Effects of stress on immune function: the good, the bad, and the beautiful
Affiliation.
- 1 Department of Psychiatry and Behavioral Sciences, Institute for Immunity, Transplantation, and Infection, Neurosciences Institute, Cancer Institute, Stanford University, 300 Pasteur Drive, MC 5135, Stanford, CA, 94305-5135, USA, [email protected].
- PMID: 24798553
- DOI: 10.1007/s12026-014-8517-0
Although the concept of stress has earned a bad reputation, it is important to recognize that the adaptive purpose of a physiological stress response is to promote survival during fight or flight. While long-term stress is generally harmful, short-term stress can be protective as it prepares the organism to deal with challenges. This review discusses the immune effects of biological stress responses that can be induced by psychological, physiological, or physical (including exercise) stressors. We have proposed that short-term stress is one of the nature's fundamental but under-appreciated survival mechanisms that could be clinically harnessed to enhance immunoprotection. Short-term (i.e., lasting for minutes to hours) stress experienced during immune activation enhances innate/primary and adaptive/secondary immune responses. Mechanisms of immuno-enhancement include changes in dendritic cell, neutrophil, macrophage, and lymphocyte trafficking, maturation, and function as well as local and systemic production of cytokines. In contrast, long-term stress suppresses or dysregulates innate and adaptive immune responses by altering the Type 1-Type 2 cytokine balance, inducing low-grade chronic inflammation, and suppressing numbers, trafficking, and function of immunoprotective cells. Chronic stress may also increase susceptibility to some types of cancer by suppressing Type 1 cytokines and protective T cells and increasing regulatory/suppressor T cell function. Here, we classify immune responses as being protective, pathological, or regulatory, and discuss "good" versus "bad" effects of stress on health. Thus, short-term stress can enhance the acquisition and/or expression of immunoprotective (wound healing, vaccination, anti-infectious agent, anti-tumor) or immuno-pathological (pro-inflammatory, autoimmune) responses. In contrast, chronic stress can suppress protective immune responses and/or exacerbate pathological immune responses. Studies such as the ones discussed here could provide mechanistic targets and conceptual frameworks for pharmacological and/or biobehavioral interventions designed to enhance the effects of "good" stress, minimize the effects of "bad" stress, and maximally promote health and healing.

Publication types
- Research Support, N.I.H., Extramural
- Research Support, Non-U.S. Gov't
- Adaptation, Physiological
- Autoimmune Diseases / etiology
- Cytokines / metabolism
- Immunity / physiology*
- Immunomodulation
- Neoplasms / etiology
- Stress, Physiological*
- Stress, Psychological*
- Time Factors
Grants and funding
- AR46299/AR/NIAMS NIH HHS/United States
- CA107498/CA/NCI NIH HHS/United States
- R01 AI048995/AI/NIAID NIH HHS/United States
- AI48995/AI/NIAID NIH HHS/United States
- R01 CA107498/CA/NCI NIH HHS/United States
The Impact of Everyday Stressors on the Immune System and Health
- Open Access
- First Online: 28 November 2019
Cite this chapter
You have full access to this open access chapter
- Annina Seiler 2 ,
- Christopher P. Fagundes 3 , 4 &
- Lisa M. Christian 5 , 6
68k Accesses
47 Citations
344 Altmetric
Research over the past three to four decades has clearly established that psychological stress affects clinically relevant immune system outcomes, including inflammatory processes, wound healing, and responses to infectious agents and other immune challenges (e.g., vaccinations, autoimmunity, cancer). Individuals vary in their ability to cope with stressful life events, and differences in perceptions of stress, mood (e.g., depressive symptoms), and adverse life events can modify the magnitude to which stressors exert a negative influence on immune function. In this chapter, we provide an overview of key findings linking everyday stressors to immune function and health. In addition, the complexity of a plausible mechanism through which chronic stress and elevated inflammation might result in serious health consequences including cardiovascular disease, diabetes, and cancer is delineated. The literature provides support for several promising avenues for interventions to prevent stress-induced immune dysregulation. Research examining stressors in everyday life also has strong implications with respect to understanding the effects of more extreme stressors, such as those encountered during space flight, a complex environmental, physiological, and psychological challenge with multiple adverse consequences for human health.
You have full access to this open access chapter, Download chapter PDF
Similar content being viewed by others
Psychoneuroimmunology: How Chronic Stress Makes Us Sick
Stress and the Immune System
Emotion, Interventions, and Immunity
1 stress, immunity, and health.
The central nervous system (CNS), endocrine system, and immune system are complex systems that interact with each other. Stressful life events and the negative emotions they generate can dysregulate the immune response by disturbing the sensitive interplay among these systems (Glaser and Kiecolt-Glaser 2005 ). Psychoneuroimmunology (PNI) is a field of investigation concerned with the interactions of psychological factors with the neuroendocrine and immune system and consequences for higher brain function and human behavior (Dantzer 2010 ).
A stressor can be defined as an event that exceeds an individual’s perceived ability to cope (Lazarus and Folkman 1984 ) and can result in an allostatic load and overload (see Chap. 4 ). Individual differences exist in the extent to which people mount a physiological stress response. Individual differences in stress physiology are, among other things, related to the brain, which plays a critical role in appraising stressors, as well as in modulating immune system reactivity to physical and social threats (Slavich and Irwin 2014 ). Additionally, certain characteristics of a situation are associated with greater stress responses, including the intensity, severity, controllability, and predictability of the stressor. Physiological reactivity to stressors are commonly observed even after repeated exposure to the same stressor (Dhabhar 2014 ).
The autonomic nervous system (ANS) and the hypothalamic-pituitary-adrenal (HPA) axis are two major stress-signaling pathways that contribute to immune dysregulation (Glaser and Kiecolt-Glaser 2005 ). Experiencing a stressful situation, as perceived by the brain, activates the HPA axis and the sympathetic-adrenal medullary axis (SAM), which provokes the release of hormones which modulate immune function including adrenocorticotropic hormone (ACTH), cortisol, growth hormone, prolactin, epinephrine, and norepinephrine (Glaser and Kiecolt-Glaser 2005 ) (see Chap. 7 ).
Immunity is the natural or acquired resistance of an organism to bacterial or viral invaders, diseases, or infections, while having adequate tolerance to avoid allergy, and autoimmune diseases. Lymphocytes, including T and B cells are the main type of cells of the immune system. T cells orchestrate the immune response via the production of cytokines and stimulate B cells to produce antibodies and signal killer cells to destroy the antigen-displaying cell (Sompayrac 2016 ). Helper T cells (Th) can be separated into Th1 cells, which primarily produce IL-2, IFN-γ and TNF, and Th2 cells, which produce IL-4, IL-5, IL-6, IL-10, and IL-13. Typically, type 1 cytokines favor the development of a strong cellular immune response, whereas type 2 cytokines favor a strong humoral immune response (Spellberg and Edwards Jr. 2001 ). Chronic stress can suppress or dysregulate innate and adaptive immune responses by altering the type 1/type 2 cytokine balance, thereby inducing low-grade inflammation and suppressing the function of immuno-protective cells (Dhabhar 2014 ).
A primary focus of the field of psychoneuroimmunology has been to understanding the link between stress and inflammatory responses. Although acute inflammation is an adaptive response to physical injury or infection, exaggerated and/or prolonged inflammatory responses are detrimental to health (Dhabhar 2014 ). Chronic inflammation secondary to long-term stress has been causally linked with risk for numerous diseases, including infectious illnesses, cardiovascular disease, diabetes, certain cancers, and autoimmune disease, as well as general frailty and mortality (Glaser and Kiecolt-Glaser 2005 ; Dhabhar 2014 ; Padro and Sanders 2014 ; Webster Marketon and Glaser 2008 ). One potential explanation for the mechanism linking chronic stress and inflammation in the onset of a wide range of diseases is that prolonged stressors result in glucocorticoid receptor resistance, which, in turn, causes dysregulated HPA axis function and interferes with the appropriate regulation of inflammation (Cohen et al. 2012 ).
Animal models have provided compelling evidence that biobehavioral stress mechanisms and their molecular and cellular pathways can cause illness behavior and illness itself. These experimental studies have conclusively demonstrated that exposure to restraint stress triggers exaggerated inflammatory responses (Korte et al. 1992 ; Ahlers et al. 1980 ; Bartolomucci et al. 2003 ). In addition, pharmacological experiments have amply demonstrated that mice injected with proinflammatory cytokines, including IL-1β or TNF, have decreased motor activity, social withdrawal, reduced food and water intake, increased slow-wave sleep, altered cognition, and increased pain sensitivity (Bluthe et al. 2000 ; Dantzer 2009 ). These experiments highlight how conditions of chronic inflammation can induce sickness and depressive-like behaviors in response to chronic stress (Dantzer et al. 2008 ).
2 Stress and Wound Healing
Wound healing is a vitally important process during recovery from either injury or surgery. Poor healing is associated with increased risks for wound infections and other complications, patient discomfort, prolonged hospital stays, and delays in one’s return to normal activities (Tevis and Kennedy 2013 ). Converging evidence from observational, experimental, and interventional studies implies that stress and other behavioral factors can impede wound healing processes and compromise immunity via multiple physiological pathways (Kiecolt-Glaser et al. 1998 ; Gouin et al. 2008 ; Ebrecht et al. 2004 ; Pinto et al. 2016 ; Walburn et al. 2009 ).
Wound healing progresses through several sequential and overlapping phases, including inflammation, proliferation, and regeneration. Cellular immunity plays an important role in the regulation of wound healing through the production of proinflammatory cytokines and chemokines (e.g., platelet derived growth factor [PDG]; transforming growth factor [TGF]; vascular endothelial growth factor [VEGF]; TNF; IFN-γ; IL-1β; IL-8), which mediate many of the complex interactions involved in wound healing. These factors act as chemo-attractants for the migration of phagocytes and other cells to the wound site, starting the proliferative phase which involves the recruitment and replication of cells necessary for tissue regeneration and capillary regrowth (Gethin 2012 ). Inflammation is a prerequisite to healing. Proinflammatory cytokines help to protect against infection and prepare injured tissue for repair by enhancing the recruitment and activation of phagocytes. Unfortunately, stress disrupts the production of proinflammatory cytokines that are essential for wound healing and, when dysregulated, impose a considerable delay in wound repair (Gouin and Kiecolt-Glaser 2011 ).
The clinical relevance of the relationship between stress and impaired wound healing has been demonstrated in several studies. In one experiment, individuals with a “slow healing” speed had higher stress and higher cortisol levels at awakening, implicating a key role of elevated cortisol levels in the process of cutaneous wound healing (Ebrecht et al. 2004 ). A meta-analysis (Walburn et al. 2009 ) corroborated these findings, synthesizing 17 articles that documented how stress is significantly associated with impaired healing and dysregulation of biomarkers crucial to wound healing. In addition to this meta-analysis, a statistically significant and moderately strong inverse correlation of r = −0.42 (95% CI = −0.51 to −0.32; p < 0.01) was calculated between the level of stress and speed of wound healing. These results confirm earlier findings by Kiecolt-Glaser et al. ( 1995 ), who observed that women experiencing the long-term stress of caring for a relative with Alzheimer’s disease, took 24% longer than controls to heal a small, standardized dermal wound. In addition, the peripheral blood leukocytes of caregivers produced less IL-1β in response to lipopolysaccharide (LPS) stimulation.
Surgical complications (e.g., postoperative pain; permanent disfigurement) pose significant challenges to surgical patients and may contribute to psychological distress, anxiety, and depression (Pinto et al. 2016 ). In an observational study involving patients undergoing coronary artery bypass graft (CABG) surgery, individuals with more depressive symptoms at discharge had more infections and poorer wound healing over the first six weeks following surgery than individuals who reported less distress (Doering et al. 2005 ). In addition, the pain associated with surgery can itself generate psychological distress, which has been shown to further influence wound healing. A prospective study involving 17 women who underwent elective gastric bypass surgery revealed that greater acute pain immediately after surgery and persistent pain in the four weeks following surgery both were associated with slower healing (McGuire et al. 2006 ).
In summary, acute and chronic stressors can negatively impact the wound healing process, by interrupting the inflammatory cascade that is fundamental for wound repair. These findings highlight the importance of addressing patients’ psychological needs in a timely manner, if possible both before and immediately after surgery, so as to prevent stress-related immune disruption.
3 Stress and Infectious Agents
Stress can also dysregulate humoral and cellular immune responses to pathogens, increasing risk for infectious illnesses including influenza and the common cold (Glaser and Kiecolt-Glaser 2005 ). The association between psychological stress and susceptibility to the common cold has long been recognized; stress suppresses the host resistance to infection and increases rates of infection (Cohen et al. 1991 ). Loneliness is another well-established risk factor for poor physical health. In a study of our own, we were able to demonstrate that loneliness predicts self-reported cold symptoms after a viral challenge, suggesting that cold symptoms are more severe among those who feel lonely (LeRoy et al. 2017 ).
Vaccination against influenza virus reduces both risk and severity of infection, thus decreasing risk for hospitalization and death. Vaccine effectiveness is of particular importance among high-risk groups, including pregnant women and older adults. However, the protective efficacy of antiviral vaccines depends upon their ability to induce both humoral and cell-mediated immune responses (Lambert et al. 2012 ).
A meta-analysis of 13 studies concluded that the effect of stress on antibody responses to influenza virus vaccination corresponded to adequate antibody responses among 41% of stressed individuals versus 59% of less-stressed individuals with similar effects among older and younger adults (Pedersen et al. 2009 ). Furthermore, psychological distress and biobehavioral vulnerabilities, which arise from being older or sedentary, have independently been found to alter immune responses to influenza vaccination (Segerstrom et al. 2012 ). In addition, studies in adults and adolescence have confirmed that negative emotions, including anxiety and depression, can modulate the antibody and T-cell responses to antiviral vaccinations, resulting in suppressed immune responses (O’Connor et al. 2014 ; Coughlin 2012 ). Interestingly, a 4-week massage intervention in students embarking on academic examinations was associated with reduced distress and enhanced antibody responses after a hepatitis B vaccine (Loft et al. 2012 ). Positive effects of other mind-body therapies, including Tai Chi, Qi Gong, meditation, and Yoga, on the immune system and virus-specific antibody responses to vaccines have also been documented in a meta-analysis of 34 studies (Morgan et al. 2014 ).
Herpes viruses, including herpes simplex virus (HSV) I and II, varicella-zoster virus (VZV), Epstein-Barr virus (EBV), and cytomegalovirus (CMV), assume a latent state after the initial infection (Grinde 2013 ). After primary infection, the herpes virus continues to reside in B lymphocytes and white blood cells for the life of the individual. Under normal health conditions, reactivation and replication of the EBV virus is prevented by the cellular immune system, largely orchestrated through specific-memory cytotoxic T cells and natural killer (NK); thus, individuals with herpesvirus infections generally remain asymptomatic (Glaser et al. 1993 ). However, under stressful conditions, suppressive immune activity may be reduced, permitting reactivation of the virus.
The relationships between neuroendocrine activity, immune function, and latent HSV type 1 reactivation were initially documented in animal studies. Among mice infected with HSV type 1, those exposed to a stressor exhibited reactivation of the latent virus, whereas nonstressed mice did not (Padgett et al. 1998 ). Today, a body of literature in humans confirms that psychosocial stressors predict reactivation of latent viruses (see Chap. 19 ). For instance, higher self-reported health was associated with lower reactivation of latent herpesviruses and inflammation (Murdock et al. 2016 ). Meanwhile, increased antibody titers against EBV viral capsid antigen (VCA) have been observed in the context of depression (Bennett et al. 2012 ), perceived stress (Brook et al. 2017 ), childhood adversity (Fagundes et al. 2013a ), bereavement or divorce (Derry et al. 2012 ), exam stress (Matalka et al. 2000 ), attachment anxiety (Fagundes et al. 2014 ), and perceived discrimination (Christian et al. 2012 ). Together, these human and animal studies show that stress can modulate the steady-state expression of latent herpesviruses, downregulating specific T-cell responses to the virus to an extent that is sufficient to result in viral activation.
Human immunodeficiency virus (HIV) is similar to herpes viruses, in that the virus remains in a latent state in the body after primary infection. As individuals infected with HIV may have lowered levels of T cells, cells that are important to fight infections, much interest exists in whether chronic stress and depression—that also are known to suppress the human immune system—may affect HIV disease progression. Indeed, there is a substantial body of evidence pointing at a relationship between chronic stress and the rate of HIV disease progression. In particular, stressful life events are considered to exert important impacts on certain biological markers of the disease: viral load and CD4 cell count (Kołodziej 2016 ). For instance, HIV-infected persons with posttraumatic stress disorders (PTSD) after Hurricane Katrina were more likely than those without PTSD to have detectable plasma viral loads and CD4 cell counts <200 mm −3 at 12 and 14 months, as well as two years post disaster (Reilly et al. 2009 ).
Major depression is highly prevalent among HIV-positive patients. Depression is associated with, among other factors, increased inflammatory markers (e.g., CRP; IL-1β; IL-6, TNF) (Slavich and Irwin 2014 ), which may alter the function of lymphocytes and decrease NK activity, contributing to HIV disease progression and mortality in these patients (Arseniou et al. 2014 ). These findings are corroborated by a study that investigated norepinephrine, cortisol, depression, hopelessness, coping, and life event stress as predictors of HIV progression in a diverse subject sample every 6 months over a period of 4 years. The authors found that norepinephrine, depression, hopelessness, and avoidant coping significantly predicted a greater rate of decrease in CD4 and increase in viral load, demonstrating a robust effect of chronic stress on HIV disease progression (Ironson et al. 2015 ).
In summary, stress can not only increase susceptibility to illness after exposure to infectious agents but also can inhibit antibody and virus-specific T cell responses to vaccines, permit reactivation of latent herpesviruses, and influence the progression of HIV-related disease.
4 Stress and Cardiovascular Disease
Cardiovascular disease (CVD) is a major cause of morbidity and mortality. Chronic low-grade inflammation is implicated in the link between stress and CVD via contributions to the early emergence, progression, and thrombotic complications of atherosclerosis (Liu et al. 2017 ). IL-6 and CRP, two important biomarkers of inflammation, are thought to be indicative and potentially predictive of atherosclerosis (Nadrowski et al. 2016 ). Of clinical importance, the biological effects of stress do not exist in isolation, and are often aggravated by unhealthy behaviors including poor diet, inadequate physical activity, tobacco use, and poor adherence to medication (Lagraauw et al. 2015 ).
Epidemiological research over the last half-century has conclusively linked chronic stress and other psychosocial factors to the increased incidence of coronary artery disease (von Kanel 2012 ). For instance, individuals exposed to work-related stressors including shiftwork, workplace conflict, and positions typified by high demands combined with low control, exhibit risk for elevations in serum CRP and IL-6 (von Kanel et al. 2008 ), as well as CVD (Kivimaki and Kawachi 2015 ). Furthermore, evidence suggests that childhood adversity, particularly severe physical and sexual abuse, confers risk for cardiovascular events, particularly among women (Garad et al. 2017 ). Similarly, among adults with greater childhood adversity/trauma, elevated risk for depressive symptoms, higher serum CRP, reduced methylation of the IL-6 promoter, and higher serum IL-6 have been observed (Janusek et al. 2017 ). These results shed light on potential epigenetic mechanisms that could link childhood adversity to disproportionally elevated risks of inflammatory disease in adulthood.
5 Stress and Metabolic Disease
Type-2 diabetes mellitus (T2DM) is a chronic metabolic disorder that results from defects in insulin secretion and insulin action (Hackett and Steptoe 2017 ). Though limited, an emerging body of literature suggests that stress plays a role in the etiology of T2DM, both as a predictor of new-onset T2DM and as a prognostic factor in individuals with existing T2DM (Hackett and Steptoe 2017 ). Stress-related biological pathways, including chronic activation of the HPA axis, which can lead to dysregulated cortisol output and neuroendocrine dysfunction, have been conjectured to contribute to the pathogenesis of T2DM (Hackett and Steptoe 2017 ). For instance, insulin resistance frequently develops during acute or chronic stress (Tsuneki et al. 2013 ). Moreover, obesity commonly co-occurs in patients with T2DM, and visceral adipose tissue (e.g., adipokines) is a major source of inflammation, including CRP, IL-1β and IL-6 (Donath and Shoelson 2011 ), supporting a link between T2DM and inflammation.
Results from meta-analyses suggest that depression further contributes to an increased risk of diabetes mellitus (Bădescu et al. 2016 ; Yu et al. 2015 ). Stress exposure during childhood has also been found to constitute a risk factor for obesity and diabetes. Experiencing an adverse childhood experience increases a child’s risk of type 1 diabetes during childhood (Nygren et al. 2015 ). Likewise, a review of literature revealed a significant association between exposure to childhood adversity and an increased risk of T2DM in adulthood (Huffhines et al. 2016 ; Hughes et al. 2017 ), with the effects of neglect and sexual abuse most prominent (Huang et al. 2015 ). Of particular note, stress can perinatally impair metabolic health in later life. Fetal exposure to high concentrations of maternal glucocorticoids, as well as obesity, have been associated with low birth weight, which in turn is associated with increased risk for hypertension, diabetes, and cardiometabolic diseases during adulthood (Zöller et al. 2015 ; Capra et al. 2013 ). However, the mechanisms for this effect are not yet fully understood. One novel potential pathway linking maternal and child weight is the transmission of obesogenic microbes from mother to child (Galley et al. 2014 ).
6 Stress and Cancer
Research over the past 30 years in the field of psychoneuroimmunology has contributed to considerable understanding of the effect of stress on cancer biology, and has identified psychosocial factors including stress, depression, and the lack of social support as risk factors for tumor progression (Moreno-Smith et al. 2010 ). Stress hormones (e.g., glucocorticoids, norepinephrine, epinephrine) have multiple effects on human tumor biology. Thus, via adrenergic- and glucocorticoid-mediated mechanisms, sympathetic nervous system (SNS)-activation may alter immune defenses mechanisms and anti-tumor immune capabilities with implications for tumor progression (Antoni et al. 2006 ; Lutgendorf and Andersen 2015 ; Armaiz-Pena et al. 2013 ). For instance, exposure to chronic stress (Lamkin et al. 2012 ) as well as the pharmacological stimulation of SNS pathways with a β-adrenergic agonist (e.g., isoproterenol) (Sloan et al. 2010 ) in tumor-bearing animals, significantly enhances tumor progression and metastasis, implying a fundamental role of stress hormones and β-adrenergic receptor signaling in both processes. Furthermore, both animal and human studies have consistently revealed that the negative effects of stress on tumor cell dissemination can be abrogated using a β2-adrenergic receptor antagonist (e.g., propranolol), supporting the use of β-blockers to modulate cancer metastasis (Sloan et al. 2010 ; Shaashua et al. 2017 ).
Chronic stress can increase inflammation and alter protective immune responses, and thereby may increase susceptibility to certain types of cancer by suppressing type 1 cytokines and protective T cells, and increasing regulatory/suppressor T-cell function (Dhabhar 2014 ). Correspondingly, increased catecholamine levels have been linked to T lymphocyte apoptosis (Radojevic et al. 2014 ), altered distribution of NK (see also Chap. 13 ) and granulocytes, and suppressed NK activity (Elenkov and Chrousos 2002 ), all important defense mechanisms against tumors and their metastasis (see also Chap. 13 ). It has become clear that cancer-related systemic inflammation is associated with poor outcomes, independent of tumor stage (Dolan et al. 2017 ). Several inflammatory mediators including IL-6, IL-12, IFN-γ, and TNF are implicated in tumor growth and progression (Cash et al. 2015 ; Landskron et al. 2014 ).
The immune system plays a critical role in the occurrence and progression of immunogenic tumors, including skin cancer (Song et al. 2016 ). For instance, an increased immune response reflected by enhanced expression of intercellular adhesion molecule (ICAM) 1 and infiltration of CD68+ cells (macrophages) surrounding, or within the tumor, have been observed during basal cell carcinoma (BCC) tumor regression following treatment (De Giorgi et al. 2009 ; Urosevic et al. 2003 ). Furthermore, immunosuppression, such as in solid-organ transplant recipients and patients with human immunodeficiency virus (HIV) or hematologic malignant neoplasms, has been clearly linked with increased incidence of non-melanoma skin cancer, including BCC, and squamous cell carcinoma (Song et al. 2016 ; Jensen et al. 2009 ).
Importantly, chronic stress can alter the anti-tumor-specific immune response to immunogenic tumors. Our own data demonstrate that emotional maltreatment in childhood and occurrence of a major life event in adulthood, showed poorer immune responses to BCC as indexed by suppressed expression of messenger RNA (mRNA) immune markers (CD25, CD3ε, ICAM-1, and CD68) to BCC (Fagundes et al. 2012 ). Animal models support these findings; mice under restraint stress developed ultraviolet-light (Illi et al. 2012 ) -induced squamous cell carcinoma more rapidly and showed a poorer immune response [as assessed by messenger RNA (mRNA) in their tumors] relative to nonstressed control mice (Saul et al. 2005 ). Taken together, these preclinical and clinical studies provide evidence that behavioral stressors can influence the tumor microenvironment.
While much initial work focused on direct effects of catecholamines and other stress mediators on cancer progression, subsequent work identified that the tumor microenvironment is a critical regulator of cancer progression and metastasis (Landskron et al. 2014 ; Wang et al. 2017 ; Berghoff and Preusser 2015 ). The tumor microenvironment has a pivotal role in regulating tumor cell growth, invasion, and metastasis, specifically through reciprocal cross-talk with infiltrating immune cells (lymphocytes, neutrophils, and macrophages), endothelial cells, mesenchymal stromal cells (fibroblasts and myofibroblasts), and their secretory products, all of which can modulate gene expression and alter the behavior of tumor cells (Mostofa et al. 2017 ).
7 Contextual Factors and Immune-Dysregulation
7.1 stressful life events.
Many investigators have studied pathways between major life events and inflammation. Caring for a loved one with a chronic medical condition, such as a spouse with dementia, is commonly characterized by significant life changes and social isolation (Holmes and Rahe 1967 ). The chronic stress of caregiving has been linked with exacerbation of typical age-related increases in serum levels of IL-6 and CRP (Gouin et al. 2012 ), providing a plausible physiological pathway via which chronic stress may lead to poor health. Analogously, the loss of a spouse is considered one of the most stressful life events one may encounter (Holmes and Rahe 1967 ). Indeed, bereavement has been associated with increased inflammation (Buckley et al. 2012 ; Cohen et al. 2015 ) as well as elevated rates of chronic inflammatory conditions, including type 2 diabetes, cardiovascular disease, and cancer within the first three years following the death (Stahl et al. 2016 ).
Particularly strong evidence indicates that trauma exposure during adulthood increases risks for psychiatric morbidity and poor health outcomes, and there is emerging evidence that inflammation contributes to this link (Flory and Yehuda 2015 ). Trauma exposure and posttraumatic stress disorder (PTSD, see Chap. 7 ) have been linked to increased risks of both depression (Dunn et al. 2017 ) and cardiovascular disorders (Edmondson and von Kanel 2017 ). The prevalence of trauma-related inflammation was addressed in a review paper, providing evidence for elevated systemic inflammation in individuals with PTSD, with this effect especially strong among those with comorbid PTSD and depression (Baker et al. 2012 ). In another study involving survivors of the World Trade Center attacks on September 11, 2001, altered salivary cortisol responses to trauma activation (induced by trauma recollections through a standardized interview) were observed, with stronger effects documented in those with comorbid PTSD and depression (Dekel et al. 2017 ).
7.2 Adverse Childhood Experiences
Early adversity confers risk for physical and mental illness (e.g., depression, cardiovascular disease, type 2 diabetes, cancer) in adulthood (Ziol-Guest et al. 2012 ; Ehrlich et al. 2016 ) with more robust effects amongst those experiencing multiple adversities (Hughes et al. 2017 ). Inflammatory pathways are implicated in these links; meta-analyses of 25 studies concluded that early life adversity contributes to significantly elevated peripheral CRP, IL-6, and TNF in adulthood (Baumeister et al. 2016 ). Most interestingly, different types of trauma exposure impacted inflammatory markers differentially: physical and sexual abuse were associated with significantly increased TNF and IL-6, but not CRP (Baumeister et al. 2016 ). Similar results were reported by Lin et al. ( 2016 ), who found that adults who had experienced childhood adversity had elevated levels of CRP and were almost three times as likely to have experienced trauma as an adult, relative to those without adverse childhood experiences.
Health behaviors (e.g., smoking and obesity) appear to partially mediate this relationship. For instance, in one study it was shown that early adversity predicted increased smoking and BMI through ongoing chronic stress in young adulthood (Raposa et al. 2014 ). In the same study, higher BMI predicted higher levels of soluble TNF receptor type II (sTNF-RII) and CRP, suggesting that early adversity contributes to inflammation, in part through ongoing stress and maladaptive health behaviors. In accordance with this, several previous studies provide evidence that specific early adversity, including low socioeconomic status in childhood (Brummett et al. 2013 ; Hagger-Johnson et al. 2012 ) and childhood abuse (Matthews et al. 2014 ), affects CRP through unhealthy behaviors and increased BMI.
Evidence also supports a role for heightened emotional and physiological reactivity to stress, which in turn drives the expression of an increasingly proinflammatory phenotype (Slavich and Irwin 2014 ). In accordance with this assumption, Shapero et al. ( 2014 ) reported that individuals with more severe childhood emotional abuse experienced greater increases in depressive symptoms when confronted with a stressor, implying the importance of emotional abuse as an indicator of reactivity to stressful life events. In addition, individuals who have experienced childhood adversities may have fewer social and psychological resources available to them for coping with stress (Fagundes et al. 2013b ).
Taken together, intense and chronic stress experienced during one’s developmental years appears to have long-lasting neurobiological effects and increases one’s risk of later morbidity (e.g., anxiety, depression, and physical disorders) and mortality (Raposa et al. 2014 ; Fagundes et al. 2013b ). Another important effect is that stress exposure during childhood might alter behavioral and physiological responses to acute and chronic stress in adulthood, which may determine one’s later risk of disease.
7.3 Pregnancy
The prenatal period is a critical time for neurodevelopment and, as such, represents a period of vulnerability during which a wide range of exposures has been found to exert long-term effects on brain development and behaviors (Christian 2012 ). Maternal psychosocial stress during pregnancy is associated with risks to maternal health and birth outcomes, as well as to various adverse health and behavioral outcomes in the offspring (Christian 2015 ). During pregnancy, the immune system undergoes substantial adaptations. Under normal circumstances, pregnancy is characterized by elevations in circulating inflammatory mediators relative to nonpregnancy (Christian and Porter 2014 ). However, excessive inflammation or deviations in inflammatory trajectories of change across pregnancy have been associated with gestational hypertension, miscarriages, preterm births, and adverse influences on fetal development (Christian 2012 ).
Stress, anxiety, and depression in pregnancy are considerable risk factors for adverse outcomes for both mothers and babies, and are associated with shorter gestation and impaired fetal neurodevelopment and child outcomes (Christian 2012 ). Inflammation is a likely mechanism by which stress may promote these negative health outcomes (Christian et al. 2009 ).
Inflammatory responses to influenza virus vaccine have been shown to be mild, transient, and generally similar in pregnant and nonpregnant women (Christian et al. 2013a ). Since it is considered safe and recommended for pregnant women, seasonal influenza vaccination provides a useful model with which to study individual differences in inflammatory responses during pregnancy. In one influenza-virus vaccine study in pregnant women, Christian et al. ( 2013a ) demonstrated that women in the highest percentile of depressive symptoms had markedly higher inflammatory responses, as indicated by elevated serum levels of macrophage migration inhibitory factor (MIF) one week post-vaccination, indicating that women with depressive symptoms may be more vulnerable to negative sequelae of infectious illness during pregnancy. Similarly, in pregnant women, greater EBV reactivation has been reported in association with maternal depression, perceived distress, and perceived racial discrimination. Notably, this effect was significantly stronger among African American women who reported greater racial discrimination (Christian et al. 2012 ).
Other risk factors can mediate the association between chronic stress and inflammation in pregnant women. Obesity, conceptualized as a physiological stress, has been linked to considerable increases in circulatory inflammatory markers, particularly IL-6, throughout pregnancy and postpartum. Moreover, psychological stress and obesity may interact synergistically, resulting in more pronounced effects among women with both risk factors (Mitchell and Christian 2018 ). In addition, obesity has been observed to increase the risks of gestational hypertension and gestational diabetes via inflammatory pathways. Most importantly, obesity-induced inflammation is transmitted to the child, and can potentially affect their immune function, metabolism, and cognitive development (Christian 2015 ). In addition, it is well established that poor sleep triggers inflammation. Accordingly, sleep-induced immune dysregulation has been found to be predictive of preterm birth. This effect, again, was especially pronounced in African American women (Blair et al. 2015 ). Indeed, the relationship between stress-induced inflammatory responses has been found to be more robust in racial minorities, placing these women at greater risk of delivering their infants preterm (Christian et al. 2013b ).
Self-rated health is a reliable predictor of health outcomes including morbidity and mortality (Idler and Benyamini 1997 ; Nielsen et al. 2008 ). Indeed, poorer self-rated health has been shown to be associated with significantly higher serum IL-1β and MIF in pregnant women during the second trimester, suggesting an influential role of inflammation on self-rated health prior to the emergence of objective and quantifiable signs of disease (Christian et al. 2013c ).
These studies suggest that pregnant women with psychosocial risk factors may experience higher daily exposure to inflammatory mediators. It is critical to identify biological markers, symptoms, and diagnostic thresholds that warrant prenatal intervention, and to develop efficient and valid screening and intervention strategies to prevent stress-related adverse health outcomes in mothers and their offspring.
Chronic stress has been shown to suppress and dysregulate immune function by affecting immunosenescence (Mathur et al. 2016 ). The term immunosenescence refers to a loss of immune function that typically occurs in elderly individuals. Declining T-cell function is a very well-characterized feature of immunosenescence, which contributes to chronic low-grade inflammation (Wu and Meydani 2008 ). Typically, elderly individuals (aged 65 years and older) compared with other age groups have two- to fourfold elevations in circulating levels of proinflammatory cytokines, such as IL-6, TNF, CRP, and serum amyloid A (SAA) (Michaud et al. 2013 ), which in turn suppresses the function of immune-protective cells and disrupts the body’s ability to defend itself against bacteria, viruses, and parasites. As a result, age-associated deterioration in immune function contributes to many illnesses and renders older individuals more vulnerable to further assaults on their immune system (e.g., stress, immunocompromising medications, infectious diseases) (Burleson et al. 2002 ). Dysregulation of the inflammatory pathway may also affect the central nervous system and the pathophysiological mechanisms of neurodegenerative disorders including Alzheimer’s disease (McCaulley and Grush 2015 ).
Epel et al. ( 2004 ) demonstrated that chronic stress in healthy premenopausal women was significantly associated with higher oxidative stress, lower telomerase activity, and shorter telomere length. Telomere length shortens with age (Rizvi et al. 2014 ). Extensive research has revealed that progressive shortening of telomeres leads to senescence, apoptosis, and carcinogenesis, which has been associated with the increased incidence of various diseases and poor survival (Shammas 2011 ). Moreover, adverse life experiences and lifestyle factors appear to affect the rate of telomere shortening over one’s lifespan (Rizvi et al. 2014 ). In accordance with these findings, in one of their studies Kiecolt-Glaser et al. ( 2011 ) demonstrated that childhood adversities have considerable consequences for cell aging in later life, and that the presence of multiple childhood adversities is linked to shorter telomeres, which underlines how adverse childhood experiences can generate continued vulnerability through to older adulthood. These findings have implications for understanding, at a cellular level, how stress gets “under the skin” and may promote the precocious onset of age-related diseases.
8 From Daily Life to Space Travel
Spaceflight conditions reflect an extreme and complex environmental challenge, with the potential for multiple aversive consequences for human health. Spaceflight, even when short in duration, can induce a wide range of adverse effects by reason of adaptations to the physical stressors of gravitational changes, radiation, malnutrition, disrupted sleep, and psychological stress (Choukèr 2012 ). As such, space travel presents an exceptional and intense combination of physical and psychological challenges that also provides a unique opportunity for investigators to examine the susceptibility of the human body to stress and explore interventions to promote psychological and physiological resilience.
In a study investigating spaceflight effects on the immune system in 30 cosmonauts, striking alterations in immune responses during and after space flight were observed, including a reduced percentage of NK, as well as suppressed NK activity by up to 85% relative to pre-flight (Rykova et al. 2008 ). Similar findings were reported in a study on long-duration spaceflight by 12 Russian cosmonauts, which included significantly suppressed T-cell immunity and exaggerated cytokine production after landing relative to before launch (Morukov et al. 2011 ). Moreover, alterations in the endocannabinoid system (ECS), which is known to play an important role in the regulation of various physiological functions, including stress regulation, behavior, mood, memory, vegetative control, and immunity, were observed following time on board of the International Space Station (ISS), resulting in an increase in circulating endocannabinoids (Strewe et al. 2012 ).
Despite the many improvements that have been made to living conditions aboard the ISS and during space travel, the clinical health risk of time in space remains high, as demonstrated by a remarkably compromised immune system and disease-fighting capabilities following exploration missions, placing cosmonauts at a greater risk for disease development, including bacterial and viral infections. In light of these results, full characterization of the shifts in the innate and adaptive immune system after space travel (see Chaps. 11 – 15 ) is critical to understand the relationship between microgravity and the stress effects of space flight in human space explorers (Morukov et al. 2011 ). In turn, studies of stressors encountered in space travel might also advance our understanding of stress in our daily lives.
9 Interventions
Given the clear negative impact of stress on immune function and health, interventions addressing stress from a psychosocial, physical, nutritional/dietary, and pharmacological perspective are of clinical importance. To appropriately manage stress in both healthy and ill individuals, comprehensive and multidisciplinary approaches that include psychopharmacological treatment, education, cognitive behavioral therapy, mindfulness-based approaches, and relaxation techniques should be provided at an early stage, particularly in physically ill patients.
A variety of stress-reduction techniques have demonstrated beneficial effects for reducing stress and improving mental health and quality of life, including cognitive behavioral therapy (Antoni et al. 2009 ), mindfulness-based stress reduction interventions (Gallegos et al. 2015 ), meditation (Rosenkranz et al. 2016 ), and yoga (Kiecolt-Glaser et al. 2010 ). Moreover, psychological interventions including cognitive behavioral stress management (Antoni et al. 2009 ; Gallegos et al. 2015 ), meditation (Rosenkranz et al. 2016 ), and yoga (Kiecolt-Glaser et al. 2010 ) have been demonstrated to improve immune function in diverse populations, including healthy individuals, women exposed to trauma, and cancer patients. These stress-reduction interventions seem to result in a healthy balance between sympathetic and parasympathetic arousal (Chaoul et al. 2014 ).
Exercise presents a promising intervention to counteract the deleterious effects of chronic stress (see also Chap. 32 ). A body of research has already examined the ability of physical/aerobic exercise to enhance immune responses when performed regularly and in moderation (Simpson et al. 2015 ). Beneficial effects of exercise and lifestyle interventions on stress reduction, inflammation, and overall well-being have been found for healthy working adults group (Kettunen et al. 2015 ), elderly individuals (Emery et al. 2005 ), patients with T2DM (Chen et al. 2015 ), and cancer patients (Zhu et al. 2016 ).
Undoubtedly, exercise is a powerful behavioral intervention with the potential to improve immune function and health outcomes in the healthy, the obese, and the elderly, as well as in patients specifically having CVD, diabetes, or cancer. Improvements in immunity, resulting from regular exercise of moderate intensity, may be due to reduced inflammation, maintained thymic mass, enhanced immuno-surveillance, reduced psychological distress, and improved overall well-being (Simpson et al. 2015 ).
In summary, a variety of interventions show promise for counteracting the negative effects of psychological stress. The particular intervention (i.e., stress management, physical activity, meditation), which is most beneficial, likely depends upon the outcome of interest and the type of stressors experienced, as well as on the individual’s personality characteristics and preexisting primary illness and comorbidities.
10 Conclusions
The findings synthesized above highlight the complex interactions that underlie the relationships among stress, neuroendocrine activity, immunity, and health outcomes. Chronic stress and its correlates affect a variety of clinically meaningful immune parameters, including wound healing, antibody responses to vaccines, susceptibility to infectious illnesses, the ability of the immune system to suppress latent viruses, and various inflammatory processes. These effects, in turn, can increase one’s risk a variety of physical and mental disorders, including cardiovascular disease, diabetes, certain cancers, and autoimmune disease, as well as general frailty and mortality. Together, these findings provide a robust pathway through which chronic stress and immune dysregulation may contribute to serious adverse health outcomes. Past research provides support for several promising avenues for interventions to prevent stress-induced immune dysfunction. However, further research is warranted to provide individualized intervention strategies.
Ahlers I, Zahumenska L, Toropila M, Smajda B, Ahlersova E (1980) The effect of season on circadian rhythm of serum and adrenal corticosterone in rats. Act Nerv Super 22:60–61
CAS Google Scholar
Antoni MH, Lutgendorf SK, Cole SW, Dhabhar FS, Sephton SE, McDonald PG, Stefanek M, Sood AK (2006) The influence of bio-behavioural factors on tumour biology: pathways and mechanisms. Nat Rev Cancer 6:240–248
Article CAS PubMed PubMed Central Google Scholar
Antoni MH, Lechner S, Diaz A, Vargas S, Holley H, Phillips K, McGregor B, Carver CS, Blomberg B (2009) Cognitive behavioral stress management effects on psychosocial and physiological adaptation in women undergoing treatment for breast cancer. Brain Behav Immun 23:580–591
Article CAS PubMed Google Scholar
Armaiz-Pena GN, Cole SW, Lutgendorf SK, Sood AK (2013) Neuroendocrine influences on cancer progression. Brain Behav Immun 30(Suppl):S19–S25
Arseniou S, Arvaniti A, Samakouri M (2014) HIV infection and depression. Psychiatry Clin Neurosci 68:96–109
Article PubMed Google Scholar
Bădescu SV, Tătaru C, Kobylinska L, Georgescu EL, Zahiu DM, Zăgrean AM, Zăgrean L (2016) The association between diabetes mellitus and depression. J Med Life 9:120–125
PubMed PubMed Central Google Scholar
Baker DG, Nievergelt CM, O’Connor DT (2012) Biomarkers of PTSD: neuropeptides and immune signaling. Neuropharmacology 62:663–673
Bartolomucci A, Palanza P, Parmigiani S, Pederzani T, Merlot E, Neveu PJ, Dantzer R (2003) Chronic psychosocial stress down-regulates central cytokines mRNA. Brain Res Bull 62:173–178
Baumeister D, Akhtar R, Ciufolini S, Pariante CM, Mondelli V (2016) Childhood trauma and adulthood inflammation: a meta-analysis of peripheral C-reactive protein, interleukin-6 and tumour necrosis factor-[alpha]. Mol Psychiatry 21:642–649
Bennett JM, Glaser R, Malarkey WB, Beversdorf DQ, Peng J, Kiecolt-Glaser JK (2012) Inflammation and reactivation of latent herpesviruses in older adults. Brain Behav Immun 26:739–746
Berghoff AS, Preusser M (2015) The inflammatory microenvironment in brain metastases: potential treatment target? Chin Clin Oncol 4:21
PubMed Google Scholar
Blair LM, Porter K, Leblebicioglu B, Christian LM (2015) Poor sleep quality and associated inflammation predict preterm birth: heightened risk among African Americans. Sleep 38:1259–1267
Article PubMed PubMed Central Google Scholar
Bluthe RM, Laye S, Michaud B, Combe C, Dantzer R, Parnet P (2000) Role of interleukin-1beta and tumour necrosis factor-alpha in lipopolysaccharide-induced sickness behaviour: a study with interleukin-1 type I receptor-deficient mice. Eur J Neurosci 12:4447–4456
CAS PubMed Google Scholar
Brook MJ, Christian LM, Hade EM, Ruffin M (2017) The effect of perceived stress on Epstein-Barr virus antibody titers in Appalachian women. Neuroimmunomodulation 24(2):67–73
Brummett BH, Babyak MA, Singh A, Jiang R, Williams RB, Harris KM, Siegler IC (2013) Socioeconomic indices as independent correlates of C-reactive protein in the National Longitudinal Study of Adolescent Health. Psychosom Med 75:882–893
Buckley T, Morel-Kopp MC, Ward C, Bartrop R, McKinley S, Mihailidou AS, Spinaze M, Chen W, Tofler G (2012) Inflammatory and thrombotic changes in early bereavement: a prospective evaluation. Eur J Prev Cardiol 19:1145–1152
Burleson MH, Poehlmann KM, Hawkley LC, Ernst JM, Berntson GG, Malarkey WB, Kiecolt-Glaser JK, Glaser R, Cacioppo JT (2002) Stress-related immune changes in middle-aged and older women: 1-year consistency of individual differences. Health Psychol 21:321–331
Capra L, Tezza G, Mazzei F, Boner AL (2013) The origins of health and disease: the influence of maternal diseases and lifestyle during gestation. Ital J Pediatr 39:7
Cash E, Sephton SE, Chagpar AB, Spiegel D, Rebholz WN, Zimmaro LA, Tillie JM, Dhabhar FS (2015) Circadian disruption and biomarkers of tumor progression in breast cancer patients awaiting surgery. Brain Behav Immun 48:102–114
Chaoul A, Milbury K, Sood AK, Prinsloo S, Cohen L (2014) Mind-body practices in cancer care. Curr Oncol Rep 16:417
Chen L, Pei JH, Kuang J, Chen HM, Chen Z, Li ZW, Yang HZ (2015) Effect of lifestyle intervention in patients with type 2 diabetes: a meta-analysis. Metab Clin Exp 64:338–347
Choukèr AE (2012) Stress challenges and immunity in space. Springer, Berlin
Book Google Scholar
Christian LM (2012) Psychoneuroimmunology in pregnancy: immune pathways linking stress with maternal health, adverse birth outcomes, and fetal development. Neurosci Biobehav Rev 36:350–361
Christian LM (2015) Stress and immune function during pregnancy: an emerging focus in mind-body medicine. Curr Dir Psychol Sci 24:3–9
Christian LM, Porter K (2014) Longitudinal changes in serum proinflammatory markers across pregnancy and postpartum: effects of maternal body mass index. Cytokine 70:134–140
Christian LM, Franco A, Glaser R, Iams JD (2009) Depressive symptoms are associated with elevated serum proinflammatory cytokines among pregnant women. Brain Behav Immun 23:750–754
Christian LM, Iams JD, Porter K, Glaser R (2012) Epstein-Barr virus reactivation during pregnancy and postpartum: effects of race and racial discrimination. Brain Behav Immun 26:1280–1287
Christian LM, Porter K, Karlsson E, Schultz-Cherry S, Iams JD (2013a) Serum proinflammatory cytokine responses to influenza virus vaccine among women during pregnancy versus non-pregnancy. Am J Reprod Immunol (New York, NY 1989) 70:45–53
Christian LM, Glaser R, Porter K, Iams JD (2013b) Stress-induced inflammatory responses in women: effects of race and pregnancy. Psychosom Med 75:658–669
Christian LM, Iams J, Porter K, Leblebicioglu B (2013c) Self-rated health among pregnant women: associations with objective health indicators, psychological functioning, and serum inflammatory markers. Ann Behav Med 46:295–309
Cohen S, Tyrrell DA, Smith AP (1991) Psychological stress and susceptibility to the common cold. N Engl J Med 325:606–612
Cohen S, Janicki-Deverts D, Doyle WJ, Miller GE, Frank E, Rabin BS, Turner RB (2012) Chronic stress, glucocorticoid receptor resistance, inflammation, and disease risk. Proc Natl Acad Sci U S A 109:5995–5999
Cohen M, Granger S, Fuller-Thomson E (2015) The association between bereavement and biomarkers of inflammation. Behav Med (Washington, DC) 41:49–59
Article Google Scholar
Coughlin SS (2012) Anxiety and depression: linkages with viral diseases. Public Health Rev 34:92
Dantzer R (2009) Cytokine, sickness behavior, and depression. Immunol Allergy Clin N Am 29:247–264
Dantzer R (2010) Psychoneuroendocrinology of stress. In: George FK, Richards FT (eds) Encyclopedia of behavioral neuroscience. Academic, Oxford, pp 126–131
Chapter Google Scholar
Dantzer R, O’Connor JC, Freund GG, Johnson RW, Kelley KW (2008) From inflammation to sickness and depression: when the immune system subjugates the brain. Nat Rev Neurosci 9:46–56
De Giorgi V, Salvini C, Chiarugi A, Paglierani M, Maio V, Nicoletti P, Santucci M, Carli P, Massi D (2009) In vivo characterization of the inflammatory infiltrate and apoptotic status in imiquimod-treated basal cell carcinoma. Int J Dermatol 48:312–321
Dekel S, Ein-Dor T, Rosen JB, Bonanno GA (2017) Differences in cortisol response to trauma activation in individuals with and without comorbid PTSD and depression. Front Psychol 8:797
Derry HM, Glaser R, Kiecolt-Glaser JK (2012) Marital status is related to Epstein-Barr virus latency in individuals undergoing cancer diagnostic procedures. Brain Behav Immun 26(Supplement 1):S30–SS1
Dhabhar FS (2014) Effects of stress on immune function: the good, the bad, and the beautiful. Immunol Res 58:193–210
Doering LV, Moser DK, Lemankiewicz W, Luper C, Khan S (2005) Depression, healing, and recovery from coronary artery bypass surgery. Am J Crit Care 14:316–324
Dolan RD, McSorley ST, Horgan PG, Laird B, McMillan DC (2017) The role of the systemic inflammatory response in predicting outcomes in patients with advanced inoperable cancer: systematic review and meta-analysis. Crit Rev Oncol Hematol 116:134–146
Donath MY, Shoelson SE (2011) Type 2 diabetes as an inflammatory disease. Nat Rev Immunol 11:98–107
Dunn EC, Nishimi K, Powers A, Bradley B (2017) Is developmental timing of trauma exposure associated with depressive and post-traumatic stress disorder symptoms in adulthood? J Psychiatr Res 84:119–127
Ebrecht M, Hextall J, Kirtley LG, Taylor A, Dyson M, Weinman J (2004) Perceived stress and cortisol levels predict speed of wound healing in healthy male adults. Psychoneuroendocrinology 29:798–809
Edmondson D, von Kanel R (2017) Post-traumatic stress disorder and cardiovascular disease. Lancet Psychiatry 4:320–329
Ehrlich KB, Miller GE, Chen E (2016) Childhood adversity and adult physical health. Developmental psychopathology. John Wiley & Sons, Inc., Hoboken, NJ
Google Scholar
Elenkov IJ, Chrousos GP (2002) Stress hormones, proinflammatory and antiinflammatory cytokines, and autoimmunity. Ann N Y Acad Sci 966:290–303
Emery CF, Kiecolt-Glaser JK, Glaser R, Malarkey WB, Frid DJ (2005) Exercise accelerates wound healing among healthy older adults: a preliminary investigation. J Gerontol A Biol Sci Med Sci 60:1432–1436
Epel ES, Blackburn EH, Lin J, Dhabhar FS, Adler NE, Morrow JD, Cawthon RM (2004) Accelerated telomere shortening in response to life stress. Proc Natl Acad Sci U S A 101:17312–17315
Fagundes CP, Glaser R, Johnson SL, Andridge RR, Yang EV, Di Gregorio MP, Chen M, Lambert DR, Jewell SD, Bechtel MA, Hearne DW, Herron JB, Kiecolt-Glaser JK (2012) Basal cell carcinoma: stressful life events and the tumor environment. Arch Gen Psychiatry 69:618–626
Fagundes CP, Glaser R, Malarkey WB, Kiecolt-Glaser JK (2013a) Childhood adversity and herpesvirus latency in breast cancer survivors. Health Psychol 32:337–344
Fagundes CP, Glaser R, Kiecolt-Glaser JK (2013b) Stressful early life experiences and immune dysregulation across the lifespan. Brain Behav Immun 27:8–12
Fagundes CP, Jaremka LM, Glaser R, Alfano CM, Povoski SP, Lipari AM, Agnese DM, Yee LD, Carson WE 3rd, Farrar WB, Malarkey WB, Chen M, Kiecolt-Glaser JK (2014) Attachment anxiety is related to Epstein-Barr virus latency. Brain Behav Immun 41:232–238
Flory JD, Yehuda R (2015) Comorbidity between post-traumatic stress disorder and major depressive disorder: alternative explanations and treatment considerations. Dialogues Clin Neurosci 17:141–150
Gallegos AM, Lytle MC, Moynihan JA, Talbot NL (2015) Mindfulness-based stress reduction to enhance psychological functioning and improve inflammatory biomarkers in trauma-exposed women: a pilot study. Psychol Trauma 7:525–532
Galley JD, Bailey M, Kamp Dush C, Schoppe-Sullivan S, Christian LM (2014) Maternal obesity is associated with alterations in the gut microbiome in toddlers. PLoS One 9:e113026
Garad Y, Maximova K, MacKinnon N, McGrath JJ, Kozyrskyj AL, Colman I (2017) Sex-specific differences in the association between childhood adversity and cardiovascular disease in adulthood: evidence from a national cohort study. Can J Cardiol 33:1013–1019
Gethin G (2012) Understanding the inflammatory process in wound healing. Br J Community Nurs Suppl:S17–S18, S20, S2
Glaser R, Kiecolt-Glaser JK (2005) Stress-induced immune dysfunction: implications for health. Nat Rev Immunol 5:243–251
Glaser R, Pearson GR, Bonneau RH, Esterling BA, Atkinson C, Kiecolt-Glaser JK (1993) Stress and the memory T-cell response to the Epstein-Barr virus in healthy medical students. Health Psychol 12:435–442
Gouin J-P, Kiecolt-Glaser JK (2011) The impact of psychological stress on wound healing: methods and mechanisms. Immunol Allergy Clin N Am 31:81–93
Gouin JP, Hantsoo L, Kiecolt-Glaser JK (2008) Immune dysregulation and chronic stress among older adults: a review. Neuroimmunomodulation 15:251–259
Gouin JP, Glaser R, Malarkey WB, Beversdorf D, Kiecolt-Glaser J (2012) Chronic stress, daily stressors, and circulating inflammatory markers. Health Psychol 31:264–268
Grinde B (2013) Herpesviruses: latency and reactivation – viral strategies and host response. J Oral Microbiol 5. https://doi.org/10.3402/jom.v5i0.22766
Article CAS Google Scholar
Hackett RA, Steptoe A (2017) Type 2 diabetes mellitus and psychological stress [mdash] a modifiable risk factor. Nat Rev Endocrinol 13:547–560
Hagger-Johnson G, Mottus R, Craig LC, Starr JM, Deary IJ (2012) Pathways from childhood intelligence and socioeconomic status to late-life cardiovascular disease risk. Health Psychol 31:403–412
Holmes TH, Rahe RH (1967) The social readjustment rating scale. J Psychosom Res 11:213–218
Huang H, Yan P, Shan Z, Chen S, Li M, Luo C, Gao H, Hao L, Liu L (2015) Adverse childhood experiences and risk of type 2 diabetes: a systematic review and meta-analysis. Metab Clin Exp 64:1408–1418
Huffhines L, Noser A, Patton SR (2016) The link between adverse childhood experiences and diabetes. Curr Diab Rep 16:54
Hughes K, Bellis MA, Hardcastle KA, Sethi D, Butchart A, Mikton C, Jones L, Dunne MP (2017) The effect of multiple adverse childhood experiences on health: a systematic review and meta-analysis. Lancet Public Health 2:e356–ee66
Idler EL, Benyamini Y (1997) Self-rated health and mortality: a review of twenty-seven community studies. J Health Soc Behav 38:21–37
Illi J, Miaskowski C, Cooper B, Levine JD, Dunn L, West C, Dodd M, Dhruva A, Paul SM, Baggott C, Cataldo J, Langford D, Schmidt B, Aouizerat BE (2012) Association between pro- and anti-inflammatory cytokine genes and a symptom cluster of pain, fatigue, sleep disturbance, and depression. Cytokine 58:437–447
Ironson G, O'Cleirigh C, Kumar M, Kaplan L, Balbin E, Kelsch CB, Fletcher MA, Schneiderman N (2015) Psychosocial and neurohormonal predictors of HIV disease progression (CD4 cells and viral load): a 4 year prospective study. AIDS Behav 19:1388–1397
Janusek LW, Tell D, Gaylord-Harden N, Mathews HL (2017) Relationship of childhood adversity and neighborhood violence to a proinflammatory phenotype in emerging adult African American men: an epigenetic link. Brain Behav Immun 60:126–135
Jensen AO, Thomsen HF, Engebjerg MC, Olesen AB, Friis S, Karagas MR, Sorensen HT (2009) Use of oral glucocorticoids and risk of skin cancer and non-Hodgkin’s lymphoma: a population-based case-control study. Br J Cancer 100:200–205
von Kanel R (2012) Psychosocial stress and cardiovascular risk : current opinion. Swiss Med Wkly 142:w13502
von Kanel R, Bellingrath S, Kudielka BM (2008) Association between burnout and circulating levels of pro- and anti-inflammatory cytokines in schoolteachers. J Psychosom Res 65:51–59
Kettunen O, Vuorimaa T, Vasankari T (2015) A 12-month exercise intervention decreased stress symptoms and increased mental resources among working adults – results perceived after a 12-month follow-up. Int J Occup Med Environ Health 28:157–168
Kiecolt-Glaser JK, Marucha PT, Malarkey WB, Mercado AM, Glaser R (1995) Slowing of wound healing by psychological stress. Lancet 346:1194–1196
Kiecolt-Glaser JK, Page GG, Marucha PT, MacCallum RC, Glaser R (1998) Psychological influences on surgical recovery. Perspectives from psychoneuroimmunology. Am Psychol 53:1209–1218
Kiecolt-Glaser JK, Christian L, Preston H, Houts CR, Malarkey WB, Emery CF, Glaser R (2010) Stress, inflammation, and yoga practice. Psychosom Med 72:113–121
Kiecolt-Glaser JK, Gouin JP, Weng NP, Malarkey WB, Beversdorf DQ, Glaser R (2011) Childhood adversity heightens the impact of later-life caregiving stress on telomere length and inflammation. Psychosom Med 73:16–22
Kivimaki M, Kawachi I (2015) Work stress as a risk factor for cardiovascular disease. Curr Cardiol Rep 17:630
Kołodziej J (2016) Effects of stress on HIV infection progression. HIV AIDS Rev 15:13–16
Korte SM, Bouws GA, Bohus B (1992) Adrenal hormones in rats before and after stress-experience: effects of ipsapirone. Physiol Behav 51:1129–1133
Lagraauw HM, Kuiper J, Bot I (2015) Acute and chronic psychological stress as risk factors for cardiovascular disease: insights gained from epidemiological, clinical and experimental studies. Brain Behav Immun 50:18–30
Lambert ND, Ovsyannikova IG, Pankratz VS, Jacobson RM, Poland GA (2012) Understanding the immune response to seasonal influenza vaccination in older adults: a systems biology approach. Expert Rev Vaccines 11:985–994
Lamkin DM, Sloan EK, Patel AJ, Chiang BS, Pimentel MA, Ma JC, Arevalo JM, Morizono K, Cole SW (2012) Chronic stress enhances progression of acute lymphoblastic leukemia via beta-adrenergic signaling. Brain Behav Immun 26:635–641
Landskron G, De la Fuente M, Thuwajit P, Thuwajit C, Hermoso MA (2014) Chronic inflammation and cytokines in the tumor microenvironment. J Immunol Res 2014:149185
Lazarus RS, Folkman S (1984) Stress, appraisal, and coping. Springer, New York, NY
LeRoy AS, Murdock KW, Jaremka LM, Loya A, Fagundes CP (2017) Loneliness predicts self-reported cold symptoms after a viral challenge. Health Psychol 36:512–520
Lin JE, Neylan TC, Epel E, O'Donovan A (2016) Associations of childhood adversity and adulthood trauma with C-reactive protein: a cross-sectional population-based study. Brain Behav Immun 53:105–112
Liu Y-Z, Wang Y-X, Jiang C-L (2017) Inflammation: the common pathway of stress-related diseases. Front Hum Neurosci 11:316
Loft P, Petrie KJ, Booth RJ, Thomas MG, Robinson E, Vedhara K (2012) Effects of massage on antibody responses after hepatitis B vaccination. Psychosom Med 74:982–987
Lutgendorf SK, Andersen BL (2015) Biobehavioral approaches to cancer progression and survival: mechanisms and interventions. Am Psychol 70:186–197
Matalka KZ, Sidki A, Abdul-Malik SM, Thewaini AJ (2000) Academic stress – influence on epstein-barr virus and cytomegalovirus reactivation, cortisol, and prolactin. Lab Med 31:163–168
Mathur MB, Epel E, Kind S, Desai M, Parks CG, Sandler DP, Khazeni N (2016) Perceived stress and telomere length: a systematic review, meta-analysis, and methodologic considerations for advancing the field. Brain Behav Immun 54:158–169
Matthews KA, Chang YF, Thurston RC, Bromberger JT (2014) Child abuse is related to inflammation in mid-life women: role of obesity. Brain Behav Immun 36:29–34
McCaulley ME, Grush KA (2015) Alzheimer’s disease: exploring the role of inflammation and implications for treatment. Int J Alzheimers Dis 2015:515248
McGuire L, Heffner K, Glaser R, Needleman B, Malarkey W, Dickinson S, Lemeshow S, Cook C, Muscarella P, Melvin WS, Ellison EC, Kiecolt-Glaser JK (2006) Pain and wound healing in surgical patients. Ann Behav Med 31:165–172
Michaud M, Balardy L, Moulis G, Gaudin C, Peyrot C, Vellas B, Cesari M, Nourhashemi F (2013) Proinflammatory cytokines, aging, and age-related diseases. J Am Med Dir Assoc 14:877–882
Mitchell AM, Christian LM (2018) Examination of the role of obesity in the association between childhood trauma and inflammation during pregnancy. Health Psychol 37(2):114–124
Moreno-Smith M, Lutgendorf SK, Sood AK (2010) Impact of stress on cancer metastasis. Fut Oncol (London) 6:1863–1881
Morgan N, Irwin MR, Chung M, Wang C (2014) The effects of mind-body therapies on the immune system: meta-analysis. PLoS One 9:e100903
Morukov B, Rykova M, Antropova E, Berendeeva T, Ponomaryov S, Larina I (2011) T-cell immunity and cytokine production in cosmonauts after long-duration space flights. Acta Astronaut 68:739–746
Mostofa AG, Punganuru SR, Madala HR, Al-Obaide M, Srivenugopal KS (2017) The process and regulatory components of inflammation in brain oncogenesis. Biomol Ther 7:pii:E34
Murdock KW, Fagundes CP, Peek MK, Vohra V, Stowe RP (2016) The effect of self-reported health on latent herpesvirus reactivation and inflammation in an ethnically diverse sample. Psychoneuroendocrinology 72:113–118
Nadrowski P, Chudek J, Skrzypek M, Puzianowska-Kuznicka M, Mossakowska M, Wiecek A, Zdrojewski T, Grodzicki T, Kozakiewicz K (2016) Associations between cardiovascular disease risk factors and IL-6 and hsCRP levels in the elderly. Exp Gerontol 85:112–117
Nielsen AB, Siersma V, Hiort LC, Drivsholm T, Kreiner S, Hollnagel H (2008) Self-rated general health among 40-year-old Danes and its association with all-cause mortality at 10-, 20-, and 29 years’ follow-up. Scand J Public Health 36:3–11
Nygren M, Carstensen J, Koch F, Ludvigsson J, Frostell A (2015) Experience of a serious life event increases the risk for childhood type 1 diabetes: the ABIS population-based prospective cohort study. Diabetologia 58:1188–1197
O’Connor TG, Moynihan JA, Wyman PA, Carnahan J, Lofthus G, Quataert SA, Bowman M, Caserta MT (2014) Depressive symptoms and immune response to meningococcal conjugate vaccine in early adolescence. Dev Psychopathol 26:1567–1576
Padgett DA, Sheridan JF, Dorne J, Berntson GG, Candelora J, Glaser R (1998) Social stress and the reactivation of latent herpes simplex virus type 1. Proc Natl Acad Sci U S A 95:7231–7235
Padro CJ, Sanders VM (2014) Neuroendocrine regulation of inflammation. Semin Immunol 26:357–368
Pedersen AF, Zachariae R, Bovbjerg DH (2009) Psychological stress and antibody response to influenza vaccination: a meta-analysis. Brain Behav Immun 23:427–433
Pinto A, Faiz O, Davis R, Almoudaris A, Vincent C (2016) Surgical complications and their impact on patients’ psychosocial well-being: a systematic review and meta-analysis. BMJ Open 6:e007224
Radojevic K, Rakin A, Pilipovic I, Kosec D, Djikic J, Bufan B, Vujnovic I, Leposavic G (2014) Effects of catecholamines on thymocyte apoptosis and proliferation depend on thymocyte microenvironment. J Neuroimmunol 272:16–28
Raposa EB, Bower JE, Hammen CL, Najman JM, Brennan PA (2014) A developmental pathway from early life stress to inflammation: the role of negative health behaviors. Psychol Sci 25:1268–1274
Reilly KH, Clark RA, Schmidt N, Benight CC, Kissinger P (2009) The effect of post-traumatic stress disorder on HIV disease progression following hurricane Katrina. AIDS Care 21:1298–1305
Rizvi S, Raza ST, Mahdi F (2014) Telomere length variations in aging and age-related diseases. Curr Aging Sci 7:161–167
Rosenkranz MA, Lutz A, Perlman DM, Bachhuber DR, Schuyler BS, MacCoon DG, Davidson RJ (2016) Reduced stress and inflammatory responsiveness in experienced meditators compared to a matched healthy control group. Psychoneuroendocrinology 68:117–125
Rykova MP, Antropova EN, Larina IM, Morukov BV (2008) Humoral and cellular immunity in cosmonauts after the ISS missions. Acta Astronaut 63:697–705
Saul AN, Oberyszyn TM, Daugherty C, Kusewitt D, Jones S, Jewell S, Malarkey WB, Lehman A, Lemeshow S, Dhabhar FS (2005) Chronic stress and susceptibility to skin cancer. J Natl Cancer Inst 97:1760–1767
Segerstrom SC, Hardy JK, Evans DR, Greenberg RN (2012) Vulnerability, distress, and immune response to vaccination in older adults. Brain Behav Immun 26:747–753
Shaashua L, Shabat-Simon M, Haldar R, Matzner P, Zmora O, Shabtai M, Sharon E, Allweis T, Barshack I, Hayman L, Arevalo JMG, Ma J, Horowitz M, Cole SW, Ben-Eliyahu S (2017) Perioperative COX-2 and β-adrenergic blockade improves metastatic biomarkers in breast cancer patients in a phase-II randomized trial. Clin Cancer Res 23(16):4651–4661
Shammas MA (2011) Telomeres, lifestyle, cancer, and aging. Curr Opin Clin Nutr Metab Care 14:28–34
Shapero BG, Black SK, Liu RT, Klugman J, Bender RE, Abramson LY, Alloy LB (2014) Stressful life events and depression symptoms: the effect of childhood emotional abuse on stress reactivity. J Clin Psychol 70:209–223
Simpson RJ, Kunz H, Agha N, Graff R (2015) Exercise and the regulation of immune functions. Prog Mol Biol Transl Sci 135:355–380
Slavich GM, Irwin MR (2014) From stress to inflammation and major depressive disorder: a social signal transduction theory of depression. Psychol Bull 140:774–815
Sloan EK, Priceman SJ, Cox BF, Yu S, Pimentel MA, Tangkanangnukul V, Arevalo JM, Morizono K, Karanikolas BD, Wu L, Sood AK, Cole SW (2010) The sympathetic nervous system induces a metastatic switch in primary breast cancer. Cancer Res 70:7042–7052
Sompayrac L (2016) How the immune system works, 5th edn. Singapore, Wiley Blackwell
Song SS, Goldenberg A, Ortiz A, Eimpunth S, Oganesyan G, Jiang SI (2016) Nonmelanoma skin cancer with aggressive subclinical extension in immunosuppressed patients. JAMA Dermatol 152:683–690
Spellberg B, Edwards JE Jr (2001) Type 1/type 2 immunity in infectious diseases. Clin Infect Dis 32:76–102
Stahl ST, Arnold AM, Chen JY, Anderson S, Schulz R (2016) Mortality after bereavement: the role of cardiovascular disease and depression. Psychosom Med 78:697–703
Strewe C, Feuerecker M, Nichiporuk I, Kaufmann I, Hauer D, Morukov B, Schelling G, Choukèr A (2012) Effects of parabolic flight and spaceflight on the endocannabinoid system in humans. Rev Neurosci 23:673
Tevis SE, Kennedy GD (2013) Postoperative complications and implications on patient-centered outcomes. J Surg Res 181:106–113
Tsuneki H, Tokai E, Sugawara C, Wada T, Sakurai T, Sasaoka T (2013) Hypothalamic orexin prevents hepatic insulin resistance induced by social defeat stress in mice. Neuropeptides 47:213–219
Urosevic M, Maier T, Benninghoff B, Slade H, Burg G, Dummer R (2003) Mechanisms underlying imiquimod-induced regression of basal cell carcinoma in vivo. Arch Dermatol 139:1325–1332
Walburn J, Vedhara K, Hankins M, Rixon L, Weinman J (2009) Psychological stress and wound healing in humans: a systematic review and meta-analysis. J Psychosom Res 67:253–271
Wang M, Zhao J, Zhang L, Wei F, Lian Y, Wu Y, Gong Z, Zhang S, Zhou J, Cao K, Li X, Xiong W, Li G, Zeng Z, Guo C (2017) Role of tumor microenvironment in tumorigenesis. J Cancer 8:761–773
Webster Marketon JI, Glaser R (2008) Stress hormones and immune function. Cell Immunol 252:16–26
Wu D, Meydani SN (2008) Age-associated changes in immune and inflammatory responses: impact of vitamin E intervention. J Leukoc Biol 84:900–914
Yu M, Zhang X, Lu F, Fang L (2015) Depression and risk for diabetes: a meta-analysis. Can J Diabetes 39:266–272
Zhu G, Zhang X, Wang Y, Xiong H, Zhao Y, Sun F (2016) Effects of exercise intervention in breast cancer survivors: a meta-analysis of 33 randomized controlled trails. OncoTargets Ther 9:2153–2168
Ziol-Guest KM, Duncan GJ, Kalil A, Boyce WT (2012) Early childhood poverty, immune-mediated disease processes, and adult productivity. Proc Natl Acad Sci U S A 109(Suppl 2):17289–17293
Zöller B, Sundquist J, Sundquist K, Crump C (2015) Perinatal risk factors for premature ischaemic heart disease in a Swedish national cohort. BMJ Open 5(6):e007308
Download references
Acknowledgement
Funding sources : Annina Seiler has received funding from the Swiss National Science Foundation (SNSF) (P2FRP1-168479). Work on this chapter was also supported by grant R01 NR013661 awarded to Lisa M. Christian by the National Institutes of Health (NIH). The content of this chapter is solely the responsibility of the authors and does not necessarily represent the official views of the National Institutes of Health.
Author information
Authors and affiliations.
Department of Consultation-Liaison-Psychiatry and Psychosomatic Medicine, University Hospital Zurich, Zurich, Switzerland
Annina Seiler
Department of Psychology, Rice University, Houston, TX, USA
Christopher P. Fagundes
Department of Behavioral Science, The University of Texas MD Anderson Cancer Center, Houston, TX, USA
Department of Psychiatry and Behavioral Health, The Ohio State University Wexner Medical Center, Columbus, OH, USA
Lisa M. Christian
The Institute for Behavioral Medicine Research, The Ohio State University Wexner Medical Center, Columbus, OH, USA
You can also search for this author in PubMed Google Scholar
Corresponding author
Correspondence to Annina Seiler .
Editor information
Editors and affiliations.
Laboratory of Translational Research Stress and Immunity Department of Anaesthesiology, Hospital of the University of Munich (LMU), Munich, Germany
Alexander Choukèr
Rights and permissions
Open Access This chapter is licensed under the terms of the Creative Commons Attribution 4.0 International License (http://creativecommons.org/licenses/by/4.0/), which permits use, sharing, adaptation, distribution and reproduction in any medium or format, as long as you give appropriate credit to the original author(s) and the source, provide a link to the Creative Commons license and indicate if changes were made.
The images or other third party material in this chapter are included in the chapter's Creative Commons license, unless indicated otherwise in a credit line to the material. If material is not included in the chapter's Creative Commons license and your intended use is not permitted by statutory regulation or exceeds the permitted use, you will need to obtain permission directly from the copyright holder.
Reprints and permissions
Copyright information
© 2020 The Author(s)
About this chapter
Seiler, A., Fagundes, C.P., Christian, L.M. (2020). The Impact of Everyday Stressors on the Immune System and Health. In: Choukèr, A. (eds) Stress Challenges and Immunity in Space. Springer, Cham. https://doi.org/10.1007/978-3-030-16996-1_6
Download citation
DOI : https://doi.org/10.1007/978-3-030-16996-1_6
Published : 28 November 2019
Publisher Name : Springer, Cham
Print ISBN : 978-3-030-16995-4
Online ISBN : 978-3-030-16996-1
eBook Packages : Medicine Medicine (R0)
Share this chapter
Anyone you share the following link with will be able to read this content:
Sorry, a shareable link is not currently available for this article.
Provided by the Springer Nature SharedIt content-sharing initiative
- Publish with us
Policies and ethics
- Find a journal
- Track your research
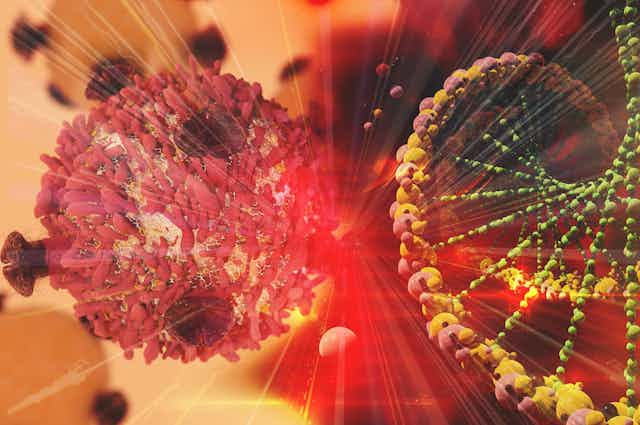
Unlocking the body’s defences: understanding immunotherapy
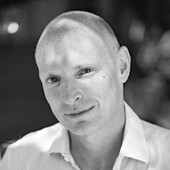
Professor of Biomedical Sciences, Anglia Ruskin University
Disclosure statement
Justin Stebbing does not work for, consult, own shares in or receive funding from any company or organisation that would benefit from this article, and has disclosed no relevant affiliations beyond their academic appointment.
Anglia Ruskin University (ARU) provides funding as a member of The Conversation UK.
View all partners
In the battle against diseases, the human body boasts an intricate defence network capable of identifying and neutralising threats – the immune system . It serves as a guardian, constantly patrolling the body to keep it safe from invaders like bacteria, viruses, and even cancer cells.
Scientists are harnessing the power of the body’s natural defence mechanism to develop immunotherapy, revolutionising the landscape of medical treatment. It enhances, redirects, or restores the body’s immune response to recognise and eliminate abnormal cells, such as cancer cells or those responsible for autoimmune diseases like multiple sclerosis, rheumatoid arthritis and type 1 diabetes.
Immunotherapy, however, is expensive. So, chemotherapy and radiation therapy are still the primary cancer treatments for most patients. But these conventional methods can damage healthy tissues as well as abnormal cells. They also tend to have debilitating side effects , such as nausea, vomiting, tiredness and hair loss.
Immunotherapy uses the body’s immune system to combat diseases with precision and minimal harm by blocking molecules – called checkpoint inhibitors – like PD-L1 or CTLA-4 that cancer cells use to turn off the immune systems.
Checkpoint inhibitors are a Nobel prize -winning discovery and they’re now one of the most widely used forms of immunotherapy. They work by blocking surface proteins that prevent immune cells from attacking cancer cells. By lifting the brakes on the immune response, these inhibitors unleash the body’s natural defence mechanism against cancer.
Hot and cold tumours
Tumours are often categorised as “hot” or “cold” based on their interaction with the immune system.
Hot tumours are characterised by a robust immune response, with infiltrating immune cells actively engaging with cancer cells. In contrast, cold tumours exhibit minimal immune activity, often evading detection by the immune system.
Immunotherapy has worked in hot tumours such as melanoma, kidney cancer and lung cancers. However, many tumours – such as most types of colon cancer – respond poorly to immunotherapy because they’re able to evade immune surveillance.
However, immunotherapies are emerging that could expand the benefits to more cancer patients , including those with cold tumours. These approaches include combination therapies using more effective immune checkpoint inhibitors with other agents, including chemotherapy and drugs in trials, to prime the immune system and enhance tumour recognition.
There are other approaches too.
CAR-T cell therapy
CAR-T cell therapy involves extracting a patient’s immune cells and genetically engineering them to produce chimeric antigen receptors – proteins on the surface of the immune cells that recognise cancer – before reintroducing them into the bloodstream. Once inside the body, the modified immune cells target and destroy cancer cells. This treatment has been used in tumour conditions like lymphomas or leukaemias but now these are moving into other cancer types .
Invariant natural killer cells
A 2024 trial used “invariant natural killer cells”, which help coordinate the body’s immune response, as immunotherapy during very severe infections, when people affected by a viral attack on their lungs could no longer breathe. The trial found that most patients recovered despite being critically unwell.
Unlike traditional vaccines that prevent infectious diseases, cancer vaccines stimulate the immune system to recognise and attack cancer cells. Cancer vaccines may contain tumour-specific markers called antigens or genetic material to train the immune system to target cancerous cells.
This means that immunotherapy can offer truly personalised medicine. There’s data, for example, on cancer vaccines from clinical trials based on the changes or mutations of a specific patient’s tumour.
Benefits beyond cancer treatment
While immunotherapy has gained widespread recognition for its efficacy in cancer treatment, its applications could extend far beyond oncology . By harnessing the immune system’s ability to distinguish self from non-self, immunotherapy offers promising avenues for combating a diverse range of ailments.
For example, researchers are exploring its potential in treating autoimmune diseases, allergic disorders, infectious diseases, and even neurological conditions like Alzheimer’s disease.
The treatment can be highly effective but it’s not everyone. For reasons we don’t yet fully understand, some people are resistant to treatment. Immunotherapy isn’t free of side effects either. Autoimmune complications can include colon and lung tissue inflammation. The current high cost of immunotherapy can prove prohibitive for many potential patients. Additionally, uptake of the treatment is limited by patient selection – choosing who would most benefit from this treatment and developing personalised treatment regimens remain critical for maximising results.
Ongoing research into immunotherapy could herald an era of targeted and tailored treatments. These include oncolytic viruses that can attack cancer directly, and microbiome modulation , which uses bacteria to enhance the activity of checkpoint inhibitors.
As our understanding of immunology continues to deepen and technology advances, immunotherapy could offer precision medicine and personalised treatments for a host of previously incurable conditions – the challenge is to make it available and accessible to more patients.
- Immunotherapy
- Cancer immunotherapy
- Immunotherapy for cancer
- Cancer vaccine

Data Manager

Research Support Officer

Director, Social Policy

Head, School of Psychology

Senior Research Fellow - Women's Health Services
- Open access
- Published: 28 May 2024
Towards personalized medicine: a scoping review of immunotherapy in sepsis
- Marleen A. Slim 1 , 2 na1 ,
- Niels van Mourik 1 , 2 na1 ,
- Lieke Bakkerus 3 ,
- Katherine Fuller 4 ,
- Lydia Acharya 4 ,
- Tatiana Giannidis 4 ,
- Joanna C. Dionne 4 , 5 , 6 , 7 ,
- Simon J. W. Oczkowski 4 , 5 , 6 ,
- Mihai G. Netea 3 ,
- Peter Pickkers 8 ,
- Evangelos J. Giamarellos-Bourboulis 9 ,
- Marcella C. A. Müller 1 ,
- Tom van der Poll 2 , 10 ,
- W. Joost Wiersinga 2 , 10 ,
- in collaboration with the ImmunoSep Consortium ,
- Alexander P. J. Vlaar 1 na2 &
- Lonneke A. van Vught 1 , 2 na2
Critical Care volume 28 , Article number: 183 ( 2024 ) Cite this article
478 Accesses
1 Altmetric
Metrics details
Despite significant progress in our understanding of the pathophysiology of sepsis and extensive clinical research, there are few proven therapies addressing the underlying immune dysregulation of this life-threatening condition. The aim of this scoping review is to describe the literature evaluating immunotherapy in adult patients with sepsis, emphasizing on methods providing a “personalized immunotherapy” approach, which was defined as the classification of patients into a distinct subgroup or subphenotype, in which a patient’s immune profile is used to guide treatment. Subgroups are subsets of sepsis patients, based on any cut-off in a variable. Subphenotypes are subgroups that can be reliably discriminated from other subgroup based on data-driven assessments. Included studies were randomized controlled trials and cohort studies investigating immunomodulatory therapies in adults with sepsis. Studies were identified by searching PubMed, Embase, Cochrane CENTRAL and ClinicalTrials.gov, from the first paper available until January 29th, 2024. The search resulted in 15,853 studies. Title and abstract screening resulted in 1409 studies (9%), assessed for eligibility; 771 studies were included, of which 282 (37%) were observational and 489 (63%) interventional. Treatment groups included were treatments targeting the innate immune response, the complement system, coagulation and endothelial dysfunction, non-pharmalogical treatment, pleiotropic drugs, immunonutrition, concomitant treatments, Traditional Chinese Medicine, immunostimulatory cytokines and growth factors, intravenous immunoglobulins, mesenchymal stem cells and immune-checkpoint inhibitors. A personalized approach was incorporated in 70 studies (9%). Enrichment was applied using cut-offs in temperature, laboratory, biomarker or genetic variables. Trials often showed conflicting results, possibly due to the lack of patient stratification or the potential influence of severity and timing on immunomodulatory therapy results. When a personalized approach was applied, trends of clinical benefit for several interventions emerged, which hold promise for future clinical trials using personalized immunotherapy.
Despite a global decrease in sepsis burden, sepsis still causes almost 20% of all deaths worldwide [ 1 ]. Over the past few decades, significant progress has been made in the understanding of the pathophysiology of sepsis [ 2 ], however, treatment is still limited to tackling the pathogens and providing supportive care. To date, limited proven therapies address the underlying mechanisms of this life-threatening condition.
The host response to infection can be dysregulated in multiple ways, resulting in a highly heterogeneous clinical presentation, treatment response, and prognosis [ 3 ]. The pathophysiology of sepsis involves dysregulation of the inflammatory response, but also catabolic, metabolic and immune-suppressive features can be present, together resulting in failure to return to homeostasis [ 3 , 4 , 5 ]. Modulating these various immune responses to infection represents a promising treatment option. Reason for the numerous failed clinical trials [ 4 , 5 , 6 ] could be the use of “one-size-fits-all” approaches, suggesting that personalized immunomodulatory treatment tailored to an individual patient’s immune profile may be a more successful treatment approach. The first step towards implementation of such a personalized strategy is providing a structured and in-depth overview of currently available evidence on immunotherapy in sepsis. The aim of this scoping review is to describe and summarize the literature evaluating immunotherapy in adult patients with sepsis, and to evaluate methods by which a personalized immunotherapy approach has been studied so far.
In line with our previously published protocol [ 7 ], studies were identified by searching PubMed, Embase, Cochrane CENTRAL and ClinicalTrials.gov from the first paper available until Janaury 29th, 2024. Inclusion criteria were: 1) randomized controlled trials (RCTs) or cohort studies (including case control studies and observational cohorts); 2) investigating immunomodulatory therapies; in 3) adult (≥ 16 years) patients with sepsis, 4) written in English or Dutch. We included studies that addressed therapies with a potential or hypothesized immunomodulatory effect (see Supplementary Methods). Exclusion criteria were: 1) case reports or systematic reviews; 2) animal studies; and 3) studies in healthy volunteers. We deviated from the previously publish protocol [ 7 ] by not including studies investigating coronavirus disease 2019 (COVID-19), since immunomodulatory treatments and patient stratification in COVID-19 is explored in a recently published review [ 8 ]. The full search strategies, screening and data extraction can be found in the Supplementary Methods. The results are organized in two steps. First, separating observational from interventional studies; and subsequently into treatment groups [ 3 ]. Ongoing trials are reported separately. The text in the main paper focusses on randomized controlled trials and studies applying a personalized approach; an overview of observational studies and non-randomized interventional studies not using a personalized approach can be found in the supplement. We defined a personalized approach as the classification of patients into a distinct subgroup or subphenotype. Subgroups are subsets of patients with the same disease or syndrome, based on any cut-off in temperature, laboratory, biomarker or genetic variables. In particular, subgroups based on age, sex or use of certain interventions (mechanical ventilation or vasopressors) were not considered subgroups for a personalized approach. Subphenotypes are subgroups that can be reliably discriminated from other subgroup based on data-driven assessments including machine learning techniques [ 9 ]. The use of disease severity scores was not considered as personalized. Individualized interventions were not included, since this review focused on personalized treatments, defined as applying specific treatment at subgroup or subphenotype level, and not at an individualized or patient level.
Study characteristics
The search resulted in 15,853 studies, including 43 studies identified through manual searching for the results of protocols, abstracts and registered studies. Our search was completed on January 29th, 2024. Title and abstract screening resulted in 1409 studies that were assessed for eligibility (Fig. 1 ). In total, 282 observational studies and 489 interventional studies were included (Figs. 1 and 2 ), of which 70 (9%) applied a personalized approach. Figure 2 depicts a timeline with an overview of the included studies in this review divided by study design, treatment group and year of publication. Figure 3 depicts an overview of all interventions discussed in this review in order to summarize all treatments that have been studied in the research field of immunotherapy in sepsis. Treatments in bold are discussed in the text and in the supplement, treatment not in bold can be found in the supplement.
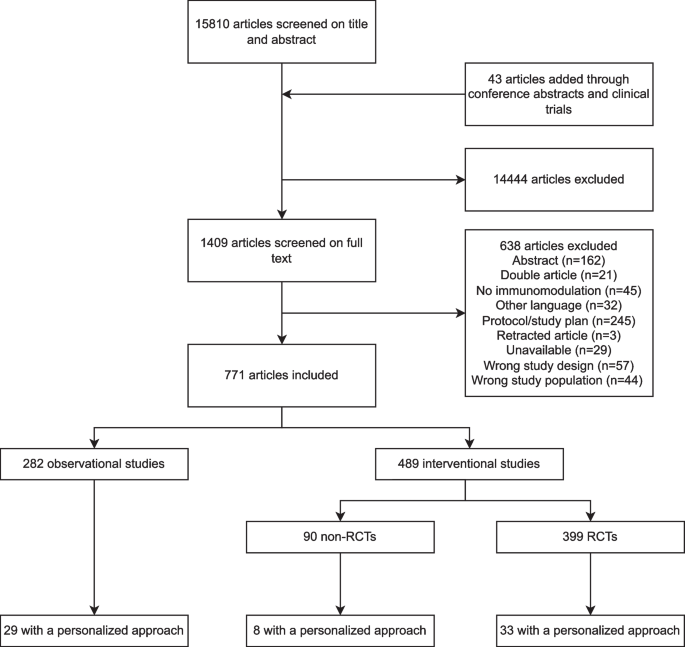
Flow diagram for study selection. RCT, randomized controlled trial
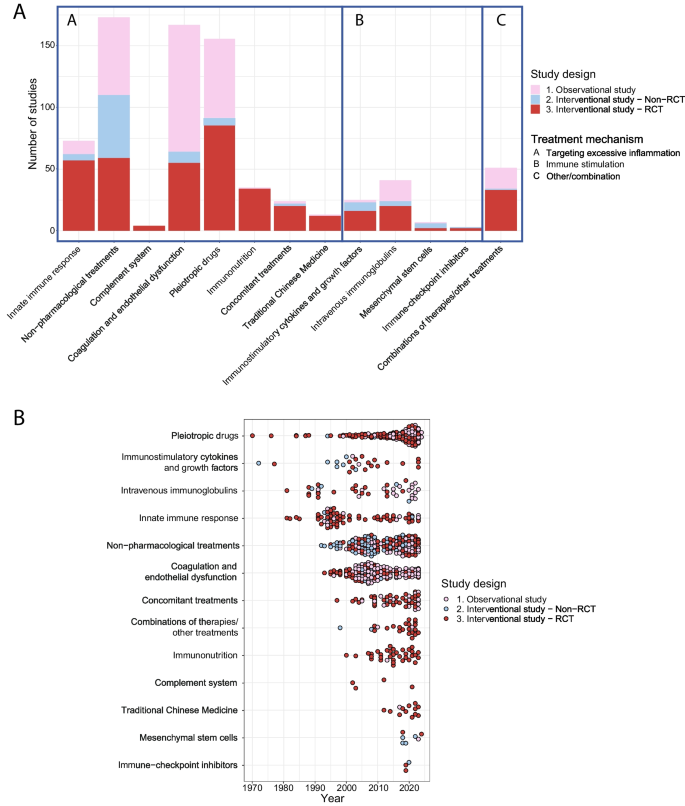
Overview of the included studies. Overview of the included studies in this review A divided per treatment strategy, study design and treatment group and B divided per year, study design and treatment group demonstrated from the earliest to the latest studies overtime. RCT, Randomized controlled trial
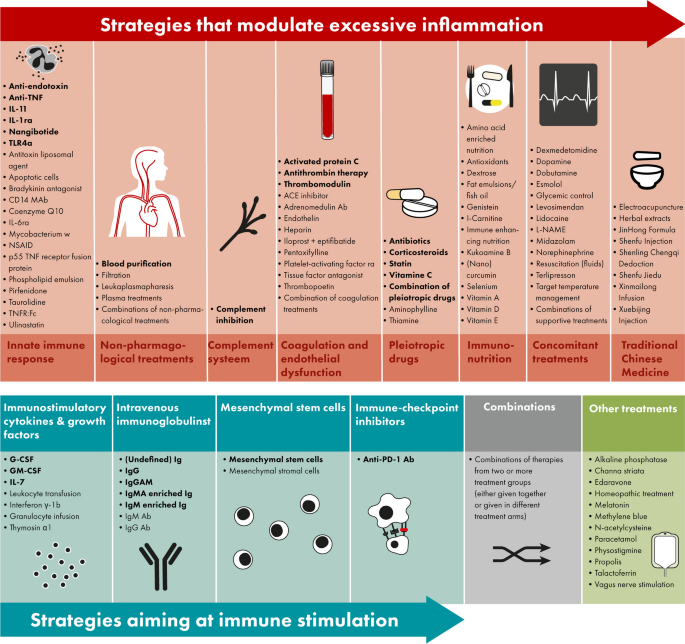
Overview of all immunomodulatory treatments studies in adult patients with sepsis. Immunomodulatory treatments investigated in sepsis patients either modulate excessive inflammation (top op panel, in red) or aim at immune stimulation (bottom of panel, blue), furthermore there are combinations of these treatment strategies (bottom of panel, in grey) or treatments not fitting into these categories (bottom of panel, in green). All treatments displayed in this figure are included in this review; the treatments in bold are discussed in the text and in the supplement, treatment not in bold can be found in the supplement. Abbreviations: Ab, antibody; ACE, angiotensin-converting-enzyme; anti-PD-1, anti-programmed cell death protein 1; G(M)-CSF, granulocyte(-macrophage) colony-stimulating factor; Ig, immunoglobulin; IL, interleukin; L-NAME, L-NG-Nitro arginine methyl ester; NSAID, non-steroidal anti-inflammatory drugs; (r)a, (receptor) antagonist; TLR, toll-like receptor; TNF(r), tumor necrosis factor (receptor)
Strategies modulating excessive inflammation
Innate immune response.
Since excessive activation of the innate immune response causes host response dysregulation leading to sepsis, there is a clear rationale to study blocking innate immune activation [ 3 ]. Treatments targeting the innate immune response were studied in 11 (15%) observational studies, 5 (7%) non-randomized interventional studies and 57 (78%) RCTs (Supplementary Table 2 ). Treatments most studied were anti-tumor necrosis factor (TNF)α antibodies (Abs) (n = 17, 23%), anti-endotoxin Abs (n = 14, 19%) and interleukin (IL)-1 receptor antagonists (ra) (n = 10, 14%),
RCTs without a personalized approach
Since 1981, RCTs studying anti-endotoxin strategies, including antiserum raised in volunteers immunized with heat-killed mutant E coli J5 (murine E5)) and humanized (HA-1A) antibodies directed against the lipid-A part of endotoxin, have been published almost without positive results. Two studies showed a lower mortality in patients with gram-negative bacteremia (30% vs. 49%, n = 197, respectively, 22% vs. 39%, n = 212) [ 10 , 11 ]. However it has been stated that the results should be interpreted cautiously [ 12 ], since this effect was restricted to gram-negative bacteremia and patients most likely to benefit are difficult to identify, and none of the other anti-endotoxin trials showed similar results (Supplementary Table 2 ). Since 2007, inhibiting Toll-like receptor-4 (TLR4) has been examined using eritoran (a synthetic lipid A antagonist blocking lipopolysaccharide (LPS) from binding at the cell surface MD2-TLR4 receptor) or TAK-242 (a small molecule-inhibitor specific for TLR4) [ 3 ]. Neither was found to be effective in large RCTs in severe sepsis patients [ 13 , 14 ]. Since 1995, anti-TNFα Abs were examined in several trials yielding disappointing results [ 15 , 16 ]. In the 1990s, Anakinra, a recombinant human IL-1RA, was studied in 6 RCTs, without effect on mortality (Supplementary Table 2 ).
Studies with a personalized approach
In a retrospective RCT subgroup analysis, showing no survival benefit of anti-TNFα Ab CB0006 in 80 unselected severe sepsis patients, the patients with increased entry TNF-levels appeared to benefit from the high dose anti-TNF Ab (survival rate 86%, n = 7) [ 17 ]. When the anti-TNF Ab afelimomab was studied in a phase-III trial including sepsis patients using stratification, only patients with IL-6 levels > 1000 pg/mL had a reduced 28-day mortality (44% vs. 48%, n = 998) [ 18 ]. In a similar trial [ 19 ], sepsis patients with IL-6 levels > 1000 pg/mL were randomized to receive either afelimomab or placebo, without mortality effect (54% vs. 58%, n = 446). One of the RCTs [ 20 ] studying anakinra in 696 sepsis patients, failed to demonstrate a mortality reduction, however in a post-hoc analysis [ 21 ] treatment with anakinra provided a 30% decrease of 28-day mortality in patients who, at the start of treatment, had both liver dysfunction and disseminated intravascular coagulation which were interpreted by the authors as traits of macrophage activation. Another post-hoc analysis of this RCT showed that patients with higher baseline IL-1 levels showed mortality reduction compared to patients with lower IL-1 [ 22 ]. In an RCT examining IL-11 therapy in patients with thrombocytopenia, a less extensive inflammatory response and lower mortality was observed (31% vs. 14%, n = 105) [ 23 ]. In an RCT with patients treated with nangibotide, a triggering receptor expressed on myeloid cells-1 (TREM-1) inhibitor, grouped according to sTREM-1 concentrations at baseline, no improvement in sequential organ failure assessment (SOFA) score was seen [ 24 ].
Non-pharmacological treatments
The primary non-pharmacological immunotherapy treatment studied in sepsis is blood purification, which may be beneficial through removal of endotoxin, altering cytokine levels, mobilization of cytokines from local tissues, or through more complex processes of immune modulation [ 25 ]. Non-pharmacological treatments were studied in 63 (36%) observational studies, 51 (29%) non-randomized interventional studies and 59 (34%) RCTs (Supplementary Table 3 ). Treatments most studies were blood purification (n = 111, 64%), blood filtration (n = 47, 27%) and plasma treatments (e.g. plasma filtration or exchange, n = 12, 7%).
The clinical effects of these studies are mixed. For instance, while in the EUPHAS trial Polymyxin B hemoperfusion reduced 28-day mortality in 64 patients with severe abdominal sepsis (32% vs. 53%, aHR 0.36; 95% CI 0.16, 0.80) [ 26 ], the ABDOMIX trial in peritonitis-induced septic shock did not show a reduction in 28-day mortality (28% vs. 20%, n = 243) [ 27 ].
The EUPHRATES trial, a multicenter RCT including 450 patients using enrichment by including patients with endotoxin activity assay (EAA) levels ≥ 0.6, did not find improvement in 28-day survival when applying Polymyxin B hemoperfusion [ 28 ]. The trial showed that in some septic shock patients the burden of endotoxin activity was extreme (EAA ≥ 0.9). Therefore, a post-hoc analysis of the EUPHRATES trial was conducted in only patients with EAA of 0.6–0.89, not leading to better survival rates [ 29 ]. In a retrospective study using the EUPHRATES trial Polymyxin B hemoadsorption was associated with higher 28-day survival in patients with PT-INR > 1.4 or lactate > 3 mmol/L (68% vs. 52%, p = 0.02) [ 30 ]. Cytokine adsorption and endotoxin hemoabsorption were studied in two observational studies including patients with septic shock and IL-6 ≥ 1000 ng/l, one study found an increased hazard of death of 1.82 (95% CI, 1.03–3.2) compared to a matched control group [ 31 ]; the other compared survivors and non-survivors and concluded that this treatment could be beneficial when applied early after onset of shock [ 32 ].
Complement system
The rationale for studying complement inhibitors is that excessive complement system activation contributes to sepsis-induced organ failure and death [ 33 ], which has been studied in 4 RCTs (Supplementary Table 4 ).
Treatment with complement (C)1-inhibitors infusion was studied in three RCTs and associated with reduced all-cause mortality (12% vs. 45% in control, n = 61) [ 34 ]. Furthermore, a phase-IIa trial on a monoclonal Anti-C5a antibody in 72 severe sepsis and septic shock patients demonstrated a dose-dependent neutralization of C5a. Complement inhibition was not studied in trials using a personalized approach.
Coagulation and endothelial dysfunction
The rationale for studies aiming at coagulation pathways and endothelial dysfunction in sepsis patients is that disseminated intravascular coagulation (DIC) and loss of endothelial barrier integrity are both key phenomena in the pathogenesis of sepsis [ 2 ]. Studies aiming at coagulation pathways and endothelial dysfunction were studied in 103 (62%) observational studies, 9 (5%) non-randomized interventional studies and 55 (33%) RCTs (Supplementary Table 5 ). The most studied treatment interventions were activated protein C (APC; n = 77, 46%), antithrombin (n = 25, 15%) and soluble thrombomodulin (n = 19, 11%).
RCT without a personalized approach
In PROWESS, a large phase-III trial in severe sepsis patients, a beneficial effect on 28-day mortality of APC was observed (25% vs. 31%, n = 1690) along with an increased risk of bleeding (3.5% versus 2.0%) [ 35 ]. In patients with septic shock, however, the phase-III PROWES-SHOCK trial did not show mortality reduction from treatment with APC (26% vs. 24%, n = 1697) [ 36 ]. Even though antithrombin therapy resulted in improvement of DIC [ 37 , 38 ], it did not result in a decreased mortality in patients with severe sepsis or septic shock (39% vs. 39%, n = 2314) [ 39 ]. In the SCARLET trial soluble thrombomodulin did not reduce mortality in unselected sepsis patient (27% vs. 29%, n = 800) [ 40 ].
In a predefined subgroup analyzing patients with severe protein C deficiency from the PROWESS-SHOCK, APC treatment did not result in differences in 28-day mortality (28.7% vs 30.8%, n = 673) [ 36 ]. However, in a retrospective cohort study in 48 patients with severe sepsis and elevated troponin, treatment with APC did improve intensive care unit (ICU)-mortality (30% vs. 72%, n = 48) [ 41 ]. A post hoc analysis of the SCARLET trial showed that patients with higher baseline thrombin generation biomarker levels showed reduced mortality when treated with recombinant human soluble thrombomodulin [ 42 ]. A study using coagulation phenotypes as a secondary analysis of multicenter registries on sepsis patients admitted to the ICU, demonstrated that in one in four phenotypes, the one with high fibrinogen/fibrin-degradation-products and D-Dimer, treatment with thrombomodulin was associated with lower mortality (adjusted risk difference -18%, 95% CI -29%,-7%, n = 323) [ 43 ]. Antithrombin supplementation therapy only reduced in-hospital mortality in sepsis patients with very low anthithrombin activity (HR 0.603, 95% CI 0.368, 0.988) [ 44 ]. When applying molecular phenotypes previously identified in acute respiratory distress syndrome (ARDS) different treatment response to activated protein C were found, with survival benefit in the hyperinflammatory and harm in the hypoinflammatory phenotype [ 45 ].
Pleiotropic drugs
Pleiotropic drugs refer to substances exerting effects other than for which it was initially developed. Corticosteroids (n = 89, 57%) and antibiotics (n = 18, 12%, mainly macrolides are known for their immunomodulatory effect [ 46 , 47 ]) are the primary pleotropic drugs used in sepsis (Supplementary Table 6 ). Pleiotropic drugs were studied in 64 (41%) observational studies, 6 (4%) non-randomized interventional studies and 85 (55%) RCTs.
Corticosteroids have been studied in sepsis patients in over 30 RCTs with contradicting results. In septic shock patients receiving hydrocortisone plus fludrocortisone compared to placebo, mortality was lower (43% vs. 49%, n = 1241) [ 48 ], however hydrocortisone alone in septic shock patients undergoing mechanical ventilation did not result in the same effect (28% vs. 29%, n = 3658) [ 49 ]. A recently published RCT showed a lower 28-day mortality among ~ 800 patients with severe community-acquired pneumonia treated in the ICU with hydrocortisone (12% vs. 6%) [ 50 ]. In an RCT in sepsis patients receiving vasopressors those who received intravenous vitamin C had a higher risk of death or persistent organ dysfunction (44.5% vs 38.5%, n = 872) [ 51 ]. Trials investigating the combination of vitamin C, thiamine, and hydrocortisone did not find positive results on ventilator-free-days [ 52 ] or mortality SPS:refid::bib53|bib54(53, 54). Treatment with clarithromycin, next to standard-of-care antimicrobial treatment, resulted in contradicting findings, the latest large trial did, however, found an association with a decreased 90-day mortality compared to placebo (43% vs. 60%, n = 200) [ 55 ].
In an RCT including patients with severe community-acquired pneumonia and C-reactive protein (CRP) > 150 mg/L methylprednisolone led to reduced treatment failure (development of shock, need for mechanical ventilation or death) compared to placebo (31% vs. 13%, n = 60) [ 56 ]. Increased mortality was observed in patients with sepsis response signature-(SRS)2 endotype compared to SRS1 in patients treated with hydrocortisone (n = 176, OR 7.9, 95% CI 1.6, 39.9) [ 57 ]. When assigning patients to two previously identified gene expression-based endotypes, corticosteroid exposure may be associated with increased mortality among septic shock endotype A patients (OR 3.1, 95% CI, 1.0 – 9.6, n = 97) [ 58 ]. When gene expression scores used to identify the immune state of shock patients; patients with the prevalent immune-adaptive state may be harmed by hydrocortisone [ 59 ]. Expression of GLCCI1 was associated with decreased time to shock reversal, and the expression of BHSD1 was associated with increased time to shock reversal (n = 494, HR 3.81 vs. 0.64 and HR 0.55 vs. 1.32, respectively) [ 60 ]. In two cohorts with > 1200 and > 2500 patients, studying the use of machine learning for corticosteroid treatment decision showed positive results [ 61 , 62 ]. Another cohort study employing machine learning identified interferon (IFN)γ/IL10 as a theranostic marker; a low serum IFNγ/IL10 ratio predicted increased survival in the hydrocortisone group whereas a high ratio predicted better survival in the placebo group [ 63 ]. One post-hoc analysis of an RCT examined the effect of simvastatin in sepsis-induced ARDS in patients with high baseline IL-18, which was associated with a higher survival probability (39% vs. 24%, n = 511) [ 64 ].
Immunonutrition, concomitant treatments and traditional chinese medicine
See the Supplementary Results for the studies regarding immunonutrition (Supplementary Table 7 ), concomitant treatments (Supplementary Table 8 ) and Traditional Chinese Medicine (Supplementary Table 9 ).
Strategies aiming at immune stimulation
Immunostimulatory cytokines and growth factors.
Immunostimulatory cytokines and growth factors have been studied in 2 (8%) observational studies, 7 (28%) interventional non-RCTs and 16 (64%) RCTs (Supplementary Table 10 ). Treatments most studies were granulocyte-colony stimulating factor (G-CSF) (n = 11, 44%) and granulocyte–macrophage colony-stimulating factor (GM-CSF) (n = 7, 28%).
Six RCTs investigating G-CSF did not show an effect on mortality (Supplementary Table 10 ). Even though likewise no survival benefit was found for GM-CSF, one RCT did demonstrate improved respiratory function (n = 18) [ 65 ].
Three RCTs studied biomarker-guided (human leukocyte antigen DR (HLA-DR) < 8000) GM-CSF treatment; one trial resulted in a shorter time of mechanical ventilation (148 ± 103 h vs. 207 ± 58 h, n = 38) [ 66 ]; another in decreased indoleamine 2,3-dioxygenase levels, possibly due to an improved antibacterial defense (35.4 ± 21.0 vs 21.6 ± 9.9 (baseline vs day 9), n = 36) [ 67 ]; another had no effect on the prevention on ICU-acquired infections (11% vs 11%, n = 98) [ 68 ]. In an RCT studying intramuscular recombinant human IL-7 (CYT107) in 27 patients with severe lymphopenia, CYT107 reversed the loss of CD4+ and CD8+ cells [ 69 ].
Intravenous immunoglobulins
Immunoglobulins can opsonize and neutralize pathogens and toxins resulting in immunostimulation and reduced inflammation. Immunoglobulins have been studied in 17 (41%) observational studies, 4 (10%) interventional non-RCTs and 20 (49%) RCTs (Supplementary Table 11 ).
RCTs without a personalized approach RCTs on immunoglobulins demonstrate contradicting results concerning improving patient outcome and decreasing mortality (Supplementary Table 11 ). For example, two RCTs on immunoglobulin (Ig)G demonstrated a lower mortality from septic shock in one trial (38% vs. 67%, n = 62) [ 70 ]; while no mortality reduction was seen in another trial (37% vs. 39%, n = 653) [ 71 ].
Studies with a personalized approach In an observation study intravenous immunoglobulins (IVIG) administration in patients with sepsis and low serum IgG levels was associated with improved prognosis (OR 0.15; 95%CI, 0.04–0.54; n = 87) [ 72 ]. In sepsis patients with neutropenia, polyclonal immunoglobulin M-enriched immunoglobulins led to a decrease in endotoxin levels in survivors, in non-survivors this was not seen [ 73 ]. In a post-hoc RCT analysis, a reduction of all-cause mortality was observed in pneumosepsis patients with high CRP and low IgM levels when administered trimodulin (polyclonal antibody) (reduction of 25%, n = 92) [ 74 ]. In an RCT studying the use of IVIG, IGMA had no effect on 28-day mortality in neutropenic patients (26% vs. 28%, n = 211) [ 75 ].
Mesenchymal stem cells
Mesenchymal stem cells enhance bacterial clearance and modulate the immune response. Mesenchymal stem cells have been studied in 1 (17%) observational study, 4 (67%) non-randomized interventional studies and 1 (17%) RCT (Supplementary Table 12 ).
RCTs with a personalized approach
One RCT showed that mesenchymal stem cells are safe and attributed to the faster hemodynamic stabilization in 30 patients with neutropenia [ 76 ].
Immune-checkpoint inhibitors
Immune-checkpoint inhibitors have been studied in 1 (33%) non-randomized interventional study and 2 (67%) RCTs (Supplementary Table 13 ).
Studies with a personalized approach Immune-checkpoint inhibitors, like anti-programmed death (PD)-1 antibodies, while not yet proven to enhance survival, also appear safe and could improve immune recovery in one non-randomized interventional study and two RCTs with patients with absolute lymphocyte count ≤ 1.1 × 10 3 cells/μL [ 77 , 78 , 79 ].
Combination of therapies and other therapies
See the Supplementary Results for studies investigating combination of therapies (Supplementary Table 14 ) and treatments that could not be classified into the previously mentioned treatment groups (Supplementary Table 15 ).
Ongoing trials
A search on ClinicalTrials.gov yielded 78 sepsis studies, reflecting ongoing research across the entire immunotherapy spectrum (Supplementary Table 16 ). Notably, sixteen studies (21%) implement a personalized approach. For the RCTs (n = 14) applying a personalized approach see Table 1 . One of these trials, employing a double-dummy design, is studying the impact of precision immunotherapy on sepsis phenotypes like hyperinflammation (using very high ferritin levels as a marker for macrophage activation-like syndrome (MALS)) and immunoparalysis (using low expression of HLA-DR on monocytes as marker of immunoparalysis) [ 80 ]. Patients, stratified by biomarkers are assigned to receive either placebo or active immunotherapy as an adjunct to standard care. The active treatments include anakinra for MALS and interferon-gamma for immunoparalysis.
This scoping review provides a comprehensive overview of immunomodulatory treatments investigated in adult patients with sepsis, highlighting studies with a personalized treatment approach. Our results show that trials often showed conflicting results. Possibly due to the lack of patient stratification, requiring the need to confirm positive findings in large multicenter populations or the potential influence of severity and timing on immunomodulatory therapy results. Several immunomodulatory treatments described in this review suggest possible efficacy, laying the groundwork for future trials to demonstrate their effectiveness. If a personalized approach is applied, clinical benefits of treatment appear to emerge in several studies. This emphasizes the need to decipher different host response endo-/phenotypes for the intervention to modulate.
Over 700 studies investigating immunotherapy in patients with sepsis have been performed and despite this body of evidence, the 2021 surviving sepsis campaign guidelines only include intravenous hydrocortisone as an immunomodulatory treatment for patients with vasopressor refractory septic shock [ 81 ]. Perhaps patient stratification might be the way forward, in which we have witnessed notable advancements in recent years, including therapies targeted by biomarker measurements. For instance, the ratio of IFN-γ to IL-10 has been used to guide corticosteroid therapy decisions [ 63 ], while HLA-DR levels on monocytes and plasma IL-10 concentrations have been used for stratification of treatment with either GM-CSF or IFN-γ [ 82 ]. In ongoing and upcoming sepsis trials, an increase in patient stratification has been observed. The personalized approach most applied is a cut-off value for inflammatory markers such as IL-6 or procalcitonin. Two studies use more complex stratification methods. One is the ImmunoSep trial (NCT04990232) which uses biomarker stratification to identify patients with either hyperinflammation or immunoparalysis [ 80 ]. Another example is the RECORDS trial, which aims at defining endotypes in sepsis adults associated with responsiveness to corticosteroids [ 83 ]. This multicenter, placebo-controlled, biomarker-guided, adaptive Bayesian design basket trial will randomly assign 1800 adults to a biomarker stratum to identify resistant or sensitive sepsis to corticosteroid treatment. In our opinion, using biomarker-based protocols for patient stratification will be the way forward in sepsis research.
The strengths of this review include the systematic search and comprehensive inclusion of all studies investigating immunomodulatory treatments in sepsis, including studies on immunonutrition, Traditional Chinese Medicine and concomitant treatments. Furthermore, it gives an extensive overview of studies that used a personalized approach, which can be used as the foundation for new study designs and aims. A few limitations should be mentioned. Given that the objective of this review was to provide a comprehensive overview of all studies examining immunomodulatory treatments in sepsis, a scoping review was considered the most suitable approach. Consequently, a risk of bias assessment was not conducted [ 84 ]. Although inevitable, different criteria for sepsis have been used over time [ 85 ], leading to heterogeneity in the population included. In this review treatments are considered personalized when a subgroup of subphenotype was selected based on biological characteristics possibly making the patient benefit more from a specific treatment, however, the is no uniform definition for ‘personalized medicine’. Since no qualitative methods such as qualitative text analysis, evidence maps or evidence gap maps, were deployed, no information on the research gaps in the field could be given. Due to the extensive body of evidence, we refrained from reporting cohort studies in a structured manner in the main text. Even though these observational studies and non-randomized interventional studies were included in the supplementary materials, not discussing them in the main text of the paper could be perceived as selective reporting bias. Lastly, since the search yielded a large amount of studies there was only limited possibility for in-depth description of important trials, including descriptions of the different dosages given.
Conclusions
Decades of extensive investigation into immunomodulatory treatments has led to over 700 studies investigating these treatment for sepsis, with often conflicting results. The lack of therapeutic efficacy appears to be related to the difficulty to enroll the right patients for the intervention. Since it is highly unlikely that one single immunomodulatory treatment will be universally effective in all sepsis patients, a personalized approach seems the way forward. To date, only a small proportion of studies have looked into enrichment strategies in sepsis, and for several interventions the therapeutic efficacy appears to emerge when a personalized approach was used. Patient stratification will play a pivotal role in the identification of patients that may benefit from targeted immunotherapy.
Availability of data and materials
Not applicable.
Abbreviations
Activated protein C
Acute respiratory distress syndrome
Coronavirus disease 2019
C-reactive protein
Disseminated intravascular coagulation
Endotoxin activity assay
Granulocyte-colony stimulating factor
Granulocyte–macrophage colony-stimulating factor
Human leukocyte antigen DR
Intensive care unit
Interleukin
(Intravenous) immunoglobulins
Lipopolysaccharide
Macrophage activation-like syndrome
Programmed death-1
Receptor antagonists
Randomized controlled trial
Sequential organ failure assessment
Toll-like receptor
Tumor necrosis factor
Triggering receptor expressed on myeloid cells-1
Rudd KE, Johnson SC, Agesa KM, Shackelford KA, Tsoi D, Kievlan DR, et al. Global, regional, and national sepsis incidence and mortality, 1990–2017: analysis for the Global Burden of Disease Study. Lancet. 2020;395(10219):200–11.
Article PubMed PubMed Central Google Scholar
Wiersinga WJ, van der Poll T. Immunopathophysiology of human sepsis. EBioMedicine. 2022;86: 104363.
Article CAS PubMed PubMed Central Google Scholar
Steinhagen F, Schmidt SV, Schewe JC, Peukert K, Klinman DM, Bode C. Immunotherapy in sepsis - brake or accelerate? Pharmacol Ther. 2020;208: 107476.
Article CAS PubMed Google Scholar
van der Poll T, van de Veerdonk FL, Scicluna BP, Netea MG. The immunopathology of sepsis and potential therapeutic targets. Nat Rev Immunol. 2017;17(7):407–20.
Article PubMed Google Scholar
Rubio I, Osuchowski MF, Shankar-Hari M, Skirecki T, Winkler MS, Lachmann G, et al. Current gaps in sepsis immunology: new opportunities for translational research. Lancet Infect Dis. 2019;19(12):e422–36.
Davies R, O’Dea K, Gordon A. Immune therapy in sepsis: are we ready to try again? J Intensive Care Soc. 2018;19(4):326–44.
Slim MA, van Mourik N, Dionne JC, Oczkowski SJW, Netea MG, Pickkers P, et al. Personalised immunotherapy in sepsis: a scoping review protocol. BMJ Open. 2022;12(5): e060411.
van de Veerdonk FL, Giamarellos-Bourboulis E, Pickkers P, Derde L, Leavis H, van Crevel R, et al. A guide to immunotherapy for COVID-19. Nat Med. 2022;28(1):39–50.
Bos LDJ, Ware LB. Acute respiratory distress syndrome: causes, pathophysiology, and phenotypes. The Lancet. 2022;400(10358):1145–56.
Article Google Scholar
Ziegler EJ, Fisher CJ Jr, Sprung CL, Straube RC, Sadoff JC, Foulke GE, et al. Treatment of gram-negative bacteremia and septic shock with HA-1A human monoclonal antibody against endotoxin A randomized, double-blind, placebo-controlled trial The HA-1A Sepsis Study Group. N Engl J Med. 1991;324(7):429–36.
Ziegler EJ, McCutchan JA, Fierer J, Glauser MP, Sadoff JC, Douglas H, Braude AI. Treatment of gram-negative bacteremia and shock with human antiserum to a mutant Escherichia coli. N Engl J Med. 1982;307(20):1225–30.
Warren HS, Danner RL, Munford RS. Anti-endotoxin monoclonal antibodies. N Engl J Med. 1992;326(17):1153–7.
Opal SM, Laterre PF, Francois B, LaRosa SP, Angus DC, Mira JP, et al. Effect of eritoran, an antagonist of MD2-TLR4, on mortality in patients with severe sepsis: the ACCESS randomized trial. JAMA. 2013;309(11):1154–62.
Rice TW, Wheeler AP, Bernard GR, Vincent JL, Angus DC, Aikawa N, et al. A randomized, double-blind, placebo-controlled trial of TAK-242 for the treatment of severe sepsis. Crit Care Med. 2010;38(8):1685–94.
Abraham E, Anzueto A, Gutierrez G, Tessler S, San Pedro G, Wunderink R, et al. Double-blind randomised controlled trial of monoclonal antibody to human tumour necrosis factor in treatment of septic shock. NORASEPT II Study Group Lancet. 1998;351(9107):929–33.
Article CAS Google Scholar
Cohen J, Carlet J. INTERSEPT: an international, multicenter, placebo-controlled trial of monoclonal antibody to human tumor necrosis factor-alpha in patients with sepsis. International Sepsis Trial Study Group. Crit Care Med. 1996;24(9):1431–40.
Fisher CJ Jr, Opal SM, Dhainaut JF, Stephens S, Zimmerman JL, Nightingale P, et al. Influence of an anti-tumor necrosis factor monoclonal antibody on cytokine levels in patients with sepsis. The CB0006 Sepsis Syndrome Study Group. Crit Care Med. 1993;21(3):318–27.
Panacek EA, Marshall JC, Albertson TE, Johnson DH, Johnson S, MacArthur RD, et al. Efficacy and safety of the monoclonal anti-tumor necrosis factor antibody F(ab’)2 fragment afelimomab in patients with severe sepsis and elevated interleukin-6 levels. Crit Care Med. 2004;32(11):2173–82.
Reinhart K, Menges T, Gardlund B, Harm Zwaveling J, Smithes M, Vincent JL, et al. Randomized, placebo-controlled trial of the anti-tumor necrosis factor antibody fragment afelimomab in hyperinflammatory response during severe sepsis: the RAMSES Study. Crit Care Med. 2001;29(4):765–9.
Opal SM, Fisher CJ Jr, Dhainaut JF, Vincent JL, Brase R, Lowry SF, et al. 1997 Confirmatory interleukin-1 receptor antagonist trial in severe sepsis: a phase III, randomized, double-blind, placebo-controlled, multicenter trial The Interleukin-1 Receptor Antagonist Sepsis Investigator Group. Crit Care Med. 1997;25(7):1115–24.
Shakoory B, Carcillo JA, Chatham WW, Amdur RL, Zhao H, Dinarello CA, et al. Interleukin-1 receptor blockade is associated with reduced mortality in sepsis patients with features of macrophage activation syndrome: reanalysis of a prior phase III trial. Crit Care Med. 2016;44(2):275–81.
Meyer NJ, Reilly JP, Anderson BJ, Palakshappa JA, Jones TK, Dunn TG, et al. Mortality benefit of recombinant human interleukin-1 receptor antagonist for sepsis varies by initial interleukin-1 receptor antagonist plasma concentration. Crit Care Med. 2018;46(1):21–8.
Wan B, Zhang H, Fu H, Chen Y, Yang L, Yin J, et al. Recombinant human interleukin-11 (IL-11) is a protective factor in severe sepsis with thrombocytopenia: a case-control study. Cytokine. 2015;76(2):138–43.
François B, Lambden S, Fivez T, Gibot S, Derive M, Grouin JM, et al. Prospective evaluation of the efficacy, safety, and optimal biomarker enrichment strategy for nangibotide, a TREM-1 inhibitor, in patients with septic shock (ASTONISH): a double-blind, randomised, controlled, phase 2b trial. Lancet Respir Med. 2023;11(10):894–904.
Zhang L, Feng Y, Fu P. Blood purification for sepsis: an overview. Precision Clinical Medicine. 2021;4(1):45–55.
Cruz DN, Antonelli M, Fumagalli R, Foltran F, Brienza N, Donati A, et al. Early use of polymyxin B hemoperfusion in abdominal septic shock: the EUPHAS randomized controlled trial. JAMA. 2009;301(23):2445–52.
Payen DM, Guilhot J, Launey Y, Lukaszewicz AC, Kaaki M, Veber B, et al. Early use of polymyxin B hemoperfusion in patients with septic shock due to peritonitis: a multicenter randomized control trial. Intensive Care Med. 2015;41(6):975–84.
Dellinger RP, Bagshaw SM, Antonelli M, Foster DM, Klein DJ, Marshall JC, et al. Effect of targeted polymyxin B hemoperfusion on 28-day mortality in patients with septic shock and elevated endotoxin level: The EUPHRATES randomized clinical trial. JAMA. 2018;320(14):1455–63.
Klein DJ, Foster D, Walker PM, Bagshaw SM, Mekonnen H, Antonelli M. Polymyxin B hemoperfusion in endotoxemic septic shock patients without extreme endotoxemia: a post hoc analysis of the EUPHRATES trial. Intensive Care Med. 2018;44(12):2205–12.
Osawa I, Goto T, Kudo D, Hayakawa M, Yamakawa K, Kushimoto S, et al. Targeted therapy using polymyxin B hemadsorption in patients with sepsis: a post-hoc analysis of the JSEPTIC-DIC study and the EUPHRATES trial. Crit Care. 2023;27(1):245.
Wendel Garcia PD, Hilty MP, Held U, Kleinert EM, Maggiorini M. Cytokine adsorption in severe, refractory septic shock. Inten Care Med. 2021;47(11):1334–6.
Ikeda T, Ikeda K, Nagura M, Taniuchi H, Matsushita M, Kiuchi S, et al. Clinical evaluation of PMX-DHP for hypercytokinemia caused by septic multiple organ failure. Ther Apher Dial. 2004;8(4):293–8.
Caliezi C, Zeerleder S, Redondo M, Regli B, Rothen HU, Zürcher-Zenklusen R, et al. C1-inhibitor in patients with severe sepsis and septic shock: beneficial effect on renal dysfunction. Crit Care Med. 2002;30(8):1722–8.
Igonin AA, Protsenko DN, Galstyan GM, Vlasenko AV, Khachatryan NN, Nekhaev IV, et al. C1-esterase inhibitor infusion increases survival rates for patients with sepsis*. Crit Care Med. 2012;40(3):770–7.
Bernard GR, Vincent JL, Laterre PF, LaRosa SP, Dhainaut JF, Lopez-Rodriguez A, et al. Efficacy and safety of recombinant human activated protein C for severe sepsis. N Engl J Med. 2001;344(10):699–709.
Ranieri VM, Thompson BT, Barie PS, Dhainaut JF, Douglas IS, Finfer S, et al. Drotrecogin alfa (activated) in adults with septic shock. N Engl J Med. 2012;366(22):2055–64.
Gando S, Saitoh D, Ishikura H, Ueyama M, Otomo Y, Oda S, et al. A randomized, controlled, multicenter trial of the effects of antithrombin on disseminated intravascular coagulation in patients with sepsis. Crit Care. 2013;17(6):R297.
Inthorn D, Hoffmann JN, Hartl WH, Mühlbayer D, Jochum M. Antithrombin III supplementation in severe sepsis: beneficial effects on organ dysfunction. Shock. 1997;8(5):328–34.
Warren BL, Eid A, Singer P, Pillay SS, Carl P, Novak I, et al. Caring for the critically ill patient High-dose antithrombin III in severe sepsis: a randomized controlled trial. JAMA. 2001;286(15):1869–78.
Vincent JL, Francois B, Zabolotskikh I, Daga MK, Lascarrou JB, Kirov MY, et al. Effect of a recombinant human soluble thrombomodulin on mortality in patients with sepsis-associated coagulopathy: the SCARLET randomized clinical trial. JAMA. 2019;321(20):1993–2002.
John J, Awab A, Norman D, Dernaika T, Kinasewitz GT. Activated protein C improves survival in severe sepsis patients with elevated troponin. Intensive Care Med. 2007;33(12):2122–8.
Levi M, Vincent JL, Tanaka K, Radford AH, Kayanoki T, Fineberg DA, et al. Effect of a recombinant human soluble thrombomodulin on baseline coagulation biomarker levels and mortality outcome in patients with sepsis-associated coagulopathy. Crit Care Med. 2020;48(8):1140–7.
Kudo D, Goto T, Uchimido R, Hayakawa M, Yamakawa K, Abe T, et al. Coagulation phenotypes in sepsis and effects of recombinant human thrombomodulin: an analysis of three multicentre observational studies. Crit Care. 2021;25(1):114.
Hayakawa M, Yamakawa K, Kudo D, Ono K. Optimal antithrombin activity threshold for initiating antithrombin supplementation in patients with sepsis-induced disseminated intravascular coagulation: a multicenter retrospective observational study. Clin Appl Thromb Hemost. 2018;24(6):874–83.
Sinha P, Kerchberger VE, Willmore A, Chambers J, Zhuo H, Abbott J, et al. Identifying molecular phenotypes in sepsis: an analysis of two prospective observational cohorts and secondary analysis of two randomised controlled trials. Lancet Respir Med. 2023;11(11):965–74.
Giamarellos-Bourboulis EJ. Immunomodulatory therapies for sepsis: unexpected effects with macrolides. Int J Antimicrob Agents. 2008;32(Suppl 1):S39-43.
Karakike E, Scicluna BP, Roumpoutsou M, Mitrou I, Karampela N, Karageorgos A, et al. Effect of intravenous clarithromycin in patients with sepsis, respiratory and multiple organ dysfunction syndrome: a randomized clinical trial. Crit Care. 2022;26(1):183.
Annane D, Renault A, Brun-Buisson C, Megarbane B, Quenot JP, Siami S, et al. Hydrocortisone plus fludrocortisone for adults with septic shock. N Engl J Med. 2018;378(9):809–18.
Venkatesh B, Finfer S, Cohen J, Rajbhandari D, Arabi Y, Bellomo R, et al. Adjunctive glucocorticoid therapy in patients with septic shock. N Engl J Med. 2018;378(9):797–808.
Dequin PF, Meziani F, Quenot JP, Kamel T, Ricard JD, Badie J, et al. Hydrocortisone in severe community-acquired pneumonia. N Engl J Med. 2023;388(21):1931–41.
Lamontagne F, Masse MH, Menard J, Sprague S, Pinto R, Heyland DK, et al. Intravenous vitamin C in adults with sepsis in the intensive care unit. N Engl J Med. 2022;386(25):2387–98.
Sevransky JE, Rothman RE, Hager DN, Bernard GR, Brown SM, Buchman TG, et al. Effect of vitamin C, thiamine, and hydrocortisone on ventilator- and vasopressor-free days in patients with sepsis: the VICTAS randomized clinical trial. JAMA. 2021;325(8):742–50.
Lyu QQ, Zheng RQ, Chen QH, Yu JQ, Shao J, Gu XH. Early administration of hydrocortisone, vitamin C, and thiamine in adult patients with septic shock: a randomized controlled clinical trial. Crit Care. 2022;26(1):295.
Mohamed A, Abdelaty M, Saad MO, Shible A, Mitwally H, Akkari AR, et al. Evaluation of hydrocortisone, vitamin c, and thiamine for the treatment of septic shock: a randomized controlled trial (the hyvits trial). Shock. 2023;59(5):697–701.
Tsaganos T, Raftogiannis M, Pratikaki M, Christodoulou S, Kotanidou A, Papadomichelakis E, et al. Clarithromycin leads to long-term survival and cost benefit in ventilator-associated pneumonia and sepsis. Antimicrob Agents Chemother. 2016;60(6):3640–6.
Torres A, Sibila O, Ferrer M, Polverino E, Menendez R, Mensa J, et al. Effect of corticosteroids on treatment failure among hospitalized patients with severe community-acquired pneumonia and high inflammatory response: a randomized clinical trial. JAMA. 2015;313(7):677–86.
Antcliffe DB, Burnham KL, Al-Beidh F, Santhakumaran S, Brett SJ, Hinds CJ, et al. Transcriptomic signatures in sepsis and a differential response to steroids from the VANISH randomized trial. Am J Respir Crit Care Med. 2019;199(8):980–6.
Wong HR, Hart KW, Lindsell CJ, Sweeney TE. External corroboration that corticosteroids may be harmful to septic shock endotype a patients. Crit Care Med. 2021;49(1):e98–101.
Yao L, Rey DA, Bulgarelli L, Kast R, Osborn J, Van Ark E, et al. Gene expression scoring of immune activity levels for precision use of hydrocortisone in vasodilatory shock. Shock. 2022;57(3):384–91.
Cohen J, Blumenthal A, Cuellar-Partida G, Evans DM, Finfer S, Li Q, et al. The relationship between adrenocortical candidate gene expression and clinical response to hydrocortisone in patients with septic shock. Intensive Care Med. 2021;47(9):974–83.
Pirracchio R, Hubbard A, Sprung CL, Chevret S, Annane D. Assessment of machine learning to estimate the individual treatment effect of corticosteroids in septic shock. JAMA Netw Open. 2020;3(12): e2029050.
Hellali R, Chelly Dagdia Z, Ktaish A, Zeitouni K, Annane D. Corticosteroid sensitivity detection in sepsis patients using a personalized data mining approach: a clinical investigation. Comput Methods Programs Biomed. 2024;245: 108017.
König R, Kolte A, Ahlers O, Oswald M, Krauss V, Roell D, et al. Use of IFNγ/IL10 ratio for stratification of hydrocortisone therapy in patients with septic shock. Front Immunol. 2021;12: 607217.
Boyle AJ, Ferris P, Bradbury I, Conlon J, Shankar-Hari M, Rogers AJ, et al. Baseline plasma IL-18 may predict simvastatin treatment response in patients with ARDS: a secondary analysis of the HARP-2 randomised clinical trial. Crit Care. 2022;26(1):164.
Presneill JJ, Harris T, Stewart AG, Cade JF, Wilson JW. A randomized phase II trial of granulocyte-macrophage colony-stimulating factor therapy in severe sepsis with respiratory dysfunction. Am J Respir Crit Care Med. 2002;166(2):138–43.
Meisel C, Schefold JC, Pschowski R, Baumann T, Hetzger K, Gregor J, et al. Granulocyte-macrophage colony-stimulating factor to reverse sepsis-associated immunosuppression: a double-blind, randomized, placebo-controlled multicenter trial. Am J Respir Crit Care Med. 2009;180(7):640–8.
Schefold JC, Zeden JP, Pschowski R, Hammoud B, Fotopoulou C, Hasper D, et al. Treatment with granulocyte-macrophage colony-stimulating factor is associated with reduced indoleamine 2,3-dioxygenase activity and kynurenine pathway catabolites in patients with severe sepsis and septic shock. Scand J Infect Dis. 2010;42(3):164–71.
Vacheron CH, Lepape A, Venet F, Monneret G, Gueyffier F, Boutitie F, et al. Granulocyte-macrophage colony-stimulating factor (GM-CSF) in patients presenting sepsis-induced immunosuppression: the GRID randomized controlled trial. J Crit Care. 2023;78: 154330.
Francois B, Jeannet R, Daix T, Walton AH, Shotwell MS, Unsinger J, et al. Interleukin-7 restores lymphocytes in septic shock: the IRIS-7 randomized clinical trial. JCI Insight. 2018. https://doi.org/10.1172/jci.insight.98960 .
Dominioni L, Dionigi R, Zanello M, Chiaranda M, Dionigi R, Acquarolo A, et al. Effects of high-dose IgG on survival of surgical patients with sepsis scores of 20 or greater. Arch Surg. 1991;126(2):236–40.
Werdan K, Pilz G, Bujdoso O, Fraunberger P, Neeser G, Schmieder RE, et al. Score-based immunoglobulin G therapy of patients with sepsis: the SBITS study. Crit Care Med. 2007;35(12):2693–701.
CAS PubMed Google Scholar
Akatsuka M, Masuda Y, Tatsumi H, Sonoda T. Efficacy of intravenous immunoglobulin therapy for patients with sepsis and low immunoglobulin G Levels: a single-center retrospective study. Clin Ther. 2022;44(2):295–303.
Behre G, Schedel I, Nentwig B, Wörmann B, Essink M, Hiddemann W. Endotoxin concentration in neutropenic patients with suspected gram-negative sepsis: correlation with clinical outcome and determination of anti-endotoxin core antibodies during therapy with polyclonal immunoglobulin M-enriched immunoglobulins. Antimicrob Agents Chemother. 1992;36(10):2139–46.
Welte T, Dellinger RP, Ebelt H, Ferrer M, Opal SM, Singer M, et al. Efficacy and safety of trimodulin, a novel polyclonal antibody preparation, in patients with severe community-acquired pneumonia: a randomized, placebo-controlled, double-blind, multicenter, phase II trial (CIGMA study). Intensive Care Med. 2018;44(4):438–48.
Hentrich M, Fehnle K, Ostermann H, Kienast J, Cornely O, Salat C, et al. IgMA-enriched immunoglobulin in neutropenic patients with sepsis syndrome and septic shock: a randomized, controlled, multiple-center trial. Crit Care Med. 2006;34(5):1319–25.
Galstyan G, Makarova P, Parovichnikova E, Kuzmina L, Troitskaya V, Ghemdzhian E. The results of the single center pilot randomized Russian clinical trial of mesenchymal stromal cells in severe neutropenic patients with septic shock (RUMCESS). Int J Blood Res Disord. 2018;5(1):33.
Google Scholar
Hotchkiss RS, Colston E, Yende S, Angus DC, Moldawer LL, Crouser ED, et al. Immune checkpoint inhibition in sepsis: a phase 1b randomized, placebo-controlled, single ascending dose study of antiprogrammed cell death-ligand 1 antibody (BMS-936559). Crit Care Med. 2019;47(5):632–42.
Hotchkiss RS, Colston E, Yende S, Crouser ED, Martin GS, Albertson T, et al. Immune checkpoint inhibition in sepsis: a Phase 1b randomized study to evaluate the safety, tolerability, pharmacokinetics, and pharmacodynamics of nivolumab. Intensive Care Med. 2019;45(10):1360–71.
Watanabe E, Nishida O, Kakihana Y, Odani M, Okamura T, Harada T, Oda S. Pharmacokinetics, pharmacodynamics, and safety of nivolumab in patients with sepsis-induced immunosuppression: a multicenter, open-label phase 1/2 study. Shock. 2020;53(6):686–94.
Kotsaki A, Pickkers P, Bauer M, Calandra T, Lupse M, Wiersinga WJ, et al. ImmunoSep (Personalised Immunotherapy in Sepsis) international double-blind, double-dummy, placebo-controlled randomised clinical trial: study protocol. BMJ Open. 2022;12(12): e067251.
Rhodes A, Evans LE, Alhazzani W, Levy MM, Antonelli M, Ferrer R, et al. Surviving sepsis campaign: international guidelines for management of sepsis and septic shock: 2016. Intensive Care Med. 2017;43(3):304–77.
Hotchkiss RS, Monneret G, Payen D. Sepsis-induced immunosuppression: from cellular dysfunctions to immunotherapy. Nat Rev Immunol. 2013;13(12):862–74.
Fleuriet J, Heming N, Meziani F, Reignier J, Declerq P-L, Mercier E, et al. Rapid rEcognition of COrticosteRoiD resistant or sensitive Sepsis (RECORDS): study protocol for a multicentre, placebo-controlled, biomarker-guided, adaptive Bayesian design basket trial. BMJ Open. 2023;13(3): e066496.
Arksey H, O’Malley L. Scoping studies: towards a methodological framework. Int J Soc Res Methodol. 2005;8(1):19–32.
Singer M, Deutschman CS, Seymour CW, Shankar-Hari M, Annane D, Bauer M, et al. The third international consensus definitions for sepsis and septic shock (Sepsis-3). JAMA. 2016;315(8):801–10.
Download references
Acknowledgements
Authors would like to gratefully thank Mrs. Faridi van Etten-Jamaludin (Medical Library, Amsterdam UMC, location AMC), who helped as an academic librarian to build the search equations, to search the databases and to exclude the duplicates and Maartje Kunen for help in the preparation of the figures.
the ImmunoSep Consortium
Bart-Jan Kullberg: [email protected]; Aline de Nooijer: [email protected]; Frank van de Veerdonk: [email protected]; Jaap ten Oever: [email protected]; Jacobien Hoogerwerf: [email protected]; Marlies Hulscher: [email protected]; Mihai Netea: [email protected]; Anke Oerlemans: [email protected]; Peter Pickkers: [email protected]; Athanasios Ziogas: [email protected]; Julie Swillens: [email protected]; Lisa van de Berg: [email protected]; Lieke Bakkerus: [email protected]; Nynke Bos: [email protected]; Matthijs Kox: [email protected]; Leda Estratiou: [email protected]; Evangelos Giamarellos-Bourboulis: [email protected]; Antigoni Kotsaki: [email protected]; Antonakos Nikolaos : [email protected]; Gregoriadis Spyros: [email protected]; Thierry Calandra: [email protected]; Sylvain Meylan : [email protected]; Tiia Snaka: [email protected]; Thierry Roger: [email protected]; Michael Bauer: [email protected]; Frank Brunkhorst: [email protected]; Frank Bloos: [email protected]; Sebastian Weis: [email protected]; Willy Hartman: [email protected]; Tom van der Poll: [email protected]; Marleen Slim : [email protected]; Niels van Mourik: [email protected]; Lonneke van Vught: [email protected]; Alexander Vlaar: [email protected]; Marcela Muller: [email protected]; Joost Wiersinga : [email protected]; Mihaela Lupse: [email protected]; Grigore Santamarean: [email protected]; Thomas Rimmele: [email protected]; Filippo Conti : [email protected]; Guillaume Monneret: [email protected]; Anna Aschenbrenner: [email protected]; Joachim Schultze: [email protected]; Martina van Uelft : [email protected]; Christoph Bock: [email protected]; Robert terHorst: [email protected]; Irit Gat-Viks: [email protected]; Einat Ron: [email protected]; Gal Yunkovitz: [email protected]; Sophie Ablott: [email protected]; Estelle Peronnet: [email protected]; Margaux Balezeaux: [email protected]; Adrien Saliou: [email protected]; Julie Hart: [email protected].
MAS, NvM, LB, MGN, PP, EJG-B, TvdP, WJW and APJV are supported by the European Commission (Horizon 2020, ImmunoSep, Grant No. 847422).
Author information
Marleen A. Slim and Niels van Mourik have contributed equally to this manuscript.
Alexander P.J. Vlaar and Lonneke A. van Vught have contributed equally to this manuscript.
Authors and Affiliations
Department of Intensive Care Medicine, Amsterdam University Medical Center, Meibergdreef 9, Room G3-220, 1105 AZ, Amsterdam, The Netherlands
Marleen A. Slim, Niels van Mourik, Marcella C. A. Müller, Alexander P. J. Vlaar & Lonneke A. van Vught
Center for Experimental and Molecular Medicine, Amsterdam University Medical Center, University of Amsterdam, Amsterdam, The Netherlands
Marleen A. Slim, Niels van Mourik, Tom van der Poll, W. Joost Wiersinga & Lonneke A. van Vught
Department of Internal Medicine and Radboud Center for Infectious Diseases, Radboud University Medical Center, Nijmegen, The Netherlands
Lieke Bakkerus & Mihai G. Netea
Department of Medicine, McMaster University, Hamilton, Canada
Katherine Fuller, Lydia Acharya, Tatiana Giannidis, Joanna C. Dionne & Simon J. W. Oczkowski
The Guidelines in Intensive Care Development and Evaluation (GUIDE) Group, Research Institute St. Joseph’s Healthcare Hamilton, Hamilton, Canada
Joanna C. Dionne & Simon J. W. Oczkowski
Department of Health Research Methods, Evidence, and Impact, McMaster University, Hamilton, Canada
Division of Gastroenterology, McMaster University, Hamilton, ON, Canada
Joanna C. Dionne
Department of Intensive Care Medicine, Radboud University Medical Center, Nijmegen, The Netherlands
Peter Pickkers
4th Department of Internal Medicine, Medical School, National and Kapodistrian University of Athens, Athens, Greece
Evangelos J. Giamarellos-Bourboulis
Department of Internal Medicine, Division of Infectious Diseases, Amsterdam University Medical Center, University of Amsterdam, Amsterdam, The Netherlands
Tom van der Poll & W. Joost Wiersinga
You can also search for this author in PubMed Google Scholar
in collaboration with the ImmunoSep Consortium
- Bart-Jan Kullberg
- , Aline Nooijer
- , Frank Veerdonk
- , Jaap Oever
- , Jacobien Hoogerwerf
- , Marlies Hulscher
- , Mihai Netea
- , Anke Oerlemans
- , Athanasios Ziogas
- , Julie Swillens
- , Lisa Berg
- , Nynke Bos
- , Matthijs Kox
- , Leda Estratiou
- , Evangelos Giamarellos-Bourboulis
- , Antigoni Kotsaki
- , Antonakos Nikolaos
- , Gregoriadis Spyros
- , Thierry Calandra
- , Sylvain Meylan
- , Tiia Snaka
- , Thierry Roger
- , Michael Bauer
- , Frank Brunkhorst
- , Frank Bloos
- , Sebastian Weis
- , Willy Hartman
- , Marleen Slim
- , Lonneke Vught
- , Alexander Vlaar
- , Marcela Muller
- , Joost Wiersinga
- , Mihaela Lupse
- , Grigore Santamarean
- , Thomas Rimmele
- , Filippo Conti
- , Guillaume Monneret
- , Anna Aschenbrenner
- , Joachim Schultze
- , Martina Uelft
- , Christoph Bock
- , Robert terHorst
- , Irit Gat-Viks
- , Einat Ron
- , Gal Yunkovitz
- , Sophie Ablott
- , Estelle Peronnet
- , Margaux Balezeaux
- , Adrien Saliou
- & Julie Hart
Contributions
MAS, TvdP, WJW, APJV and LAvV initiated the project, MAS and NvM lead the project. MAS, NvM, JCD, SJWO, MGN, MCAM, APJV and LAvV contributed to the conception and design of the study. JCD and SJWO helped as methodologists to design the study and present the results. All authors contributed to the acquisition of data, or the analysis and interpretation of the data; MAS, NvM, LB, KF, LA, TG, JCD, SJWO and LAvV drafted the manuscript. All authors provided critical revision of the article and provided final approval of the version submitted for publication.
Corresponding author
Correspondence to Marleen A. Slim .
Ethics declarations
Ethics approval and consent to participate, consent for publication, competing interests.
SJWO has received travel support from Fisher & Paykel Healthcare. MGN is a scientific founder of TTxD, Lemba and Biotrip. PP has received travel reimbursements and consulting fees from AM-Pharma, Adrenomed, EBI Paion, Sphingotec, and 4Teen4. EJG-B has received honoraria from Abbott Products Operations AG, bioMérieux, Brahms GmbH, GSK, InflaRx GmbH, Sobi and Xbiotech Inc; independent educational grants from Abbott Products Operations, bioMérieux Inc, InflaRx GmbH, Johnson & Johnson, MSD, UCB, Swedish Orphan Biovitrum AB; and funding from the Horizon 2020 European Grants ImmunoSep and RISCinCOVID and the Horizon Health grant EPIC-CROWN-2 (granted to the Hellenic Institute for the Study of Sepsis). WJW reports a project grants from EU, Amsterdam UMC and NOW/ZonMW and receives ad hoc consulting fees from GSK, Pfizer, Sobi, AstraZeneca paid to the institution. APJV receives consultant fees from InflaRx paid to the institution. LAvV was supported by the Netherlands Organisation for Health Research and Development ZonMW (Nederlandse Organisatie voor Wetenschappelijk Onderzoek NWO) VENI grant 09150161910033.
Additional information
Publisher's note.
Springer Nature remains neutral with regard to jurisdictional claims in published maps and institutional affiliations.
Supplementary Information
Supplementary material 1., rights and permissions.
Open Access This article is licensed under a Creative Commons Attribution 4.0 International License, which permits use, sharing, adaptation, distribution and reproduction in any medium or format, as long as you give appropriate credit to the original author(s) and the source, provide a link to the Creative Commons licence, and indicate if changes were made. The images or other third party material in this article are included in the article's Creative Commons licence, unless indicated otherwise in a credit line to the material. If material is not included in the article's Creative Commons licence and your intended use is not permitted by statutory regulation or exceeds the permitted use, you will need to obtain permission directly from the copyright holder. To view a copy of this licence, visit http://creativecommons.org/licenses/by/4.0/ . The Creative Commons Public Domain Dedication waiver ( http://creativecommons.org/publicdomain/zero/1.0/ ) applies to the data made available in this article, unless otherwise stated in a credit line to the data.
Reprints and permissions
About this article
Cite this article.
Slim, M.A., van Mourik, N., Bakkerus, L. et al. Towards personalized medicine: a scoping review of immunotherapy in sepsis. Crit Care 28 , 183 (2024). https://doi.org/10.1186/s13054-024-04964-6
Download citation
Received : 13 March 2024
Accepted : 20 May 2024
Published : 28 May 2024
DOI : https://doi.org/10.1186/s13054-024-04964-6
Share this article
Anyone you share the following link with will be able to read this content:
Sorry, a shareable link is not currently available for this article.
Provided by the Springer Nature SharedIt content-sharing initiative
- Immunomodulation
- Immunotherapy
- Personalized
Critical Care
ISSN: 1364-8535
- Submission enquiries: [email protected]
- U.S. Department of Health & Human Services

- Virtual Tour
- Staff Directory
- En Español
You are here
News releases.
Media Advisory
Thursday, May 30, 2024
Novel vaccine concept generates immune responses that could produce multiple types of HIV broadly neutralizing antibodies
NIH-funded animal model results will inform vaccine development in humans.

Using a combination of cutting-edge immunologic technologies, researchers have successfully stimulated animals’ immune systems to induce rare precursor B cells of a class of HIV broadly neutralizing antibodies (bNAbs). The findings, published today in Nature Immunology , are an encouraging, incremental step in developing a preventive HIV vaccine.
HIV is genetically diverse making the virus difficult to target with a vaccine, but bNAbs may overcome that hurdle because they bind to parts of the virus that remain constant even when it mutates. Germline targeting is an immune system-stimulating approach that guides naïve (precursor) B cells to develop into mature B cells that can produce bNAbs. A class of bNAbs called 10E8 is a priority for HIV vaccine development because it neutralizes a particularly broad range of HIV variants. The 10E8 bNAb binds to a conserved region of the glycoprotein gp41 on HIV’s surface involved in its entry into human immune cells. Designing an immunogen—a molecule used in a vaccine that elicits a specific immune system response—to stimulate production of 10E8 bNAbs has been challenging because that key region of gp41 is hidden in a recessed crevice on HIV’s surface. Prior vaccine immunogens have not generated bNAbs with the physical structure to reach and bind to gp41.
To address this challenge, the researchers engineered immunogens on nanoparticles that mimic the appearance of a specific part of gp41. They vaccinated rhesus macaque monkeys and mice with those immunogens and elicited specific responses from the 10E8 B cell precursors and induced antibodies that showed signs of maturing into bNAbs that could reach the hidden gp41 region. They observed similar responses when they used mRNA-encoded nanoparticles in mice. The researchers also found that the same immunogens produced B cells that could mature to produce an additional type of gp41-directed bNAb called LN01. Finally, their laboratory analysis of human blood samples found that 10E8-class bNAb precursors occurred naturally in people without HIV, and that their immunogens bound to and isolated naïve human B cells with 10E8-like features. Together these observations suggest that the promising immunization data from mice and macaques has the potential for translation to humans.
The research was conducted by the Scripps Consortium for HIV/AIDS Vaccine Development, one of two consortia supported by the National Institutes of Health’s (NIH) National Institute of Allergy and Infectious Diseases (NIAID). The research also was supported by collaborating partners including the Bill & Melinda Gates Foundation and other NIH Institutes and Offices. According to the authors, these findings support the development of the immunogens as the first part of a multi-step vaccine regimen for humans. Their work further supports research in developing a germline-targeting strategy for priming the immune system to elicit a bNAb called VRC01. This bNAb was discovered by NIAID researchers almost 15 years ago. The goal of this line of research is to develop an HIV vaccine that generates multiple classes of bNAbs to prevent HIV.
Schiffner et al . Vaccination induces broadly neutralizing antibody precursors to HIV gp41. Nature Immunology DOI: 10.1038/s41590-024-01833-w (2024).
Angela Malaspina, program officer in NIAID’s Division of AIDS, is available to discuss this study.
NIAID conducts and supports research—at NIH, throughout the United States, and worldwide—to study the causes of infectious and immune-mediated diseases, and to develop better means of preventing, diagnosing and treating these illnesses. News releases, fact sheets and other NIAID-related materials are available on the NIAID website .
About the National Institutes of Health (NIH): NIH, the nation's medical research agency, includes 27 Institutes and Centers and is a component of the U.S. Department of Health and Human Services. NIH is the primary federal agency conducting and supporting basic, clinical, and translational medical research, and is investigating the causes, treatments, and cures for both common and rare diseases. For more information about NIH and its programs, visit www.nih.gov .
NIH…Turning Discovery Into Health ®
Connect with Us
- More Social Media from NIH
Suggestions or feedback?

MIT News | Massachusetts Institute of Technology
- Machine learning
- Social justice
- Black holes
- Classes and programs
Departments
- Aeronautics and Astronautics
- Brain and Cognitive Sciences
- Architecture
- Political Science
- Mechanical Engineering
Centers, Labs, & Programs
- Abdul Latif Jameel Poverty Action Lab (J-PAL)
- Picower Institute for Learning and Memory
- Lincoln Laboratory
- School of Architecture + Planning
- School of Engineering
- School of Humanities, Arts, and Social Sciences
- Sloan School of Management
- School of Science
- MIT Schwarzman College of Computing
Adhesive coatings can prevent scarring around medical implants
Press contact :, media download.
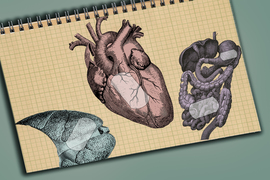
*Terms of Use:
Images for download on the MIT News office website are made available to non-commercial entities, press and the general public under a Creative Commons Attribution Non-Commercial No Derivatives license . You may not alter the images provided, other than to crop them to size. A credit line must be used when reproducing images; if one is not provided below, credit the images to "MIT."
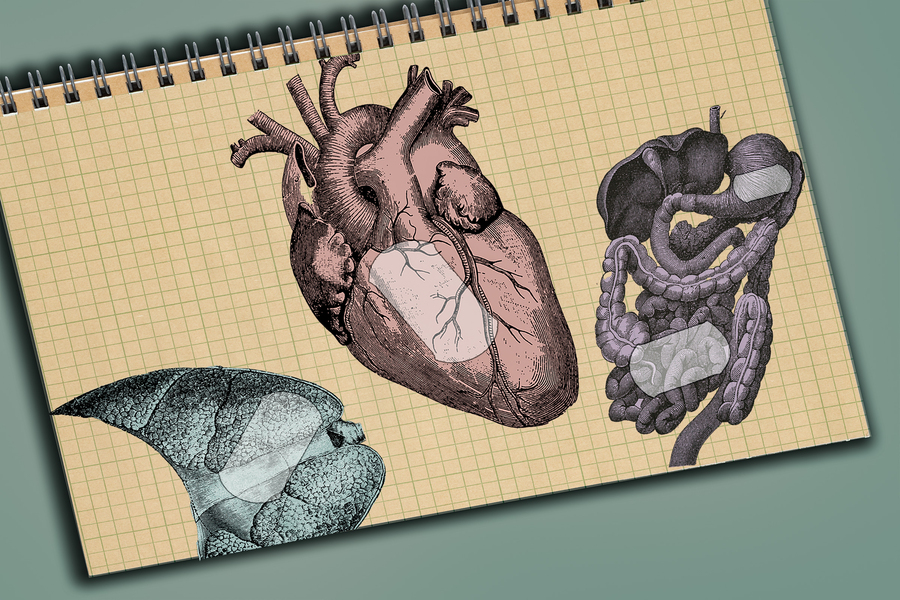
Previous image Next image
When medical devices such as pacemakers are implanted in the body, they usually provoke an immune response that leads to buildup of scar tissue around the implant. This scarring, known as fibrosis, can interfere with the devices’ function and may require them to be removed.
In an advance that could prevent that kind of device failure, MIT engineers have found a simple and general way to eliminate fibrosis by coating devices with a hydrogel adhesive. This adhesive binds the devices to tissue and prevents the immune system from attacking it.
“The dream of many research groups and companies is to implant something into the body that over the long term the body will not see, and the device can provide therapeutic or diagnostic functionality. Now we have such an ‘invisibility cloak,’ and this is very general: There’s no need for a drug, no need for a special polymer,” says Xuanhe Zhao, an MIT professor of mechanical engineering and of civil and environmental engineering.
The adhesive that the researchers used in this study is made from cross-linked polymers called hydrogels, and is similar to a surgical tape they previously developed to help seal internal wounds. Other types of hydrogel adhesives can also protect against fibrosis, the researchers found, and they believe this approach could be used for not only pacemakers but also sensors or devices that deliver drugs or therapeutic cells.
Zhao and Hyunwoo Yuk SM ’16, PhD ’21, a former MIT research scientist who is now the chief technology officer at SanaHeal, are the senior authors of the study, which appears today in Nature . MIT postdoc Jingjing Wu is the lead author of the paper.
Preventing fibrosis
In recent years, Zhao’s lab has developed adhesives for a variety of medical applications, including double-sided and single-sided tapes that could be used to heal surgical incisions or internal injuries. These adhesives work by rapidly absorbing water from wet tissues, using polyacrylic acid, an absorbent material used in diapers. Once the water is cleared, chemical groups called NHS esters embedded in the polyacrylic acid form strong bonds with proteins at the tissue surface. This process takes about five seconds.
Several years ago, Zhao and Yuk began exploring whether this kind of adhesive could also help keep medical implants in place and prevent fibrosis from occurring.
To test this idea, Wu coated polyurethane devices with their adhesive and implanted them on the abdominal wall, colon, stomach, lung, or heart of rats. Weeks later, they removed the device and found that there was no visible scar tissue. Additional tests with other animal models showed the same thing: Wherever the adhesive-coated devices were implanted, fibrosis did not occur, for up to three months.
“This work really has identified a very general strategy, not only for one animal model, one organ, or one application,” Wu says. “Across all of these animal models, we have consistent, reproducible results without any observable fibrotic capsule.”
Using bulk RNA sequencing and fluorescent imaging, the researchers analyzed the animals’ immune response and found that when devices with adhesive coatings were first implanted, immune cells such as neutrophils began to infiltrate the area. However, the attacks quickly quenched out before any scar tissue could form.
“For the adhered devices, there is an acute inflammatory response because it is a foreign material,” Yuk says. “However, very quickly that inflammatory response decayed, and then from that point you do not have this fibrosis formation.”
One application for this adhesive could be coatings for epicardial pacemakers — devices that are placed on the heart to help control the heart rate. The wires that contact the heart often become fibrotic, but the MIT team found that when they implanted adhesive-coated wires in rats, they remained functional for at least three months, with no scar tissue formation.
“The formation of fibrotic tissue at the interface between implanted medical devices and the target tissue is a longstanding problem that routinely causes failure of the device. The demonstration that robust adhesion between the device and the tissue obviates fibrotic tissue formation is an important observation that has many potential applications in the medical device space,” says David Mooney, a professor of bioengineering at Harvard University, who was not involved in the study.
Mechanical cues
The researchers also tested a hydrogel adhesive that includes chitosan, a naturally occurring polysaccharide, and found that this adhesive also eliminated fibrosis in animal studies. However, two commercially available tissue adhesives that they tested did not show this antifibrotic effect because the commercially available adhesives eventually detached from the tissue and allowed the immune system to attack.
In another experiment, the researchers coated implants in hydrogel adhesives but then soaked them in a solution that removed the polymers’ adhesive properties, while keeping their overall chemical structure the same. After being implanted in the body, where they were held in place by sutures, fibrotic scarring occurred. This suggests that there is something about the mechanical interaction between the adhesive and the tissue that prevents the immune system from attacking, the researchers say.
“Previous research in immunology has been focused on chemistry and biochemistry, but mechanics and physics may play equivalent roles, and we should pay attention to those mechanical and physical cues in immunological responses,” says Zhao, who now plans to further investigate how those mechanical cues affect the immune system.
Yuk, Zhao, and others have started a company called SanaHeal, which is now working on further developing tissue adhesives for medical applications.
“As a team, we are interested in reporting this to the community and sparking speculation and imagination as to where this can go,” Yuk says. “There are so many scenarios in which people want to interface with foreign or manmade material in the body, like implantable devices, drug depots, or cell depots.”
The research was funded by the National Institutes of Health and the National Science Foundation.
Share this news article on:
Press mentions, interesting engineering.
MIT engineers have developed a new adhesive, low-cost hydrogel that can stop fibrosis often experienced by people with pacemakers and other medical devices, reports for Maria Bolevich Interesting Engineering . “These findings may offer a promising strategy for long-term anti-fibrotic implant–tissue interfaces,” explains Prof. Xuanhe Zhao.
Previous item Next item
Related Links
- Xuanhe Zhao
- Hyunwoo Yuk
- Jingjing Wu
- Department of Mechanical Engineering
- Department of Civil and Environmental Engineering
Related Topics
- Mechanical engineering
- Civil and environmental engineering
- Biomedical engineering
- Medical devices
- National Institutes of Health (NIH)
- National Science Foundation (NSF)
Related Articles
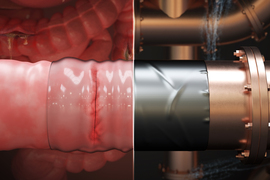
Engineers develop surgical “duct tape” as an alternative to sutures
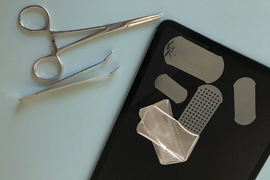
Double-sided tape for tissues could replace surgical sutures
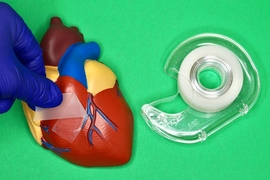
Super-strong surgical tape detaches on demand
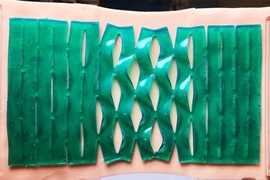
Paper-folding art inspires better bandages
More mit news.
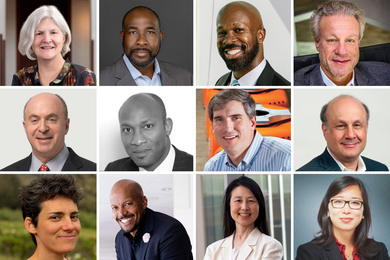
MIT Corporation elects 10 term members, two life members
Read full story →
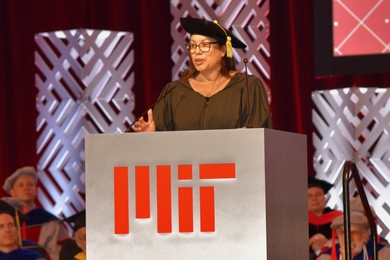
Diane Hoskins ’79: How going off-track can lead new SA+P graduates to become integrators of ideas
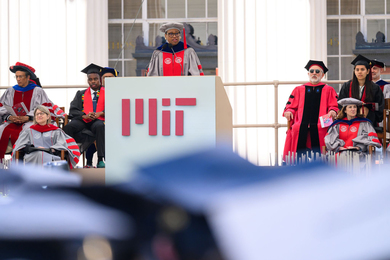
Chancellor Melissa Nobles’ address to MIT’s undergraduate Class of 2024
Noubar Afeyan PhD ’87 gives new MIT graduates a special assignment
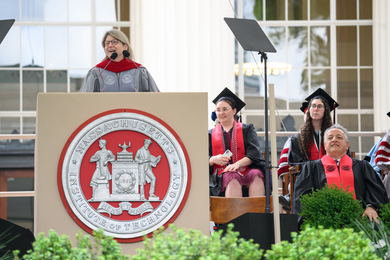
President Sally Kornbluth’s charge to the Class of 2024
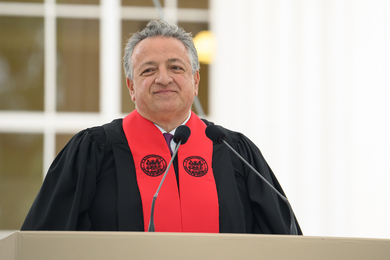
Commencement address by Noubar Afeyan PhD ’87
- More news on MIT News homepage →
Massachusetts Institute of Technology 77 Massachusetts Avenue, Cambridge, MA, USA
- Map (opens in new window)
- Events (opens in new window)
- People (opens in new window)
- Careers (opens in new window)
- Accessibility
- Social Media Hub
- MIT on Facebook
- MIT on YouTube
- MIT on Instagram

An official website of the United States government
The .gov means it’s official. Federal government websites often end in .gov or .mil. Before sharing sensitive information, make sure you’re on a federal government site.
The site is secure. The https:// ensures that you are connecting to the official website and that any information you provide is encrypted and transmitted securely.
- Publications
- Account settings
Preview improvements coming to the PMC website in October 2024. Learn More or Try it out now .
- Advanced Search
- Journal List
- Elsevier - PMC COVID-19 Collection

Immune response in COVID-19: A review
Mohammad asaduzzaman chowdhury.
a Department of Mechanical Engineering, Dhaka University of Engineering and Technology, Gazipur, Gazipur 1707, Bangladesh
Nayem Hossain
b Department of Mechanical Engineering, IUBAT—International University of Business Agriculture and Technology, Bangladesh
Mohammod Abul Kashem
c Department of Computer Science and Engineering, Dhaka University of Engineering and Technology, Gazipur, Gazipur 1707,Bangladesh
Md. Abdus Shahid
d Department of Textile Engineering, Dhaka University of Engineering and Technology, Gazipur, Gazipur 1707, Bangladesh
Ashraful Alam
e University of South Asia, House 76 & 78, Rd No.14, Dhaka 1212, Bangladesh
The immune system protects against viruses and diseases and produces antibodies to kill pathogens. This review presents a brief overview of the immune system regarding its protection of the human body from COVID-19; illustrates the process of the immune system, how it works, and its mechanism to fight virus; and presents information on the most recent COVID-19 treatments and experimental data. Various types of potential challenges for the immunes system are also discussed. At the end of the article, foods to consume and avoid are suggested, and physical exercise is encouraged. This article can be used worldwide as a state of the art in this critical moment for promising alternative solutions related to surviving the coronavirus.
Introduction
The earth is relaxing but humans are dying. As of April 18, 2020, more than 154,000 have people died, 2.2 million have been affected, and at least 185 countries have been affected by the coronavirus. The world experienced coronavirus for the first time in 2002–2003 through severe acute respiratory syndrome (SARS), and in 2011, Middle East respiratory syndrome (MERS) for the first time. The causative agents for both cases (SARS-CoV and MERS-CoV,) were newly identified coronaviruses of zoonotic origin in the genus Beta coronavirus [ 1 ]. The present coronavirus (SARS-CoV-2) COVID-19 appeared for the first time in Wuhan, China, at the end of 2019. People are being affected by human-to-human transmission due to close contact [ 2 , 3 ], and people affected by COVID-19 suffer from severe respiratory illness [ 4 ]. People who are elderly and have many comorbidities are the most vulnerable to COVID-19 [ 5 , 6 ].
There is no registered treatment or vaccine for this disease [ 7 ]. For the treatment of affected people, limited urgent use of chloroquine and hydroxychloroquine have been approved by the United States Food and Drug Administration. The use of an antiviral drug called Favilavir as a treatment for coronavirus has been approved by the National Medical Products Administration of China. The drug has shown efficacy in treating the disease, with very low side effects in a clinical trial involving 70 patients. The clinical trial has been ongoing in Shenzhen, Guangdong province [ 8 ]. This review article reported the recent observations regarding the development of the immunity level in the human body for resisting the coronavirus as an alternative solution before the invention of drugs and vaccinations.
Process of the immune system in the human body
The body contains the organs of the immune system ( Fig. 1 ), which protects against diseases [ 9 , 10 ]. It plays a key role to maintain health and pathogenesis. It also protects the body from harmful substances, germs, and cell changes (neoplasm) [ 11 ]. The key player in the immune system is the white blood cells, which can travel throughout the body through the blood vessels. To monitor for invading microbes, the body exchanges cells and fluids between blood and lymphatic vessels and enables the lymphatic system. The lymphatic vessels carry lymph. Each lymph node contains specialized compartments where they can encounter antigens. Through the incoming lymphatic vessels, the immune cells and foreign particles enter the lymph nodes. When they are in the bloodstream, they are transported to tissues throughout the body. They continue the cycle all over by patrolling for foreign antigens everywhere and then gradually drift back into the lymphatic system. The immune cells gather, work, and serve to confront antigens in lymph nodes and the spleen’s compartments [ 12 ].

The organs of the immune system are positioned throughout the body [ 12 ].
Impacts of Covid-19 on the human body
COVID-19 is an RNA virus with a crown-like appearance. Its diameter is approximately 60–140 nm. On one side, it has a concave surface with a ridge. It makes a larger binding interface, as well as more contacts with ACE2. It can make better contact with the N-terminal helix of ACE2 and have higher affinity [ 13 ]. It is transmitted through respiratory droplets from coughing and sneezing and enters the nasal system by inhaling and starts replicating. ACE2 is the main receptor for the COVID-19 virus [ 14 ]. The spike protein (S protein) present on the surface of COVID-19 is pinched inside the host cell binding to the ACE2 receptor. Here, the enzyme furin is present in the host cell and plays a vital role for the virus to enter, which was absent in SARS-CoV [ 15 ]. Next, the virus starts to propagate with limited innate immune response and can be detected by nasal swabs. The virus then propagates and reaches the respiratory tract, where it faces a more robust innate immune response. At this stage, the disease is clinically manifest and an innate response cytokine may be predictive of the subsequent clinical course [ 16 ]. For beta and lambda infections, viral-infected epithelial cells are a major source [ 17 ]. The disease will be mild for 80% of the infected patients and mostly restricted to the upper and conducting airways [ 18 ]. With conservative symptomatic therapy, these individuals may be monitored and monitored at home. Approximately 20% of the infected patients develop pulmonary infiltrates and some of these develop very severe disease [ 19 ]. The mortality rate of severe patients with COVID-19 can be as high as 49%, based on a recent epidemiological by China CDC [ 20 ]. In Wuhan, 292 patients with COVID-19 were studied. Age was the risk factor of patients with a severe condition, as shown by the Lasso algorithm. When the age of patients with a severe condition increased by 5 years, the risk increased by 15.15%. Most of the patients with COVD-19 were elderly patients in the severe group, with basic diseases. Chronic obstructive pulmonary disease, hypertension, malignant tumor, coronary heart disease, and chronic kidney disease were more frequent in the severe group than in the mild group. Of 145 severe cases, 51 patients died (34.69%), and 90.2% of the patients who dies were over 60 years old. Forty patients had basic disease out of 51 deaths (78.43%). Reports have demonstrated that patients aged older than 60 years who have comorbidities, especially hypertension, are at risk for severe disease and death from SARS-CoV-2 infection [ [21] , [22] , [23] ].
Mechanism of immune systems in the human body against COVID-19
Because there is no registered medicine or vaccine against COVID-19, the immune system is the best defense because it supports the body’s natural ability to defend against pathogens (eg, viruses, bacteria, fungi, protozoan, and worms [ 24 , 25 ]) and resists infections. As long as the immune system is functioning normally, infections such as COVID-19 go unnoticed. The three types of immunity are innate immunity (rapid response), adaptive immunity (slow response), and passive immunity ( Fig. 2 ). Passive immunity has two types: natural immunity, received from the maternal side, and artificial immunity, received from medicine. Skin and inflammatory responses begin when the body is affected [ 26 , 27 ]. However, when the body encounters germs or viruses for the first time, the immune system cannot work properly, and illness can occur. This scenario is what has occurred in the case of COVID-19 [ 28 ].

Innate and adaptive immune system [ 28 ].
When the cells of the immune system become educated, they complete their jobs by recirculating between central and peripheral lymphoid organs and migrating it and from sites of injury via blood ( Fig. 3 ). Blood carries naïve and educated immune cells from one site to another, as it flows throughout the body, and acts as a pipeline for the immune system. The cells again enter into the bloodstream to be transported to tissues throughout the body after exiting these nodes through outgoing lymphatic vessels [ 29 ].

Blood in the pipeline of the immune system [ 29 ].
Many molecular and cellular profiling assays are now available for the study of the human immune system ( Fig. 4 ). The level of advancement of instruments has increased (e.g., polychromatic flow cytometers have improved). In the fields of genomics and proteomics, major technological breakthroughs have also occurred, creating a unique facility for the study of human beings in health and disease where inherent heterogeneity dictates that large collections of samples be analyzed [ 29 ].

Immune profiling armamentarium [ 29 ].
After being affected by virus immune responses to mediate antibody. The B cells are assisted by T cells to differentiate into plasma cells, which then produce antibodies specific to a viral antigen. A neutralizing nature antibody is efficient in fully blocking the virus from entering into host cells to limit the infection and plays a very intense protective role at the later stage of infection and prevents relapse of infection. By contrast, a cellular immunity response can be observed inside the infected cells, which is mediated by T-lymphocytes. The overall adaptive immune response is directed by helper T cells, and cytotoxic T cells play a vital role in the clearance and cleaning of viral-infected cells [ 30 ].
Information from SARS-CoV and MERS-CoV may allow the exploration of knowledge to understand how SARS-CoV-2 escapes the host’s immune response, because data on SARS-CoV-2 remain limited. Notably, 80% of the RNA sequence of SARS-CoV and 50% of the RNA sequence of MERS-CoV match the RNA of SARS-CoV-2 [ 31 ], and SARS-CoV-2 exhibits additional genomic regions. Compared with SARS-CoV and other closely related coronaviruses, its S protein is 20–30 amino acids longer. Thus, SARS-CoV-2 has similar immune evasion strategies, but an additional mechanism remains undiscovered [ 32 , 33 ].
The synopsis of Shi et al. [ 34 ] is based on clinical common sense. They proposed some normal approaches for the treatment of patients with COVID-19 ( Fig. 5 ). They posited that the two-phase immune defense-based protective phase and inflammation-driven damaging phase division are essential. In the first phase, doctors should attempt to boost immune response, and in the second phase, they should attempt to suppress it. Vitamin B3 should be used immediately after the coughing begins because it is highly lung protective. When breathing difficulty starts, hyaluronidase can be given intratracheally and simultaneously, 4-MU can be used to inhibit HAS2. Clearly, susceptibility information will be provided by HLA typing for strategizing prevention, treatment, vaccination, and clinical approaches.

Progression of COVID-19 infection and potential adjuvant interventions [ 34 ].
Reasons for failure
The leading cause for mortality of patients with COVID-19 is respiratory failure from acute respiratory distress syndrome [ 35 ]. Secondary hemophagocytic lymphohistiocytosis (sHLH) is characterized by fulminant and fatal hypercytokinemia with multiorgan failure, and it is underrecognized. Viral infection triggers sHLH and occurs in 3.7%–4.3% of sepsis cases in adults [ 36 , 37 ]. sHLH, resembled by a cytokine profile, is associated with COVID-19 disease severity, characterized by increased interleukin (IL)-2, IL-7, interferon-inducible protein 10, granulocyte-colony stimulating factor, macrophage inflammatory protein 1-, monocyte chemoattractant protein 1, and tumor necrosis factor- (TNF-) [ 38 ]. A recent retrospective fatality predictor’s multicenter study of 150 confirmed COVID-19 cases in Wuhan, China, included elevated ferritin and IL-6, suggesting that mortality might due to virally driven hyperinflammation [ 39 ].
Treatment for patients with COVID-19
Research is ongoing worldwide to develop a vaccine against COVID-19. According to a report [ 40 ], 115 vaccine candidates are being developed. Among them, 78 are confirmed as active and 37 are unconfirmed; 73 are in exploratory out of 78 confirmed active projects. The most advanced candidates have been moved into clinical development. Table 1 shows the clinical phase vaccine candidates for COVID-19.
Clinical phase vaccine candidates for COVID-19 [ 40 ].
According to another report [ 41 ], 108 adults have received a low, middle, or high dose of the vaccine, given as an intramuscular injection. All those adults were not affected by SARS-CoV-2, and their age was in between 18 and 90 years. Their mean age was 36.3, and 51% of them were male and observed for 28 days. Live virus or pseudovirus neutralization assays can detect neutralizing antibodies, in addition to binding antibodies measured by ELISA at approximately 14 days.
At 28 days, dose-dependent antibody responses peaked, with seroconversion documented in 50%–75% of the participants in the middle- and high-dose groups. Moreover, specific T cell responses toward the spike glycoprotein were shown by interferon enzyme-linked immunospot and flow-cytometry. Among 83%–97% of participants, dose-dependent responses were detectable starting from 14 days. The most common adverse effects were fever, fatigue, headache, and muscle pain. One study demonstrated that [ 42 ] for the treatment of COVID-19, convalescent plasma therapy is effective. The survival rate of patients with SARS of viral etiology has been improved with this treatment [ 43 ]. Pre-donation assessment is performed to ensure compliance with the current regulations for plasma donors [ 44 ]. Individuals aged between 18 and 65 years who have recovered and not been infected by COVID-19 for the last 14 days are the convalescent donors. Individuals from the tropical disease areas were also excluded. Plasma, approximately 400–800 mL, was collected from each donor, stored in units of 200 or 250 mL, and frozen within 24 h of collection to be used for further transfusions [ 45 ]. The safety of using convalescent plasma is another issue. Any adverse event did not associate during the epidemic of influenza, SARS-CoV, and MARS-CoV but did occur for Ebola. Reports say treatment with convalescent plasma for patients with COVID-19 is safe without any major adverse events [ 46 ]. Table 2 shows the associated adverse events to convalescent plasma in different epidemics.
Associated adverse events to convalescent plasma in different epidemics.
Because there is no definite and specific treatment for patients with COVID-19, some antiviral agents are prescribed to the patients, depending on their condition and location. Among the antiviral agents, remdesivir is the most well-known potential drug for the treatment of patients with COVID-19. For the treatment of Ebola virus infection in 2017, Gilead Sciences synthesized and developed remdesivir, and it is a phosphoramidate prodrug of an adenosine C-nucleoside and a broad spectrum antiviral agent [ 65 ].
Hydroxychloroquine and chloroquine are other drugs that have a long history of clinical use and similar chemical structures and are often used for the treatment of malaria erythematosus and rheumatoid arthritis [ 66 ]. Lopinavir is another drug, which was administered and marketed in combination with ritonavir by Abbott under the brand name Kaletra in 2000. Lopinavir is a protease inhibitor with high specificity with HIV-1 protease [ 67 ]. Another drug, umifenovir, was first developed in Russia and used in Russia and China for the treatment of prophylaxis, infections associated with influenza A and B, and other arbovirus [ 68 ]. Favipiravir was developed by Fujifilm Toyama Chemical, Japan, in 2014, for treating avian influenza resistant to neuraminidase inhibitors [ 69 ]. Oseltamivir is used to treat influenza A and B. This drug targets the neuraminidase distributed on the surface of the influenza virus to inhibit the spread of the influenza virus in the human body [ [70] , [71] , [72] ]. Table 3 presents the off-label drugs against SARS-CoV-2 and COVID-19.
Off-label drugs against SARS-CoV-2 and COVID-19 disease [ 92 ].
Recent observations of COVID-19 treatment improving the immune system: case study
Researchers are attempting to improve the immune system against COVID-19 and here some of the data reviewed. Ten proteins are encoded by the COVID-19 genome; one of them is the S protein, as aforementioned, because a glycoprotein exists in the virus-infected region ( Fig. 6 ). The S protein is a significant therapeutic target, ensured its location, and targetable using antibodies [ 93 ]. The formation of neutralizing antibodies’ immunization of animals with S protein-oriented vaccines is very effective in preventing infection by homologous coronavirus [ 94 ]. If human cells are infected by virus entities, epitopes from any of that viruses’ proteins can theoretically be bound and presented by MHC-1 receptors on host cell surfaces, leading to the stimulation of CD4 and CD8 T cells to provoke antibody-mediated and cell-mediated immune responses.

Adaptive immune response against coronavirus requires stimulation of B cell and T cell epitopes [ 95 ].
Case study-1
At the University of Copenhagen, researchers net MHC made in-silicon predictions of epitopes presented by 11 MHC-1 alleles that covered approx. 90% of the Asian population. Using that approach, they compiled a list of 100 candidate epitopes for the following MHC alleles—A*0101, A*0201, A*0301, A*11:01, A*2402, B*40:01, C*0401, C*0701, C*0702, and DRB1*0401—resulting in 1100 MHC/peptide binding studies. For the project, they partnered with Intavis and Intavis, who synthesized the COVID-19 epitopes assessed in the study. Using their unique neoscreen technology, they performed in vitro binding studies of the epitopes. The study identified 159 epitopes that stably bind the MHC-1 allele and 22 that bind the tested MHC-II alleles [ 95 ]. The relevant data are listed in Table 4 , Table 5 .
Reference peptides.
Number of epitopes with a minimum 60% stability [ 95 ].
Case study-2 [ 105 ]
As of March 13, 2020, outside China, there were 32 countries with more than 100 COVID-19 cases [ 104 ]. The highest number of infections was found in seven countries: the United States (n = 2294), France (n = 3671), Germany (n = 3675), Spain (n = 5232), South Korea (n = 8086), Iran (n = 11,364), and Italy (n = 17,660). The number of confirmed cases in the other 25 countries was fewer than 1200 [ 105 ]. The related data is presented in Table 3 .
The change of R 0 and R t is connected to the proportion of individuals who have immunity in their body to that pathogen in that population. The alternative method of estimating R t for a pathogen including the population is by multiplying R 0 through the proportion of the population of individuals considered non-immune to that pathogen. In this perception, R 0 will only have a similar level of R t if there are no immune persons in the population. It indicates that any partial pre-existing immunity to the infecting elements is able to decrease the number of expected secondary cases emerging.
Whenever this perception is applied in case of herd immunity to control the COVID-19 epidemic, the fatality rate of the coronavirus is between 0.25% and 3.0% of the estimated population (ie, the measured number of people who may die from affecting this virus), but when the population attains the P crit herd immunity level, it can be difficult to accept ( Table 6 ).
Estimates of SARS-CoV-2 effective reproduction number (Rt) of 32 study countries (as of March 13, 2020), and the minimum proportion (P crit , as % of population) necessary to have recovered from COVID-19 with subsequent immunity, to halt the epidemic in that population. [ 105 ].
Case study-3
Dong et al. [ 106 ] experimented on patients by using various methods. Initially, they used sera, but no significant result was observed. Subsequently, the team focused on NP and S-RBD. To determine optical dilutions, the serum from a patient and human AB serum was titrated. For IgM a dilution of 1:50 and for IgG a dilution of 1:150 were used. Compared with healthy donor groups, NP- and S-RBD-specific IgM and IgG antibodies were both detected in the area of newly discharged patients ( Fig. 7 ). Compared with those of healthy donors, Anti-SARS-CoV-2 IgG antibodies were also more clearly observed than IgM in the followed-up patients. These findings indicate that patients with COVID-19 mounted IgG and IgM responses to SARS-CoV-2 proteins, especially NP and S-RBD, and suggest that patients who are infected could maintain their IgG levels, at least for 2 weeks. Because the RBD domain of the S protein has been shown to bind to the human receptor ACE2, the existence of antibodies against it may suggest the neutralization of SARS-CoV-2 infection. To assess that phenomenon, they performed a pseudovirus particle-based neutralization assay. Patients #1, 2, 4, and 5, all within the discharged group, had high levels of neutralizing antibody titers. Those findings demonstrate that most recently discharged patients had protective humoral immunity to SARS-CoV-2. Except for patient #11, the followed-up patients had lower levels of neutralizing antibody titers than the recently discharged other patients, and all were positive except for patient #7, who was negative.

Detection of antibody responses to recombinant SARS-CoV-2 proteins in patients with COVID-19. (A) Serological responses of 12 patients with COVID-19 to recombinant NP (top) and S-RBD (bottom). The experiment was performed in duplicate. (B) Measurement of neutralizing antibody titers by pseudovirus-based assay. The experiment was performed in triplicate. NP, nucleocapsid protein. S-RBD receptor binding domain of spike protein. HD, healthy donor. Pt, patient. HD#1, serum was collected in 2018. HD#2–4, the sera were from close contact. *P < **P [ 106 ].
Potential challenges in immune system development
An effective immune system must have the ability to interpret changes in the world around it and respond properly; however, it must overcome challenges to work in different environments with different pathogens ( Fig. 8 ). Most of the time, the immune system encounters something new and considers it harmless, but in some cases, that response can be dangerous. An efficient immune system must have the ability to distinguish this. It should have the ability to adapt to strange environmental changes to fight against infections. A healthy immune system has symbiotic microbial farms and reacts to a harmful infection. When pathogens enter the body, they attempt to use it as a host, and the immune system poses many threats. A different door is used for every infection to enter a cell, and blocking these routes of entry can stop an infection before it begins. The immune system neutralizes an infection by producing antibodies; however, this must be conducted at the proper time. An immune system—ideally—must stop an infection before it has established a foothold in the body [ [107] , [108] , [109] , [110] , [111] ].

The adaptive immune response to infection [ 111 ].
Suggested food, vaccination, drugs, and supplementary for the immune system for COVID-19
According to the World Health Organization, healthy foods and hydration are vital. Individuals consuming a well-balanced diet are healthier with a strong immune system and have a reduced risk of chronic illness, infectious diseases. Vitamins and minerals are vital. Vitamin B, insoluble in water, protects from infection. Vitamin C protects from flu-like symptoms [ 112 ]. Insufficient vitamin D and vitamin E can lead to coronavirus infection [ 113 ]. Vitamin D can be found in sunlight, and vitamin E can be found in, for example, oil, seeds, and fruits. Insufficient iron and excess iron can lead to risk [ 114 , 115 ]. Zinc is necessary for maintaining the immune system [ 116 ]. Food rich in protein should be the top priority because it has immune properties (immunoglobulin production) and potential antiviral activity [ [117] , [118] , [119] ]. Therefore, in a regular meal, individuals should eat fruit, vegetables, legumes, nuts, whole grains, and foods from animal sources ( Fig. 9 ). Food from plants containing vitamin A should be consumed, and 8–10 cups of water should be drunk daily. Malnutrition is dangerous for patients with COVID-19 and thus proper nutrition should be provided [ 120 , 121 ]. Fruit juice, tea, and coffee can also be consumed. Too much caffeine, sweetened fruit juices, fruit juice concentrates, syrups, fizzy drinks, and still drinks must be avoided. Unsaturated fats, white meats, and fish should be consumed. Saturated fat, red meat, more than 5 g salt per day, and industry processed food should be avoided [ 122 ]. Along with diet, physical activity is another factor. Individuals should be active and perform physical exercise regularly to boost the immune system and should have proper sleep [123]. Although there is no registered medicine for COVID-19 treatment, hydroxychloroquine and remdesivir are prescribed and are partially effective [124].<-- -->

Nutrition advice for adults during the COVID-19 outbreak [ 122 ].
Conclusions
This review on boosting the immune system is a potential resource for the treatment of patients with COVID-19. The process and mechanism of the immune system can be a good source of knowledge for immune system development. Further research could focus on the most recent observations regarding COVID-19 treatment. If the potential challenges can be overcome, this would be a substantial achievement. Finally, nutrition (eg, dietary recommendations) to boost the immune system should be explored and recommended because no registered medicine is available for COVID-19 treatment.
No funding sources.
Competing interests
The authors declared that there is no conflict of interest.
Ethical approval
Not required.
Thank you for visiting nature.com. You are using a browser version with limited support for CSS. To obtain the best experience, we recommend you use a more up to date browser (or turn off compatibility mode in Internet Explorer). In the meantime, to ensure continued support, we are displaying the site without styles and JavaScript.
- View all journals
- My Account Login
- Explore content
- About the journal
- Publish with us
- Sign up for alerts
- Review Article
- Open access
- Published: 09 March 2020
A systematic review of the applications of artificial intelligence and machine learning in autoimmune diseases
- I. S. Stafford ORCID: orcid.org/0000-0003-1666-1906 1 , 2 ,
- M. Kellermann ORCID: orcid.org/0000-0002-2304-5856 1 ,
- E. Mossotto 1 , 2 ,
- R. M. Beattie 3 ,
- B. D. MacArthur ORCID: orcid.org/0000-0002-5396-9750 2 &
- S. Ennis ORCID: orcid.org/0000-0003-2648-0869 1
npj Digital Medicine volume 3 , Article number: 30 ( 2020 ) Cite this article
28k Accesses
133 Citations
25 Altmetric
Metrics details
- Autoimmune diseases
- Machine learning
- Predictive medicine
Autoimmune diseases are chronic, multifactorial conditions. Through machine learning (ML), a branch of the wider field of artificial intelligence, it is possible to extract patterns within patient data, and exploit these patterns to predict patient outcomes for improved clinical management. Here, we surveyed the use of ML methods to address clinical problems in autoimmune disease. A systematic review was conducted using MEDLINE, embase and computers and applied sciences complete databases. Relevant papers included “machine learning” or “artificial intelligence” and the autoimmune diseases search term(s) in their title, abstract or key words. Exclusion criteria: studies not written in English, no real human patient data included, publication prior to 2001, studies that were not peer reviewed, non-autoimmune disease comorbidity research and review papers. 169 (of 702) studies met the criteria for inclusion. Support vector machines and random forests were the most popular ML methods used. ML models using data on multiple sclerosis, rheumatoid arthritis and inflammatory bowel disease were most common. A small proportion of studies (7.7% or 13/169) combined different data types in the modelling process. Cross-validation, combined with a separate testing set for more robust model evaluation occurred in 8.3% of papers (14/169). The field may benefit from adopting a best practice of validation, cross-validation and independent testing of ML models. Many models achieved good predictive results in simple scenarios (e.g. classification of cases and controls). Progression to more complex predictive models may be achievable in future through integration of multiple data types.
Similar content being viewed by others
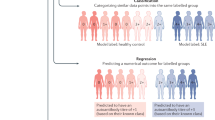
An introduction to machine learning and analysis of its use in rheumatic diseases
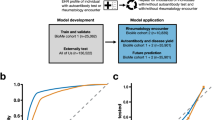
A machine learning model identifies patients in need of autoimmune disease testing using electronic health records
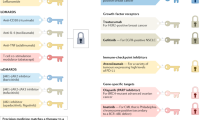
Precision medicine: the precision gap in rheumatic disease
Introduction, autoimmune disease.
Three elements contribute to autoimmune disease development: genetic predisposition, environmental factors and immune system dysregulation (Fig. 1 ). Due to the heterogeneity of onset and progression, diagnosis and prognosis for autoimmune disease is unpredictable.
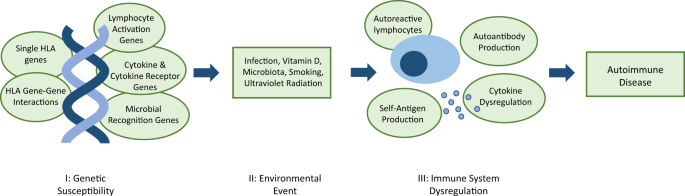
I genetic susceptibility is conferred by a combination of genes that may include genes encoding human leukocyte antigen (HLA) innate and adaptive immune proteins, and directly or indirectly affect the regulation of the immune system. II examples of potential environmental triggers for dysregulation. III autoantibody production alone will not always result in disease development; self-antigen production and subsequent elevated immune response is necessary. 3
A predisposition to autoimmunity is strongly linked to genetics, and caused by defects in mechanisms that result in loss of self-tolerance. 1 Autoimmune disease develops after further immune system dysregulation, in both the innate and adaptive immune system. 2 Microbial antigens, foreign antigens and cytokine dysregulation, can cause induction of self-reactive lymphocytes. 3 Moreover, hyper-activation of T and B cells may occur, along with a change in the duration and quality of their response, which further disrupts the homeostasis of the immune system. 2
The prevalence of autoimmune disease is difficult to estimate; diseases are variably represented across different studies and no definitive list exists. 4 , 5 , 6 There is a reported prevalence rate of between 4.5% 5 and 9.4%, 4 across all autoimmune diseases.
The importance of personalised medicine
Personalised care is valuable for autoimmune disease, with variability within the disorders, 7 and presence of autoimmune comorbidities for 15–29% of patients. 8 , 9 , 10 , 11 Arguably, patients with multiple autoimmune comorbidities would particularly benefit from personalised healthcare for the causal molecular mechanism as opposed to specialist treatment of symptoms.
The data revolution
Standard patient care generates diverse clinical data types. Examples of such data include laboratory test results from blood or urinary samples, symptoms at diagnosis and images obtained using colonoscopies and magnetic resonance imaging (MRI). The majority of these data are reproduced longitudinally over a chronic disease course.
In addition to this wealth of clinical data, ‘omic data—such as patients’ genomic, transcriptomic and proteomic profiles—are now increasingly available. ‘Omic data are large, as molecular measurements are made on a genome-wide scale, 12 and high throughput omics technologies have allowed fast analysis of these data. The inclusion of multiple types of ‘omic data into machine learning models may give a more complete picture of autoimmune disease, leading to novel insights.
The need for artificial intelligence and machine learning
Combined clinical and ‘omic data have limited utility without methods for interpretation. Artificial intelligence and machine learning techniques have the capacity to identify clinically relevant patterns amongst an abundance of information, 13 fulfilling an unmet need. The ability to stratify patient’s using these data has implications for their care, from estimation of autoimmune disease risk, diagnosis, initial and ongoing management, monitoring, treatment response and outcome.
Defining artificial intelligence and machine learning
The terms “machine learning” (ML) and “artificial intelligence” (AI) are often conflated. Artificial intelligence is the study of methods to imitate intelligent human behaviour (for example to make decisions under conditions of uncertainty). Machine learning is a subset of AI that focuses on the study of algorithms that enable a computer to perform specific tasks (typically classification or regression) without specific instructions, but instead inferring patterns from data. 14 Both AI and ML differ from traditional statistical methods as they focus on prediction and classification from high-dimensional data, rather than inference. Successful ML requires robust data from which it can learn. These data must be sufficiently abundant to enable the model to be robust and generalisable to unseen data.
Supervised and unsupervised machine learning
Two types of ML are discussed here: supervised and unsupervised learning. During supervised learning, an algorithm is trained on a “training dataset” to recognise the patterns that are associated with specific “labels” (for example, healthy or diseased). Once predictive patterns have been learned from training data, the ML algorithm is then able to assign labels to unseen “test data”. In a well-trained model, the patterns identified in the training data will generalise to the test data. Brief descriptions of some of the most common supervised ML techniques referred to in this review are summarised in Box 1 .
For unsupervised learning, training data are unlabelled, and the algorithm instead attempts to find and represent patterns within the data, for example by identifying clusters based upon the similarity of the examples. Other types of ML exist, but are reviewed elsewhere. 15 Some of the more common unsupervised methods discussed in this review include hierarchical clustering and self-organising maps.
Box 1 Popular supervised machine learning methods
Neural networks: outputs are learned from inputs via a series of nested nonlinear functions, encoded in a network of “neurons”, which may vary in its topology. 59
Decision trees: outputs are learned from inputs via a series of yes/no questions that successively divide the predictor space into discrete piece. 175
Random forest: a simple ensemble method that grows a large number of decision trees, each of which see only a subset of the data, and learns output from input by combining the predictions. 79
k nearest neighbours: learns output from input by comparing the identity of each data point to its (k) nearest neighbours. 117
Support vector machine: a binary classification method that can be adapted to multiclass classification or regression. They seek to partition the predictor space into two, such that data points from each class are concentrated on one side of the decision boundary. 118
Natural language processing: a set of advanced ML methods that seek to extract sentiment from text. 19
The pros and cons of alternative machine learning models
Recommendations cannot be made on the best model to use in general, as this is always situation specific and dependent on data type, size and dimensionality. Decision trees are simple and highly interpretable, but they rarely achieve performance accuracies higher than other algorithms. Using the random forest method can improve performance, at the cost of losing some interpretability. K nearest neighbours is a non-parametric method, and copes well when complex boundaries separate classes, but this flexibility can lead to poor classification results due to overfitting. 16 Neural networks and support vector machines have similar strengths and weakness: they achieve high accuracies, and can extract linear combinations of features, but interpretability is poor, scaling to very large data can be difficult, and they are not robust to outliers. 17
Technical aspects regarding the operation and fitting of machine learning algorithms are outside the scope of this systematic review, but comprehensively discussed elsewhere. 16 , 17
Avoiding overfitting
Machine learning models are often complicated, and can involve optimizing many free parameters. For this reason, they are prone to overfitting. Overfitting is the process by which the algorithm learns patterns that are specific to the training data but do not generalise to test data. For example, there may be some random technical error in the training data that is not of clinical relevance, yet is learned by the algorithm. Training any model accurately while avoiding overfitting is a central part of an ML pipeline. If data are abundant and/or the ML model is computationally expensive to train then the standard strategy is to remove a portion of the data for training, optimize the model on the remaining portion, and finally determine the model performance by comparison with the unseen test portion. If data are not abundant, a process known as cross-validation is typically employed (Fig. 2 ). There are many variations of cross-validation but they are all essentially generalisations of the training/test splitting process described above. For example, in k-fold cross validation, the data are randomly split into k subsets, with all but one subset used to train an ML model, and the remaining subset used to test the model. This process leads to the generation of k ML models. Each subset of the data is used only once as a test set, and overall model performance is determined by averaging the performance of the k models (Box 2 describes model evaluation metrics). 18
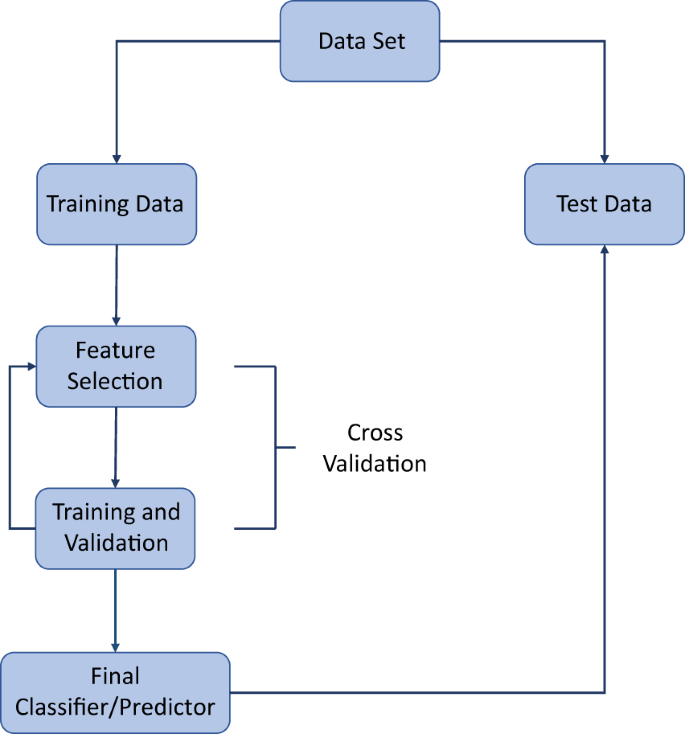
This includes the cycle of feature selection, training and validation that is required to avoid overfitting (cross validation).
Box 2 Metrics for ML method evaluation
Accuracy: percentage of correct predictions. 198
Area under the receiver-operator curve (AUC): appropriate for binary classification problems, this method uses a plot of sensitivity versus specificity to determine model performance. 16
Balanced accuracy: measure of the total number of correct predictions in either class, therefore taking into account an unbalanced dataset. 198
F-score: an accuracy measure calculated using precision and recall. 199
Out-of-bag error: this metric applies to tree-based ensemble methods, and measures the test error by comparing predictions with true labels for samples that were not used in the construction of a particular decision tree. 16
Precision: equivalent to positive predictive value. 16
Recall: another term for sensitivity. 16
R 2 : measures the amount of variation explained by the model regression. 16
Sensitivity: correctly identified true positives. 16
Artificial intelligence, machine learning and autoimmune disease
This systematic review aims to inform on the current status of the application of artificial intelligence and machine learning methods to autoimmune disease to improve patient care. To the best knowledge of the researchers, this is the first study on this topic. The review identifies the most common methods, data and applications, the issues surrounding this exciting interdisciplinary approach, and promising future possibilities.
Summary of results
Of 702 papers identified in database searches, 169 were selected for inclusion in the analysis, 227 duplicates were removed, 273 records were excluded based on the abstract and 33 were excluded after reading the full article (Fig. 3 ) using the criteria described above. A summary and detailed information for qualifying studies are described in Table 1 and Supplementary Table 1 , respectively. Six diseases included in the database search returned no studies that met the inclusion and exclusion criteria (Addison disease, myasthenia gravis, polymyalgia rheumatica, Sjӧgren syndrome, systemic vasculitis and uveitis).
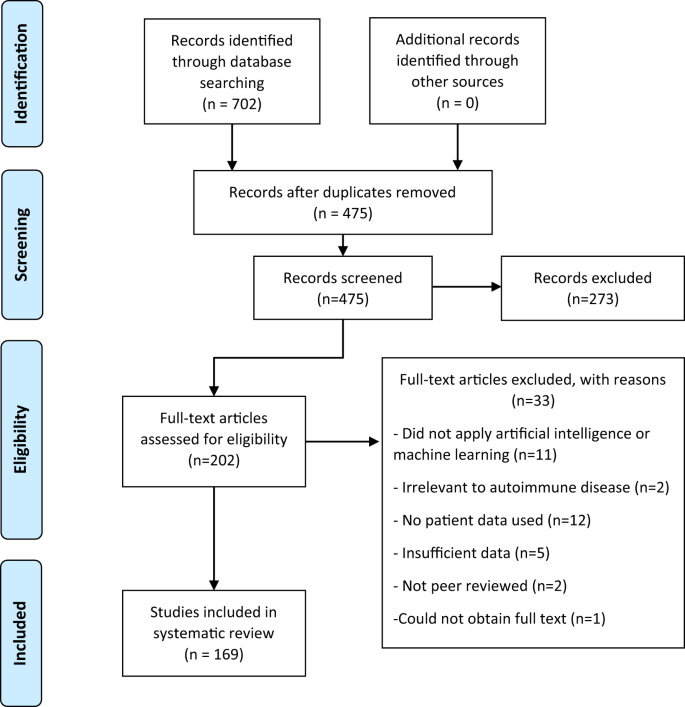
The inclusion and exclusion criteria are applied to the title and abstract at the screening step and to the full article at the eligibility step. During the screening step, it was unclear from some abstracts if the article fulfilled the criteria, and therefore a full read is completed at the eligibility step to clarify the status of those records. Two reviewers completed screening independently, and where consensus could not be reached, a third reviewer assessed these articles and decided whether they were included or excluded.
Machine learning and artificial intelligence are most commonly applied to multiple sclerosis (MS), rheumatoid arthritis (RA) and inflammatory bowel disease (IBD). MS, IBD and RA models used the most types of data, including 13 studies generating models using two data types (always including clinical data). Random forests and support vector machines were the most commonly used methods throughout diseases and applications. Clinical data were used in models for every type of autoimmune disease, and models using genetic data were created for the majority of disorders. The variety in methodological approaches, applications and data, as well as use of validation methods (Supplementary Table 1 ) renders meta-analysis of these methods inappropriate.
The applications for ML can be categorised into six broad topics: patient identification, risk prediction, diagnosis, disease subtype classification, disease progression and outcome and monitoring and management.
Identification of patients
Studies utilised ML methods to identify patients with autoimmune diseases from electronic medical records, 19 , 20 , 21 , 22 , 23 , 24 , 25 and employed natural language processing. Gronsbell et al. worked to improve the efficiency of algorithms for this purpose. 26 , 27 These algorithms are intended to replace International Classification of Diseases billing codes, which have error rates of between 17.1–76.9% due to inconsistent terminology. 19 Electronic medical records also identified comorbidities associated with alopecia and vitiligo using natural language processing. This identified similar autoimmune comorbidities for both diseases. 28 , 29
Identifying and assessing autoimmune disease risk
Prediction of disease risk 30 , 31 , 32 , 33 , 34 , 35 , 36 , 37 , 38 , 39 and identification of novel risk factors through feature selection 40 , 41 , 42 , 43 , 44 was documented for IBD, type 1 diabetes (T1D), RA, systemic lupus erythematosus (SLE) and MS. Fifteen studies employed genetic data, using either sequencing arrays (GWAS) or exome data (nine studies), individual SNPs 38 within in the HLA regions 37 , 45 or pre-selected genes, 41 or gene expression data. 30 , 43 Only one study employed clinical data, 31 and two others combined clinical and genomic data. 30 , 45 Popular models included random forest, support vector machine and logistic regression.
Patient diagnosis was the most frequent ML application, and this approach was used for all autoimmune diseases. Distinguishing cases from healthy controls was an aim for 27 studies. Diagnostic classification models used patients with other autoimmune diseases as controls, 46 , 47 , 48 , 49 differentiated between diseases with overlapping or similar symptoms or phenotype, for example stratifying coeliac disease and irritable bowel syndrome, 50 , 51 , 52 , 53 , 54 , 55 , 56 or examined classification of multiple autoimmune diseases. 57 , 58 ML specifically for early diagnosis was specified by seven studies for the later onset degenerative conditions MS and RA. 48 , 59 , 60 , 61 , 62 , 63 , 64 Other diagnostic applications included distinguishing coeliac disease from an at-risk group 65 , 66 and differentiating those who have complications in T1D. 67 , 68 Random forests and support vector machine most frequently utilised.
Classifying disease subtypes
Disease subtypes in one RA, two IBD, and six MS studies were classified by ML. Three types of unsupervised clustering were used by these studies: hierarchical clustering for identifying novel IBD subtypes; 69 consensus clustering to identify high, low and mixed levels of inflammation in RA; 70 and agglomerative hierarchical clustering to cluster MS by genetic signature. 71 Two of these studies employed support vector machine, 69 , 70 which is a popular supervised method in general, as well as random forest. There was wide variation in data types used. These included clinical (in particular MRI), genetic, RNA sequencing and gene expression data.
Disease progression and outcome
Disease progression and outcome was a focus for 27 studies. Other considered issues were disease severity 72 , 73 , 74 , 75 , 76 , 77 , 78 in psoriasis, RA, IBD and coeliac disease; treatment response 79 , 80 , 81 , 82 , 83 , 84 , 85 , 86 , 87 in IBD, RA and primary biliary cirrhosis (PBC); and survival prediction 88 , 89 , 90 in PBC, RA and SLE. Other models focused on improved image segmentation to aid prognoses 91 , 92 , 93 , 94 , 95 , 96 for IBD and MS. Disease progression and outcome was the second-most prevalent area for model development. Throughout, the most common models were support vector machines, random forest and neural networks. The majority of data used was clinical, with very few papers utilising ‘omic data. 86 , 97 , 98 , 99
Monitoring and management
Ten different studies of type 1 diabetes (T1D) used ML for monitoring and management: four predicted blood glucose level, four identified or predicted hypoglycaemic events, and two supported decision making using case-based reasoning or decision support systems. The majority of models used clinical data. Three models were developed using activity measurements for monitoring movement in MS, and one in RA. Support vector regression was used most frequently. 100 , 101 , 102 , 103 , 104
Validation and independent testing
Eighteen studies only used hold-out validation, not including studies with random forest models, where cross validation is unnecessary, or neural networks, where this process can be too computationally intensive. Eleven studies did not use any validation method, and so model integrity and applicability is unconfirmed. Methods that use hold-out validation have the potential to provide useful information, but it is accepted that unless the dataset is very large, these models are not as robustly validated as those that have used k-fold or leave-one-out cross validation, or a combination of cross-validation and testing on an independent dataset.
Only 14 of 169 studies combined cross-validation with independent test data for evaluating their models. These studies did not have any model types or applications in common. Clinical and genomic data were most common inputs for these studies. Models that used cross-validation and independent test data were applied to a number of the autoimmune diseases.
The research reviewed here demonstrates that, much like the disease studied, the ML models and methods used are heterogeneous. It can be difficult then, to determine which methods should be taken forward to clinical application. Alternatively, models from existing studies could be combined. Models have utilised different types of ‘omic data, including proteomic, metagenomic and exome data. More popular has been sequencing array (SNP/GWAS) data, particularly when predicting autoimmune disease risk. By far the most prevalent type of data is the use of clinical and laboratory data.
To optimise the use of these data types, accessibility is key, and EMRs allow easy extraction of these data. Some researchers have moved beyond only storing medical data in these systems. The eMERGE (electronic medical records and genomics) network combines the genomic and EMR repositories to further genomic medicine research. 105 Other studies such as SPOKE (Scalable Precision Medicine Oriented Knowledge Engine), wish to integrate these data within the storage platform, by building a knowledge network using unsupervised machine learning that informs on how data types such as GWAS, gene ontology, pathways and drug data are connected to EMRs. 106 Improving knowledge of how these data are related is a key step towards implementing precision medicine.
Many models were created for autoimmune disease diagnosis, more specifically classifying those with disease and controls. The majority achieved high classifier performance (where any combination of the following metrics are over these thresholds: accuracy > 81%, AUC > 0.95, Sensitivity > 82, Specificity > 84), and provided evidence of machine learning’s utility in diagnostics.
Identifying the molecular diagnosis to inform tailored treatment strategies has revolutionised cancer prognoses, improving patient outcomes and quality of life, along with economic benefits to the treatment provider. Targeted therapies such as monoclonal antibodies and small molecule inhibitors transformed treatment of some cancers, or improved patient survival times. 107 Key to precision treatment has been the identification of the driver mutations specific to the cancer type. 108 Machine learning has been utilised for cancer classification 109 , 110 and discovery of relevant pathways. 109 Across the spectrum of autoimmune diseases, there has traditionally been a one-size-fits-all approach to patient therapeutics. The expectation is that machine learning represents a necessary key tool that will use ‘big’ data to stratify patients and move towards personalised treatment approaches that have proven so effective in cancer. Proof of this concept has already been demonstrated through machine learning to stratify patient’s inflammation status in RA, 70 and further investigate IBD subtypes. 69
Six models from the evaluated studies returned more than one of the following measures as either 1 or 100%: AUC, accuracy, precision and recall, sensitivity and specificity. 59 , 67 , 68 , 111 , 112 , 113 This perfect performance indicates that a model may not be required, as there exists data that classifies the groups without error. An alternative explanation of apparently optimal performance may reside in poor implementation of cross-validation strategies.
Common metrics reported are accuracy, AUC, and sensitivity and specificity. However, accuracy is inferior to AUC, particularly when imbalanced datasets are used. 114 The AUC measure is unaffected by imbalanced data, but precision-recall curves may reflect model performance more accurately. 115 Dataset rebalancing methods should potentially be utilised more for a thorough review of model performance.
When creating and evaluating a model, increasing focus could be placed on which measure is more important, sensitivity or specificity. Scully et al. demonstrated this, where a lesion segmentation model could achieve high specificity (99.9%) through labelling all tissue as non-lesion. 96
An ML model by Ahmed et al. 62 provides evidence for using an additional independent test dataset subsequent to cross validation. In their study, the AUC dropped by 0.25, indicating decreased model performance on new data.
Studies included in this systematic review have shown that artificial intelligence and machine learning models provide useful insight, despite the heterogeneity of presentation, diagnosis, disease course and patient outcome. However, the heterogeneity in data used, models and model evaluation cause difficulties in obtaining consensus. Furthermore, the number of autoimmune diseases this literature search focussed on was restricted, and may have resulted in an incomplete picture of ML applied to autoimmune diseases.
From this analysis, it seems appropriate to advocate for standardised methods of model evaluation, by utilising a combination of cross validation and independent test data for model validation. Increased confidence in model results allows for more complex model creation, by layering data types or combining classifiers. These models could be applied to more difficult tasks that reflect the complexity of autoimmune disease. With these advances, AI and ML have the potential to bring personalised medicine closer for patients with complex and chronic disease.
Autoimmune disease selection
Autoimmune diseases selected for the systematic review are based on prevalence estimates 4 and include Addison disease, alopecia, Coeliac disease, Crohn’s disease, ulcerative colitis, type 1 diabetes, autoimmune liver diseases, hyper- and hypo-thyroidism, multiple sclerosis, myasthenia gravis, polymyalgia rheumatica, psoriasis, psoriatic arthritis, rheumatoid arthritis, Sjӧgren syndrome, systemic sclerosis, systemic lupus erythematosus, systemic vasculitis, uveitis and vitiligo.
Literature search
The literature search was performed electronically with OvidSP using MEDLINE from 1946, and EMBASE from 1974. A search was also performed on the Computers & Applied Sciences Complete database available on EBSCO. The literature search was completed in December 2018. All searches conformed to the same structure: the words “machine learning” or “artificial intelligence” combined with the chosen search term(s) for each autoimmune disease (see Table 2 ). Boolean operators OR and AND (for combining search terms) were used in order to streamline the procedure. In both databases, the title, abstract and subject terms/keyword headings assigned by authors were searched (last search 17/12/2018).
Inclusion and exclusion criteria
Studies that applied ML methods to autoimmune diseases listed above, or to complications that arise from autoimmune diseases were included. Studies not written in English, published prior to 2001, that did not use real human patient data, were not peer reviewed, or were review papers were also excluded. This systematic review conforms to the Preferred Reporting Items for Systematic Reviews and Meta-Analyses (PRISMA) standards. 116
Reporting summary
Further information on research design is available in the Nature Research Reporting Summary linked to this article.
Data availability
The data (papers) that support the findings of this study are available publicly. Full list of records identified through database searching are available on reasonable request from the authors.
Goodnow, C. C., Sprent, J., de St Groth, B. F. & Vinuesa, C. G. Cellular and genetic mechanisms of self tolerance and autoimmunity. Nature 435 , 590–597 (2005).
Article CAS PubMed Google Scholar
Kuchroo, V. K., Ohashi, P. S., Sartor, R. B. & Vinuesa, C. G. Dysregulation of immune homeostasis in autoimmune diseases. Nat. Med. 18 , 42–47 (2012).
Male, D. K., Roitt, I. M., Roth, D. B., Roitt, I. M. Immunology . 8th edn. (Saunders, 2013).
Cooper, G. S., Bynum, M. L. & Somers, E. C. Recent insights in the epidemiology of autoimmune diseases: improved prevalence estimates and understanding of clustering of diseases. J. Autoimmun. 33 , 197–207 (2009).
Article PubMed PubMed Central Google Scholar
Hayter, S. M. & Cook, M. C. Updated assessment of the prevalence, spectrum and case definition of autoimmune disease. Autoimmun. Rev. 11 , 754–765 (2012).
Article PubMed Google Scholar
Eaton, W. W., Rose, N. R., Kalaydjian, A., Pedersen, M. G. & Mortensen, P. B. Epidemiology of autoimmune diseases in Denmark. J. Autoimmun. 29 , 1–9 (2007).
Cho, J. H. & Feldman, M. Heterogeneity of autoimmune diseases: pathophysiologic insights from genetics and implications for new therapies. Nat. Med. 21 , 730 (2015).
Article CAS PubMed PubMed Central Google Scholar
Simon, T. A. et al. Prevalence of co-existing autoimmune disease in rheumatoid arthritis: a cross-sectional study. Adv. Ther. 34 , 2481–2490 (2017).
Gilhus, N. E., Nacu, A., Andersen, J. B. & Owe, J. F. Myasthenia gravis and risks for comorbidity. Eur. J. Neurol. 22 , 17–23 (2015).
Ruggeri, R. M. et al. Autoimmune comorbidities in Hashimoto’s thyroiditis: different patterns of association in adulthood and childhood/adolescence. Eur. J. Endocrinol. 176 , 133 (2017).
Gill, L. et al. Comorbid autoimmune diseases in patients with vitiligo: a cross-sectional study. J. Am. Acad. Dermatol. 74 , 295–302 (2016).
Teschendorff, A. E. Avoiding common pitfalls in machine learning omic data science. Nat. Mater. 18 , 422–427 (2019).
Jiang, F. et al. Artificial intelligence in healthcare: past, present and future. Stroke Vasc. Neurol. 2 , 230–243 (2017).
Kersting, K. Machine learning and artificial intelligence: two fellow travelers on the quest for intelligent behavior in machines. Front. Big Data. 1 , 6 (2018). https://doi.org/10.3389/fdata.2018.00006 .
Fatima, M. & Pasha, M. Survey of machine learning algorithms for disease diagnostic. J. Intell. Learn. Syst. Appl. 09 , 1–16 (2017).
Google Scholar
James, G., Witten, D., Hastie, T. & Tibshirani, R. An Introduction to Statistical Learning with Applications in R. 1 ed, Vol. XIV , (426. Springer-Verlag, New York, 2013).
Hastie, T., Tibshirani, R. & Friedman, J. H. The elements of statistical learning: data mining, inference, and prediction . 2nd ed. ed. (Springer, New York, 2009).
Figueroa, R. L., Zeng-Treitler, Q., Kandula, S. & Ngo, L. H. Predicting sample size required for classification performance. BMC Med. Inf. Decis. Mak. 12 , 8 (2012).
Article Google Scholar
Turner, C. A. et al. Word2Vec inversion and traditional text classifiers for phenotyping lupus. BMC Med. Inform. Decis. Mak. 17 , 126 (2017).
Zhou, S. M. et al. Defining disease phenotypes in primary care electronic health records by a machine learning approach: a case study in identifying rheumatoid arthritis. PLoS ONE [Electron. Resour.]. 11 , e0154515 (2016).
Article PubMed CAS Google Scholar
Lin, C. et al. Automatic identification of methotrexate-induced liver toxicity in patients with rheumatoid arthritis from the electronic medical record. J. Am. Med. Inf. Assoc. 22 , e151–e161 (2015).
Chen, Y. et al. Applying active learning to high-throughput phenotyping algorithms for electronic health records data. J. Am. Med. Inf. Assoc. 20 , e253–e259 (2013).
Murray, S. G., Avati, A., Schmajuk, G. & Yazdany, J. Automated and flexible identification of complex disease: building a model for systemic lupus erythematosus using noisy labeling. J. Am. Med. Inf. Assoc. 26 , 61–65 (2018).
Chen, W., Huang, Y., Boyle, B. & Lin, S. The utility of including pathology reports in improving the computational identification of patients. J. Pathol. Inform. 7 , 46 (2016).
Ludvigsson, J. F. et al. Use of computerized algorithm to identify individuals in need of testing for celiac disease. J. Am. Med. Inf. Assoc. 20 , e306–e310 (2013).
Gronsbell, J., Minnier, J., Yu, S., Liao, K., Cai, T. Automated feature selection of predictors in electronic medical records data. Biometrics . https://onlinelibrary.wiley.com/doi/abs/10.1111/biom.12987 (2018).
Gronsbell, J. L. & Cai, T. Semi‐supervised approaches to efficient evaluation of model prediction performance. J. R. Stat. Soc. Ser. B (Stat. Methodol.). 80 , 579–594 (2018).
Huang, K. P., Mullangi, S., Guo, Y. & Qureshi, A. A. Autoimmune, atopic, and mental health comorbid conditions associated with alopecia areata in the United States.[Erratum appears in JAMA Dermatol. 2014 Jun;150(6):674]. JAMA Dermatol. 149 , 789–794 (2013).
Sheth, V. M., Guo, Y. & Qureshi, A. A. Comorbidities associated with vitiligo: a ten-year retrospective study. Dermatology 227 , 311–315 (2013).
Corvol, J. C. et al. Abrogation of T cell quiescence characterizes patients at high risk for multiple sclerosis after the initial neurological event. Proc. Natl Acad. Sci. USA 105 , 11839–11844 (2008).
Chin, C. Y., Hsieh, S. Y., Tseng, V. S. EDram: Effective early disease risk assessment with matrix factorization on a large-scale medical database: a case study on rheumatoid arthritis. PLoS ONE 13 , e0207579 (2018).
Liu, C., Ackerman, H. H. & Carulli, J. P. A genome-wide screen of gene-gene interactions for rheumatoid arthritis susceptibility. Hum. Genet. 129 , 473–485 (2011).
Wei, Z. et al. Large sample size, wide variant spectrum, and advanced machine-learning technique boost risk prediction for inflammatory bowel disease. Am. J. Hum. Genet. 92 , 1008–1012 (2013).
Daneshjou, R. et al. Working toward precision medicine: predicting phenotypes from exomes in the Critical Assessment of Genome Interpretation (CAGI) challenges. Hum. Mutat. 38 , 1182–1192 (2017).
Giollo, M. et al. Crohn disease risk prediction-Best practices and pitfalls with exome data. Hum. Mutat. 38 , 1193–1200 (2017).
Pal, L. R., Kundu, K., Yin, Y. & Moult, J. CAGI4 Crohn’s exome challenge: marker SNP versus exome variant models for assigning risk of Crohn disease. Hum. Mutat. 38 , 1225–1234 (2017).
Zhao, L. P., Bolouri, H., Zhao, M., Geraghty, D. E. & Lernmark, A. An object-oriented regression for building disease predictive models with multiallelic HLA genes. Genet. Epidemiol. 40 , 315–332 (2016).
Nguyen, C., Varney, M. D., Harrison, L. C. & Morahan, G. Definition of high-risk type 1 diabetes HLA-DR and HLA-DQ types using only three single nucleotide polymorphisms. Diabetes 62 , 2135–2140 (2013).
Wei, Z. et al. From disease association to risk assessment: an optimistic view from genome-wide association studies on type 1 diabetes. PLoS Genet . 5 (2009).
Negi, S. et al. A genome-wide association study reveals ARL15, a novel non-HLA susceptibility gene for rheumatoid arthritis in North Indians. Arthritis Rheumatism 65 , 3026–3035 (2013).
Briggs, F. B. S. et al. Supervised machine learning and logistic regression identifies novel epistatic risk factors with PTPN22 for rheumatoid arthritis. Genes Immun. 11 , 199–208 (2010).
Gonzalez-Recio, O., de Maturana, E. L., Vega, A. T., Engelman, C. D. & Broman, K. W. Detecting single-nucleotide polymorphism by single-nucleotide polymorphism interactions in rheumatoid arthritis using a two-step approach with machine learning and a Bayesian threshold least absolute shrinkage and selection operator (LASSO) model. BMC Proc. 3 , S63 (2009).
Isakov, O., Dotan, I. & Ben-Shachar, S. Machine learning-based gene prioritization identifies novel candidate risk genes for inflammatory bowel disease. Inflamm. Bowel Dis. 23 , 1516–1523 (2017).
Davis, N. A. et al. Encore: genetic association interaction network centrality pipeline and application to SLE exome data. Genet. Epidemiol. 37 , 614–621 (2013).
Mowry, E. M. et al. Incorporating machine learning approaches to assess putative environmental risk factors for multiple sclerosis. Mult. Scler. Relat. Disord. 24 , 135–141 (2018).
Niu, Q. et al. Specific serum protein biomarkers of rheumatoid arthritis detected by MALDI-TOF-MS combined with magnetic beads. Int. Immunol. 22 , 611–618 (2010).
Geurts, P. et al. Proteomic mass spectra classification using decision tree based ensemble methods. Bioinformatics 21 , 3138–3145 (2005).
De Seny, D. et al. Discovery of new rheumatoid arthritis biomarkers using the surface-enhanced laser desorption/ionization time-of-flight mass spectrometry proteinchip approach. Arthritis Rheumatism 52 , 3801–3812 (2005).
Huang, Z. et al. MALDI-TOF MS combined with magnetic beads for detecting serum protein biomarkers and establishment of boosting decision tree model for diagnosis of systemic lupus erythematosus. Rheumatology 48 , 626–631 (2009).
Alaqtash, M. et al. Automatic classification of pathological gait patterns using ground reaction forces and machine learning algorithms. Conf. Proc. IEEE Eng. Med Biol. Soc. 2011 , 453–457 (2011).
Ohanian, D. et al. Identifying key symptoms differentiating myalgic encephalomyelitis and chronic fatigue syndrome from multiple sclerosis. Neurol. (E-Cronico.). 4 , 41–45 (2016).
Singh, S., Kumar, A., Panneerselvam, K. & Vennila, J. J. Diagnosis of arthritis through fuzzy inference system. J. Med. Syst. 36 , 1459–1468 (2012).
Cowen, E. W. et al. Differentiation of tumour-stage mycosis fungoides, psoriasis vulgaris and normal controls in a pilot study using serum proteomic analysis. Br. J. Dermatol. 157 , 946–953 (2007).
Arasaradnam, R. P. et al. Differentiating coeliac disease from irritable bowel syndrome by urinary volatile organic compound analysis—A pilot study. PLoS ONE . 9 , e107312 (2014).
Berks, M. et al. An automated system for detecting and measuring nailfold capillaries. Medical image computing and computer-assisted intervention: MICCAI International Conference on Medical Image Computing and Computer-Assisted Intervention. 17, 658–665 (2014).
Armananzas, R. et al. Microarray analysis of autoimmune diseases by machine learning procedures. IEEE transactions on information technology in biomedicine: a publication of the IEEE Engineering in Medicine and Biology. Society 13 , 341–350 (2009).
Forbes, J. D. et al. A comparative study of the gut microbiota in immune-mediated inflammatory diseases-does a common dysbiosis exist? Microbiome 6 , 221 (2018).
Iwasawa, K. et al. Dysbiosis of the salivary microbiota in pediatric-onset primary sclerosing cholangitis and its potential as a biomarker. Sci. Rep. 8 , 5480 (2018).
Article PubMed PubMed Central CAS Google Scholar
Heard, B. J. et al. A computational method to differentiate normal individuals, osteoarthritis and rheumatoid arthritis patients using serum biomarkers. J. R. Soc. Interface 11 , 20140428 (2014).
Saccà, V. et al. Evaluation of machine learning algorithms performance for the prediction of early multiple sclerosis from resting-state FMRI connectivity data. Brain Imaging Behav . https://link.springer.com/article/10.1007%2Fs11682-018-9926-9 (2018).
Yoo, Y. et al. Deep learning of joint myelin and T1w MRI features in normal-appearing brain tissue to distinguish between multiple sclerosis patients and healthy controls. NeuroImage: Clin. 17 , 169–178 (2018).
Ahmed, U., Anwar, A., Savage, R. S., Thornalley, P. J. & Rabbani, N. Protein oxidation, nitration and glycation biomarkers for early-stage diagnosis of osteoarthritis of the knee and typing and progression of arthritic disease. Arthritis Res. Ther. 18 , 250 (2016).
Scheel, A. K. et al. Laser imaging techniques for follow-up analysis of joint inflammation in patients with rheumatoid arthritis. Med. Laser Appl. 18 , 198–205 (2003).
Wyns, B. et al. Prediction of arthritis using a modified Kohonen mapping and case based reasoning. Eng. Appl. Artif. Intell. 17 , 205 (2004).
Hujoel, I. A. et al. Machine learning in detection of undiagnosed celiac disease. Clin. Gastroenterol. Hepatol. 16 , 1354–1355.e1351 (2018).
Tenorio, J. M. et al. Artificial intelligence techniques applied to the development of a decision-support system for diagnosing celiac disease. Int. J. Med. Inform. 80 , 793–802 (2011).
Maulucci, G. et al. Phase separation of the plasma membrane in human red blood cells as a potential tool for diagnosis and progression monitoring of type 1 diabetes mellitus. PLoS ONE 12 , (2017).
Cordelli, E. et al. A decision support system for type 1 diabetes mellitus diagnostics based on dual channel analysis of red blood cell membrane fluidity. Comput. Methods Prog. Biomed. 162 , 263–271 (2018).
Mossotto, E. et al. Classification of paediatric inflammatory bowel disease using machine learning. Sci. Rep. 7 , 2427 (2017).
Orange, D. E. et al. Identification of three rheumatoid arthritis disease subtypes by machine learning integration of synovial histologic features and RNA sequencing data. Arthritis Rheumatol. 70 , 690–701 (2018).
Lopez, C., Tucker, S., Salameh, T. & Tucker, C. An unsupervised machine learning method for discovering patient clusters based on genetic signatures. J. Biomed. Inform. 85 , 30–39 (2018).
Lin, C. et al. Automatic prediction of rheumatoid arthritis disease activity from the electronic medical records. PLoS ONE 8 , (2013).
Niehaus, K. E., Uhlig H. H., Clifton D. A. Phenotypic characterisation of Crohn’s disease severity. Conference proceedings:. Annual International Conference of the IEEE Engineering in Medicine and Biology Society. IEEE Engineering in Medicine and Biology Society. Annual Conference. 2015, 7023–7026 (2015).
George, Y., Aldeen, M. & Garnavi, R. Psoriasis image representation using patch-based dictionary learning for erythema severity scoring. Comput. Med. Imaging Graph. 66 , 44–55 (2018).
Shrivastava, V. K., Londhe, N. D., Sonawane, R. S. & Suri, J. S. A novel and robust Bayesian approach for segmentation of psoriasis lesions and its risk stratification. Comput. Methods Prog. Biomed. 150 , 9–22 (2017).
Shrivastava, V. K., Londhe, N. D., Sonawane, R. S. & Suri, J. S. A novel approach to multiclass psoriasis disease risk stratification: Machine learning paradigm. Biomed. Signal Process. Control. 28 , 27–40 (2016).
Raina, A. et al. Objective measurement of erythema in psoriasis using digital color photography with color calibration. Ski. Res. Technol. 22 , 375–380 (2016).
Article CAS Google Scholar
Amirkhani, A., Mosavi, M. R., Mohammadi, K. & Papageorgiou, E. I. A novel hybrid method based on fuzzy cognitive maps and fuzzy clustering algorithms for grading celiac disease. Neural Comput. Appl. 30 , 1573–1588 (2018).
Waljee, A. K. et al. Predicting corticosteroid-free endoscopic remission with vedolizumab in ulcerative colitis. Alimentary Pharmacol. Thera. 47 , 763–772 (2018).
Miyoshi, F. et al. A novel method predicting clinical response using only background clinical data in RA patients before treatment with infliximab. Mod. Rheumatol. 26 , 813–816 (2016).
Nair, S. S., French, R. M., Laroche, D. & Thomas, E. The application of machine learning algorithms to the analysis of electromyographic patterns from arthritic patients. IEEE Trans. Neural Syst. Rehabil. Eng. 18 , 174–184 (2010).
Van Looy, S. et al. Prediction of dose escalation for rheumatoid arthritis patients under infliximab treatment. Eng. Appl. Artif. Intell. 19 , 819–828 (2006).
Waljee, A. K. et al. Machine learning algorithms for objective remission and clinical outcomes with thiopurines. J. Crohn’s Colitis 11 , 801–810 (2017).
Kang, T., Ding, W., Zhang, L., Ziemek, D. & Zarringhalam, K. A biological network-based regularized artificial neural network model for robust phenotype prediction from gene expression data. BMC Bioinforma. 18 , 565 (2017).
Waljee, A. K. et al. Algorithms outperform metabolite tests in predicting response of patients with inflammatory bowel disease to thiopurines. Clin. Gastroenterol. Hepatol. 8 , 143–150 (2010).
Doherty, M. K. et al. Fecal microbiota signatures are associated with response to ustekinumab therapy among Crohn’s disease patients. mBio 9 , e02120-02117 (2018).
Weiss, J., Kuusisto, F., Boyd, K., Liu, J. & Page, D. Machine learning for treatment assignment: improving individualized risk attribution. AMIA Annu. Symp. Proc. AMIA Symp. 2015 , 1306–1315 (2015).
PubMed Google Scholar
Lezcano-Valverde, J. M. et al. Development and validation of a multivariate predictive model for rheumatoid arthritis mortality using a machine learning approach. Sci. Rep. 7 , 10189 (2017).
Tang, H. et al. Predicting three-year kidney graft survival in recipients with systemic lupus erythematosus. ASAIO J. (Am. Soc. Artif. Intern. Organs: 1992) 57 , 300–309 (2011).
Tsujitani, M. & Sakon, M. Analysis of survival data having time-dependent covariates. IEEE Trans. Neural Netw. 20 , 389–394 (2009).
Sweeney, E. M. et al. A comparison of supervised machine learning algorithms and feature vectors for MS lesion segmentation using multimodal structural MRI. PLOS ONE. 9 , e95753 (2014).
Commowick, O. et al. Objective evaluation of multiple sclerosis lesion segmentation using a data management and processing infrastructure. Sci. Rep. 8 , 13650 (2018).
Cabezas, M. et al. BOOST: a supervised approach for multiple sclerosis lesion segmentation. J. Neurosci. Methods 237 , 108–117 (2014).
Mahapatra, D., Vos, F. M. & Buhmann, J. M. Active learning based segmentation of Crohns disease from abdominal MRI. Comput Methods Prog. Biomed. 128 , 75–85 (2016).
Mahapatra, D. Combining multiple expert annotations using semi-supervised learning and graph cuts for medical image segmentation. Computer Vis. Image Underst. 151 , 114–123 (2016).
Scully, M. et al. An Automated Method for Segmenting White Matter Lesions through Multi-Level Morphometric Feature Classification with Application to Lupus. Front Hum. Neurosci. 4 , 27 (2010).
PubMed PubMed Central Google Scholar
Joo, Y. B. et al. Biological function integrated prediction of severe radiographic progression in rheumatoid arthritis: A nested case control study. Arthritis and Rheumatology Conference: American College of Rheumatology/Association of Rheumatology Health Professionals Annual Scientific Meeting, ACR/ARHP. 19, 244 (2017).
Douglas, G. M. et al. Multi-omics differentially classify disease state and treatment outcome in pediatric Crohn’s disease. Microbiome . 6 , 13 (2018).
Patrick, M. T. et al. Genetic signature to provide robust risk assessment of psoriatic arthritis development in psoriasis patients. Nat. Commun . 9 , 4178 (2018). https://doi.org/10.1038/s41467-018-06672-6 .
Supratak, A. et al. Remote monitoring in the home validates clinical gait measures for multiple sclerosis. Front. Neurol . 9 , 561 (2018). https://doi.org/10.3389/fneur.2018.00561 .
McGinnis, R. S. et al. A machine learning approach for gait speed estimation using skin-mounted wearable sensors: From healthy controls to individuals with multiple sclerosis. PLoS ONE 12 , e0178366 (2017).
Georga, E. I., Protopappas, V. C., Ardigo, D., Polyzos, D. & Fotiadis, D. I. A Glucose Model Based on Support Vector Regression for the Prediction of Hypoglycemic Events Under Free-Living Conditions. Diabetes Technol. Ther. 15 , 634–643 (2013).
Marling, C. R., Struble, N. W., Bunescu, R. C., Shubrook, J. H. & Schwartz, F. L. A consensus-perceived glycemic variability metric. J. Diabetes Sci. Technol. 7 , 871–879 (2013).
Georga, E. I., Protopappas, V. C., Polyzos, D. & Fotiadis, D. I. Evaluation of short-term predictors of glucose concentration in type 1 diabetes combining feature ranking with regression models. Med Biol. Eng. Comput. 53 , 1305–1318 (2015).
Gottesman, O. et al. The Electronic Medical Records and Genomics (eMERGE) Network: past, present, and future. Genet. Med. 15 , 761–771 (2013).
Nelson, C. A., Butte, A. J. & Baranzini, S. E. Integrating biomedical research and electronic health records to create knowledge-based biologically meaningful machine-readable embeddings. Nat. Commun. 10 , 3045 (2019).
Gerber, D. E. Targeted therapies: a new generation of cancer treatments. Am. Fam. Physician 77 , 311–319 (2008).
Raphael, B. J., Dobson, J. R., Oesper, L. & Vandin, F. Identifying driver mutations in sequenced cancer genomes: computational approaches to enable precision medicine. Genome Med. 6 , 5 (2014).
Zeng, Z. et al. Cancer classification and pathway discovery using non-negative matrix factorization. J. Biomed. Inform. 96 , 103247 (2019).
Zhang, X., Guan, N., Jia, Z., Qiu, X. & Luo, Z. Semi-supervised projective non-negative matrix factorization for cancer classification. PLoS ONE 10 , e0138814 (2015).
Lotsch, J. et al. Machine-learning based lipid mediator serum concentration patterns allow identification of multiple sclerosis patients with high accuracy. Sci. Rep. 8 , 14884 (2018).
Shrivastava, V. K., Londhe, N. D., Sonawane, R. S. & Suri, J. S. Computer-aided diagnosis of psoriasis skin images with HOS, texture and color features: a first comparative study of its kind. Comput Methods Prog. Biomed. 126 , 98–109 (2016).
Zhu, H. et al. Integration of Genome-Wide DNA Methylation and Transcription Uncovered Aberrant Methylation-Regulated Genes and Pathways in the Peripheral Blood Mononuclear Cells of Systemic Sclerosis. Int. J. Rheumatol . 2018 , https://doi.org/10.1155/2018/7342472 (2018).
Huang, J. & Ling, C. X. Using AUC and accuracy in evaluating learning algorithms. Ieee T Knowl. Data En. 17 , 299–310 (2005).
Jeni, L. A., Cohn, J. F., Torre, FDL, (eds) Facing Imbalanced Data–Recommendations for the Use of Performance Metrics. 2013 Humaine Association Conference on Affective Computing and Intelligent Interaction; 2013 2-5 Sept. 2013.
Moher, D., Liberati, A., Tetzlaff, J. & Altman, D. G. The PG. Preferred Reporting Items for Systematic Reviews and Meta-Analyses: The PRISMA Statement. PLOS Med. 6 , e1000097 (2009).
Zhang, Y. et al. Comparison of machine learning methods for stationary wavelet entropy-based multiple sclerosis detection: decision tree, k-nearest neighbors, and support vector machine. Simulation 92 , 861–871 (2016).
Zhao, Y. et al. Exploration of machine learning techniques in predicting multiple sclerosis disease course. PLOS ONE. 12 , e0174866 (2017).
Briggs, F. B. S. et al. Multiple sclerosis risk factors contribute to onset heterogeneity. Mult. Scler. Relat. Disord. 28 , 11–16 (2019).
Ahmadi, A., Davoudi, S. & Daliri, M. R. Computer aided diagnosis system for multiple sclerosis disease based on phase to amplitude coupling in covert visual attention. Comput Methods Prog. Biomed. 169 , 9–18 (2019).
Zhang, H. et al. Predicting conversion from clinically isolated syndrome to multiple sclerosis–An imaging-based machine learning approach. NeuroImage: Clin. 21 , 101593 (2019).
Zurita, M. et al. Characterization of relapsing-remitting multiple sclerosis patients using support vector machine classifications of functional and diffusion MRI data. NeuroImage: Clin. 20 , 724–730 (2018).
Wang, S.-H. et al. Multiple sclerosis identification by 14-layer convolutional neural network with batch normalization, dropout, and stochastic pooling. Front. Neuroscience . 12 , 818 (2018) https://doi.org/10.3389/fnins.2018.00818 .
Neeb, H. & Schenk, J. Multivariate prediction of multiple sclerosis using robust quantitative MR-based image metrics. Zeitschrift für Medizinische Physik. https://www.sciencedirect.com/science/article/pii/S0939388918300680?via%3Dihub (2018).
Tacchella, A. et al. Collaboration between a human group and artificial intelligence can improve prediction of multiple sclerosis course: a proof-of-principle study. F1000Research. 6 , 2172 (2017).
Kiiski, H. et al. Machine learning EEG to predict cognitive functioning and processing speed over a 2-year Period in multiple sclerosis patients and controls. Brain Topogr. 31 , 346–363 (2018).
Fiorini, S. et al. A machine learning pipeline for multiple sclerosis course detection from clinical scales and patient reported outcomes. Conf. Proc. IEEE Eng. Med Biol. Soc. 2015 , 4443–4446 (2015).
Zhong, J. et al. Combined structural and functional patterns discriminating upper limb motor disability in multiple sclerosis using multivariate approaches. Brain Imaging Behav. 11 , 754–768 (2017).
Lötsch, J. et al. Machine-learned data structures of lipid marker serum concentrations in multiple sclerosis patients differ from those in healthy subjects. Int. J. Mol. Sci. 18 , 1217 (2017).
Article PubMed Central CAS Google Scholar
Karaca, Y., Zhang, Y. D., Cattani, C. & Ayan, U. The differential diagnosis of multiple sclerosis using convex combination of infinite kernels. CNS Neurol. Disord. Drug Targets 16 , 36–43 (2017).
Ostmeyer, J. et al. Statistical classifiers for diagnosing disease from immune repertoires: a case study using multiple sclerosis. BMC Bioinforma. 18 , 401 (2017).
Ion-Mărgineanu, A. et al. Machine learning approach for classifying multiple sclerosis courses by combining clinical data with lesion loads and magnetic resonance metabolic features. Front. Neurosci . 11 , 398 (2017). https://doi.org/10.3389/fnins.2017.00398 .
Kocevar, G. et al. Graph theory-based brain connectivity for automatic classification of multiple sclerosis clinical courses. Front. Neurosci . 10 , 478 (2016). https://doi.org/10.3389/fnins.2016.00478 .
Kosa, P. et al. Development of a sensitive outcome for economical drug screening for progressive multiple sclerosis treatment. Front. Neurol . 7 , 131 (2016). https://doi.org/10.3389/fneur.2016.00131 .
Baranzini, S. E. et al. Prognostic biomarkers of IFNb therapy in multiple sclerosis patients. Mult. Scler. (Houndmills, Basingstoke, Engl.). 21 , 894–904 (2015).
Wottschel, V. et al. Predicting outcome in clinically isolated syndrome using machine learning. Neuroimage Clin. 7 , 281–287 (2015).
Crimi, A. et al. Predictive value of imaging markers at multiple sclerosis disease onset based on gadolinium- and USPIO-enhanced MRI and machine learning. PLoS ONE 9 , e93024 (2014).
Taschler, B. et al. editors. Spatial modeling of multiple sclerosis for disease subtype prediction. (Springer International Publishing, 2014).
Goldstein, B. A., Hubbard, A. E., Cutler, A. & Barcellos, L. F. An application of Random Forests to a genome-wide association dataset: methodological considerations & new findings. BMC Genet. 11 , 49 (2010).
Briggs, F. B. et al. Evidence for CRHR1 in multiple sclerosis using supervised machine learning and meta-analysis in 12,566 individuals. Hum. Mol. Genet. 19 , 4286–4295 (2010).
Salem, M. et al. A supervised framework with intensity subtraction and deformation field features for the detection of new T2-w lesions in multiple sclerosis. NeuroImage: Clin. 17 , 607–615 (2018).
Birenbaum, A. & Greenspan, H. Multi-view longitudinal CNN for multiple sclerosis lesion segmentation. Eng. Appl. Artif. Intell. 65 , 111–118 (2017).
Morrison, C. et al. Assessing multiple sclerosis with kinect: designing computer vision systems for real-world use. Hum.-Computer Interact. 31 , 191–226 (2016).
Liu, J., Brodley, C. E., Healy, B. C. & Chitnis, T. Removing confounding factors via constraint-based clustering: An application to finding homogeneous groups of multiple sclerosis patients. Artif. Intell. Med. 65 , 79–88 (2015).
Chocholova, E. et al. Glycomics meets artificial intelligence - Potential of glycan analysis for identification of seropositive and seronegative rheumatoid arthritis patients revealed. Clin. Chim. Acta 481 , 49–55 (2018).
Wu, H. et al. Metagenomics biomarkers selected for prediction of three different diseases in Chinese population. BioMed Res. Int. (2018) https://www.ncbi.nlm.nih.gov/pmc/articles/PMC5820663/ .
Andreu-Perez, J. et al. Developing fine-grained actigraphies for rheumatoid arthritis patients from a single accelerometer using machine learning. Sensors 17 , 2113 (2017).
Article PubMed Central Google Scholar
Yeo, L. et al. Expression of chemokines CXCL4 and CXCL7 by synovial macrophages defines an early stage of rheumatoid arthritis. Ann. Rheum. Dis. 75 , 763–771 (2016).
Pratt, A. G. et al. A CD4 T cell gene signature for early rheumatoid arthritis implicates interleukin 6-mediated STAT3 signalling, particularly in anti-citrullinated peptide antibody-negative disease. Ann. Rheum. Dis. 71 , 1374–1381 (2012).
Kruppa, J., Ziegler, A. & Konig, I. R. Risk estimation and risk prediction using machine-learning methods. Hum. Genet. 131 , 1639–1654 (2012).
Gossec, L. et al. Detection of flares by decrease in physical activity, collected using wearable activity trackers, in rheumatoid arthritis or axial spondyloarthritis: an application of Machine-Learning analyses in rheumatology. Arthritis Care Res (Hoboken). 22 , 22 (2018).
Waljee, A. K. et al. Predicting hospitalization and outpatient corticosteroid use in inflammatory bowel disease patients using machine learning. Inflamm. Bowel Dis. 24 , 45–53 (2018).
Maeda, Y. et al. Fully automated diagnostic system with artificial intelligence using endocytoscopy to identify the presence of histologic inflammation associated with ulcerative colitis (with video). Gastrointest. Endosc. 89 , 408–415 (2018).
Jain, S. et al. Predictors of long-term outcomes in patients with acute severe colitis: a northern Indian cohort study. J. Gastroenterol. Hepatol. (Aust.). 33 , 615–622 (2018).
Eck, A. et al. Interpretation of microbiota-based diagnostics by explaining individual classifier decisions. BMC Bioinforma. 18 , 441 (2017).
Menti, E. et al. Bayesian Machine Learning Techniques for revealing complex interactions among genetic and clinical factors in association with extra-intestinal Manifestations in IBD patients. AMIA Annu. Symp. Proc. AMIA Symp. 2016 , 884–893 (2016).
CAS PubMed Google Scholar
Hubenthal, M. et al. Sparse modeling reveals miRNA signatures for diagnostics of inflammatory bowel disease. PLoS ONE . 10 , e0140155 (2015). https://doi.org/10.1371/journal.pone.0140155 .
Cui, H. & Zhang, X. Alignment-free supervised classification of metagenomes by recursive SVM. BMC Genomics . 14 , 641 (2013). https://doi.org/10.1186/1471-2164-14-641 .
Firouzi, F. et al. A decision tree-based approach for determining low bone mineral density in inflammatory bowel disease using WEKA software. Eur. J. Gastroenterol. Hepatol. 19 , 1075–1081 (2007).
Ozawa, T. et al. Novel computer-assisted diagnosis system for endoscopic disease activity in patients with ulcerative colitis. Gastrointestinal Endoscopy . (2018). https://www.sciencedirect.com/science/article/pii/S0016510718331936?via%3Dihub .
Reddy, B. K., Delen, D., Agrawal, R. K. Predicting and explaining inflammation in Crohn’s disease patients using predictive analytics methods and electronic medical record data. Health Inform J. 1460458217751015 (2018).
Han, L. et al. A probabilistic pathway score (PROPS) for classification with applications to inflammatory bowel disease. Bioinformatics 34 , 985–993 (2018).
Yu, S. et al. Surrogate-assisted feature extraction for high-throughput phenotyping. J. Am. Med. Inf. Assoc. 24 , e143–e149 (2017).
Wisittipanit, N. et al. Classification methods for the analysis of LH-PCR data associated with inflammatory bowel disease patients. Int J. Bioinform Res. Appl. 11 , 111–129 (2015).
Ahmed, S. et al. Effect of fuzzy partitioning in Crohn’s disease classification: a neuro-fuzzy-based approach. Med. Biol. Eng. Comput. 55 , 101–115 (2017).
Stawiski, K., Pietrzak, I., Mlynarski, W., Fendler, W. & Szadkowska, A. NIRCa: An artificial neural network-based insulin resistance calculator. Pediatr. Diabetes 19 , 231–235 (2018).
Ben Ali, J. et al. Continuous blood glucose level prediction of Type 1 Diabetes based on Artificial Neural Network. Biocybern. Biomed. Eng. 38 , 828–840 (2018).
Siegel, A. P. et al. Analyzing breath samples of hypoglycemic events in type 1 diabetes patients: towards developing an alternative to diabetes alert dogs. J. Breath Res . 11 , 026007 (2017). https://doi.org/10.1088/1752-7163/aa6ac6 .
Georga, E. I., Protopappas, V. C., Polyzos, D., Fotiadis, D. I. Online prediction of glucose concentration in type 1 diabetes using extreme learning machines. Conference proceedings:. Annual International Conference of the IEEE Engineering in Medicine and Biology Society. IEEE Engineering in Medicine and Biology Society. Annual Conference. 2015, 3262–3265 (2015).
Jensen, M. H. et al. Evaluation of an algorithm for retrospective hypoglycemia detection using professional continuous glucose monitoring data. J. Diabetes Sci. Technol. 8 , 117–122 (2014).
Schwartz, F. L., Shubrook, J. H. & Marling, C. R. Use of case-based reasoning to enhance intensive management of patients on insulin pump therapy. J. Diabetes Sci. Technol. 2 , 603–611 (2008).
Sampath, S., Tkachenko, P., Renard, E. & Pereverzev, S. V. Glycemic control indices and their aggregation in the prediction of nocturnal hypoglycemia from intermittent blood glucose measurements. J. Diabetes Sci. Technol. 10 , 1245–1250 (2016).
Ling, S. H., San, P. P. & Nguyen, H. T. Non-invasive hypoglycemia monitoring system using extreme learning machine for Type 1 diabetes. ISA Trans. 64 , 440–446 (2016).
Perez-Gandia, C. et al. Decision support in diabetes care: the challenge of supporting patients in their daily living using a mobile glucose predictor. J. Diabetes Sci. Technol. 12 , 243–250 (2018).
Guy, R. T., Santago, P. & Langefeld, C. D. Bootstrap aggregating of alternating decision trees to detect sets of SNPs that associate with disease. Genet. Epidemiol. 36 , 99–106 (2012).
Ceccarelli, F. et al. Biomarkers of erosive arthritis in systemic lupus erythematosus: application of machine learning models. PLoS ONE 13 , e0207926 (2018). https://doi.org/10.1371/journal.pone.0207926 .
Ceccarelli, F. et al. Prediction of chronic damage in systemic lupus erythematosus by using machine-learning models. PLoS ONE 12 , e0174200 (2017). https://doi.org/10.1371/journal.pone.0174200 .
Kan, H. et al. Longitudinal treatment patterns and associated outcomes in patients with newly diagnosed systemic lupus erythematosus. Clin. Ther. 38 , 610–624 (2016).
Wolf, B. J. et al. Development of biomarker models to predict outcomes in lupus nephritis. Arthritis Rheumatol. 68 , 1955–1963 (2016).
Armañanzas, R. et al. Microarray analysis of autoimmune diseases by machine learning procedures. IEEE Trans. Inf. Technol. Biomedicine. 13 , 341–350 (2009).
Reddy, B. K. & Delen, D. Predicting hospital readmission for lupus patients: an RNN-LSTM-based deep-learning methodology. Comput Biol. Med. 101 , 199–209 (2018).
Tang, Y. et al. Lupus nephritis pathology prediction with clinical indices. Sci. Rep. 8 , 10231 (2018).
Wang, Y. et al. Random Bits Forest: a Strong Classifier/Regressor for Big Data. Sci. Rep. 6 , 30086 (2016).
Shrivastava, V. K., Londhe, N. D., Sonawane, R. S. & Suri, J. S. Exploring the color feature power for psoriasis risk stratification and classification: a data mining paradigm. Comput. Biol. Med. 65 , 54–68 (2015).
Shrivastava, V. K., Londhe, N. D., Sonawane, R. S. & Suri, J. S. Reliable and accurate psoriasis disease classification in dermatology images using comprehensive feature space in machine learning paradigm. Expert Syst. Appl. 42 , 6184–6195 (2015).
Shrivastava, V. K., Londhe, N. D., Sonawane, R. S. & Suri, J. S. Reliability analysis of psoriasis decision support system in principal component analysis framework. Data Knowl. Eng. 106 , 1–17 (2016).
Choung, R. S. et al. Synthetic neoepitopes of the transglutaminase-deamidated gliadin complex as biomarkers for diagnosing and monitoring celiac disease. Gastroenterology 156 , 582–591.e581 (2019).
Ahmad, W., Ahmad, A., Lu, C., Khoso, B. A. & Huang, L. A novel hybrid decision support system for thyroid disease forecasting. Soft Computing - A Fusion of Foundations. Methodologies Appl. 22 , 5377–5383 (2018).
Baccour, L. Amended fused TOPSIS-VIKOR for classification (ATOVIC) applied to some UCI data sets. Expert Syst. Appl. 99 , 115–125 (2018).
Morejón, R., Viana, M. & Lucena, C. An approach to generate software agents for health data mining. Int. J. Softw. Eng. Knowl. Eng. 27 , 1579–1589 (2017).
Temurtas, F. A comparative study on thyroid disease diagnosis using neural networks. Expert Syst. Appl. 36 , 944–949 (2009).
Polat, K., Şahan, S. & Güneş, S. A novel hybrid method based on artificial immune recognition system (AIRS) with fuzzy weighted pre-processing for thyroid disease diagnosis. Expert Syst. Appl. 32 , 1141–1147 (2007).
Keleş, A. & Keleş, A. ESTDD: Expert system for thyroid diseases diagnosis. Expert Syst. Appl. 34 , 242–246 (2008).
Singh, A. & Pandey, B. A KLD-LSSVM based computational method applied for feature ranking and classification of primary biliary cirrhosis stages. Int. J. Comput. Biol. Drug Des. 10 , 24–38 (2017).
Eaton, J. E. et al. Primary sclerosing cholangitis risk estimate tool (PREsTo) predicts outcomes in PSC: a derivation & validation study using machine learning. Hepatology (2018). https://www.ncbi.nlm.nih.gov/pubmed/29742811 .
Taroni, J. N., Martyanov, V., Mahoney, J. M. & Whitfield, M. L. A functional genomic meta-analysis of clinical trials in systemic sclerosis: toward precision medicine and combination therapy. J. Invest. Dermatol. 137 , 1033–1041 (2017).
Huang, H. et al. A methodology for exploring biomarker–phenotype associations: application to flow cytometry data and systemic sclerosis clinical manifestations. BMC Bioinforma. 16 , 293 (2015).
Brodersen, K. H., Ong, C. S., Stephan, K. E., Buhmann, J. M., (eds) The Balanced Accuracy and Its Posterior Distribution. 2010 20th International Conference on Pattern Recognition; 2010 23–26 Aug. 2010.
Goutte, C., Gaussier, E., (eds) A Probabilistic Interpretation of Precision, Recall and F-Score, with Implication for Evaluation . (Springer Berlin Heidelberg, 2005).
Download references
Acknowledgements
This study was supported by the Institute for Life Sciences, University of Southampton, and the National Institute for Health Research (NIHR) Southampton Biomedical Centre. The views expressed are those of the author(s) and not necessarily those of the NIHR or the Department of Health and Social Care.
Author information
Authors and affiliations.
Department of Human Genetics and Genomic Medicine, University of Southampton, Southampton, UK
I. S. Stafford, M. Kellermann, E. Mossotto & S. Ennis
Institute for Life Sciences, University of Southampton, Southampton, UK
I. S. Stafford, E. Mossotto & B. D. MacArthur
Department of Paediatric Gastroenterology, Southampton Children’s Hospital, Southampton, UK
R. M. Beattie
You can also search for this author in PubMed Google Scholar
Contributions
I.S.S., S.E. and E.M. formed the search strategy and inclusion and exclusion criteria. I.S.S. performed database searches and reviewed all records for eligibility (title and abstract, full record if necessary). M.K. reviewed all records (title and abstract). E.M. reviewed records where agreement could not be reached between I.S.S. and M.K. regarding study inclusion. I.S.S. read and evaluated all included studies, with analysis and interpretation assistance from E.M., B.D.M. (M.L. perspective) S.E. and R.M.B. (clinical perspective). I.S.S. wrote the manuscript with input from M.K., E.M., B.D.M., R.M.B. and S.E.
Corresponding author
Correspondence to S. Ennis .
Ethics declarations
Competing interests.
The authors declare no competing interests.
Additional information
Publisher’s note Springer Nature remains neutral with regard to jurisdictional claims in published maps and institutional affiliations.
Supplementary information
Supplementary information, reporting summary, rights and permissions.
Open Access This article is licensed under a Creative Commons Attribution 4.0 International License, which permits use, sharing, adaptation, distribution and reproduction in any medium or format, as long as you give appropriate credit to the original author(s) and the source, provide a link to the Creative Commons license, and indicate if changes were made. The images or other third party material in this article are included in the article’s Creative Commons license, unless indicated otherwise in a credit line to the material. If material is not included in the article’s Creative Commons license and your intended use is not permitted by statutory regulation or exceeds the permitted use, you will need to obtain permission directly from the copyright holder. To view a copy of this license, visit http://creativecommons.org/licenses/by/4.0/ .
Reprints and permissions
About this article
Cite this article.
Stafford, I.S., Kellermann, M., Mossotto, E. et al. A systematic review of the applications of artificial intelligence and machine learning in autoimmune diseases. npj Digit. Med. 3 , 30 (2020). https://doi.org/10.1038/s41746-020-0229-3
Download citation
Received : 12 August 2019
Accepted : 17 January 2020
Published : 09 March 2020
DOI : https://doi.org/10.1038/s41746-020-0229-3
Share this article
Anyone you share the following link with will be able to read this content:
Sorry, a shareable link is not currently available for this article.
Provided by the Springer Nature SharedIt content-sharing initiative
This article is cited by
Machine learning approaches to identify systemic lupus erythematosus in anti-nuclear antibody-positive patients using genomic data and electronic health records.
- Chih-Wei Chung
- Seng-Cho Chou
- Yi-Ming Chen
BioData Mining (2024)
Early identification of macrophage activation syndrome secondary to systemic lupus erythematosus with machine learning
Arthritis Research & Therapy (2024)
Collating the voice of people with autoimmune diseases: Methodology for the Third Phase of the COVAD Studies
- Mahnoor Javaid
- Latika Gupta
Rheumatology International (2024)
Decoding the mitochondrial connection: development and validation of biomarkers for classifying and treating systemic lupus erythematosus through bioinformatics and machine learning
- Haoguang Li
- Xinwang Duan
BMC Rheumatology (2023)
- Iain S. Forrest
- Ben O. Petrazzini
Nature Communications (2023)
Quick links
- Explore articles by subject
- Guide to authors
- Editorial policies
Sign up for the Nature Briefing newsletter — what matters in science, free to your inbox daily.


IMAGES
VIDEO
COMMENTS
The immune system is a fascinating world of cells, soluble factors, interacting cells, and tissues, all of which are interconnected. ... Extensive research has focused on deciphering the role of ...
Tackling infections is the job of different types of white blood cell. Early in an immune response, the most important of these are the innate immune system cells neutrophils and macrophages, which are the first at the scene of an environmental breach, such as an insect bite. Both of these cell types are effective killers in their own right.
The immune system also plays a critical role in maintaining homeostasis by mediating tissue repair and controlling inflammation. Unlike other organ systems that are contained within a specific tissue or structure (for example, the heart and blood vessels in the cardiovascular system), the immune system is comprised of a diverse population of ...
Abstract. Beyond structural and chemical barriers to pathogens, the immune system has two fundamental lines of defense: innate immunity and adaptive immunity. Innate immunity is the first immunological mechanism for fighting against an intruding pathogen. It is a rapid immune response, initiated within minutes or hours after aggression, that ...
The immune system is a complex network of cells, tissues and organs that work together to protect the body from harmful substances and organisms and defend against disease. ... Research Highlights ...
The interplay between the commensal microbiota and the mammalian immune system development and function includes multifold interactions in homeostasis and disease. The microbiome plays critical ...
Acquired Immune Responses. References. The immune system is an organization of cells and molecules with specialized roles in defending against infection. There are two fundamentally different ...
The immune system is highly time-of-day dependent. Pioneering studies in the 1960s were the first to identify immune responses to be under a circadian control. ... In 2019, three papers were published exploring the role of the circadian clock in ILC3 homeostasis ... Research in the laboratory is funded by the European Research Council (ERC CoG ...
Essays Biochem. 2016 Oct 31;60(3):275-301. doi: 10.1042/EBC20160017. Author Lindsay B Nicholson 1 ... From bacteria through to primates, the presence of some kind of effective immune system has gone hand in hand with evolutionary success. This article focuses on mammalian immunity, the challenges that it faces, the mechanisms by which these are ...
The mammalian immune system is a remarkable sensory system for the detection and neutralization of pathogens. History is replete with the devastating effects of plagues, and the coronavirus disease 2019 (COVID-19) pandemic is a defining global health crisis of our time. Although the development of effective vaccines has saved many lives, the ...
In contrast, long-term stress suppresses or dysregulates innate and adaptive immune responses by altering the Type 1-Type 2 cytokine balance, inducing low-grade chronic inflammation, and suppressing numbers, trafficking, and function of immunoprotective cells. Chronic stress may also increase susceptibility to some types of cancer by ...
The human immune system mobilizes a broad repertoire of innate and adaptive responses to protect against the universe of pathogens it encounters. Central to these protective responses are its mechanisms to distinguish self from nonself. This overview describes the major mechanisms used by the immune system to respond to invading microbes and identifies settings in which disturbed immune ...
Specific brain circuits recruited during stress contribute to differential immune responses and affect how the immune system handles viral and autoimmune challenges. For the past two years, the ...
Background Regular physical activity is the prime modality for the prevention of numerous non-communicable diseases and has also been advocated for resilience against COVID-19 and other infectious diseases. However, there is currently no systematic and quantitative evidence synthesis of the association between physical activity and the strength of the immune system. Objective To examine the ...
The central nervous system (CNS), endocrine system, and immune system are complex systems that interact with each other. Stressful life events and the negative emotions they generate can dysregulate the immune response by disturbing the sensitive interplay among these systems (Glaser and Kiecolt-Glaser 2005).Psychoneuroimmunology (PNI) is a field of investigation concerned with the ...
Immune Tolerance. Tolerance is the prevention of an immune response against a particular antigen. For instance, the immune system is generally tolerant of self-antigens, so it does not usually attack the body's own cells, tissues, and organs. However, when tolerance is lost, disorders like autoimmune disease or food allergy may occur.
immune-system--rsv-vaccine.jpg. In 2023, due in part to over 60 years of NIH-supported research, FDA approved a safe and effective vaccine for respiratory syncytial virus (RSV), which reduces the risk of severe disease by 94.1% in people over 60. RSV causes up to 160,000 adults over the age of 65 to be hospitalized per year in the U.S.
Published: May 16, 2024 1:27pm EDT. Until now, chemo and radiation therapy have been main cancer treatments but they can also damage healthy tissues. Immunotherapy uses the body's immune system ...
Within the physiological parameters of a protective immune response, inflammation is essential for efficient immunity, which includes tissue healing and return to homeostasis. ... Research into ...
Despite significant progress in our understanding of the pathophysiology of sepsis and extensive clinical research, there are few proven therapies addressing the underlying immune dysregulation of this life-threatening condition. The aim of this scoping review is to describe the literature evaluating immunotherapy in adult patients with sepsis, emphasizing on methods providing a ...
Selenium. Selenium supports the function of many immune cell types (see Table 1) [ 42, 43] and helps to control oxidative stress and inflammation. Extensive research in mice has shown that selenium deficiency impairs multiple immune responses and increases susceptibility to viral infection [ 44 ].
Their work further supports research in developing a germline-targeting strategy for priming the immune system to elicit a bNAb called VRC01. This bNAb was discovered by NIAID researchers almost 15 years ago. The goal of this line of research is to develop an HIV vaccine that generates multiple classes of bNAbs to prevent HIV. Article ...
Immunology is the branch of biomedical sciences concerned with all aspects of the immune system in all multicellular organisms. The 2016 Zika virus (ZIKV) epidemic catalyzed a global effort to ...
This adhesive binds the devices to tissue and prevents the immune system from attacking it. "The dream of many research groups and companies is to implant something into the body that over the long term the body will not see, and the device can provide therapeutic or diagnostic functionality. ... Paper-folding art inspires better bandages.
The immune system is a key player in maintaining physiological homeostasis and in sustaining health over disease in the human body. ... What distinguishes the paper at hand is its focus on inhalation as the only way of taking in the biogenic substances analysed. ... Very limited research has so far been carried out to address the question of ...
Their work further supports research in developing a germline-targeting strategy for priming the immune system to elicit a bNAb called VRC01. This bNAb was discovered by NIAID researchers almost 15 years ago. The goal of this line of research is to develop an HIV vaccine that generates multiple classes of bNAbs to prevent HIV. ARTICLE:
For a long time, research on immune cell engagers has focused almost exclusively on T lymphocytes in cancer treatment, probably because of the extremely important role of these cells in the ...
Tumors of the central nervous system (CNS) are severe and refractory diseases with poor prognosis, especially for patients with malignant glioblastoma and brain metastases. Currently, numerous studies have explored the potential role of bacteria and intestinal flora in tumor development and treatment. Bacteria can penetrate the blood-brain barrier (BBB), targeting the hypoxic ...
The immune system protects against viruses and diseases and produces antibodies to kill pathogens. This review presents a brief overview of the immune system regarding its protection of the human body from COVID-19; illustrates the process of the immune system, how it works, and its mechanism to fight virus; and presents information on the most recent COVID-19 treatments and experimental data.
A predisposition to autoimmunity is strongly linked to genetics, and caused by defects in mechanisms that result in loss of self-tolerance. 1 Autoimmune disease develops after further immune ...