- AI & NLP
- Churn & Loyalty
- Customer Experience
- Customer Journeys
- Customer Metrics
- Feedback Analysis
- Product Experience
- Product Updates
- Sentiment Analysis
- Surveys & Feedback Collection
- Try Thematic
Welcome to the community
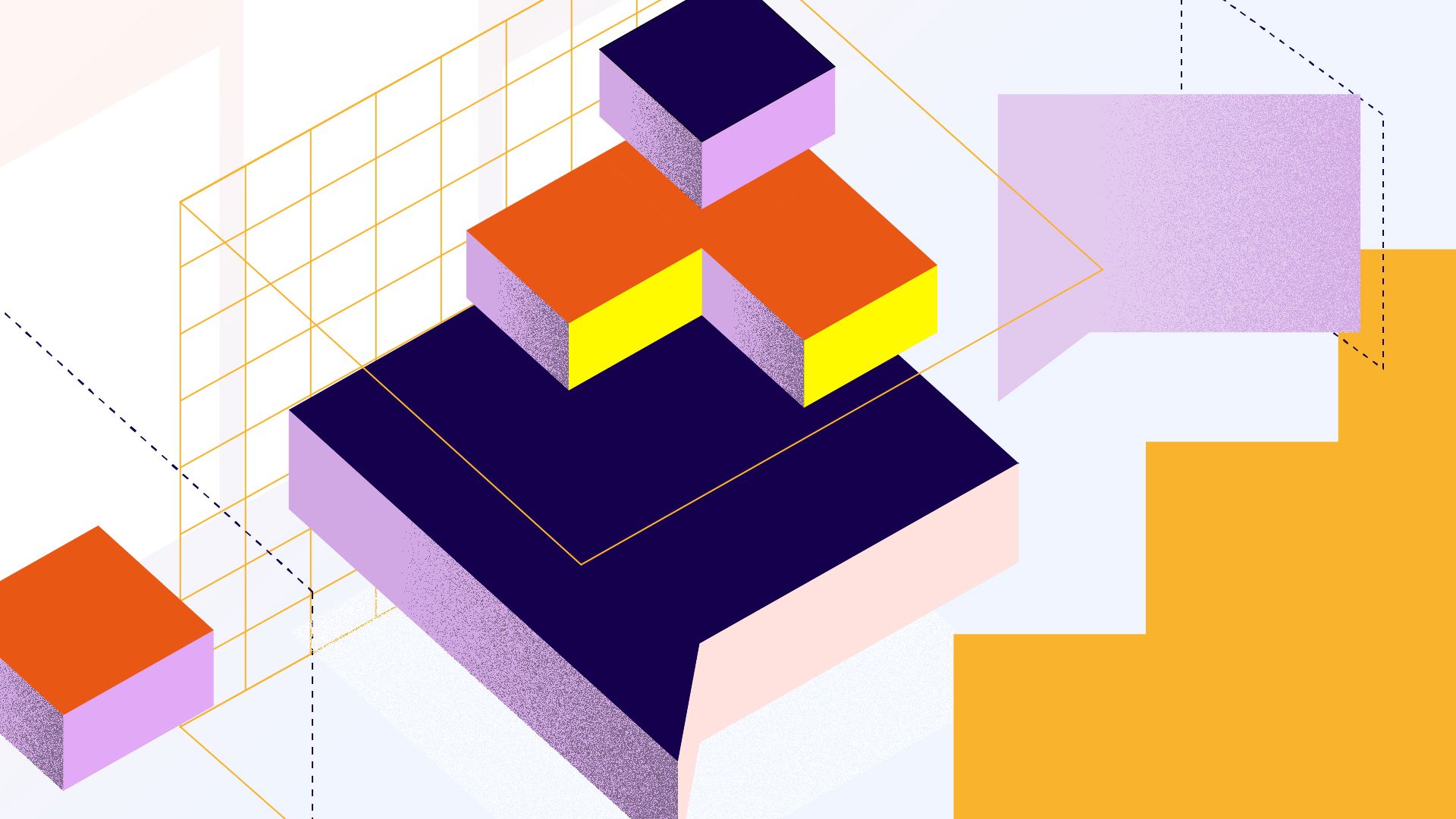

Qualitative Data Analysis: Step-by-Step Guide (Manual vs. Automatic)
When we conduct qualitative methods of research, need to explain changes in metrics or understand people's opinions, we always turn to qualitative data. Qualitative data is typically generated through:
- Interview transcripts
- Surveys with open-ended questions
- Contact center transcripts
- Texts and documents
- Audio and video recordings
- Observational notes
Compared to quantitative data, which captures structured information, qualitative data is unstructured and has more depth. It can answer our questions, can help formulate hypotheses and build understanding.
It's important to understand the differences between quantitative data & qualitative data . But unfortunately, analyzing qualitative data is difficult. While tools like Excel, Tableau and PowerBI crunch and visualize quantitative data with ease, there are a limited number of mainstream tools for analyzing qualitative data . The majority of qualitative data analysis still happens manually.
That said, there are two new trends that are changing this. First, there are advances in natural language processing (NLP) which is focused on understanding human language. Second, there is an explosion of user-friendly software designed for both researchers and businesses. Both help automate the qualitative data analysis process.
In this post we want to teach you how to conduct a successful qualitative data analysis. There are two primary qualitative data analysis methods; manual & automatic. We will teach you how to conduct the analysis manually, and also, automatically using software solutions powered by NLP. We’ll guide you through the steps to conduct a manual analysis, and look at what is involved and the role technology can play in automating this process.
More businesses are switching to fully-automated analysis of qualitative customer data because it is cheaper, faster, and just as accurate. Primarily, businesses purchase subscriptions to feedback analytics platforms so that they can understand customer pain points and sentiment.
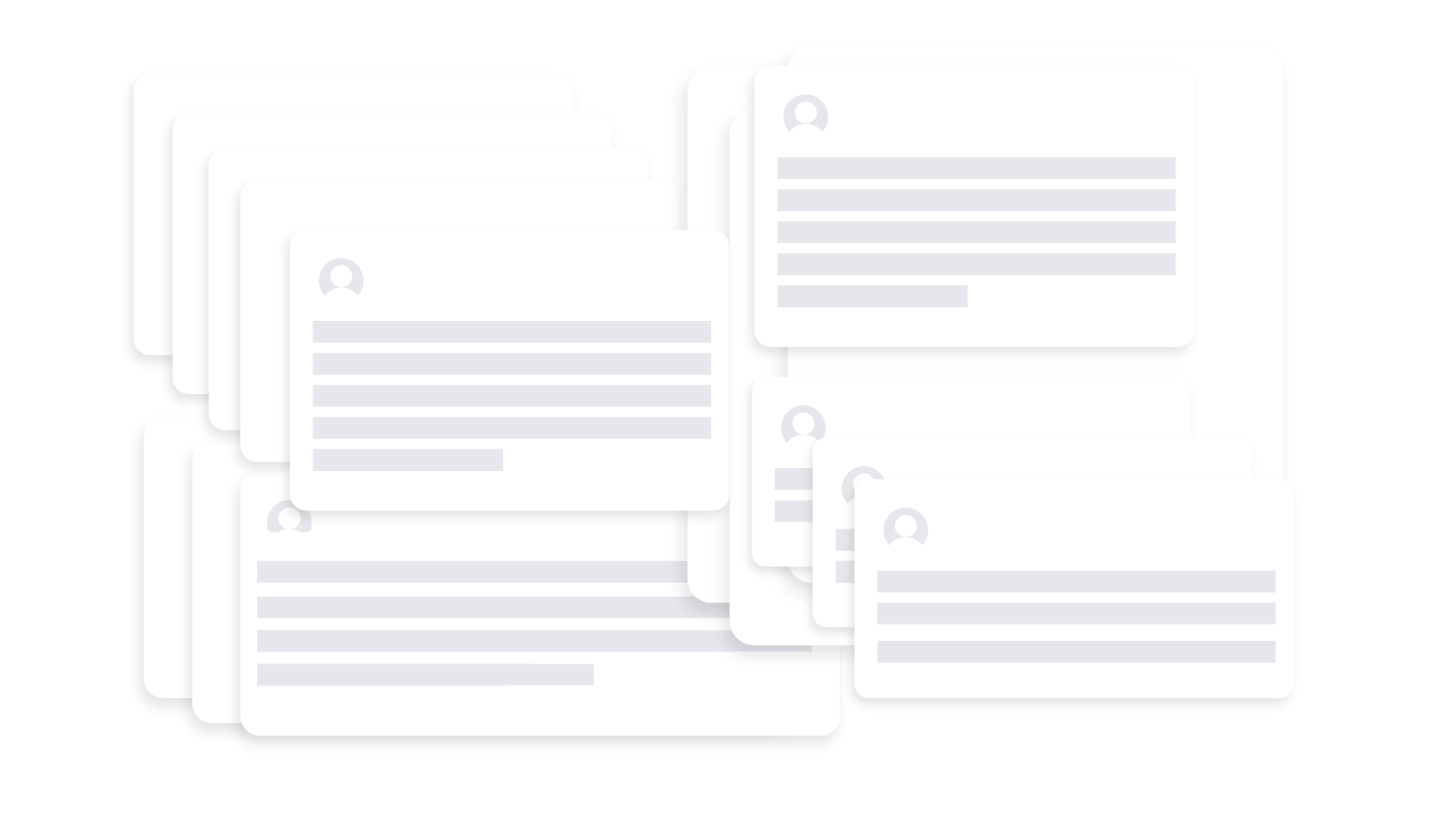
We’ll take you through 5 steps to conduct a successful qualitative data analysis. Within each step we will highlight the key difference between the manual, and automated approach of qualitative researchers. Here's an overview of the steps:
The 5 steps to doing qualitative data analysis
- Gathering and collecting your qualitative data
- Organizing and connecting into your qualitative data
- Coding your qualitative data
- Analyzing the qualitative data for insights
- Reporting on the insights derived from your analysis
What is Qualitative Data Analysis?
Qualitative data analysis is a process of gathering, structuring and interpreting qualitative data to understand what it represents.
Qualitative data is non-numerical and unstructured. Qualitative data generally refers to text, such as open-ended responses to survey questions or user interviews, but also includes audio, photos and video.
Businesses often perform qualitative data analysis on customer feedback. And within this context, qualitative data generally refers to verbatim text data collected from sources such as reviews, complaints, chat messages, support centre interactions, customer interviews, case notes or social media comments.
How is qualitative data analysis different from quantitative data analysis?
Understanding the differences between quantitative & qualitative data is important. When it comes to analyzing data, Qualitative Data Analysis serves a very different role to Quantitative Data Analysis. But what sets them apart?
Qualitative Data Analysis dives into the stories hidden in non-numerical data such as interviews, open-ended survey answers, or notes from observations. It uncovers the ‘whys’ and ‘hows’ giving a deep understanding of people’s experiences and emotions.
Quantitative Data Analysis on the other hand deals with numerical data, using statistics to measure differences, identify preferred options, and pinpoint root causes of issues. It steps back to address questions like "how many" or "what percentage" to offer broad insights we can apply to larger groups.
In short, Qualitative Data Analysis is like a microscope, helping us understand specific detail. Quantitative Data Analysis is like the telescope, giving us a broader perspective. Both are important, working together to decode data for different objectives.
Qualitative Data Analysis methods
Once all the data has been captured, there are a variety of analysis techniques available and the choice is determined by your specific research objectives and the kind of data you’ve gathered. Common qualitative data analysis methods include:
Content Analysis
This is a popular approach to qualitative data analysis. Other qualitative analysis techniques may fit within the broad scope of content analysis. Thematic analysis is a part of the content analysis. Content analysis is used to identify the patterns that emerge from text, by grouping content into words, concepts, and themes. Content analysis is useful to quantify the relationship between all of the grouped content. The Columbia School of Public Health has a detailed breakdown of content analysis .
Narrative Analysis
Narrative analysis focuses on the stories people tell and the language they use to make sense of them. It is particularly useful in qualitative research methods where customer stories are used to get a deep understanding of customers’ perspectives on a specific issue. A narrative analysis might enable us to summarize the outcomes of a focused case study.
Discourse Analysis
Discourse analysis is used to get a thorough understanding of the political, cultural and power dynamics that exist in specific situations. The focus of discourse analysis here is on the way people express themselves in different social contexts. Discourse analysis is commonly used by brand strategists who hope to understand why a group of people feel the way they do about a brand or product.
Thematic Analysis
Thematic analysis is used to deduce the meaning behind the words people use. This is accomplished by discovering repeating themes in text. These meaningful themes reveal key insights into data and can be quantified, particularly when paired with sentiment analysis . Often, the outcome of thematic analysis is a code frame that captures themes in terms of codes, also called categories. So the process of thematic analysis is also referred to as “coding”. A common use-case for thematic analysis in companies is analysis of customer feedback.
Grounded Theory
Grounded theory is a useful approach when little is known about a subject. Grounded theory starts by formulating a theory around a single data case. This means that the theory is “grounded”. Grounded theory analysis is based on actual data, and not entirely speculative. Then additional cases can be examined to see if they are relevant and can add to the original grounded theory.
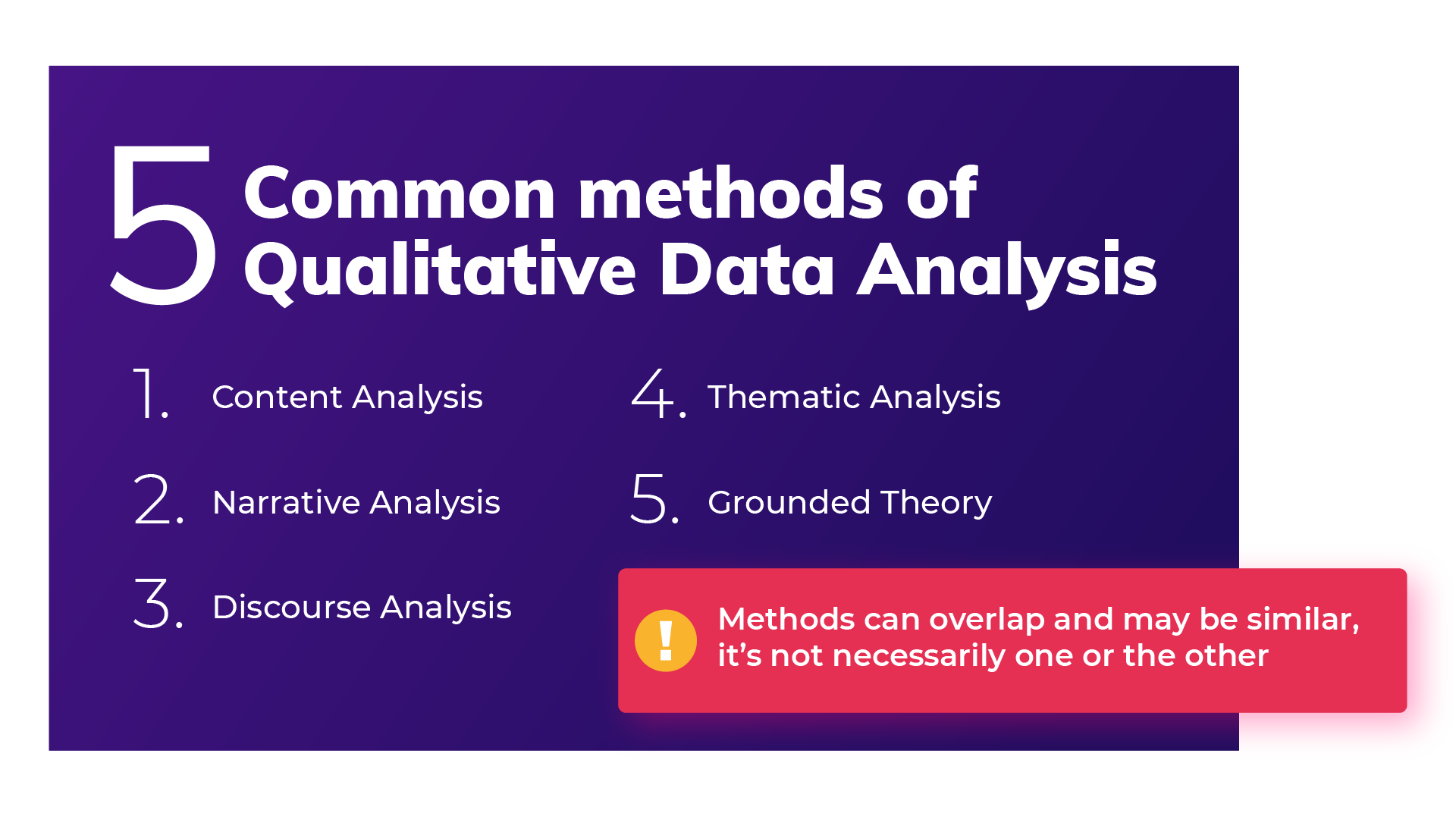
Challenges of Qualitative Data Analysis
While Qualitative Data Analysis offers rich insights, it comes with its challenges. Each unique QDA method has its unique hurdles. Let’s take a look at the challenges researchers and analysts might face, depending on the chosen method.
- Time and Effort (Narrative Analysis): Narrative analysis, which focuses on personal stories, demands patience. Sifting through lengthy narratives to find meaningful insights can be time-consuming, requires dedicated effort.
- Being Objective (Grounded Theory): Grounded theory, building theories from data, faces the challenges of personal biases. Staying objective while interpreting data is crucial, ensuring conclusions are rooted in the data itself.
- Complexity (Thematic Analysis): Thematic analysis involves identifying themes within data, a process that can be intricate. Categorizing and understanding themes can be complex, especially when each piece of data varies in context and structure. Thematic Analysis software can simplify this process.
- Generalizing Findings (Narrative Analysis): Narrative analysis, dealing with individual stories, makes drawing broad challenging. Extending findings from a single narrative to a broader context requires careful consideration.
- Managing Data (Thematic Analysis): Thematic analysis involves organizing and managing vast amounts of unstructured data, like interview transcripts. Managing this can be a hefty task, requiring effective data management strategies.
- Skill Level (Grounded Theory): Grounded theory demands specific skills to build theories from the ground up. Finding or training analysts with these skills poses a challenge, requiring investment in building expertise.
Benefits of qualitative data analysis
Qualitative Data Analysis (QDA) is like a versatile toolkit, offering a tailored approach to understanding your data. The benefits it offers are as diverse as the methods. Let’s explore why choosing the right method matters.
- Tailored Methods for Specific Needs: QDA isn't one-size-fits-all. Depending on your research objectives and the type of data at hand, different methods offer unique benefits. If you want emotive customer stories, narrative analysis paints a strong picture. When you want to explain a score, thematic analysis reveals insightful patterns
- Flexibility with Thematic Analysis: thematic analysis is like a chameleon in the toolkit of QDA. It adapts well to different types of data and research objectives, making it a top choice for any qualitative analysis.
- Deeper Understanding, Better Products: QDA helps you dive into people's thoughts and feelings. This deep understanding helps you build products and services that truly matches what people want, ensuring satisfied customers
- Finding the Unexpected: Qualitative data often reveals surprises that we miss in quantitative data. QDA offers us new ideas and perspectives, for insights we might otherwise miss.
- Building Effective Strategies: Insights from QDA are like strategic guides. They help businesses in crafting plans that match people’s desires.
- Creating Genuine Connections: Understanding people’s experiences lets businesses connect on a real level. This genuine connection helps build trust and loyalty, priceless for any business.
How to do Qualitative Data Analysis: 5 steps
Now we are going to show how you can do your own qualitative data analysis. We will guide you through this process step by step. As mentioned earlier, you will learn how to do qualitative data analysis manually , and also automatically using modern qualitative data and thematic analysis software.
To get best value from the analysis process and research process, it’s important to be super clear about the nature and scope of the question that’s being researched. This will help you select the research collection channels that are most likely to help you answer your question.
Depending on if you are a business looking to understand customer sentiment, or an academic surveying a school, your approach to qualitative data analysis will be unique.
Once you’re clear, there’s a sequence to follow. And, though there are differences in the manual and automatic approaches, the process steps are mostly the same.
The use case for our step-by-step guide is a company looking to collect data (customer feedback data), and analyze the customer feedback - in order to improve customer experience. By analyzing the customer feedback the company derives insights about their business and their customers. You can follow these same steps regardless of the nature of your research. Let’s get started.
Step 1: Gather your qualitative data and conduct research (Conduct qualitative research)
The first step of qualitative research is to do data collection. Put simply, data collection is gathering all of your data for analysis. A common situation is when qualitative data is spread across various sources.
Classic methods of gathering qualitative data
Most companies use traditional methods for gathering qualitative data: conducting interviews with research participants, running surveys, and running focus groups. This data is typically stored in documents, CRMs, databases and knowledge bases. It’s important to examine which data is available and needs to be included in your research project, based on its scope.
Using your existing qualitative feedback
As it becomes easier for customers to engage across a range of different channels, companies are gathering increasingly large amounts of both solicited and unsolicited qualitative feedback.
Most organizations have now invested in Voice of Customer programs , support ticketing systems, chatbot and support conversations, emails and even customer Slack chats.
These new channels provide companies with new ways of getting feedback, and also allow the collection of unstructured feedback data at scale.
The great thing about this data is that it contains a wealth of valubale insights and that it’s already there! When you have a new question about user behavior or your customers, you don’t need to create a new research study or set up a focus group. You can find most answers in the data you already have.
Typically, this data is stored in third-party solutions or a central database, but there are ways to export it or connect to a feedback analysis solution through integrations or an API.
Utilize untapped qualitative data channels
There are many online qualitative data sources you may not have considered. For example, you can find useful qualitative data in social media channels like Twitter or Facebook. Online forums, review sites, and online communities such as Discourse or Reddit also contain valuable data about your customers, or research questions.
If you are considering performing a qualitative benchmark analysis against competitors - the internet is your best friend. Gathering feedback in competitor reviews on sites like Trustpilot, G2, Capterra, Better Business Bureau or on app stores is a great way to perform a competitor benchmark analysis.
Customer feedback analysis software often has integrations into social media and review sites, or you could use a solution like DataMiner to scrape the reviews.
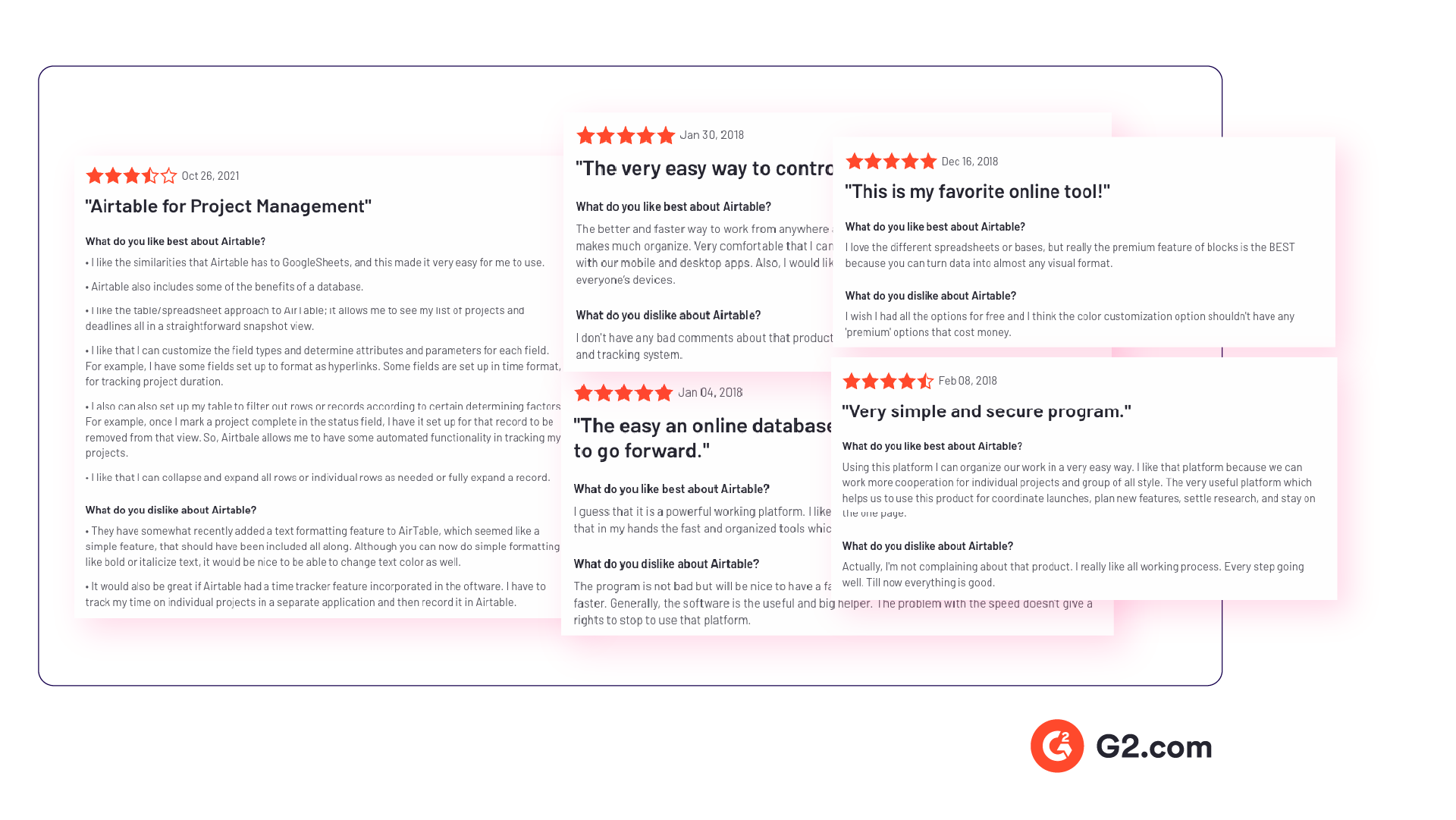
Step 2: Connect & organize all your qualitative data
Now you all have this qualitative data but there’s a problem, the data is unstructured. Before feedback can be analyzed and assigned any value, it needs to be organized in a single place. Why is this important? Consistency!
If all data is easily accessible in one place and analyzed in a consistent manner, you will have an easier time summarizing and making decisions based on this data.
The manual approach to organizing your data
The classic method of structuring qualitative data is to plot all the raw data you’ve gathered into a spreadsheet.
Typically, research and support teams would share large Excel sheets and different business units would make sense of the qualitative feedback data on their own. Each team collects and organizes the data in a way that best suits them, which means the feedback tends to be kept in separate silos.
An alternative and a more robust solution is to store feedback in a central database, like Snowflake or Amazon Redshift .
Keep in mind that when you organize your data in this way, you are often preparing it to be imported into another software. If you go the route of a database, you would need to use an API to push the feedback into a third-party software.
Computer-assisted qualitative data analysis software (CAQDAS)
Traditionally within the manual analysis approach (but not always), qualitative data is imported into CAQDAS software for coding.
In the early 2000s, CAQDAS software was popularised by developers such as ATLAS.ti, NVivo and MAXQDA and eagerly adopted by researchers to assist with the organizing and coding of data.
The benefits of using computer-assisted qualitative data analysis software:
- Assists in the organizing of your data
- Opens you up to exploring different interpretations of your data analysis
- Allows you to share your dataset easier and allows group collaboration (allows for secondary analysis)
However you still need to code the data, uncover the themes and do the analysis yourself. Therefore it is still a manual approach.
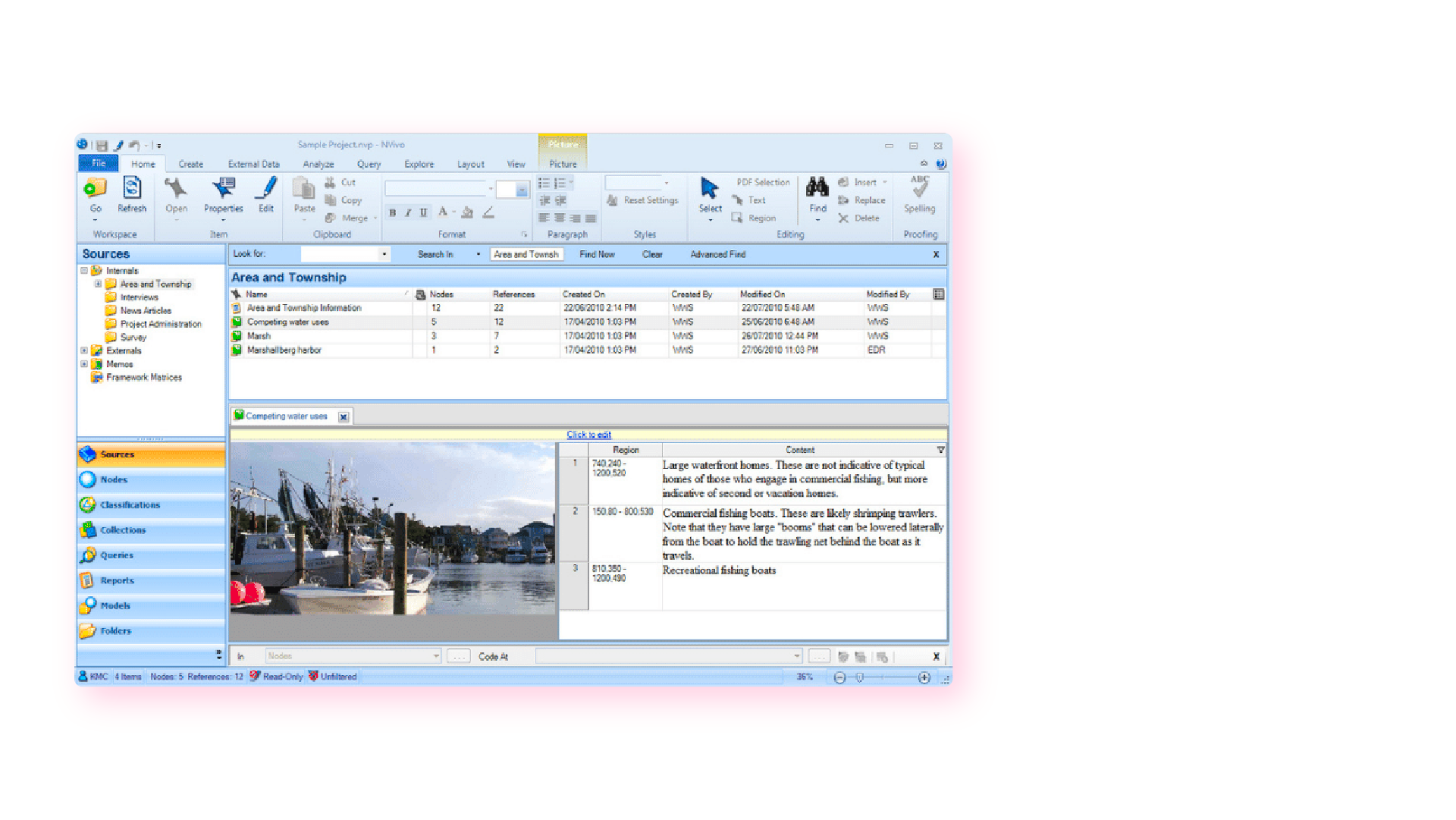
Organizing your qualitative data in a feedback repository
Another solution to organizing your qualitative data is to upload it into a feedback repository where it can be unified with your other data , and easily searchable and taggable. There are a number of software solutions that act as a central repository for your qualitative research data. Here are a couple solutions that you could investigate:
- Dovetail: Dovetail is a research repository with a focus on video and audio transcriptions. You can tag your transcriptions within the platform for theme analysis. You can also upload your other qualitative data such as research reports, survey responses, support conversations, and customer interviews. Dovetail acts as a single, searchable repository. And makes it easier to collaborate with other people around your qualitative research.
- EnjoyHQ: EnjoyHQ is another research repository with similar functionality to Dovetail. It boasts a more sophisticated search engine, but it has a higher starting subscription cost.
Organizing your qualitative data in a feedback analytics platform
If you have a lot of qualitative customer or employee feedback, from the likes of customer surveys or employee surveys, you will benefit from a feedback analytics platform. A feedback analytics platform is a software that automates the process of both sentiment analysis and thematic analysis . Companies use the integrations offered by these platforms to directly tap into their qualitative data sources (review sites, social media, survey responses, etc.). The data collected is then organized and analyzed consistently within the platform.
If you have data prepared in a spreadsheet, it can also be imported into feedback analytics platforms.
Once all this rich data has been organized within the feedback analytics platform, it is ready to be coded and themed, within the same platform. Thematic is a feedback analytics platform that offers one of the largest libraries of integrations with qualitative data sources.
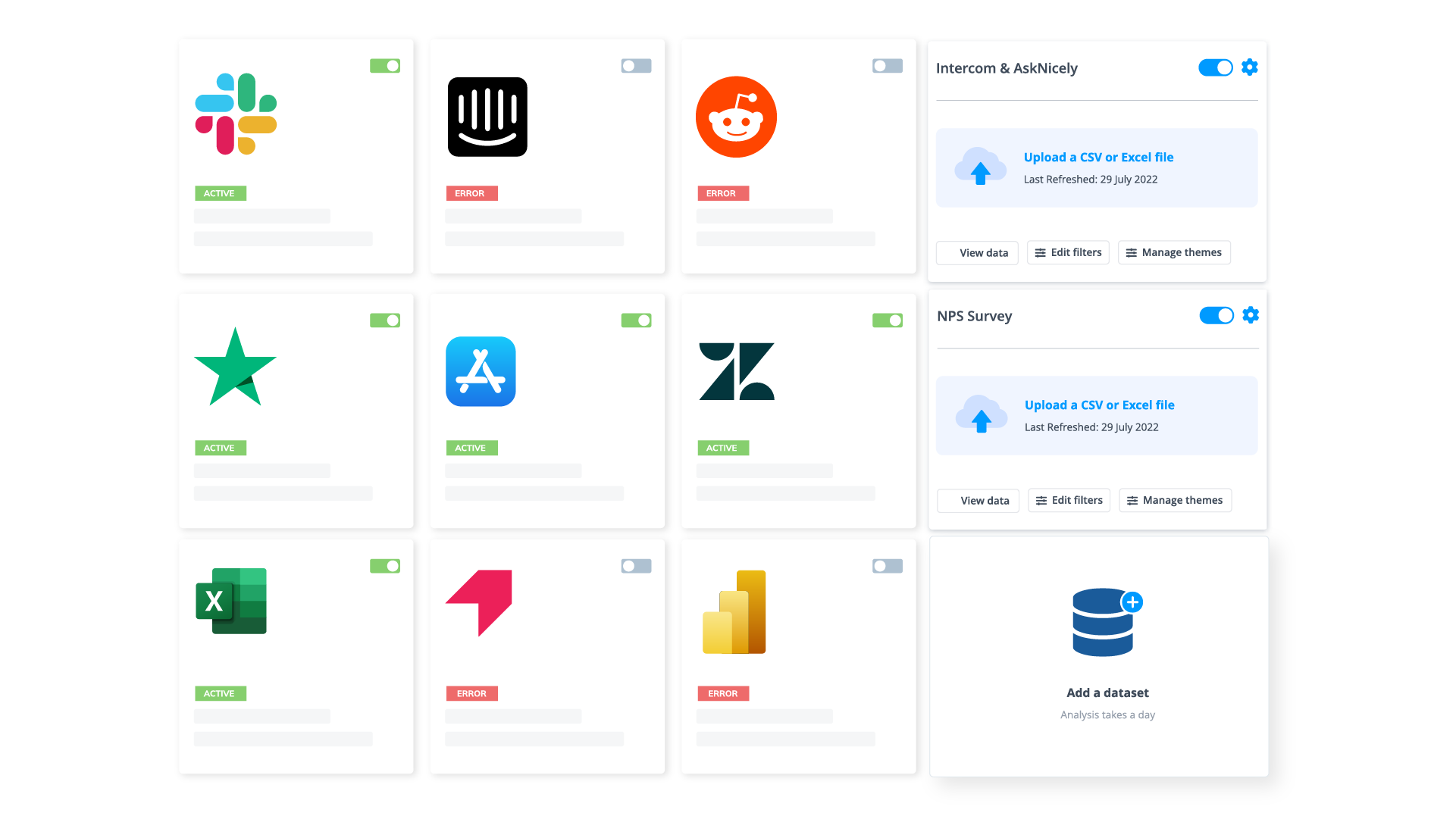
Step 3: Coding your qualitative data
Your feedback data is now organized in one place. Either within your spreadsheet, CAQDAS, feedback repository or within your feedback analytics platform. The next step is to code your feedback data so we can extract meaningful insights in the next step.
Coding is the process of labelling and organizing your data in such a way that you can then identify themes in the data, and the relationships between these themes.
To simplify the coding process, you will take small samples of your customer feedback data, come up with a set of codes, or categories capturing themes, and label each piece of feedback, systematically, for patterns and meaning. Then you will take a larger sample of data, revising and refining the codes for greater accuracy and consistency as you go.
If you choose to use a feedback analytics platform, much of this process will be automated and accomplished for you.
The terms to describe different categories of meaning (‘theme’, ‘code’, ‘tag’, ‘category’ etc) can be confusing as they are often used interchangeably. For clarity, this article will use the term ‘code’.
To code means to identify key words or phrases and assign them to a category of meaning. “I really hate the customer service of this computer software company” would be coded as “poor customer service”.
How to manually code your qualitative data
- Decide whether you will use deductive or inductive coding. Deductive coding is when you create a list of predefined codes, and then assign them to the qualitative data. Inductive coding is the opposite of this, you create codes based on the data itself. Codes arise directly from the data and you label them as you go. You need to weigh up the pros and cons of each coding method and select the most appropriate.
- Read through the feedback data to get a broad sense of what it reveals. Now it’s time to start assigning your first set of codes to statements and sections of text.
- Keep repeating step 2, adding new codes and revising the code description as often as necessary. Once it has all been coded, go through everything again, to be sure there are no inconsistencies and that nothing has been overlooked.
- Create a code frame to group your codes. The coding frame is the organizational structure of all your codes. And there are two commonly used types of coding frames, flat, or hierarchical. A hierarchical code frame will make it easier for you to derive insights from your analysis.
- Based on the number of times a particular code occurs, you can now see the common themes in your feedback data. This is insightful! If ‘bad customer service’ is a common code, it’s time to take action.
We have a detailed guide dedicated to manually coding your qualitative data .
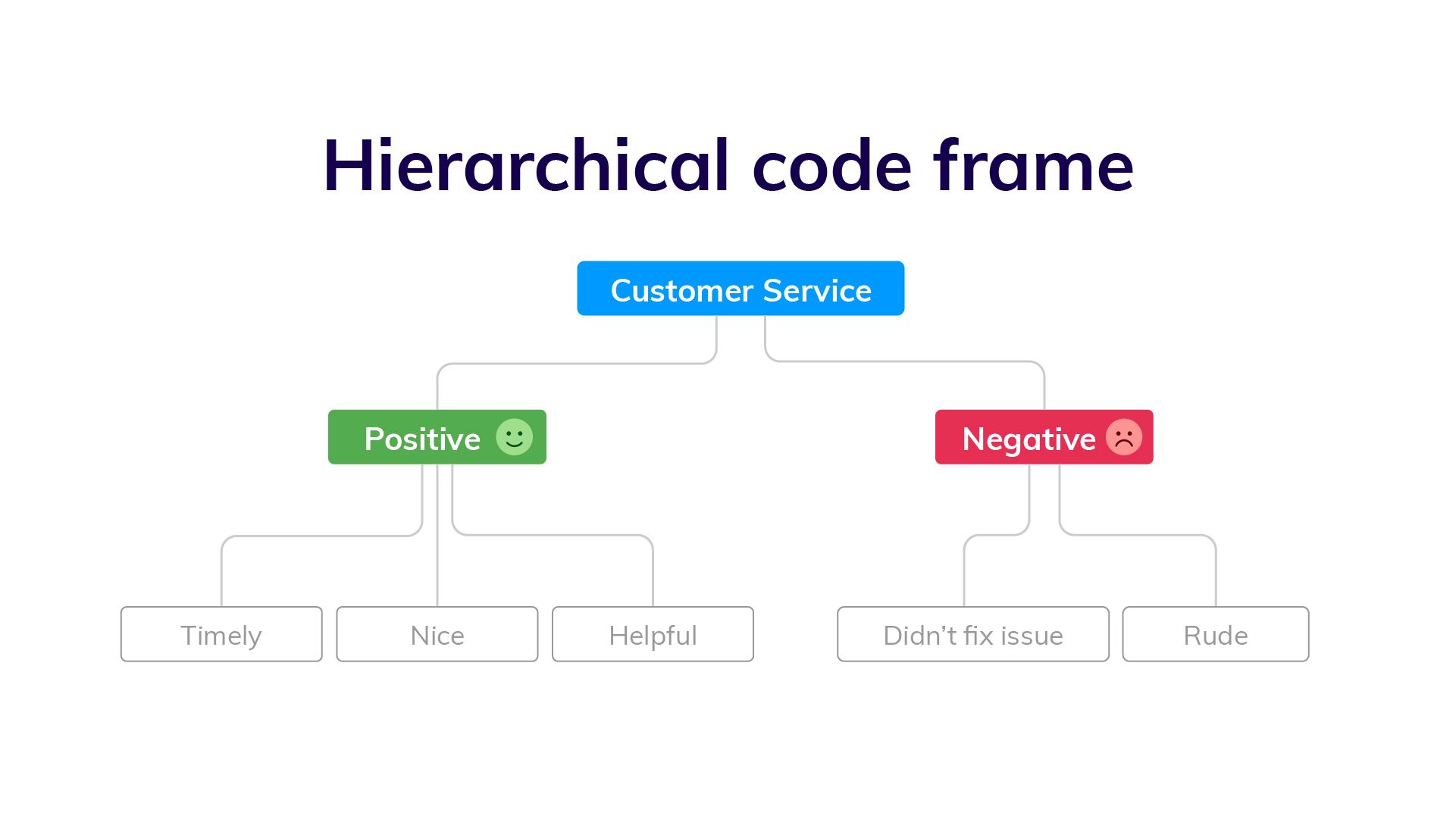
Using software to speed up manual coding of qualitative data
An Excel spreadsheet is still a popular method for coding. But various software solutions can help speed up this process. Here are some examples.
- CAQDAS / NVivo - CAQDAS software has built-in functionality that allows you to code text within their software. You may find the interface the software offers easier for managing codes than a spreadsheet.
- Dovetail/EnjoyHQ - You can tag transcripts and other textual data within these solutions. As they are also repositories you may find it simpler to keep the coding in one platform.
- IBM SPSS - SPSS is a statistical analysis software that may make coding easier than in a spreadsheet.
- Ascribe - Ascribe’s ‘Coder’ is a coding management system. Its user interface will make it easier for you to manage your codes.
Automating the qualitative coding process using thematic analysis software
In solutions which speed up the manual coding process, you still have to come up with valid codes and often apply codes manually to pieces of feedback. But there are also solutions that automate both the discovery and the application of codes.
Advances in machine learning have now made it possible to read, code and structure qualitative data automatically. This type of automated coding is offered by thematic analysis software .
Automation makes it far simpler and faster to code the feedback and group it into themes. By incorporating natural language processing (NLP) into the software, the AI looks across sentences and phrases to identify common themes meaningful statements. Some automated solutions detect repeating patterns and assign codes to them, others make you train the AI by providing examples. You could say that the AI learns the meaning of the feedback on its own.
Thematic automates the coding of qualitative feedback regardless of source. There’s no need to set up themes or categories in advance. Simply upload your data and wait a few minutes. You can also manually edit the codes to further refine their accuracy. Experiments conducted indicate that Thematic’s automated coding is just as accurate as manual coding .
Paired with sentiment analysis and advanced text analytics - these automated solutions become powerful for deriving quality business or research insights.
You could also build your own , if you have the resources!
The key benefits of using an automated coding solution
Automated analysis can often be set up fast and there’s the potential to uncover things that would never have been revealed if you had given the software a prescribed list of themes to look for.
Because the model applies a consistent rule to the data, it captures phrases or statements that a human eye might have missed.
Complete and consistent analysis of customer feedback enables more meaningful findings. Leading us into step 4.
Step 4: Analyze your data: Find meaningful insights
Now we are going to analyze our data to find insights. This is where we start to answer our research questions. Keep in mind that step 4 and step 5 (tell the story) have some overlap . This is because creating visualizations is both part of analysis process and reporting.
The task of uncovering insights is to scour through the codes that emerge from the data and draw meaningful correlations from them. It is also about making sure each insight is distinct and has enough data to support it.
Part of the analysis is to establish how much each code relates to different demographics and customer profiles, and identify whether there’s any relationship between these data points.
Manually create sub-codes to improve the quality of insights
If your code frame only has one level, you may find that your codes are too broad to be able to extract meaningful insights. This is where it is valuable to create sub-codes to your primary codes. This process is sometimes referred to as meta coding.
Note: If you take an inductive coding approach, you can create sub-codes as you are reading through your feedback data and coding it.
While time-consuming, this exercise will improve the quality of your analysis. Here is an example of what sub-codes could look like.
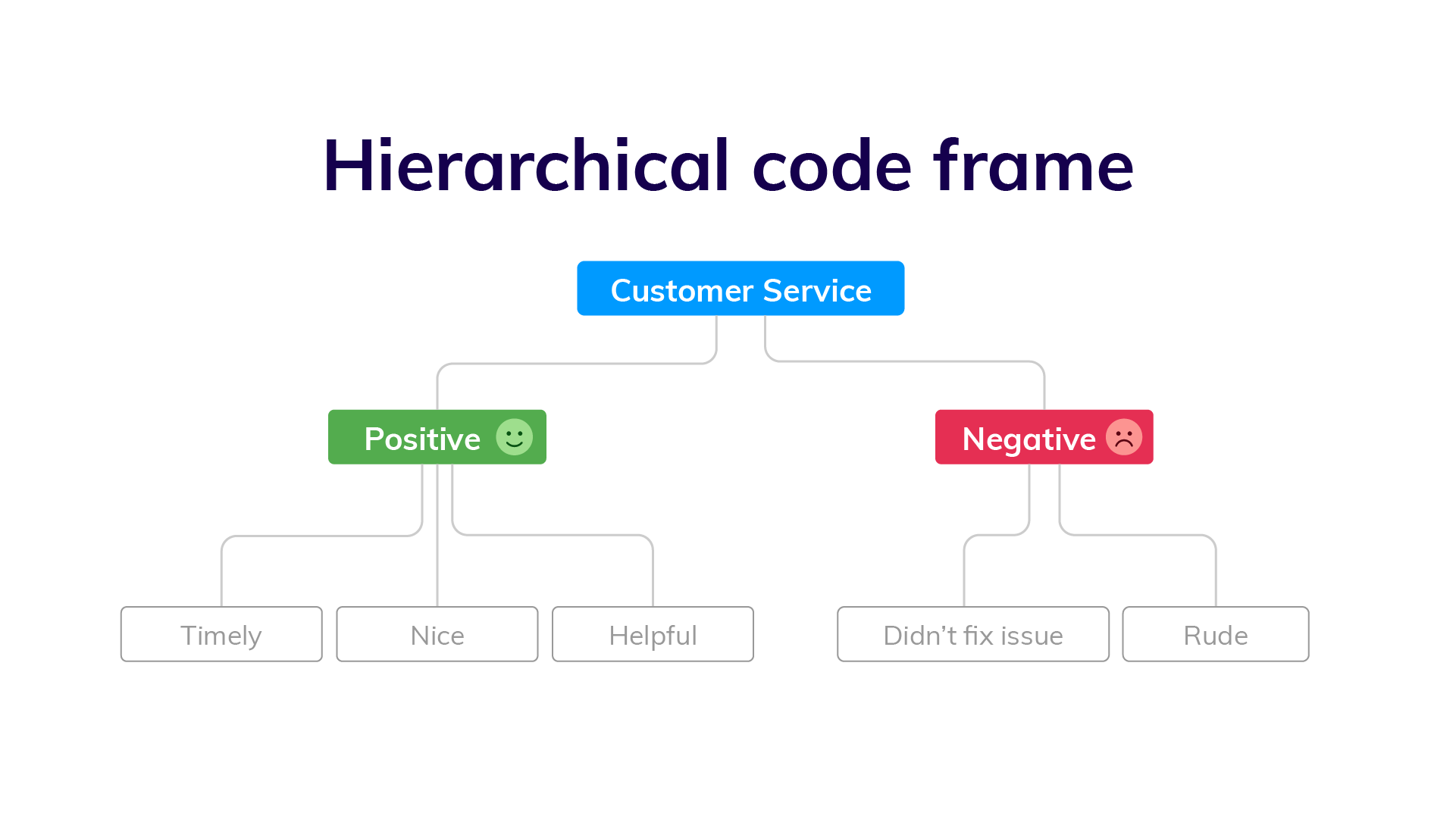
You need to carefully read your qualitative data to create quality sub-codes. But as you can see, the depth of analysis is greatly improved. By calculating the frequency of these sub-codes you can get insight into which customer service problems you can immediately address.
Correlate the frequency of codes to customer segments
Many businesses use customer segmentation . And you may have your own respondent segments that you can apply to your qualitative analysis. Segmentation is the practise of dividing customers or research respondents into subgroups.
Segments can be based on:
- Demographic
- And any other data type that you care to segment by
It is particularly useful to see the occurrence of codes within your segments. If one of your customer segments is considered unimportant to your business, but they are the cause of nearly all customer service complaints, it may be in your best interest to focus attention elsewhere. This is a useful insight!
Manually visualizing coded qualitative data
There are formulas you can use to visualize key insights in your data. The formulas we will suggest are imperative if you are measuring a score alongside your feedback.
If you are collecting a metric alongside your qualitative data this is a key visualization. Impact answers the question: “What’s the impact of a code on my overall score?”. Using Net Promoter Score (NPS) as an example, first you need to:
- Calculate overall NPS
- Calculate NPS in the subset of responses that do not contain that theme
- Subtract B from A
Then you can use this simple formula to calculate code impact on NPS .
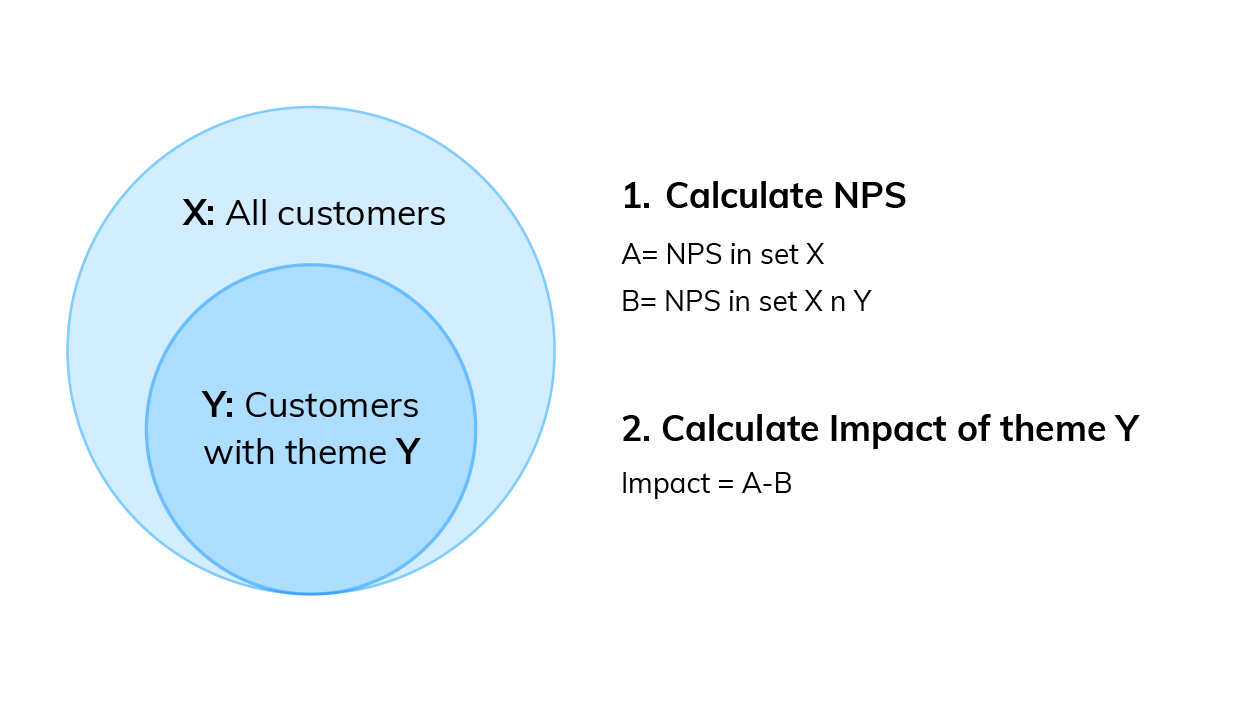
You can then visualize this data using a bar chart.
You can download our CX toolkit - it includes a template to recreate this.
Trends over time
This analysis can help you answer questions like: “Which codes are linked to decreases or increases in my score over time?”
We need to compare two sequences of numbers: NPS over time and code frequency over time . Using Excel, calculate the correlation between the two sequences, which can be either positive (the more codes the higher the NPS, see picture below), or negative (the more codes the lower the NPS).
Now you need to plot code frequency against the absolute value of code correlation with NPS. Here is the formula:
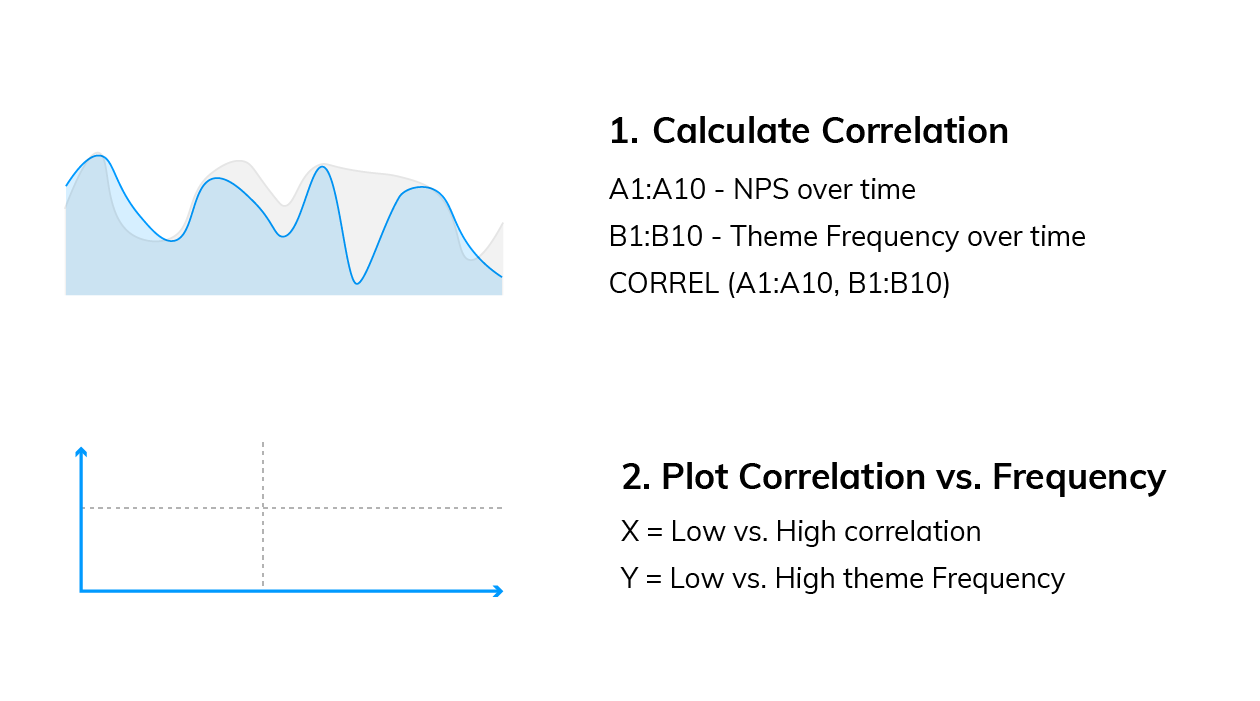
The visualization could look like this:
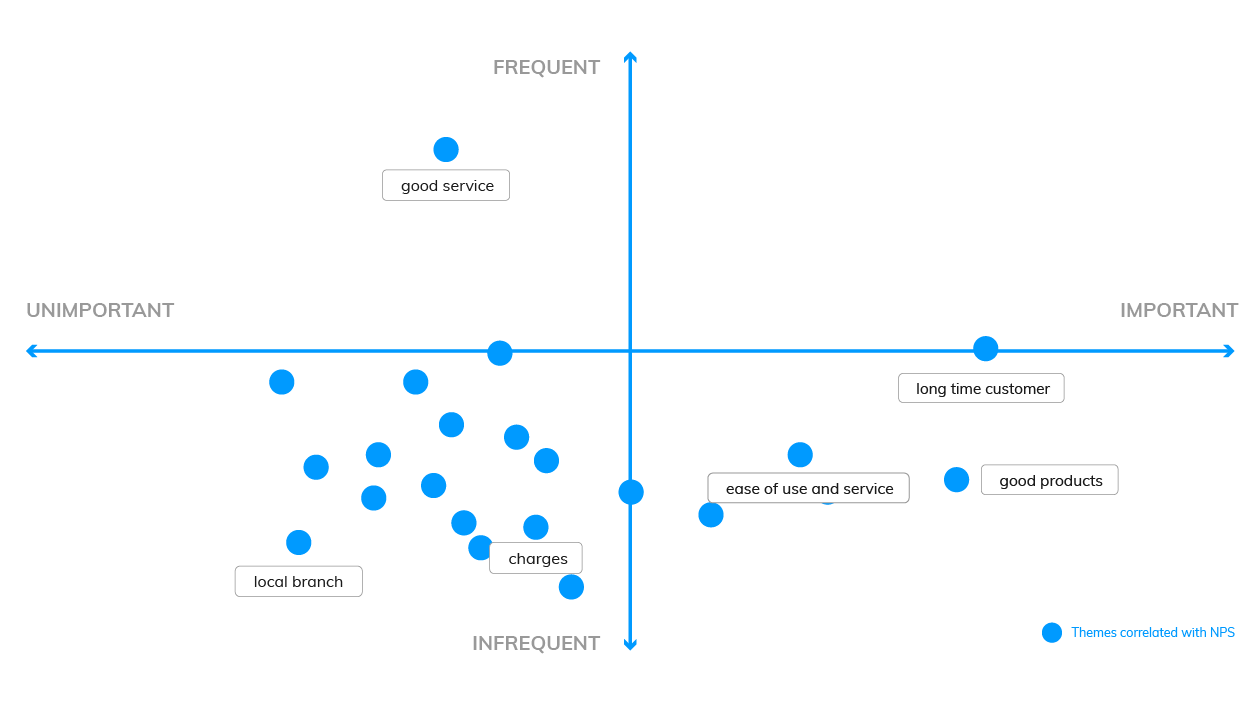
These are two examples, but there are more. For a third manual formula, and to learn why word clouds are not an insightful form of analysis, read our visualizations article .
Using a text analytics solution to automate analysis
Automated text analytics solutions enable codes and sub-codes to be pulled out of the data automatically. This makes it far faster and easier to identify what’s driving negative or positive results. And to pick up emerging trends and find all manner of rich insights in the data.
Another benefit of AI-driven text analytics software is its built-in capability for sentiment analysis, which provides the emotive context behind your feedback and other qualitative textual data therein.
Thematic provides text analytics that goes further by allowing users to apply their expertise on business context to edit or augment the AI-generated outputs.
Since the move away from manual research is generally about reducing the human element, adding human input to the technology might sound counter-intuitive. However, this is mostly to make sure important business nuances in the feedback aren’t missed during coding. The result is a higher accuracy of analysis. This is sometimes referred to as augmented intelligence .
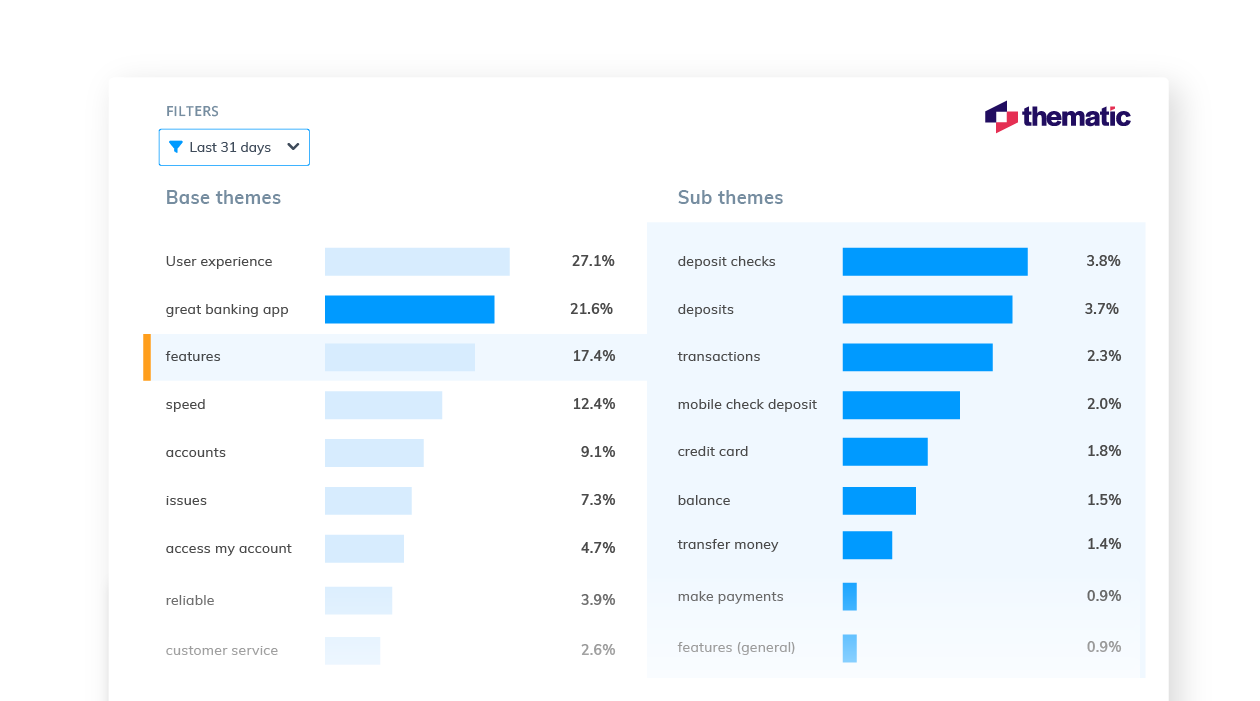
Step 5: Report on your data: Tell the story
The last step of analyzing your qualitative data is to report on it, to tell the story. At this point, the codes are fully developed and the focus is on communicating the narrative to the audience.
A coherent outline of the qualitative research, the findings and the insights is vital for stakeholders to discuss and debate before they can devise a meaningful course of action.
Creating graphs and reporting in Powerpoint
Typically, qualitative researchers take the tried and tested approach of distilling their report into a series of charts, tables and other visuals which are woven into a narrative for presentation in Powerpoint.
Using visualization software for reporting
With data transformation and APIs, the analyzed data can be shared with data visualisation software, such as Power BI or Tableau , Google Studio or Looker. Power BI and Tableau are among the most preferred options.
Visualizing your insights inside a feedback analytics platform
Feedback analytics platforms, like Thematic, incorporate visualisation tools that intuitively turn key data and insights into graphs. This removes the time consuming work of constructing charts to visually identify patterns and creates more time to focus on building a compelling narrative that highlights the insights, in bite-size chunks, for executive teams to review.
Using a feedback analytics platform with visualization tools means you don’t have to use a separate product for visualizations. You can export graphs into Powerpoints straight from the platforms.
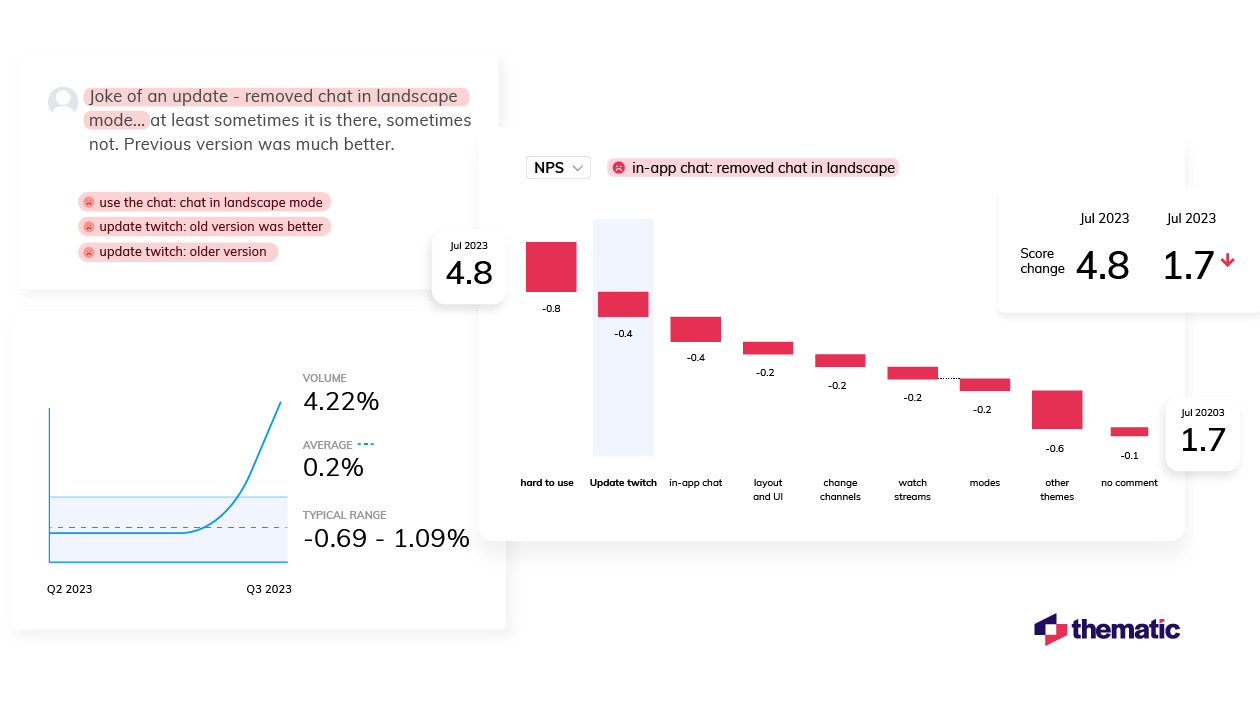
Conclusion - Manual or Automated?
There are those who remain deeply invested in the manual approach - because it’s familiar, because they’re reluctant to spend money and time learning new software, or because they’ve been burned by the overpromises of AI.
For projects that involve small datasets, manual analysis makes sense. For example, if the objective is simply to quantify a simple question like “Do customers prefer X concepts to Y?”. If the findings are being extracted from a small set of focus groups and interviews, sometimes it’s easier to just read them
However, as new generations come into the workplace, it’s technology-driven solutions that feel more comfortable and practical. And the merits are undeniable. Especially if the objective is to go deeper and understand the ‘why’ behind customers’ preference for X or Y. And even more especially if time and money are considerations.
The ability to collect a free flow of qualitative feedback data at the same time as the metric means AI can cost-effectively scan, crunch, score and analyze a ton of feedback from one system in one go. And time-intensive processes like focus groups, or coding, that used to take weeks, can now be completed in a matter of hours or days.
But aside from the ever-present business case to speed things up and keep costs down, there are also powerful research imperatives for automated analysis of qualitative data: namely, accuracy and consistency.
Finding insights hidden in feedback requires consistency, especially in coding. Not to mention catching all the ‘unknown unknowns’ that can skew research findings and steering clear of cognitive bias.
Some say without manual data analysis researchers won’t get an accurate “feel” for the insights. However, the larger data sets are, the harder it is to sort through the feedback and organize feedback that has been pulled from different places. And, the more difficult it is to stay on course, the greater the risk of drawing incorrect, or incomplete, conclusions grows.
Though the process steps for qualitative data analysis have remained pretty much unchanged since psychologist Paul Felix Lazarsfeld paved the path a hundred years ago, the impact digital technology has had on types of qualitative feedback data and the approach to the analysis are profound.
If you want to try an automated feedback analysis solution on your own qualitative data, you can get started with Thematic .
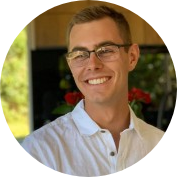
Community & Marketing
Tyler manages our community of CX, insights & analytics professionals. Tyler's goal is to help unite insights professionals around common challenges.
We make it easy to discover the customer and product issues that matter.
Unlock the value of feedback at scale, in one platform. Try it for free now!
- Questions to ask your Feedback Analytics vendor
- How to end customer churn for good
- Scalable analysis of NPS verbatims
- 5 Text analytics approaches
- How to calculate the ROI of CX
Our experts will show you how Thematic works, how to discover pain points and track the ROI of decisions. To access your free trial, book a personal demo today.
Recent posts
Watercare is New Zealand's largest water and wastewater service provider. They are responsible for bringing clean water to 1.7 million people in Tamaki Makaurau (Auckland) and safeguarding the wastewater network to minimize impact on the environment. Water is a sector that often gets taken for granted, with drainage and
Become a qualitative theming pro! Creating a perfect code frame is hard, but thematic analysis software makes the process much easier.
Qualtrics is one of the most well-known and powerful Customer Feedback Management platforms. But even so, it has limitations. We recently hosted a live panel where data analysts from two well-known brands shared their experiences with Qualtrics, and how they extended this platform’s capabilities. Below, we’ll share the
Have a language expert improve your writing
Run a free plagiarism check in 10 minutes, automatically generate references for free.
- Knowledge Base
- Methodology
- Case Study | Definition, Examples & Methods
Case Study | Definition, Examples & Methods
Published on 5 May 2022 by Shona McCombes . Revised on 30 January 2023.
A case study is a detailed study of a specific subject, such as a person, group, place, event, organisation, or phenomenon. Case studies are commonly used in social, educational, clinical, and business research.
A case study research design usually involves qualitative methods , but quantitative methods are sometimes also used. Case studies are good for describing , comparing, evaluating, and understanding different aspects of a research problem .
Table of contents
When to do a case study, step 1: select a case, step 2: build a theoretical framework, step 3: collect your data, step 4: describe and analyse the case.
A case study is an appropriate research design when you want to gain concrete, contextual, in-depth knowledge about a specific real-world subject. It allows you to explore the key characteristics, meanings, and implications of the case.
Case studies are often a good choice in a thesis or dissertation . They keep your project focused and manageable when you don’t have the time or resources to do large-scale research.
You might use just one complex case study where you explore a single subject in depth, or conduct multiple case studies to compare and illuminate different aspects of your research problem.
Prevent plagiarism, run a free check.
Once you have developed your problem statement and research questions , you should be ready to choose the specific case that you want to focus on. A good case study should have the potential to:
- Provide new or unexpected insights into the subject
- Challenge or complicate existing assumptions and theories
- Propose practical courses of action to resolve a problem
- Open up new directions for future research
Unlike quantitative or experimental research, a strong case study does not require a random or representative sample. In fact, case studies often deliberately focus on unusual, neglected, or outlying cases which may shed new light on the research problem.
If you find yourself aiming to simultaneously investigate and solve an issue, consider conducting action research . As its name suggests, action research conducts research and takes action at the same time, and is highly iterative and flexible.
However, you can also choose a more common or representative case to exemplify a particular category, experience, or phenomenon.
While case studies focus more on concrete details than general theories, they should usually have some connection with theory in the field. This way the case study is not just an isolated description, but is integrated into existing knowledge about the topic. It might aim to:
- Exemplify a theory by showing how it explains the case under investigation
- Expand on a theory by uncovering new concepts and ideas that need to be incorporated
- Challenge a theory by exploring an outlier case that doesn’t fit with established assumptions
To ensure that your analysis of the case has a solid academic grounding, you should conduct a literature review of sources related to the topic and develop a theoretical framework . This means identifying key concepts and theories to guide your analysis and interpretation.
There are many different research methods you can use to collect data on your subject. Case studies tend to focus on qualitative data using methods such as interviews, observations, and analysis of primary and secondary sources (e.g., newspaper articles, photographs, official records). Sometimes a case study will also collect quantitative data .
The aim is to gain as thorough an understanding as possible of the case and its context.
In writing up the case study, you need to bring together all the relevant aspects to give as complete a picture as possible of the subject.
How you report your findings depends on the type of research you are doing. Some case studies are structured like a standard scientific paper or thesis, with separate sections or chapters for the methods , results , and discussion .
Others are written in a more narrative style, aiming to explore the case from various angles and analyse its meanings and implications (for example, by using textual analysis or discourse analysis ).
In all cases, though, make sure to give contextual details about the case, connect it back to the literature and theory, and discuss how it fits into wider patterns or debates.
Cite this Scribbr article
If you want to cite this source, you can copy and paste the citation or click the ‘Cite this Scribbr article’ button to automatically add the citation to our free Reference Generator.
McCombes, S. (2023, January 30). Case Study | Definition, Examples & Methods. Scribbr. Retrieved 14 May 2024, from https://www.scribbr.co.uk/research-methods/case-studies/
Is this article helpful?
Shona McCombes
Other students also liked, correlational research | guide, design & examples, a quick guide to experimental design | 5 steps & examples, descriptive research design | definition, methods & examples.
Qualitative case study data analysis: an example from practice
Affiliation.
- 1 School of Nursing and Midwifery, National University of Ireland, Galway, Republic of Ireland.
- PMID: 25976531
- DOI: 10.7748/nr.22.5.8.e1307
Aim: To illustrate an approach to data analysis in qualitative case study methodology.
Background: There is often little detail in case study research about how data were analysed. However, it is important that comprehensive analysis procedures are used because there are often large sets of data from multiple sources of evidence. Furthermore, the ability to describe in detail how the analysis was conducted ensures rigour in reporting qualitative research.
Data sources: The research example used is a multiple case study that explored the role of the clinical skills laboratory in preparing students for the real world of practice. Data analysis was conducted using a framework guided by the four stages of analysis outlined by Morse ( 1994 ): comprehending, synthesising, theorising and recontextualising. The specific strategies for analysis in these stages centred on the work of Miles and Huberman ( 1994 ), which has been successfully used in case study research. The data were managed using NVivo software.
Review methods: Literature examining qualitative data analysis was reviewed and strategies illustrated by the case study example provided. Discussion Each stage of the analysis framework is described with illustration from the research example for the purpose of highlighting the benefits of a systematic approach to handling large data sets from multiple sources.
Conclusion: By providing an example of how each stage of the analysis was conducted, it is hoped that researchers will be able to consider the benefits of such an approach to their own case study analysis.
Implications for research/practice: This paper illustrates specific strategies that can be employed when conducting data analysis in case study research and other qualitative research designs.
Keywords: Case study data analysis; case study research methodology; clinical skills research; qualitative case study methodology; qualitative data analysis; qualitative research.
- Case-Control Studies*
- Data Interpretation, Statistical*
- Nursing Research / methods*
- Qualitative Research*
- Research Design
Case Study Research in Software Engineering: Guidelines and Examples by Per Runeson, Martin Höst, Austen Rainer, Björn Regnell
Get full access to Case Study Research in Software Engineering: Guidelines and Examples and 60K+ other titles, with a free 10-day trial of O'Reilly.
There are also live events, courses curated by job role, and more.
DATA ANALYSIS AND INTERPRETATION
5.1 introduction.
Once data has been collected the focus shifts to analysis of data. It can be said that in this phase, data is used to understand what actually has happened in the studied case, and where the researcher understands the details of the case and seeks patterns in the data. This means that there inevitably is some analysis going on also in the data collection phase where the data is studied, and for example when data from an interview is transcribed. The understandings in the earlier phases are of course also valid and important, but this chapter is more focusing on the separate phase that starts after the data has been collected.
Data analysis is conducted differently for quantitative and qualitative data. Sections 5.2 – 5.5 describe how to analyze qualitative data and how to assess the validity of this type of analysis. In Section 5.6 , a short introduction to quantitative analysis methods is given. Since quantitative analysis is covered extensively in textbooks on statistical analysis, and case study research to a large extent relies on qualitative data, this section is kept short.
5.2 ANALYSIS OF DATA IN FLEXIBLE RESEARCH
5.2.1 introduction.
As case study research is a flexible research method, qualitative data analysis methods are commonly used [176]. The basic objective of the analysis is, as in any other analysis, to derive conclusions from the data, keeping a clear chain of evidence. The chain of evidence means that a reader ...
Get Case Study Research in Software Engineering: Guidelines and Examples now with the O’Reilly learning platform.
O’Reilly members experience books, live events, courses curated by job role, and more from O’Reilly and nearly 200 top publishers.
Don’t leave empty-handed
Get Mark Richards’s Software Architecture Patterns ebook to better understand how to design components—and how they should interact.
It’s yours, free.
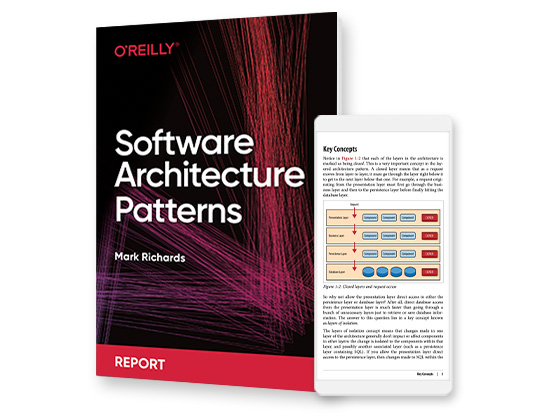
Check it out now on O’Reilly
Dive in for free with a 10-day trial of the O’Reilly learning platform—then explore all the other resources our members count on to build skills and solve problems every day.
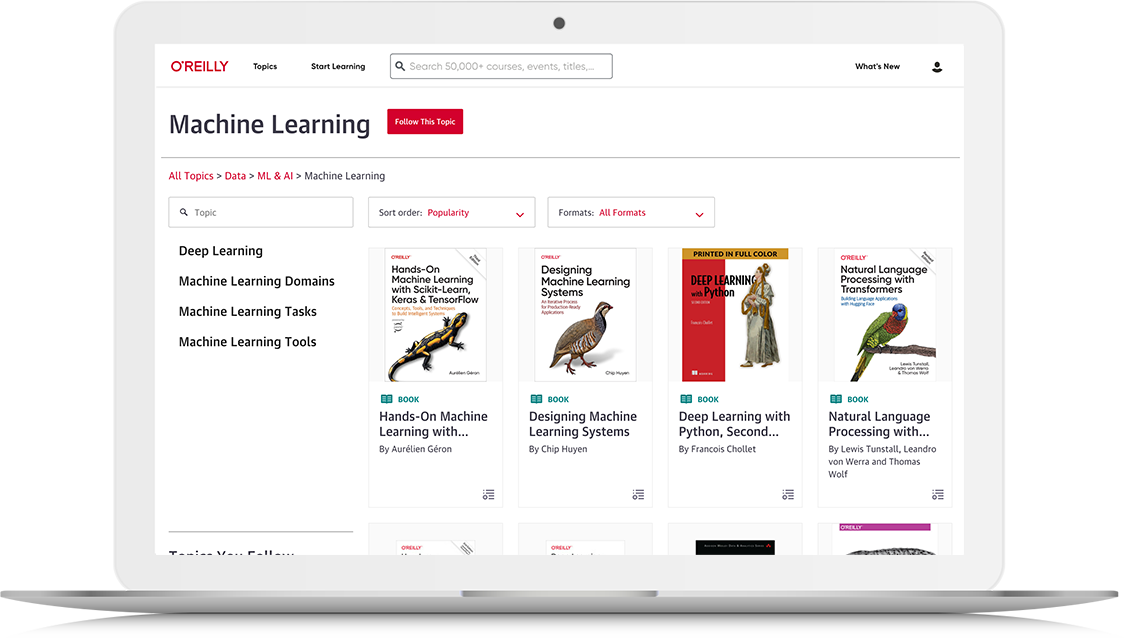
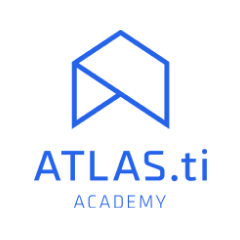
The Ultimate Guide to Qualitative Research - Part 1: The Basics
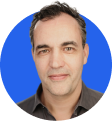
- Introduction and overview
- What is qualitative research?
- What is qualitative data?
- Examples of qualitative data
- Qualitative vs. quantitative research
- Mixed methods
- Qualitative research preparation
- Theoretical perspective
- Theoretical framework
- Literature reviews
Research question
- Conceptual framework
- Conceptual vs. theoretical framework
Data collection
- Qualitative research methods
- Focus groups
- Observational research

What is a case study?
Applications for case study research, what is a good case study, process of case study design, benefits and limitations of case studies.
- Ethnographical research
- Ethical considerations
- Confidentiality and privacy
- Power dynamics
- Reflexivity
Case studies
Case studies are essential to qualitative research , offering a lens through which researchers can investigate complex phenomena within their real-life contexts. This chapter explores the concept, purpose, applications, examples, and types of case studies and provides guidance on how to conduct case study research effectively.
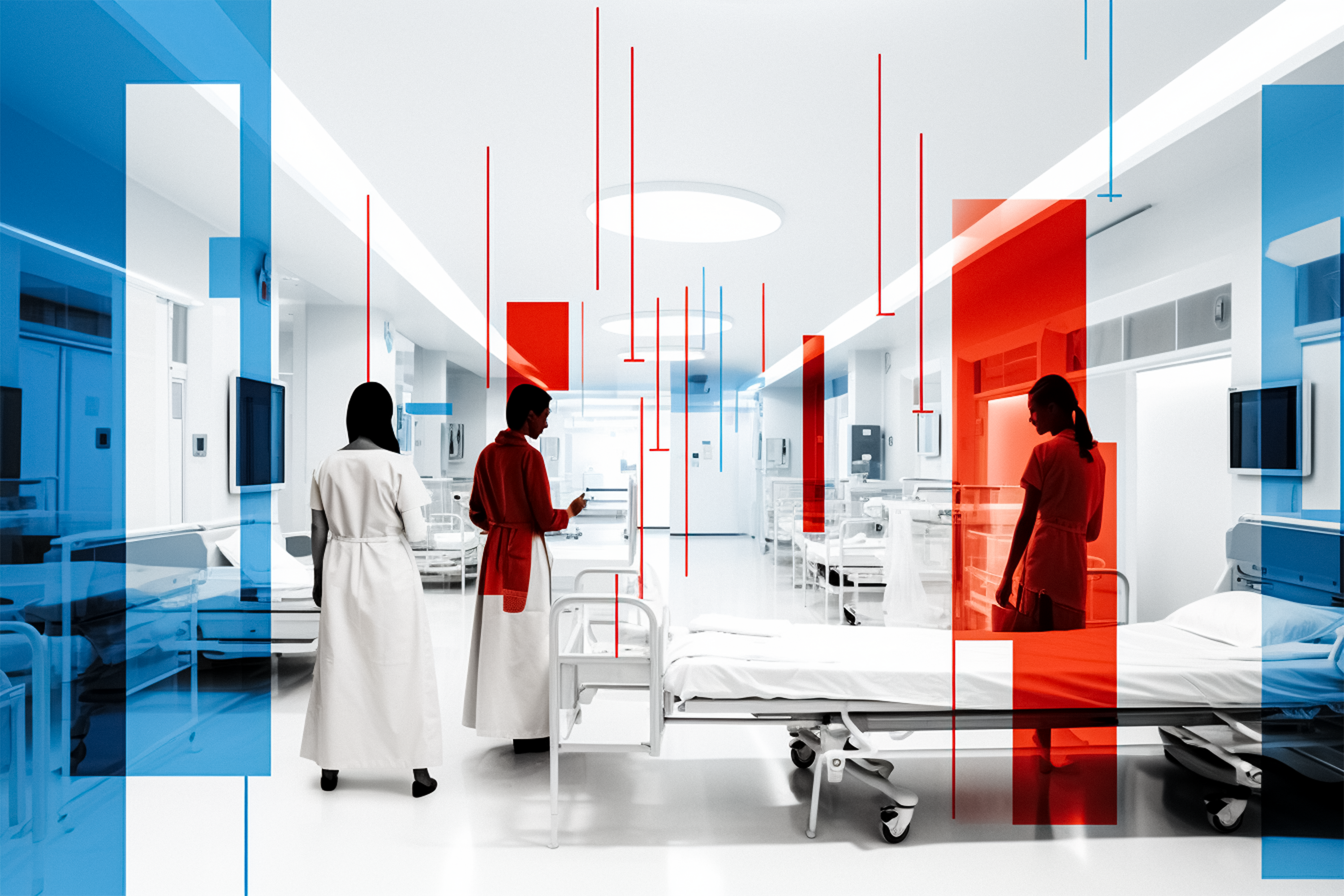
Whereas quantitative methods look at phenomena at scale, case study research looks at a concept or phenomenon in considerable detail. While analyzing a single case can help understand one perspective regarding the object of research inquiry, analyzing multiple cases can help obtain a more holistic sense of the topic or issue. Let's provide a basic definition of a case study, then explore its characteristics and role in the qualitative research process.
Definition of a case study
A case study in qualitative research is a strategy of inquiry that involves an in-depth investigation of a phenomenon within its real-world context. It provides researchers with the opportunity to acquire an in-depth understanding of intricate details that might not be as apparent or accessible through other methods of research. The specific case or cases being studied can be a single person, group, or organization – demarcating what constitutes a relevant case worth studying depends on the researcher and their research question .
Among qualitative research methods , a case study relies on multiple sources of evidence, such as documents, artifacts, interviews , or observations , to present a complete and nuanced understanding of the phenomenon under investigation. The objective is to illuminate the readers' understanding of the phenomenon beyond its abstract statistical or theoretical explanations.
Characteristics of case studies
Case studies typically possess a number of distinct characteristics that set them apart from other research methods. These characteristics include a focus on holistic description and explanation, flexibility in the design and data collection methods, reliance on multiple sources of evidence, and emphasis on the context in which the phenomenon occurs.
Furthermore, case studies can often involve a longitudinal examination of the case, meaning they study the case over a period of time. These characteristics allow case studies to yield comprehensive, in-depth, and richly contextualized insights about the phenomenon of interest.
The role of case studies in research
Case studies hold a unique position in the broader landscape of research methods aimed at theory development. They are instrumental when the primary research interest is to gain an intensive, detailed understanding of a phenomenon in its real-life context.
In addition, case studies can serve different purposes within research - they can be used for exploratory, descriptive, or explanatory purposes, depending on the research question and objectives. This flexibility and depth make case studies a valuable tool in the toolkit of qualitative researchers.
Remember, a well-conducted case study can offer a rich, insightful contribution to both academic and practical knowledge through theory development or theory verification, thus enhancing our understanding of complex phenomena in their real-world contexts.
What is the purpose of a case study?
Case study research aims for a more comprehensive understanding of phenomena, requiring various research methods to gather information for qualitative analysis . Ultimately, a case study can allow the researcher to gain insight into a particular object of inquiry and develop a theoretical framework relevant to the research inquiry.
Why use case studies in qualitative research?
Using case studies as a research strategy depends mainly on the nature of the research question and the researcher's access to the data.
Conducting case study research provides a level of detail and contextual richness that other research methods might not offer. They are beneficial when there's a need to understand complex social phenomena within their natural contexts.
The explanatory, exploratory, and descriptive roles of case studies
Case studies can take on various roles depending on the research objectives. They can be exploratory when the research aims to discover new phenomena or define new research questions; they are descriptive when the objective is to depict a phenomenon within its context in a detailed manner; and they can be explanatory if the goal is to understand specific relationships within the studied context. Thus, the versatility of case studies allows researchers to approach their topic from different angles, offering multiple ways to uncover and interpret the data .
The impact of case studies on knowledge development
Case studies play a significant role in knowledge development across various disciplines. Analysis of cases provides an avenue for researchers to explore phenomena within their context based on the collected data.
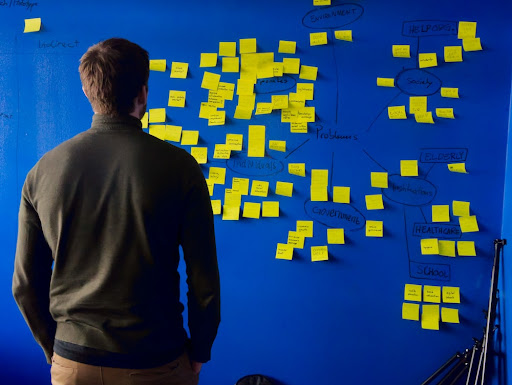
This can result in the production of rich, practical insights that can be instrumental in both theory-building and practice. Case studies allow researchers to delve into the intricacies and complexities of real-life situations, uncovering insights that might otherwise remain hidden.
Types of case studies
In qualitative research , a case study is not a one-size-fits-all approach. Depending on the nature of the research question and the specific objectives of the study, researchers might choose to use different types of case studies. These types differ in their focus, methodology, and the level of detail they provide about the phenomenon under investigation.
Understanding these types is crucial for selecting the most appropriate approach for your research project and effectively achieving your research goals. Let's briefly look at the main types of case studies.
Exploratory case studies
Exploratory case studies are typically conducted to develop a theory or framework around an understudied phenomenon. They can also serve as a precursor to a larger-scale research project. Exploratory case studies are useful when a researcher wants to identify the key issues or questions which can spur more extensive study or be used to develop propositions for further research. These case studies are characterized by flexibility, allowing researchers to explore various aspects of a phenomenon as they emerge, which can also form the foundation for subsequent studies.
Descriptive case studies
Descriptive case studies aim to provide a complete and accurate representation of a phenomenon or event within its context. These case studies are often based on an established theoretical framework, which guides how data is collected and analyzed. The researcher is concerned with describing the phenomenon in detail, as it occurs naturally, without trying to influence or manipulate it.
Explanatory case studies
Explanatory case studies are focused on explanation - they seek to clarify how or why certain phenomena occur. Often used in complex, real-life situations, they can be particularly valuable in clarifying causal relationships among concepts and understanding the interplay between different factors within a specific context.
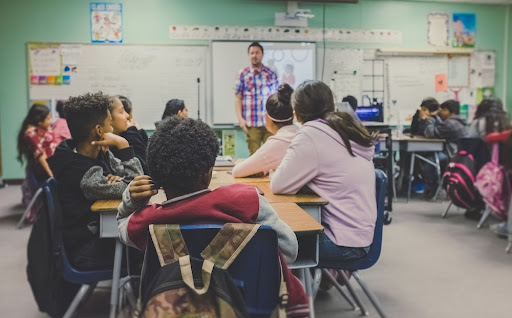
Intrinsic, instrumental, and collective case studies
These three categories of case studies focus on the nature and purpose of the study. An intrinsic case study is conducted when a researcher has an inherent interest in the case itself. Instrumental case studies are employed when the case is used to provide insight into a particular issue or phenomenon. A collective case study, on the other hand, involves studying multiple cases simultaneously to investigate some general phenomena.
Each type of case study serves a different purpose and has its own strengths and challenges. The selection of the type should be guided by the research question and objectives, as well as the context and constraints of the research.
The flexibility, depth, and contextual richness offered by case studies make this approach an excellent research method for various fields of study. They enable researchers to investigate real-world phenomena within their specific contexts, capturing nuances that other research methods might miss. Across numerous fields, case studies provide valuable insights into complex issues.
Critical information systems research
Case studies provide a detailed understanding of the role and impact of information systems in different contexts. They offer a platform to explore how information systems are designed, implemented, and used and how they interact with various social, economic, and political factors. Case studies in this field often focus on examining the intricate relationship between technology, organizational processes, and user behavior, helping to uncover insights that can inform better system design and implementation.
Health research
Health research is another field where case studies are highly valuable. They offer a way to explore patient experiences, healthcare delivery processes, and the impact of various interventions in a real-world context.
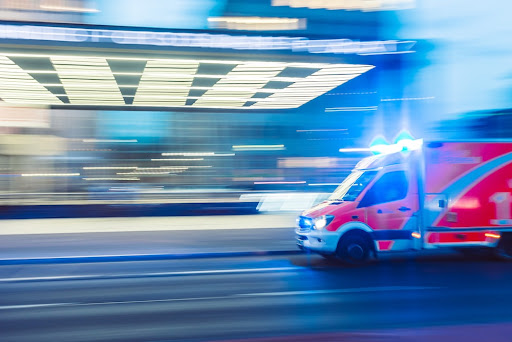
Case studies can provide a deep understanding of a patient's journey, giving insights into the intricacies of disease progression, treatment effects, and the psychosocial aspects of health and illness.
Asthma research studies
Specifically within medical research, studies on asthma often employ case studies to explore the individual and environmental factors that influence asthma development, management, and outcomes. A case study can provide rich, detailed data about individual patients' experiences, from the triggers and symptoms they experience to the effectiveness of various management strategies. This can be crucial for developing patient-centered asthma care approaches.
Other fields
Apart from the fields mentioned, case studies are also extensively used in business and management research, education research, and political sciences, among many others. They provide an opportunity to delve into the intricacies of real-world situations, allowing for a comprehensive understanding of various phenomena.
Case studies, with their depth and contextual focus, offer unique insights across these varied fields. They allow researchers to illuminate the complexities of real-life situations, contributing to both theory and practice.
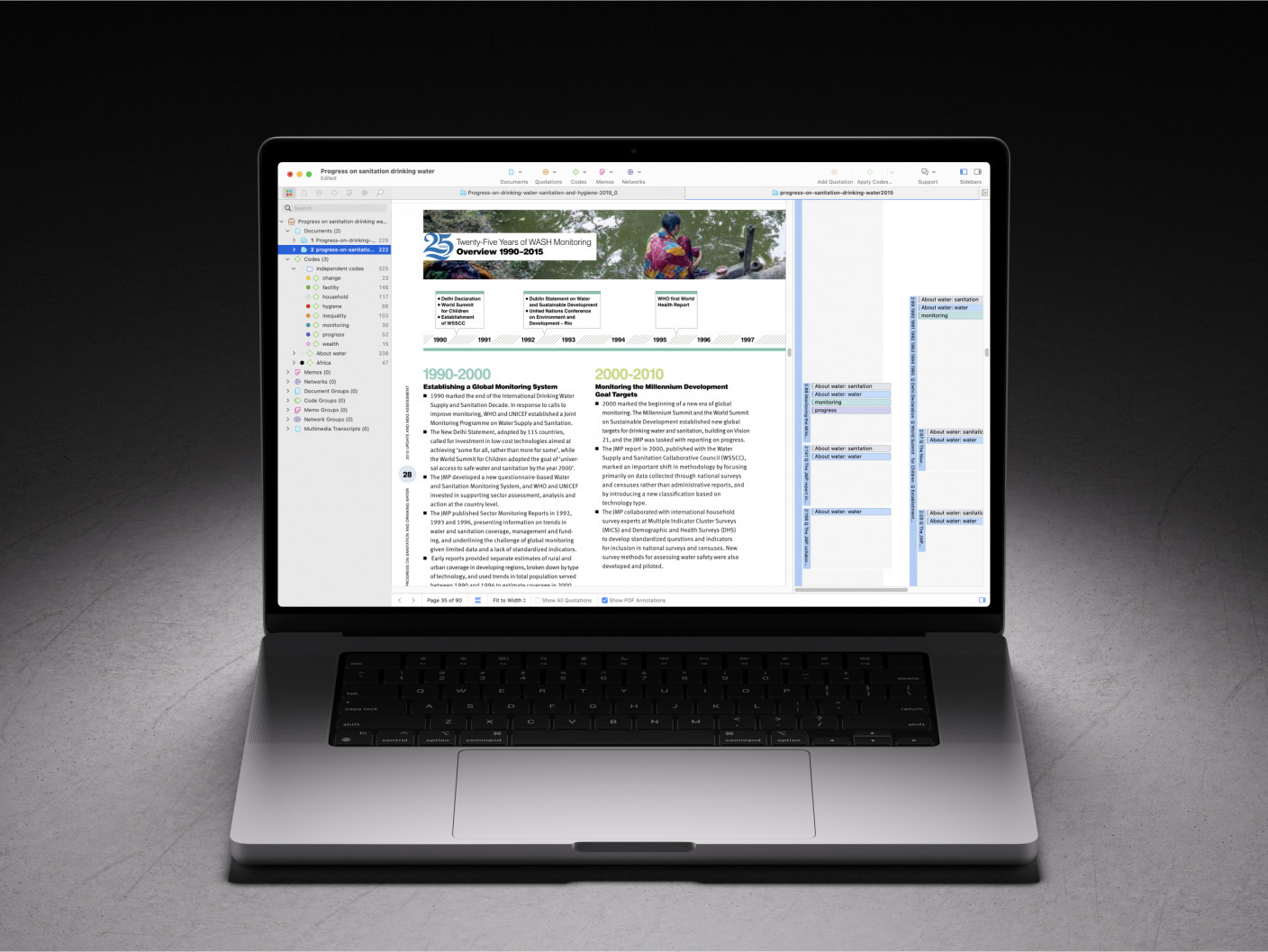
Whatever field you're in, ATLAS.ti puts your data to work for you
Download a free trial of ATLAS.ti to turn your data into insights.
Understanding the key elements of case study design is crucial for conducting rigorous and impactful case study research. A well-structured design guides the researcher through the process, ensuring that the study is methodologically sound and its findings are reliable and valid. The main elements of case study design include the research question , propositions, units of analysis, and the logic linking the data to the propositions.
The research question is the foundation of any research study. A good research question guides the direction of the study and informs the selection of the case, the methods of collecting data, and the analysis techniques. A well-formulated research question in case study research is typically clear, focused, and complex enough to merit further detailed examination of the relevant case(s).
Propositions
Propositions, though not necessary in every case study, provide a direction by stating what we might expect to find in the data collected. They guide how data is collected and analyzed by helping researchers focus on specific aspects of the case. They are particularly important in explanatory case studies, which seek to understand the relationships among concepts within the studied phenomenon.
Units of analysis
The unit of analysis refers to the case, or the main entity or entities that are being analyzed in the study. In case study research, the unit of analysis can be an individual, a group, an organization, a decision, an event, or even a time period. It's crucial to clearly define the unit of analysis, as it shapes the qualitative data analysis process by allowing the researcher to analyze a particular case and synthesize analysis across multiple case studies to draw conclusions.
Argumentation
This refers to the inferential model that allows researchers to draw conclusions from the data. The researcher needs to ensure that there is a clear link between the data, the propositions (if any), and the conclusions drawn. This argumentation is what enables the researcher to make valid and credible inferences about the phenomenon under study.
Understanding and carefully considering these elements in the design phase of a case study can significantly enhance the quality of the research. It can help ensure that the study is methodologically sound and its findings contribute meaningful insights about the case.
Ready to jumpstart your research with ATLAS.ti?
Conceptualize your research project with our intuitive data analysis interface. Download a free trial today.
Conducting a case study involves several steps, from defining the research question and selecting the case to collecting and analyzing data . This section outlines these key stages, providing a practical guide on how to conduct case study research.
Defining the research question
The first step in case study research is defining a clear, focused research question. This question should guide the entire research process, from case selection to analysis. It's crucial to ensure that the research question is suitable for a case study approach. Typically, such questions are exploratory or descriptive in nature and focus on understanding a phenomenon within its real-life context.
Selecting and defining the case
The selection of the case should be based on the research question and the objectives of the study. It involves choosing a unique example or a set of examples that provide rich, in-depth data about the phenomenon under investigation. After selecting the case, it's crucial to define it clearly, setting the boundaries of the case, including the time period and the specific context.
Previous research can help guide the case study design. When considering a case study, an example of a case could be taken from previous case study research and used to define cases in a new research inquiry. Considering recently published examples can help understand how to select and define cases effectively.
Developing a detailed case study protocol
A case study protocol outlines the procedures and general rules to be followed during the case study. This includes the data collection methods to be used, the sources of data, and the procedures for analysis. Having a detailed case study protocol ensures consistency and reliability in the study.
The protocol should also consider how to work with the people involved in the research context to grant the research team access to collecting data. As mentioned in previous sections of this guide, establishing rapport is an essential component of qualitative research as it shapes the overall potential for collecting and analyzing data.
Collecting data
Gathering data in case study research often involves multiple sources of evidence, including documents, archival records, interviews, observations, and physical artifacts. This allows for a comprehensive understanding of the case. The process for gathering data should be systematic and carefully documented to ensure the reliability and validity of the study.
Analyzing and interpreting data
The next step is analyzing the data. This involves organizing the data , categorizing it into themes or patterns , and interpreting these patterns to answer the research question. The analysis might also involve comparing the findings with prior research or theoretical propositions.
Writing the case study report
The final step is writing the case study report . This should provide a detailed description of the case, the data, the analysis process, and the findings. The report should be clear, organized, and carefully written to ensure that the reader can understand the case and the conclusions drawn from it.
Each of these steps is crucial in ensuring that the case study research is rigorous, reliable, and provides valuable insights about the case.
The type, depth, and quality of data in your study can significantly influence the validity and utility of the study. In case study research, data is usually collected from multiple sources to provide a comprehensive and nuanced understanding of the case. This section will outline the various methods of collecting data used in case study research and discuss considerations for ensuring the quality of the data.
Interviews are a common method of gathering data in case study research. They can provide rich, in-depth data about the perspectives, experiences, and interpretations of the individuals involved in the case. Interviews can be structured , semi-structured , or unstructured , depending on the research question and the degree of flexibility needed.
Observations
Observations involve the researcher observing the case in its natural setting, providing first-hand information about the case and its context. Observations can provide data that might not be revealed in interviews or documents, such as non-verbal cues or contextual information.
Documents and artifacts
Documents and archival records provide a valuable source of data in case study research. They can include reports, letters, memos, meeting minutes, email correspondence, and various public and private documents related to the case.
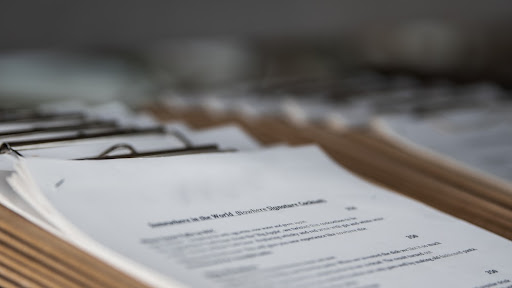
These records can provide historical context, corroborate evidence from other sources, and offer insights into the case that might not be apparent from interviews or observations.
Physical artifacts refer to any physical evidence related to the case, such as tools, products, or physical environments. These artifacts can provide tangible insights into the case, complementing the data gathered from other sources.
Ensuring the quality of data collection
Determining the quality of data in case study research requires careful planning and execution. It's crucial to ensure that the data is reliable, accurate, and relevant to the research question. This involves selecting appropriate methods of collecting data, properly training interviewers or observers, and systematically recording and storing the data. It also includes considering ethical issues related to collecting and handling data, such as obtaining informed consent and ensuring the privacy and confidentiality of the participants.
Data analysis
Analyzing case study research involves making sense of the rich, detailed data to answer the research question. This process can be challenging due to the volume and complexity of case study data. However, a systematic and rigorous approach to analysis can ensure that the findings are credible and meaningful. This section outlines the main steps and considerations in analyzing data in case study research.
Organizing the data
The first step in the analysis is organizing the data. This involves sorting the data into manageable sections, often according to the data source or the theme. This step can also involve transcribing interviews, digitizing physical artifacts, or organizing observational data.
Categorizing and coding the data
Once the data is organized, the next step is to categorize or code the data. This involves identifying common themes, patterns, or concepts in the data and assigning codes to relevant data segments. Coding can be done manually or with the help of software tools, and in either case, qualitative analysis software can greatly facilitate the entire coding process. Coding helps to reduce the data to a set of themes or categories that can be more easily analyzed.
Identifying patterns and themes
After coding the data, the researcher looks for patterns or themes in the coded data. This involves comparing and contrasting the codes and looking for relationships or patterns among them. The identified patterns and themes should help answer the research question.
Interpreting the data
Once patterns and themes have been identified, the next step is to interpret these findings. This involves explaining what the patterns or themes mean in the context of the research question and the case. This interpretation should be grounded in the data, but it can also involve drawing on theoretical concepts or prior research.
Verification of the data
The last step in the analysis is verification. This involves checking the accuracy and consistency of the analysis process and confirming that the findings are supported by the data. This can involve re-checking the original data, checking the consistency of codes, or seeking feedback from research participants or peers.
Like any research method , case study research has its strengths and limitations. Researchers must be aware of these, as they can influence the design, conduct, and interpretation of the study.
Understanding the strengths and limitations of case study research can also guide researchers in deciding whether this approach is suitable for their research question . This section outlines some of the key strengths and limitations of case study research.
Benefits include the following:
- Rich, detailed data: One of the main strengths of case study research is that it can generate rich, detailed data about the case. This can provide a deep understanding of the case and its context, which can be valuable in exploring complex phenomena.
- Flexibility: Case study research is flexible in terms of design , data collection , and analysis . A sufficient degree of flexibility allows the researcher to adapt the study according to the case and the emerging findings.
- Real-world context: Case study research involves studying the case in its real-world context, which can provide valuable insights into the interplay between the case and its context.
- Multiple sources of evidence: Case study research often involves collecting data from multiple sources , which can enhance the robustness and validity of the findings.
On the other hand, researchers should consider the following limitations:
- Generalizability: A common criticism of case study research is that its findings might not be generalizable to other cases due to the specificity and uniqueness of each case.
- Time and resource intensive: Case study research can be time and resource intensive due to the depth of the investigation and the amount of collected data.
- Complexity of analysis: The rich, detailed data generated in case study research can make analyzing the data challenging.
- Subjectivity: Given the nature of case study research, there may be a higher degree of subjectivity in interpreting the data , so researchers need to reflect on this and transparently convey to audiences how the research was conducted.
Being aware of these strengths and limitations can help researchers design and conduct case study research effectively and interpret and report the findings appropriately.
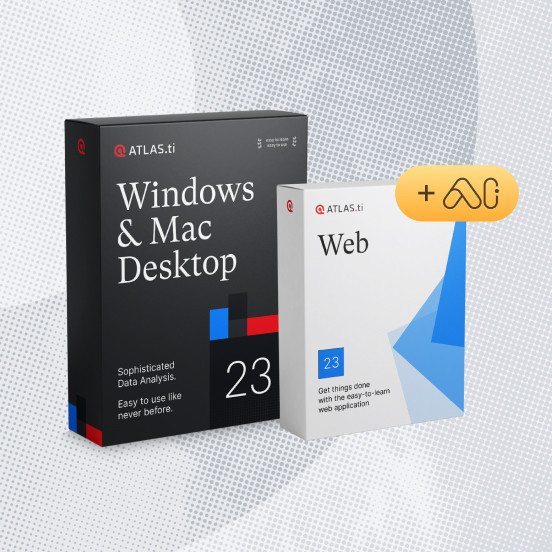
Ready to analyze your data with ATLAS.ti?
See how our intuitive software can draw key insights from your data with a free trial today.

- SUGGESTED TOPICS
- The Magazine
- Newsletters
- Managing Yourself
- Managing Teams
- Work-life Balance
- The Big Idea
- Data & Visuals
- Reading Lists
- Case Selections
- HBR Learning
- Topic Feeds
- Account Settings
- Email Preferences
What the Case Study Method Really Teaches
- Nitin Nohria

Seven meta-skills that stick even if the cases fade from memory.
It’s been 100 years since Harvard Business School began using the case study method. Beyond teaching specific subject matter, the case study method excels in instilling meta-skills in students. This article explains the importance of seven such skills: preparation, discernment, bias recognition, judgement, collaboration, curiosity, and self-confidence.
During my decade as dean of Harvard Business School, I spent hundreds of hours talking with our alumni. To enliven these conversations, I relied on a favorite question: “What was the most important thing you learned from your time in our MBA program?”
- Nitin Nohria is the George F. Baker Professor of Business Administration, Distinguished University Service Professor, and former dean of Harvard Business School.
Partner Center

An official website of the United States government
The .gov means it’s official. Federal government websites often end in .gov or .mil. Before sharing sensitive information, make sure you’re on a federal government site.
The site is secure. The https:// ensures that you are connecting to the official website and that any information you provide is encrypted and transmitted securely.
- Publications
- Account settings
Preview improvements coming to the PMC website in October 2024. Learn More or Try it out now .
- Advanced Search
- Journal List
- Ann Indian Acad Neurol
- v.16(4); Oct-Dec 2013
Design and data analysis case-controlled study in clinical research
Sanjeev v. thomas.
Department of Neurology, Sree Chitra Tirunal Institute for Medical Sciences and Technology, Trivandrum, Kerala, India
Karthik Suresh
1 Department of Pulmonary and Critical Care Medicine, Johns Hopkins University School of Medicine, Louiseville, USA
Geetha Suresh
2 Department of Justice Administration, University of Louisville, Louiseville, USA
Clinicians during their training period and practice are often called upon to conduct studies to explore the association between certain exposures and disease states or interventions and outcomes. More often they need to interpret the results of research data published in the medical literature. Case-control studies are one of the most frequently used study designs for these purposes. This paper explains basic features of case control studies, rationality behind applying case control design with appropriate examples and limitations of this design. Analysis of sensitivity and specificity along with template to calculate various ratios are explained with user friendly tables and calculations in this article. The interpretation of some of the laboratory results requires sound knowledge of the various risk ratios and positive or negative predictive values for correct identification for unbiased analysis. A major advantage of case-control study is that they are small and retrospective and so they are economical than cohort studies and randomized controlled trials.
Introduction
Clinicians think of case-control study when they want to ascertain association between one clinical condition and an exposure or when a researcher wants to compare patients with disease exposed to the risk factors to non-exposed control group. In other words, case-control study compares subjects who have disease or outcome (cases) with subjects who do not have the disease or outcome (controls). Historically, case control studies came into fashion in the early 20 th century, when great interest arose in the role of environmental factors (such as pipe smoke) in the pathogenesis of disease. In the 1950s, case control studies were used to link cigarette smoke and lung cancer. Case-control studies look back in time to compare “what happened” in each group to determine the relationship between the risk factor and disease. The case-control study has important advantages, including cost and ease of deployment. However, it is important to note that a positive relationship between exposure and disease does not imply causality.
At the center of the case-control study is a collection of cases. [ Figure 1 ] This explains why this type of study is often used to study rare diseases, where the prevalence of the disease may not be high enough to permit for a cohort study. A cohort study identifies patients with and without an exposure and then “looks forward” to see whether or not greater numbers of patients with an exposure develop disease.

Comparison of cohort and case control studies
For instance, Yang et al . studied antiepileptic drug (AED) associated rashes in Asians in a case-control study.[ 1 ] They collected cases of confirmed anti-epileptic induced severe cutaneous reactions (such as Stevens Johnson syndrome) and then, using appropriate controls, analyzed various exposures (including type of [AED] used) to look for risk factors to developing AED induced skin disease.
Choosing controls is very important aspect of case-control study design. The investigator must weigh the need for the controls to be relevant against the tendency to over match controls such that potential differences may become muted. In general, one may consider three populations: Cases, the relevant control population and the population at large. For the study above, the cases include patients with AED skin disease. In this case, the relevant control population is a group of Asian patients without skin disease. It is important for controls to be relevant: In the anti-epileptic study, it would not be appropriate to choose a population across ethnicities since one of the premises of the paper revolves around particularly susceptibility to AED drug rashes in Asian populations.
One popular method of choosing controls is to choose patients from a geographic population at large. In studying the relationship between non-steroidal anti-inflammatory drugs and Parkinson's disease (PD), Wahner et al . chose a control population from several rural California counties.[ 2 ] There are other methods of choosing controls (using patients without disease admitted to the hospital during the time of study, neighbors of disease positive cases, using mail routes to identify disease negative cases). However, one must be careful not to introduce bias into control selection. For instance, a study that enrolls cases from a clinic population should not use a hospital population as control. Studies looking at geography specific population (e.g., Neurocysticercosis in India) cannot use controls from large studies done in other populations (registries of patients from countries where disease prevalence may be drastically different than in India). In general, geographic clustering is probably the easiest way to choose controls for case-control studies.
Two popular ways of choosing controls include hospitalized patients and patients from the general population. Choosing hospitalized, disease negative patients offers several advantages, including good rates of response (patients admitted to the hospital are generally already being examined and evaluated and often tend to be available to further questioning for a study, compared with the general population, where rates of response may be much lower) and possibly less amnestic bias (patients who are already in the hospital are, by default, being asked to remember details of their presenting illnesses and as such, may more reliably remember details of exposures). However, using hospitalized patients has one large disadvantage; these patients have higher severity of disease since they required hospitalization in the first place. In addition, patients may be hospitalized for disease processes that may share features with diseases under study, thus confounding results.
Using a general population offers the advantage of being a true control group, random in its choosing and without any common features that may confound associations. However, disadvantages include poor response rates and biasing based on geography. Administering long histories and questions regarding exposures are often hard to accomplish in the general population due to the number of people willing (or rather, not willing) to undergo testing. In addition, choosing cases from the general population from particular geographic areas may bias the population toward certain characteristics (such as a socio-economic status) of that geographic population. Consider a study that uses cases from a referral clinic population that draws patients from across socio-economic strata. Using a control group selected from a population from a very affluent or very impoverished area may be problematic unless the socio-economic status is included in the final analysis.
In case-controls studies, cases are usually available before controls. When studying specific diseases, cases are often collected from specialty clinics that see large numbers of patients with a specific disease. Consider for example, the study by Garwood et al .[ 3 ] which looked at patients with established PD and looked for associations between prior amphetamine use and subsequent development various neurologic disorders. Patients in this study were chosen from specialty clinics that see large numbers of patients with certain neurologic disorders. Case definitions are very important when planning to choose cases. For instance, in a hypothetical study aiming to study cases of peripheral neuropathy, will all patients who carry a diagnosis of peripheral neuropathy be included? Or, will only patients with definite electromyography evidence of neuropathy be included? If a disease process with known histopathology is being studied, will tissue diagnosis be required for all cases? More stringent case definitions that require multiple pieces of data to be present may limit the number of cases that can be used in the study. Less stringent criteria (for instance, counting all patients with the diagnosis of “peripheral neuropathy” listed in the chart) may inadvertently choose a group of cases that are too heterogeneous.
The disease history status of the chosen cases must also be decided. Will the cases being chosen have newly diagnosed disease, or will cases of ongoing/longstanding disease also be included? Will decedent cases be included? This is important when looking at exposures in the following fashion: Consider exposure X that is associated with disease Y. Suppose that exposure X negatively affects disease Y such that patients that are X + have more severe disease. Now, a case-control study that used only patients with long-standing or ongoing disease might miss a potential association between X and Y because X + patients, due to their more aggressive course of disease, are no longer alive and therefore were not included in the analysis. If this particular confounding effect is of concern, it can be circumvented by using incident cases only.
Selection bias occurs when the exposure of interest results in more careful screening of a population, thus mimicking an association. The classic example of this phenomenon was noted in the 70s, when certain studies noted a relationship between estrogen use and endometrial cancer. However, on close analysis, it was noted that patients who used estrogen were more likely to experience vaginal bleeding, which in turn is often a cause for close examination by physicians to rule out endometrial cancer. This is often seen with certain drug exposures as well. A drug may produce various symptoms, which lead to closer physician evaluation, thus leading to more disease positive cases. Thus, when analyzed in a retrospective fashion, more of the cases may have a particular exposure only insofar as that particular exposure led to evaluations that resulted in a diagnosis, but without any direct association or causality between the exposure and disease.
One advantage of case-control studies is the ability to study multiple exposures and other risk factors within one study. In addition, the “exposure” being studied can be biochemical in nature. Consider the study, which looked at a genetic variant of a kinase enzyme as a risk factor for development of Alzheimer's disease.[ 4 ] Compare this with the study mentioned earlier by Garwood et al .,[ 3 ] where exposure data was collected by surveys and questionnaires. In this study, the authors drew blood work on cases and controls in order to assess their polymorphism status. Indeed, more than one exposure can be assessed in the same study and with planning, a researcher may look at several variables, including biochemical ones, in single case-control study.
Matching is one of three ways (along with exclusion and statistical adjustment) to adjust for differences. Matching attempts to make sure that the control group is sufficiently similar to the cases group, with respects to variables such as age, sex, etc., Cases and controls should not be matched on variables that will be analyzed for possible associations to disease. Not only should exposure variables not be included, but neither should variables that are closely related to these variables. Lastly, overmatching should be avoided. If the control group is too similar to the cases group, the study may fail to detect the difference even if one exists. In addition, adding matching categories increases expense of the study.
One measure of association derived from case control studies are sensitivity and specificity ratios. These measures are important to a researcher, to understand the correct classification. A good understanding of sensitivity and specificity is essential to understand receiver operating characteristic curve and in distinguishing correct classification of positive exposure and disease with negative exposure and no disease. Table 1 explains a hypothetical example and method of calculation of specificity and sensitivity analysis.
Hypothetical example of sensitivity, specificity and predictive values

Interpretation of sensitivity, specificity and predictive values
Sensitivity and specificity are statistical measures of the performance of a two by two classification of cases and controls (sick or healthy) against positives and negatives (exposed or non-exposed).[ 5 ] Sensitivity measures or identifies the proportion of actual positives identified as the percentage of sick people who are correctly identified as sick. Specificity measures or identifies the proportion of negatives identified as the percentage of healthy people who are correctly identified as healthy. Theoretically, optimum prediction aims at 100% sensitivity and specificity with a minimum of margin of error. Table 1 also shows false positive rate, which is referred to as Type I error commonly stated as α “Alpha” is calculated using the following formula: 100 − specificity, which is equal to 100 − 90.80 = 9.20% for Table 1 example. Type 1 error is also known as false positive error is referred to as a false alarm, indicates that a condition is present when it is actually not present. In the above mentioned example, a false positive error indicates the percent falsely identified healthy as sick. The reason why we want Type 1 error to be as minimum as possible is because healthy should not get treatment.
The false negative rate, which is referred to as Type II error commonly stated as β “Beta” is calculated using the following formula: 100 − sensitivity which is equal to 100 − 73.30 = 26.70% for Table 1 example. Type II error is also known as false negative error indicates that a condition is not present when it should have been present. In the above mentioned example, a false negative error indicates percent falsely identified sick as healthy. A Type 1 error unnecessarily treats a healthy, which in turn increases the budget and Type II error would risk the sick, which would act against study objectives. A researcher wants to minimize both errors, which not a simple issue because an effort to decrease one type of error increases the other type of error. The only way to minimize both type of error statistically is by increasing sample size, which may be difficult sometimes not feasible or expensive. If the sample size is too low it lacks precision and it is too large, time and resources will be wasted. Hence, the question is what should be the sample size so that the study has the power to generalize the result obtained from the study. The researcher has to decide whether, the study has enough power to make a judgment of the population from their sample. The researcher has to decide this issue in the process of designing an experiment, how large a sample is needed to enable reliable judgment.
Statistical power is same as sensitivity (73.30%). In this example, large number of false positives and few false negatives indicate the test conducted alone is not the best test to confirm the disease. Higher statistical power increase statistical significance by reducing Type 1 error which increases confidence interval. In other words, larger the power more accurately the study can mirror the behavior of the study population.
The positive predictive values (PPV) or the precision rate is referred to as the proportion of positive test results, which means correct diagnoses. If the test correctly identifies all positive conditions then the PPV would be 100% and negative predictive value (NPV) would be 0. The calculative PPV in Table 1 is 11.8%, which is not large enough to predict cases with test conducted alone. However, the NPV 99.9% indicates the test correctly identifies negative conditions.
Clinical interpretation of a test
In a sample, there are two groups those who have the disease and those who do not have the disease. A test designed to detect that disease can have two results a positive result that states that the disease is present and a negative result that states that the disease is absent. In an ideal situation, we would want the test to be positive for all persons who have the disease and test to be negative for all persons who do not have the disease. Unfortunately, reality is often far from ideal. The clinician who had ordered the test has the result as positive or negative. What conclusion can he or she make about the disease status for his patient? The first step would be to examine the reliability of the test in statistical terms. (1) What is the sensitivity of the test? (2) What is the specificity of the test? The second step is to examine it applicability to his patient. (3) What is the PPV of the test? (4) What is the NPV of the test?
Suppose the test result had come as positive. In this example the test has a sensitivity of 73.3% and specificity of 90.8%. This test is capable of detecting the disease status in 73% of cases only. It has a false positivity of 9.2%. The PPV of the test is 11.8%. In other words, there is a good possibility that the test result is false positive and the person does not have the disease. We need to look at other test results and the clinical situation. Suppose the PPV of this test was close to 80 or 90%, one could conclude that most likely the person has the disease state if the test result is positive.
Suppose the test result had come as negative. The NPV of this test is 99.9%, which means this test gave a negative result in a patient with the disease only very rarely. Hence, there is only 0.1% possibility that the person who tested negative has in fact the disease. Probably no further tests are required unless the clinical suspicion is very high.
It is very important how the clinician interprets the result of a test. The usefulness of a positive result or negative result depends upon the PPV or NPV of the test respectively. A screening test should have high sensitivity and high PPV. A confirmatory test should have high specificity and high NPV.
Case control method is most efficient, for the study of rare diseases and most common diseases. Other measures of association from case control studies are calculation of odds ratio (OR) and risk ratio which is presented in Table 2 .
Different ratio calculation templates with sample calculation

Absolute risk means the probability of an event occurring and are not compared with any other type of risk. Absolute risk is expressed as a ratio or percent. In the example, absolute risk reduction indicates 27.37% decline in risk. Relative risk (RR) on the other hand compares the risk among exposed and non-exposed. In the example provided in Table 2 , the non-exposed control group is 69.93% less likely compared to exposed cases. Reader should keep in mind that RR does not mean increase in risk. This means that while a 100% likely risk among those exposed cases, unexposed control is less likely by 69.93%. RR does not explain actual risk but is expressed as relative increase or decrease in risk of exposed compared to non-exposed.
OR help the researcher to conclude whether the odds of a certain event or outcome are same for two groups. It calculates the odds of a health outcome when exposed compared to non-exposed. In our example an OR of. 207 can be interpreted as the non-exposed group is less likely to experience the event compared to the exposed group. If the OR is greater than 1 (example 1.11) means that the exposed are 1.11 times more likely to be riskier than the non-exposed.
Event rate for cases (E) and controls (C) in biostatistics explains how event ratio is a measure of how often a particular statistical exposure results in occurrence of disease within the experimental group (cases) of an experiment. This value in our example is 11.76%. This value or percent explains the extent of risk to patients exposed, compared with the non-exposed.
The statistical tests that can be used for ascertain an association depends upon the variable characteristics also. If the researcher wants to find the association between two categorical variables (e.g., a positive versus negative test result and disease state expressed as present or absent), Cochran-Armitage test, which is same as Pearson Chi-squared test can be used. When the objective is to find the association between two interval or ratio level (continuous) variables, correlation and regression analysis can be performed. In order to evaluate statistical significant difference between the means of cases and control, a test of group difference can be performed. If the researcher wants to find statically significant difference among means of more than two groups, analysis of variance can be performed. A detailed explanation and how to calculate various statistical tests will be published in later issues. The success of the research directly and indirectly depends on how the following biases or systematic errors, are controlled.
When selecting cases and controls, based on exposed or not-exposed factors, the ability of subjects to recall information on exposure is collected retrospectively and often forms the basis for recall bias. Recall bias is a methodological issue. Problems of recall method are: Limitations in human ability to recall and cases may remember their exposure with more accuracy than the controls. Other possible bias is the selection bias. In case-control studies, the cases and controls are selected from the same inherited characteristics. For instance, cases collected from referral clinics often exposed to selection bias cases. If selection bias is not controlled, the findings of association, most likely may be due to of chance resulting from the study design. Another possible bias is information bias, which arises because of misclassification of the level of exposure or misclassification of disease or other symptoms of outcome itself.
Case control studies are good for studying rare diseases, but they are not generally used to study rare exposures. As Kaelin and Bayona explains[ 6 ] if a researcher want to study the risk of asthma from working in a nuclear submarine shipyard, a case control study may not be a best option because a very small proportion of people with asthma might be exposed. Similarly, case-control studies cannot be the best option to study multiple diseases or conditions because the selection of the control group may not be comparable for multiple disease or conditions selected. The major advantage of case-control study is that they are small and retrospective and so they are economical than cohort studies and randomized controlled trials.
Source of Support: Nil
Conflict of Interest: Nil

- eLibrary Home
- eLibrary Login
- < Previous
Home > CHAPTERS > ACIS > ACIS 2002 > 38
ACIS 2002 Proceedings
Four steps to analyse data from a case study method.
John Atkinson , Charles Sturt University
Four steps are proposed to assist the novice researcher analyse their data that has been collected using a case study method. The first step proposes the creation of a data repository using basic relational database theory. The second step involves creating codes to identify the respective ‘chunks’ of data. These resulting codes are then analysed and rationalised. The third step involves analysing the case study data by generating a variety of reports. The fourth step generates the final propositions by linking the rationalised codes back to the initial propositions and where appropriate new propositions are generated. The outcome of these steps is a series of propositions that reflect the nature of the data associated with the case studies data.
Recommended Citation
Atkinson, John, "Four Steps to Analyse Data from a Case Study Method" (2002). ACIS 2002 Proceedings . 38. https://aisel.aisnet.org/acis2002/38
Since August 21, 2008
Advanced Search
- Notify me via email or RSS
- ACIS 2002 Website
- All Content
Author Corner
- eLibrary FAQ
Home | About | FAQ | My Account | Accessibility Statement
Privacy Copyright
- Future Students
- Current Students
- Faculty/Staff

News and Media
- News & Media Home
- Research Stories
- School's In
- In the Media
You are here
70 years after brown v. board of education, new research shows rise in school segregation.

As the nation prepares to mark the 70th anniversary of the landmark U.S. Supreme Court ruling in Brown v. Board of Education , a new report from researchers at Stanford and USC shows that racial and economic segregation among schools has grown steadily in large school districts over the past three decades — an increase that appears to be driven in part by policies favoring school choice over integration.
Analyzing data from U.S. public schools going back to 1967, the researchers found that segregation between white and Black students has increased by 64 percent since 1988 in the 100 largest districts, and segregation by economic status has increased by about 50 percent since 1991.
The report also provides new evidence about the forces driving recent trends in school segregation, showing that the expansion of charter schools has played a major role.
The findings were released on May 6 with the launch of the Segregation Explorer , a new interactive website from the Educational Opportunity Project at Stanford University. The website provides searchable data on racial and economic school segregation in U.S. states, counties, metropolitan areas, and school districts from 1991 to 2022.
“School segregation levels are not at pre- Brown levels, but they are high and have been rising steadily since the late 1980s,” said Sean Reardon , the Professor of Poverty and Inequality in Education at Stanford Graduate School of Education and faculty director of the Educational Opportunity Project. “In most large districts, school segregation has increased while residential segregation and racial economic inequality have declined, and our findings indicate that policy choices – not demographic changes – are driving the increase.”
“There’s a tendency to attribute segregation in schools to segregation in neighborhoods,” said Ann Owens , a professor of sociology and public policy at USC. “But we’re finding that the story is more complicated than that.”
Assessing the rise
In the Brown v. Board decision issued on May 17, 1954, the U.S. Supreme Court ruled that racially segregated public schools violated the Equal Protection Clause of the Fourteenth Amendment and established that “separate but equal” schools were not only inherently unequal but unconstitutional. The ruling paved the way for future decisions that led to rapid school desegregation in many school districts in the late 1960s and early 1970s.
Though segregation in most school districts is much lower than it was 60 years ago, the researchers found that over the past three decades, both racial and economic segregation in large districts increased. Much of the increase in economic segregation since 1991, measured by segregation between students eligible and ineligible for free lunch, occurred in the last 15 years.
White-Hispanic and white-Asian segregation, while lower on average than white-Black segregation, have both more than doubled in large school districts since the 1980s.
Racial-economic segregation – specifically the difference in the proportion of free-lunch-eligible students between the average white and Black or Hispanic student’s schools – has increased by 70 percent since 1991.
School segregation is strongly associated with achievement gaps between racial and ethnic groups, especially the rate at which achievement gaps widen during school, the researchers said.
“Segregation appears to shape educational outcomes because it concentrates Black and Hispanic students in higher-poverty schools, which results in unequal learning opportunities,” said Reardon, who is also a senior fellow at the Stanford Institute for Economic Policy Research and a faculty affiliate of the Stanford Accelerator for Learning .
Policies shaping recent trends
The recent rise in school segregation appears to be the direct result of educational policy and legal decisions, the researchers said.
Both residential segregation and racial disparities in income declined between 1990 and 2020 in most large school districts. “Had nothing else changed, that trend would have led to lower school segregation,” said Owens.
But since 1991, roughly two-thirds of districts that were under court-ordered desegregation have been released from court oversight. Meanwhile, since 1998, the charter sector – a form of expanded school choice – has grown.
Expanding school choice could influence segregation levels in different ways: If families sought schools that were more diverse than the ones available in their neighborhood, it could reduce segregation. But the researchers found that in districts where the charter sector expanded most rapidly in the 2000s and 2010s, segregation grew the most.
The researchers’ analysis also quantified the extent to which the release from court orders accounted for the rise in school segregation. They found that, together, the release from court oversight and the expansion of choice accounted entirely for the rise in school segregation from 2000 to 2019.
The researchers noted enrollment policies that school districts can implement to mitigate segregation, such as voluntary integration programs, socioeconomic-based student assignment policies, and school choice policies that affirmatively promote integration.
“School segregation levels are high, troubling, and rising in large districts,” said Reardon. “These findings should sound an alarm for educators and policymakers.”
Additional collaborators on the project include Demetra Kalogrides, Thalia Tom, and Heewon Jang. This research, including the development of the Segregation Explorer data and website, was supported by the Russell Sage Foundation, the Robert Wood Johnson Foundation, and the Bill and Melinda Gates Foundation.
More Stories

⟵ Go to all Research Stories
Get the Educator
Subscribe to our monthly newsletter.
Stanford Graduate School of Education
482 Galvez Mall Stanford, CA 94305-3096 Tel: (650) 723-2109
- Contact Admissions
- GSE Leadership
- Site Feedback
- Web Accessibility
- Career Resources
- Faculty Open Positions
- Explore Courses
- Academic Calendar
- Office of the Registrar
- Cubberley Library
- StanfordWho
- StanfordYou
Improving lives through learning

- Stanford Home
- Maps & Directions
- Search Stanford
- Emergency Info
- Terms of Use
- Non-Discrimination
- Accessibility
© Stanford University , Stanford , California 94305 .

IMAGES
VIDEO
COMMENTS
Step 1: Gather your qualitative data and conduct research (Conduct qualitative research) The first step of qualitative research is to do data collection. Put simply, data collection is gathering all of your data for analysis. A common situation is when qualitative data is spread across various sources.
136 CASE STUDY RESEARCH data, and rival explanations. All four strategies underlie the analytic techniques to be described below. Without such strategies (or alternatives to them), case study analysis will proceed with difficulty. The remainder of this chapter covers the specific analytic techniques, to be
Notably, about half of the articles reported analyzing their qualitative data via content analysis and a constant comparative method, which was also commonly referred to as a grounded theory approach and/or inductive analysis. Four studies did not identify data analysis methods, and no mention was made of using a qualitative data analysis ...
Qualitative analysis involves analyzing non-numerical data from sources like interviews, observations, documents, and images in a case study. It helps explore context, meaning, and patterns to ...
Case studies tend to focus on qualitative data using methods such as interviews, observations, and analysis of primary and secondary sources (e.g., newspaper articles, photographs, official records). Sometimes a case study will also collect quantitative data. ... Step 4: Describe and analyze the case. In writing up the case study, you need to ...
Case study protocol is a formal document capturing the entire set of procedures involved in the collection of empirical material . It extends direction to researchers for gathering evidences, empirical material analysis, and case study reporting . This section includes a step-by-step guide that is used for the execution of the actual study.
Case studies tend to focus on qualitative data using methods such as interviews, observations, and analysis of primary and secondary sources (e.g., newspaper articles, photographs, official records). Sometimes a case study will also collect quantitative data. Example: Mixed methods case study. For a case study of a wind farm development in a ...
Data sources: The research example used is a multiple case study that explored the role of the clinical skills laboratory in preparing students for the real world of practice. Data analysis was conducted using a framework guided by the four stages of analysis outlined by Morse ( 1994 ): comprehending, synthesising, theorising and recontextualising.
How to Analyze a Case Study Adapted from Ellet, W. (2007). The case study handbook. Boston, MA: Harvard Business School. A business case simulates a real situation and has three characteristics: 1. a significant issue, 2. enough information to reach a reasonable conclusion, 3. no stated conclusion. A case may include 1. irrelevant information 2.
Besides discussing case study design, data collection, and analysis, the refresher addresses several key features of case study research. First, an abbreviated definition of a "case study" will help identify the circumstances when you might choose to use the case study method instead of (or as a complement to) some other research method.
As case study research is a flexible research method, qualitative data analysis methods are commonly used [176]. The basic objective of the analysis is, as in any other analysis, to derive conclusions from the data, keeping a clear chain of evidence.
Case study is also described as a method, given particular approaches used to collect and analyze data. Case study research is conducted by almost every social science discipline: business, education, sociology, psychology. Case study research, with its reliance on multiple sources, is also a natural choice for researchers interested in trans ...
Steps. Download Article. 1. Examine and describe the business environment relevant to the case study. Describe the nature of the organization under consideration and its competitors. Provide general information about the market and customer base.
Data analysis. Analyzing case study research involves making sense of the rich, detailed data to answer the research question. This process can be challenging due to the volume and complexity of case study data. However, a systematic and rigorous approach to analysis can ensure that the findings are credible and meaningful.
A case study protocol should have the following constituent elements: (a) an overview of the entire study including its objectives, (b) a detailed description of field procedures including the techniques of data collection to be employed, and how one plans to move ahead and operate in the field, (c) a clearly and sharply developed questions ...
The data collection method should be selected based on the research questions and the nature of the case study phenomenon. Analyze the data: The data collected from the case study should be analyzed using various techniques, such as content analysis, thematic analysis, or grounded theory. The analysis should be guided by the research questions ...
only way case study data can be analysed (Barry, 1998) and it is recommended that they be used in conjunction with the overall case study design frameworks proposed by Yin (1994); and Miles and Huberman (1994). Create data repository To be able to analyse the data from the case studies it has to be in a format that allows for easy manipulation.
Case study is unbounded and relies on gathering external information; case analysis is a self-contained subject of analysis. The scope of a case study chosen as a method of research is bounded. However, the researcher is free to gather whatever information and data is necessary to investigate its relevance to understanding the research problem.
Step 1: With Data Analytics Case Studies, Start by Making Assumptions. Hint: Start by making assumptions and thinking out loud. With this question, focus on coming up with a metric to support the hypothesis. If the question is unclear or if you think you need more information, be sure to ask.
Step 1: Define your qualitative research questions. The qualitative analysis research process starts with defining your research questions. It's important to be as specific as possible, as this will guide the way you choose to collect qualitative research data and the rest of your analysis. Examples are:
Identify the key problems and issues in the case study. Formulate and include a thesis statement, summarizing the outcome of your analysis in 1-2 sentences. Background. Set the scene: background information, relevant facts, and the most important issues. Demonstrate that you have researched the problems in this case study. Evaluation of the Case
What the Case Study Method Really Teaches. Summary. It's been 100 years since Harvard Business School began using the case study method. Beyond teaching specific subject matter, the case study ...
Introduction. Clinicians think of case-control study when they want to ascertain association between one clinical condition and an exposure or when a researcher wants to compare patients with disease exposed to the risk factors to non-exposed control group. In other words, case-control study compares subjects who have disease or outcome (cases ...
Four steps are proposed to assist the novice researcher analyse their data that has been collected using a case study method. The first step proposes the creation of a data repository using basic relational database theory. The second step involves creating codes to identify the respective 'chunks' of data. These resulting codes are then analysed and rationalised. The third step involves ...
Humana's Automated Data Analysis Case Study. The key thing to note here is that the approach to creating a successful data program varies from industry to industry. Let's start with one to demonstrate the kind of value you can glean from these kinds of success stories. Humana has provided health insurance to Americans for over 50 years.
The main difference between big data analytics and traditional data analytics is the type of data handled and the tools used to analyze it. Traditional analytics deals with structured data, typically stored in relational databases.This type of database helps ensure that data is well-organized and easy for a computer to understand.
In 2023 K-12 schools experienced a rise in cyberattacks, underscoring the need to implement strong systems to safeguard student data. Technology is "requiring people to check their assumptions ...
Yuval Atsmon: When people talk about artificial intelligence, they include everything to do with analytics, automation, and data analysis. Marvin Minsky, the pioneer of artificial intelligence research in the 1960s, talked about AI as a "suitcase word"—a term into which you can stuff whatever you want—and that still seems to be the case.
As the nation prepares to mark the 70th anniversary of the landmark U.S. Supreme Court ruling in Brown v. Board of Education, a new report from researchers at Stanford and USC shows that racial and economic segregation among schools has grown steadily in large school districts over the past three decades — an increase that appears to be driven in part by policies favoring
The methodology of empirical research as applied in this study, along with the corresponding data analysis conducted in the case study of Nangou Village, aims to reveal the influencing factors for ...