- Open access
- Published: 14 May 2024

15 years of Big Data: a systematic literature review
- Davide Tosi 1 ,
- Redon Kokaj 1 &
- Marco Roccetti 2
Journal of Big Data volume 11 , Article number: 73 ( 2024 ) Cite this article
724 Accesses
Metrics details
Big Data is still gaining attention as a fundamental building block of the Artificial Intelligence and Machine Learning world. Therefore, a lot of effort has been pushed into Big Data research in the last 15 years. The objective of this Systematic Literature Review is to summarize the current state of the art of the previous 15 years of research about Big Data by providing answers to a set of research questions related to the main application domains for Big Data analytics; the significant challenges and limitations researchers have encountered in Big Data analysis, and emerging research trends and future directions in Big Data. The review follows a predefined procedure that automatically searches five well-known digital libraries. After applying the selection criteria to the results, 189 primary studies were identified as relevant, of which 32 were Systematic Literature Reviews. Required information was extracted from the 32 studies and summarized. Our Systematic Literature Review sketched the picture of 15 years of research in Big Data, identifying application domains, challenges, and future directions in this research field. We believe that a substantial amount of work remains to be done to align and seamlessly integrate Big Data into data-driven advanced software solutions of the future.
Introduction
Over the past 15 years, Big Data has emerged as a foundational pillar providing support to an extensive range of different scientific fields, from medicine and healthcare [ 1 ] to engineering [ 2 ], finance and marketing [ 3 , 4 , 5 ], politics [ 6 ], social networks analysis [ 7 , 8 ], and telecommunications [ 9 ], to cite only a few examples. This 15-year period has witnessed a significant increase in research efforts aimed at unraveling the major problems in Big Data, with an almost innumerable array of potential solutions and data sources [ 10 , 11 , 12 , 13 ]. This has resulted in a boundless world of scientific papers that, in the end, have demonstrated the twofold, ambivalent nature of Big Data. On one side, in fact, we have had a confirmation of the pivotal role played by this scientific field in shaping the technological advancements of our time. On the other side, an approach to the comprehension of Big Data, based on this endless universe of ten of thousand technical papers, each specializing in its specific sector, however natural it might seem, has become not sustainable because it has often made researchers confuse (or mixing) the theory (of Big Data) with the practice or use of it. We cannot ignore that there have also been numerous active attempts to describe the general landscape of Big Data through survey papers. Nonetheless, again, given the vastness of the subject, the majority of them did not shun the trap of pre-formed models and have tried to respond, as closely as possible, to the concrete requirements coming from just one sub-field or from the point of view of a few perspectives. In this complex context, to take at least one step further into the knowledge of the state of the art of Big Data research over the above-mentioned period of time, we have decided to conduct a different form of comprehensive exploration which was not biased by the specificity of some given sectors or confounded by single technical perspectives. To do that, we have adopted the methodology termed systematic literature review (SLR), as proposed by Kitchenham and Charters [ 14 ] in the field of software engineering [ 15 , 16 ]. Although SLR proceeds through a set of well-defined steps, also in this case, an initial choice has to be made regarding the most crucial parameters through which the subject of investigation should be explored. In the case of Big Data, our primary focus has been on gaining insights into the principal application domains of Big Data, unraveling the major challenges and limitations encountered by researchers in the analysis of the typically enormous datasets they manage, and unveiling the emerging trends and directions in future Big Data research.
Guided by the structured methodology imposed by SLR, we hence started with three research questions that matched the points raised before: essentially, (i) most common application domains, (ii) current research challenges and limitations, and (iii) emerging future trends and directions. From this point on, we proceeded following the SLR steps. Basically: first, we translated the three research questions above into specific search terms, through which five different digital libraries were investigated, namely: Scopus , IEEE Explore , ACM Digital Library , SpringerLink and Google Scholar . Upon completion of the search activity (detailed in the following section of this paper), 189 primary studies that matched our generic search criteria were identified. Of these 189, only 32 of these studies were actually reviews. Since the target of our study was to provide a panoramic view of this 15-year Big Data research period, (a) shedding light on the prevalent application domains, (b) highlighting the hurdles faced by researchers, and (c) finally outlining the potential trajectories for future research, we focused on the analysis of just these 32 survey studies.
With this paper, we do not want to conduct a traditional literature review on a very extensive topic like Big Data. Traditional scientific surveys can include many more studies and corresponding papers, and they are mainly built with an eye toward generalizability and inclusion rather than selectivity and relevance. As a consequence, those approaches often bring to us no much more than a mere summary of the topic of interest. SLRs, instead, start from the legitimate presumption to be more than merely a summary of a topic. In essence, they distinguish themselves from ordinary surveys of the available literature because they are specifically built to add to the identification of all publications on a topic also all the following activities: explicit formulation of a search objective, identification and description of a search procedure, definition of criteria for inclusion and exclusion of publications, literature selection, and information extraction only based on a transparent evaluation of the quality of publications. Not only this, but an SLR should also provide insightful information on the current state of research on a topic, starting from a given set of research questions and following a formal methodological procedure, designed to reduce distortions caused by an overly generous and restrictive selection of the literature, while guaranteeing the reliability of the selected publications. Hence, to pursue these objectives, an SLR should start with the definition of the criteria for determining what should be included/excluded before conducting the search. Not to mention that, typically, an SLR should be performed mainly using electronic literature databases. It should be also noticed that such a structured approach should document all the information gathered (and the steps taken as part of this process), with the aim of making the paper selection process completely visible and reproducible [ 17 ].
In the end, we know very well that a point-to-point analysis of the set of almost 320 papers from which we have started our SLR could have brought more (generic) information than that provided by the circa 30 papers finally selected by our SLR. Nonetheless, it is highly likely that this information would have been somewhat redundant, more prone to defects and personal biases, and finally, also more boring to read.
With this SLR, we aim to contribute, in a focused and structured way, to Big Data research in several ways: from one side, we provide researchers with a clear picture of how Big Data application domains changed over time; then, we highlight challenges faced by academia and industry and their evolution in the last 15 years; finally, we sketch a set of open points that researchers will take into consideration in the next future.
We can conclude that, while our collective understanding of Big Data has grown after this investigation, this analysis has underscored again the fact that in this field, a kind of optimal stability emerges in terms of research interests through the even distribution among applications domains/challenges/future trends. From one side, we observe a pervasive adoption of Big Data solutions in all everyday life domains (such as Energy [ 18 ], Smart Cities [ 9 ], and Healthcare [ 19 ].) On the other hand, researchers have spent a lot of effort managing data quality, designing and developing advanced frameworks to manage Big Data in real-time, focusing on security and privacy. However, many challenges still remain open to seamlessly integrate Big Data into data-driven advanced software solutions of the future, such as mitigating energy consumption, optimizing algorithms, increasing framework security with privacy and ethical focus, intersecting Artificial Intelligence and Machine Learning technologies, opening data sets, improving interoperability among different stakeholders, and considering societal and business changes.
The remainder of this paper is organized as follows: in Sect " Research method ", we run the SLR methodology on our Big Data use case (with the definition of our research questions, the search strategy, the inclusion/exclusion criteria, the study quality assessment questionnaire, and the data extraction from primary studies). All this is in the dual attempt to explain the abstract methodology, as well as its application in our field. Section " SLR: implementation " describes how we conducted the review and the results obtained in each stage and step of our SLR; Section " SLR: results " shows our findings, briefly summarizing each of our selected primary studies; Section " Discussion " discusses critically those findings garnering special attention in our analytical process; Section " Threats to validity " discusses the possible threats to the validity of our study; Section " Conclusion " demonstrates the conclusions we drew for our SLR.
A taxonomy of key concepts for Big Data evolution over the last 15 years is presented in Fig. 1 .
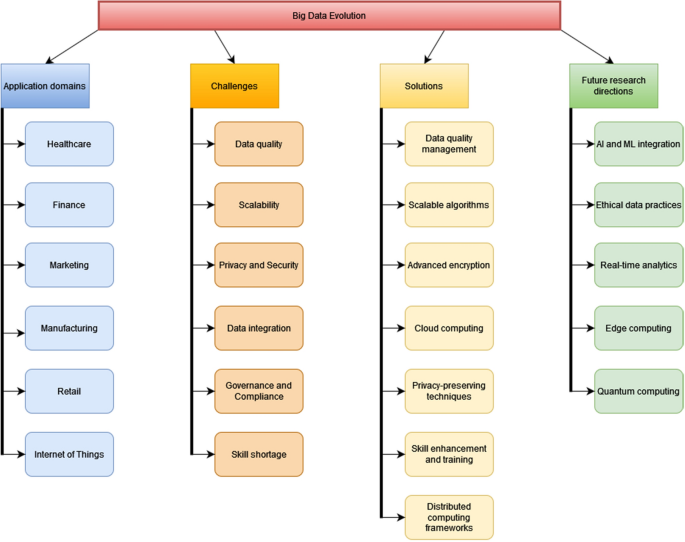
Taxonomy of Big Data evolution over the last 15 years
Research method
Research questions.
This SLR has been conducted following the procedure defined by Kitchenham and Charters. As such, in the first step, we defined the research questions (RQ) that will drive the entire review methodology.
As we define the research questions that will guide our SLR, it is crucial to establish a balance between the breadth and depth of our investigation. After careful consideration and to ensure that our review maintains a focused and meaningful scope, it has been decided to narrow down our research questions to the following three:
RQ1 : what are the most common application domains for Big Data analytics, and how have they evolved over time?
RQ2 : what are the major challenges and limitations that researchers have encountered in Big Data analysis, and how have they been addressed?
RQ3 : what are the emerging research trends and directions in Big Data that will likely shape the field in the next 5 to 10 years?
Search strategy
SLR begins by looking for relevant studies related to our research questions. To do this, we find appropriate search terms using the method outlined by Kitchenham and Charters, which suggests to consider three aspects: Population (P), Interventions (I), and Outcomes (O).
We identified the following relevant search terms for each aspect in our review:
Population : Big Data, real-time data analytics, large datasets.
Intervention : methodologies, techniques, domains, architectures, solutions.
Outcomes : research trends, future directions, emerging technologies, challenges, SLR, Systematic Literature Review.
The search string was constructed as follows:
P refers to population terms, I refers to intervention terms and O refers to outcome terms, all of which are connected through boolean operators AND and OR.
Searches string may take the exemplar form like the following:
(“big data” OR “real-time data analytics” OR “large datasets”) AND (“methodologies” OR “techniques” OR “domains” OR “architectures” OR “solutions”) AND (“research trends” OR “future directions” OR “emerging technologies” OR “challenges” OR “SLR” OR “Systematic Literature Review”)
Since we need to find and study primary studies related to our research questions, the selection of appropriate digital libraries/search engines to search for the articles needed is essential. For this reason, it has been decided to use the following state-of-the-art sources:
Scopus : a multidisciplinary database that covers a broad range of research fields.
IEEE Xplore : an invaluable resource for technology and engineering-related SLR.
ACM Digital Library : a comprehensive collection of relevant articles, conference papers, and journals focused on computer science and information technology.
SpringerLink : an extensive collection of academic articles in the fields that align closely with our research interests.
Google Scholar : a freely accessible web search engine that indexes scholarly literature across various disciplines.
We aim to ensure a comprehensive and focused literature search by utilizing these sources, thereby facilitating a thorough and methodical research.
Inclusion/Exclusion criteria
In this stage of the SLR, we need to make an accurate selection of the studies extracted. To do this, we must define some rigorous inclusion/exclusion criteria, to decide which studies are going to be useful for our purpose. To achieve this, studies were excluded based on the following criteria:
Studies published before the 15-year time frame
Studies in languages other than English
Exclude non-academic sources, including blogs, news articles, marketing materials, and reports from non-academic organizations
Studies that are only marginally related to Big Data or the specific topics within our research questions.
In conclusion, all those studies that are not cut off by the exclusion criteria above are to be considered as included. They are called “Primary Studies” (PS).
Study quality assessment
Kitchenham and Charters stresses the necessity of assessing the quality of primary studies to reduce bias and enhance the validity of the evaluation process. In our research, we employ a study quality assessment to make sure that we have only the most relevant results for our research.
To achieve this, we formulated a five question study quality questionnaire, which serves as the foundation for assessing the quality of the primary studies:
QA1 : has the primary study established a well-defined research objective?
QA2 : did the primary study comprehensively describe its research methods and data sources?
QA3 : has the technique or approach undergone a trustworthy validation?
QA4 : has the primary study effectively identified and discussed the significant challenges and limitations encountered in Big Data analysis?
QA5 : are the findings, research trends, and directions clearly presented and directly connected to the study’s objectives or goals?
Hence, we applied the formulated questionnaire to the included PSs to assess their quality. The output of this SLR stage will be discussed in Section 4.
Data extraction
The data extraction process entails gathering relevant information from the chosen primary studies to address the research questions. To facilitate this process, we have created a dedicated data extraction form, as shown in Table 1 . As suggested in Kitchenham and Charters, we used the test-retest process to check the consistency and accuracy of the extracted data with respect to the original sources. After finishing the data extraction for all the selected studies, we randomly selected 3 primary studies and performed a second extraction of the data. No inconsistencies were detected.
SLR: implementation
In this section, we describe step-by-step the implementation and execution of the different stages of our SLR. Figure 2 depicts the search stages followed and the resulting number of primary studies for each stage.
In stage 1, an automated search was performed by applying the search string to the digital libraries. The software used for the management of the references is Zotero (www.zotero.org), a popular choice for SLRs. We began the research using the following research string:
(“big data” OR “real-time data analytics” OR “large datasets”) AND (“methodologies” OR “techniques” OR “domains” OR “architectures” OR “solutions”) AND (“research trends” OR “future directions” OR “emerging technologies” OR “challenges” OR “SLR” OR “Systematic Literature Review”). As a result, we found a total of 4204 studies. The reason for this many results could be attributed mostly to the main topic of this SLR being “Big Data”, a hugely popular field, especially in the last few years.
In stage 2, we used the Zotero’s duplicate identification tool, and we found a total of 25 duplicates. Additionally, 1 duplicate was found manually, bringing the total number of results to 4178 articles.
In stage 3, studies were excluded based on the title and the language. Fortunately, all the documents were in English, so we just needed to focus on the title, eliminating what had no use for our research. This cut down the total number to 553.
In stage 4, we eliminated the articles whose abstracts had marginal or no interest at all to us. At the end of the process, 189 Primary Studies were left, 32 of which were SLRs.
To ensure the best quality possible for our SLR, we have collected generic information on all the 189 studies that passed the Primary Study check. This information is depicted in Figs. 3 and 4 . We then proceeded with an in-depth full-text review for the 32 PSs, which are the main subject of our SLR.
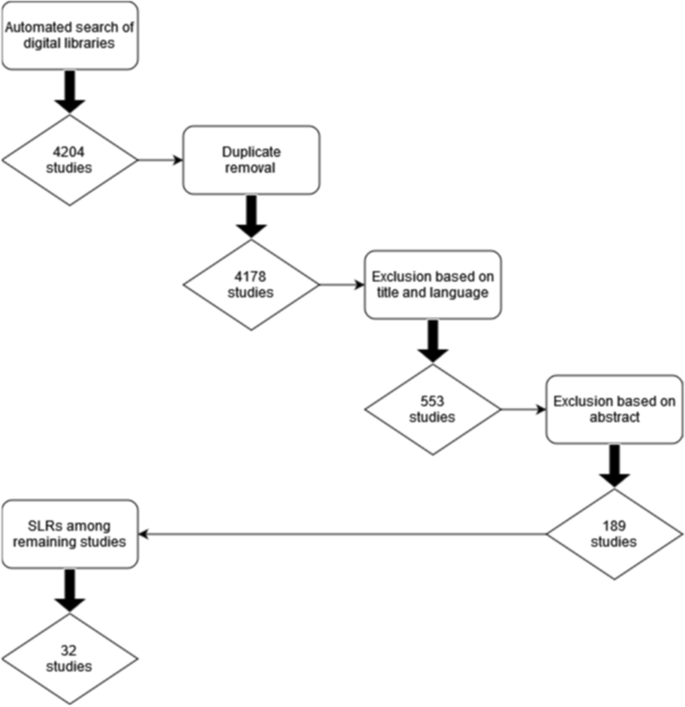
Stages of the applied search strategy
Figure 3 depicts the distribution per year for all the 189 studies. Our SLR focuses on the evolution of Big Data in the last 15 years. In any case, no studies before 2012 were detected. The reason for this could be attributed to the fact that before then Big Data, as a research topic, was not as popular.
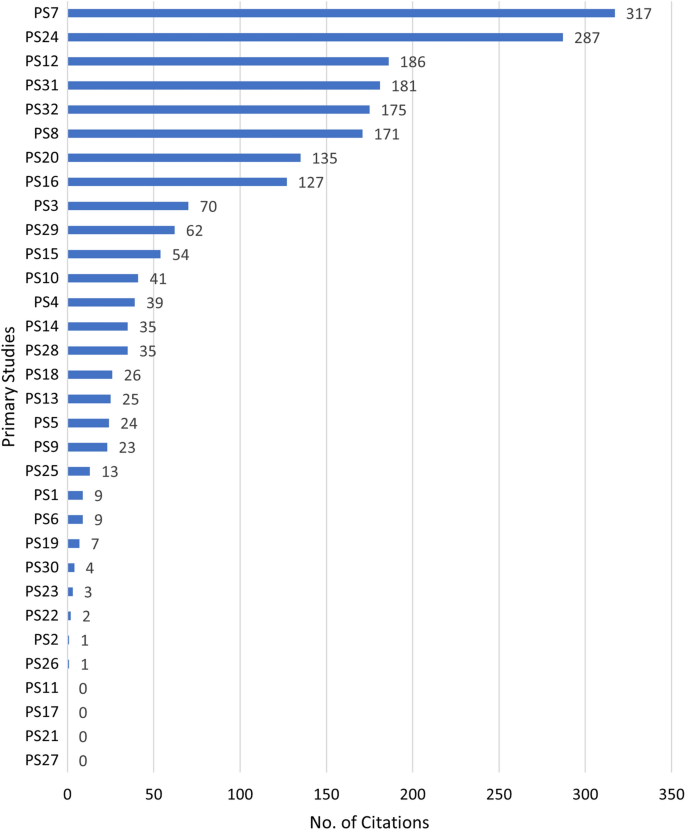
Number of filtered primary studies and number of total citations
Figure 4 represents the total number of citations per year for our selected 32 Primary Studies. The graph clearly shows that the most recent studies have not been cited as much. Particularly, even though the studies released in the last two years compose about one third of our selected primary studies (11 out of 32), we can see that they have not been cited as much in comparison to the previous years. The lower citation rate may indicate that recently, researchers have focused more on understudied areas or more recent emerging trends, suggesting that the field of Big Data is currently undergoing an evolution. However, further analysis of the quality, methodology and context of these studies is necessary for more concrete conclusions.
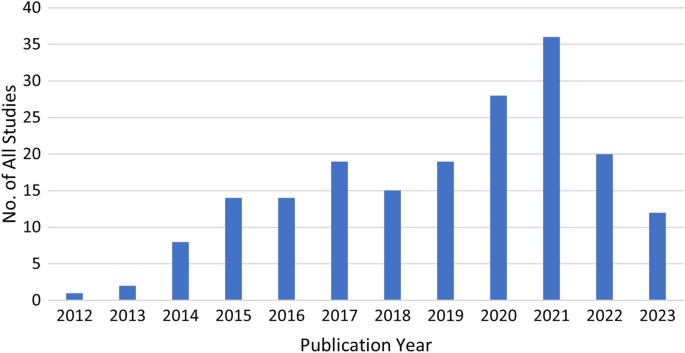
Number of total PSs per year
For further clarity, we elaborated Table 2 to represent the chosen articles by highlighting the first author’s family name, the venue, the title of each PS, and a short introduction that highlights the main findings of each PS. Note that the ”J” indicates that the article has been published in a journal.
To better understand the influence of the selected Primary Studies over time, we created a bubble chart to show the most cited documents by aggregating the PSs with the same publication year (see Fig. 5 ). The size of each bubble is proportional to the number of citations.
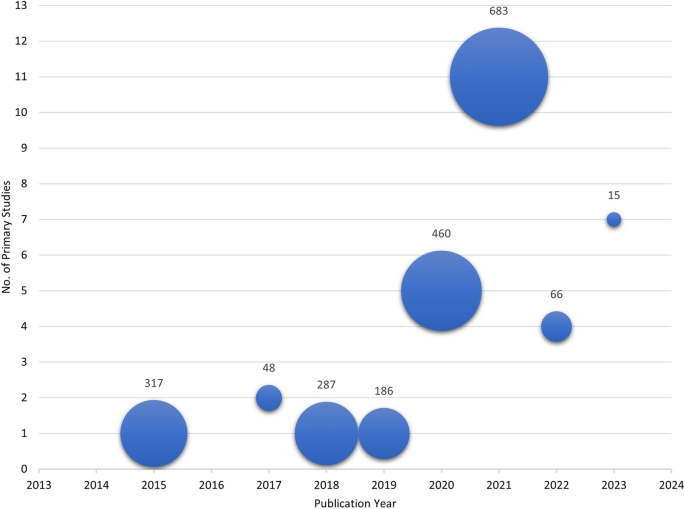
Bubble chart showing the number of primary studies and total citations per year of publication
SLR: results
The study of the PSs allowed us to pinpoint exactly which research question (RQ1-RQ3) is answered by each primary study. Table 3 summarizes our findings.
As previously stated, it is important to assess the quality of each study. In subsect. " Study quality assessment ", we developed a brief questionnaire that would help us determine the quality of a primary study. Table 4 shows the results of this quality check. It uses a simple “Yes,” “No,” or “na” (used when we don’t have enough information to answer) to fill out the Quality Assessment questionnaire.
From now on, we will briefly summarize each study and its findings.
PS1—A comprehensive and systematic literature review on the Big Data management techniques in the internet of things [ 20 ]
In this article, the authors explored the Big Data management techniques applied to the internet of things. Big Data was initially applied for healthcare monitoring, smart cities, and industrial systems. Over time, with the evolution of IoT, it expanded to include broader topics: healthcare applications involved health state monitoring and predictive modeling, smart cities encompassed traffic management, energy efficiency and security, while industrial systems employed Big Data to improve scalability and security. The application landscape broadened emphasizing the importance of quality attributes such as performance, efficiency, reliability, and scalability in ensuring the success of Big Data Analytics systems in IoT across ever-evolving domains.
The challenges and open issues in Big Data Analytics within IoT span various dimensions, including centralized architectures, energy consumption in data collection, blockchain limitations, communication challenges, and diverse data features.
For future research, the exploration of AI for intelligent mobile data collection will take on a more relevant role, combining compressive sensing with AI for communication challenges and utilizing new optimization algorithms for data processing. To ensure security and privacy in IoT, Big Data Analytics could involve cryptography mechanisms, a data perception layer and a lightweight framework with AI. Addressing these challenges is essential for advancing Big Data Analytics in the evolving landscape of IoT applications.
PS2—A comprehensive review on Big Data for industries challenges and opportunities [ 21 ]
The article explores the transformative impact of Big Data Analytics in power systems, mineral industries, and manufacturing. In power systems, it revolutionizes fault detection, enables early warning systems and predicts future electricity demand, enhancing reliability and decision-making. For mineral industries, Big Data improves data storage, processing and analytics, optimizing exploration, extraction, and resource management. In manufacturing, it facilitates data-driven decision-making, comprehensive product quality assessment, and streamlined supply chain management for increased operational efficiency.
The study also highlights challenges in implementing Big Data Analytics, emphasizing the crucial need for precise data quality assessment models and secure frameworks. Machine learning and data analytics play a pivotal role in overcoming challenges, particularly in fault detection, load forecasting, and reservoir management. The call for open-source databases and integration with machine learning addresses the scarcity of datasets, reflecting challenges in maximizing Big Data’s potential.
Furthermore, the paper recommends future research trends, including advanced data quality assessment models, frameworks for high-dimensional data and solutions for secure communication. Emphasizing open-source databases and integrating machine learning promotes a collaborative and transparent approach. The call for interpretable models reflects a trend toward understanding and optimizing Big Data Analytics. Overall, these recommendations shape the future direction of Big Data applications in diverse industries.
PS3—A survey on IoT Big Data current status, 13 V’s challenges, and future directions [ 22 ]
The document delves into the landscape of Big Data Analytics, particularly exploring its integration with the Internet of Things. Application domains such as energy, healthcare, transportation, and smart cities emerge prominently. The discussion unfolds how these domains have evolved, signalling a shift towards IoT-driven intelligent applications.
Within this expansive terrain, the study identifies and elucidates 13 major challenges encapsulated by the “13 V’s”. These challenges span traditional aspects like volume, velocity, and variety, extending to less common concerns like vagueness and location-aware data processing. The document also offers innovative solutions, like edge-based processing and semantic representation, as strategies to manage these complex challenges.
In regards to the future, the document outlines emerging trends anticipated to define the Big Data landscape in the coming 5 to 10 years. These include a focus on energy-efficient data acquisition, the integration of machine learning and deep learning for advanced analytics, a strategic emphasis on edge and fog infrastructures, the evolving paradigm of multi-cloud data management, a shift towards data-oriented network addressing, and the increasing adoption of blockchain technology. These trends collectively indicate a trajectory towards more efficient, scalable, and secure practices in Big Data Analytics, particularly within the realm of IoT applications.
PS4—A systematic literature review on features of deep learning in Big Data analytics [ 23 ]
The document navigates the evolution of Big Data, emphasizing challenges and the rise of machine learning, particularly Deep Learning. Machine learning’s widespread use, observed in areas like healthcare and finance, underscores its crucial role. Even in complex data scenarios, its effectiveness is evident, as demonstrated by the U.S. Department of Homeland Security’s success in identifying threats.
Recognizing a gap in existing research, the document proposes a review focusing on Deep Learning in Big Data Analytics. The goal is to explore features like hierarchical layers and high-level abstraction. The study emphasizes Deep Learning’s strength in handling extensive datasets, its versatility, and its ability to prevent over fitting.
This exploration into Big Data’s journey underscores the central role of machine learning. The proposed review, specifically focusing on Deep Learning in Big Data Analytics, not only captures current advancements but also suggests there’s more to discover in the future where Big Data and machine learning intersect.
PS5—A systematic survey of data mining and Big Data analysis in internet of things [ 24 ]
The document navigates through diverse applications of Big Data Analytics, illustrating its transformative journey across sectors. Notably, it tracks the evolution within healthcare and finance, showcasing how Big Data has become integral to these domains over time.
Going further, the research dives into the various challenges of Big Data analysis. It identifies three main challenges: dealing with societal changes, understanding how businesses use IoT, and solving technical issues like security and connectivity. The study emphasizes the need to adapt to society’s changing needs, categorize IoT uses in business and front technical problems for effective Big Data analysis.
Moreover, the research anticipates future trends, in particular the rising importance of Big Data frameworks in handling expansive IoT-generated data. The intersection of these frameworks with data mining in the IoT domain emerges as a pivotal focus, pointing toward exciting possibilities and potential paths for future research in the realm of Big Data.
PS6—Access methods for Big Data: current status and future directions [ 25 ]
The document explores diverse applications of Big Data Analytics in research, education, urban planning, transportation, environmental modeling, energy conservation, and homeland security, emphasizing its transformative potential.
It addresses challenges like heterogeneity, scale, timeliness, privacy, and the evolving processing paradigms due to data volume surpassing computational resources.
Future directions include the need for systems handling structured and unstructured data, embedded analytics for real-time processing, innovative paradigms, application frameworks, and advanced databases ensuring transactional semantics. The research underscores the importance of tools addressing ethical, security, and privacy concerns.
PS7—An industrial Big Data pipeline for data-driven analytics maintenance applications in large-scale smart manufacturing facilities [ 26 ]
This research introduces an innovative Big Data pipeline designed for industrial analytics in manufacturing.
The pipeline excels in integrating legacy and smart devices, ensuring cross-network communication, and adhering to open standards, marking a significant evolution in the field. The document showcases the pipeline’s ability to handle complexities, integrate older systems, ensure reliability, and scale efficiently in industrial data analytics.
The future plan involves implementing the pipeline to validate its architecture, particularly in predictive maintenance for Wind Turbines and Air Handling Units, contributing to the evolving landscape of Big Data Analytics.
PS8—Applications of Big Data in emerging management disciplines: a literature review using text mining [ 27 ]
This study explores diverse applications of Big Data Analytics across twelve emerging management domains, emphasizing their dynamic nature over time.
It addresses adoption challenges, focusing on data quality, resource management, and distinguishing between the ability and capability of organizations in using Big Data Analytics. The research underscores the thoughtful adoption of Big Data Analytics and the importance of measuring its business value comprehensively. It acknowledges the difficulty of translating insights into real-time actionable items.
Looking forward, the study proposes a framework connecting emerging management domains with conventional practices, suggesting future research areas in human resources, marketing, sales, strategy, and services. The research emphasizes the need for in-depth exploration to integrate emerging domains into established management practices, providing valuable insights for research and practical application.
PS9—Applying Big Data analytics in higher education: a systematic mapping study [ 28 ]
The document conducts a thorough exploration of Big Data Analytics (BDA) in Higher Education Institutions from 2010 to 2020. It uncovers diverse BDA applications in three domains: Educational Quality, Decision-Making Process, and Information Management.
Challenges in BDA adoption include handling large data volumes, addressing privacy concerns, and dealing with resource constraints. The study emphasizes the need for practical outcomes, automated tools, and validated frameworks.
Despite robust research interest, the field exhibits immaturity, with a prevalence of conference papers indicating an early development stage. The study calls for increased empirical research to fortify the evidence base and foster a more mature BDA integration in higher education.
PS10—Artificial intelligence approaches and mechanisms for Big Data analytics: a systematic study [ 29 ]
The SLR explores AI-driven Big Data Analytics, emphasizing machine learning, knowledge-based reasoning, decision-making algorithms, and search methods. Applications, notably in supervised learning, aim to enhance precision and efficiency but grapple with complexity and scalability issues.
Challenges encompass processing vast, heterogeneous data, ensuring system security, and addressing qualitative parameters. Fog computing emerges as a potential solution, yet security concerns remain under-explored.
Emerging trends spotlight Big Data Analytics for IoT through fog computing, the need for enhanced algorithms handling extensive data, and the necessity to address data quality issues in unstructured formats.
PS11—Bibliometric mining of research directions and trends for Big Data [ 30 ]
The research identifies key application domains, with particular focus on China, and emerging directions such as Machine Learning and Healthcare.
Navigating challenges, the study introduces a semi-automatic method, utilizing blacklists and thesauri to enhance precision in identifying research directions. This favors a balance between automation and expert input.
The study forecasts Big Data’s future using a growth rate criterion, emphasizing Machine Learning and Deep Learning. Moreover, the study suggests applying its methodology not only to Big Data but also to various research areas, such as Machine Learning, showcasing its potential applicability in diverse research areas.
PS12—Big Data adoption: state of the art and research challenges [ 31 ]
The study explores the widespread adoption of Big Data Analytics across diverse sectors such as finance, education, healthcare, and more. It identifies a need for increased research in untapped areas like education and healthcare, suggesting potential transformative effects.
Challenges in current Big Data research include the need for refined theoretical models, adaptable data collection methods, and larger sample sizes to ensure accuracy. The study recommends a mixed-method approach to address these challenges effectively.
The study, although not explicitly stating upcoming trends, suggests a changing research focus in both developing and developed countries. It indicates a growing awareness of untapped opportunities, hinting at a future emphasis on specific situations and new factors in Big Data adoption.
PS13—Big Data analytics for data-driven industry: a review of data sources, tools, challenges, solutions, and research directions [ 32 ]
This research provides a comprehensive overview of Big Data Analytics. Exploring application domains, it traces Big Data’s historical integration across education, healthcare, finance, national security, and Industry 4.0 components like IoT and smart cities.
Delving into challenges, the research highlights skill shortages, dataset management, privacy, scalability, and intellectual property issues. Solutions range from software-defined data management to innovative truthfulness and privacy preservation methods.
Looking ahead, the study identifies some emerging trends: sourcing data from education and diverse IoT devices, refining pre-processing, advancing data management, enhancing privacy, and exploring deep learning methods. These trends forecast a dynamic future for Big Data Analytics, shaping the field in the next years.
PS14—Big Data analytics in healthcare: a systematic literature review and road map for practical implementation [ 33 ]
The paper conducts a thorough examination of Big Data Analytics (BDA) applications in healthcare, introducing the novel Med-BDA architecture.
Notably, the work addresses challenges inherent in BDA (such as increased costs, difficulty in acquiring a relevant skill set, rapidly expanding technology stack, and heightened management overhead), presenting a comprehensive road map to alleviate issues such as cost escalation and skill acquisition hurdles.
The document concludes by outlining the potential for extensions to Med-BDA and its applicability to diverse Big Data domains, showcasing a forward-looking perspective in BDA research and application.
PS15—Big Data analytics in telecommunications: literature review and architecture recommendations [ 34 ]
The document explores Big Data Analytics in TELCO, introducing LambdaTel as a proposed solution for batch and streaming data processing. It discusses Big Data Analytics applications like CRM and Customer Attrition.
Challenges, such as the lack of standardized architecture, are acknowledged. LambdaTel addresses these challenges through a structured approach, emphasizing security and recommending the usage Python.
While not explicitly talking about future trends, the document suggests a commitment to ongoing adaptation, seen in recommendations like Python usage, Dockerized implementation and the application of LambdaTel in a local Telco company for cross-selling/up-selling.
PS16—Big Data analytics meets social media: a systematic review of techniques, open issues, and future directions [ 35 ]
The document highlights social media’s transformative impact in healthcare, emphasizing its role in patient support and disease tracking. It emphasizes leveraging social platforms for patient support, disease prevention, and real-time tracking of contagious diseases.
The review highlights challenges in both content and network-oriented approaches, such as privacy concerns, scalability limitations, and accuracy enhancement with incomplete data. Comprehensive resolution remains an open frontier, requiring innovative solutions for privacy preservation and accurate predictions.
The paper also highlights emerging trends in Big Data Analytics, emphasizing real-time and predictive analysis, and addressing challenges in sentiment analysis. It identifies under explored areas like political and e-commerce applications, underscoring the expanding trajectory of Big Data Analytics. Furthermore, it emphasizes the evolving complexities of linguistic analysis, underlining the need for domain-dependent sentiment analysis, and addressing challenges like sarcasm detection.
PS17—Big Data and its future in computational biology: a literature review [ 36 ]
The document underscores the growing significance of Big Data in computational biology and healthcare, particularly in the conversion of healthcare records into digital formats. It highlights the major application domains, focusing on optimizing health and medical care through electronic health data.
Challenges include the under-utilization of electronic health data and the need to convert raw data into actionable information. Despite increasing interest, the field lacks comprehensive literature reviews.
The document outlines emerging trends in Big Data for computational biology and bio informatics. It emphasizes the pivotal role of volume, variety, and velocity in defining Big Data’s impact on bio informatics. Key technologies, including Hadoop and MapReduce, are discussed, illustrating their significance in the field. The integration of Big Data technology is shown to enhance biological findings and facilitate real-time identification of high-risk patients. However, limitations, such as narrow study focuses, are noted.
PS18—Big Data and sentiment analysis: a comprehensive and systematic literature review [ 37 ]
The document delves into the diverse applications of Big Data Analytics, spotlighting its evolution, notably in sentiment analysis for marketing and disaster response.
Challenges identified include data quality issues and the absence of standardized disaster-related datasets. The limitations of centralized data mining algorithms for distributed systems are acknowledged, urging exploration into other platforms (YARN is directly cited as an example). The analysis underscores the need for immediate and improved performance, emphasizing real-time analysis.
In the future, it is important for researchers to carefully look into specific methods like Hadoop, MapReduce, and deep learning. This will help us better understand what these methods are good at and where they might struggle.
PS19—Big Data applications on the internet of things: a systematic literature review [ 38 ]
This document explores the evolving applications of Big Data, from understanding customer sentiments to enhancing disaster response. Hadoop emerges as a popular framework.
Challenges include robust data acquisition from IoT devices, addressing security concerns and optimizing system scalability.
Future directions involve improving algorithms for efficiency, addressing energy consumption, and exploring the synergy of Big Data and machine learning for emergency systems.
PS20—Big Data in education: a state of the art, limitations, and future research directions [ 39 ]
The paper talks about how Big Data Analytics is used in various areas, especially in education, with a noticeable increase in publications from 2014 to 2019. It highlights important topics like how students behave, creating models, using data for education, improving systems, and adding Big Data (as a topic) to study plans.
Researchers face challenges in employing qualitative methods and data collection techniques, highlighting the need for quantitative approaches and more robust methodologies.
Future research should emphasize quantifying Big Data’s impact, adopting efficient solutions, exploring new tools and developing frameworks for educational applications. Integrating the concept of Big Data into study plans requires significant restructuring and well-designed learning activities.
PS21—Big Data in healthcare—a comprehensive bibliometric analysis of current research trends [ 40 ]
This document unveils the dynamic evolution of Big Data Analytics across diverse application domains, with a notable surge in research activities within the healthcare sector since 2012.
While the study discusses various related studies and challenges in Big Data analysis, it does not directly address or provide specific solutions to those challenges.
Looking ahead, the document reveals emerging trends and directions shaping the future of Big Data Analytics over the next 5 to 10 years. Key themes include data analytics, predictive analytics, and collaborative networks, providing a glimpse into the evolving landscape of research endeavors.
PS22—Big Data life cycle in shop-floor-trends and challenges [ 41 ]
The document explores Big Data Analytics in manufacturing, emphasizing its application domains like maintenance, automation, and decision-making.
Challenges include data measurement errors, high-frequency sampling issues, and the need for real-time processing. The study notes a shift to scalable storage options and highlights the importance of efficient data management.
Emerging trends involve the prominent role of AI and statistical approaches in data processing, coupled with a growing emphasis on data privacy. The study concludes with a call for future work focused on developing a consolidated framework for the Big Data life cycle in manufacturing.
PS23—Big Data testing techniques: taxonomy, challenges and future trends [ 42 ]
The paper explores the shift from traditional to advanced testing methods to address challenges in ETL processes, data quality, and node failures.
Addressing major challenges in Big Data analysis, the paper emphasizes the inadequacy of traditional testing, highlighting specific difficulties like ETL testing, node failure prevention, and unit-level debugging. It showcases evolving strategies employed by researchers to ensure the quality of Big Data systems.
Looking ahead, the document outlines emerging research trends shaping the future of Big Data Analytics. It identifies trends such as combinatorial testing techniques, fault tolerance testing, and model-driven entity reconciliation testing as key areas for future exploration.
PS24—Big Data with cognitive computing: a review for the future [ 43 ]
The paper explores the application domains of Big Data Analytics, highlighting its early stage in conjunction with cognitive computing, particularly in healthcare.
Challenges in adoption are attributed to a perceived lack of strategic value. The study categorizes issues into data, process, and management challenges, emphasizing the potential of integrating cognitive computing to overcome barriers.
Regarding emerging trends, there’s a rising interest in cognitive computing. The research encourages more global collaboration and highlights a gap in understanding how Big Data studies impact decision-making processes.
PS25—Current approaches for executing Big Data science projects-a systematic literature review [ 44 ]
The paper explores the landscape of Big Data Analytics. Regarding the common application domains and their evolution, the study notes a significant increase in articles. Workshops play a crucial role in shaping the trajectory, reflecting a robust and expanding interest in Big Data Analytics, influenced by technological advancements.
It also addresses challenges in Big Data analysis, with a focus on workflows and agility. While acknowledging the conceptual nature of agility papers, a gap between theoretical benefits and practical implementation is underscored, necessitating further exploration to optimize agile frameworks for data science projects.
The study highlights emerging trends in Big Data, emphasizing the need for integrated frameworks in data science. It points out a research gap in standardized approaches, urging further exploration for innovative methodologies.
PS26—Data quality affecting Big Data analytics in smart factories: research themes, issues and methods [ 45 ]
This review explores the growing applications of Big Data Analytics in Smart Factories, emphasizing an upsurge in empirical case studies on production, process monitoring, and quality tracing.
Challenges involve key data quality issues (missing, anomalous, noisy, and old data), as well as ISO-defined data quality dimensions. While technical methods prevail, an integrated approach combining technical and non-technical methods for comprehensive data quality management is highlighted. Theoretical insights focus on data quality dimensions, issues, and resolutions, while practical implications underscore the need for collaboration and integrated methods.
The study calls for future research in frameworks, data quality requirements, and emerging scenarios, contributing to Big Data Analytics evolution in Smart Factories.
PS27—Harnessing Big Data analytics for healthcare: a comprehensive review of frameworks, implications, applications, and impacts [ 46 ]
The study meticulously explores the landscape of Big Data Analytics in healthcare. Noteworthy application domains, such as multi modal data analysis and fusion, natural language processing, and electronic health records, emerge from this exploration.
Some challenges faced in Big Data analysis are presented in the document, highlighting issues like data quality, privacy concerns, and a shortage of skilled professionals. It emphasizes the necessity for interoperability and standardization while identifying ongoing challenges in multi modality, ethical considerations, and bias mitigation.
The research outlines emerging trends and directions in Big Data, emphasizing the importance of ongoing exploration in areas like multi modality, data mining, precision medicine, ethical considerations, and the broader understanding of the Big Data Ecosystem.
PS28—Leveraging Big Data in smart cities: a systematic review [ 47 ]
Big Data Analytics has evolved across diverse domains, expanding from finance and healthcare to smart cities and e-commerce. This evolution has been marked by a transformative impact on industries.
Challenges in Big Data, including security, privacy, and scalability issues, have prompted innovative solutions. Advanced encryption, anonymization techniques, and scalable computing frameworks address these concerns.
Looking ahead, emerging trends highlight the fusion of Big Data with AI, machine learning, and technologies like edge computing. Ethical considerations gain prominence and quantum computing’s potential is explored for handling massive datasets.
PS29—Roles and capabilities of enterprise architecture in Big Data analytics technology adoption and implementation [ 48 ]
The document explores the evolution and current state of Big Data Analytics, highlighting its diverse applications in domains like healthcare and finance.
Researchers have grappled with challenges such as data privacy and scalability, addressing them through innovations like advanced encryption and scalable algorithms.
Looking forward, emerging trends include the integration of Artificial Intelligence and Machine Learning for enhanced analytics and a growing focus on ethics and responsible data use. The intersection of Big Data with edge computing and IoT also opens new frontiers for real-time analytics.
PS30—Security and privacy challenges of Big Data adoption: a qualitative study in telecommunication industry [ 49 ]
The research investigates the evolution of Big Data Analytics applications across diverse domains, emphasizing healthcare, finance, marketing, and telecommunications.
Challenges include data security and privacy, addressed through advanced encryption and privacy-preserving techniques.
In the future, emerging trends highlight explainable AI, ethical data practices, and innovations in handling streaming data, graph databases, and blockchain integration.
PS31—The role of AI, machine learning, and Big Data in digital twinning: a systematic literature review, challenges, and opportunities [ 50 ]
The document explores diverse applications of Big Data Analytics across industries like healthcare, energy, and manufacturing. It underscores the evolution of these applications, highlighting a focus on optimization, diagnostics, and predictive analytics.
Challenges include data collection difficulties, picking the right AI models that are both accurate and fast and the ongoing need for standardization in digital twinning.
The document anticipates future trends, emphasizing the integration of AI, Machine Learning, and Big Data, particularly in digital twinning. It sets the stage for ongoing research in optimizing industrial processes, predictive analytics, healthcare, and smart city implementations.
PS32—The state of the art and taxonomy of Big Data analytics: view from new Big Data framework [ 51 ]
The document extensively explores the landscape of Big Data Analytics, emphasizing the dominant role of Hadoop while acknowledging the rise of Apache Spark in recent years.
Major challenges in the field involve handling diverse data formats, optimizing algorithms for evolving hardware configurations, and bridging the gap between complex systems and end-users through user-friendly visualization techniques.
It anticipates future advancements in applications, specifically in domains like e-commerce and the IoT, while expressing optimism about increased investments in Big Data technology.
In the last 15 years, Big Data has found applications across various domains, evolving over time in line with the evolution of technologies and new business needs. Some of the most common application domains for Big Data Analytics include:
Business and Finance, for example, to detect fraud detection by analyzing large datasets and identifying patterns indicative of fraudulent activities or to study customer behavior, preferences, and trends to improve marketing strategies.
Healthcare, for example, to forecast disease outbreaks, patient admission rates, and treatment outcomes, or to personalize medicine with the analysis of genetic data for ad-hoc treatments.
Retail, for example, to automatically manage and optimize inventories, and stock levels by predicting demands, or to create recommender systems to targeted and segmented customers’ profiles.
Manufacturing, for example, to predict and schedule maintenance needs and potential equipment failures by analyzing sensor data, or to improve product quality by monitoring and analyzing production processes.
Telecommunications, for example, to optimize at real-time network performance and areas for improvement, or to predict customer churn by identifying factors and customers’ behaviors that contribute to customer churn.
Government, Public Services, and Transportation, for example, to plan efficient urban mobility, traffic management, and resource allocation in Smart Cities, or to predict and prevent criminal activities, or to optimize energy distribution and reduce wastage, or to optimize transportation routes, reduce delivery times, and vehicle fleets for efficiency and cost savings.
Media, Entertainment, and Education, for example, to recommend movies, music, or articles based on users’ behaviors and preferences, or to tailor content and advertising by studying users’ behaviors, or to improve educational impact by analyzing student performance.
In Fig. 6 , we show the distribution of the studies addressing the three research questions (RQ1-RQ3), from which we has started initially our investigation: 31 PSs discuss common application domains where the use of Big Data solutions is relevant (RQ1); 30 PSs analyze research challenges and limitations of Big Data (RQ2); 28 PSs highlight emerging research trends and directions in Big Data (RQ3). The total number of papers addressing the 3 RQs is different from the number of the selected 32 PSs, since we observed overlaps and intersections (e.g., a PS can address multiple RQs.)
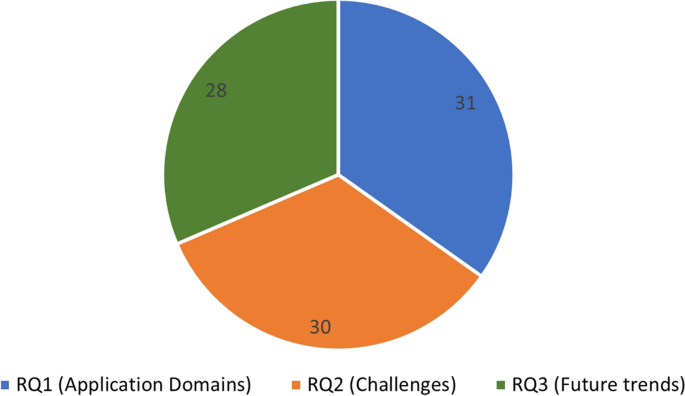
Distribution of studies addressing the three research questions
To better understand the main focus of the PSs, Fig. 7 shows the distribution of studies addressing the three research questions, but this time, we made it avoiding intersections (i.e., each primary study can only be part of one of the 3 categories.) We can classify 12 PSs as papers that mainly focus on RQ1, 10 PSs mainly focus on RQ2, and 10 PSs on RQ3. The homogeneous distribution of the primary studies allows us to be optimistic about the results of our research since we had a good number of studies to answer each of our research questions.
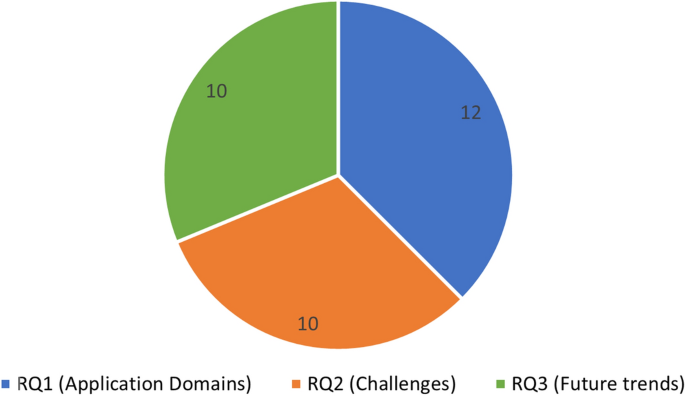
Distribution of studies mainly addressing the three research questions
To further make clear the main focuses of our studies, we decided to categorize each one. Figures 8 , 9 , and 10 show the focus of the documents for each Research Question (note that the sum of the categorized documents may be greater than the number of studies that answer that RQ, because they may overlap and be part of more than one category).
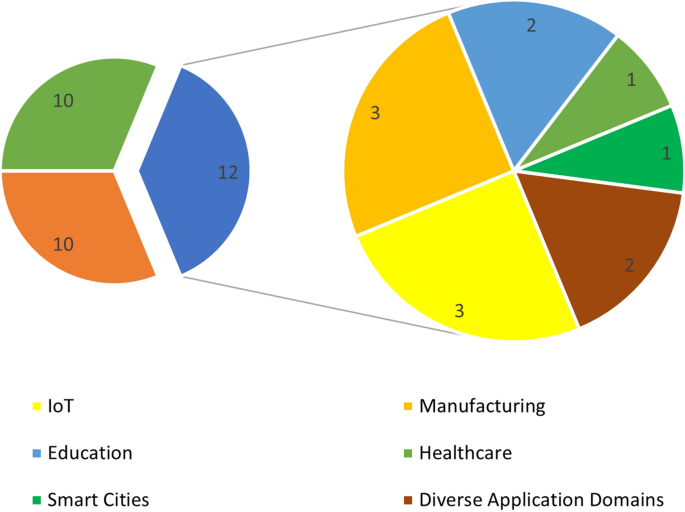
Categorization of RQ1 studies
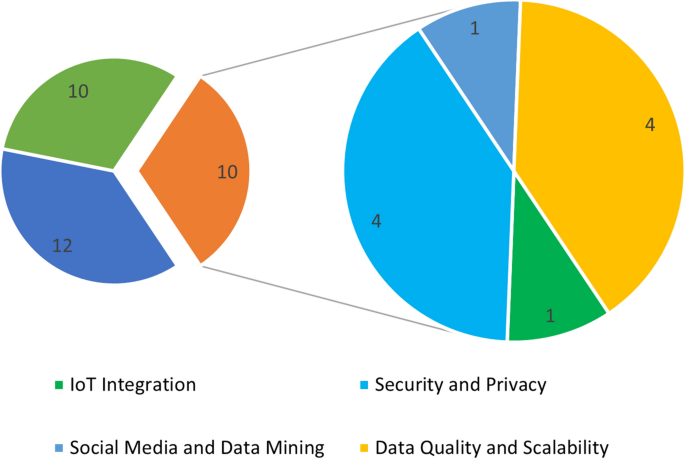
Categorization of RQ2 studies
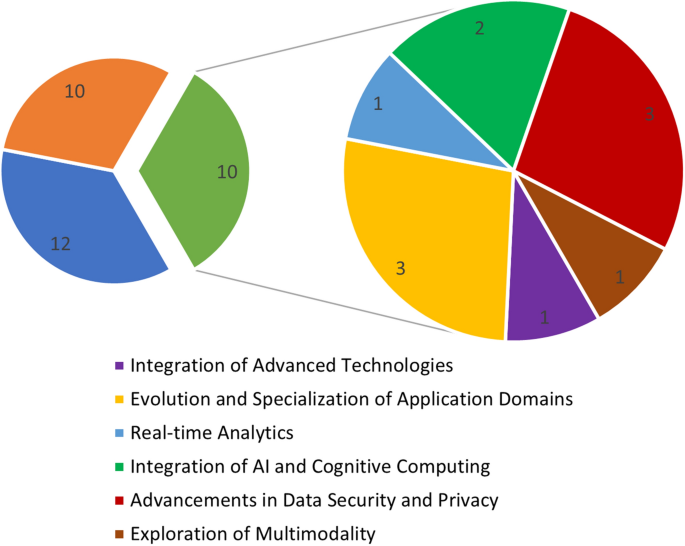
Categorization of RQ3 studies
Having clarified this, we now discuss the findings of our SLR. We divided this discussion in three sections, one for each Research Question, so that we could clearly define which elements answer which question.
RQ1: what are the most common application domains for Big Data analytics, and how have they evolved over time?
Delving into the realm of Big Data across various sectors over the last 15 years reveals a narrative of evolution and adaptation. Initially rooted in finance, healthcare and marketing, the domain of Big Data analytics has undergone a metamorphosis, embracing applications from computational biology to education and manufacturing, expanding into the avant-garde concept of digital twinning. This dynamic evolution is evident in studies investigating Big Data management techniques on the Internet of Things, where the focus has shifted from basic health state monitoring to sophisticated predictive modeling. This evolution signifies a maturation of Big Data analytics, with an increased focus on nuanced attributes like performance, efficiency, reliability, and scalability.
RQ2: what are the major challenges and limitations that researchers have encountered in Big Data analysis, and how have they been addressed?
Shifting our focus to the challenges within the Big Data analytics landscape, a complex history of persistent hurdles and inventive solutions comes into focus. The studies converge on a common thread, unraveling ongoing challenges encapsulated in the trio of data quality, scalability, and privacy/security concerns. Researchers faced with these challenges have become architects of innovative solutions, leveraging advanced algorithms, distributed frameworks, and privacy-preserving techniques. These solutions reflect a commitment to advancing the field in response to the complexities of handling vast and dynamic datasets.
In the implementation of Big Data Analytics, diverse challenges emerge. A dedicated study on industries points to crucial issues in data quality assessment models and secure frameworks. Here, the role of machine learning and data analytics, particularly in fault detection and reservoir management, becomes pivotal. The interconnected nature of these challenges emphasizes the importance of a comprehensive approach to implementation. Beyond technological challenges, ethical considerations surrounding data privacy and security take center stage. Researchers stress the significance of tools addressing ethical concerns, underlining that responsible deployment is intrinsic to the ethical use of Big Data Analytics.
In response to these challenges, the industry advocates for innovative solutions, emphasizing AI-driven approaches, cryptography mechanisms, and lightweight frameworks with AI. This recognition underscores the need for inventive strategies to navigate the intricate integration of Big Data into rapidly evolving technological landscapes.
RQ3: what are the emerging research trends and directions in Big Data that will likely shape the field in the next 5 to 10 years?
Looking into the next 5 to 10 years, several trends are expected to shape the landscape of Big Data Analytics. One significant trend involves making data acquisition more energy-efficient, a move that aligns with broader sustainability goals. The integration of machine learning and deep learning techniques is anticipated to enhance the analytical capabilities of Big Data systems, enabling more accurate predictions and insights. Another noteworthy trend is the emphasis on edge and fog infrastructures, signifying a shift towards decentralized processing for faster data processing and decision-making, especially relevant in the context of the Internet of Things. Importantly, these trends extend beyond technological advancements to include ethical considerations. As Big Data assumes a pivotal role in decision-making processes, these ethical dimensions must be at the forefront. This involves dealing with the tricky ethical issues that come with having such a big influence through data analytics.
In essence, the trajectory of Big Data analytics in the coming years is a dual journey, one that advances technologically with a keen eye on efficiency and, concurrently, prioritizes ethical practices. It’s a future where innovation and responsibility go hand in hand, defining a landscape that reflects both progress and ethical consciousness.
Threats to validity
Ensuring the validity of a SLR is essential for the development of a reliable study. For this reason, in this section, we examine potential threats to construct, internal and external validity, aiming to maintain the robustness of our findings.
Construct validity determines whether the implementation of the SLR aligns with its initial objectives. The efficacy of our search process and the relevance of search terms are crucial concerns. While our search terms were derived from well-defined research questions and adjusted based on that, the completeness and comprehensiveness of these terms may be subject to limitations. Additionally, the use of different keywords might have returned other relevant studies that have not been taken into consideration. A potential language bias may also exist due to the exclusion of non-English articles, representing a limitation that should be acknowledged in the overall validity of the research.
Internal validity assesses the extent to which the design and execution of the study minimize systematic errors. A key focus is on the process of data extraction from the selected primary studies. Some required data may not have been explicitly expressed or were entirely missing, posing a potential threat to internal validity. To minimize this risk, the SLR process has been supervised by another person in order to minimize error into the process.
External validity examines the extent to which the observed effects of the study can be applied beyond its scope. In this SLR, we concentrated on research questions and quality assessments to mitigate the risk of limited generalizability. However, the study’s focus on the specific domain of Big Data research may limit external validity. Moreover, the dynamic nature of Big Data and the predefined time frame (last 15 years) could affect the generalizability of findings. Recognizing these constraints, the outcomes of this SLR are considered generalizable within the specified context of Big Data research.
By acknowledging these potential threats to validity, we strive to enhance the credibility and reliability of our SLR, contributing valuable insights to the evolving landscape of Big Data research.
Over the past 15 years, Big Data has become a crucial player in various fields, adapting to technological shifts and meeting the changing needs of businesses. This review has taken a closer look at how Big Data has been applied, its challenges, and what we can expect in the near future. 189 studies were ultimately found, 32 of which were SLRs analyzed for this study.
Big Data started in areas like Business, Healthcare, and Marketing, but its influence has ultimately grown. Now, it helps predict disease outbreaks, manage retail inventory, forecast equipment failures in manufacturing, improve network performance, optimize urban planning, personalize media content, and enhance education.
Dealing with Big Data hasn’t been without challenges. Issues like ensuring data quality, handling scalability, and maintaining privacy and security have been persistent. Researchers have responded with creative solutions, using advanced algorithms and privacy measures.
Looking to the future, the trends suggest exciting developments. Making data acquisition more energy-efficient and integrating advanced machine learning techniques are on the horizon. There is a shift toward decentralized processing, especially with the Internet of Things in mind. Importantly, these trends aren’t just about technology; they also emphasize ethical considerations. Ethical issues need careful attention as Big Data becomes more influential in decision-making processes.
To summarize, the future of Big Data is a journey that combines technological progress with a strong ethical stance. It’s a path where innovation and responsibility walk hand in hand, shaping a landscape that advances both technologically and ethically. The last 15 years have set the stage and the road ahead invites us to keep exploring and engaging with the ever-evolving world of Big Data.
Data availability
No datasets were generated or analysed during the current study.
Bibliography
Tosi D, Campi AS. How schools affected the covid-19 pandemic in Italy: data analysis for Lombardy Region, Campania Region, and Emilia Region. Future Internet. 2021. https://doi.org/10.3390/fi13050109 .
Article Google Scholar
Davoudian A, Liu M. Big Data systems: a software engineering perspective. ACM Comput Surv. 2020. https://doi.org/10.1145/3408314 .
Kushwaha AK, Kar AK. Language model-driven chatbot for business to address marketing and selection of products. In: Sharma SK, Dwivedi YK, Metri B, Rana NP, editors. Re-imagining diffusion and adoption of information technology and systems: a continuing conversation. Cham: Springer; 2020. p. 16–28. https://doi.org/10.1007/978-3-030-64849-7_3 .
Chapter Google Scholar
Kushwaha AK, Kar AK. Micro-foundations of artificial intelligence adoption in business: making the shift. In: Sharma SK, Dwivedi YK, Metri B, Rana NP, editors. Re-imagining diffusion and adoption of information technology and systems: a continuing conversation. Cham: Springer; 2020. p. 249–60. https://doi.org/10.1007/978-3-030-64849-7_22 .
Dong W, Liao S, Zhang Z. Leveraging financial social media data for corporate fraud detection. J Manag Inf Syst. 2018;35(2):461–87. https://doi.org/10.1080/07421222.2018.1451954 .
Kushwaha AK, Mandal S, Pharswan R, Kar AK, Ilavarasan PV. Studying online political behaviours as rituals: a study of social media behaviour regarding the CAA. In: Sharma SK, Dwivedi YK, Metri B, Rana NP, editors. Re-imagining diffusion and adoption of information technology and systems: a continuing conversation. Cham: Springer; 2020. p. 315–26. https://doi.org/10.1007/978-3-030-64861-9_28 .
Fronzetti Colladon A, Gloor P, Iezzi DF. Editorial introduction: the power of words and networks. Int J Inf Manag. 2020;51: 102031. https://doi.org/10.1016/j.ijinfomgt.2019.10.016 .
Kushwaha AK, Kar AK, Ilavarasan PV. Predicting retweet class using deep learning. In: Piuri V, Raj S, Genovese A, Srivastava R, editors. Trends in deep learning methodologies: hybrid computational intelligence for pattern analysis. Cambridge: Academic Press; 2021. p. 89–112. https://doi.org/10.1016/B978-0-12-822226-3.00004-0 .
Tosi D. Cell phone Big Data to compute mobility scenarios for future smart cities. Int J Data Sci Anal. 2017;4:265–84. https://doi.org/10.1007/s41060-017-0061-2 .
Chen H, Chiang RHL, Storey VC. Business intelligence and analytics: from Big Data to big impact. MIS Q. 2012;36(4):1165–88. https://doi.org/10.2307/41703503 .
Wamba SF, Ngai E, Riggins F, Akter S. Big Data and business analytics adoption and use: a step toward transforming operations and production management? Bingley: Emerald Group Publishing Limited; 2017.
Google Scholar
George G, Osinga EC, Lavie D, Scott BA. Big Data and data science methods for management research. Acad Manag. 2016. https://doi.org/10.5465/amj.2016.4005 .
Curtin J, Kauffman RJ, Riggins FJ. Making the ‘MOST’ out of RFID technology: a research agenda for the study of the adoption, usage and impact of RFID. Inf Technol Manag. 2007;8(2):87–110. https://doi.org/10.1007/s10799-007-0010-1 .
Kitchenham BA, Charters S. Guidelines for performing systematic literature reviews in software engineering. Technical Report EBSE 2007-001, Keele University and Durham University Joint Report. 2007. https://www.elsevier.com/__data/promis_misc/525444systematicreviewsguide.pdf . Accessed 15 Jan 2024
Tosi D, Morasca S. Supporting the semi-automatic semantic annotation of web services: a systematic literature review. Inf Softw Technol. 2015;61:16–32. https://doi.org/10.1016/j.infsof.2015.01.007 .
Tahir A, Tosi D, Morasca S. A systematic review on the functional testing of semantic web services. J Syst Softw. 2013;86(11):2877–89. https://doi.org/10.1016/j.jss.2013.06.064 .
Briner RB, Denyer D. 112 systematic review and evidence synthesis as a practice and scholarship tool. In: Rousseau DM, editor. The Oxford handbook of evidence-based management. Oxford: Oxford University Press; 2012. https://doi.org/10.1093/oxfordhb/9780199763986.013.0007 .
Tosi D, Marzorati S, La Rosa M, Dondossola G, Terruggia R. Big Data from cellular networks: how to estimate energy demand at real-time. In: 2015 IEEE International Conference on Data Science and Advanced Analytics (DSAA), IEEE. 2015. pp. 1–10. https://doi.org/10.1109/DSAA.2015.7344881 .
Cappi R, Casini L, Tosi D, Roccetti M. Questioning the seasonality of SARS-COV-2: a Fourier spectral analysis. BMJ Open. 2022. https://doi.org/10.1136/bmjopen-2022-061602 .
Naghib A, Jafari Navimipour N, Hosseinzadeh M, Sharifi A. A comprehensive and systematic literature review on the Big Data management techniques in the internet of things. Wirel Netw. 2023;29(3):1085–144. https://doi.org/10.1007/s11276-022-03177-5 .
Sarker S, Arefin MS, Kowsher M, Bhuiyan T, Dhar PK, Kwon O-J. A comprehensive review on Big Data for industries: challenges and opportunities. IEEE Access. 2023;11:744–69. https://doi.org/10.1109/ACCESS.2022.3232526 .
Bansal M, Chana I, Clarke S. A survey on IoT Big Data: current status, 13 V’s challenges, and future directions. ACM Comput Surv. 2020. https://doi.org/10.1145/3419634 .
Hordri NF, Samar A, Yuhaniz SS, Shamsuddin SM. A systematic literature review on features of deep learning in Big Data analytics. Int J Adv Soft Comput Appl. 2017;9(1):32–49.
Zhong Y, Chen L, Dan C, Rezaeipanah A. A systematic survey of data mining and Big Data analysis in internet of things. J Supercomput. 2022;78(17):18405–53. https://doi.org/10.1007/s11227-022-04594-1 .
Rashid ANMB. Access methods for Big Data: current status and future directions. EAI Endorsed Trans Scal Inf Syst. 2017;4(15):1–14. https://doi.org/10.4108/eai.28-12-2017.153520 .
O’Donovan P, Leahy K, Bruton K, O’Sullivan DTJ. An industrial Big Data pipeline for data-driven analytics maintenance applications in large-scale smart manufacturing facilities. J Big Data. 2015. https://doi.org/10.1186/s40537-015-0034-z .
Kushwaha AK, Kar AK, Dwivedi YK. Applications of Big Data in emerging management disciplines: a literature review using text mining. Int J Inf Manag Data Insights. 2021. https://doi.org/10.1016/j.jjimei.2021.100017 .
Alkhalil A, Abdallah MAE, Alogali A, Aljaloud A. Applying Big Data analytics in higher education: a systematic mapping study. Int J Inf Commun Technol Educ. 2021;17(3):29–51. https://doi.org/10.4018/IJICTE.20210701.oa3 .
Rahmani AM, Azhir E, Ali S, Mohammadi M, Ahmed OH, Ghafour MY, Ahmed SH, Hosseinzadeh M. Artificial intelligence approaches and mechanisms for Big Data analytics: a systematic study. PeerJ Computer Sci. 2021;7:1–28. https://doi.org/10.7717/peerj-cs.488 .
Lundberg L. Bibliometric mining of research directions and trends for Big Data. J Big Data. 2023. https://doi.org/10.1186/s40537-023-00793-6 .
Baig MI, Shuib L, Yadegaridehkordi E. Big Data adoption: state of the art and research challenges. Inf Process Manag. 2019. https://doi.org/10.1016/j.ipm.2019.102095 .
Ikegwu AC, Nweke HF, Anikwe CV, Alo UR, Okonkwo OR. Big Data analytics for data-driven industry: a review of data sources, tools, challenges, solutions, and research directions. Clust Comput. 2022;25(5):3343–87. https://doi.org/10.1007/s10586-022-03568-5 .
Imran S, Mahmood T, Morshed A, Sellis T. Big Data analytics in healthcare: a systematic literature review and roadmap for practical implementation. IEEE/CAA J Autom Sin. 2021;8(1):1–22. https://doi.org/10.1109/JAS.2020.1003384 .
Zahid H, Mahmood T, Morshed A, Sellis T. Big Data analytics in telecommunications: literature review and architecture recommendations. IEEE/CAA J Autom Sin. 2020;7(1):18–38. https://doi.org/10.1109/JAS.2019.1911795 .
Bazzaz Abkenar S, Haghi Kashani M, Mahdipour E, Jameii SM. Big Data analytics meets social media: a systematic review of techniques, open issues, and future directions. Telemat Inf. 2021. https://doi.org/10.1016/j.tele.2020.101517 .
ElSayed IA, ElDahshan K, Hefny H, ElSayed EK. Big Data and its future in computational biology: a literature review. J Computer Sci. 2021;17(12):1222–8. https://doi.org/10.3844/jcssp.2021.1222.1228 .
Hajiali M. Big Data and sentiment analysis: a comprehensive and systematic literature review. Concurr Comput Pract Exp. 2020. https://doi.org/10.1002/cpe.5671 .
Ahmadova U, Mustafayev M, Kiani Kalejahi B, Saeedvand S, Rahmani AM. Big Data applications on the internet of things: a systematic literature review. Int J Commun Syst. 2021. https://doi.org/10.1002/dac.5004 .
Baig MI, Shuib L, Yadegaridehkordi E. Big Data in education: a state of the art, limitations, and future research directions. Int J Educ Technol High Educ. 2020;17(1):1–23.
Reshi AA, Shah ARIF, Shafi S, Qadri MH. Big Data in healthcare a comprehensive bibliometric analysis of current research trends. Scal Comput. 2023;24(3):531–49. https://doi.org/10.12694/scpe.v24i3.2155 .
Pulikottil T, Estrada-Jimenez LA, Abadía JJP, Carrera-Rivera A, Torayev A, Rehman HU, Mo F, Nikghadam-Hojjati S, Barata J. Big Data life cycle in shop-floor-trends and challenges. IEEE Access. 2023;11:30008–26. https://doi.org/10.1109/ACCESS.2023.3253286 .
Arshad I, Alsamhi SH, Afzal W. Big Data testing techniques: taxonomy, challenges and future trends. Computers Mater Contin. 2023;74(2):2739–70. https://doi.org/10.32604/cmc.2023.030266 .
Gupta S, Kar AK, Baabdullah A, Al-Khowaiter WAA. Big Data with cognitive computing: a review for the future. Int J Inf Manag. 2018;42:78–89. https://doi.org/10.1016/j.ijinfomgt.2018.06.005 .
Saltz JS, Krasteva I. Current approaches for executing Big Data science projects-a systematic literature review. PeerJ Computer Sci. 2022. https://doi.org/10.7717/PEERJ-CS.862 .
Liu C, Peng G, Kong Y, Li S, Chen S. Data quality affecting Big Data analytics in smart factories: research themes, issues and methods. Symmetry. 2021. https://doi.org/10.3390/sym13081440 .
Ahmed A, Xi R, Hou M, Shah SA, Hameed S. Harnessing Big Data analytics for healthcare: a comprehensive review of frameworks, implications, applications, and impacts. IEEE Access. 2023;11:112891–928. https://doi.org/10.1109/ACCESS.2023.3323574 .
Karimi Y, Haghi Kashani M, Akbari M, Mahdipour E. Leveraging Big Data in smart cities: a systematic review. Concurr Comput Pract Exp. 2021. https://doi.org/10.1002/cpe.6379 .
Gong Y, Janssen M. Roles and capabilities of enterprise architecture in Big Data analytics technology adoption and implementation. J Theor Appl Electron Commer Res. 2021;16(1):37–51. https://doi.org/10.4067/S0718-18762021000100104 .
Anawar S, Othman NF, Selamat SR, Ayop Z, Harum N, Rahim FA. Security and privacy challenges of Big Data adoption: a qualitative study in telecommunication industry. Int J Interact Mob Technol. 2022;16(19):81–97. https://doi.org/10.3991/ijim.v16i19.32093 .
Rathore MM, Shah SA, Shukla D, Bentafat E, Bakiras S. The role of AI, machine learning, and Big Data in digital twinning: a systematic literature review, challenges, and opportunities. IEEE Access. 2021;9:32030–52. https://doi.org/10.1109/ACCESS.2021.3060863 .
Mohamed A, Najafabadi MK, Wah YB, Zaman EAK, Maskat R. The state of the art and taxonomy of Big Data analytics: view from new Big Data framework. Artif Intell Rev. 2020;53(2):989–1037. https://doi.org/10.1007/s10462-019-09685-9 .
Li Y, Liu Z, Zhu H. Enterprise search in the Big Data era: recent developments and open challenges. Proc VLDB Endow. 2014;7(13):1717–8. https://doi.org/10.14778/2733004.2733071 .
Lee D, Camacho D, Jung JJ. Smart mobility with Big Data: approaches, applications, and challenges. Appl Sci. 2023. https://doi.org/10.3390/app13127244 .
Himeur Y, Elnour M, Fadli F, Meskin N, Petri I, Rezgui Y, Bensaali F, Amira A. AI-Big Data analytics for building automation and management systems: a survey, actual challenges and future perspectives. Artif Intell Rev. 2023;56(6):4929–5021. https://doi.org/10.1007/s10462-022-10286-2 .
Cesario E. Big Data analytics and smart cities: applications, challenges, and opportunities. Front Big Data. 2023. https://doi.org/10.3389/fdata.2023.1149402 .
Zwilling M. Big Data challenges in social sciences: an NLP analysis. J Computer Inf Syst. 2023;63(3):537–54. https://doi.org/10.1080/08874417.2022.2085211 .
Rani R, Khurana M, Kumar A, Kumar N. Big Data dimensionality reduction techniques in IoT: review, applications and open research challenges. Clust Comput. 2022;25(6):4027–49. https://doi.org/10.1007/s10586-022-03634-y .
Jagatheesaperumal SK, Rahouti M, Ahmad K, Al-Fuqaha A, Guizani M. The duo of artificial intelligence and Big Data for industry 4.0: applications, techniques, challenges, and future research directions. IEEE Internet Things J. 2022;9(15):12861–85. https://doi.org/10.1109/JIOT.2021.3139827 .
Lundberg L, Grahn H. Research trends, enabling technologies and application areas for Big Data. Algorithms. 2022. https://doi.org/10.3390/a15080280 .
Ali TAL, Khafagy MH, Farrag MH. Big Data challenges: preserving techniques for privacy violations. J Theor Appl Inf Technol. 2022;100(8):2505–17.
Latifian A. How does cloud computing help businesses to manage Big Data issues. Kybernetes. 2022;51(6):1917–48. https://doi.org/10.1108/K-05-2021-0432 .
Rehman A, Naz S, Razzak I. Leveraging Big Data analytics in healthcare enhancement: trends, challenges and opportunities. Multimed Syst. 2022;28(4):1339–71. https://doi.org/10.1007/s00530-020-00736-8 .
Al-Zahrani A, Al-Hebbi M. Big Data major security issues: challenges and defense strategies. Tehnicki Glasnik. 2022;16(2):197–204. https://doi.org/10.31803/tg-20220124135330 .
Song X, Zhang H, Akerkar R, Huang H, Guo S, Zhong L, Ji Y, Opdahl AL, Purohit H, Skupin A, Pottathil A, Culotta A. Big Data and emergency management: concepts, methodologies, and applications. IEEE Trans Big Data. 2022;8(2):397–419. https://doi.org/10.1109/TBDATA.2020.2972871 .
Singh N, Singh DP, Pant B. Big Data knowledge discovery as a service: recent trends and challenges. Wirel Pers Commun. 2022;123(2):1789–807. https://doi.org/10.1007/s11277-021-09213-5 .
Mohammadi E, Karami A. Exploring research trends in Big Data across disciplines: a text mining analysis. J Inf Sci. 2022;48(1):44–56. https://doi.org/10.1177/0165551520932855 .
Ambeth Kumar VD, Varadarajan V, Gupta MK, Rodrigues JJPC, Janu N. AI empowered Big Data analytics for industrial applications. J Univers Computer Sci. 2022;28(9):877–81. https://doi.org/10.3897/jucs.94155 .
Kumari S, Muthulakshmi P. Transformative effects of Big Data on advanced data analytics: open issues and critical challenges. J Computer Sci. 2022;18(6):463–79. https://doi.org/10.3844/jcssp.2022.463.479 .
Tang S, He B, Yu C, Li Y, Li K. A survey on spark ecosystem: Big Data processing infrastructure, machine learning, and applications. IEEE Trans Knowl Data Eng. 2022;34(1):71–91. https://doi.org/10.1109/TKDE.2020.2975652 .
Reyes-Veras PF, Renukappa S, Suresh S. Challenges faced by the adoption of Big Data in the Dominican Republic construction industry: an empirical study. J Inf Technol Constr. 2021;26:812–31. https://doi.org/10.36680/J.ITCON.2021.044 .
Bentotahewa V, Hewage C, Williams J. Solutions to Big Data privacy and security challenges associated with COVID-19 surveillance systems. Front Big Data. 2021. https://doi.org/10.3389/fdata.2021.645204 .
Escobar CA, McGovern ME, Morales-Menendez R. Quality 4.0: a review of Big Data challenges in manufacturing. J Intell Manuf. 2021;32(8):2319–34. https://doi.org/10.1007/s10845-021-01765-4 .
Mwitondi KS, Said RA. Dealing with randomness and concept drift in large datasets. Data. 2021. https://doi.org/10.3390/data6070077 .
Kusal S, Patil S, Kotecha K, Aluvalu R, Varadarajan V. Ai based emotion detection for textual Big Data: techniques and contribution. Big Data Cognit Comput. 2021. https://doi.org/10.3390/bdcc5030043 .
Lee E, Jang J. Research trend analysis for sustainable QR code use: focus on Big Data analysis. KSII Trans Internet Inf Syst. 2021;15(9):3221–42. https://doi.org/10.3837/tiis.2021.09.008 .
Rhahla M, Allegue S, Abdellatif T. Guidelines for GDPR compliance in Big Data systems. J Inf Secur Appl. 2021. https://doi.org/10.1016/j.jisa.2021.102896 .
Amović M, Govedarica M, Radulović A, Janković I. Big Data in smart city: management challenges. Appl Sci. 2021. https://doi.org/10.3390/app11104557 .
Hoozemans J, Peltenburg J, Nonnemacher F, Hadnagy A, Al-Ars Z, Hofstee HP. FPGA acceleration for Big Data analytics: challenges and opportunities. IEEE Circuits Syst Mag. 2021;21(2):30–47. https://doi.org/10.1109/MCAS.2021.3071608 .
Jalali SMJ, Park HW, Vanani IR, Pho K-H. Research trends on Big Data domain using text mining algorithms. Digit Scholarsh Hum. 2021;36(2):361–70. https://doi.org/10.1093/llc/fqaa012 .
Almutairi MM. Role of Big Data in education in KSA. Int J Inf Technol. 2021;13(1):367–73. https://doi.org/10.1007/s41870-020-00489-7 .
Ardagna D, Barbierato E, Gianniti E, Gribaudo M, Pinto TBM, Silva APC, Almeida JM. Predicting the performance of Big Data applications on the cloud. J Supercomput. 2021;77(2):1321–53. https://doi.org/10.1007/s11227-020-03307-w .
Mkrttchian V, Gamidullaeva L, Finogeev A, Chernyshenko S, Chernyshenko V, Amirov D, Potapova I. Big Data and internet of things (IoT) technologies’ influence on higher education: current state and future prospects. Int J Web-Based Learn Teach Technol. 2021;16(5):137–57. https://doi.org/10.4018/IJWLTT.20210901.oa8 .
Mourtzis D. Towards the 5th industrial revolution: a literature review and a framework for process optimization based on Big Data analytics and semantics. J Mach Eng. 2021;21(3):5–39. https://doi.org/10.36897/jme/141834 .
Dias MNR, Hassan S, Shahzad A. The impact of Big Data utilization on Malaysian government hospital healthcare performance. Int J eBus eGov Stud. 2021;13(1):50–77. https://doi.org/10.34111/ijebeg.202113103 .
Babar M, Alshehri MD, Tariq MU, Ullah F, Khan A, Uddin MI, Almasoud AS. IoT-enabled Big Data analytics architecture for multimedia data communications. Wirel Commun Mob Comput. 2021. https://doi.org/10.1155/2021/5283309 .
Bhat SA, Huang N-F. Big Data and AI revolution in precision agriculture: survey and challenges. IEEE Access. 2021;9:110209–22. https://doi.org/10.1109/ACCESS.2021.3102227 .
Zainab A, Ghrayeb A, Syed D, Abu-Rub H, Refaat SS, Bouhali O. Big Data management in smart grids: technologies and challenges. IEEE Access. 2021;9:73046–59. https://doi.org/10.1109/ACCESS.2021.3080433 .
Jabir B, Falih N. Big Data analytics opportunities and challenges for the smart enterprise. Int J Tech Phys Probl Eng. 2021;13(2):20–6.
Zineb EF, Najat R, Jaafar A. An intelligent approach for data analysis and decision making in Big Data: a case study on e-commerce industry. Int J Adv Computer Sci Appl. 2021;12(7):723–36. https://doi.org/10.14569/IJACSA.2021.0120783 .
Syed D, Zainab A, Ghrayeb A, Refaat SS, Abu-Rub H, Bouhali O. Smart grid Big Data analytics: survey of technologies, techniques, and applications. IEEE Access. 2021;9:59564–85. https://doi.org/10.1109/ACCESS.2020.3041178 .
Talebkhah M, Sali A, Marjani M, Gordan M, Hashim SJ, Rokhani FZ. IoT and Big Data applications in smart cities: recent advances, challenges, and critical issues. IEEE Access. 2021;9:55465–84. https://doi.org/10.1109/ACCESS.2021.3070905 .
Dubuc T, Stahl F, Roesch EB. Mapping the Big Data landscape: technologies, platforms and paradigms for real-time analytics of data streams. IEEE Access. 2021;9:15351–74. https://doi.org/10.1109/ACCESS.2020.3046132 .
Ang KL-M, Seng JKP. Big Data and machine learning with hyperspectral information in agriculture. IEEE Access. 2021;9:36699–718. https://doi.org/10.1109/ACCESS.2021.3051196 .
Zeadally S, Siddiqui F, Baig Z, Ibrahim A. Smart healthcare: challenges and potential solutions using internet of things (IoT) and Big Data analytics. PSU Res Rev. 2020;4(2):149–68. https://doi.org/10.1108/PRR-08-2019-0027 .
Thudumu S, Branch P, Jin J, Singh JJ. A comprehensive survey of anomaly detection techniques for high dimensional Big Data. J Big Data. 2020. https://doi.org/10.1186/s40537-020-00320-x .
Trang NH. Limitations of Big Data partitions technology. J Appl Data Sci. 2020;1(1):11–9. https://doi.org/10.47738/jads.v1i1.7 .
Caíno-Lores S, Lapin A, Carretero J, Kropf P. Applying Big Data paradigms to a large scale scientific workflow: lessons learned and future directions. Future Gener Computer Syst. 2020;110:440–52. https://doi.org/10.1016/j.future.2018.04.014 .
Awaysheh FM, Alazab M, Gupta M, Pena TF, Cabaleiro JC. Next-generation Big Data federation access control: a reference model. Future Gener Computer Syst. 2020;108:726–41. https://doi.org/10.1016/j.future.2020.02.052 .
Valencia-Parra A, Varela-Vaca AJ, Parody L, Gomez-Lopez MT. Unleashing constraint optimisation problem solving in Big Data environments. J Comput Sci. 2020. https://doi.org/10.1016/j.jocs.2020.101180 .
Article MathSciNet Google Scholar
López-Martínez F, Núñez-Valdez ER, García-Díaz V, Bursac Z. A case study for a Big Data and machine learning platform to improve medical decision support in population health management. Algorithms. 2020. https://doi.org/10.3390/A13040102 .
Iqbal R, Doctor F, More B, Mahmud S, Yousuf U. Big Data analytics and computational intelligence for cyber-physical systems: recent trends and state of the art applications. Future Gener Computer Syst. 2020;105:766–78. https://doi.org/10.1016/j.future.2017.10.021 .
Carnevale L, Celesti A, Fazio M, Villari M. A Big Data analytics approach for the development of advanced cardiology applications. Information. 2020. https://doi.org/10.3390/info11020060 .
Shukla AK, Muhuri PK, Abraham A. A bibliometric analysis and cutting-edge overview on fuzzy techniques in Big Data. Eng Appl Artif Intell. 2020. https://doi.org/10.1016/j.engappai.2020.103625 .
Karim A, Siddiqa A, Safdar Z, Razzaq M, Gillani SA, Tahir H, Kiran S, Ahmed E, Imran M. Big Data management in participatory sensing: issues, trends and future directions. Future Gener Computer Syst. 2020;107:942–55. https://doi.org/10.1016/j.future.2017.10.007 .
Humayun M. Role of emerging IoT Big Data and cloud computing for real time application. Int J Adv Computer Sci Appl. 2020;11(4):494–506.
Rabanal F, Martínez C. Cryptography for Big Data environments: current status, challenges, and opportunities. Comput Math Methods. 2020. https://doi.org/10.1002/cmm4.1075 .
Ramesh T, Santhi V. Exploring Big Data analytics in health care. Int J Intell Netw. 2020;1:135–40. https://doi.org/10.1016/j.ijin.2020.11.003 .
Gautam A, Chatterjee I. Big Data and cloud computing: a critical review. Int J Oper Res Inf Syst. 2020;11(3):19–38. https://doi.org/10.4018/IJORIS.2020070102 .
Bajaber F, Sakr S, Batarfi O, Altalhi A, Barnawi A. Benchmarking Big Data systems: a survey. Computer Commun. 2020;149:241–51. https://doi.org/10.1016/j.comcom.2019.10.002 .
Maksimov P, Koiranen T. Application of novel Big Data processing techniques in process industries. Int J Computer Appl Technol. 2020;62(3):200–15. https://doi.org/10.1504/IJCAT.2020.106591 .
Dash S, Shakyawar SK, Sharma M, Kaushik S. Big Data in healthcare: management, analysis and future prospects. J Big Data. 2019. https://doi.org/10.1186/s40537-019-0217-0 .
Nagalakshmi N, Anand Babu GL, Reddy KS, Ashalatha T. Security challenges associated with Big Data in health care system. Int J Eng Adv Technol. 2019;9(1):4057–60. https://doi.org/10.35940/ijeat.A1296.109119 .
Dai H-N, Wong RC-W, Wang H, Zheng Z, Vasilakos AV. Big Data analytics for large-scale wireless networks: challenges and opportunities. ACM Comput Surv. 2019. https://doi.org/10.1145/3337065 .
Barika M, Garg S, Zomaya AY, Wang L, Moorsel AVAN, Ranjan R. Orchestrating Big Data analysis workflows in the cloud: research challenges, survey, and future directions. ACM Comput Surv. 2019. https://doi.org/10.1145/3332301 .
Hariri RH, Fredericks EM, Bowers KM. Uncertainty in Big Data analytics: survey, opportunities, and challenges. J Big Data. 2019. https://doi.org/10.1186/s40537-019-0206-3 .
Latif Z, Lei W, Latif S, Pathan ZH, Ullah R, Jianqiu Z. Big Data challenges: prioritizing by decision-making process using analytic network process technique. Multimed Tools Appl. 2019;78(19):27127–53. https://doi.org/10.1007/s11042-017-5161-4 .
Kumari A, Tanwar S, Tyagi S, Kumar N. Verification and validation techniques for streaming Big Data analytics in internet of things environment. IET Netw. 2019;8(3):155–63. https://doi.org/10.1049/iet-net.2018.5187 .
Singh SP, Nayyar A, Kumar R, Sharma A. Fog computing: from architecture to edge computing and Big Data processing. J Supercomput. 2019;75(4):2070–105. https://doi.org/10.1007/s11227-018-2701-2 .
Raufi B, Ismaili F, Ajdari J, Zenuni X. Web personalization issues in Big Data and semantic web: challenges and opportunities. Turk J Electr Eng Computer Sci. 2019;27(4):2379–94. https://doi.org/10.3906/elk-1812-25 .
Rahman NA, Nor NM. Healthcare using social media: Big Data analytics perspective. J Adv Res Dyn Control Syst. 2019;11(8 Special Issue):1169–79.
Mishra S, Pattnaik S, Mishra BB. Application of Big Data analysis in supply chain management: future challenges. J Adv Res Dyn Control Syst. 2019;11(8 Special Issue):2541–8.
Ivanovic M, Klasnja-Milicevic A. Big Data and collective intelligence. Int J Embed Syst. 2019;11(5):573–83. https://doi.org/10.1504/IJES.2019.102430 .
Qolomany B, Al-Fuqaha A, Gupta A, Benhaddou D, Alwajidi S, Qadir J, Fong AC. Leveraging machine learning and Big Data for smart buildings: a comprehensive survey. IEEE Access. 2019;7:90316–56. https://doi.org/10.1109/ACCESS.2019.2926642 .
Shah SA, Seker DZ, Hameed S, Draheim D. The rising role of Big Data analytics and IoT in disaster management: recent advances, taxonomy and prospects. IEEE Access. 2019;7:54595–614. https://doi.org/10.1109/ACCESS.2019.2913340 .
Lin W, Zhang Z, Peng S. Academic research trend analysis based on Big Data technology. Int J Comput Sci Eng. 2019;20(1):31–9. https://doi.org/10.1504/ijcse.2019.103247 .
Hong L, Luo M, Wang R, Lu P, Lu W, Lu L. Big Data in health care: applications and challenges. Data Inf Manag. 2018;2(3):175–97. https://doi.org/10.2478/dim-2018-0014 .
Pal D, Triyason T, Padungweang P. Big Data in smart-cities: current research and challenges. Indones J Electr Eng Inf. 2018;6(4):351–60. https://doi.org/10.11591/ijeei.v6i4.543 .
Li N, Mahalik NP. A Big Data and cloud computing specification, standards and architecture: agricultural and food informatics. Int J Inf Commun Technol. 2019;14(2):159–74. https://doi.org/10.1504/IJICT.2019.097687 .
Chiroma H, Abdullahi UA, Abdulhamid SM, Abdulsalam Alarood A, Gabralla LA, Rana N, Shuib L, Targio Hashem IA, Gbenga DE, Abubakar AI, Zeki AM, Herawan T. Progress on artificial neural networks for Big Data analytics: a survey. IEEE Access. 2019;7:70535–51. https://doi.org/10.1109/ACCESS.2018.2880694 .
Waheed H, Hassan S-U, Aljohani NR, Wasif M. A bibliometric perspective of learning analytics research landscape. Behav Inf Technol. 2018;37(10–11):941–57. https://doi.org/10.1080/0144929X.2018.1467967 .
Ray J, Johnny O, Trovati M, Sotiriadis S, Bessis N. The rise of Big Data science: a survey of techniques, methods and approaches in the field of natural language processing and network theory. Big Data Cognit Comput. 2018;2(3):1–18. https://doi.org/10.3390/bdcc2030022 .
Mantelero A. AI and Big Data: a blueprint for a human rights, social and ethical impact assessment. Computer Law Secur Rev. 2018;34(4):754–72. https://doi.org/10.1016/j.clsr.2018.05.017 .
Sultan K, Ali H, Zhang Z. Big Data perspective and challenges in next generation networks. Future Internet. 2018. https://doi.org/10.3390/fi10070056 .
Li Q, Chen Y, Wang J, Chen Y, Chen H. Web media and stock markets : a survey and future directions from a Big Data perspective. IEEE Trans Knowl Data Eng. 2018;30(2):381–99. https://doi.org/10.1109/TKDE.2017.2763144 .
Jabbar S, Malik KR, Ahmad M, Aldabbas O, Asif M, Khalid S, Han K, Ahmed SH. A methodology of real-time data fusion for localized Big Data analytics. IEEE Access. 2018;6:24510–20. https://doi.org/10.1109/ACCESS.2018.2820176 .
Darwish TSJ, Abu Bakar K. Fog based intelligent transportation Big Data analytics in the internet of vehicles environment: motivations, architecture, challenges, and critical issues. IEEE Access. 2018;6:15679–701. https://doi.org/10.1109/ACCESS.2018.2815989 .
Zheng S, Chen S, Yang L, Zhu J, Luo Z, Hu J, Yang X. Big Data processing architecture for radio signals empowered by deep learning: concept, experiment, applications and challenges. IEEE Access. 2018;6:55907–22. https://doi.org/10.1109/ACCESS.2018.2872769 .
Stefanowski J, Krawiec K, Wrembel R. Exploring complex and Big Data. Int J Appl Math Computer Sci. 2017;27(4):669–79. https://doi.org/10.1515/amcs-2017-0046 .
Harerimana G, Jang B, Kim JW, Park HK. Health Big Data analytics: a technology survey. IEEE Access. 2018;6:65661–78. https://doi.org/10.1109/ACCESS.2018.2878254 .
Ravi S, Jeyaprakash T. Combined ideas on the necessity of Big Data on internet of things and researchers point of view and its challenges, future directions. J Adv Res Dyn Control Syst. 2018;10(9 Special Issue):2140–4.
Neggers J, Allix O, Hild F, Roux S. Big Data in experimental mechanics and model order reduction: today’s challenges and tomorrow’s opportunities. Arch Comput Methods Eng. 2018;25(1):143–64. https://doi.org/10.1007/s11831-017-9234-3 .
Khan S, Liu X, Shakil KA, Alam M. A survey on scholarly data: from Big Data perspective. Inf Process Manag. 2017;53(4):923–44. https://doi.org/10.1016/j.ipm.2017.03.006 .
Costa C, Santos MY. Big Data: state-of-the-art concepts, techniques, technologies, modeling approaches and research challenges. IAENG Int J Computer Sci. 2017;44(3):285–301.
Lv Z, Song H, Basanta-Val P, Steed A, Jo M. Next-generation Big Data analytics: state of the art, challenges, and future research topics. IEEE Trans Ind Inf. 2017;13(4):1891–9. https://doi.org/10.1109/TII.2017.2650204 .
Memon MA, Soomro S, Jumani AK, Kartio MA. Big Data analytics and its applications. Ann Emerg Technol Comput. 2017;1(1):45–54. https://doi.org/10.33166/AETiC.2017.01.006 .
Mantelero A. Regulating Big Data The guidelines of the Council of Europe in the context of the European data protection framework. Computer Law Secur Rev. 2017;33(5):584–602. https://doi.org/10.1016/j.clsr.2017.05.011 .
Zhou L, Pan S, Wang J, Vasilakos AV. Machine learning on Big Data: opportunities and challenges. Neurocomputing. 2017;237:350–61. https://doi.org/10.1016/j.neucom.2017.01.026 .
Yan J, Meng Y, Lu L, Li L. Industrial Big Data in an industry 4.0 environment: challenges, schemes, and applications for predictive maintenance. IEEE Access. 2017;5:23484–91. https://doi.org/10.1109/ACCESS.2017.2765544 .
Gonçalves ME. The EU data protection reform and the challenges of Big Data: remaining uncertainties and ways forward. Inf Commun Technol Law. 2017;26(2):90–115. https://doi.org/10.1080/13600834.2017.1295838 .
Peng S, Wang G, Xie D. Social influence analysis in social networking Big Data: opportunities and challenges. IEEE Netw. 2017;31(1):11–7. https://doi.org/10.1109/MNET.2016.1500104NM .
L’Heureux A, Grolinger K, Elyamany HF, Capretz MAM. Machine learning with Big Data: challenges and approaches. IEEE Access. 2017;5:7776–97. https://doi.org/10.1109/ACCESS.2017.2696365 .
Manikyam NRH, Mohan Kumar S. Methods and techniques to deal with Big Data analytics and challenges in cloud computing environment. Int J Civil Eng Technol. 2017;8(4):669–78.
El-Seoud SA, El-Sofany HF, Abdelfattah M, Mohamed R. Big Data and cloud computing: trends and challenges. Int J Interact Mob Technol. 2017;11(2):34–52. https://doi.org/10.3991/ijim.v11i2.6561 .
Zúñiga H, Diehl T. Citizenship, social media, and Big Data: current and future research in the social sciences. Soc Sci Computer Rev. 2017;35(1):3–9. https://doi.org/10.1177/0894439315619589 .
Wang H, Xu Z, Pedrycz W. An overview on the roles of fuzzy set techniques in Big Data processing: trends, challenges and opportunities. Knowl-Based Syst. 2017;118:15–30. https://doi.org/10.1016/j.knosys.2016.11.008 .
Choi T-M, Chan HK, Yue X. Recent development in Big Data analytics for business operations and risk management. IEEE Trans Cybern. 2017;47(1):81–92. https://doi.org/10.1109/TCYB.2015.2507599 .
Zhong RY, Newman ST, Huang GQ, Lan S. Big Data for supply chain management in the service and manufacturing sectors: challenges, opportunities, and future perspectives. Comput Ind Eng. 2016;101:572–91. https://doi.org/10.1016/j.cie.2016.07.013 .
Bajaber F, Elshawi R, Batarfi O, Altalhi A, Barnawi A, Sakr S. Big Data 2.0 processing systems: taxonomy and open challenges. J Grid Comput. 2016;14(3):379–405. https://doi.org/10.1007/s10723-016-9371-1 .
De Gennaro M, Paffumi E, Martini G. Big Data for supporting low-carbon road transport policies in europe: applications, challenges and opportunities. Big Data Res. 2016;6:11–25. https://doi.org/10.1016/j.bdr.2016.04.003 .
Wang H, Xu Z, Fujita H, Liu S. Towards felicitous decision making: an overview on challenges and trends of Big Data. Inf Sci. 2016;367–368:747–65. https://doi.org/10.1016/j.ins.2016.07.007 .
Rodríguez-Mazahua L, Rodríguez-Enríquez C-A, Sánchez-Cervantes JL, Cervantes J, García-Alcaraz JL, Alor-Hernández G. A general perspective of Big Data: applications, tools, challenges and trends. J Supercomput. 2016;72(8):3073–113. https://doi.org/10.1007/s11227-015-1501-1 .
Bello-Orgaz G, Jung JJ, Camacho D. Social Big Data: recent achievements and new challenges. Inf Fus. 2016;28:45–59. https://doi.org/10.1016/j.inffus.2015.08.005 .
Zheng X, Chen W, Wang P, Shen D, Chen S, Wang X, Zhang Q, Yang L. Big Data for social transportation. IEEE Trans Intell Transp Syst. 2016;17(3):620–30. https://doi.org/10.1109/TITS.2015.2480157 .
Sahay S. Big Data and public health: challenges and opportunities for low and middle income countries. Commun Assoc Inf Syst. 2016;39(1):419–38. https://doi.org/10.17705/1cais.03920 .
Sharma N, Namratha B. Towards addressing the challenges of data intensive computing in Big Data analytics. Int J Control Theor Appl. 2016;9(23):57–62.
Yu S. Big privacy: challenges and opportunities of privacy study in the age of Big Data. IEEE Access. 2016;4:2751–63. https://doi.org/10.1109/ACCESS.2016.2577036 .
Chen C-M. Use cases and challenges in telecom Big Data analytics. APSIPA Trans Signal Inf Process. 2016. https://doi.org/10.1017/ATSIP.2016.20 .
Anagnostopoulos I, Zeadally S, Exposito E. Handling Big Data: research challenges and future directions. J Supercomput. 2016;72(4):1494–516. https://doi.org/10.1007/s11227-016-1677-z .
Jothi B, Pushpalatha M, Krishnaveni S. Significance and challenges in Big Data: a survey. Int J Control Theor Appl. 2016;9(34):235–43.
Huang Y, Schuehle J, Porter AL, Youtie J. A systematic method to create search strategies for emerging technologies based on the Web of Science: illustrated for ‘Big Data’. Scientometrics. 2015;105(3):2005–22. https://doi.org/10.1007/s11192-015-1638-y .
Xu Z, Shi Y. Exploring Big Data analysis: fundamental scientific problems. Ann Data Sci. 2015;2(4):363–72. https://doi.org/10.1007/s40745-015-0063-7 .
Olshannikova E, Ometov A, Koucheryavy Y, Olsson T. Visualizing Big Data with augmented and virtual reality: challenges and research agenda. J Big Data. 2015. https://doi.org/10.1186/s40537-015-0031-2 .
Assunção MD, Calheiros RN, Bianchi S, Netto MAS, Buyya R. Big Data computing and clouds: trends and future directions. J Parallel Distrib Comput. 2015;79–80:3–15. https://doi.org/10.1016/j.jpdc.2014.08.003 .
Najafabadi MM, Villanustre F, Khoshgoftaar TM, Seliya N, Wald R, Muharemagic E. Deep learning applications and challenges in Big Data analytics. J Big Data. 2015. https://doi.org/10.1186/s40537-014-0007-7 .
Nativi S, Mazzetti P, Santoro M, Papeschi F, Craglia M, Ochiai O. Big Data challenges in building the global earth observation system of systems. Environ Model Softw. 2015;68:1–26. https://doi.org/10.1016/j.envsoft.2015.01.017 .
Tian X, Han R, Wang L, Lu G, Zhan J. Latency critical Big Data computing in finance. J Finance Data Sci. 2015;1(1):33–41. https://doi.org/10.1016/j.jfds.2015.07.002 .
Perera C, Ranjan R, Wang L, Khan SU, Zomaya AY. Big Data privacy in the internet of things era. IT Prof. 2015;17(3):32–9. https://doi.org/10.1109/MITP.2015.34 .
Jin X, Wah BW, Cheng X, Wang Y. Significance and challenges of Big Data research. Big Data Res. 2015;2(2):59–64. https://doi.org/10.1016/j.bdr.2015.01.006 .
Mao R, Xu H, Wu W, Li J, Li Y, Lu M. Overcoming the challenge of variety: Big Data abstraction, the next evolution of data management for AAL communication systems. IEEE Commun Mag. 2015;53(1):42–7. https://doi.org/10.1109/MCOM.2015.7010514 .
Philip Chen CL, Zhang C-Y. Data-intensive applications, challenges, techniques and technologies: a survey on Big Data. Inf Sci. 2014;275:314–47. https://doi.org/10.1016/j.ins.2014.01.015 .
Ma Y, Wu H, Wang L, Huang B, Ranjan R, Zomaya A, Jie W. Remote sensing Big Data computing: challenges and opportunities. Future Gener Computer Syst. 2015;51:47–60. https://doi.org/10.1016/j.future.2014.10.029 .
Jeong SR, Ghani I. Semantic computing for Big Data: approaches, tools, and emerging directions (2011–2014). KSII Trans Internet Inf Syst. 2014;8(6):2022–42. https://doi.org/10.3837/tiis.2014.06.012 .
Sun D, Liu C, Ren D. Prospects, challenges and latest developments in designing a scalable Big Data stream computing system. Int J Wirel Mob Comput. 2015;9(2):155–60. https://doi.org/10.1504/IJWMC.2015.072567 .
Dobre C, Xhafa F. Intelligent services for Big Data science. Future Gener Computer Syst. 2014;37:267–81. https://doi.org/10.1016/j.future.2013.07.014 .
Qin HF, Li ZH. Research on the method of Big Data analysis. Inf Technol J. 2013;12(10):1974–80. https://doi.org/10.3923/itj.2013.1974.1980 .
Ji C, Li Y, Qiu W, Jin Y, Xu Y, Awada U, Li K, Qu W. Big Data processing: big challenges. J Interconnect Netw. 2012. https://doi.org/10.1142/S0219265912500090 .
Kambatla K, Kollias G, Kumar V, Grama A. Trends in Big Data analytics. J Parallel Distrib Comput. 2014;74(7):2561–73. https://doi.org/10.1016/j.jpdc.2014.01.003 .
Dong XL, Srivastava D. Big Data integration. Proc VLDB Endow. 2013;6(11):1188–9. https://doi.org/10.14778/2536222.2536253 .
Yin H, Jiang Y, Lin C, Luo Y, Liu Y. Big Data: transforming the design philosophy of future internet. IEEE Netw. 2014;28(4):14–9. https://doi.org/10.1109/MNET.2014.6863126 .
Nti IK, Quarcoo JA, Aning J, Fosu GK. A mini-review of machine learning in Big Data analytics: applications, challenges, and prospects. Big Data Min Anal. 2022;5(2):81–97. https://doi.org/10.26599/BDMA.2021.9020028 .
Yu Y, Li M, Liu L, Li Y, Wang J. Clinical Big Data and deep learning: applications, challenges, and future outlooks. Big Data Min Analy. 2019;2(4):288–305. https://doi.org/10.26599/BDMA.2019.9020007 .
Amalina F, Targio Hashem IA, Azizul ZH, Fong AT, Firdaus A, Imran M, Anuar NB. Blending Big Data analytics: review on challenges and a recent study. IEEE Access. 2020;8:3629–45. https://doi.org/10.1109/ACCESS.2019.2923270 .
Chen X-W, Lin X. Big Data deep learning: challenges and perspectives. IEEE Access. 2014;2:514–25. https://doi.org/10.1109/ACCESS.2014.2325029 .
Alam A, Ullah I, Lee Y-K. Video Big Data analytics in the cloud: a reference architecture, survey, opportunities, and open research issues. IEEE Access. 2020;8:152377–422. https://doi.org/10.1109/ACCESS.2020.3017135 .
Pham Q-V, Nguyen DC, Huynh-The T, Hwang W-J, Pathirana PN. Artificial intelligence (AI) and Big Data for coronavirus (COVID-19) pandemic: a survey on the state-of-the-arts. IEEE Access. 2020;8:130820–39. https://doi.org/10.1109/ACCESS.2020.3009328 .
Aydin AA. A comparative perspective on technologies of Big Data value chain. IEEE Access. 2023;11:112133–46. https://doi.org/10.1109/ACCESS.2023.3323160 .
Kalantari A, Kamsin A, Kamaruddin HS, Ale Ebrahim N, Gani A, Ebrahimi A, Shamshirband S. A bibliometric approach to tracking Big Data research trends. J Big Data. 2017. https://doi.org/10.1186/s40537-017-0088-1 .
Tsai C-W, Lai C-F, Chao H-C, Vasilakos AV. Big Data analytics: a survey. J Big Data. 2015. https://doi.org/10.1186/s40537-015-0030-3 .
Raghupathi W, Raghupathi V. Big Data analytics in healthcare: promise and potential. Health Inf Sci Syst. 2014;2(1):3. https://doi.org/10.1186/2047-2501-2-3 .
Ram Mohan Rao P, Murali Krishna S, Siva Kumar AP. Privacy preservation techniques in Big Data analytics: a survey. J Big Data. 2018. https://doi.org/10.1186/s40537-018-0141-8 .
Ali A, Qadir J, Rasool RU, Sathiaseelan A, Zwitter A, Crowcroft J. Big Data for development: applications and techniques. Big Data Anal. 2016. https://doi.org/10.1186/s41044-016-0002-4 .
Hasan MM, Popp J, Oláh J. Current landscape and influence of Big Data on finance. J Big Data. 2020. https://doi.org/10.1186/s40537-020-00291-z .
Seyedan M, Mafakheri F. Predictive Big Data analytics for supply chain demand forecasting: methods, applications, and research opportunities. J Big Data. 2020. https://doi.org/10.1186/s40537-020-00329-2 .
Chang V, Muñoz VM, Ramachandran M. Emerging applications of internet of things, Big Data, security, and complexity: special issue on collaboration opportunity for IoTBDS and COMPLEXIS. Computing. 2020;102(6):1301–4. https://doi.org/10.1007/s00607-020-00811-y .
Biswas S, Khare N, Agrawal P, Jain P. Machine learning concepts for correlated Big Data privacy. J Big Data. 2021. https://doi.org/10.1186/s40537-021-00530-x .
Belcastro L, Cantini R, Marozzo F, Orsino A, Talia D, Trunfio P. Programming Big Data analysis: principles and solutions. J Big Data. 2022. https://doi.org/10.1186/s40537-021-00555-2 .
Abdalla HB. A brief survey on Big Data: technologies, terminologies and data-intensive applications. J Big Data. 2022. https://doi.org/10.1186/s40537-022-00659-3 .
Download references
Acknowledgments
Not applicable.
Author information
Authors and affiliations.
Department of Theoretical and Applied Sciences, Università degli Studi dell’Insubria, Via Mazzini 5, 21100, Varese, Italy
Davide Tosi & Redon Kokaj
Department of Computer Science and Engineering, University of Bologna, Mura Anteo Zamboni 7, 40126, Bologna, Italy
Marco Roccetti
You can also search for this author in PubMed Google Scholar
Contributions
D.T. designed the SLR and wrote the main manuscript text R.K. conducted the SLR M.R. contributed to the Introduction, Challenges, and Conclusions All authors reviewed the manuscript.
Corresponding author
Correspondence to Davide Tosi .
Ethics declarations
Competing interests.
The authors declare no competing interests.
Additional information
Publisher's note.
Springer Nature remains neutral with regard to jurisdictional claims in published maps and institutional affiliations.

Rights and permissions
Open Access This article is licensed under a Creative Commons Attribution 4.0 International License, which permits use, sharing, adaptation, distribution and reproduction in any medium or format, as long as you give appropriate credit to the original author(s) and the source, provide a link to the Creative Commons licence, and indicate if changes were made. The images or other third party material in this article are included in the article’s Creative Commons licence, unless indicated otherwise in a credit line to the material. If material is not included in the article’s Creative Commons licence and your intended use is not permitted by statutory regulation or exceeds the permitted use, you will need to obtain permission directly from the copyright holder. To view a copy of this licence, visit http://creativecommons.org/licenses/by/4.0/ .
Reprints and permissions
About this article
Cite this article.
Tosi, D., Kokaj, R. & Roccetti, M. 15 years of Big Data: a systematic literature review. J Big Data 11 , 73 (2024). https://doi.org/10.1186/s40537-024-00914-9
Download citation
Received : 05 February 2024
Accepted : 07 April 2024
Published : 14 May 2024
DOI : https://doi.org/10.1186/s40537-024-00914-9
Share this article
Anyone you share the following link with will be able to read this content:
Sorry, a shareable link is not currently available for this article.
Provided by the Springer Nature SharedIt content-sharing initiative
- Systematic literature review
- Data analysis
- Artificial intelligence
Open Access is an initiative that aims to make scientific research freely available to all. To date our community has made over 100 million downloads. It’s based on principles of collaboration, unobstructed discovery, and, most importantly, scientific progression. As PhD students, we found it difficult to access the research we needed, so we decided to create a new Open Access publisher that levels the playing field for scientists across the world. How? By making research easy to access, and puts the academic needs of the researchers before the business interests of publishers.
We are a community of more than 103,000 authors and editors from 3,291 institutions spanning 160 countries, including Nobel Prize winners and some of the world’s most-cited researchers. Publishing on IntechOpen allows authors to earn citations and find new collaborators, meaning more people see your work not only from your own field of study, but from other related fields too.
Brief introduction to this section that descibes Open Access especially from an IntechOpen perspective
Want to get in touch? Contact our London head office or media team here
Our team is growing all the time, so we’re always on the lookout for smart people who want to help us reshape the world of scientific publishing.
Home > Books > Social Media and Machine Learning
Literature Review on Big Data Analytics Methods
Submitted: 18 February 2019 Reviewed: 14 May 2019 Published: 24 October 2019
DOI: 10.5772/intechopen.86843
Cite this chapter
There are two ways to cite this chapter:
From the Edited Volume
Social Media and Machine Learning
Edited by Alberto Cano
To purchase hard copies of this book, please contact the representative in India: CBS Publishers & Distributors Pvt. Ltd. www.cbspd.com | [email protected]
Chapter metrics overview
1,783 Chapter Downloads
Impact of this chapter
Total Chapter Downloads on intechopen.com

Total Chapter Views on intechopen.com
Companies and industries are faced with a huge amount of raw data, which have information and knowledge in their hidden layer. Also, the format, size, variety, and velocity of generated data bring complexity for industries to apply them in an efficient and effective way. So, complexity in data analysis and interpretation incline organizations to deploy advanced tools and techniques to overcome the difficulties of managing raw data. Big data analytics is the advanced method that has the capability for managing data. It deploys machine learning techniques and deep learning methods to benefit from gathered data. In this research, the methods of both ML and DL have been discussed, and an ML/DL deployment model for IOT data has been proposed.
- big data analytics
- machine learning
- deep learning
Author Information
Iman raeesi vanani.
- Information Technology Management, Allameh Tabataba’i University, Iran
Setareh Majidian *
*Address all correspondence to: [email protected]
1. Introduction
Digital era with its opportunity and complexity overwhelms industries and markets that are faced with a huge amount of potential information in each transaction. Being aware of the value of gathered data and benefitting from hidden knowledge create a new paradigm in this era, which redefines the meaning of power for corporation. The power of information leads organizations toward being agile and to hit the goals. Big data analytics (BDA) enforces industries to describe, diagnose, predict, prescribe, and cognate the hidden growth opportunities and leads them toward gaining business value [ 68 ]. BDA deploys advanced analytical techniques to create knowledge from exponentially increasing amount of data, which will affect the decision-making process in decreasing complexity of the process [ 43 ]. BDA needs novel and sophisticated algorithms that process and analyze real-time data and result in high-accuracy analytics. Machine and deep learning allocate their complex algorithms in this process considering the problem approach [ 28 ].
In this research, a literature review on big data analytics, deep learning and its algorithms, and machine learning and related methods has been considered. As a result, a conceptual model is provided to show the relation of the algorithms that helps researchers and practitioners in deploying BDA on IOT data.
The process of discussing over DL and ML methods has been shown in Figure 1 .

The big data analytics methods in this research.
2. Big data and big data analytics
One of the vital consequences of the digital world is creating a collection of bulk of raw data. Managing such valuable capital with different shape and size on the basis of organizations’ needs the manager’s attention. Big data has the power to affect all parts of society from social aspect to education and all in between. As the amount of data increases especially in technology-based companies, the matter of managing raw data becomes much more important. Facing with features of raw data like variety, velocity, and volume of big data entitles advanced tools to overcome the complexity and hidden body of them. So, big data analytics has been proposed for “experimentation,” “simulations,” “data analysis,” and “monitoring.” Machine learning as one of the BDA tools creates a ground to have predictive analysis on the basis of supervised and unsupervised data input. In fact, a reciprocal relation has existed between the power of machine learning analytics and data input; the more exact and accurate data input, the more effective the analytical performance. Also, deep learning as a subfield of machine learning is deployed to extract knowledge from hidden trends of data [ 28 ].
3. Big data analytics
In digital era with growing rate of data production, big data has been introduced, which is known by big volume, variety, veracity, velocity, and high value. It brings hardness in analyzing with itself which entitled organization to deploy a new approach and tools in analytical aspects to overcome the complexity and massiveness of different types of data (structured, semistructured, and unstructured). So, a sophisticated technique that aims to cope with complexity of big data by analyzing a huge volume of data is known as big data analytics [ 50 ]. Big data analytics for the first time was coined by Chen Chiang (2012) who pointed out the relation between business intelligence and analytics that has strong ties with data mining and statistical analysis [ 11 ].
Big data analytics supports organizations in innovation, productivity, and competition [ 16 ]. Big data analytics has been defined as techniques that are deployed to uncover hidden patterns and bring insight into interesting relations in understanding contexts by examining, processing, discovering, and exhibiting the result [ 69 ]. Complexity reduction and handling cognitive burden in knowledge-based society create a path toward gaining advantages of big data analytics. Also, the most vital feature that led big data analytics toward success is feature identification. This means that the crucial features that have important affection on results should be defined. It is followed by identifying of corelations between input and a dynamic given point, which may change during times [ 69 ].
As a result of fast evolution of big data analytics, e-business and dense connectivity globally have flourished. Governments, also, take advantages of big data analytics to serve better services to their citizens [ 69 ].
Big data in business context can be managed and analyzed through big data analytics, which is known as a specific application of this field. Also, big data gained from social media can be managed efficiently through big data analytics process. In this way, customer behavior can be understood and five features of big data, which are enumerated as volume, velocity, value, variety, and veracity, can be handled. Big data analytics not only helps business to create a comprehensive view toward consumer behavior but also helps organizations to be more innovative and effective in deploying strategies [ 14 ]. Small and medium size company use big data analytics to mine their semistructured big data, which results in better quality of product recommendation systems and improved website design [ 19 ]. As Ref. [ 9 ] cited, big data analytics gains advantages of deploying technology and techniques on their massive data to improve a firm’s performance.
According to Ref. [ 19 ], the importance of big data analytics has been laid in the fact that decision-making process is supported by insight, which is the result of processing diverse data. This will turn decision-making process into an evidence-based field. Insight extraction from big data has been divided into two main processes, namely data management and data analytics with the former referring to technology support for gathering, storing, and preparing data for analyzing purpose and the latter is about techniques deployed for data analyzing and extracting knowledge from them. Thus, big data analytics has been known as a subprocess of insight extraction. Big data analytics tools are text analytics, audio analytics, video analytics, social media analytics, and predictive analytics. It can be inferred that big data analytics is the main tool for analyzing and interpreting all kinds of digital information [ 35 ]. And the processes involved are data storage, data management, data analyzing, and data visualization [ 9 ].
Big data analytics has the potential for creating effective and efficient value in both operational and strategic approach for organization and it plays as a game changer in augmenting productivity [ 20 ].
Industry practitioners believe that big data analytics is the next ‘blue ocean’ that brings opportunities for organizations [ 33 ], and it is known as “the fourth paradigm of science” [ 70 ].
Fields of machine learning (ML) and deep learning (DL) were expanded to deal with BDA. Different fields like “medicine,” “Internet of Things (IOT),” and “search engines” deploy ML for exploration of predictive features of big data. In other words, it generalizes learnt patterns to predict future data. Feature construction and data representation are two main elements of ML. Also, useful data extraction from big data is the reason for deploying DL, which is a human-brain inspired technique for processing neural signals as a subfield of ML [ 28 ].
4. Big data analytics and deep learning
In 1940s, deep learning was been introduced [ 71 ], but the birth of deep learning algorithms has been determined in year 2006 when layer-wise-greedy-learning method was introduced by Hinton to overcome the deficiency of neural network (NN) method in finding optimized point by trapping in optima local point that is exacerbated when the size of training data was not enough. The underlying thought of proposed method by Hinton is to use unsupervised learning before layer-by-layer training happens [ 72 ].
Inspiring from hierarchical structure of human brain, deep learning algorithms extract complex hidden features with a high level of abstraction. When massive amounts of unstructured data represent, the layered architecture of deep learning algorithms works effectively. The goal of deep learning is to deploy multiple transformation layers where in every layer output representation is occurred [ 42 ]. Big data analytics comprises the whole learnt untapped knowledge gained from deep learning. The main feature of big data analytics, which is extracting underlying features in huge amounts of data, makes it a beneficial tool for big data analytics [ 42 ].
convolutional neural networks (CNN)
restricted Boltzmann machines
autoencoder
sparse coding [ 24 ]
4.1 Convolutional neural networks (CNN)
CNN inspired from neural network model as a type of deep learning algorithm has a “convolutional layer” and “subsampling layer” architecture. Multi-instance data is deployed as a bag of instances in which each data point is a set of instances [ 73 ].
CNN has been known with three features namely “local field,” “subsampling,” and “weight sharing” and comprised of three layers, which are input, hidden that consists of “convolutional layer” and “subsampling layer” and output layer. In hidden layer, each “convolutional layer” comes after “subsampling layer.” CNN training process has been done in two phases of “feed forward” in which the result of previous level entered into next level and “back propagation” pass, which is about modification of errors and deviation through a process of spreading training errors backward and in a hierarchical process [ 74 ]. In the first layer, convolution operation is deployed that is to take various filtering phases in each instances, and then, nonlinear transformation function takes place as the result of previous phase transforming into a nonlinear space. After that, the transformed nonlinear space is considered in max-pooling layer, which represents the bag of instances. This step has been done by considering the maximum response of each instance, which was in filtering step. The representation creates a strong pie with the maximum response that can be deployed by predicting instances’ status in each class. This will lead to constructing a classification model [ 73 ].
CNN is comprised of feature identifier, which is an automatic learning process from extracted features from data with two components of convolutional and pooling layers. Another element of CNN is multilayer perception, which is about taking features that were learned into classification phase [ 3 ].
4.2 Deep neural network (DNN)
A deep architecture in supervised data has been introduced with advances in computation algorithm and method, which is called deep neural network (DNN) [ 3 ]. It originates from shallow artificial neural networks (SANN) that are related to artificial intelligence (AI) [ 30 ].
As hierarchical architecture of DL can constitute nonlinear information in the set of layers, DNN deploys a layered architecture with complex function to deal with complexity and high number of layers [ 3 ].
DNN is known as one of the most prominent tools for classifying [ 49 ] because of its outstanding classification performance in complex classification matters. One of the most challenging issues in DNN is training performance of it, as in optimization problems it tries to minimize an objective function with high amount of parameters in a multidimensional searching space. So, fining and training a proper DNN optimization algorithm requires in high level of attention. DNN is constructed of structure stacked denoising auto encoder (SDAE) [ 75 ] and has a number of cascade auto encoder layers and softmax classifier. The first one deploys raw data to generate novel features, and with the help of softmax, the process of feature classification is performed in an accurate way. The cited features are complementary to each other that helps DNN do its main performance, which is classification in an effective way. Gradient descent (GD) algorithm, which is an optimization method, can be deployed in linear problems with no complex objective function especially in DNN training, and the main condition of this procedure is that the amount of optimization parameter is near to optimal solution [ 6 ]. According to Ref. [ 30 ], DNN with the feature of deep architecture is deployed as a prediction model [ 30 ].
4.3 Recurrent neural network (RNN)
RNN, a network of nodes that are similar to neurons, was developed in 1980s. Each neuron-like node is interconnected with each other, and it can be divided into categories of input, hidden, and output neurons. The data will receive, transform, and generate results in this triple process. Each neuron has the feature of time-varying real-valued activation and every synapse is real-valued weight justifiable [ 66 ]. A classifier for neural networks has outstanding performance in not only learning and approximating [ 105 ] but also in dynamic system modeling with nonlinear approach by using present data [ 29 , 52 ]. RNN with the background of human brain–inspired algorithm has been derived from artificial neural network but they are slightly different from each other. Various fields of “associative memories,” “image processing,” “pattern recognition,” “signal processing,” “robotics,” and “control” have been in the center of focus in research of RNN [ 67 ]. RNN with its feedback and feed forward relations can take a comprehensive view from past information and deploy it for adjusting with sudden changes. Also, RNN has the capability of using time-varying data in a recursive way, which simplified the neural network architecture. Its simplicity and dynamic features work effectively in real-time problems [ 40 ]. RNN has the ability to process temporal data in hierarchy method and take multilayer of abstract data to show dynamical features, which is another capability of RNN [ 18 ]. RNN has the potential to make connection between signals in different levels, which brings significant processing power with huge amounts of memory space [ 45 ].
5. Big data analytics and machine learning
Machine learning has been defined as predictive algorithms by data interpretation, which is followed by learning algorithm in an unstructured program. Three main categories of ML are supervised, unsupervised, and reinforcement learning [ 47 ], which is done during “data preprocessing,” “learning,” and “evaluation phase.” Preprocessing is related to transformation of raw data into right form that can be deployed in learning phase, which comprises of some levels like cleaning the data, extracting, transforming, and combining it. In the evaluation phase, data set will be selected, and evaluation of performance, statistical tests, and estimation of errors or deviation occur. This may lead to modifying selected parameters from learning process [ 76 ]. The first one refers to analyzing features that are critical for classification through a given training data. The data deployed in training algorithm will then become trained and then it will be used in testing of unlabeled data. After interpreting unlabeled data, the output will be generated, which can be classified as discrete or regression if it is continuous. On the other hand, ML can be deployed in pattern identification without training process, which is called unsupervised ML. In this category, when pattern of characteristics are used to group the data, cluster analysis is formed, and if the hidden rules of data have been recognized, another form of ML, which is association, will be formed [ 77 ]. In the other words, the main process of unsupervised ML or clustering is to find natural grouping from those data, which is unlabeled. In this process, K cluster in a set number of data is much more similar in comparison with other clusters considering similarity measure. Three categories of unsupervised ML are “hierarchical,” “partitioned,” and “overlapping” techniques. “Agglomerative” and “divisive” are two kinds of hierarchical methods. The first one is referred to an element that creates a separate cluster with tendency to get involved with larger cluster; however, the second one is a comprehensive set that is going to divide into some smaller clusters. “Partitioned” methods begin with creating several disjoint clusters from data set without considering any hierarchical structure, and “overlapping” techniques are defined as methods that try to find fuzzy or deffuzy partitioning, which is done by “relaxing the mutually disjoint constraint.” Among all unsupervised learning techniques, K-means grabs attention. “Simplicity” and “effectiveness” are two main characteristics of unsupervised techniques [ 47 ].
5.1 Machine learning and fuzzy logic
Fuzzy logic proposed by Lotfi Zadeh (1965) has been deployed in many fields from engineering to data analysis and all in between. Machine learning also gains advantage from fuzzy logic as fuzzy takes inductive inference. The changes happened in such grounds like “fuzzy rule induction,” “fuzzy decision trees,” “fuzzy nearest neighbor estimation,” or “fuzzy support vector machines” [ 27 ].
5.2 Machine learning and classification methods
One of the most critical aspects of ML is classifications [ 23 ], which is the initial phase in data analytics [ 17 ]. Prior studies found new fields that can deploy this aspect like face recognition or even recognition of hand writing. According to [ 23 ], operating algorithm of classification has been divided into two categories: offline and online. In offline approach, static dataset is deployed for training. The training process will be stopped by classifiers after training process is finished and modification of data structured will not be allowed. On the other hand, online category is defined as a “one-pass” type, which is learning from new data. The prominent features of data will be stored in memory and will be kept until the processed training data is erased. Incremental and evolving processes (changing data pattern in unstable environment, which is a result of evolutionary system structure, and continuously updating meta-parameters) are two main approaches for online category [ 23 ].
Support vector machine (SVM) was proposed in 1995 by Cortes and Vapnik to solve problems related to multidimensional classification and regression issues as its outstanding learning performance [ 64 ]. In this process, SVM constructs a high-dimensional hyperplane that divides data into binary categories, and finding greatest margin in binary categories considering the hyperplane space is the main objective of this method [ 10 ]. “Statistical learning theory,” “Vapnik-Chervonenkis (VC) dimension,” and the “kernel method” are underlying factors of development of SVM [ 78 ], which deploys limited number of learning patterns to desirable generalization considering a risk minimization structure [ 22 ].
It is highly dependent on the value of K parameter, which is a gauge for determination of neighborhood space.
The method lacks discrimination ability to differentiate between far and close neighbors.
Overlapping or noise may happen when neighbor are close [ 80 ].
KNN as one of the most important data mining algorithms was first introduced for classification problems, which are expanded to pattern recognition and machine learning research. Expert systems take advantage of KNN classification problems. Three main KNN classifiers that put focus on k-nearest vector neighbor in every class of test sample are as follows:
“Local mean-based k-nearest neighbor classifier (LMKNN)”: despite the fact that existing outlier negative influence can be solved by this method, LMKNN is prone to misclassification because of taking single value of k considering neighborhood size per class and applying it in all classes.
“Local mean-based pseudo nearest neighbor classifier (LMPNN)”: LMKNN and PNN methods create LMPNN, which is known as a good classifier in “multi-local mean vectors of k-nearest neighbors and pseudo nearest neighbor based on the multi-local mean vectors for each class.” Outlier points in addition to k sensitivity have been more considered in this technique. However, differentiation of information in nearest sample of classification cannot recognize widely as weight of all classes are the same [ 81 ].
“Multi-local means-based k-harmonic nearest neighbor classifier (MLMKHNN)”: MLMKHNN as an extension to KNN takes harmonic mean distance for classification of decision rule. It deploys multi-local mean vectors of k-nearest neighbors per class of every query sample and harmonic mean distance will be deployed as the result of this phase [ 82 ]. These methods are designed in order to find different classification decisions [ 81 ].
In 2006, Huang et al. proposed extreme learning machine (ELM) as a classification method that works by a hidden single layer feedback in neural network [ 92 ]. In this layer, the input weight and deviation will be randomly generated and least square method will be deployed to determine output weight analytically [ 17 ], which differentiates this method from traditional methods. In this phase, learning happens followed by finding transformation matrix [ 93 , 94 , 95 , 96 , 97 , 98 , 99 , 100 , 101 , 102 , 103 ]. It is deployed to minimize the sum-of-squares error function. The result of minimizing function will then be used in classification or reduction of dimension [ 48 ]. Neural networks are divided into two categories of feed forward neural network and feedback neural networks and ELM is on the first category, which has a strong learning ability specially in solving nonlinear functions with high complexity. ELM uses this feature in addition to fast learning methods to solve traditional feed forward neural network problems in a mathematical change without iteration with higher speed in comparison with traditional neural network [ 13 ].
Despite the efficiency of ELM in classification problems, binary classification problems emerge as the deficiency of ELM; as in these problems, a parallel training phase on ELM is needed. In twin extreme learning machine (TELM), the problems will be solved by a simultaneous train and two nonparallel classification hyperplanes, which are deployed for classification. Every hyperplane enters into a minimization function to minimize the distance of it with one class, which is located far away from other classes [ 60 ]. ELM is at the center of attention in data stream classification research [ 83 ].
5.3 Machine learning and clustering
Clustering as a supervised learning method aims to create groups of clusters, which members of it are in common with each other in characteristics and dissimilar with other cluster members [ 84 ]. The calculated interpoint distance of every observation in a cluster is small in comparison with its distance to a point in other clusters [ 36 ]. “Exploratory pattern-analysis,” “grouping,” “decision-making,” and “machine-learning situations” are some main applications of clustering technique. Five groups of clustering are “hierarchical clustering,” “partitioning clustering,” “density-based clustering,” “grid-based clustering,” and “model-based clustering” [ 84 ]. Clustering problems are divided into two categories: generative and discriminative approaches. The first one refers to maximizing the probability of sample generation, which is used in learning from generated models, and the other is related to deploying pairwise similarities, which maximize intercluster similarities and minimize similarities of clusters in between [ 63 ].
There are important clustering methods like K-means clustering, kernel K means, spectral clustering, and density-based clustering algorithms that are at the center of research topics for several decades. In K-means clustering, data is assigned to the nearest center, which results from being unable to detect nonspherical clusters. Kernel k-means and spectral clustering create a link between the data and feature space and after that k-means clustering is deployed. Obtaining feature space is done by using kernel function and graph model by kernel k-means and spectral clustering, respectively. Also spectral clustering deploys Eigen-decomposition techniques additionally [ 26 ]. K-means clustering works effectively in clustering of numerical data, which is multidimensional [ 85 ].
Density-based clustering is represented by DBSCAN, and clusters tend to be separate from data set and be as higher density area. This method does not deploy one cluster for clusters recognition in the data a priori. It considers user-defined parameter to create clusters, which has a bit deviation from cited parameter in clustering process [ 84 ].
5.4 Machine learning and evolutionary methods
The main goal of optimization problems is to find an optimal solution among a set of alternatives. Providing the best solution has become difficult if the searching area is large. Heuristic algorithm proposed different techniques to find the optimal solution, but they lack finding the best solution. However, population-based algorithm was generated to overcome the cited deficiency, which is considered to find the best alternative [ 7 ].
5.5 Genetic algorithms (GA)
GA is defined as a randomized search, which tries to find near-optimal solution in complex and high-dimensional environment. In GA, a bunch of genes that are called chromosomes are the main parameters in the technique. These chromosomes are deployed as a search space. A number of chromosomes that seem as a collection are called population. The creation of a random population will be followed by representing the goodness degree of objective and fitness function related to each string. The result of this step that will be a few of selected string with a number of copies will be entered into the mating pool. By deploying cross-over and mutation process, a new generation of string will be created from the string. This process will be continued until a termination condition is found. “Image processing,” “neural network,” and “machine learning” are some examples of application fields for genetic algorithms [ 38 ]. GA as nature-inspired algorithm is based on genetic and natural selection algorithms [ 31 ].
GA tries to find optimal solution without considering the starting point [ 104 ]; also, GA has the potential to find optimal clustering considering clustering metrics [ 38 ]. Filter and wrapper search are two main approaches of GA in the field of feature selection. The first one aims to investigate the value of features by deploying heuristic-based data characteristics like correlation, and the second one assesses the goodness of GA solution by using machine learning algorithm [ 53 ]. In K-means algorithm, optimized local point is found on the basis of initializing seed values and the generated cluster is on the basis of initial seed values. GA by the aim of finding near-optimal or optimal clustering searches for initial seed values, outperforms K-mean algorithm, and covers the lack of K-mean algorithm [ 4 ]. Gaining knowledge from data base is another ground for GA, which plays the role of building “classifier system” and “mining association rules” [ 58 ].
Feature selection is a vital problem in big data as it usually contains many features that describe target concepts and chooses proper amount of feature for pre-processing traditionally as a main matter was done by data mining. Feature selection is divided into two groups: independent of learning algorithm, which deploys filter approach, and dependent on learning algorithm, which uses a wrapper approach. However, filter approach is independent of learning algorithm, and the optimal set of feature may be dependent on learning algorithm, which is one of the main drawbacks of filter selection. In contrast, wrapper approach by deploying learning algorithm in evaluation of every feature set works better. A main problem of this approach is complexity in computation field, which is overcome by using GA in feature selection as learning algorithm [ 56 ].
5.6 Ant colony optimization (ACO)
Ant colony optimization method was proposed by Dorigo [ 17 ] as a population-based stochastic method [ 15 ]. The method has been created biologically from real ant behavior in food-seeking pattern. In other words, this bionic algorithm has been deployed for finding the optimal path [ 44 ]. The process is that when ants start to seek food they deposit a chemical material on the ground, which is known as pheromone while they are moving toward food source. As the path between the food source and nest become shorter, the amount of pheromone will become larger. New ants in this system tend to choose the path with greater amount of pheromone. By passing time, all ants follow the positive feedback and choose the shortest path, which is signed by greatest amount of pheromone [ 86 ]. The applications of ant colony optimization in recent research have been declared as traveling salesman problem, scheduling, structural and concrete engineering, digital image processing, electrical engineering, clustering, routing optimization algorithm [ 41 ], data mining [ 32 ], robot path planning [ 87 ], and deep learning [ 39 ].
Less complexity in integration of this method with other algorithms
Gain advantage of distributed parallel computing (e.g., intelligent search)
Work better in optimization in comparison with swarm intelligence
High speed and high accuracy
Robustness in finding a quasi-optimal solution [ 41 ]
As it is stated, the emitted material called pheromone causes clustering between species around optimal position. In big data analytics, ant colony clustering is deployed on the grid board to cluster the data objects [ 21 ].
Initializing pheromone trail
Deploying pheromone trail to construct solution
Updating trail pheromone
On the basis of probabilistic state transition rule, which depends on the state of the pheromone, a complete solution is made by each ant. Two steps of evaporation and reinforcement phase are passed in pheromone updating procedure, where evaporation of pheromone fraction happens and emitting of pheromone that shows the level of solution fitness is determined, respectively, which is followed by finalizing condition [ 46 ].
Ant colony decision tree (ACDT) is a branch of ant colony decision that aims to develop decision tress that are created in running algorithm, but as a nondeterministic algorithm in every execution, different decision tree is created. A pheromone trail on the edge and heuristics used in classical algorithm is the principle of ACDT algorithm.
The multilayered ant colony algorithm has been proposed after the disability of one layer ant colony optimization has been declared in finding optimal solution. As an item, value with massive amount of quantity takes too long to grow. In this way, through transactions, maximum quantities of an item is determined and a rough set of membership function will be set, which will be improved by refining process at subsequent levels by reduction in search space. As a result, search ranges will be differing considering the levels. Solution derived from every level is an input for next level, which is considered in the cited approach but with a smaller search space that is necessary for modifying membership functions [ 88 ]. Tsang and Kwong proposed ant colony clustering in anomaly detection [ 65 ].
5.7 Bee colony optimization (BCO)
BCO algorithm works on inspiration from honey bee’s behavior, which is widely used in optimization problems like “traveling salesman problem,” “internet hosting center,” vehicle routing, and the list goes on. Karaboga in 2005 proposed artificial bee colony (ABC) algorithm. The main features of artificial bee colony (ABC) algorithm are simplicity, easy used and has few elements which need to be controlled in optimization problems. “Face recognition,” “high-dimensional gene expression,” and “speech segment classification” are some examples that ABC and ACO use to select features and optimize them by having a big search space. In ABC algorithms, three types of bees called “employed bees (EBees),” “onlooker bees (OBees),” and “scout bees deployed” are deployed. In this process, food sources are positioned and then EBees, where their numbers are equal to number of food source, pass the nectar information to OBees. They are equal to the number of EBees. The information is taken to exploit the food source till the finishing amount. Scouts in exhausted food source are employed to search for new food source. The nectar amount is a factor that shows solution quality [ 25 , 55 ].
This method is comprised of two steps: step forward, which is exploring new information by bees, and step back, which is related to sharing information considering new alternative by bee of hives.
In this method, exploration is started by a bee that tries to discover a full path for its travel. When it leaves the hive, it comes across with random dances of other bees, which are equipped with movement array of other bees that is known as “preferred path.” This will lead in foraging process and it comprises of a full path, which was previously discovered by its partner who guides the bee to the final destination. The process of moving from one node to another will be continued till the final destination is reached. For choosing the node by bees, a heuristic algorithm is used, which involves two factors of arc fitness and the distance heuristic. The shortest distance has the possibility to be selected by bees [ 7 ]. In BCO algorithm, two values of alpha and beta will be considered, which are exploitation and exploration processes, respectively [ 8 ].
5.8 Particle swarm optimization (PSO)
PSO was generated from inspiration from biological organisms, particularly the ability of a grouped animal to work together in order to find the desired location in particular area. The method was introduced by Kennedy and Eberhart in 1995 as a stochastic population-based algorithm, which is known by features like trying to find global optimize point and easy implementation with taking a small amount of parameters in adjusting process. It takes benefit from a very productive searching algorithm, which makes it a best tool to work on different optimization research area and problems [ 59 ].
The searching process is led toward solving a nonlinear optimization problem in a real value search space. In this process, an iterative searching happens to find the destination, which is the optimal point. In other words, each particle has a multidimensional search with a specific space, which is updated by particle experience or the best neighbor’s space and the objective function assesses the fitness value of each particle. The best solution, which is found in each iteration, will be kept in memory. If the optimal solution is found by particle, it is called local best or pbest and the optimal point among the particle neighbors is called global best or gbest [ 89 ]. In this algorithm, every potential solution is considered as a particle, which has several features like the current position and velocity. The balance between global and local search can be adjusted by adopting different inertia weight. One of critical success factors in PSO is a trade-off between global and local search in iteration [ 59 ]. Artificial neural network, pattern classification, and fuzzy control are some area for deploying PSO [ 5 ]. Social interaction and communication metaphor like “birds flock and fish schooling” developed this algorithm and it works on the basis of improving social information sharing, which is done among swarm particles [ 12 ].
5.9 Firefly algorithm (FA)
Firefly algorithm was been introduced by Yang [ 16 ]. The main idea of FA is that each firefly has been assumed as unisexual, which is attracted toward other firefly regardless of the gender. Brightness is the main attraction for firefly that stimulates the less bright to move toward brighter ones. The attractiveness and brightness are opposed to distance. The brightness of a firefly has been determined by the area of fitness function [ 90 ]. As the brightness of firefly increased, the level of goodness of solution increased. A full attraction model has been proposed that shows all fireflies will be attracted to brighter ones and similarity of all fireflies will occur if a great number of fireflies attract to a brighter one, which is measured by fitness value. So, convergence rate during the search method will occur in a slow pace.
FA has been inspired from the lightening feature of fireflies and known as swarm intelligence algorithm. FA better works in comparison with genetic algorithm (GA) and PSO in some cases. “Unit commitment,” “energy conservation,” and “complex networks” are some examples of working area of FA [ 61 ]. Fluctuation may occur when huge numbers of fireflies attract to light emission source and the searching process becomes time-consuming. To overcome these issues, neighborhood attraction FA (NaFA) is introduced, which shows that fireflies are just attracted to only some brighter points, which are outlined by previous neighbor [ 62 ].
5.10 Tabu search algorithm (TS)
Tabu search is a meta-heuristic, which was proposed by y Glover and Laguna (1997) on the basis of edge projection and making it better and it tries to make a progress in local search, which leads to a global optimized solution by taking possibility on consecutive algorithm iterations. Local heuristic search process is taken to find solution that can be deployed to combinatorial optimization paradigm [ 2 ]. The searching process in this methodology is flexible as it takes adaptive memory. The process is done during different iterations. In each iteration, a solution is found. The solution has a neighbor point that can be reached via “move.” In every move, a better solution is found, which can be stopped when no better answer is found [ 37 ]. In TS, the aspiration criteria are critical factors that lead the searching process by not considering forbidden solutions that are known by TS. In each solution, the constraints of the objective are met. So, the solutions are both feasible and time-consuming. TS process is continued by using a tabu list (TL), which is a short-term history. The short memory just keeps the recent movement, which is done by deleting the old movement when the memory is full to the maximum level [ 1 ].
The main idea of TS is to move toward solution space, which remains unexplored, which would be an opportunity to keep away from local solution. So, “tabu” movements that are recent movements are kept forbidden, which prevents from visiting previous solution points. This is proved that the method brings high-quality solutions in its iterations [ 57 ].
6. Big data analytics and Internet of Things (IOT)
Internet of things (IOT) put focus on creating an intelligent environment in which things socialize with each other by sensing, processing, communicating, and actuating activities. As IOT sensors gathered a huge amount of raw data, which is needed to be processed and analyzed, powerful tools will enforce the analytics process. This will stimulate to deploy BDA and its methods on IOT-based data. Ref. [ 51 ] proposed a four-layer model to show how BDA can help IOT-based system to work better. This model comprised of data generation, sensor communication, data processing, and data interpretation [ 51 ]. It is cited that beyond 2020 cognitive processing and optimization will be considered on IOT data processing [ 34 ]. In IOT-based systems, acquired signals from sensors are gathered and deployed for processing in frame-by-frame or batch mode. Also, gathered data in IOT system will be deployed in feature extraction, which is followed by classification stage. Machine learning algorithms will be used in data classifying [ 54 ]. Machine learning classification can be deployed on three types of data, which are supervised, semisupervised, and unsupervised [ 54 ]. In decision-making level, which is comprised of pattern recognition, deep learning methods, namely, RNN, DNN, CNN, and ANN can be used for discovering knowledge. Optimization process in IOT can be used to create an optimized cluster in IOT data [ 91 ].
In Figure 2 , the process of IOT is shown. Data is gathered from sensors. Data enters the filtering process. In this level, denoising and data cleansing happen. Also, in this level, feature extraction is considered for classification phase. After preprocessing, decision making happens on the basis of deep learning methodology ( Table 1 ). Deep learning and machine learning algorithms can be used in analyzing of data generated through IOT device, especially in the classification and decision-making phase. Both supervised and unsupervised techniques can be used in classification phase considering the data type. However, both deep learning and machine learning algorithms are eligible in deploying in decision-making phase.

IOT process.
Deep learning and machine learning techniques on IOT phases.
7. Future research directions
For feature endeavors, it is proposed to work on application of big data analytics methods on IOT fog and edge computing. It is useful to extract patterns from hidden knowledge of data gathered from sensors deploying powerful analytical tools. Fog computing is defined as a technology that is implemented in near distance to end user, which provides local processing and storage to support different devices and sensors. Health care systems gain advantage from IOT for fog computing, which supports mobility and reliability in such systems. Health care data acquisition, processing, and storage of real-time data are done in edge, cloud, and fog layer [ 47 ]. In future research, the area that machine learning algorithms can provide techniques for fog computing can be on the focus. IOT data captured from smart houses needs analytical algorithms to overcome the complexity of offline and online data gathered in processing, classification, and also next best action, or even pattern recognition [ 81 ]. Hospital information system creates “life sciences data,” “clinical data,” “administrative data,” and “social network data.” These data sources are overwhelmed with illness predictions, medical research, or even management and control of disease [ 39 ]. Big data analytics can be a future subject by helping HIS to cover data processing and disease pattern recognition.
Smart house creates ground for real-time data with high complexity, which entitles big data analytics to overcome such sophistication. Classical methods of data analyzing lost their ability in front of evolutionary methods of classification and clustering. So graphic processing unit (GPU) for machine learning and data mining purposes bring advantage for large scale dataset [ 7 ], which leads the applications into lower cost of data analytics. Another way to create future research is to work over different frameworks like Spark, which is an in-memory computation, and with the help of big data analytics, optimization problems can be solved [ 20 ].
Deployment of natural language processing (NLP) in text classification can be accompanied by different methods like CNN and RNN. These methods can gain the result with higher accuracy and lower time (Li et al., 2018).
Predictive analytics offered by big data analytics works on developing predictive models to analyze large volume data both structured and unstructured with the goal of identifying hidden patterns and relations between variables in near future [ 76 ]. Big data analytics can help cognitive computing, and behavior pattern recognition deploys deep learning technique to predict future action as it is used to predict cancer in health care system [ 59 ]. It also leads organizations to understand their problems [ 13 ].
So, future research can be focused on both the new area for application of different machine learning or deep learning algorithm for censored data gathered and also mixture of techniques that can create globally optimal solution with higher accuracy and lower cost. Researchers can put focus on existing problems of industries through mixed application of machine learning and deep learning techniques, which may results in optimize solution with lower cost and higher speed. They also can take identified algorithms in new area of industries to solve problems, create insight, and identify hidden patterns.
In summary, future research can be done as it is shown in Figure 3 .

Future research on big data analytics (BDA).
8. Conclusion
This chapter has been attempted to give an overview on big data analytics and its subfields, which are machine learning and deep learning techniques. As it is cited before, big data analytics has been generated to overcome the complexity of data managing and also create and bring knowledge into organizations to empower the performances. In this chapter, DNN, RNN, and CNN have been introduced as deep learning methods, and classification, clustering, and evolutionary techniques have been overviewed. Also, a glance at some techniques of every field has been given. Also, the application of machine learning and deep learning in IOT-based data is shown in order to make IOT data analytics much more powerful in phase of classification and decision-making. It has been identified that on the basis of rapid speed of data generation through IOT sensors, big data analytics methods have been widely used for analyzing real-time data, which can solve the problem of complexity of data processing. Hospital information systems (HIS), smart cities, and smart houses take benefits of to-the-point data processing by deploying fog and cloud platforms. The methods are not only deployed to create a clear picture of clusters and classifications of data but also to create insight for future behavior by pattern recognition. A wide variety of future research has been proposed by researchers, from customer pattern recognition to predict illness like cancer and all in between are comprised in area of big data analytics algorithms.
Acknowledgments
This research received no specific grant from any funding agency in the public, commercial, or not-for-profit sectors.
- 1. Bożejko W et al. Parallel tabu search for the cyclic job shop scheduling problem. Computers & Industrial Engineering. 2018; 113 :512-524
- 2. Kiziloz H, Dokeroglu T. A robust and cooperative parallel tabu search algorithm for the maximum vertex weight clique problem. Computers & Industrial Engineering. 2018; 118 :54-66
- 3. Acharya U et al. Automated detection of coronary artery disease using different durations of ECG segments with convolutional neural network. Knowledge-Based Systems. 2017; 132 :62-71
- 4. Babu GP, Murty M. A near-optimal initial seed value selection in K-means algorithm using a genetic algorithm. Pattern Recognition Letters. 1993; 14 (10):763-769
- 5. Bonyadi MR, Michalewicz Z. Particle swarm optimization for single objective continuous space problems: A review. Evolutionary Computation. 2017; 25 (1):1-54
- 6. Caliskan A et al. Classification of high resolution hyperspectral remote sensing data using deep neural networks. Engineering Applications of Artificial Intelligence. 2018; 67 :14-23
- 7. Cano A. A survey on graphic processing unit computing for large-scale data mining. WIREs Data Mining and Knowledge Discovery. 2017; 8 (1):e1232. DOI: 10.1002/widm.1232
- 8. Caraveo C et al. Optimization of fuzzy controller design using a new bee colony algorithm with fuzzy dynamic parameter adaptation. Applied Soft Computing. 2016; 43 :131-142
- 9. Castillo O, Amador-Angulo L. A generalized type-2 fuzzy logic approach for dynamic parameter adaptation in bee colony optimization applied to fuzzy controller design. Information Sciences. 2018; 460-461 :476-496
- 10. Chen J et al. The synergistic effects of IT-enabled resources on organizational capabilities and firmperformance. Information and Management. 2012; 49 (34):140-152
- 11. Chou J et al. Metaheuristic optimization within machine learning-based classification system for early warnings related to geotechnical problems. Automation in Construction. 2016; 68 :65-80
- 12. Côrte-Real A et al. Assessing business value of Big Data Analytics in European firms. Journal of Business Research. 2017; 70 :379-390
- 13. Côrte-Real N et al. Unlocking the drivers of big data analytics value in firms. Journal of Business Research. 2019; 97 :160-173
- 14. Delice Y et al. A modified particle swarm optimization algorithm to mixed-model two-sided assembly line balancing. Journal of Intelligent Manufacturing. 2017; 28 (1):23-36
- 15. Ding S et al. Extreme learning machine: Algorithm, theory and applications. Artificial Intelligent Review. 2015; 44 (1):103-115
- 16. Dong J, Yang C. Business value of big data analytics: A systems-theoretic approach and empirical test. In: Information & Management. 2018. [In Press]
- 17. Dorigo M. Ant Colony Optimization: New Optimization Techniques in Engineering. Berlin Heidelberg: Springer-Verlag; 1991. pp. 101-117
- 18. Esposito C et al. A knowledge-based platform for Big Data analytics based on publish/subscribe services and stream processing. Knowledge-Based Systems. 2015; 79 :3-17
- 19. Feng L et al. Rough extreme learning machine: A new classification method based on uncertainty measure. Neurocomputing. 2019; 325 :269-282
- 20. Gonzalez-Lopez J et al. Distributed nearest neighbor classification for large-scale multi-label data on spark. Future Generation Computer Systems. 2018; 87 :66-82
- 21. Gallicchio C et al. Deep reservoir computing: A critical experimental analysis. Neurocomputing. 2017; 268 :87-99
- 22. Gandomi A, Haider M. Beyond the hype: Big data concepts, methods, and analytics. International Journal of Information Management. 2015; 35 (2):137-144
- 23. German F et al. Do retailers benefit from deploying customer analytics? Journal of Retailing. 2014; 90 :587-593
- 24. Ghosh A et al. Aggregation pheromone density based data clustering. Information Sciences. 2008; 178 :2816-2831
- 25. Gonzalez-Abril L et al. Handling binary classification problems with a priority class by using support vector machines. Applied Soft Computing. 2017; 61 :661-669
- 26. Gu X, Angelov P. Self-organizing fuzzy logic classifier. Information Sciences. 2018; 447 :36-51
- 27. Guo Y et al. Deep learning for visual understanding: A review. Neurocomputing. 2016; 187 :27-48
- 28. Harfouchi F et al. Modified multiple search cooperative foraging strategy for improved artificial bee colony optimization with robustness analysis. Soft Computing. 2017; 22 (19)
- 29. Huang J et al. A clustering method based on extreme learning machine. Neurocomputing. 2018; 227 :108-119
- 30. Hüllermeier E. Does machine learning need fuzzy logic? Fuzzy Sets and Systems. 2015; 281 :292-299
- 31. Jan B et al. Deep learning in big data analytics: A comparative study. Computers and Electrical Engineering. 2017; 75 :1-13
- 32. Jiang P, Chen J. Displacement prediction of landslide based on generalized regression neural networks with K-fold cross-validation. Neurocomputing. 2016; 198 :40-47
- 33. Jiang S et al. Modified genetic algorithm-based feature selection combined with pre-trained deep neural network for demand forecasting in outpatient department. Expert Systems With Applications. 2017; 82 :216-230
- 34. Ko Y. How to use negative class information for Naive Bayes classification. Information Processing and Management. 2017; 53 (6):1255-1268
- 35. Koonce D, Tsaib S. Using data mining to find patterns in genetic algorithm solutions to a job shop schedule. Computers & Industrial Engineering. 2000; 38 (3):361-374
- 36. Kozak J, Boryczka U. Collective data mining in the ant colony decision tree approach. Information Sciences. 2016; 372 :126-147
- 37. Kwon O et al. Data quality management, data usage experience and acquisition intention of big data analytics. International Journal of Information Management. 2014; 34 (3):387-394
- 38. Lee I, Lee K. The Internet of Things (IoT): Applications, investments, and challenges for enterprises. Business Horizons. 2015; 58 (4):1-10
- 39. Li J et al. Medical big data analysis in hospital information system. In: Big Data on Real-World Applications. 2016. Chapter 4
- 40. Loebbecke C, Picot A. Reflections on societal and business model transformation arising from digitization and big data analytics: A research agenda. Journal of Strategic Information Systems. 2015; 24 (3):149-157
- 41. Lohrmann C, Luukka P. A novel similarity classifier with multiple ideal vectors based on k-means clustering. Decision Support Systems. 2018; 111 :27-37
- 42. Martí R et al. Tabu search for the dynamic bipartite drawing problem. Computers and Operations Research. 2018; 91 :1-12
- 43. Maulik U et al. Genetic algorithm-based clustering technique. Pattern Recognition. 2000; 33 (9):1455-1465
- 44. Mavrovounioti M, Yang S. Training neural networks with ant colony optimization algorithms for pattern classification. Journal of Soft Computing. 2015; 19 (6):1511-1522
- 45. Miao Z et al. Robust tracking control of uncertain dynamic nonholonomic systems using recurrent neural networks. Neurocomputing. 2014; 142 :216-227
- 46. Mohan B, Baskaran R. A survey: Ant colony optimization based recent research and implementation on several engineering domain. Expert Systems with Applications. 2012; 39 (4):4618-4627
- 47. Mutlag AA et al. Enabling technologies for fog computing in health care IoT systems. Future Generation Computer Systems. 2019; 90 :62-78
- 48. Najafabadi M et al. Deep learning applications and challenges in big data analytics. Journal of Big Data. 2015:1-21. DOI: 10.1186/s40537-014-0007-7
- 49. Nguyen T et al. Big data analytics in supply chain management: A state-of-the-art literature review. Computers and Operations Research. 2018; 98 :254-264
- 50. Ning J et al. A best-path-updating information-guided ant colony optimization algorithm. Information Sciences. 2018; 433-434 :142-162
- 51. Osipov V, Osipova M. Space–time signal binding in recurrent neural networks with controlled elements. Neurocomputing. 2018; 308 :194-204
- 52. Panda M, Abraham A. Hybrid evolutionary algorithms for classification data mining. In: Neural Computing & Applications. 2014; 26 (3):507-523
- 53. Peng H et al. An unsupervised learning algorithm for membrane computing. Information Sciences. 2015; 304 :80-91
- 54. Peng Y et al. Orthogonal extreme learning machine for image classification. Neurocomputing. 2017; 266 :458-464
- 55. Qawaqneh Z et al. Age and gender classification from speech and face images by jointly fine-tuned deep neural networks. Expert Systems With Applications. 2017; 85 :78-86
- 56. Ramsingh J, Bhuvaneswari V. An efficient map reduce-based hybrid NBC-TFIDF algorithm to mine the public sentiment on diabetes mellitus—A big data approach. Journal of King Saud University – Computer and Information Sciences. 2018. [In Press]
- 57. Rathore M et al. Urban planning and building smart cities based on the Internet of Things using Big Data analytics. Computer Networks. 2016; 101 :63-80
- 58. Ruan X, Zhang Y. Blind sequence estimation of MPSK signals using dynamically driven recurrent neural networks. Neurocomputing. 2014; 129 :421-427
- 59. Sekaran K et al. Deep learning convolutional neural network (CNN) with Gaussian mixture model for predicting pancreatic cancer. Multimedia Tools and Applications. 2019:1-15. DOI: 10.1007/s11042-019-7419-5
- 60. Shah S, Kusiak A. Data mining and genetic algorithm based gene/SNP selection. Artificial Intelligence in Medicine. 2004; 31 (3):183-196
- 61. Shanthamallu U et al. A brief survey of machine learning methods and their sensor and IoT applications. In: 2017 8th International Conference on Information, Intelligence, Systems & Applications (IISA). 2017. DOI: 10.1109/IISA.2017.8316459
- 62. Shunmugapriya P, Kanmani S. A hybrid algorithm using ant and bee colony optimization for feature selection and classification (AC-ABC Hybrid). Swarm and Evolutionary Computation. 2017; 36 :27-36
- 63. Sikora R, Piramuthu S. Framework for efficient feature selection in genetic algorithm based data mining. European Journal of Operational Research. 2007; 180 (2):723-737
- 64. Silva M, Cunha C. A tabu search heuristic for the uncapacitated single allocation p-hub maximal covering problem. European Journal of Operational Research. 2017; 262 (3):954-965
- 65. Srinivasa KG et al. A self-adaptive migration model genetic algorithm for data mining applications. Information Sciences. 2007; 177 (20):4295-4313
- 66. Taherkhani M, Safabakhsh R. A novel stability-based adaptive inertia weight for particle swarm optimization. Applied Soft Computing. 2016; 38 :281-295
- 67. Wan Y et al. Twin extreme learning machines for pattern classification. Neurocomputing. 2017; 260 :235-244
- 68. Wang H et al. Firefly algorithm with neighborhood attraction. Information Sciences. 2017; 382-383 :374-387
- 69. Wang H et al. Randomly attracted firefly algorithm with neighborhood search and dynamic parameter adjustment mechanism. Journal of Soft Computing. 2017; 21 (18):5325-5339
- 70. Wang Q et al. Local kernel alignment based multi-view clustering using extreme learning machine. Neurocomputing. 2018; 275 :1099-1111
- 71. Wu J et al. A patent quality analysis and classification system using self-organizing maps with support vector machine. Applied Soft Computing. 2016; 41 :305-316
- 72. Zhang L, Zhang Q. A novel ant-based clustering algorithm using the kernel method. Information Sciences. 2011; 181 :4658-4672
- 73. Zhang X et al. An overview of recent developments in Lyapunov–Krasovskii functionals and stability criteria for recurrent neural networks with time-varying delays. Neurocomputing. 2018; 313 :392-401
- 74. Zhu S, Shen Y. Robustness analysis for connection weight matrix of global exponential stability recurrent neural networks. Neurocomputing. 2013; 101 :370-374
- 75. Wang Y et al. Integrated big data analytics-enabled transformation model: Application to health care. Information and Management. 2018; 55 (1):64-79
- 76. Wang Y, Hajli N. Exploring the path to big data analytics success in healthcare. Journal of Business Research. 2017; 70 :287-299
- 77. Iqbal R et al. Big data analytics: Computational intelligence techniques and application areas. Technological Forecasting & Social Change. 2018. [In Press]
- 78. Wamba S et al. Big data analytics and firm performance: Effects of dynamic capabilities. Journal of Business Research. 2017; 70 :356-365
- 79. Zhang Q et al. A survey on deep learning for big data. Information Fusion. 2018; 42 :146-157
- 80. Liu W et al. A survey of deep neural network architectures and their applications. Neurocomputing. 2017; 234 :11-26
- 81. Yassine A et al. IoT big data analytics for smart homes with fog and cloud computing. Future Generation Computer Systems. 2019; 91 :563-573
- 82. Yin Z et al. A-optimal convolutional neural network. Neural Computings & Applications. 2016; 30 (7):2295-2304
- 83. Wang S et al. Convolutional neural network-based hidden Markov models for rolling element bearing fault identification. Knowledge-Based Systems. 2018; 144 :65-76
- 84. Shi X et al. Tracking topology structure adaptively with deep neural networks. Neural Computing & Application. 2017; 30 (11):3317-3326
- 85. Zhou L et al. Machine learning on big data: Opportunities and challenges. Neurocomputing Journal. 2017; 237 :350-361
- 86. Tack C. Artificial intelligence and machine learning | applications in musculoskeletal physiotherapy. Musculoskeletal Science and Practice. 2018; 39 :164-169
- 87. Tang L et al. A novel perspective on multiclass classification: Regular simplex support vector machine. Information Sciences. 2018; 480 :324-338
- 88. Xia M et al. A hybrid method based on extreme learning machine and k-nearest neighbor for cloud classification of ground-based visible cloud image. Neurocomputing. 2015; 160 :238-249
- 89. Onan A. A fuzzy-rough nearest neighbor classifier combined with consistency-based subset evaluation and instance selection for automated diagnosis of breast cancer. Expert Systems with Applications. 2015; 42 (20):6844-6852
- 90. Gou J et al. A generalized mean distance-based k-nearest neighbor classifier. Expert Systems With Applications. 2019; 115 :356-372
- 91. Pan Z et al. A new k-harmonic nearest neighbor classifier based on the multi-local means. Expert Systems With Applications. 2017; 67 :115-125
- 92. Xu S, Wang J. Dynamic extreme learning machine for data stream classification. Neurocomputing. 2017; 238 :433-449
- 93. Du G et al. Study on density peaks clustering based on k-nearest neighbors and principal component analysis. Knowledge-Based Systems. 2016; 99 :135-145
- 94. Yu S et al. Two improved k-means algorithms. Applied Soft Computing. 2018; 68 :747-755
- 95. Tabakhi S et al. Gene selection for microarray data classification using a novel ant colony optimization. Neurocomputing. 2015; 168 :1024-1036
- 96. Liu H et al. A path planning approach for crowd evacuation in buildings based on improved artificial bee colony algorithm. Applied Soft Computing. 2018; 68 :360-376
- 97. Hong T et al. A multi-level ant-colony mining algorithm for membership functions. Information Sciences. 2012; 182 (1):3-14
- 98. Kuo RJ et al. Integration of growing self-organizing map and bee colony optimization algorithm for part clustering. Computers & Industrial Engineering. 2018; 120 :251-265
- 99. Verma O et al. Opposition and dimensional based modified firefly algorithm. Expert Systems With Applications. 2016; 44 :168-176
- 100. Janakiraman S. A hybrid ant colony and artificial bee colony optimization algorithm-based cluster head selection for IoT. Procedia Computer Science. 2018; 143 :360-366
- 101. Tsai C et al. Metaheuristic algorithms for healthcare: Open issues and challenges. Computers and Electrical Engineering. 2016; 53 :421-434
- 102. Villarrubia G et al. Artificial neural networks used in optimization problems. Neurocomputing. 2018; 272 :10-16
- 103. Wari E, Zhu W. A survey on metaheuristics for optimization in food manufacturing. Applied Soft Computing. 2016; 46 :328-343
- 104. Wu J et al. Evolving RBF neural networks for rainfall prediction using hybrid particle swarm optimization and genetic algorithm. Neurocomputing. 2015; 148 :136-142
- 105. Yang F et al. A new approach to non-fragile state estimation for continuous neural networks with time-delays. Neurocomputing. 2016; 197 :205-211
© 2019 The Author(s). Licensee IntechOpen. This chapter is distributed under the terms of the Creative Commons Attribution 3.0 License , which permits unrestricted use, distribution, and reproduction in any medium, provided the original work is properly cited.
Continue reading from the same book
Published: 19 February 2020
By Nityashree Nadar and R. Kamatchi
897 downloads
By Alberto Cano
965 downloads
By Leila Kerkeni, Youssef Serrestou, Mohamed Mbarki, ...
6204 downloads

An official website of the United States government
The .gov means it’s official. Federal government websites often end in .gov or .mil. Before sharing sensitive information, make sure you’re on a federal government site.
The site is secure. The https:// ensures that you are connecting to the official website and that any information you provide is encrypted and transmitted securely.
- Publications
- Account settings
Preview improvements coming to the PMC website in October 2024. Learn More or Try it out now .
- Advanced Search
- Journal List
- Healthcare (Basel)

Towards the Use of Big Data in Healthcare: A Literature Review
Associated data.
Not applicable.
The interest in new and more advanced technological solutions is paving the way for the diffusion of innovative and revolutionary applications in healthcare organizations. The application of an artificial intelligence system to medical research has the potential to move toward highly advanced e-Health. This analysis aims to explore the main areas of application of big data in healthcare, as well as the restructuring of the technological infrastructure and the integration of traditional data analytical tools and techniques with an elaborate computational technology that is able to enhance and extract useful information for decision-making. We conducted a literature review using the Scopus database over the period 2010–2020. The article selection process involved five steps: the planning and identification of studies, the evaluation of articles, the extraction of results, the summary, and the dissemination of the audit results. We included 93 documents. Our results suggest that effective and patient-centered care cannot disregard the acquisition, management, and analysis of a huge volume and variety of health data. In this way, an immediate and more effective diagnosis could be possible while maximizing healthcare resources. Deriving the benefits associated with digitization and technological innovation, however, requires the restructuring of traditional operational and strategic processes, and the acquisition of new skills.
1. Introduction
The adoption of Fourth Industrial Revolution technologies, particularly artificial intelligence (AI) and big data (BD), has been a major challenge for all industries [ 1 ]. The increasing technological progress has initiated a digital transformation process in many sectors, including healthcare [ 2 ], which is already moving toward Healthcare 4.0 due to the impact of smart technologies [ 3 , 4 ] such as the Internet of Things (IoT) paradigm [ 5 ], cloud and fog computing [ 5 ], and big data analytics (BDA) [ 6 ].
Healthcare institutions often face many challenges, ranging from epidemics to determining the most suitable therapies for treating diseases. If an AI technology system is applied to medical research, owing to the development, validation, and deployment of various machine learning algorithms for industrial applications with sustainable performance [ 7 ], it has the potential to diagnose, find vaccines, and personalize healthcare services, moving toward highly advanced e-Health [ 8 ].
Patient-centered care cannot ignore the continuous expansion of data in terms of its volume, variety, and velocity, propelling it toward a new technological paradigm, now widely called BD [ 9 , 10 ]. The analysis of the enormous volume, heterogeneity, and velocity of the information provided by BD allows for the extraction of the greatest value from collected data and successfully solving and analyzing the relationships between different variables that describe a patient’s vital functions and that can affect their health [ 11 ]. These data stimulate healthcare organizations to invest heavily in data analysis to facilitate decision-making [ 12 , 13 ]. Integrating data on an individual’s unique characteristics, clinical phenotypes, and biological information obtained from diagnostic imaging to laboratory tests and medical records enables precision medicine to operate under predictive and preventive conditions [ 14 ]. Having abundant data is crucial, especially in critical care environments, to be able to rapidly identify diagnoses and specific treatments for particular or rare pathological cases [ 15 ]. The improvement of critical stages of diagnosis and the personalization of therapeutic treatments for various diseases are spreading rapidly due to the emerging technological development of BD and the use of social media and IoT that allow for collecting various kinds of data generated by a huge number of devices. In particular, these are biomedical sensors and intelligent devices that, during the diagnosis and monitoring of a patient, collect data related to their health and make them accessible through interconnected and integrated systems, facilitating the transmission of information [ 5 , 16 ].
Currently, the health emergency situation caused by the spread of the COVID-19 disease is increasing the need to develop a BD information system for epidemic- and rapid problem-oriented BD acquisition and integration [ 17 , 18 ]. Previous studies examining BD in healthcare predominantly focused on informatics [ 16 , 19 , 20 ] and medical aspects [ 21 , 22 ]. Few studies have analyzed this topic from a managerial point of view [ 23 , 24 ]. Therefore, this paper contributes to this stream of literature by exploring which tangible and intangible elements are needed to draw the maximum benefits from BD.
Scopus has been used to select the most relevant studies on the role of BD in healthcare to generate knowledge even from the most remote contributions to the literature [ 25 ]. The study shows exponential growth in publications, especially since 2020, highlighting the growing criticality and urgency of healthcare and Industry 4.0 integration. Given the increasing interest in BD in healthcare management, a literature review is useful to understand the challenges and opportunities of BD’s use [ 22 ] for future applications in healthcare.
2. Background
BD is a large collection of data from various healthcare sources that enables increasingly personalized treatments, evaluations of their effectiveness, and a reduction of clinical risk through innovative ways of managing and controlling processes [ 26 , 27 , 28 ].
The literature identifies the main features that characterize BD, also known as the 3Vs [ 28 , 29 ]:
- Volume: amount of data generated every second;
- Variety: different types of data generated, accumulated, and used, even unstructured or semi-structured; and
- Velocity: referring to the generation of data (which is always increasing).
To these first 3Vs, another 3Vs were later added [ 30 , 31 ]:
- Veracity or uncertainty of data;
- Value: BD analytics technologies increase the value of data by transforming it into useful information; and
- Variability: data on the same topic can have differences related to their format or mode of collection, and this is often a limitation.
Then a seventh additional characteristic was found:
- Complexity: the larger the size of the dataset, the greater the complexity of the data to be managed.
Although the importance of BD has emerged particularly in finance, banking, and insurance, one of the most promising and interesting areas in which it can effect significant change is healthcare, although its adoption has been slow [ 32 , 33 ]. Global BD in the healthcare market was expected to grow at a CAGR (Compounded Average Growth Rate) of 20.69% between 2015 and 2021 [ 34 ]. BD is transforming the way healthcare is managed, enabling a revolution in knowledge management and data analytics [ 35 ]. The analysis of the large amount of data generated by a single patient related to diagnosis, treatment pathways, drugs, medical devices, digital images, and laboratory analysis results, to be meaningful, requires that these data be validated, processed, and integrated into processing systems that allow for creating new value in the organization of health services [ 36 ]. The data, therefore, are stored in databases and are efficiently managed [ 9 , 37 ], providing useful insights that otherwise would not have been possible and identifying better solutions in terms of health quality and timely decisions [ 23 , 38 ].
The increasing influence of BD has prompted healthcare organizations to use AI and the skills needed to effectively exploit BDA [ 1 , 24 ]. The quantity of data to manage, analyze, and archive is so large and complex that traditional methods of data processing are inadequate [ 39 ]. The potential acquisition and analysis of BD, in fact, requires the restructuring of the technological infrastructure and integration of traditional data analytical tools and techniques with computational technology that is able to enhance and extract information that will be useful for decision-making [ 2 , 36 , 40 ].
The most relevant sources from which to acquire BD in healthcare are medical recordings (e.g., electronic healthcare records, clinical decision support systems, biomedical data, etc.) [ 41 ] as well as external data sources (laboratories, pharmacies, patient-reported data, biometric and other data received directly from patients, etc.). Additional data sources are increasingly available, such us data derived from Internet use (social media) and smart applications [ 42 , 43 , 44 , 45 ]. For the management and processing of these data, many healthcare sectors have adopted cloud computing. It is a solution for receiving and storing huge amounts of patient data and managing electronic medical records [ 46 ]. These heterogeneous data, when properly integrated with the most relevant health data, allow for the monitoring of patients’ health status in various contexts (hospitals, nursing homes, private homes) [ 5 , 16 , 47 ]. This is an aspect of crucial relevance because the main errors that can lead to a misdiagnosis and case fatality occur due to improper monitoring and administration of therapeutic treatment, as well as to drug non-adherence [ 48 ]. Integrating data on an individual’s unique characteristics, clinical phenotypes, and biological information obtained from imaging to laboratory tests and medical records enables individualized diagnostic or therapeutic solutions [ 14 ].
However, there are still many challenges to be faced. The use of cloud computing and other BD analysis tools and techniques in general, in fact, encounters a number of difficulties represented by network failures, security and privacy issues of patient data, and network downtime [ 46 , 49 , 50 ].
The proliferation of increasingly fast network infrastructures is a phenomenon that is directly proportional to the expansion of possibilities of conveying and exchanging health information, opening up scenarios that were unimaginable until a few years ago. However, today, medicine and scientific research in the medical field are no longer carried out with traditional devices but also, for example, through the so-called smart devices that are increasingly becoming essential elements of daily life [ 51 ].
A product of the technological revolution that began prior to 2000 with the explosion of the Internet, and later with the huge spread of new generation devices connected to it (IoT), is e-Health. In fact, e-Health has huge potential for improving the efficiency of the health system (cutting costs) and effectiveness in the management of patients (understood as the quality of healthcare) [ 52 , 53 ]. The convergence toward a health system, Healthcare 4.0 [ 3 , 4 ] based on smart technologies, IoT [ 5 ], data sharing between different actors [ 6 ], robotics, and cloud computing [ 54 ] can lead to improved healthcare delivery. These IoT devices and sensors also play an essential role in analyzing and predicting new diseases, such as COVID-19 [ 55 ].
The affirmation of new technologies has determined the creation of the Digital Imaging and Communication in Medicine (DICOM) standard, which defines the rules for the storage and sharing of images, going beyond the old generation of analog machines. Another AI application relates to digital reporting techniques—that is, electronic health records (EHR), which in a few years will replace paper media [ 56 ]. To protect patient privacy, EHR must be stored as sensitive information in a secure and reliable manner [ 57 ].
The main health benefits of BD are found in disease prevention, in identifying the main health risk factors, and in designing more effective healthcare measures [ 15 , 58 , 59 ]. The rational use of information and communications technology (ICT) represents a revitalization lever for health systems challenged especially during the COVID-19 health emergency. Enhancing decision-making and operational capabilities, reducing errors, and saving resources are the key benefits. In this view, BD is proving to be an important source with new characteristics, potential, and limitations [ 60 , 61 ].
BD and AI technologies have high predictive capabilities in their application in the treatment of cardiovascular diseases. Studies [ 62 , 63 ] have shown that it is mainly machine learning techniques (k-nearest neighbor, decision tree, linear regression, and support vector machine [SVM]) that improve the accuracy of heart disease detection.
Combinations of machine learning methods with deep learning approaches also enhance the use of neuroimaging data to classify and predict Alzheimer’s disease [ 64 ]. Alzheimer’s is a degenerative neurological disease that impairs a person’s ability to function independently, making early diagnosis critical. Sharma et al. proposed a Hadoop-based BD system for early indicators of the disease. Such a system involves combining data obtained from noninvasive magnetic resonance imaging (MRI), spectrography, magnetic resonance spectroscopy (MRS), and neuropsychological test results [ 65 ]. Kautzky et al. (2018), however, developed a prediction model based on a single diagnostic factor that allows early detection of brain abnormalities even before the onset of symptoms [ 66 ].
An additional degenerative disease in which to employ machine learning is Parkinson’s disease, which affects the neurological system and limits mobility. For early detection of disease symptoms, some studies have used k-nearest neighbor, random forest, and decision tree algorithms [ 67 , 68 ]. Sivaparthipan et al. (2019) also highlighted the importance of data collection using cell phones to recognize the gait of Parkinson’s patients [ 69 ].
Different machine learning (ML) techniques are also used to improve the prediction results for cancer, a major cause of mortality globally [ 70 ]. Torkey et al. (2021) proposed two survival prediction models based on deep learning that can guide physicians in determining breast cancer treatment options and avoid ineffective treatments [ 71 ]. In another study, Torkey et al. (2021) used an ML model that, through the construction of a DNA microarray dataset, allows for the identification of discriminative features that influence the classification of different kinds of cancer and facilitate their early diagnosis [ 72 ].
3. Materials and Methods
A systematic literature review was conducted over the period 2010–2021 to explore the main areas of application of BD in healthcare and the organizational changes needed to address the challenges of applying BD in this area, as well as to illustrate the potential benefits in light of the COVID-19 health emergency that, with its extemporaneity and unpredictability, has severely affected the healthcare management [ 73 ]. A review was also conducted of works from 2022, considering that the scientific production on the investigated topic is already significant.
To ensure a transparent and high-quality process, the analysis comprised four phases [ 68 ]:
- Planning and identification of studies
- Article evaluation
- Extraction of results
- Summary and dissemination of audit results
The analysis was carried out using the Scopus database [ 74 ], and the articles were selected by searching for both “Big Data” and “Healthcare” in the title, abstract, or keywords of an article.
The research conducted, without any restriction on the type of contribution, was delimited with respect to the year of publication and the research area of business, management, and accounting. Subsequently, screening was carried out to assess suitability with respect to the inclusion criteria, first analyzing the relevance of the title, abstract, and then the full text of an article [ 36 ]. Works that were not directly related to the definition, process, and use of BD in healthcare management were excluded. Finally, the remaining 93 papers met all inclusion criteria.
Table 1 shows the steps followed in the search strategy:
Review Strategy.
Table 2 shows the journals that have published the most articles. In particular, International Journal Recent Technology and Engineering presents the highest number of publications (25), covering articles in the areas of computer science and engineering; information technology; electrical and electronics engineering; telecommunication; mechanical, civil, and textile engineering; and all interdisciplinary streams of engineering sciences. This is followed by International Journal of Scientific & Technology Research with 11 publications and Lecture Notes in Business Information Processing with 10 papers in the fields of engineering, science, technology, and industrial application software development. There are many other journals with one paper each.
Top five sources.
Applications of BDA in healthcare are gradually increasing with the growing volume of BD in this context since 2014, with new research areas evolving and applications being explored ( Figure 1 ).

Annual distribution of publications.
The recent literature regarding BD in healthcare discusses the following three themes:
- - BD and health awareness
- - BD and digital transformation
- - BD and analytical skills
This section presents the results of the analysis, highlighting the benefits, challenges, and risks of BD’s use in the healthcare sector.
4.1. Big Data and Health Awareness
The main health benefits of BD are found in disease prevention, in identifying the main health risk factors and in designing more effective healthcare measures [ 58 , 59 , 75 ]. BD, in fact, supports the digitization of all medical records by making all data related to each patient’s medical history available [ 76 ].
The areas that could gain more than others from the benefits of technology and, in particular, from the preservation and sharing of large amounts of clinical data are predictivity, timely diagnosis, and personalized treatment, also favoring the development of precision medicine (patient-centered care) [ 38 , 39 ]. Taking advantage of new biotechnological discoveries allows for going beyond the traditional concept of a “standard patient” to treating the “individual” in their uniqueness [ 77 , 78 ], owing to the analysis of interactions between the different variables that describe the patient’s vital functions and that may affect their health [ 11 ], with enormous benefits for medical functions [ 72 , 73 , 74 , 75 , 76 , 77 , 78 , 79 ]. This improves efficiency and is more patient-centric, yielding consistent, suitable, safe, and flexible solutions [ 39 , 80 ] due to the vigorous analysis by applying various machine learning techniques [ 2 ].
The predictive use of BDA tools allows, especially in cases of health emergencies, for the prompt reporting of high-risk patients and ensuring more effective and efficient care, thus improving overall healthcare outcomes [ 9 , 81 , 82 ]. In fact, the heterogeneity, volume, and velocity of the data contribute to the monitoring of population flows and trends, which are of crucial importance both for early diagnoses and for personalized healthcare services [ 8 , 12 , 13 ]. El Samad et al. (2021), in this regard, conducted a study showing that BD management is considered a key prerogative for the quality of medical services and conditions [ 83 ].
AI-based diagnosis systems and algorithms to detect new outbreaks are just some of the tools that could limit the spread of the SARS-CoV-2 virus and related disease COVID-19, thus maximizing healthcare resources [ 13 , 17 , 84 ] and contributing to the containment of pandemic risk on national territory [ 85 , 86 ]. Abdel-Basset et al. (2021) demonstrated the relevant role that disruptive technologies for COVID-19 analysis, such as AI, Industry 4.0, IoT, Internet of Medical Things (IoMT), BD, virtual reality (VR), drone technology and autonomous robots, 5G, and blockchain, have played in limiting the spread of COVID-19 outbreaks [ 87 ]. Another technology that is being widely deployed is Wireless Body Area Networks (WBANs). This is an innovative solution that can restructure healthcare and make pervasive support available to patients [ 87 , 88 ].
The evolution of digital healthcare into mobile healthcare (mobile health) has also made it possible to manage information via apps that a patient can download directly to their smartphone or tablet [ 89 ], allowing them to monitor their health status independently and share it with their doctor [ 44 , 90 ]. Therefore, IoT allows a much better and timely diagnosis of the patient’s status and offers medical services via telemedicine, even in remote locations [ 58 , 59 , 60 , 61 , 62 , 63 , 64 , 65 , 66 , 67 , 68 , 69 , 70 , 71 , 72 , 73 , 74 , 75 , 76 , 77 , 78 , 79 , 80 , 81 , 82 , 83 , 84 , 85 , 86 , 87 , 88 , 89 , 90 , 91 ] still underdeveloped, where the number of specialists in health services is insufficient [ 92 ]. The use of telemedicine is of fundamental importance in this SARS-CoV-2 pandemic period for preventing and managing COVID-19 infection [ 93 ].
The many applications of medical IoT in the field of monitoring include sensors to monitor vital parameters, such as blood pressure, heart rate, etc.; smart tags (i.e., chips inserted in clothing) with monitoring and data scanning functions; bracelets or other wearable devices capable of detecting vital parameters and forwarding emergency calls in case of anomalies; and Real-Time Location System (RTLS) (i.e., Global Positioning System [GPS]), which is a satellite-based navigation system used to locate ambulances, patients, doctors, etc. [ 5 , 9 ].
Most of these systems are set for patients suffering from dementia [ 94 ] or diabetes [ 95 ] and with vascular pathologies [ 96 ].
The combination and wise and competent use of these BD sources can help health operators undertaking various individual or collective activities, summarized in precision medicine, predictive medicine, and prevention.
Fanta et al. (2021) argued that digital technologies are also a supporting tool in the healthcare sector’s transition to a circular economy. Indeed, such tools, and IoT in particular, can support the collection of end-of-life healthcare products as well as their recycling, regeneration, and disposal [ 97 , 98 ].
Table 3 shows top ten articles per citations on theme “Big Data and awareness”.
Top ten articles per citations on theme 1: Big Data and awareness.
4.2. Big Data and Digital Transformation
The literature review showed that the digital health phenomenon is a true paradigm of innovation that allows for increasing the quality of health services and shaping them according to the needs of the patient, proceeding to the control of their health in real time regardless of geographical location [ 99 , 100 ]. It also requires the main political, legal, and medical players to reconsider the risks associated with the processing of data in the health sector; promote a cultural change, even more than an organizational one, in digital transformation; and investigate new protocols for a more efficient and secure transmission of sensitive health data [ 97 , 98 , 99 , 100 , 101 ].
To manage data in a structured way and address the privacy system effectively and more robustly, healthcare organizations are looking for AI and analytics techniques that will enable them to consolidate organizational resources and develop new data-driven and integrated governance [ 102 ]. Managing an integrated healthcare solution requires security of medical data, which can be achieved with the cryptosystem, which has been found to be highly secure against attacks and interference [ 103 ]. The goal is to allow the development of ways to monitor the health conditions of the population using a huge volume, variety, and velocity of data from a wide range of healthcare networks in an aggregate and anonymous form [ 104 , 105 , 106 ] and improve its performance.
The optimal use of resources has a critically important role for healthcare operators in assessing the quality of the healthcare service provided and also requires appropriate technologies to ensure the rational use of resources [ 107 , 108 , 109 ]. Benzidia et al. (2021) claim that extracting new insights from existing volumes of structured and unstructured data related to medical treatments and products improves decision-making and enables a better understanding of each patient’s costs [ 93 , 110 ].
The result may be an important analytical capability of BD through the definition of previously unobserved patterns and improved resource efficiency through the identification of costly healthcare services, such as unnecessary diagnostic tests and additional treatments [ 19 , 111 ].
Introducing advanced digital solutions to explore huge amounts of heterogeneous and unstructured data requires the design of a clear and integrated strategy across all areas of innovation. It is appropriate to start with understanding the actual level of digital maturity to explore its potential benefits driven by BD analytics and to create value for their healthcare organizations [ 112 , 113 ].
Ultimately, healthcare organizations must begin to develop a concrete analysis of how to apply emerging technologies to methods such as diagnostic procedures, treatment protocol development, patient monitoring, drug development, patient diagnose, and epidemic forecasting [ 45 , 46 , 47 , 48 , 49 , 50 , 51 ]. In this way, risks can be minimized and decisions can be made from the perspective of improved effectiveness and efficiency [ 114 , 115 ].
To accelerate digitalization, hospitals must invest in technology to automate processes and streamline operations, moving in two distinct directions: focusing on the organizational level (moving from episodic to coordinated care), where telemedicine is prioritized, and introducing digital solutions to enable new models of care (progressing toward personalized care and increasing the focus on prevention and wellness) [ 93 , 94 , 95 , 96 , 97 , 98 , 99 , 100 , 101 , 102 , 103 , 104 , 105 , 106 , 107 , 108 , 109 , 110 , 111 , 112 , 113 , 114 , 115 , 116 ].
Implementing a Healthcare 4.0 system requires the careful consideration of process improvement as part of the overall plan to achieve maximum benefits from technology adoption [ 117 ]. In the absence of a strategy that indicates a precise and coherent direction for the evolution of organizational and technological models, it is easy to get lost within an ever-increasing range of technologies, and perhaps end up choosing and introducing advanced digital solutions in an organizational context that is unable to grasp all the advantages to transform the competitive landscape and improve organizational performance [ 52 ]. New technologies enable the creation of high-quality datasets and extract value from them [ 118 , 119 ] but with a rethinking of existing business models [ 120 , 121 ].
Table 4 shows top ten articles per citations on theme “Big Data and digital transformation”.
Top ten articles per citations on theme 2: Big Data and digital transformation.
4.3. Big Data and Analytical Skills
BD can offer a major competitive advantage for healthcare providers, especially with regard to reducing therapeutic mistakes by analyzing patient data [ 120 , 122 ]. In fact, software that uses BD efficiently can flag any inconsistencies between a patient’s medical history and their drug allergies with the medications they are taking, thus alerting the referring physician to discontinue therapy. BD can also identify chronically ill citizens, providing them with preventive actions to avoid clogging emergency channels, such as the emergency room [ 117 , 118 , 123 ].
To seize these important opportunities, however, it is necessary to make a considerable investment in healthcare organizations, for example, in the hiring of analytics experts—that is, professionals capable of identifying problems from the data and proposing the most appropriate solutions [ 23 , 124 , 125 ]. Gravili et al. (2021) highlighted the crucial role of intangible elements, especially of the intellectual capital in the health sector. The dissemination of new knowledge and specialized skills promotes the sharing of best practices in the health sector. The result is a reduction in mortality rates, better outcomes in terms of cost minimization, and a reduction in hospitalization periods [ 115 , 126 , 127 ]. De Mauro et al. (2018) proposed a classification of job roles and skill sets needed in the BD and AI era. This classification provides valuable support for business leaders and human resource managers in the selection process and in developing the skills needed to make the most of BD [ 72 , 128 ].
The management of a highly variable amount of data in real time requires not only new tools and methods but also the development of new knowledge and skills that are essential for converting data into a strategic resource and for implementing new management practices or a new organizational culture across the entire organization. A lack of data analytics skills among existing employees may increase data entry errors that could result in placing information in the wrong record, losing valuable information, and limiting the value a business can derive from the data that it captures [ 129 ]. BD analysis is essential in defining the patient diagnosis. Therefore, doctors and nurses’ understanding of data undoubtedly has a positive impact on the rapid recovery of patients in hospitals [ 62 , 63 ]. These are professionals with technical skills and multidisciplinary knowledge who can manage a huge volume of data and extract useful information to ensure adequate social and healthcare and support the restructuring of healthcare processes [ 64 , 65 ].
Furthermore, process innovation and efficient scheduling are key to addressing bottlenecks in healthcare management [ 64 , 119 ].
Table 5 shows top ten articles per citations on theme 3 “Big Data and analytical skills”.
Top ten articles per citations on theme 3: Big Data and analytical skills.
5. Discussion and Conclusions
In recent years, there has been a process of digitalization and technological innovation in the healthcare sector to enable the transformation of a huge volume of data into valuable health BD, optimize resources, and improve both the patient experience and organizational performance [ 66 ]. The main sources of health data are EHR [ 38 ], medical data [ 130 ], laboratory information systems [ 131 , 132 , 133 , 134 , 135 ], biometric sources, patient-reported data, and social media (wearable devices and sensors that provide information about a patient’s lifestyle) [ 22 , 130 ].
The rapid deployment of new emergency devices (i.e., wireless communications, mobile computing, and mobile devices) and patient monitoring systems has allowed for the focus to be on the design and delivery of digital health services that, leveraging real-time data, foster integrated and effective governance. It is essential to ensure a personalized health service, early disease diagnosis, and support for patient undergoing online care treatments [ 132 ]. The gradual implementation of advanced digital solutions will support the clinical team’s decisions and release time for the most value-added clinical activities and treatment of the most complex cases. BD and AI not only have great potential in the fight against infectious diseases but can also be used for rapid drug and vaccine development [ 130 ].
Despite the important strides made in healthcare digitalization, there are numerous challenges to making the healthcare sector more resilient in the face of health crises. In this regard, it is necessary not only to strengthen the system but also to change its architecture toward a connected care model in which the organization, care, and assistance processes are redefined from a digital perspective and allow for making informed decisions using cutting-edge technology and BDA [ 22 , 134 , 135 ].
The transformation in health information acquisition and informed decision-making using cutting-edge technologies, however, must compromise with the mitigation of privacy associated with patient risks and data confidentiality protection [ 131 , 132 ]. The COVID-19 health emergency has illuminated the need for the careful consideration of the evolving relationship between privacy and public health and the relevance of the public interest in personal data processing activities [ 18 ]. These exceptional and contingent circumstances have highlighted the importance of data protection regulations and cybersecurity investment plans aimed at channeling the flow of BD into healthcare. Indeed, the collection and use of health-related data have been indispensable tools in the effort to counter and contain the pandemic [ 17 ].
Moreover, the evolution of technologies and the competitive environment require the development of new skills in the field of data analysis. In the Fourth Industrial Revolution, people continue to be the most strategic and important component in the business, and it is becoming increasingly strategic to be able to acquire analytical skills to analyze and transform consolidated data from existing fragmented data sources into valuable information for business decision-makers. In this way, it is possible to gain a competitive advantage through timely and more informed decisions based on adequate knowledge of descriptive analytics and predictive analytics, analytical techniques that are ideal for analyzing a large proportion of text-based health documents and other unstructured clinical data [ 135 ].
In conclusion, personalization of care, reduction of hospitalization, and effectiveness and cost containment of services and waiting lists are benefits unquestionably linked to digitalization and technological innovation but that require a review of the systems of traceability and control with a revolution of traditional ICT systems.
This is the challenge that healthcare must overcome. In fact, over the years, analyzing these data and sharing the results with managers and healthcare operators has made it possible to improve the level of knowledge of the system, the sustainability of the healthcare system, its accountability and transparency, and the quality and equity of care.
Our work has theoretical and practical implications. From the theoretical perspective, the paper, by proposing a literature review with a strong focus on managerial aspects, extends the literature by enriching a growing field of research. From the practical perspective, the paper reveals the need to develop new skills and redesign operational and strategic processes to consciously use heterogeneous data in future scenarios.
This paper presents several limitations. First, we used a defined set of keywords and only one database. Second, the research was conducted without programs like VOSviewer that could be used in future studies to identify new clusters related to this topic.
Funding Statement
This research received no external funding.
Author Contributions
Conceptualization, G.G. and G.D.; methodology, M.S.; validation, G.D. and A.M.; formal analysis, G.G.; investigation, G.G.; data curation, M.S.; writing—original draft preparation, G.G.; writing—review and editing, A.M. and G.G.; visualization, M.S.; supervision, G.D. and A.M. All authors have read and agreed to the published version of the manuscript.
Institutional Review Board Statement
Informed consent statement, data availability statement, conflicts of interest.
The authors declare no conflict of interest.
Publisher’s Note: MDPI stays neutral with regard to jurisdictional claims in published maps and institutional affiliations.
A Literature Review on Big Data Analytics Capabilities
Ieee account.
- Change Username/Password
- Update Address
Purchase Details
- Payment Options
- Order History
- View Purchased Documents
Profile Information
- Communications Preferences
- Profession and Education
- Technical Interests
- US & Canada: +1 800 678 4333
- Worldwide: +1 732 981 0060
- Contact & Support
- About IEEE Xplore
- Accessibility
- Terms of Use
- Nondiscrimination Policy
- Privacy & Opting Out of Cookies
A not-for-profit organization, IEEE is the world's largest technical professional organization dedicated to advancing technology for the benefit of humanity. © Copyright 2024 IEEE - All rights reserved. Use of this web site signifies your agreement to the terms and conditions.
Systematic Literature Review of Cloud Computing Research Between 2010 and 2023
- Conference paper
- First Online: 21 May 2024
- Cite this conference paper
- Shailaja Jha 10 &
- Devina Chaturvedi ORCID: orcid.org/0009-0004-1242-2099 11
Part of the book series: Lecture Notes in Business Information Processing ((LNBIP,volume 508))
Included in the following conference series:
- Workshop on e-Business
We present a meta-analysis of cloud computing research in information systems. The study includes 152 referenced journal articles published between January 2010 to June 2023. We take stock of the literature and the associated research themes, research frameworks, the employed research methodology, and the geographical distribution of the articles. This review provides holistic insights into trends in cloud computing research based on themes, frameworks, methodology, geographical focus, and future research directions. The results indicate that the extant literature tends to skew toward themes related to business issues, which is an indicator of the maturing and widespread use of cloud computing. This trend is evidenced in the more recent articles published between 2016 to 2023.
This is a preview of subscription content, log in via an institution to check access.
Access this chapter
- Available as PDF
- Read on any device
- Instant download
- Own it forever
- Available as EPUB and PDF
- Compact, lightweight edition
- Dispatched in 3 to 5 business days
- Free shipping worldwide - see info
Tax calculation will be finalised at checkout
Purchases are for personal use only
Institutional subscriptions
The conference proceedings were primarily used to assess the year-on-year numerical trends in publications, and they have not been used for detailed analysis.
Abdalla Mikhaeil, C., James, T.L.: Examining the case of French hesitancy toward IDaaS solutions: technical and social contextual factors of the organizational IDaaS privacy calculus. Inform. Manage. 60 (4), 103779 (2023)
Google Scholar
Allen, B., et al.: Software as a service for data scientists. Commun. ACM 55 (2), 81–88 (2012)
Andrade-Rojas, M.G., Kathuria, A., Lee, H.-H.: Multilevel synergy of IT operational integration: competition networks and operating performance. Prod. Oper. Manage. (forthcoming) (2024)
Andrade-Rojas, M.G., Saldanha, T., Kathuria, A., Khuntia, J., Boh, W.F.: How IT overcomes deficiencies for innovation in SMEs: closed innovation versus open innovation. Inform. Syst. Res. (forthcoming) (2024)
Anthes, G.: Security in the cloud. Commun. ACM 53 , 16–18 (2010)
Armbrust, M., et al.: A view of cloud computing. Commun. ACM 53 , 50–58 (2010)
August, T., Niculescu, M.F., Shin, H.: Cloud implications on software network structure and security risks. Inform. Syst. Res. 25 , 489–510 (2014)
Bandara, W., Furtmueller, E., Gorbacheva, E., Miskon, S., Beekhuyzen, J.: Achieving rigor in literature reviews: insights from qualitative data analysis and tool-support. Commun. Assoc. Inform. Syst. 37 (8), 154–204 (2015). http://aisel.aisnet.org/cais/vol37/iss1/8
Benlian, A.: Is traditional, open-source, or on-demand first choice? Developing an AHP-based framework for the comparison of different software models in office suites selection. Eur. J. Inform. Syst. 20 , 542–559 (2011)
Benlian, A., Kettinger, W.J., Sunyaev, A., Winkler, T.J.: Special section: the transformative value of cloud computing: a decoupling, platformization, and recombination theoretical framework. J. Manage. Inform. Syst. 35 , 719–739 (2018)
Benlian, A., Koufaris, M., Hess, T.: The role of SaaS service quality for continued SaaS use: Empirical insights from SaaS using firms (2010)
Bhattacherjee, A., Park, S.C.: Why end-users move to the cloud: a migration-theoretic analysis. Eur. J. Inform. Syst. 23, 357–372 (2014)
Chaturvedi, D., Kathuria, A., Andrade, M., Saldanha, T.: Navigating the Paradox of IT Novelty and Strategic Conformity: The Moderating Role of Industry Dynamism (2023)
Chen, F., Lu, A., Wu, H., Li, M.: Compensation and pricing strategies in cloud service SLAs: considering participants’ risk attitudes and consumer quality perception. Electron. Commerce Res. Appl. 56 , 101215 (2022)
Cheng, H.K., Li, Z., Naranjo, A.: Research note—cloud computing spot pricing dynamics: latency and limits to arbitrage. Inform. Syst. Res. 27 , 145–165 (2016)
Choudhary, V., Vithayathil, J.: The impact of cloud computing: should the IT department be organized as a cost center or a profit center? J. Manage. Inform. Syst. 30 , 67–100 (2013)
Choudhary, V., Zhang, Z.: Research note—patching the cloud: the impact of SaaS on patching strategy and the timing of software release. Inform. Syst. Res. 26 , 845–858 (2015)
Dasgupta, A., Karhade, P., Kathuria, A., Konsynski, B.: Holding space for voices that do not speak: design reform of rating systems for platforms in GREAT economies (2021)
Demirkan, H., Cheng, H.K., Bandyopadhyay, S.: Coordination strategies in an SaaS supply chain. J. Manage. Inform. Syst. 26 , 119–143 (2010)
Demirkan, H., Delen, D.: Leveraging the capabilities of service-oriented decision support systems: putting analytics and big data in cloud. Decis. Support Syst. 55 , 412–421 (2013)
Dierks, L., Seuken, S.: Cloud pricing: the spot market strikes back. Manage. Sci. 68 (1), 105–122 (2022)
Article Google Scholar
Ding, S., Xia, C., Wang, C., Desheng, Wu., Zhang, Y.: Multi-objective optimization based ranking prediction for cloud service recommendation. Decis. Support. Syst. 101 , 106–114 (2017)
Dong, L., Shu, W., Sun, D., Li, X., Zhang, L.: Pre-alarm system based on real-time monitoring and numerical simulation using internet of things and cloud computing for tailings dam in mines. IEEE Access 5 , 21080–21089 (2017)
Xin, Du., Tang, S., Zhihui, Lu., Gai, K., Jie, Wu., Hung, P.C.K.: Scientific workflows in IoT environments: a data placement strategy based on heterogeneous edge-cloud computing. ACM Trans. Manage. Inform. Syst. 13 (4), 1–26 (2022)
Ermakova, T., Fabian, B., Kornacka, M., Thiebes, S., Sunyaev, A.: Security and privacy requirements for cloud computing in healthcare: elicitation and prioritization from a patient perspective. ACM Trans. Manage. Inform. Syst. 11 (2), 1–29 (2020)
Garrison, G., Kim, S., Wakefield, R.L.: Success factors for deploying cloud computing. Commun. ACM 55 (9), 62–68 (2012)
Giessmann, A., Legner, C.: Designing business models for cloud platforms. Inf. Syst. J. 26 (5), 551–579 (2016). https://doi.org/10.1111/isj.12107
Gray, A.: Conflict of laws and the cloud. Comput. Law Secur. Rev. 29 (1), 58–65 (2013)
Hosseini, L., Tang, S., Mookerjee, V., Sriskandarajah, C.: A switch in time saves the dime: a model to reduce rental cost in cloud computing. Inform. Syst. Res. 31 (3), 753–775 (2020)
Huang, K.-W., Sundararajan, A.: Pricing digital goods: discontinuous costs and shared infrastructure. Inf. Syst. Res. 22 (4), 721–738 (2011)
Iosup, A., Ostermann, S., Yigitbasi, M.N., Prodan, R., Fahringer, T., Epema, D.H.J.: Performance analysis of cloud computing services for many-tasks scientific computing. IEEE Trans. Parallel Distrib. Syst. 22 , 931–945 (2011)
Iyer, B., Henderson, J.C.: Preparing for the future: understanding the seven capabilities cloud computing. MIS Q. Exec. 9 , 2 (2010)
Jha, S. and Kathuria, A. Size Matters for Cloud Capability and Performance (2022)
Jha, S., Kathuria, A.: How firm age and size influence value creation from cloud computing (2023)
Joe-Wong, C., Sen, S.: Harnessing the power of the cloud: revenue, fairness, and cloud neutrality. J. Manage. Inf. Syst. 35 , 813–836 (2018)
Joint, A., Baker, E.: Knowing the past to understand the present–issues in the contracting for cloud based services. Comput. Law Secur. Rev. 27 (4), 407–415 (2011)
Karhade, P., Kathuria, A.: Missing impact of ratings on platform participation in India: a call for research in GREAT domains. Commun. Assoc. Inf. Syst. 47 (1), 19 (2020)
Karhade, P., Kathuria, A., Dasgupta, A., Malik, O., Konsynski, B.R.: Decolonization of digital platforms: a research agenda for GREAT domains. In: Garimella, A., Karhade, P., Kathuria, A., Liu, X., Xu, J., Zhao, K. (eds.) The Role of e-Business during the Time of Grand Challenges. LNBIP, vol. 418, pp. 51–58. Springer, Cham (2021). https://doi.org/10.1007/978-3-030-79454-5_5
Chapter Google Scholar
Karhade, P., Kathuria, A., Konsynski, B.: When choice matters: assortment and participation for performance on digital platforms (2021)
Kathuria, A., Karhade, P.P., Konsynski, B.R.: In the realm of hungry ghosts: multi-level theory for supplier participation on digital platforms. J. Manag. Inf. Syst. 37 (2), 396–430 (2020)
Kathuria, A., Mann, A., Khuntia, J., Saldanha, T.J.V., Kauffman, R.J.: A strategic value appropriation path for cloud computing. J. Manage. Inf. Syst. 35 (3), 740–775 (2018). https://doi.org/10.1080/07421222.2018.1481635
Kaur, J., Kaur, P.D.: CE-GMS: A cloud IoT-enabled grocery management system. Electron. Commer. Res. Appl. 28 , 63–72 (2018)
Kepes, B.: 30% of servers are sitting “Comatose” according to research. Forbes https://forbes.com/sites/benkepes/2015/06/03/30-of-servers-are-sitting-comatose-according-to-research (2015)
Khokhar, R.H., Fung, B.C.M., Iqbal, F., Alhadidi, D., Bentahar, J.: Privacy-preserving data mashup model for trading person-specific information. Electron. Commer. Res. Appl. 17 , 19–37 (2016)
Khuntia, J., Kathuria, A., Andrade-Rojas, M.G., Saldanha, T., Celly, N.: How foreign and domestic firms differ in leveraging IT-enabled supply chain information integration in BOP markets: the role of supplier and client business collaboration. J. Assoc. Inf. Syst. 22 (3), 6 (2021)
King, W.R., He, J.: Understanding the role and methods of meta-analysis in IS Research. Commun. Assoc. Inf. Syst. 16, 665–686 (2005)
Krancher, O., Luther, P., Jost, M.: Key affordances of Platform-as-a-Service: self-organization and continuous feedback. J. Manage. Inf. Syst. 35 , 776–812 (2018)
Kumar, C., Marston, S., Sen, R., Narisetty, A.: Greening the cloud: a load balancing mechanism to optimize cloud computing networks. J. Manage. Inf. Syst. 39 ,, 513–541 (2022)
Kung, L., Cegielski, C.G., Kung, H.-J.: An integrated environmental perspective on software as a service adoption in manufacturing and retail firms. J. Inf. Technol. 30 , 352–363 (2015)
Lansing, J., Benlian, A., Sunyaev, A.: Unblackboxing” decision makers’ interpretations of IS certifications in the context of cloud service certifications. J. Assoc. Inf. Syst. 19 (11), 1064–1096 (2018)
Lansing, J., Siegfried, N., Sunyaev, A., Benlian, A.: Strategic signaling through cloud service certifications: Comparing the relative importance of certifications’ assurances to companies and consumers. J. Strateg. Inf. Syst. 28 , 101579 (2019)
Lansing, J., Sunyaev, A.: Trust in cloud computing. ACM SIGMIS Database DATABASE Adv. Inform. Syst. 47 , 58–96 (2016)
Lee, J., Cho, D., Lim, G.: Design and validation of the bright internet. J. Assoc. Inform. Syst. 19 , 63–85 (2018)
Lee, M.H., Han, S.P., Park, S., Oh, W.: Positive demand spillover of popular app adoption: implications for platform owners’ management of complements. Inf. Syst. Res. 34 (3), 961–995 (2023)
Li, S., Chen, W., Chen, Y., Chen, C. and Zheng, Z.: Makespan-minimized computation offloading for smart toys in edge-cloud computing. Electron. Commerce Res. Appl. 37 , 100884 (2019)
Li, S., Cheng, H.K., Duan, Y., Yang, Y.-C.: A study of enterprise software licensing models. J. Manag. Inf. Syst. 34 (1), 177–205 (2017)
Lins, S., Schneider, S., Szefer, J., Ibraheem, S., Ali, A.: Designing monitoring systems for continuous certification of cloud services: deriving meta-requirements and design guidelines. Commun. Assoc. Inf. Syst. 44 (1), 460–510 (2019)
Liu, Y., Sheng, X., Marston, S.R.: The impact of client-side security restrictions on the competition of cloud computing services. Int. J. Electron. Comm. 19 (3), 90–117 (2015)
Ma, D., Seidmann, A.: Analyzing software as a service with per-transaction charges. Inf. Syst. Res. 26 , 360–378 (2015)
Malik, O., Jaiswal, A., Kathuria, A., Karhade, P.: Leveraging BI systems to overcome infobesity: a comparative analysis of incumbent and new entrant firms (2022)
Mani, D., Srikanth, K., Bharadwaj, A.: Efficacy of R&D work in offshore captive centers: an empirical study of task characteristics, coordination mechanisms, and performance. Inf. Syst. Res. 25 (4), 846–864 (2014)
Mann, A., Kathuria, A., Khuntia, J., Saldanha, T.: Cloud-integration and business flexibility: the mediating role of cloud functional capabilities (2016)
Marston, S., Li, Z., Bandyopadhyay, S., Zhang, J., Ghalsasi, A.: Cloud computing — the business perspective. Decis. Support. Syst. 51 (1), 176–189 (2011)
Mell, P.M., Grance, T.: The NIST definition of cloud computing. National Institute of Standards and Technology (2011)
Metz, C.: The epic story of dropboxs exodus from the amazon cloud empire (2016)
Mithas, R., Sambamurthy,: How information management capability influences firm performance. MIS Q. 35 (1), 237 (2011)
Mithas, T., Bardhan, G.: Information technology and firm profitability: mechanisms and empirical evidence. MIS Q. 36 (1), 205 (2012)
Muhic, M., Bengtsson, L., Holmström, J.: Barriers to continuance use of cloud computing: evidence from two case studies. Inf. Manage. 60 , 103792 (2023)
Mukherjee, A., Sundarraj, R.P., Dutta, K.: Time-preference-based on-spot bundled cloud-service provisioning. Decis. Support. Syst. 151 , 113607 (2021)
Müller, S.D., Holm, S.R., Søndergaard, J.: Benefits of cloud computing: literature review in a maturity model perspective. Commun. Assoc. Inform. Syst. 37 , 851–878 (2015)
Ojala, A.: Business models and opportunity creation: how IT entrepreneurs create and develop business models under uncertainty. Inf. Syst. J. 26 , 451–476 (2015)
Oliveira, T., Thomas, M., Espadanal, M.: Assessing the determinants of cloud computing adoption: An analysis of the manufacturing and services sectors. Inf. Manage. 51 , 497–510 (2014)
Owens, D. Securing elasticity in the cloud. Communications of the ACM , 53, 6 (2010/06 2010), 46–51 (2010)
Pang, M.-S., Tanriverdi, H.: Strategic roles of IT modernization and cloud migration in reducing cybersecurity risks of organizations: the case of U.S. federal government. J. Strat. Inf. Syst. 31 , 101707 (2022)
Park, J., Han, K., Lee, B.: Green cloud? An empirical analysis of cloud computing and energy efficiency. Manage. Sci. 69 , 1639–1664 (2023)
Parno, B., Howell, J., Gentry, C., Raykova, M.: Pinocchio. Commun. ACM 59 , 103–112 (2016)
Pye, J., Rai, A., Dong, J.Q.: Business value of information technology capabilities: an institutional governance perspective. Inf. Syst. Res. 35 , 28–44 (2023)
Ramakrishnan, T., Kathuria, A., Khuntia, J., Konsynski, B.: IoT value creation through supply chain analytics capability (2022)
Retana, G., Forman, C., Narasimhan, S., Niculescu, M.F., Wu, D.J.: Technical support, knowledge transfer, and service demand: evidence from the cloud. SSRN Electron. J. (2012)
Rodrigues, J., Ruivo, P., Oliveira, T.: Mediation role of business value and strategy in firm performance of organizations using software-as-a-service enterprise applications. Inf. Manag. 58 (1), 103289 (2021)
Saldanha, T.J., Andrade-Rojas, M.G., Kathuria, A., Khuntia, J., Krishnan, M.: How the locus of uncertainty shapes the influence of CEO long-term compensation on IT capital investments. MIS Q. (2023)
Sambhara, C., Rai, A., Xu, S.X.: Configuring the enterprise systems portfolio: the role of information risk. Inf. Syst. Res. 33 (2), 446–463 (2022)
Sarker, S., Chatterjee, S., Xiao, X., Elbanna, A.: The sociotechnical axis of cohesion for the IS discipline: its historical legacy and its continued relevance. MIS Q. 43 (3), 695–720 (2019)
Schlagwein, D., Thorogood, A., Willcocks, L.P.: How commonwealth bank of Australia gained benefits using a standards-based, multi-provider cloud model. MIS Q. Exec. 13 (4), 209–222 (2014)
Schneider, S., Sunyaev, A.: Determinant factors of cloud-sourcing decisions: reflecting on the IT outsourcing literature in the era of cloud computing. J. Inf. Technol. 31 (1), 1–31 (2016). https://doi.org/10.1057/jit.2014.25
Schneider, S., Wollersheim, J., Krcmar, H., Sunyaev, A.: How do Requirements evolve over Time? A case study investigating the role of context and experiences in the evolution of enterprise software requirements. J. Inf. Technol. 33 (2), 151–170 (2018)
Schniederjans, D.G., Hales, D.N.: Cloud computing and its impact on economic and environmental performance: a transaction cost economics perspective. Decis. Support. Syst. 86 , 73–82 (2016)
Schreieck, M., Wiesche, M., Krcmar, H.: Capabilities for value co-creation and value capture in emergent platform ecosystems: a longitudinal case study of SAP’s cloud platform. J. Inf. Technol. 36 (4), 365–390 (2021)
Shiau, W.-L., Chau, P.Y.K.: Understanding behavioral intention to use a cloud computing classroom: a multiple model comparison approach. Inf. Manag. 53 (3), 355–365 (2016)
Singh, V.K., Shivendu, S., Dutta, K.: Spot instance similarity and substitution effect in cloud spot market. Decis. Support. Syst. 159 , 113815 (2022)
Soh, F., Setia, P.: The impact of dominant IT infrastructure in multi-establishment firms: the moderating role of environmental dynamism. J. Assoc. Inf. Syst. 23 (6), 1603–1633 (2022)
Son, I., Lee, D., Lee, J.-N., Chang, Y.B.: Market perception on cloud computing initiatives in organizations: an extended resource-based view. Inf. Manag. 51 (6), 653–669 (2014)
Srinivasan, S.: Is security realistic in cloud computing? J. Int. Technol. Inf. Manag. 22 (4), 3 (2013). https://doi.org/10.58729/1941-6679.1020
Article MathSciNet Google Scholar
Sun, T., Shi, L., Viswanathan, S., Zheleva, E.: Motivating effective mobile app adoptions: evidence from a large-scale randomized field experiment. Inf. Syst. Res. 30 (2), 523–539 (2019)
Templier, M., Paré, G.: Transparency in literature reviews: an assessment of reporting practices across review types and genres in top IS journals. Eur. J. Inf. Syst. 27 (5), 503–550 (2017). https://doi.org/10.1080/0960085X.2017.1398880
Trenz, M., Huntgeburth, J., Veit, D.: Uncertainty in cloud service relationships: uncovering the differential effect of three social influence processes on potential and current users. Inf. Manage. 55, 971–983 (2018)
van de Weerd, I., Mangula, I.S., Brinkkemper, S.: Adoption of software as a service in Indonesia: examining the influence of organizational factors. Inf. Manage. 53 (7), 915–928 (2016)
Venkatesh, V., Bala, H., Sambamurthy, V.: Implementation of an information and communication technology in a developing country: a multimethod longitudinal study in a Bank in India. Inf. Syst. Res. 27 (3), 558–579 (2016)
Venkatesh, V., Sykes, T.A.: Digital divide initiative success in developing countries: a longitudinal field study in a Village in India. Inf. Syst. Res. 24 (2), 239–260 (2013)
Venters, W., Whitley, E.A.: A critical review of cloud computing: researching desires and realities. J. Inf. Technol. 27 (3), 179–197 (2012)
Wang, N., Huigang Liang, Yu., Jia, S.G., Xue, Y., Wang, Z.: Cloud computing research in the IS discipline: a citation/co-citation analysis. Decis. Support. Syst. 86 , 35–47 (2016)
Wang, X., Wang, X.: Multimedia data delivery based on IoT clouds. Commun. ACM 64 (8), 80–86 (2021)
Winkler, T.J., Benlian, A., Piper, M., Hirsch, H.: Bayer healthcare delivers a dose of reality for cloud payoff mantras in multinationals. MIS Q. Exec. 13 , 4 (2014)
Winkler, T.J., Brown, C.V.: Horizontal allocation of decision rights for on-premise applications and Software-as-a-Service. J. Manage. Inf. Syst. 30 (3), 13–48 (2013)
Wright, R.T., Roberts, N., Wilson, D.: The role of context in IT assimilation: a multi-method study of a SaaS platform in the US nonprofit sector. Eur. J. Inf. Syst. 26 (5), 509–539 (2017). https://doi.org/10.1057/s41303-017-0053-2
Wulf, F., Lindner, T., Strahringer, S., Westner, M.: IaaS, PaaS, or SaaS? The why of cloud computing delivery model selection: vignettes on the post-adoption of cloud computing. In: The Proceedings of Proceedings of the 54th Hawaii International Conference on System Sciences, pp. 6285–6294 (2021)
Xiong, Hu., Wang, Yi., Li, W., Chen, C.-M.: Flexible, efficient, and secure access delegation in cloud computing. ACM Trans. Manage. Inf. Syst. 10 (1), 1–20 (2019)
Yang, H., Tate, M.: A descriptive literature review and classification of cloud computing research. Commun. Assoc. Inf. Syst. 31 (1), 2 (2012)
Yaraghi, N., Du, A.Y., Sharman, R., Gopal, R.D., Ramesh, R.: Health Information exchange as a multisided platform: adoption, usage, and practice involvement in service co-production. Inf. Syst. Res. 26 (1), 1–18 (2015)
Yuan, S., Sanjukta Das, R., Ramesh, C.Q.: Service agreement trifecta: backup resources, price and penalty in the availability-aware cloud. Inf. Syst. Res. 29 (4), 947–964 (2018)
Zhang, G., Ravishankar, M.N.: Exploring vendor capabilities in the cloud environment: a case study of Alibaba cloud computing. Inf. Manage. 56 , 343–355 (2019)
Zhang, X., Yue, W.: Integration of on-premises and cloud-based software: the product bundling perspective. J. Assoc. Inform. Syst. 21 , 1507–1551 (2020)
Zorrilla, M., García-Saiz, D.: A service oriented architecture to provide data mining services for non-expert data miners. Decis. Support. Syst.. Support. Syst. 55 (1), 399–411 (2013). https://doi.org/10.1016/j.dss.2012.05.045
Download references
Author information
Authors and affiliations.
SP Jain Institute of Management and Research, Mumbai, India
Shailaja Jha
Indian School of Business, Hyderabad, India
Devina Chaturvedi
You can also search for this author in PubMed Google Scholar
Corresponding author
Correspondence to Devina Chaturvedi .
Editor information
Editors and affiliations.
#6104, Indian School of Business, Hyderabad, Telangana, India
Abhishek Kathuria
Chinese University of Hong Kong, Sha Tin District, Hong Kong
Prasanna P. Karhade
University of North Carolina at Charlotte, Charlotte, NC, USA
Indian School of Business, Hyderabad, Telangana, India
Rights and permissions
Reprints and permissions
Copyright information
© 2024 The Author(s), under exclusive license to Springer Nature Switzerland AG
About this paper
Cite this paper.
Jha, S., Chaturvedi, D. (2024). Systematic Literature Review of Cloud Computing Research Between 2010 and 2023. In: Kathuria, A., Karhade, P.P., Zhao, K., Chaturvedi, D. (eds) Digital Transformation in the Viral Age. WeB 2022. Lecture Notes in Business Information Processing, vol 508. Springer, Cham. https://doi.org/10.1007/978-3-031-60003-6_5
Download citation
DOI : https://doi.org/10.1007/978-3-031-60003-6_5
Published : 21 May 2024
Publisher Name : Springer, Cham
Print ISBN : 978-3-031-60002-9
Online ISBN : 978-3-031-60003-6
eBook Packages : Computer Science Computer Science (R0)
Share this paper
Anyone you share the following link with will be able to read this content:
Sorry, a shareable link is not currently available for this article.
Provided by the Springer Nature SharedIt content-sharing initiative
- Publish with us
Policies and ethics
- Find a journal
- Track your research

IMAGES
VIDEO
COMMENTS
Big Data is still gaining attention as a fundamental building block of the Artificial Intelligence and Machine Learning world. Therefore, a lot of effort has been pushed into Big Data research in the last 15 years. The objective of this Systematic Literature Review is to summarize the current state of the art of the previous 15 years of research about Big Data by providing answers to a set of ...
In particular, our review uses three major criteria (big-data business model types, dimensions, and deployment) to assess the state of the big-data business model literature and identify shortcomings in this literature. On this basis, we derive and discuss five central research perspectives (supply chain, stakeholder, ethics, national, and ...
2.2. Analysis and synthesis of the literature. Our analysis focused on summarizing and analyzing existing theories on big data value realization, highlighting prevailing debates related to this topic, and identifying supporting evidence and gaps in the literature (Jones and Gatrell, 2014).Our aim was to provide new insights that can contribute to future research and thus, to go beyond merely ...
Abstract. In the information era, enormous amounts of data have become available on hand to decision makers. Big data refers to datasets that are not only big, but also high in variety and velocity, which makes them difficult to handle using traditional tools and techniques. Due to the rapid growth of such data, solutions need to be studied and ...
This poses a novel perspective on big data literature, since the vast majority focuses on tools, technical methods (e.g. data mining, textual analysis, and sentiment analysis), network analytics, and infrastructure. ... The main argument of this systematic literature review is that the value of big data does not solely rely on the technologies ...
2.1. Characteristics of big data. The concept of BDA overarches several data-intensive approaches to the analysis and synthesis of large-scale data (Galetsi, Katsaliaki, and Kumar Citation 2020; Mergel, Rethemeyer, and Isett Citation 2016).Such large-scale data derived from information exchange among different systems is often termed 'big data' (Bahri et al. Citation 2018; Khanra, Dhir ...
Systematic literature review (SLR) is a research methodology that examines data and findings of the researchers relative to specified questions [46, 47].It aims to find as much relevant research on the defined questions as possible and to use explicit methods to identify what can reliably be said based on these studies [48, 49].This section provides an SLR to understand the BDM techniques in ...
In this research, a literature review on big data analytics, deep learning and its algorithms, and machine learning and related methods has been considered. As a result, a conceptual model is provided to show the relation of the algorithms that helps researchers and practitioners in deploying BDA on IOT data.
Big Data Analytic s: A Literature Review Pape r. Nada Elgendy and Ahmed Elragal. Department of Busi ness Informatics & Operations, German University in Cairo (GUC), Cairo, Egypt. {nada.el-gendy ...
Abstract. Big data technology has revolutionized the research paradigm of economic forecasting regardless of the data source, forecasting method, or forecasting result. This study evaluates the current literature on economic forecasting using big data and employs bibliometric approaches to offer a comprehensive analysis.
This paper reviews the literature on the relationship between big data, analytics (BDA) and the performance in and of organizations with three bibliometric methods (co-citation analysis, algorithmic historiography and bibliographic coupling). ... (i.e. performance in organizations). Potentially, as a result, our review does not replicate the ...
Abstract. With the boom in Internet techniques and computer science, a variety of big data have been introduced into forecasting research, bringing new knowledge and improving prediction models. This paper is the first attempt to conduct a literature review on full-scale big data in forecasting research.
3. Materials and Methods. A systematic literature review was conducted over the period 2010-2021 to explore the main areas of application of BD in healthcare and the organizational changes needed to address the challenges of applying BD in this area, as well as to illustrate the potential benefits in light of the COVID-19 health emergency that, with its extemporaneity and unpredictability ...
4.3.1. Methods based on statistical analysis. The use of big data in sustainable tourism research should enable local communities to use statistical modelling and forecasting to look forward to what their needs are and mitigate the negative impacts in the future (Caringal et al., 2017 ). Tables 6.
Currently, enormous publications of big data analytics make it difficult for practitioners and researchers to find topics they are interested in and track up to date. This paper aims to present an overview of big data analytics' content, scope and findings as well as opportunities provided by the application of big data analytics.
The study is a comprehensive literature review which does not discuss the big data system architectures in depth, rather focuses on the business and practical aspects of the big data systems. "A general perspective of Big Data: applications, tools, challenges and trends" is another study presenting the main trends, technical domains and ...
the mechanisms and processes through which big data can add business value to companies, and to have a clear picture of the different elements and their interde-pendencies. To this end, the present paper aims to provide a systematic literature review that can help to explain the mechanisms through which big data analytics (BDA) lead to ...
by conducting an in-depth literature review. We adopted a systematic literature review approach and studied academic articles published between 2010 and 2018. We used Scopus and Web of Science (WoS) databases to find published studies related to big data analytics capabilities, twenty-five (25) of which met the selection criteria.
Literatu re Review on Big Data Anal ytics Methods DOI: h p:// dx.doi. org/1 0. 57 72/intec hopen.8684 3 has a n eighbor point that can be rea ched via "move.
The study aims to investigate the big data software architectures based on appli-cation domains assessing the evidence considering the interrelation among the data extraction area and the quality attributes with the systematic literature review methodology which is the suitable research method.
Literature Review Big Data Analytics in the Hospitality Industry. The concept of "Big Data" was initially defined in 2001 by Doug Laney, who identified three primary characteristics known as the "3Vs": Volume (size of data), Variety (different formats/structures of data), and Velocity (rapidity of data generation, modification, and ...
Big data analytics in cancer disease-based systematic literature review is offered, acting as a road map for experts in the area to spot and deal with problems caused by new developments. A comprehensive analysis of the issues and challenges posed by deep learning-based healthcare big data analytics is given, along with a look ahead.
A literature review approach is suitable for emerging topics, such as big data, because, this approach classifies the literature of a research domain, helps understanding a topic in comprehensive perspective, and sheds light on research gaps aiming moving a theme forward (Fosso Wamba et al., 2015; Gunasekaran, Ngai, & McGaughey, 2006; Ngai ...
Revolutionizing Cardiology through Artificial Intelligence—Big Data from Proactive Prevention to Precise Diagnostics and Cutting-Edge Treatment—A Comprehensive Review of the Past 5 Years ... We conducted a comprehensive review of current literature including original articles that studied various clinical applications of AI in cardiology ...
Industrial symbiosis is an urgent priority to circular economy, thus it becomes the frontier and focus of sustainable development research. In this regard, this paper uses knowledge graph visualization technology to analyze the features of industrial symbiosis Publications from 1997 to 2020.The amount of publications, the authors and their affiliations, the journals as well as the Citation ...
Despite recent advances in computer sciences and the rising availability of big data for environmental monitoring and management, this literature review evidenced that the implementation of advanced complex system methods for cumulative risk assessment remains limited. ... Through an iterative scientometric and systematic literature review ...
A literature review can be conducted in different ways: narrative review, descriptive review ... Delen, D.: Leveraging the capabilities of service-oriented decision support systems: putting analytics and big data in cloud. Decis. Support Syst. 55, 412-421 (2013) Google Scholar Dierks, L., Seuken, S.: Cloud pricing: the spot market strikes ...