rights and permissions.
Open Access This article is licensed under a Creative Commons Attribution 4.0 International License, which permits use, sharing, adaptation, distribution and reproduction in any medium or format, as long as you give appropriate credit to the original author(s) and the source, provide a link to the Creative Commons licence, and indicate if changes were made. The images or other third party material in this article are included in the article’s Creative Commons licence, unless indicated otherwise in a credit line to the material. If material is not included in the article’s Creative Commons licence and your intended use is not permitted by statutory regulation or exceeds the permitted use, you will need to obtain permission directly from the copyright holder. To view a copy of this licence, visit http://creativecommons.org/licenses/by/4.0/ . The Creative Commons Public Domain Dedication waiver ( http://creativecommons.org/publicdomain/zero/1.0/ ) applies to the data made available in this article, unless otherwise stated in a credit line to the data.
Reprints and permissions
About this article
Cite this article.
Cao, ZY., Lin, F. & Feng, C. Interpretation of course conceptual structure and student self-efficacy: an integrated strategy of knowledge graphs with item response modeling. BMC Med Educ 24 , 563 (2024). https://doi.org/10.1186/s12909-024-05401-6
Download citation
Received : 22 November 2023
Accepted : 08 April 2024
Published : 23 May 2024
DOI : https://doi.org/10.1186/s12909-024-05401-6
Share this article
Anyone you share the following link with will be able to read this content:
Sorry, a shareable link is not currently available for this article.
Provided by the Springer Nature SharedIt content-sharing initiative
- Knowledge points (KPs), Item Response Theory (IRT)
- Knowledge graphs (KG)
- Teaching evaluation
- Physical therapy
BMC Medical Education
ISSN: 1472-6920
- Submission enquiries: [email protected]
- General enquiries: [email protected]
Peer relationship instructions in inclusive educational settings in Korea: a meta-analysis
- Review Article
- Published: 20 May 2024
Cite this article
- Jechun An ORCID: orcid.org/0000-0003-1746-4154 1 ,
- Seohyeon Choi ORCID: orcid.org/0000-0003-1721-4956 1 &
- Jin Hyung Lim ORCID: orcid.org/0000-0002-2250-0052 2
42 Accesses
Explore all metrics
This study aimed to identify the effects of peer relationship instructions between students with and without disabilities in inclusive education settings in South Korea. We conducted a meta-analysis using journal articles published over the last 20 years. From a total of 1419 student data within 33 primary studies, we found an overall effect size of 0.78 (Hedges’ g ), which indicates a strong effect size of intervention efforts on peer relations of students with disabilities. With respect to outcomes, we found higher effect sizes ( g = 0.82) particularly on collaboration than self-directedness ( g = 0.47). We also found that instructions were highly effective for both students with ( g = 0.93) and without disabilities ( g = 0.76). It would be encouraged for educators to put consistent effort into implementing instructions that target to improve peer relationships and consider instruction variables such as length of program while balancing the quantitative and qualitative aspects of peer relationship.
This is a preview of subscription content, log in via an institution to check access.
Access this article
Price includes VAT (Russian Federation)
Instant access to the full article PDF.
Rent this article via DeepDyve
Institutional subscriptions
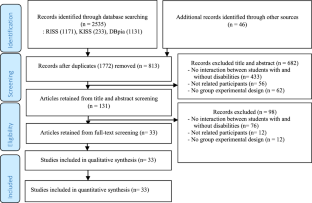
Similar content being viewed by others
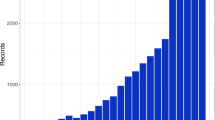
The Impact of Peer Assessment on Academic Performance: A Meta-analysis of Control Group Studies
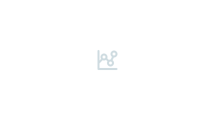
The justification for inclusive education in Australia
The role of school in adolescents’ identity development. a literature review.
Antia, S. (1999). The roles of special educators and classroom teachers in an inclusive school. Journal of Deaf Studies and Deaf Education, 4 (3), 203–214. https://doi.org/10.1093/deafed/4.3.203
Article Google Scholar
Appelbaum, M., Cooper, H., Kline, R. B., Mayo-Wilson, E., Nezu, A. M., & Rao, S. M. (2018). Journal article reporting standards for quantitative research in psychology: The APA publications and communications board task force report. American Psychologist, 73 , 3–25. https://doi.org/10.1037/amp0000191
Ballard, S. L., & Dymond, S. K. (2017). Addressing the general education curriculum in general education settings with students with severe disabilities. Research and Practice for Persons with Severe Disabilities, 42 (3), 155–170. https://doi.org/10.1177/1540796917698832
Boivin, M., & Hymel, S. (1997). Peer experiences and social self-perceptions: A sequential model. Developmental Psychology, 33 (1), 135. https://doi.org/10.1037/0012-1649.33.1.135
Borenstein, M., Hedges, L. V., Higgins, J. P., & Rothstein, H. R. (2011). Introduction to meta-analysis. Wiley . https://doi.org/10.1002/9780470743386
Bossaert, G., de Boer, A. A., Frostad, P., Pijl, S. J., & Petry, K. (2015). Social participation of students with special educational needs in different educational systems. Irish Educational Studies, 34 (1), 43–54. https://doi.org/10.1080/03323315.2015.1010703
Buysse, V., Goldman, B. D., & Skinner, M. L. (2003). Friendship formation in inclusive early childhood classrooms: What is the teacher’s role? Early Childhood Research Quarterly, 18 (4), 485–501. https://doi.org/10.1016/j.ecresq.2003.09.007
Caldarella, P., & Merrell, K. W. (1997). Common dimensions of social skills of children and adolescents: A taxonomy of positive behaviors. School Psychology Review, 26 (2), 264–278. https://doi.org/10.1080/02796015.1997.12085865
Chang, J. E., & Lee, S. H. (2019). The effects of class-wide social skill intervention based on the preschool curriculum on young children’s prosocial behavior and social acceptance toward peers with disabilities, and peer interaction of young children with disabilities. Korean Journal of Special Education, 54 (3), 19–47.
Cheng, L., Wang, X., & Ritzhaupt, A. D. (2023). The effects of computational thinking integration in STEM on students’ learning performance in K-12 education: A meta-analysis. Journal of Educational Computing Research, 61 (2), 416–443. https://doi.org/10.1177/07356331221114183
Cheung, M. W. L. (2014). Modeling dependent effect sizes with three-level meta-analyses: A structural equation modeling approach. Psychological Methods, 19 (2), 211–229. https://doi.org/10.1037/a0032968
Choi, J. (2009a). A review on class-wide peer tutoring for the students with disabilities and without disabilities in the inclusion classroom. Korean Journal of Special Education, 8 (1), 185–205.
Google Scholar
Choi, W. (2009). The effects of a classroom community development program: elementary school students’ perception on building friendships with students with disabilities. control, peer support, and class participation in elementary inclusive classrooms. [Master’s thesis, Ewha Womans University].
Cillessen, A. H. N., & Bukowski, W. M. (2018). Sociometric perspectives. In W. M. Bukowski, B. Laursen, & K. H. Rubin (Eds.), Handbook of peer interactions, relationships, and groups (pp. 64–83). Guilford Press.
Cooley, C. H. (1902). Human nature and the social order . NY: Scribner. https://doi.org/10.4324/9780203789513
Book Google Scholar
Cresswell, L., Hinch, R., & Cage, E. (2019). The experiences of peer relationships amongst autistic adolescents: A systematic review of the qualitative evidence. Research in Autism Spectrum Disorders, 61 , 45–60. https://doi.org/10.1016/j.rasd.2019.01.003
Duval, S., & Tweedie, R. (2000). Trim and fill: A simple funnel-plot–based method of testing and adjusting for publication bias in meta-analysis. Biometrics, 56 , 455–463. https://doi.org/10.1111/j.0006-341X.2000.00455.x
Erwin, P. (1993). Friendship and peer relations in children . Wiley.
Farmer, T. W., Dawes, M., Hamm, J. V., Lee, D., Mehtaji, M., Hoffman, A. S., & Brooks, D. S. (2018a). Classroom social dynamics management: Why the invisible hand of the teacher matters for special education. Remedial and Special Education, 39 (3), 177–192. https://doi.org/10.1177/0741932517718359
Farmer, T. W., Lines, M. M., & Hamm, J. V. (2011). Revealing the invisible hand: The role of teachers in children’s peer experiences. Journal of Applied Developmental Psychology, 32 (5), 247–256. https://doi.org/10.1016/j.appdev.2011.04.006
Farmer, T. W., Talbott, B., Dawes, M., Huber, H. B., Brooks, D. S., & Powers, E. E. (2018b). Social dynamics management: What is it and why is it important for intervention? Journal of Emotional and Behavioral Disorders, 26 (1), 3–10. https://doi.org/10.1177/1063426617752139
Florian, L., Rose, R., & Tilstone, C. (Eds.). (2002). Promoting inclusive practice. Routledge.
Frostad, P., & Pijl, S. J. (2007). Does being friendly help in making friends? The relation between the social position and social skills of pupils with special needs in mainstream education. European Journal of Special Needs Education, 22 (1), 15–30. https://doi.org/10.1080/08856250601082224
Garrote, A., Dessemontet, R. S., & Opitz, E. M. (2017). Facilitating the social participation of pupils with special educational needs in mainstream schools: A review of school-based interventions. Educational Research Review, 20 , 12–23. https://doi.org/10.1016/j.edurev.2016.11.001
Gersten, R., Fuchs, L. S., Compton, D., Coyne, M., Greenwood, C., & Innocenti, M. S. (2005). Quality indicators for group experimental and quasi-experimental research in special education. Exceptional Children, 71 (2), 149–164. https://doi.org/10.1177/001440290507100202
Gresham, F. M., & Elliott, S. N. (1990). The social skills rating system . American Guidance Service.
Haegele, J. A., & Sutherland, S. (2015). Perspectives of students with disabilities toward physical education: A qualitative inquiry review. Quest, 67 (3), 255–273. https://doi.org/10.1080/00336297.2015.1050118
Hall, L. J., & McGregor, J. A. (2000). A follow-up study of the peer relationships of children with disabilities in an inclusive school. Journal of Special Education, 34 (3), 114–126. https://doi.org/10.1177/002246690003400301
Han, D. K., Kang, Y. S., & Yang, H. N. (2009). Developing a physical activity program to improve peer relationship of children with disabilities in inclusive education classes. Korean Journal of Special Education: Theory and Practice, 10 (2), 57–84.
Hay, D. F. (2005). Early peer relations and their impact on children’s development. Encyclopedia on Early Childhood Development, 1 (1), 1–6.
Hedges, L. V. (1981). Distribution theory for Glass’s estimator of effect size and related estimators. Journal of Educational Statistics, 6 (2), 107–128.
Heo, H., & Park, S. (2011). Effects of a class-wide peer supporter program on the friendship between children with and without disabilities and on the school adjustment of children with disabilities. Special Education Research, 10 (1), 155–186.
Inderbitzen, H. M., & Foster, S. L. (1992). The teenage inventory of social skills: Development, reliability, and validity. Psychological Assessment, 4 (4), 451.
Jang, M., & Kim, E. (2013). The trend of experimental studies on peer-mediated intervention for children with autism spectrum disorders. Korean Journal of Emotional & Behavioral Disorders, 29 (3), 311–336.
Kalymon, K., Gettinger, M., & Hanley-Maxwell, C. (2010). Middle school boys’ perspectives on social relationships with peers with disabilities. Remedial and Special Education, 31 (4), 305–316. https://doi.org/10.1177/0741932508327470
Kim, D., & Jung, K. (2005). The trends of inclusion program articles in Korea. Korean Journal of Special Education, 40 (2), 333–353.
Kim, D., Lim, J. H., & An, J. (2020). A Meta-analysis on effects of sociality interventions for individuals with intellectual disabilities. Korean Journal of Mental Retardation, 22 (4), 1–25.
Kim, D., Lim, J. H., & An, J. (2022). The quality and effectiveness of Social-Emotional Learning (SEL) intervention studies in Korea: A meta-analysis. PLoS ONE, 17 (6), e0269996. https://doi.org/10.1371/journal.pone.0269996
Kim, S. A., Park, C. W., & Lee, H. G. (2003). Characteristics of academic performance educational needs of college students with disabilities. Korean Journal of Special Education, 37 (4), 335–357.
Koster, M., Nakken, H., Pijl, S. J., Van Houten, E. J., & Lutje Spelberg, J. C. (2008). Assessing social participation of pupils with special needs in inclusive education: The construction of a teacher questionnaire. Educational Research and Evaluation, 14 , 395–409. https://doi.org/10.1080/13803610802337657
Koster, M., Timmerman, M. E., Nakken, H., Pijl, S. J., & van Houten, E. J. (2009). Evaluating social participation of pupils with special needs in regular primary schools: Examination of a teacher questionnaire. European Journal of Psychological Assessment, 25 (4), 213–222. https://doi.org/10.1027/1015-5759.25.4.213
Kuutti, T., Sajaniemi, N., Björn, P. M., Heiskanen, N., & Reunamo, J. (2022). Participation, involvement and peer relationships in children with special educational needs in early childhood education. European Journal of Special Needs Education, 37 (4), 587–602. https://doi.org/10.1080/08856257.2021.1920214
Lee, J. H. (2011). An analysis of research trends for intervention to promote relationships of young children with disabilities and without disabilities in inclusive educational environments: Focusing on experimental studies in Korea. Korean Journal of Special Education: Theory and Practice, 12 (2), 1–28.
Lee, J. E., & Lee, S. H. (2015). The impact of conflict resolution-oriented school violence prevention programs on use of conflict resolution strategies and attitudes toward school violence and students with disabilities in inclusive elementary school classes. Korean Journal of Intellectual Disabilities, 17 (1), 319–348.
Lee, K. R., & Park, J. K. (2007). A study on characteristics of social networks for handicapped college students and support ways. Korean Journal of Physical, Multiple, & Health Disabilities, 50 (1), 1–20.
Lee, S. J. (2007). A review of Korean research for intervention to support peer relationships of young children with disabilities in inclusive classrooms. Special Education Research, 6 (2), 47–65.
Lee, S. J., & Lee, S. H. (2011). The effects of disability awareness and inclusion activities for preschool classrooms embedded in preschool routine/activities on social acceptance and social engagement of children with and without disabilities. Korean Journal of Early Childhood Special Education, 11 (2), 1–32.
Special Education Act for Individuals with Disabilities and Others. (2008). Act No. 8852. https://elaw.klri.re.kr/eng_mobile/viewer.do?hseq=46388&type=part&key=16
Moon, H. W., & Park, J. Y. (2014). The effects of class-wide implementation of enhanced skill streaming on social skill, problem behaviors and interaction with peers in young children at risk for emotional and behavioral disorders. Special Education Research, 13 (1), 29–57.
Moon, S., Hong, J., & Kim, Y. (2016). A systematic review on peer relation intervention for children with autism spectrum disorders. Journal of the Korean Association for Persons with Autism, 16 (2), 83–109. https://doi.org/10.1111/1471-3802.12471
Ochoa, S. H., & Olivarez, A., Jr. (1995). A meta-analysis of peer rating sociometric studies of pupils with learning disabilities. Korean Journal of Special Education, 29 (1), 1–19. https://doi.org/10.1177/002246699502900101
Odom, S. L., Brantlinger, E., Gersten, R., Horner, R. H., Thompson, B., & Harris, K. R. (2005). Research in special education: Scientific methods and evidence-based practices. Exceptional Children, 71 (2), 137–148. https://doi.org/10.1177/001440290507100201
Odom, S. L., & Wolery, M. (2003). A unified theory of practice in early intervention/early childhood special education: Evidence-based practices. Journal of Special Education, 37 (3), 164–173. https://doi.org/10.1177/00224669030370030601
Okagaki, L., Diamond, K. E., Kontos, S. J., & Hestenes, L. L. (1998). Correlates of young children’s interactions with classmates with disabilities. Early Childhood Research Quarterly, 13 (1), 67–86. https://doi.org/10.1016/S0885-2006(99)80026-X
Orsmond, G. I., Krauss, M. W., & Seltzer, M. M. (2004). Peer relationships and social and recreational activities among adolescents and adults with autism. Journal of Autism and Developmental Disorders, 34 , 245–256. https://doi.org/10.1023/B:JADD.0000029547.96610.df
Orsmond, G. I., Shattuck, P. T., Cooper, B. P., Sterzing, P. R., & Anderson, K. A. (2013). Social participation among young adults with an autism spectrum disorder. Journal of Autism and Developmental Disorders, 43 , 2710–2719. https://doi.org/10.1007/s10803-013-1833-8
Park, Y. K., & Lee, J. Y. (2015). The effects of peer tutoring on students with disabilities in Korean context: A meta-analysis. Korean Journal of Special Education & Rehabilitation Science, 54 (4), 101–119.
Pearl, R., & Donahue, M. L. (2004). Peer relationships and learning disabilities. Learning about learning disabilities (pp. 133–165). Academic Press.
Chapter Google Scholar
Schoop-Kasteler, N., & Müller, C. M. (2020a). Peer relationships of students with intellectual disabilities in special needs classrooms–a systematic review. Journal of Research in Special Educational Needs, 20 (2), 130–145. https://doi.org/10.1111/1471-3802.12471
Schoop-Kasteler, N., & Müller, C. M. (2020b). Peer relationships of students with intellectual disabilities in special needs classrooms—a systematic review. Journal of Research in Special Educational Needs, 20 (2), 130–145.
Shin, H., & Kim, S. (2013). The effect of social story intervention including peer support on task-performance behavior of children with intellectual disabilities and their friendship relationship with general students. Korean Journal of Developmental Disabilities, 17 (4), 29–55.
Spence, S. (1995). Social skills training: Enhancing social competence with children and adolescents . Windsor: NFER-Nelson.
Sterne, J. A., & Egger, M. (2001). Funnel plots for detecting bias in meta-analysis: Guidelines on choice of axis. Journal of Clinical Epidemiology, 54 (10), 1046–1055. https://doi.org/10.1016/S0895-4356(01)00377-8
United Nations Educational. (2017). Scientific and Cultural Organization (UNESCO) . UNESCO.
van de Wetering, J., Leijten, P., Spitzer, J., & Thomaes, S. (2022). Does environmental education benefit environmental outcomes in children and adolescents? A Meta-Analysis. Journal of Environmental Psychology, 81 , 101782. https://doi.org/10.1016/j.jenvp.2022.101782
Vaughn, S., & Linan-Thompson, S. (2003). What is special about special education for students with learning disabilities? Journal of Special Education, 37 (3), 140–147. https://doi.org/10.1177/00224669030370030301
Vetoniemi, J., & Kärnä, E. (2021). Being included–experiences of social participation of pupils with special education needs in mainstream schools. International Journal of Inclusive Education, 25 (10), 1190–1204. https://doi.org/10.1080/13603116.2019.1603329
Webster, A. A., & Carter, M. (2007). Social relationships and friendships of children with developmental disabilities: Implications for inclusive settings. A systematic review. Journal of Intellectual and Developmental Disability, 32 (3), 200–213. https://doi.org/10.1080/13668250701549443
Wiener, J. (2004). Do peer relationships foster behavioral adjustment in children with learning disabilities? Learning Disability Quarterly, 27 (1), 21–30. https://doi.org/10.2307/1593629
Yang, Y. R., & Oh, K. J. (2005). Development of peer relational skills scale for 4th-9th grades. Korean Journal of Clinical Psychology, 24 (4), 961–971.
Yoo, G., & Yi, M. (2015). Validation of the friendship quality scale for pre-and early adolescence. Korean Journal of School Psychology, 12 (1), 57–71.
References included in a meta-analysis
An, E. J., Won, J. R., & Kim, S. H. (2017). The effect of the promoting relationship program embedded in daily routines on the social interaction and the peer acceptance of children with disabilities. Korean Journal of Early Childhood Special Education, 17 (4), 277–297. https://doi.org/10.21214/kecse.2017.17.4.277
Cha, Y. N. (2015). The effects of the social skills improvement program connected with the moral curriculum on social skills, peer relationships, and interpersonal problem-solving ability of elementary students with intellectual disabilities and their peers in inclusive classes . [Master’s thesis, Ewha Womans University].
Choi, J. H., Kim, H., Lee, J. S., & Yoo, D. H. (2018). Effect of sensory integration group therapy on sensory processing, peer interaction and task performance of children with intellectual disabilities. Journal of Korean Society of Occupational Therapy, 26 (4), 111–125. https://doi.org/10.14519/jksot.2018.26.4.09
Han, K. I. (2020). Effects of the situation-based empathic communication program implemented in a classroom on the elementary inclusive students’ empathy abilities, peer networks, disabilities acceptance attitudes, and empathic conversational behaviors of students. [Doctoral dissertation, Ewha Womans University].
Han, K. I., & Park, S. H. (2021). Effects of a situation-based empathic communication program on elementary students’ empathy abilities peer networks, attitudes toward students with disabilities, and empathic conversational behaviors. Korean Journal of Special Education, 56 (1), 105–138. https://doi.org/10.15861/kjse.2021.56.1.105
Hwang, B. S. (2012). The effects of a peer-buddy program on perception to social adjustment of university students with disabilities during leisure activities. Special Education Research, 11 (1), 147–169.
Jang, Y. (2011). The effects of peer relation improvement programs on peer competence and acceptance toward children with disabilities. [Master’s thesis, Kongju National University].
Kang, K. S., Choi, S. M., & Oh, K. Y. (2009). The effects of Integrative arts therapy on peer relation between children with/without disabilities. Korean Journal of Play Therapy, 13 (2), 99–112.
Kang, M. Y. (2016). The effects of musical production club activities on the friendship relationship of students with intellectual disabilities and students without disabilities. [Master’s thesis, Gyeongin National University of Education].
Kim, E. Y., Noh, S., Namgung, Y., & Kim, K. M. (2012). The effects of group sensory integrative intervention on sensory integration dysfunction children’s social interaction with peers and therapists. Journal Korean Academy of Sensory Integration, 10 (1), 1–10.
Kim, H. S., & Park, J. (2015). The effects of the ‘multi-level harmony program’ for social inclusion of students with disabilities on pro-social behavior, peer acceptance and class climate of elementary inclusive class students. Korean Journal of Emotional & Behavioral Disorders, 31 (3), 177–201.
Kwon, N. Y. (2019). The effect of school violence prevention UCC production club activity on social skills and self-expression of disabled students and non-disabled students' attitude towards school violence and disability. [Master’s thesis, Ewha Womans University].
Lee, J. W., & Jeon, S. S. (2018). Effects of small-group social skills training programs on social skills and peer play behavior of children with autism spectrum disorder in an inclusive environment. Korean Journal of Special Education, 34 (2), 43–72.
Lee, S. H. (2013). The effects of peer-supporter activity on friendship between the disabled student and the general students in an integrated classroom. [Master’s thesis, Kongju National University].
Moon, D. J. (2018). The effects of classwide compliment tree activities on peer relationship and classmate likability of children at risk for ADHD. [Master’s thesis, Gyeongin National University of Education].
Nam, K. (2009). The effects of collaborative art activities based on the theory of multiple intelligence for general children's acceptance attitude toward children with disabilities and the friendship between children with disabilities. [Master’s thesis, Gyeongin National University of Education].
Oh, K. Y. (2008). The effects of integrative arts therapy on peer relation, adaptive behavior and social skills. [Master’s thesis, Wan-gwang University].
Oh, S. Y. (2000). The effects of peer support network programs on friendships between disabled and nondisabled children. [Master’s thesis, Ewha Womans University].
Park, H. S. (2007). The impact of a peer-led interventional program on the student with disabilities and students without disabilities. [Master’s thesis, Ajou University].
Park. M. H. (2008). The effectiveness of a class-wide peer network program on the friendship between children with and without disabilities and general children's attitudes toward disabled people. [Master’s thesis, Gyeongin National University of Education].
Park, S., & Park, J. (2015). Effects of play-based self-expression training on the emotional competency and peer relationships of withdrawn children with intellectual disabilities and their peers in inclusive classrooms. Journal of Yeolin Education, 23 (3), 65–85.
Pyo, Y. H. (2020). The effects of integrated subjects activity with peers on the interaction and happiness of students with severe and multiple disabilities. Korean Journal of Physical, Multiple & Health Disabilities, 63 (1), 19–41. https://doi.org/10.20971/kcpmd.2020.63.1.19
Roh, H. Y. (2014). The effects of a self-regulation enhancement program on students’ self- control, peer support, and class participation in elementary inclusive classrooms . [Doctoral dissertation, Ewha Womans University].
Yang, E. (2005). A study on the effect of 'good friend program' on the friendship between students with and without disabilities at high school. [Master’s thesis, Ewha Womans University].
Yoo, H. (2017). The effectiveness of perspective taking ability improvement programs on peer relations of children with and without disabilities. [Master’s thesis, Gyeongin National University of Education].
Yoon, S. R. (2003). The effects of the social skills improvement program connected with the moral curriculum on social skills, peer relationships, and interpersonal problem-solving ability of elementary students with intellectual disabilities and their peers inclusive classes. [Master’s thesis, Kongju National University].
Download references
Author information
Authors and affiliations.
Department of Educational Psychology, University of Minnesota Twin Cities, Minneapolis, United States
Jechun An & Seohyeon Choi
School of Education, University of California, Berkeley, Berkeley, United States
Jin Hyung Lim
You can also search for this author in PubMed Google Scholar
Corresponding author
Correspondence to Jechun An .
Ethics declarations
Conflict of interest.
No conflict of interested declared by the authors with respect to the research, authorship, and/or publication of this article.
Ethical approval
This is a meta-analysis paper which synthesized data from previous published studies in which informed consent was obtained by primary investigators will be retrieved and analyzed. Therefore, ethics approval was not required for this meta-analysis paper.
Additional information
Publisher's note.
Springer Nature remains neutral with regard to jurisdictional claims in published maps and institutional affiliations.
Supplementary Information
Below is the link to the electronic supplementary material.
Supplementary file1 (DOCX 17 KB)
Rights and permissions.
Springer Nature or its licensor (e.g. a society or other partner) holds exclusive rights to this article under a publishing agreement with the author(s) or other rightsholder(s); author self-archiving of the accepted manuscript version of this article is solely governed by the terms of such publishing agreement and applicable law.
Reprints and permissions
About this article
An, J., Choi, S. & Lim, J.H. Peer relationship instructions in inclusive educational settings in Korea: a meta-analysis. Asia Pacific Educ. Rev. (2024). https://doi.org/10.1007/s12564-024-09971-4
Download citation
Received : 02 June 2023
Revised : 03 March 2024
Accepted : 26 April 2024
Published : 20 May 2024
DOI : https://doi.org/10.1007/s12564-024-09971-4
Share this article
Anyone you share the following link with will be able to read this content:
Sorry, a shareable link is not currently available for this article.
Provided by the Springer Nature SharedIt content-sharing initiative
- Peer relationship
- Inclusive education
- Students with disabilities
- Meta-analysis
- Find a journal
- Publish with us
- Track your research

IMAGES
VIDEO
COMMENTS
In peer instruction, instructors pose a challenging question to students, students answer the question individually, students work with a partner in the class to discuss their answers, and finally students answer the question again. A large body of evidence shows that peer instruction benefits student learning. To determine the mechanism for these benefits, we collected semester-long data from ...
Peer teaching among students has a powerful role in the learning process and has been studied and recognized as an effective teaching method. Research on peer teaching applied in different contexts has shown that learning through the process of teaching others increases students' academic performance.
David Boud of Stanford University explored the concepts of peer teaching, learning, and reciprocal peer learning in a short overview of existing research-which is limited. Though the context he discusses is primarily in the higher-ed domain where peer teaching is a literal component of most university learning models, the concepts transfer to ...
PEER LEARNING: WHAT THE RESEARCH SAYS Peer learning" can refer to any number of situations in which students interact with each other to learn, including one-on-one tutoring, classroom groups, writing workshops, semester-long project ... Handbook in the work's title Lmajor developments and syntheses in education research
Other research on peer instruction shows the same patterns: 41% of incorrect answers are switched to correct ones, ... Henderson C, Dancy MH. The impact of physics education research on the teaching of introductory quantitative physics in the United States. Physical Review Special Topics: Physics Education Research. 2009; 5 (2):020107. doi: ...
peer-teaching is reciprocal peer-learning; students simultaneously learning and sharing other students' learning and making equal contributions (Boud, 2001). ... is ample research to support the positive impact, peer-teaching has on a student 'self-confidence, and other leadership attributes (Morrison, 2004; Johnson, 2015; Lockie, &
WHAT IS LACKING? PEER TO PEER LEARNING. At the Micro level, four factors (Kumar et al., Citation 2019) that interact with each other are: Technology, Students, Courses, and Professors working toward achieving desired learning outcomes (Figure 1).For students, the sub-factors include motivation, culture, learning style, and IT skill level. For professors, the component factors include, but are ...
This is therefore a key area for future research. A recent review of peer education and peer counselling for health and well-being highlights how peer education interventions are inherently difficult to quality control and evaluate , partly due to what makes peer education attractive; peer education defies the conventions of traditional formal ...
University teachers do not comprise the view of peer teaching necessarily resulting in greater academic achievement gains or deep learning. University teachers identify and esteem other pedagogical benefits such as improving students': critical thinking, learning autonomy, motivation, collaborative and communicative skills.
Elias Avramidis. In this collaborative action research project three researchers and six primary teachers in two Greek mainstream schools developed a peer tutoring programme for 130 students, 11 of whom were students with special educational needs and disabilities (SEND). Through exploring new roles for researchers and teachers, the aim of this ...
Peer learning is an education method that helps students solidify their knowledge by teaching each other. One student tutoring another in a supervised environment can result in better learning and retention. Why? Because to teach another, one must first fully understand a concept themselves. Verbalizing a concept and sharing the information ...
Peer instruction, a form of active learning, is generally defined as an opportunity for peers to discuss ideas or to share answers to questions in an in-class environment, where they also have opportunities for further interactions with their instructor. When implementing peer instruction, instructors have many choices to make about group design, assignment format, and grading, among others ...
The peer teaching environment facilitated student interaction which allowed students to assume the role of active participants. Students laughed, talked, and interacted throughout the lessons, ... explore student perceptions of peer teaching. The research objectives were written to examine qualitatively three distinct aspects of peer teaching ...
This paper reports on the feedback of a case study on peer teaching acti vity in a third year unive r-. sity mathematics course. Th e objective of the peer -teaching activity was to motivate ...
Email address: [email protected]. Abstract — Peer learning is not only a teaching method but also a learning method created by educationists based on the psychological and. sociological ...
Research in Education provides a space for fully peer-reviewed, critical, trans-disciplinary, debates on theory, policy and practice in relation to Education. International in scope, we publish challenging, well-written and theoretically innovative contributions that question and explore the concept, practice and institution of Education as an object of study.
wide range of subject areas, education levels, and assessment types, most of them conclude that while peer grading was beneficial for tertiary students, it could be not beneficial for primary or secondary school students Double et.al (2019). In his research Kennedy (2006) also provided comments on the responses of students to a peer
Peer education (PE) Peer education is known as sharing of information and experiences among individuals with something in common (16, 17).It aims to assist young people in developing the knowledge, attitudes, and skills that are necessary for positive behavior modification through the establishment of accessible and inexpensive preventive and psychosocial support.
Peer review of teaching (PRT) has long been the subject of attention in higher education research. Some institutions use this as a mechanism for continuous professional development (CPD), while others as an indicator of teaching quality. It is evident that substantial work has been conducted in understanding PRT frameworks globally.
Peer education, whereby peers ('peer educators') teach their other peers ('peer learners') about aspects of health is an approach growing in popularity across school contexts, possibly due to adolescents preferring to seek help for health-related concerns from their peers rather than adults or professionals. Peer education interventions cover a wide range of health areas but their ...
discussed earlier in the study. Some main. benefits of peer tutoring which have a. considerable impact on learning include the. following. It provides opportunity to the students to. interact ...
Peer Observation of Teaching (PoT) that specifically supports a peer review and collegial approach can be a valuable tool to scaffold professional dialogue about, and reflection on, practice. ... This article explores PoT as a model to structure cross-institutional conversations about teaching and learning. Our research explored how to foster ...
Yanzheng Li and Zorka Karanxha examine the relationship between one specific model (transformational leadership) and student achievement. Focusing only on peer-reviewed journals published in English between 2006 and 2019, they found 14 articles.
Peer observation of teaching is a collaborative and formative process where instructors observe each other's teaching methods and classroom interactions in order to provide constructive feedback. It's an opportunity for mutual professional development, fostering an environment of continuous improvement and sharing of best practices among educators.
Background There is a scarcity of studies that quantitatively assess the difficulty and importance of knowledge points (KPs) depending on students' self-efficacy for learning (SEL). This study aims to validate the practical application of psychological measurement tools in physical therapy education by analyzing student SEL and course conceptual structure. Methods From the "Therapeutic ...
The Standards for Excellence in Education Research (SEER) Learn more about the Standards and access resources for making education research more transformational. Dashboards on Learning Modes and School Responses to COVID-19. Updates on modes of instruction offered, student enrollment, and schools' responses to the COVID-19 pandemic.
Alexandre Ardichvili is Professor and Hellervik Endowed Chair in Leadership and Adult Career Development at the University of Minnesota. He served as President of the University Council for Workforce and Human Resource Education, and Editor-in-chief of the Human Resource Development International. Alexandre received the Academy of Human Resource Development Outstanding HRD Scholar Award and ...
This study aimed to identify the effects of peer relationship instructions between students with and without disabilities in inclusive education settings in South Korea. We conducted a meta-analysis using journal articles published over the last 20 years. From a total of 1419 student data within 33 primary studies, we found an overall effect size of 0.78 (Hedges' g), which indicates a strong ...
Social work scholars have increasingly adopted community-based participatory research (CBPR) approaches to center community voice in research and action to promote youth's healthy development. While valuable contributions have emerged to engage in further dialectical learning processes, limited research has examined in depth the participation, critical reflection, and training of social work ...
an effective teaching method. Research on peer teaching applied in different contexts has shown that learning through the process of teaching others increases students' academic performance. In Indonesia, some research on peer teaching has been conducted, but there has been no research on peer teaching in the context of theological studies ...