
An official website of the United States government
The .gov means it’s official. Federal government websites often end in .gov or .mil. Before sharing sensitive information, make sure you’re on a federal government site.
The site is secure. The https:// ensures that you are connecting to the official website and that any information you provide is encrypted and transmitted securely.
- Publications
- Account settings
Preview improvements coming to the PMC website in October 2024. Learn More or Try it out now .
- Advanced Search
- Journal List
- CBE Life Sci Educ
- v.20(1); Spring 2021

To What Extent Do Study Habits Relate to Performance?
Elise m. walck-shannon.
† Biology Department, Washington University in St. Louis, St. Louis, MO 63130
Shaina F. Rowell
‡ Center for Integrative Research on Cognition, Learning, and Education (CIRCLE), Washington University in St. Louis, St. Louis, MO 63130
Regina F. Frey
§ Department of Chemistry, University of Utah, Salt Lake City, UT 84112
Associated Data
Students’ study sessions outside class are important learning opportunities in college courses. However, we often depend on students to study effectively without explicit instruction. In this study, we described students’ self-reported study habits and related those habits to their performance on exams. Notably, in these analyses, we controlled for potential confounds, such as academic preparation, self-reported class absences, and self-reported total study time. First, we found that, on average, students used approximately four active strategies to study and that they spent about half of their study time using active strategies. In addition, both the number of active strategies and the proportion of their study time using active strategies positively predicted exam performance. Second, on average, students started studying 6 days before an exam, but how early a student started studying was not related to performance on in-term (immediate) or cumulative (delayed) exams. Third, on average, students reported being distracted about 20% of their study time, and distraction while studying negatively predicted exam performance. These results add nuance to lab findings and help instructors prioritize study habits to target for change.
INTRODUCTION
One of our goals in college courses is to help students develop into independent, self-regulated learners. This requires students to perform several metacognitive tasks on their own, including setting goals, choosing strategies, monitoring and reflecting on performance, and modifying those steps over time ( Zimmerman, 2002 ). There are many challenges that learners encounter in developing self-regulation. One such challenge is that students often misjudge their learning during the monitoring and reflection phases ( Kornell and Bjork, 2007 ). Often, students feel that they learn more from cognitively superficial tasks than from cognitively effortful tasks. As one example, students may feel that they have learned more if they reread a text passage multiple times than if they are quizzed on that same material ( Karpicke and Roediger, 2008 ). In contrast to students’ judgments, many effortful tasks are highly effective for learning. R. A. Bjork defines these effective, effortful tasks as desirable difficulties ( Bjork, 1994 ). In the present study, we investigated the frequency with which students reported carrying out effortful (active) or superficial (passive) study habits in a large introductory biology course. Additionally, we examined the relationship between study habits and performance on exams while controlling for prior academic preparation and total study time.
THEORETICAL FRAMEWORK
Why would difficulties be desirable.
During learning, the goal is to generate knowledge or skills that are robustly integrated with related knowledge and easily accessible. Desirable difficulties promote cognitive processes that either aid forming robust, interconnected knowledge or skills or retrieving that knowledge or skill ( Bjork, 1994 ; also see Marsh and Butler, 2013 , for a chapter written for educators). Learners employing desirable difficulties may feel that they put in more effort and make more mistakes, but they are actually realizing larger gains toward long-term learning than learners using cognitively superficial tasks.
Which Study Habits Are Difficult in a Desirable Way?
Study habits can include a wide variety of behaviors, from the amount of time that students study, to the strategies that they use while studying, to the environment in which they study. The desirable difficulties framework ( Bjork and Bjork, 2011 ), describes two main kinds of effective habits that apply to our study: 1) using effortful study strategies or techniques that prompt students to generate something or test themselves during studying and 2) distributing study time into multiple sessions to avoid “cramming” near the exam. In the following two paragraphs, we expand upon these study habits of interest.
The desirable difficulties framework suggests that study strategies whereby students actively generate a product or test themselves promote greater long-term learning than study strategies whereby students passively consume presentations. This is supported by strong evidence for the “generation effect,” in which new knowledge or skills are more robustly encoded and retrieved if you generate a solution, explanation, or summary, rather than looking it up ( Jacoby, 1978 ). A few generative strategies that are commonly reported among students—summarization, self-explanation, and practice testing—are compared below. Summarization is a learning strategy in which students identify key points and combine them into a succinct explanation in their own words. As predicted by the generation effect, evidence suggests that summarization is more effective than rewriting notes (e.g., laboratory study by Bretzing and Kulhavy, 1979 ) or reviewing notes (e.g., classroom study by King, 1992 ). Self-explanation is a learning strategy wherein students ask “how” and “why” questions for material as they are being exposed to the material or shortly after ( Berry, 1983 ). This is one form of elaborative interrogation, a robust memory technique in which learners generate more expansive details for new knowledge to help them remember that information ( Pressley et al. , 1987 ). Self-explanation requires little instruction and seems to be helpful for a broad array of tasks, including recall, comprehension, and transfer. Further, it is more effective than summarization (e.g., classroom study by King, 1992 ), perhaps because it prompts students to make additional connections between new and existing knowledge. Practice testing is supported by evidence of the “testing effect,” for which retrieving information itself actually promotes learning ( Karpicke and Roediger, 2008 ). The memory benefits of the “testing effect” can be achieved with any strategy in which students complete problems or practice retrieval without relying on external materials (quizzing, practice testing, flashcards, etc.). In this study, we refer to these strategies together as “self-quizzing.” Self-quizzing is especially effective at improving performance on delayed tests, even as long as 9–11 months after initial learning ( Carpenter, 2009 ). Additionally, in the laboratory, self-quizzing has been shown to be effective on a range of tasks from recall to inference ( Karpicke and Blunt, 2011 ). Overall, research suggests that active, more effortful strategies—such as self-quizzing, summarization, and self-explanation—are more effective for learning than passive strategies—such as rereading and rewriting notes. In this study, we asked whether these laboratory findings would extend to students’ self-directed study time, focusing especially on the effectiveness of effortful (herein, “active”) study strategies.
The second effective habit described by the desirable difficulties framework is to avoid cramming study time near exam time. The “spacing effect” describes the phenomenon wherein, when given equal study time, spacing study out into multiple sessions promotes greater long-term learning than massing (i.e., cramming) study into one study session. Like the “testing effect,” the “spacing effect” is especially pronounced for longer-term tests in the laboratory ( Rawson and Kintsch, 2005 ). Based on laboratory studies, we would expect that, in a course context, cramming study time into fewer sessions close to an exam would be less desirable for long-term learning than distributing study time over multiple sessions, especially if that learning is measured on a delay.
However, estimating spacing in practice is more complicated. Classroom studies have used two main methodologies to estimate spacing, either asking the students to report their study schedules directly ( Susser and McCabe, 2013 ) or asking students to choose whether they describe their pattern of study as spaced out or occurring in one session ( Hartwig and Dunlosky, 2012 ; Rodriguez et al. , 2018 ). The results from these analyses have been mixed; in some cases, spacing has been a significant, positive predictor of performance ( Rodriquez et al. , 2018 ; Susser and McCabe, 2013 ), but in other cases it has not ( Hartwig and Dunlosky, 2012 ).
In the present study, we do not claim measure spacing directly. Lab definitions of spacing are based on studying the same topic over multiple sessions. But, because our exams have multiple topics, some students who start studying early may not revisit the same topic in multiple sessions. Rather, in this study, we measure what we refer to as “spacing potential.” For example, if students study only on the day before the exam, there is little potential for spacing. If, instead, they are studying across 7 days, there is more potential for spacing. We collected two spacing potential measurements: (1) cramming , or the number of days in advance that a student began studying for the exam; and (2) consistency , or the number of days in the week leading up to an exam that a student studied. Based on our measurements, students with a higher spacing potential would exhibit less cramming and study more consistently than students with lower spacing potential. Because not every student with a high spacing potential may actually space out the studying of a single topic into multiple sessions, spacing potential is likely to underestimate the spacing effect; however, it is a practical way to indirectly estimate spacing in practice.
Importantly, not all difficult, or effortful, study tasks are desirable ( Bjork and Bjork, 2011 ). For example, in the present study, we examined students’ level of distraction while studying. Distraction can come in many forms, commonly “multitasking,” or splitting one’s attention among multiple tasks (e.g., watching lectures while also scrolling through social media). However, multitasking has been shown to decrease working memory for the study tasks at hand ( May and Elder, 2018 ). Thus, it may make a task more difficult, but in a way that interferes with learning rather than contributing to it.
In summary, available research suggests that active, effortful study strategies are more effective than passive ones; that cramming is less effective than distributing studying over time; and that focused study is more effective than distracted study. Whether students choose to use these more effective practices during their independent study time is a separate question.
How Do Students Actually Study for Their Courses?
There have been several studies surveying students’ general study habits. When asked free-response questions about their study strategies in general, students listed an average of 2.9 total strategies ( Karpicke et al. , 2009 ). In addition, few students listed active strategies, such as self-quizzing, but many students listed more passive strategies, such as rereading.
There have also been studies asking whether what students actually do while they are studying is related to their achievement. Hartwig and Dunlosky (2012) surveyed 324 college students about their general study habits and found that self-quizzing and rereading were positively correlated with grade point average (GPA). Other studies have shown that using Facebook or texting during study sessions was negatively associated with college GPA ( Junco, 2012 ; Junco and Cotten, 2012 ). While these findings are suggestive, we suspect that the use of study strategies and the relationship between study strategies and achievement may differ from discipline to discipline. The research we have reviewed thus far has been conducted for students’ “general” study habits, rather than for specific courses. To learn about how study habits relate to learning biology, it is necessary to look at study habits within the context of biology courses.
How Do Students Study for Biology Courses?
Several prior qualitative studies carried out within the context of specific biology courses have shown that students often report ineffective habits, such as favoring passive strategies or cramming. Hora and Oleson ( 2017 ) found that, when asked about study habits in focus groups, students in science, technology, engineering, and mathematics (STEM) courses (including biology) used predominantly passive strategies such as reviewing notes or texts, practices that in some cases were unchanged from high school. Tomanek and Montplaisir (2004) found that the majority of 13 interviewed students answered questions on old exams (100% of students) and reread lecture slides (92.3% of students) or the textbook (61.5% of students) to study for a biology exam, but only a small minority participated in deeper tasks such as explaining concepts to a peer (7.7% of students) or generating flashcards for retrieval practice (7.7% of students). We can also learn indirectly about students’ study habits by analyzing what they would change upon reflection. For example, in another study within an introductory biology classroom, Stanton and colleagues ( 2015 ) asked students what they would change about their studying for the next exam. In this context, 13.5% of students said that using active strategies would be more effective for learning, and 55.5% said that they wanted to spend more time studying, many of whom reported following through by studying earlier for the next exam ( Stanton et al. , 2015 ). In the current study, we extended prior research by exploring the prevalence of multiple study habits simultaneously, including the use of active study strategy and study timing, in a large sample of introductory biology students.
In addition to characterizing students’ study habits, we also aimed to show how those study habits were related to performance in a biology classroom. In one existing study, there were positive associations between exam performance and some (but not all) active strategies—such as completing practice exams and taking notes—but no significant associations between performance and some more passive strategies—such as reviewing notes/screencasts or reviewing the textbook ( Sebesta and Bray Speth, 2017 ). In another study, both self-reported study patterns (e.g., spacing studies into multiple sessions or one single session) and self-quizzing were positively related to overall course grade in a molecular biology course ( Rodriguez et al. , 2018 ). We build on this previous work by asking whether associations between performance and a wide variety of study habits still hold when controlling for confounding variables, such as student preparation and total study time.
In this study, we asked whether students actually use cognitive psychologists’ recommendations from the desirable difficulty framework in a specific biology course, and we investigated whether students who reported using those recommendations during studying performed differently on exams than those who did not. We wanted to focus on how students spend their study time, rather than the amount of time that they study, their level of preparation, or engagement. Therefore, we used regression analyses to hold preparation (i.e., ACT math and the course pretest scores), self-reported class absences, and overall study time equal. In this way, we estimated the relationship between particular study habit variables—including the strategies that students use, their timing of using those strategies, and their level of distraction while studying—and exam performance.
Based on previous research and the desirable difficulties framework, we hypothesized that:
- Students would use a combination of active and passive strategies, but those who used more active study strategies or who devoted more of their study time to active strategies would perform higher on their exams than those who used fewer active strategies or devoted less time to active strategies.
- Students would vary in their study timing, but those with less spacing potential (e.g., crammed their study time or studied less consistently) would perform worse, especially on long-term tests (final exam and course posttest), than students with more spacing potential.
- Students would report at least some distraction during their studying, but those who reported being distracted for a smaller percent of their study time would score higher on exams than students who reported being distracted for a larger percent of their study time.
Context and Participants
Data for this study were gathered from a large-enrollment introductory biology course (total class size was 623) during the Spring 2019 semester at a selective, private institution in the Midwest. This course covers basic biochemistry and molecular genetics. It is the first semester of a two-semester sequence. Students who take this course are generally interested in life science majors and/or have pre-health intentions. The data for this study came from an on-campus repository; both the repository and this study have been approved by our internal review board (IRB ID: 201810007 for this study; IRB ID: 201408004 for the repository). There were no exclusion criteria for the study. Anyone who gave consent and for whom all variables were available was considered for the analyses. However, because the variables were different in each analysis, the sample differed slightly from analysis to analysis. When we compared students who were included in the first hypothesis’s analyses to students who gave consent but were not included, we found no significant differences between participants and nonparticipants for ACT math score, pretest score, year in school, sex, or race (Supplemental Table 1). This suggested that our sample did not dramatically differ from the class as a whole.
Other than those analyses labeled “post hoc,” analyses were preplanned before data were retrieved.
Timeline of Assignments Used in This Study
Figure 1 shows a timeline of the assignments analyzed in this study, which included the exam 1 and 2 reflections (both online), exams 1 and 2 (both in person), the course pre and post knowledge tests (both online), and a cumulative final exam (in person). As shown in the text boxes within Figure 1 , the majority (85.7% [430/502] or greater) of students completed each of the assignments that were used in this study.

Timeline of assignments used in this study organized by mode of submission (online vs. in person) and grading (completion vs. accuracy). Exam days are indicated by thick lines. There were other course assignments (including a third exam), but they are not depicted here, because they were not analyzed in this study. Exam return is indicated by dotted lines. Light gray boxes represent weeks that class was in session. The number of consenting students who completed each assignment is indicated in the corresponding assignment box; the total number of consenting students was 502.
Exam Reflections
Students’ responses to exam 1 and 2 study habits reflections were central to all of our hypotheses. In these reflection assignments, students were asked to indicate their study habits leading up to the exam (see Supplemental Item 1 for prompts), including the timing of studying and type of study strategies. The list of strategies for students to choose from came from preliminary analysis of open-response questions in previous years. To increase the likelihood that students accurately remembered their study habits, we made the exercise available online immediately after each exam for 5 days. The reflection assignment was completed before exam grades were returned to students so that their performance did not bias their memory of studying. Students received 0.20% of the total course points for completion of each reflection.
Exams in this course contained both structured-response (multiple-choice, matching, etc.) and free-response questions. The exams were given in person and contained a mixture of lower-order cognitive level (i.e., recall and comprehension) and higher-order cognitive level (i.e., application, analysis, synthesis, or evaluation) questions. Two independent (A.B and G. Y.) raters qualitatively coded exam questions by cognitive level using a rubric slightly modified from Crowe et al. (2008) to bin lower-order and higher-order level questions. This revealed that 38% of exam points were derived from higher-order questions. Each in-term exam was worth 22.5% of the course grade, and the cumulative final exam was worth 25% of the course grade. To prepare for the exams, students were assigned weekly quizzes and were given opportunities for optional practice quizzing and in-class clicker questions as formative assessment. Students were also provided with weekly learning objectives and access to the previous year’s exams. None of the exam questions were identical to questions presented previously in problem sets, old exams, or quizzes. Additionally, in the first week of class, students were given a handout about effective study strategies that included a list of active study techniques along with content-specific examples. Further, on the first quiz, students were asked to determine the most active way to use a particular resource from a list of options. The mean and SD of these exams, and all other variables used in this analysis, can be found in Supplemental Table 2. Pairwise correlations for all variables can be found in Supplemental Table 3
Pre and Post Knowledge Test
As described previously ( Walck-Shannon et al. , 2019 ), the pre/posttest is a multiple-choice test that had been developed by the instructor team. The test contained 38 questions, but the percentage of questions correct is reported here for ease of interpretation. The same test was given online in the first week of classes and after class sessions had ended. One percent extra credit was given to students who completed both tests. To encourage students to participate fully, we presented the pre and posttests as learning opportunities in the course to foreshadow topics for the course (pretest) or review topics for the final (posttest). Additionally, we told students that “reasonable effort” was required for credit. Expressing this rationale seemed to be effective for participation rates. While others have found that participation is low when extra credit is offered as an incentive (38%, Padilla-Walker et al. , 2005 ), we found participation rates for the pre- and posttests to be high; 97.4% of students completed the pretest and 85.9% of students completed the posttest.
Statistical Analyses
To test our three hypotheses, we used hierarchical regression. We controlled for potential confounding variables in step 1 and factored in the study variable of interest at step 2 for each model. We performed the following steps to check that the assumptions of linear regression were met for each model: first, we made scatter plots and found that the relationship was roughly linear, rather than curved; second, we plotted the histogram of residuals and found that they were normally distributed and centered around zero; and finally, we checked for multicollinearity by verifying that no two variables in the model were highly correlated (greater than 0.8). All statistical analyses were performed in JMP Pro (SAS Institute).
Base Model Selection
The purpose of the base model was to account for potential confounding variables. Thus, we included variables that we theoretically expected to explain some variance in exam performance based on previous studies. First, based on a meta-analysis ( Westrick et al. , 2015 ) and our own previous study with a different cohort in this same course ( Walck-Shannon et al. , 2019 ), we expected academic preparation to predict performance. Therefore, we included ACT math and biology pretest scores in our base model. Second, the negative relationship between self-reported class absences and exam or course performance is well documented ( Gump, 2005 ; Lin and Chen, 2006 ; Credé et al. , 2010 ). Therefore, we included the number of class sessions missed in our base model. Finally, our research questions focus on how students use their study time, rather than the relationship between study time itself and performance. Because others have found a small but significant relationship between total study time and performance ( Credé and Kuncel, 2008 ), we controlled for the total number of hours spent studying in our base model. In summary, theoretical considerations of confounds prompted us to include ACT math score, biology pretest score, self-reported class absences, and self-reported exam study time as the base for each model.
Calculated Indices
In the following sections we provide descriptions of variables that were calculated from the reported data. If variables were used directly as input by the student (e.g., class absences, percent of study time distracted) or directly as reported by the registrar (e.g., ACT score), they are not listed below.
Total Exam Study Time.
In students’ exam reflections, they were asked to report both the number of hours that they studied each day in the week leading up to the exam and any hours that they spent studying more than 1 week ahead of the exam. The total exam study time was the sum of these study hours.
Number of Active Strategies Used.
To determine the number of active strategies used, we first had to define which strategies were active. To do so, all authors reviewed literature about desirable difficulties and effective study strategies (also reviewed in Bjork and Bjork, 2011 , and Dunlosky et al. , 2013 , respectively). Then, each author categorized the strategies independently. Finally, we met to discuss until agreement was reached. The resulting categorizations are given in Table 1 . Students who selected “other” and wrote a text description were recoded into existing categories. After the coding was in place, we summed the number of active strategies that each student reported to yield the number of active strategies variable.
Specific study strategy prompts from exam reflections, listed in prevalence of use for exam 1 a
a The classification of the strategy into active and passive is stated in “type.” Prevalences for exam 1 ( n = 424) and exam 2 ( n = 471) are reported.
Proportion of Study Time Using Active Strategies.
In addition to asking students which strategies they used, we also asked them to estimate the percentage of their study time they spent using each strategy. To calculate the proportion of study time using active strategies, we summed the percentages of time using each of the active strategies, then divided by the sum of the percentages for all strategies. For most students (90.0% for exam 1 and 92.8% for exam 2), the sum of all percentages was 100%. However, there were some students whose reported percentages did not add to 100%. If the summed percentages added to between 90 and 110%, they were still included in analyses. If, for example, the sum of all percentages was 90%, and 40% of that was using active strategies, this would become 0.44 (40/90). If the summed percentages were lower than 90% or higher than 110%, students were excluded from the analyses involving the proportion of active study time index.
Number of Days in Advance Studying Began.
In the exam 2 reflection, we asked students to report: 1) their study hours in the week leading up to the exam; and 2) if they began before this time, the total number of hours and date that they began studying. If students did not report any study hours earlier than the week leading up to the exam, we used their first reported study hour as the first day of study. If students did report study time before the week before the exam, we used the reported date that studying began as the first day of study. To get the number of days in advance variable, we counted the number of days between the first day of study and the day of the exam. If a student began studying on exam day, this would be recorded as 0. All students reported some amount of studying.
Number of Days Studied in Week Leading Up to the Exam.
As a measure of studying consistency, we counted the number of days that each student reported studying in the week leading up to exam 2. More specifically, the number of days with nonzero reported study hours were summed to give the number of days studied.
The study strategies that students selected, the timing with which they implemented those strategies, and the level of distraction they reported while doing so are described below. We depict the frequencies with which certain study variables were reported and correlate those study variables to exam 1 and exam 2 scores. For all performance analyses described in the Results section, we first controlled for a base model described below.
We attempted to control for some confounding variables using a base model, which included preparation (ACT math and course pretest percentage), self-reported class absences, and self-reported total study hours. For each analysis, we included all consenting individuals who responded to the relevant reflection questions for the model. Thus, the sample size and values for the variables in the base model differed slightly from analysis to analysis. For brevity, only the first base model is reported in the main text; the other base models included the same variables and are reported in Supplemental Tables 5A, 7A, and 8A.
The base model significantly predicted exam 1 score and exam 2 score for all analyses. Table 2 shows these results for the first analysis; exam 1: R 2 = 0.327, F (4, 419) = 51.010, p < 0.0001; exam 2: R 2 = 0.219, F(4, 466) = 32.751, p < 0.0001. As expected, all individual predictor terms were significant for both exams, with preparation and study time variables positively associated and absences negatively associated. For means and SDs of all continuous variables in this study, see Supplemental Table 2. We found that the preparatory variables were the most predictive, with the course pretest being more predictive than ACT math score. Total study time and class absences were predictive of performance to a similar degree. In summary, our base model accounted for a substantial proportion (32.7%) of the variance due to preparation, class absences, and study time, which allowed us to interpret the relationship between particular study habits and performance more directly.
Base model for hierarchical regression analyses in Table 3 for exam 1 ( n = 424) and exam 2 ( n = 471) a
Did Students Who Used More Active Study Strategies Perform Better on Exams?
We first investigated the specific study strategies listed in Table 1 . Then, we examined the total amount of time spent on active strategies to test our hypothesis that students who spent more time actively studying performed better on exams. Further, we counted the number of different types of active strategies that students used to test whether students who used a more diverse set of active strategies performed better on exams than those who used fewer active strategies.
Study Strategies Differed in Their Frequency of Use and Effectiveness.
The frequency with which specific study strategies were employed is reported in Table 1 . Almost all students reported reading notes. The next most prevalent strategies were active in nature, including that students (in order of prevalence) completed problem sets, completed old exams, self-quizzed, synthesized notes, explained concepts, and made diagrams. Surprisingly, each of these active strategies was used by the majority of students (54.7–86.1%) for both exams 1 and 2 ( Table 1 ). Less frequently used strategies included those more passive in nature, including that students (in order of prevalence) watched lectures, reviewed online content, read the textbook, and rewrote notes. A relatively infrequent strategy was attending review sessions, office hours, and help sessions. Because student engagement varied dramatically in these different venues, we classified this category as mixed. In summary, our results showed that, after reading notes, the most frequently used strategies were active strategies.
Next, we wondered whether the types of strategies that students reported using were related to exam performance. For these analyses, we added whether a student used a specific strategy (0 or 1) into the model, after controlling for the base model reported in Table 2 . When holding preparation, class absences, and total study time equal, we found that, on average, students who reported having completed problem sets, explained concepts, self-quizzed, or attended review sessions earned 4.0–7.7% higher on average on both exams 1 and 2 than students who did not report using the strategy (see b unstd. in Table 3 ). Notably, these strategies were active in nature, except for the category attending review session, which was mixed in nature. The remaining active strategies were positively correlated to performance for only one of the exams. Additionally, we observed that the strategies categorized as passive were either nonsignificant or negatively related to performance on at least one exam. Together, these results suggest that active strategies tended to be positively related to exam performance. In our sample, each of these active strategies was used by the majority (more than half) of the students.
Relating specific study strategy use to performance on exam 1 ( n = 424) and exam 2 ( n = 471) when controlling for preparation, class absences, and total study hours (base model) a
The Proportion of Time Spent Using Active Strategies Positively Predicted Exam Score.
To further understand how active strategies related to performance, we investigated the proportion of study time that students spent using active strategies. On average, students spent about half of their study time using active strategies for exam 1 (M = 0.524, SD = 0.244) and exam 2 (M = 0.548, SD = 0.243), though values varied from 0 to 1 ( Figure 2 ). Importantly, students who spent a larger proportion of their study time on active strategies tended to perform better on exams 1 and 2. More specifically, after accounting for our base model (Supplemental Table 5A), the proportion of time students spent using active strategies added significant additional predictive value for exam 1, F (1, 416) = 8.770, p = 0.003, Δ R 2 = 0.014; and exam 2, F (1, 450) = 14.848, p = 0.0001, Δ R 2 = 0.024. When holding preparation, class absences, and total study time equal, we found that students who spent all of their study time on active strategies scored 5.5% higher and 10.0% higher on exams 1 and 2, respectively, than those who spent none of their study time on active strategies ( Table 4 ). Overall, these two results suggested that, on average, students spent about half of their study time using active strategies and students who devoted more study time to active strategies tended to perform better on exams.

Distribution of the proportion of time that students devoted to active study for exam 1 ( n = 422) and exam 2 ( n = 456). Percentages of students in each bin are indicated.
Relating active study strategy use to performance on exam 1 ( n = 422) and exam 2 ( n = 456) when controlling for preparation, class absences, and total study hours (base model) a
The Number of Active Strategies Used Positively Predicted Exam Score.
We next investigated the number of active strategies used by each student. On average, students used approximately four active strategies for exam 1 (M = 4.212, SD = 1.510) and exam 2 (M = 4.239, SD = 1.501). Very few students used no active strategies and most students (73%) used four or more active strategies ( Figure 3 ). Further, those students who used more active strategies tended to perform higher on exams 1 and 2. More specifically, after accounting for our base model, the number of active strategies students used added significant additional predictive value for exam 1, ( F (1, 416) = 33.698, p < 0.0001 Δ R 2 = 0.024; and exam 2, F (1, 450) = 91.083, p < 0.0001, Δ R 2 = 0.066. When holding preparation, class absences, and total study time equal, we found that, for each additional active strategy used, students scored 1.9% and 2.8% higher on exams 1 and 2, respectively. Students who used all six active strategies scored 11.1% higher and 16.6% higher on exams 1 and 2, respectively, than those who used no active strategies ( Table 4 , See Supplemental Table 5A for base model). In summary, students who used a greater diversity of active strategies tended to perform better on exams.

Distribution of the number of active strategies that each student used for exam 1 ( n = 422) and exam 2 ( n = 456). Percentages of students in each bin are indicated.
Post Hoc Analysis 1: Are Certain Active Strategies Uniquely Predictive of Performance?
Though it was not part of our planned analyses, the previous finding that the number of active strategies is predictive of performance made us question whether certain active strategies are uniquely predictive or whether they each have overlapping benefits. To test this, we added all six of the active strategies into the model as separate variables in the same step. When doing so, we found that the following active strategies were distinctly predictive for both exams 1 and 2: explaining concepts, self-quizzing, and completing problem sets (Supplemental Table 6). In other words, the portion of exam-score variance explained by certain active strategies was non-overlapping.
Did Study Timing Predict Performance on Immediate or Delayed Exams?
We next characterized students’ spacing potential using two indices: 1) the number of days in advance that studying began (cramming) and 2) the number of days in the week leading up to the exam that a student studied (consistency). Notably, in these results, we adjusted for our base model, which included total study time. In this way, we addressed the timing of studying while holding the total amount of studying equal. We examined outcomes at two different times: exam 2, which came close after studying; and the cumulative final exam and the posttest, which came after about a 5-week delay.
Cramming Was Not a Significant Predictor of Exam 2, the Final Exam, or the Posttest.
While there was variation in the degree of cramming among students, this was not predictive of exam score on either immediate or delayed tests. On average, students began studying 5.842 d in advance of exam 2 (SD = 4.377). About a third of students began studying 0–3 days before the exam, and another third began studying 4–6 days before the exam ( Figure 4 A). When holding preparation, class absences, and total study time equal, we found that the number of days in advance that studying began was not a significant predictor of in-term exam 2, the posttest, or the cumulative final ( Table 5 ; see Supplemental Table 7A for base model).

Distributions of spacing potential variables for exam 2 ( n = 450). (A) The distribution of the days in advance that exam 2 studying began (cramming); (B) the distribution of the number of days studied in the week before exam 2 (consistency). Percentages of students in each bin are indicated.
Relating spacing potential to performance on in-term exam 2 ( n = 447), the posttest ( n = 392), and the cumulative final exam ( n = 450) when controlling for preparation, class absences, and total study hours (base model) a
Studying Consistency Was Not a Significant Predictor of Exam 2, the Final Exam, or the Posttest.
While there was variation in how consistently students studied in the week leading up to exam 2, this consistency was not predictive of exam score either immediately or on delayed tests. On average, students studied 5 of the 8 days leading up to the exam (M = 5.082, SD = 1.810 ). Sixteen percent of students studied every day, and no students studied fewer than 2 days in the week leading up to the exam ( Figure 4 B). When holding preparation, class absences, and total study time equal, we found that the number of days studied in the week leading up to the exam was not a significant predictor of in-term exam 2, the posttest, or the cumulative final ( Table 5 ; see Supplemental Table 7A for base model).
In summary, our students varied in both the degree of cramming and the consistency of their studying. Even so, when holding preparation, class absences, and study time equal as part of our base model, neither of these spacing potential measures were predictive of performance on immediate or delayed tests.
Did Students Who Reported Being Less Distracted while Studying Perform Better on Exams?
In addition to the timing of studying, another factor that contextualizes the study strategies is how focused students are during study sessions. In the exam reflections, we asked students how distracted they were while studying. Here, we relate those estimates to exam scores while controlling for our base model of preparation, class absences, and total study time.
Distraction while Studying Was a Negative Predictor of Exam Score.
On average, students reported being distracted during 20% of their exam 1 and exam 2 study time (exam 1: M = 20.733, SD = 16.478; exam 2: M = 20.239, SD = 15.506) . Sixty-one percent of students reported being distracted during more than 10% of their study time ( Figure 5 ). Further, students who were more distracted while studying tended to perform lower on exams 1 and 2. After accounting for our base model, the percent of study time that students reported being distracted added significant additional predictive value for exam 1 and exam 2; exam 1: F (1, 429) = 12.365, p = 0.000, Δ R 2 = 0.019; exam 2: F (1, 467) = 8.942, p = 0.003, Δ R 2 = 0.015. When holding preparation, class absences, and total study time equal, we found that students who reported being distracted 10% more than other students scored about 1% lower on exams 1 and 2 ( Table 6 ; see Supplemental Table 8A for base model). In summary, this suggests that not only was it common for students to be distracted while studying, but this was also negatively related to exam performance.

Distribution of the percent of time students reported being distracted while studying for exam 1 ( n = 435) and exam 2 ( n = 473). Percentages of students in each bin are indicated.
Relating study distraction to performance on exam 1 ( n = 435) and exam 2 ( n = 473) when controlling for preparation, class absences, and total study hours (base model) a
Students’ independent study behaviors are an important part of their learning in college courses. When holding preparation, class absences, and total study time equal, we found that students who spent more time on effortful, active study strategies and used a greater number of active strategies had higher scores for exams. Yet neither students who started studying earlier nor those who studied over more sessions scored differently than students who started later or studied over fewer sessions. Additionally, students who were more distracted while studying tended to perform worse than students who were less distracted. In other words, both the degree to which students employed desirably difficult strategies while studying and their level of focus when doing so were important for performance.
Specific Study Strategies (Hypothesis 1)
Our finding that more time and diversity of active study strategies were associated with higher exam grades was consistent with our hypothesis based on the desirable difficulties framework, laboratory, and classroom research studies ( Berry, 1983 ; King, 1992 ; Bjork, 1994 ; Karpicke and Roediger, 2008 ; Karpicke and Blunt, 2011 ; Hartwig and Dunlosky, 2012 ). Our study brought together lab research about effective strategies with what students did during self-directed study in an actual course. In doing so, we affirmed the lab findings that active strategies are generally effective, but also uncovered further nuances that highlight the value of investigating course-specific study strategies.
First, our study, when combined with other work, may have revealed that certain study strategies are more common than course-nonspecific surveys would predict. For example, compared with surveys of general study habits, our students reported relatively high use of active strategies. We found that the majority of students (73%) reported using four or more active strategies, which was more than the 2.9 average total strategies listed by students in a survey about general study habits at this same institution ( Karpicke et al. , 2009 ). In particular, we found that two-thirds of students reported the active study strategy of self-quizzing. This was considerably higher than what was found in a free-response survey about general habits not focused on a specific course at the same institution ( Karpicke et al. , 2009 ). In this survey, only 10.7% reported self-testing and 40.1% reported using flashcards. This higher frequency of self-quizzing behaviors may be due to a combination of factors in the course, the measures, and/or the students. In this course, we attempted to make self-quizzing easier by reopening the weekly quiz questions near exam time ( Walck-Shannon et al. , 2019 ). We also used a course-specific survey rather than the more general, course-nonspecific surveys used in the previous research. Additionally, it is possible that, in recent years, more students have become more aware of the benefits of self-testing and so are using this strategy with greater frequency. When we compared our frequencies of several categories to analogous categories from course-specific surveys of introductory biology students ( Sebesta and Bray Speth, 2017 ) and molecular biology students ( Rodriguez et al. , 2018 ), we saw similar results. Combined with our work, these studies suggest that when students focused on a particular course, they reported more active strategies than when prompted about studying in general.
Second, the opportunity to control for potential confounding variables in our study, including total study time, allowed us to better estimate the relationships between specific strategies and performance. This approach was important, given concerns raised by others that in classroom studies, benefits of certain strategies, such as explanation, could simply have been due to greater total study time ( Dunlosky et al. , 2013 ). Our results showed that, even when controlling for total study time, self-explanation and other strategies were still significant predictors of performance. This helped illustrate that the strategies themselves, and not just the time on task, were important considerations of students’ study habits.
Third, we were surprised by how predictive the diversity of active strategies was of performance. While we found that the proportion of active study time and the number of active strategies were both important predictors of performance, we found that the latter was a stronger predictor. This suggests that, if total study time was held equal, students who used a larger number of active strategies tended to perform better than those that used a smaller number of active strategies. This finding also deserves to be followed up in subsequent study to determine whether any of the active strategies that students use tend to co-occur in a “suite,” and whether any of those suites are particularly predictive of performance. We suspect that there is some limit to the benefit of using diverse strategies, as some strategies take a considerable amount of time to master ( Bean and Steenwyk, 1984 ; Armbruster et al. , 1987 ; Wong et al. , 2002 ), and students need to devote enough time to each strategy to learn how to use it well.
Additionally, we found that particular active study strategies—explanation, self-quizzing, and answering problem sets—were uniquely predictive of higher performance in a biology course context. Undergraduate biology courses introduce a large amount of discipline-specific terminology, in addition to requiring the higher-order prediction and application skills found among STEM courses ( Wandersee, 1988 ; Zukswert et al. , 2019 ). This is true for the course studied here, which covers biochemistry and molecular genetics, and the assessments that we used as our outcomes reflect this combination of terminology, comprehension, prediction, and application skills. Our results support the finding that active, effortful strategies can be effective on a variety of cognitive levels ( Butler, 2010 ; Karpicke and Blunt, 2011 ; Smith and Karpicke, 2014 ); and this work extends support of the desirable difficulties framework into biology by finding unique value for distinct generative or testing strategies.
Study Timing (Hypothesis 2)
Inconsistent with our second hypothesis that students with less spacing potential would perform worse than students with more spacing potential, we found no relationship between study timing and performance on in-term or cumulative exams. Because we knew that spacing was difficult to estimate, we analyzed two spacing potential indices, the degree of cramming (i.e., the number of days in advance that students started studying) and the consistency of studying (i.e., the number of days studied in the week leading up to the exam). We controlled for total study time, because the spacing effect is defined as identical study time spread over multiple sessions rather than fewer, massed sessions. When doing so, neither of these measures were significantly related to performance.
There are a few possible explanations why we may not have observed a “spacing effect.” First, as explained in the Introduction , we measured spacing potential. It could be that students with high spacing potential may have arranged their studies to mass studying each topic, rather than spacing it out, which would lead us to underestimate the spacing effect. Second, students likely studied again before our cumulative final. This delayed test is where we expected to see the largest effect, and restudying may have masked any spacing effect that did exist. Third, we asked students to directly report their study time, and some may have struggled to remember the exact dates that they studied. While this has the advantage that it results in more sensitive and direct measures of students’ spacing potential than asking students to interpret for themselves whether they binarily spaced their studies or crammed ( Hartwig and Dunlosky, 2012 ; Rodriguez et al. , 2018 ), students who did not remember their study schedules may have reported idealized study schedules with greater spacing, rather than realistic schedules with more cramming ( Susser and McCabe, 2013 ), thus minimizing the expected spacing effect.
Despite the lack of a spacing effect in our data, we certainly do not advocate that students cram their studying, as we find it likely that students who started studying earlier may also have tended to study more. Also, those same students who studied earlier may have felt less stressed and gotten more sleep. In other words, even though our estimation of spacing potential did not capture performance benefits, benefits of spacing for well-being may be multifaceted and not wholly captured by our study.
Distraction (Hypothesis 3)
Consistent with our third hypothesis, we found a negative relationship between distraction while studying and performance. This finding agreed with the few available studies that related distraction during self-directed out-of-class studying and grade, but differs in that our students reported a lower level of distraction than other published studies ( Junco, 2012 ; Junco and Cotten, 2012 ). One possible reason for our low distraction estimate may have been that students were inadvertently underestimating their distraction, as has been reported ( Kraushaar and Novak, 2010 ). In addition, some students may not have been including multitasking as a type of distraction, and this habit of multitasking while studying will likely be difficult to change, as students tend to underestimate how negatively it will affect performance ( Calderwood et al. , 2016 ).
Implications for Instruction
How can we leverage these results to help students change their habits? We present a few ideas of course structural changes that follow from some of the results from this study:
- To encourage students to use more active study strategies, try asking students to turn in the output of the strategy as a low-stakes assignment. For example, to encourage self-explanation, you could ask students to turn in a short video of themselves verbally explaining a concept for credit. To encourage practice quizzing, try to publish or reopen quizzes near exam time ( Walck-Shannon et al. , 2019 ) and ask students to complete them for credit.
- To encourage students to use active study strategies effectively, model those strategies during class. For example, when doing a clicker question, explicitly state your approach to answering the question and self-explain your reasoning out loud. This also gives you an opportunity to add the rationale for why certain strategies are effective or provide advice about carrying them out. In addition to modeling a strategy, remind students to do it often. Simply prompting students to explain their reasoning to their neighbors or themselves during a clicker question helps shifts students’ conversations toward explanation ( Knight et al. , 2013 ).
- To encourage students to stay focused during studying, provide voluntary, structured study sessions. These could include highly structured peer-led team-learning sessions during which students work through a packet of new questions ( Hockings et al. , 2008 ; Snyder et al. , 2015 ) or more relaxed sessions during which students work through problems that have already been provided ( Kudish et al. , 2016 ).
Limitations and Future Directions
There are multiple caveats to these analyses, which may be addressed in future studies. First, our data about study behaviors were self-reported. While we opened the reflection exercise immediately after the exam to mitigate students forgetting their behaviors, some may still have misremembered. Further, some students may not have forgotten, but rather were unable to accurately self-report certain behaviors. As stated earlier, one behavior that is especially prone to this is distraction. But, similarly, we suspect that some students had trouble estimating the percent of study time that they spent using each strategy, while their binary report of whether they used it or not may be more accurate. This may be one reason why the number of active strategies has more explanatory power than the percent of time using an active strategy. Separately, although students were told that we would not analyze their responses until after the semester had ended, some may have conformed their responses to what they thought was desirable. However, there is not strong evidence that students conform their study habit responses to their beliefs about what is effective. For example, Blasiman and colleagues found that, even though students believed rereading was an ineffective strategy, they still reported using it more than other strategies ( Blasiman et al. , 2017 ). Another limitation due to self-reporting is that we lack knowledge of the exact, nuanced behaviors that a student carried out. Thus, a student who chose a strategy that we defined as active—such as “completing problem sets”—may have actually performed more passive behaviors. Specifically, while we did use verbal reminders and delay the release of a key when encouraging students to complete the problem sets and old exams before looking at the answers, some students may have looked up answers prematurely or may have read passively through portions of the key. These more passive behaviors may have underestimated the importance of active strategies. A second limitation is that these data were collected from a course at a selective research-intensive institution and may not be applicable to all student populations. A third limitation is that our analyses are correlational. While we have carefully selected potential confounds, there may be other important confounding variables that we did not account for. Finally, it was beyond the scope of this study to ask whether certain subgroups of students employed different strategies or whether strategies were more or less predictive of performance for different subgroups of students.
Despite these caveats, the main point is clear. Students’ course-specific study habits predict their performance. While many students in our sample reported using effective strategies, some students still had room to improve, especially with their level of distraction. One open question that remains is how we can encourage these students to change their study habits over time.
Supplementary Material
Acknowledgments.
We would like to thank April Bednarski, Kathleen Weston-Hafer, and Barbara Kunkel for their flexibility and feedback on the exam reflection exercises. We would also like to acknowledge Grace Yuan and Ashton Barber for their assistance categorizing exam questions. This research was supported in part by an internal grant titled “Transformational Initiative for Educators in STEM,” which aimed to foster the adoption of evidence-based teaching practices in science classrooms at Washington University in St. Louis.
- Armbruster, B. B., Anderson, T. H., Ostertag, J. (1987). Does text structure/summarization instruction facilitate learning from expository text? Reading Research Quarterly , 22 ( 3 ), 331. [ Google Scholar ]
- Bean, T. W., Steenwyk, F. L. (1984). The effect of three forms of summarization instruction on sixth graders’ summary writing and comprehension . Journal of Reading Behavior , 16 ( 4 ), 297–306. [ Google Scholar ]
- Berry, D. C. (1983). Metacognitive experience and transfer of logical reasoning . Quarterly Journal of Experimental Psychology Section A , 35 ( 1 ), 39–49. [ Google Scholar ]
- Bjork, E. L., Bjork, R. A. (2011). Making things hard on yourself, but in a good way: Creating desirable difficulties to enhance learning . In Gernsbacher, M. A., Pew, R. W., Hough, L. M., Pomerantz, J. R. (Eds.) & FABBS Foundation, Psychology and the real world: Essays illustrating fundamental contributions to society (pp. 56–64). New York, NY: Worth Publishers. [ Google Scholar ]
- Bjork, R. A. (1994). Memory and metamemory considerations in the training of human beings . In Metcalfe, J., Shimamura, A. P. (Eds.), Metacognition: Knowing about knowing (pp. 185–205). Cambridge, MA: Worth Publishers. [ Google Scholar ]
- Blasiman, R. N., Dunlosky, J., Rawson, K. A. (2017). The what, how much, and when of study strategies: Comparing intended versus actual study behaviour . Memory , 25 ( 6 ), 784–792. [ PubMed ] [ Google Scholar ]
- Bretzing, B. H., Kulhavy, R. W. (1979). Notetaking and depth of processing . Contemporary Educational Psychology , 4 ( 2 ), 145–153. [ Google Scholar ]
- Butler, A. C. (2010). Repeated testing produces superior transfer of learning relative to repeated studying . Journal of Experimental Psychology: Learning, Memory, and Cognition , 36 ( 5 ), 1118–1133. [ PubMed ] [ Google Scholar ]
- Calderwood, C., Green, J. D., Joy-Gaba, J. A., Moloney, J. M. (2016). Forecasting errors in student media multitasking during homework completion . Computers and Education , 94 , 37–48. [ Google Scholar ]
- Carpenter, S. K. (2009). Cue strength as a moderator of the testing effect: The benefits of elaborative retrieval . Journal of Experimental Psychology: Learning, Memory, and Cognition , 35 ( 6 ), 1563–1569. [ PubMed ] [ Google Scholar ]
- Credé, M., Kuncel, N. R. (2008). Study habits, skills, and attitudes: The third pillar supporting collegiate academic performance . Perspectives on Psychological Science , 3 ( 6 ), 425–453. [ PubMed ] [ Google Scholar ]
- Credé, M., Roch, S. G., Kieszczynka, U. M. (2010). Class attendance in college . Review of Educational Research , 80 ( 2 ), 272–295. [ Google Scholar ]
- Crowe, A., Dirks, C., Wenderoth, M. P. (2008). Biology in Bloom: Implementing Bloom’s taxonomy to enhance student learning in biology . CBE—Life Sciences Education , 7 ( 4 ), 368–381. [ PMC free article ] [ PubMed ] [ Google Scholar ]
- Dunlosky, J., Rawson, K. A., Marsh, E. J., Nathan, M. J., Willingham, D. T. (2013). Improving students’ learning with effective learning techniques . Psychological Science in the Public Interest , 14 ( 1 ), 4–58. [ PubMed ] [ Google Scholar ]
- Gump, S. E. (2005). The cost of cutting class: Attendance as a predictor of success . College Teaching , 53 ( 1 ), 21–26. [ Google Scholar ]
- Hartwig, M. K., Dunlosky, J. (2012). Study strategies of college students: Are self-testing and scheduling related to achievement? Psychonomic Bulletin & Review , 19 ( 1 ), 126–134. [ PubMed ] [ Google Scholar ]
- Hockings, S. C., DeAngelis, K. J., Frey, R. F. (2008). Peer-led team learning in general chemistry: Implementation and evaluation . Journal of Chemical Education , 85 ( 7 ), 990. [ Google Scholar ]
- Hora, M. T., Oleson, A. K. (2017). Examining study habits in undergraduate STEM courses from a situative perspective . International Journal of STEM Education , 4 ( 1 ), 1. [ Google Scholar ]
- Jacoby, L. L. (1978). On interpreting the effects of repetition: Solving a problem versus remembering a solution . Journal of Verbal Learning and Verbal Behavior , 17 ( 6 ), 649–667. [ Google Scholar ]
- Junco, R. (2012). Too much face and not enough books: The relationship between multiple indices of Facebook use and academic performance . Computers in Human Behavior , 28 ( 1 ), 187–198. [ Google Scholar ]
- Junco, R., Cotten, S. R. (2012). No A 4 U: The relationship between multitasking and academic performance . Computers & Education , 59 ( 2 ), 505–514. [ Google Scholar ]
- Karpicke, J. D., Blunt, J. R. (2011). Retrieval practice produces more learning than elaborative studying with concept mapping . Science , 331 ( 6018 ), 772–775. [ PubMed ] [ Google Scholar ]
- Karpicke, J. D., Butler, A. C., Roediger, H. L. (2009). Metacognitive strategies in student learning: Do students practise retrieval when they study on their own? Memory , 17 ( 4 ), 471–479. [ PubMed ] [ Google Scholar ]
- Karpicke, J. D., Roediger, H. L. (2008). The critical importance of retrieval for learning . Science , 319 ( 5865 ), 966–968. [ PubMed ] [ Google Scholar ]
- King, A. (1992). Comparison of self-questioning, summarizing, and notetaking-review as strategies for learning from lectures . American Educational Research Journal , 29 ( 2 ), 303. [ Google Scholar ]
- Knight, J. K., Wise, S. B., Southard, K. M. (2013). Understanding clicker discussions: Student reasoning and the impact of instructional cues . CBE—Life Sciences Education , 12 ( 4 ), 645–654. [ PMC free article ] [ PubMed ] [ Google Scholar ]
- Kornell, N., Bjork, R. A. (2007). The promise and perils of self-regulated study . Psychonomic Bulletin & Review , 14 ( 2 ), 219–224. [ PubMed ] [ Google Scholar ]
- Kraushaar, J. M., Novak, D. (2010). Examining the effects of student multitasking with laptops during the lecture . Journal of Information Systems Education , 21 ( 2 ), 241–251. [ Google Scholar ]
- Kudish, P., Shores, R., McClung, A., Smulyan, L., Vallen, E. A., Siwicki, K. K. (2016). Active learning outside the classroom: Implementation and outcomes of peer-led team-learning workshops in introductory biology . CBE—Life Sciences Education , 15 ( 3 ), ar31. [ PMC free article ] [ PubMed ] [ Google Scholar ]
- Lin, T. F., Chen, J. (2006). Cumulative class attendance and exam performance . Applied Economics Letters , 13 ( 14 ), 937–942. [ Google Scholar ]
- Marsh, E. J., Butler, A. C. (2013). Memory in educational settings . In Reisberg, D. (Ed.), Oxford library of psychology. The Oxford handbook of cognitive psychology (pp. 299–317). Washington, DC: Oxford University Press. [ Google Scholar ]
- May, K. E., Elder, A. D. (2018). Efficient, helpful, or distracting? A literature review of media multitasking in relation to academic performance . International Journal of Educational Technology in Higher Education , 15 ( 1 ), 13. [ Google Scholar ]
- Padilla-Walker, L. M., Thompson, R. A., Zamboanga, B. L., Schmersal, L. A. (2005). Extra credit as incentive for voluntary research participation . Teaching of Psychology , 32 ( 3 ), 150–153. [ Google Scholar ]
- Pressley, M., McDaniel, M. A., Turnure, J. E., Wood, E., Ahmad, M. (1987). Generation and precision of elaboration: Effects on intentional and incidental learning . Journal of Experimental Psychology: Learning, Memory, and Cognition , 13 ( 2 ), 291–300. [ Google Scholar ]
- Rawson, K. A., Kintsch, W. (2005). Rereading effects depend on time of test . Journal of Educational Psychology , 97 ( 1 ), 70–80. [ Google Scholar ]
- Rodriquez, F., Rivas, M. J., Matsumura, L. H., Warschauer, M., Sato, B. K. (2018). How do students study in STEM courses? Findings from a light-touch intervention and its relevance for underrepresented students . PLoS ONE , 13 ( 7 ), e0200767. [ PMC free article ] [ PubMed ] [ Google Scholar ]
- Sebesta, A. J., Bray Speth, E. (2017). How should I study for the exam? Self-regulated learning strategies and achievement in introductory biology . CBE—Life Sciences Education , 16 ( 2 ), ar30. [ PMC free article ] [ PubMed ] [ Google Scholar ]
- Smith, M. A., Karpicke, J. D. (2014). Retrieval practice with short-answer, multiple-choice, and hybrid tests . Memory , 22 ( 7 ), 784–802. [ PubMed ] [ Google Scholar ]
- Snyder, J. J., Elijah Carter, B., Wiles, J. R. (2015). Implementation of the peer-led team-learning instructional model as a stopgap measure improves student achievement for students opting out of laboratory . CBE—Life Sciences Education , 14 ( 1 ), ar2. [ PMC free article ] [ PubMed ] [ Google Scholar ]
- Stanton, J. D., Neider, X. N., Gallegos, I. J., Clark, N. C. (2015). Differences in metacognitive regulation in introductory biology students: When prompts are not enough . CBE—Life Sciences Education , 14 ( 2 ), ar15. [ PMC free article ] [ PubMed ] [ Google Scholar ]
- Susser, J. A., McCabe, J. (2013). From the lab to the dorm room: Metacognitive awareness and use of spaced study . Instructional Science , 41 ( 2 ), 345–363. [ Google Scholar ]
- Tomanek, D., Montplaisir, L. (2004). Students’ studying and approaches to learning in introductory biology . Cell Biology Education , 3 ( 4 ), 253–262. [ PMC free article ] [ PubMed ] [ Google Scholar ]
- Walck-Shannon, E. M., Cahill, M. J., McDaniel, M. A., Frey, R. F. (2019). Participation in voluntary re-quizzing is predictive of increased performance on cumulative assessments in introductory biology . CBE—Life Sciences Education , 18 ( 2 ), ar15. [ PMC free article ] [ PubMed ] [ Google Scholar ]
- Wandersee, J. H. (1988). The terminology problem in biology education: A reconnaissance . American Biology Teacher , 50 ( 2 ), 97–100. [ Google Scholar ]
- Westrick, P. A., Le, H., Robbins, S. B., Radunzel, J. M. R., Schmidt, F. L. (2015). College performance and retention: A meta-analysis of the predictive validities of ACT® scores, high school grades, and SES . Educational Assessment , 20 ( 1 ), 23–45. [ Google Scholar ]
- Wong, R. M. F., Lawson, M. J., Keeves, J. (2002). The effects of self-explanation training on students’ problem solving in high-school mathematics . Learning and Instruction , 12 ( 2 ), 233–262. [ Google Scholar ]
- Zimmerman, B. J. (2002). Becoming a self-regulated learner: An overview . Theory into Practice , 41 ( 2 ), 64–70. [ Google Scholar ]
- Zukswert, J. M., Barker, M. K., McDonnell, L. (2019). Identifying troublesome jargon in biology: Discrepancies between student performance and perceived understanding . CBE—Life Sciences Education , 18 ( 1 ), ar6. [ PMC free article ] [ PubMed ] [ Google Scholar ]
Thank you for visiting nature.com. You are using a browser version with limited support for CSS. To obtain the best experience, we recommend you use a more up to date browser (or turn off compatibility mode in Internet Explorer). In the meantime, to ensure continued support, we are displaying the site without styles and JavaScript.
- View all journals
- My Account Login
- Explore content
- About the journal
- Publish with us
- Sign up for alerts
- Open access
- Published: 02 December 2020
Enhancing senior high school student engagement and academic performance using an inclusive and scalable inquiry-based program
- Locke Davenport Huyer ORCID: orcid.org/0000-0003-1526-7122 1 , 2 na1 ,
- Neal I. Callaghan ORCID: orcid.org/0000-0001-8214-3395 1 , 3 na1 ,
- Sara Dicks 4 ,
- Edward Scherer 4 ,
- Andrey I. Shukalyuk 1 ,
- Margaret Jou 4 &
- Dawn M. Kilkenny ORCID: orcid.org/0000-0002-3899-9767 1 , 5
npj Science of Learning volume 5 , Article number: 17 ( 2020 ) Cite this article
42k Accesses
4 Citations
13 Altmetric
Metrics details
The multi-disciplinary nature of science, technology, engineering, and math (STEM) careers often renders difficulty for high school students navigating from classroom knowledge to post-secondary pursuits. Discrepancies between the knowledge-based high school learning approach and the experiential approach of future studies leaves some students disillusioned by STEM. We present Discovery , a term-long inquiry-focused learning model delivered by STEM graduate students in collaboration with high school teachers, in the context of biomedical engineering. Entire classes of high school STEM students representing diverse cultural and socioeconomic backgrounds engaged in iterative, problem-based learning designed to emphasize critical thinking concomitantly within the secondary school and university environments. Assessment of grades and survey data suggested positive impact of this learning model on students’ STEM interests and engagement, notably in under-performing cohorts, as well as repeating cohorts that engage in the program on more than one occasion. Discovery presents a scalable platform that stimulates persistence in STEM learning, providing valuable learning opportunities and capturing cohorts of students that might otherwise be under-engaged in STEM.
Similar content being viewed by others
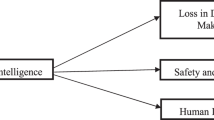
Impact of artificial intelligence on human loss in decision making, laziness and safety in education
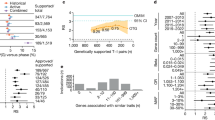
Refining the impact of genetic evidence on clinical success
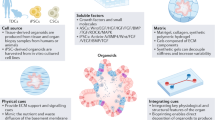
Introduction
High school students with diverse STEM interests often struggle to understand the STEM experience outside the classroom 1 . The multi-disciplinary nature of many career fields can foster a challenge for students in their decision to enroll in appropriate high school courses while maintaining persistence in study, particularly when these courses are not mandatory 2 . Furthermore, this challenge is amplified by the known discrepancy between the knowledge-based learning approach common in high schools and the experiential, mastery-based approaches afforded by the subsequent undergraduate model 3 . In the latter, focused classes, interdisciplinary concepts, and laboratory experiences allow for the application of accumulated knowledge, practice in problem solving, and development of both general and technical skills 4 . Such immersive cooperative learning environments are difficult to establish in the secondary school setting and high school teachers often struggle to implement within their classroom 5 . As such, high school students may become disillusioned before graduation and never experience an enriched learning environment, despite their inherent interests in STEM 6 .
It cannot be argued that early introduction to varied math and science disciplines throughout high school is vital if students are to pursue STEM fields, especially within engineering 7 . However, the majority of literature focused on student interest and retention in STEM highlights outcomes in US high school learning environments, where the sciences are often subject-specific from the onset of enrollment 8 . In contrast, students in the Ontario (Canada) high school system are required to complete Level 1 and 2 core courses in science and math during Grades 9 and 10; these courses are offered as ‘applied’ or ‘academic’ versions and present broad topics of content 9 . It is not until Levels 3 and 4 (generally Grades 11 and 12, respectively) that STEM classes become subject-specific (i.e., Biology, Chemistry, and/or Physics) and are offered as “university”, “college”, or “mixed” versions, designed to best prepare students for their desired post-secondary pursuits 9 . Given that Levels 3 and 4 science courses are not mandatory for graduation, enrollment identifies an innate student interest in continued learning. Furthermore, engagement in these post-secondary preparatory courses is also dependent upon achieving successful grades in preceding courses, but as curriculum becomes more subject-specific, students often yield lower degrees of success in achieving course credit 2 . Therefore, it is imperative that learning supports are best focused on ensuring that those students with an innate interest are able to achieve success in learning.
When given opportunity and focused support, high school students are capable of successfully completing rigorous programs at STEM-focused schools 10 . Specialized STEM schools have existed in the US for over 100 years; generally, students are admitted after their sophomore year of high school experience (equivalent to Grade 10) based on standardized test scores, essays, portfolios, references, and/or interviews 11 . Common elements to this learning framework include a diverse array of advanced STEM courses, paired with opportunities to engage in and disseminate cutting-edge research 12 . Therein, said research experience is inherently based in the processes of critical thinking, problem solving, and collaboration. This learning framework supports translation of core curricular concepts to practice and is fundamental in allowing students to develop better understanding and appreciation of STEM career fields.
Despite the described positive attributes, many students do not have the ability or resources to engage within STEM-focused schools, particularly given that they are not prevalent across Canada, and other countries across the world. Consequently, many public institutions support the idea that post-secondary led engineering education programs are effective ways to expose high school students to engineering education and relevant career options, and also increase engineering awareness 13 . Although singular class field trips are used extensively to accomplish such programs, these may not allow immersive experiences for application of knowledge and practice of skills that are proven to impact long-term learning and influence career choices 14 , 15 . Longer-term immersive research experiences, such as after-school programs or summer camps, have shown successful at recruiting students into STEM degree programs and careers, where longevity of experience helps foster self-determination and interest-led, inquiry-based projects 4 , 16 , 17 , 18 , 19 .
Such activities convey the elements that are suggested to make a post-secondary led high school education programs successful: hands-on experience, self-motivated learning, real-life application, immediate feedback, and problem-based projects 20 , 21 . In combination with immersion in university teaching facilities, learning is authentic and relevant, similar to the STEM school-focused framework, and consequently representative of an experience found in actual STEM practice 22 . These outcomes may further be a consequence of student engagement and attitude: Brown et al. studied the relationships between STEM curriculum and student attitudes, and found the latter played a more important role in intention to persist in STEM when compared to self-efficacy 23 . This is interesting given that student self-efficacy has been identified to influence ‘motivation, persistence, and determination’ in overcoming challenges in a career pathway 24 . Taken together, this suggests that creation and delivery of modern, exciting curriculum that supports positive student attitudes is fundamental to engage and retain students in STEM programs.
Supported by the outcomes of identified effective learning strategies, University of Toronto (U of T) graduate trainees created a novel high school education program Discovery , to develop a comfortable yet stimulating environment of inquiry-focused iterative learning for senior high school students (Grades 11 & 12; Levels 3 & 4) at non-specialized schools. Built in strong collaboration with science teachers from George Harvey Collegiate Institute (Toronto District School Board), Discovery stimulates application of STEM concepts within a unique term-long applied curriculum delivered iteratively within both U of T undergraduate teaching facilities and collaborating high school classrooms 25 . Based on the volume of medically-themed news and entertainment that is communicated to the population at large, the rapidly-growing and diverse field of biomedical engineering (BME) were considered an ideal program context 26 . In its definition, BME necessitates cross-disciplinary STEM knowledge focused on the betterment of human health, wherein Discovery facilitates broadening student perspective through engaging inquiry-based projects. Importantly, Discovery allows all students within a class cohort to work together with their classroom teacher, stimulating continued development of a relevant learning community that is deemed essential for meaningful context and important for transforming student perspectives and understandings 27 , 28 . Multiple studies support the concept that relevant learning communities improve student attitudes towards learning, significantly increasing student motivation in STEM courses, and consequently improving the overall learning experience 29 . Learning communities, such as that provided by Discovery , also promote the formation of self-supporting groups, greater active involvement in class, and higher persistence rates for participating students 30 .
The objective of Discovery , through structure and dissemination, is to engage senior high school science students in challenging, inquiry-based practical BME activities as a mechanism to stimulate comprehension of STEM curriculum application to real-world concepts. Consequent focus is placed on critical thinking skill development through an atmosphere of perseverance in ambiguity, something not common in a secondary school knowledge-focused delivery but highly relevant in post-secondary STEM education strategies. Herein, we describe the observed impact of the differential project-based learning environment of Discovery on student performance and engagement. We identify the value of an inquiry-focused learning model that is tangible for students who struggle in a knowledge-focused delivery structure, where engagement in conceptual critical thinking in the relevant subject area stimulates student interest, attitudes, and resulting academic performance. Assessment of study outcomes suggests that when provided with a differential learning opportunity, student performance and interest in STEM increased. Consequently, Discovery provides an effective teaching and learning framework within a non-specialized school that motivates students, provides opportunity for critical thinking and problem-solving practice, and better prepares them for persistence in future STEM programs.
Program delivery
The outcomes of the current study result from execution of Discovery over five independent academic terms as a collaboration between Institute of Biomedical Engineering (graduate students, faculty, and support staff) and George Harvey Collegiate Institute (science teachers and administration) stakeholders. Each term, the program allowed senior secondary STEM students (Grades 11 and 12) opportunity to engage in a novel project-based learning environment. The program structure uses the problem-based engineering capstone framework as a tool of inquiry-focused learning objectives, motivated by a central BME global research topic, with research questions that are inter-related but specific to the curriculum of each STEM course subject (Fig. 1 ). Over each 12-week term, students worked in teams (3–4 students) within their class cohorts to execute projects with the guidance of U of T trainees ( Discovery instructors) and their own high school teacher(s). Student experimental work was conducted in U of T teaching facilities relevant to the research study of interest (i.e., Biology and Chemistry-based projects executed within Undergraduate Teaching Laboratories; Physics projects executed within Undergraduate Design Studios). Students were introduced to relevant techniques and safety procedures in advance of iterative experimentation. Importantly, this experience served as a course term project for students, who were assessed at several points throughout the program for performance in an inquiry-focused environment as well as within the regular classroom (Fig. 1 ). To instill the atmosphere of STEM, student teams delivered their outcomes in research poster format at a final symposium, sharing their results and recommendations with other post-secondary students, faculty, and community in an open environment.
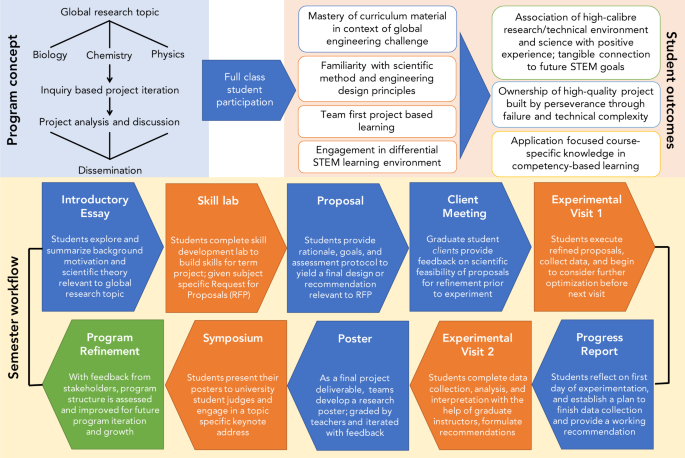
The general program concept (blue background; top left ) highlights a global research topic examined through student dissemination of subject-specific research questions, yielding multifaceted student outcomes (orange background; top right ). Each program term (term workflow, yellow background; bottom panel ), students work on program deliverables in class (blue), iterate experimental outcomes within university facilities (orange), and are assessed accordingly at numerous deliverables in an inquiry-focused learning model.
Over the course of five terms there were 268 instances of tracked student participation, representing 170 individual students. Specifically, 94 students participated during only one term of programming, 57 students participated in two terms, 16 students participated in three terms, and 3 students participated in four terms. Multiple instances of participation represent students that enrol in more than one STEM class during their senior years of high school, or who participated in Grade 11 and subsequently Grade 12. Students were surveyed before and after each term to assess program effects on STEM interest and engagement. All grade-based assessments were performed by high school teachers for their respective STEM class cohorts using consistent grading rubrics and assignment structure. Here, we discuss the outcomes of student involvement in this experiential curriculum model.
Student performance and engagement
Student grades were assigned, collected, and anonymized by teachers for each Discovery deliverable (background essay, client meeting, proposal, progress report, poster, and final presentation). Teachers anonymized collective Discovery grades, the component deliverable grades thereof, final course grades, attendance in class and during programming, as well as incomplete classroom assignments, for comparative study purposes. Students performed significantly higher in their cumulative Discovery grade than in their cumulative classroom grade (final course grade less the Discovery contribution; p < 0.0001). Nevertheless, there was a highly significant correlation ( p < 0.0001) observed between the grade representing combined Discovery deliverables and the final course grade (Fig. 2a ). Further examination of the full dataset revealed two student cohorts of interest: the “Exceeds Expectations” (EE) subset (defined as those students who achieved ≥1 SD [18.0%] grade differential in Discovery over their final course grade; N = 99 instances), and the “Multiple Term” (MT) subset (defined as those students who participated in Discovery more than once; 76 individual students that collectively accounted for 174 single terms of assessment out of the 268 total student-terms delivered) (Fig. 2b, c ). These subsets were not unrelated; 46 individual students who had multiple experiences (60.5% of total MTs) exhibited at least one occasion in achieving a ≥18.0% grade differential. As students participated in group work, there was concern that lower-performing students might negatively influence the Discovery grade of higher-performing students (or vice versa). However, students were observed to self-organize into groups where all individuals received similar final overall course grades (Fig. 2d ), thereby alleviating these concerns.
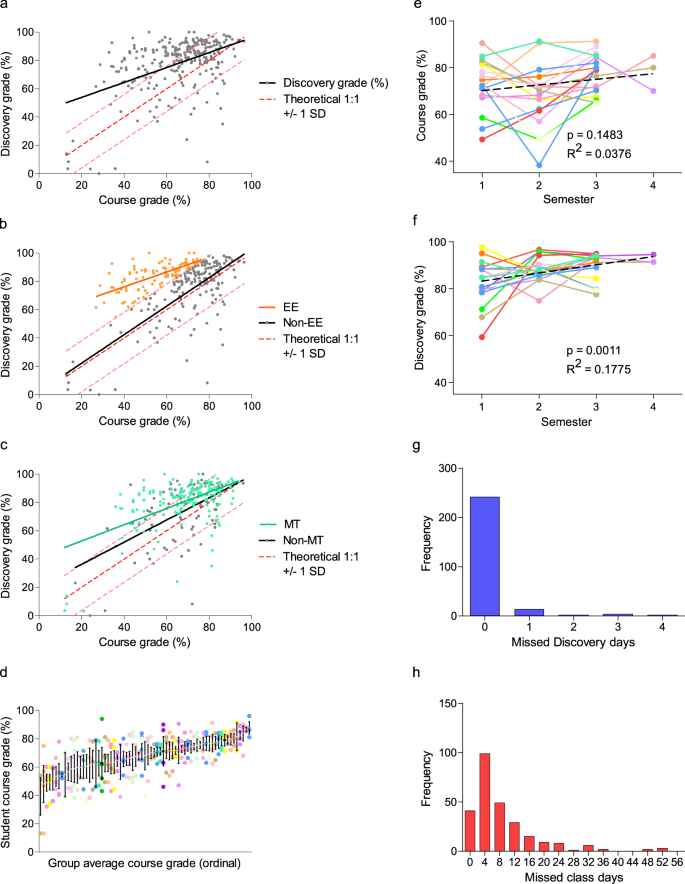
a Linear regression of student grades reveals a significant correlation ( p = 0.0009) between Discovery performance and final course grade less the Discovery contribution to grade, as assessed by teachers. The dashed red line and intervals represent the theoretical 1:1 correlation between Discovery and course grades and standard deviation of the Discovery -course grade differential, respectively. b , c Identification of subgroups of interest, Exceeds Expectations (EE; N = 99, orange ) who were ≥+1 SD in Discovery -course grade differential and Multi-Term (MT; N = 174, teal ), of which N = 65 students were present in both subgroups. d Students tended to self-assemble in working groups according to their final course performance; data presented as mean ± SEM. e For MT students participating at least 3 terms in Discovery , there was no significant correlation between course grade and time, while ( f ) there was a significant correlation between Discovery grade and cumulative terms in the program. Histograms of total absences per student in ( g ) Discovery and ( h ) class (binned by 4 days to be equivalent in time to a single Discovery absence).
The benefits experienced by MT students seemed progressive; MT students that participated in 3 or 4 terms ( N = 16 and 3, respectively ) showed no significant increase by linear regression in their course grade over time ( p = 0.15, Fig. 2e ), but did show a significant increase in their Discovery grades ( p = 0.0011, Fig. 2f ). Finally, students demonstrated excellent Discovery attendance; at least 91% of participants attended all Discovery sessions in a given term (Fig. 2g ). In contrast, class attendance rates reveal a much wider distribution where 60.8% (163 out of 268 students) missed more than 4 classes (equivalent in learning time to one Discovery session) and 14.6% (39 out of 268 students) missed 16 or more classes (equivalent in learning time to an entire program of Discovery ) in a term (Fig. 2h ).
Discovery EE students (Fig. 3 ), roughly by definition, obtained lower course grades ( p < 0.0001, Fig. 3a ) and higher final Discovery grades ( p = 0.0004, Fig. 3b ) than non-EE students. This cohort of students exhibited program grades higher than classmates (Fig. 3c–h ); these differences were significant in every category with the exception of essays, where they outperformed to a significantly lesser degree ( p = 0.097; Fig. 3c ). There was no statistically significant difference in EE vs. non-EE student classroom attendance ( p = 0.85; Fig. 3i, j ). There were only four single day absences in Discovery within the EE subset; however, this difference was not statistically significant ( p = 0.074).
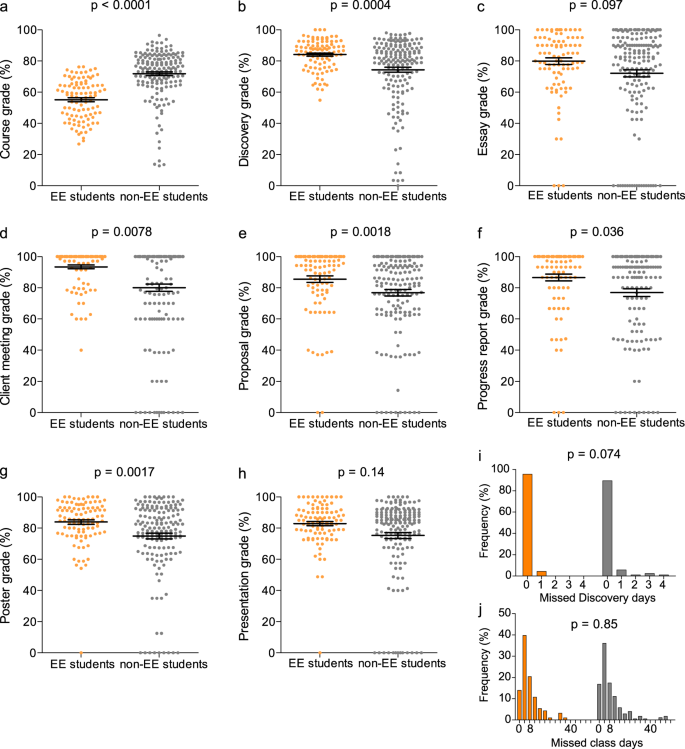
The “Exceeds Expectations” (EE) subset of students (defined as those who received a combined Discovery grade ≥1 SD (18.0%) higher than their final course grade) performed ( a ) lower on their final course grade and ( b ) higher in the Discovery program as a whole when compared to their classmates. d – h EE students received significantly higher grades on each Discovery deliverable than their classmates, except for their ( c ) introductory essays and ( h ) final presentations. The EE subset also tended ( i ) to have a higher relative rate of attendance during Discovery sessions but no difference in ( j ) classroom attendance. N = 99 EE students and 169 non-EE students (268 total). Grade data expressed as mean ± SEM.
Discovery MT students (Fig. 4 ), although not receiving significantly higher grades in class than students participating in the program only one time ( p = 0.29, Fig. 4a ), were observed to obtain higher final Discovery grades than single-term students ( p = 0.0067, Fig. 4b ). Although trends were less pronounced for individual MT student deliverables (Fig. 4c–h ), this student group performed significantly better on the progress report ( p = 0.0021; Fig. 4f ). Trends of higher performance were observed for initial proposals and final presentations ( p = 0.081 and 0.056, respectively; Fig. 4e, h ); all other deliverables were not significantly different between MT and non-MT students (Fig. 4c, d, g ). Attendance in Discovery ( p = 0.22) was also not significantly different between MT and non-MT students, although MT students did miss significantly less class time ( p = 0.010) (Fig. 4i, j ). Longitudinal assessment of individual deliverables for MT students that participated in three or more Discovery terms (Fig. 5 ) further highlights trend in improvement (Fig. 2f ). Greater performance over terms of participation was observed for essay ( p = 0.0295, Fig. 5a ), client meeting ( p = 0.0003, Fig. 5b ), proposal ( p = 0.0004, Fig. 5c ), progress report ( p = 0.16, Fig. 5d ), poster ( p = 0.0005, Fig. 5e ), and presentation ( p = 0.0295, Fig. 5f ) deliverable grades; these trends were all significant with the exception of the progress report ( p = 0.16, Fig. 5d ) owing to strong performance in this deliverable in all terms.
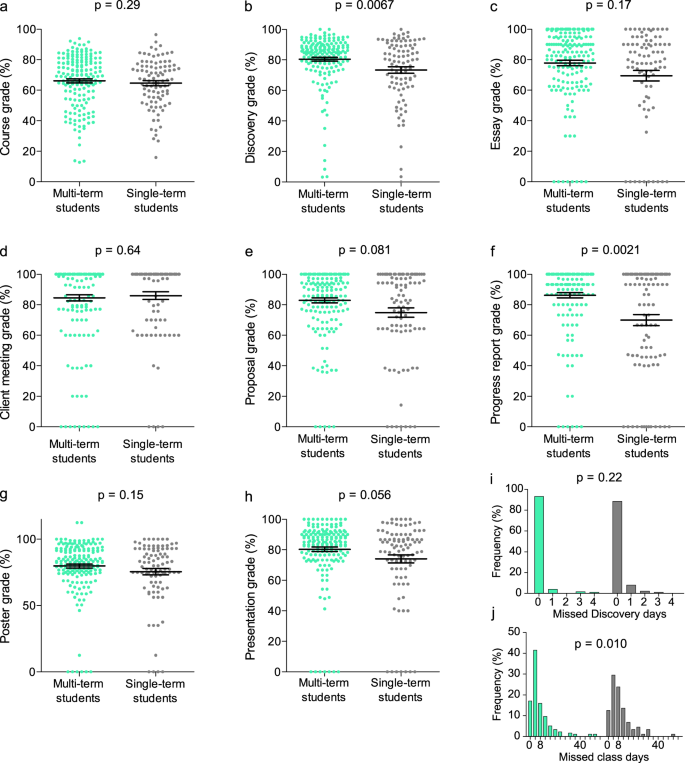
The “multi-term” (MT) subset of students (defined as having attended more than one term of Discovery ) demonstrated favorable performance in Discovery , ( a ) showing no difference in course grade compared to single-term students, but ( b outperforming them in final Discovery grade. Independent of the number of times participating in Discovery , MT students did not score significantly differently on their ( c ) essay, ( d ) client meeting, or ( g ) poster. They tended to outperform their single-term classmates on the ( e ) proposal and ( h ) final presentation and scored significantly higher on their ( f ) progress report. MT students showed no statistical difference in ( i ) Discovery attendance but did show ( j ) higher rates of classroom attendance than single-term students. N = 174 MT instances of student participation (76 individual students) and 94 single-term students. Grade data expressed as mean ± SEM.
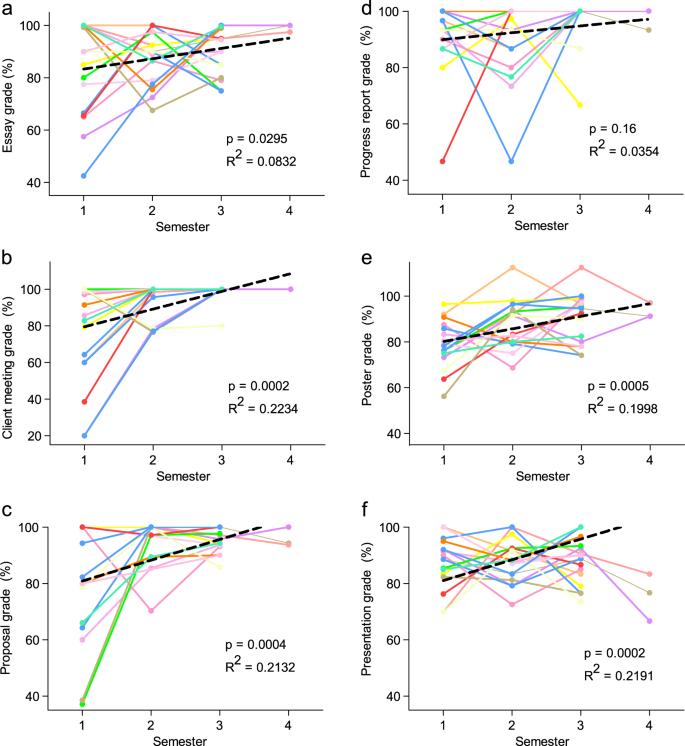
Longitudinal assessment of a subset of MT student participants that participated in three ( N = 16) or four ( N = 3) terms presents a significant trend of improvement in their ( a ) essay, ( b ) client meeting, ( c ) proposal, ( e ) poster, and ( f ) presentation grade. d Progress report grades present a trend in improvement but demonstrate strong performance in all terms, limiting potential for student improvement. Grade data are presented as individual student performance; each student is represented by one color; data is fitted with a linear trendline (black).
Finally, the expansion of Discovery to a second school of lower LOI (i.e., nominally higher aggregate SES) allowed for the assessment of program impact in a new population over 2 terms of programming. A significant ( p = 0.040) divergence in Discovery vs. course grade distribution from the theoretical 1:1 relationship was found in the new cohort (S 1 Appendix , Fig. S 1 ), in keeping with the pattern established in this study.
Teacher perceptions
Qualitative observation in the classroom by high school teachers emphasized the value students independently placed on program participation and deliverables. Throughout the term, students often prioritized Discovery group assignments over other tasks for their STEM courses, regardless of academic weight and/or due date. Comparing within this student population, teachers spoke of difficulties with late and incomplete assignments in the regular curriculum but found very few such instances with respect to Discovery -associated deliverables. Further, teachers speculated on the good behavior and focus of students in Discovery programming in contrast to attentiveness and behavior issues in their school classrooms. Multiple anecdotal examples were shared of renewed perception of student potential; students that exhibited poor academic performance in the classroom often engaged with high performance in this inquiry-focused atmosphere. Students appeared to take a sense of ownership, excitement, and pride in the setting of group projects oriented around scientific inquiry, discovery, and dissemination.
Student perceptions
Students were asked to consider and rank the academic difficulty (scale of 1–5, with 1 = not challenging and 5 = highly challenging) of the work they conducted within the Discovery learning model. Considering individual Discovery terms, at least 91% of students felt the curriculum to be sufficiently challenging with a 3/5 or higher ranking (Term 1: 87.5%, Term 2: 93.4%, Term 3: 85%, Term 4: 93.3%, Term 5: 100%), and a minimum of 58% of students indicating a 4/5 or higher ranking (Term 1: 58.3%, Term 2: 70.5%, Term 3: 67.5%, Term 4: 69.1%, Term 5: 86.4%) (Fig. 6a ).
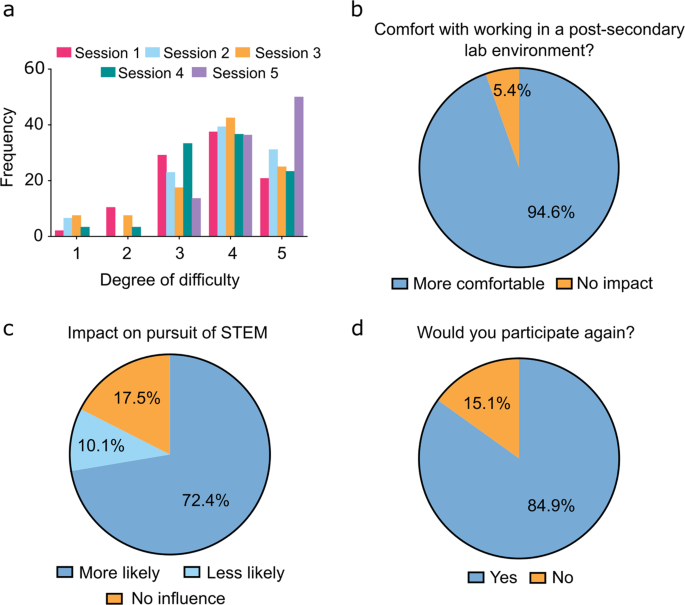
a Histogram of relative frequency of perceived Discovery programming academic difficulty ranked from not challenging (1) to highly challenging (5) for each session demonstrated the consistently perceived high degree of difficulty for Discovery programming (total responses: 223). b Program participation increased student comfort (94.6%) with navigating lab work in a university or college setting (total responses: 220). c Considering participation in Discovery programming, students indicated their increased (72.4%) or decreased (10.1%) likelihood to pursue future experiences in STEM as a measure of program impact (total responses: 217). d Large majority of participating students (84.9%) indicated their interest for future participation in Discovery (total responses: 212). Students were given the opportunity to opt out of individual survey questions, partially completed surveys were included in totals.
The majority of students (94.6%) indicated they felt more comfortable with the idea of performing future work in a university STEM laboratory environment given exposure to university teaching facilities throughout the program (Fig. 6b ). Students were also queried whether they were (i) more likely, (ii) less likely, or (iii) not impacted by their experience in the pursuit of STEM in the future. The majority of participants (>82%) perceived impact on STEM interests, with 72.4% indicating they were more likely to pursue these interests in the future (Fig. 6c ). When surveyed at the end of term, 84.9% of students indicated they would participate in the program again (Fig. 6d ).
We have described an inquiry-based framework for implementing experiential STEM education in a BME setting. Using this model, we engaged 268 instances of student participation (170 individual students who participated 1–4 times) over five terms in project-based learning wherein students worked in peer-based teams under the mentorship of U of T trainees to design and execute the scientific method in answering a relevant research question. Collaboration between high school teachers and Discovery instructors allowed for high school student exposure to cutting-edge BME research topics, participation in facilitated inquiry, and acquisition of knowledge through scientific discovery. All assessments were conducted by high school teachers and constituted a fraction (10–15%) of the overall course grade, instilling academic value for participating students. As such, students exhibited excitement to learn as well as commitment to their studies in the program.
Through our observations and analysis, we suggest there is value in differential learning environments for students that struggle in a knowledge acquisition-focused classroom setting. In general, we observed a high level of academic performance in Discovery programming (Fig. 2a ), which was highlighted exceptionally in EE students who exhibited greater academic performance in Discovery deliverables compared to normal coursework (>18% grade improvement in relevant deliverables). We initially considered whether this was the result of strong students influencing weaker students; however, group organization within each course suggests this is not the case (Fig. 2d ). With the exception of one class in one term (24 participants assigned by their teacher), students were allowed to self-organize into working groups and they chose to work with other students of relatively similar academic performance (as indicated by course grade), a trend observed in other studies 31 , 32 . Remarkably, EE students not only excelled during Discovery when compared to their own performance in class, but this cohort also achieved significantly higher average grades in each of the deliverables throughout the program when compared to the remaining Discovery cohort (Fig. 3 ). This data demonstrates the value of an inquiry-based learning environment compared to knowledge-focused delivery in the classroom in allowing students to excel. We expect that part of this engagement was resultant of student excitement with a novel learning opportunity. It is however a well-supported concept that students who struggle in traditional settings tend to demonstrate improved interest and motivation in STEM when given opportunity to interact in a hands-on fashion, which supports our outcomes 4 , 33 . Furthermore, these outcomes clearly represent variable student learning styles, where some students benefit from a greater exchange of information, knowledge and skills in a cooperative learning environment 34 . The performance of the EE group may not be by itself surprising, as the identification of the subset by definition required high performers in Discovery who did not have exceptionally high course grades; in addition, the final Discovery grade is dependent on the component assignment grades. However, the discrepancies between EE and non-EE groups attendance suggests that students were engaged by Discovery in a way that they were not by regular classroom curriculum.
In addition to quantified engagement in Discovery observed in academic performance, we believe remarkable attendance rates are indicative of the value students place in the differential learning structure. Given the differences in number of Discovery days and implications of missing one day of regular class compared to this immersive program, we acknowledge it is challenging to directly compare attendance data and therefore approximate this comparison with consideration of learning time equivalence. When combined with other subjective data including student focus, requests to work on Discovery during class time, and lack of discipline/behavior issues, the attendance data importantly suggests that students were especially engaged by the Discovery model. Further, we believe the increased commute time to the university campus (students are responsible for independent transit to campus, a much longer endeavour than the normal school commute), early program start time, and students’ lack of familiarity with the location are non-trivial considerations when determining the propensity of students to participate enthusiastically in Discovery . We feel this suggests the students place value on this team-focused learning and find it to be more applicable and meaningful to their interests.
Given post-secondary admission requirements for STEM programs, it would be prudent to think that students participating in multiple STEM classes across terms are the ones with the most inherent interest in post-secondary STEM programs. The MT subset, representing students who participated in Discovery for more than one term, averaged significantly higher final Discovery grades. The increase in the final Discovery grade was observed to result from a general confluence of improved performance over multiple deliverables and a continuous effort to improve in a STEM curriculum. This was reflected in longitudinal tracking of Discovery performance, where we observed a significant trend of improved performance. Interestingly, the high number of MT students who were included in the EE group suggests that students who had a keen interest in science enrolled in more than one course and in general responded well to the inquiry-based teaching method of Discovery , where scientific method was put into action. It stands to reason that students interested in science will continue to take STEM courses and will respond favorably to opportunities to put classroom theory to practical application.
The true value of an inquiry-based program such as Discovery may not be based in inspiring students to perform at a higher standard in STEM within the high school setting, as skills in critical thinking do not necessarily translate to knowledge-based assessment. Notably, students found the programming equally challenging throughout each of the sequential sessions, perhaps somewhat surprising considering the increasing number of repeat attendees in successive sessions (Fig. 6a ). Regardless of sub-discipline, there was an emphasis of perceived value demonstrated through student surveys where we observed indicated interest in STEM and comfort with laboratory work environments, and desire to engage in future iterations given the opportunity. Although non-quantitative, we perceive this as an indicator of significant student engagement, even though some participants did not yield academic success in the program and found it highly challenging given its ambiguity.
Although we observed that students become more certain of their direction in STEM, further longitudinal study is warranted to make claim of this outcome. Additionally, at this point in our assessment we cannot effectively assess the practical outcomes of participation, understanding that the immediate effects observed are subject to a number of factors associated with performance in the high school learning environment. Future studies that track graduates from this program will be prudent, in conjunction with an ever-growing dataset of assessment as well as surveys designed to better elucidate underlying perceptions and attitudes, to continue to understand the expected benefits of this inquiry-focused and partnered approach. Altogether, a multifaceted assessment of our early outcomes suggests significant value of an immersive and iterative interaction with STEM as part of the high school experience. A well-defined divergence from knowledge-based learning, focused on engagement in critical thinking development framed in the cutting-edge of STEM, may be an important step to broadening student perspectives.
In this study, we describe the short-term effects of an inquiry-based STEM educational experience on a cohort of secondary students attending a non-specialized school, and suggest that the framework can be widely applied across virtually all subjects where inquiry-driven and mentored projects can be undertaken. Although we have demonstrated replication in a second cohort of nominally higher SES (S 1 Appendix , Supplementary Fig. 1 ), a larger collection period with more students will be necessary to conclusively determine impact independent of both SES and specific cohort effects. Teachers may also find this framework difficult to implement depending on resources and/or institutional investment and support, particularly if post-secondary collaboration is inaccessible. Offerings to a specific subject (e.g., physics) where experiments yielding empirical data are logistically or financially simpler to perform may be valid routes of adoption as opposed to the current study where all subject cohorts were included.
As we consider Discovery in a bigger picture context, expansion and implementation of this model is translatable. Execution of the scientific method is an important aspect of citizen science, as the concepts of critical thing become ever-more important in a landscape of changing technological landscapes. Giving students critical thinking and problem-solving skills in their primary and secondary education provides value in the context of any career path. Further, we feel that this model is scalable across disciplines, STEM or otherwise, as a means of building the tools of inquiry. We have observed here the value of differential inclusive student engagement and critical thinking through an inquiry-focused model for a subset of students, but further to this an engagement, interest, and excitement across the body of student participants. As we educate the leaders of tomorrow, we suggest that use of an inquiry-focused model such as Discovery could facilitate growth of a data-driven critical thinking framework.
In conclusion, we have presented a model of inquiry-based STEM education for secondary students that emphasizes inclusion, quantitative analysis, and critical thinking. Student grades suggest significant performance benefits, and engagement data suggests positive student attitude despite the perceived challenges of the program. We also note a particular performance benefit to students who repeatedly engage in the program. This framework may carry benefits in a wide variety of settings and disciplines for enhancing student engagement and performance, particularly in non-specialized school environments.
Study design and implementation
Participants in Discovery include all students enrolled in university-stream Grade 11 or 12 biology, chemistry, or physics at the participating school over five consecutive terms (cohort summary shown in Table 1 ). Although student participation in educational content was mandatory, student grades and survey responses (administered by high school teachers) were collected from only those students with parent or guardian consent. Teachers replaced each student name with a unique coded identifier to preserve anonymity but enable individual student tracking over multiple terms. All data collected were analyzed without any exclusions save for missing survey responses; no power analysis was performed prior to data collection.
Ethics statement
This study was approved by the University of Toronto Health Sciences Research Ethics Board (Protocol # 34825) and the Toronto District School Board External Research Review Committee (Protocol # 2017-2018-20). Written informed consent was collected from parents or guardians of participating students prior to the acquisition of student data (both post-hoc academic data and survey administration). Data were anonymized by high school teachers for maintenance of academic confidentiality of individual students prior to release to U of T researchers.
Educational program overview
Students enrolled in university-preparatory STEM classes at the participating school completed a term-long project under the guidance of graduate student instructors and undergraduate student mentors as a mandatory component of their respective course. Project curriculum developed collaboratively between graduate students and participating high school teachers was delivered within U of T Faculty of Applied Science & Engineering (FASE) teaching facilities. Participation allows high school students to garner a better understanding as to how undergraduate learning and career workflows in STEM vary from traditional high school classroom learning, meanwhile reinforcing the benefits of problem solving, perseverance, teamwork, and creative thinking competencies. Given that Discovery was a mandatory component of course curriculum, students participated as class cohorts and addressed questions specific to their course subject knowledge base but related to the defined global health research topic (Fig. 1 ). Assessment of program deliverables was collectively assigned to represent 10–15% of the final course grade for each subject at the discretion of the respective STEM teacher.
The Discovery program framework was developed, prior to initiation of student assessment, in collaboration with one high school selected from the local public school board over a 1.5 year period of time. This partner school consistently scores highly (top decile) in the school board’s Learning Opportunities Index (LOI). The LOI ranks each school based on measures of external challenges affecting its student population therefore schools with the greatest level of external challenge receive a higher ranking 35 . A high LOI ranking is inversely correlated with socioeconomic status (SES); therefore, participating students are identified as having a significant number of external challenges that may affect their academic success. The mandatory nature of program participation was established to reach highly capable students who may be reluctant to engage on their own initiative, as a means of enhancing the inclusivity and impact of the program. The selected school partner is located within a reasonable geographical radius of our campus (i.e., ~40 min transit time from school to campus). This is relevant as participating students are required to independently commute to campus for Discovery hands-on experiences.
Each program term of Discovery corresponds with a five-month high school term. Lead university trainee instructors (3–6 each term) engaged with high school teachers 1–2 months in advance of high school student engagement to discern a relevant overarching global healthcare theme. Each theme was selected with consideration of (a) topics that university faculty identify as cutting-edge biomedical research, (b) expertise that Discovery instructors provide, and (c) capacity to showcase the diversity of BME. Each theme was sub-divided into STEM subject-specific research questions aligning with provincial Ministry of Education curriculum concepts for university-preparatory Biology, Chemistry, and Physics 9 that students worked to address, both on-campus and in-class, during a term-long project. The Discovery framework therefore provides students a problem-based learning experience reflective of an engineering capstone design project, including a motivating scientific problem (i.e., global topic), subject-specific research question, and systematic determination of a professional recommendation addressing the needs of the presented problem.
Discovery instructors were volunteers recruited primarily from graduate and undergraduate BME programs in the FASE. Instructors were organized into subject-specific instructional teams based on laboratory skills, teaching experience, and research expertise. The lead instructors of each subject (the identified 1–2 trainees that built curriculum with high school teachers) were responsible to organize the remaining team members as mentors for specific student groups over the course of the program term (~1:8 mentor to student ratio).
All Discovery instructors were familiarized with program expectations and trained in relevant workspace safety, in addition to engagement at a teaching workshop delivered by the Faculty Advisor (a Teaching Stream faculty member) at the onset of term. This workshop was designed to provide practical information on teaching and was co-developed with high school teachers based on their extensive training and experience in fundamental teaching methods. In addition, group mentors received hands-on training and guidance from lead instructors regarding the specific activities outlined for their respective subject programming (an exemplary term of student programming is available in S 2 Appendix) .
Discovery instructors were responsible for introducing relevant STEM skills and mentoring high school students for the duration of their projects, with support and mentorship from the Faculty Mentor. Each instructor worked exclusively throughout the term with the student groups to which they had been assigned, ensuring consistent mentorship across all disciplinary components of the project. In addition to further supporting university trainees in on-campus mentorship, high school teachers were responsible for academic assessment of all student program deliverables (Fig. 1 ; the standardized grade distribution available in S 3 Appendix ). Importantly, trainees never engaged in deliverable assessment; for continuity of overall course assessment, this remained the responsibility of the relevant teacher for each student cohort.
Throughout each term, students engaged within the university facilities four times. The first three sessions included hands-on lab sessions while the fourth visit included a culminating symposium for students to present their scientific findings (Fig. 1 ). On average, there were 4–5 groups of students per subject (3–4 students per group; ~20 students/class). Discovery instructors worked exclusively with 1–2 groups each term in the capacity of mentor to monitor and guide student progress in all project deliverables.
After introducing the selected global research topic in class, teachers led students in completion of background research essays. Students subsequently engaged in a subject-relevant skill-building protocol during their first visit to university teaching laboratory facilities, allowing opportunity to understand analysis techniques and equipment relevant for their assessment projects. At completion of this session, student groups were presented with a subject-specific research question as well as the relevant laboratory inventory available for use during their projects. Armed with this information, student groups continued to work in their classroom setting to develop group-specific experimental plans. Teachers and Discovery instructors provided written and oral feedback, respectively , allowing students an opportunity to revise their plans in class prior to on-campus experimental execution.
Once at the relevant laboratory environment, student groups executed their protocols in an effort to collect experimental data. Data analysis was performed in the classroom and students learned by trial & error to optimize their protocols before returning to the university lab for a second opportunity of data collection. All methods and data were re-analyzed in class in order for students to create a scientific poster for the purpose of study/experience dissemination. During a final visit to campus, all groups presented their findings at a research symposium, allowing students to verbally defend their process, analyses, interpretations, and design recommendations to a diverse audience including peers, STEM teachers, undergraduate and graduate university students, postdoctoral fellows and U of T faculty.
Data collection
Teachers evaluated their students on the following associated deliverables: (i) global theme background research essay; (ii) experimental plan; (iii) progress report; (iv) final poster content and presentation; and (v) attendance. For research purposes, these grades were examined individually and also as a collective Discovery program grade for each student. For students consenting to participation in the research study, all Discovery grades were anonymized by the classroom teacher before being shared with study authors. Each student was assigned a code by the teacher for direct comparison of deliverable outcomes and survey responses. All instances of “Final course grade” represent the prorated course grade without the Discovery component, to prevent confounding of quantitative analyses.
Survey instruments were used to gain insight into student attitudes and perceptions of STEM and post-secondary study, as well as Discovery program experience and impact (S 4 Appendix ). High school teachers administered surveys in the classroom only to students supported by parental permission. Pre-program surveys were completed at minimum 1 week prior to program initiation each term and exit surveys were completed at maximum 2 weeks post- Discovery term completion. Surveys results were validated using a principal component analysis (S 1 Appendix , Supplementary Fig. 2 ).
Identification and comparison of population subsets
From initial analysis, we identified two student subpopulations of particular interest: students who performed ≥1 SD [18.0%] or greater in the collective Discovery components of the course compared to their final course grade (“EE”), and students who participated in Discovery more than once (“MT”). These groups were compared individually against the rest of the respective Discovery population (“non-EE” and “non-MT”, respectively ). Additionally, MT students who participated in three or four (the maximum observed) terms of Discovery were assessed for longitudinal changes to performance in their course and Discovery grades. Comparisons were made for all Discovery deliverables (introductory essay, client meeting, proposal, progress report, poster, and presentation), final Discovery grade, final course grade, Discovery attendance, and overall attendance.
Statistical analysis
Student course grades were analyzed in all instances without the Discovery contribution (calculated from all deliverable component grades and ranging from 10 to 15% of final course grade depending on class and year) to prevent correlation. Aggregate course grades and Discovery grades were first compared by paired t-test, matching each student’s course grade to their Discovery grade for the term. Student performance in Discovery ( N = 268 instances of student participation, comprising 170 individual students that participated 1–4 times) was initially assessed in a linear regression of Discovery grade vs. final course grade. Trends in course and Discovery performance over time for students participating 3 or 4 terms ( N = 16 and 3 individuals, respectively ) were also assessed by linear regression. For subpopulation analysis (EE and MT, N = 99 instances from 81 individuals and 174 instances from 76 individuals, respectively ), each dataset was tested for normality using the D’Agostino and Pearson omnibus normality test. All subgroup comparisons vs. the remaining population were performed by Mann–Whitney U -test. Data are plotted as individual points with mean ± SEM overlaid (grades), or in histogram bins of 1 and 4 days, respectively , for Discovery and class attendance. Significance was set at α ≤ 0.05.
Reporting summary
Further information on research design is available in the Nature Research Reporting Summary linked to this article.
Data availability
The data that support the findings of this study are available upon reasonable request from the corresponding author DMK. These data are not publicly available due to privacy concerns of personal data according to the ethical research agreements supporting this study.
Holmes, K., Gore, J., Smith, M. & Lloyd, A. An integrated analysis of school students’ aspirations for STEM careers: Which student and school factors are most predictive? Int. J. Sci. Math. Educ. 16 , 655–675 (2018).
Article Google Scholar
Dooley, M., Payne, A., Steffler, M. & Wagner, J. Understanding the STEM path through high school and into university programs. Can. Public Policy 43 , 1–16 (2017).
Gilmore, M. W. Improvement of STEM education: experiential learning is the key. Mod. Chem. Appl. 1, e109. https://doi.org/10.4172/2329-6798.1000e109 (2013).
Roberts, T. et al. Students’ perceptions of STEM learning after participating in a summer informal learning experience. Int. J. STEM Educ. 5 , 35 (2018).
Gillies, R. M. & Boyle, M. Teachers’ reflections on cooperative learning: Issues of implementation. Teach. Teach. Educ. 26 , 933–940 (2010).
Nasir, M., Seta, J. & Meyer, E.G. Introducing high school students to biomedical engineering through summer camps. Paper presented at the ASEE Annual Conference & Exposition, Indianapolis, IN. https://doi.org/10.18260/1-2-20701 (2014).
Sadler, P. M., Sonnert, G., Hazari, Z. & Tai, R. Stability and volatility of STEM career interest in high school: a gender study. Sci. Educ. 96 , 411–427 (2012).
Sarikas, C. The High School Science Classes You Should Take . https://blog.prepscholar.com/the-high-school-science-classes-you-should-take (2020).
Ontario, G. o. The ontario curriculum grades 11 and 12. Science http://www.edu.gov.on.ca/eng/curriculum/secondary/2009science11_12.pdf (2008).
Scott, C. An investigation of science, technology, engineering and mathematics (STEM) focused high schools in the US. J. STEM Educ.: Innov. Res. 13 , 30 (2012).
Google Scholar
Erdogan, N. & Stuessy, C. L. Modeling successful STEM high schools in the United States: an ecology framework. Int. J. Educ. Math., Sci. Technol. 3 , 77–92 (2015).
Pfeiffer, S. I., Overstreet, J. M. & Park, A. The state of science and mathematics education in state-supported residential academies: a nationwide survey. Roeper Rev. 32 , 25–31 (2009).
Anthony, A. B., Greene, H., Post, P. E., Parkhurst, A. & Zhan, X. Preparing university students to lead K-12 engineering outreach programmes: a design experiment. Eur. J. Eng. Educ. 41 , 623–637 (2016).
Brown, J. S., Collins, A. & Duguid, P. Situated cognition and the culture of learning. Educ. researcher 18 , 32–42 (1989).
Reveles, J. M. & Brown, B. A. Contextual shifting: teachers emphasizing students’ academic identity to promote scientific literacy. Sci. Educ. 92 , 1015–1041 (2008).
Adedokun, O. A., Bessenbacher, A. B., Parker, L. C., Kirkham, L. L. & Burgess, W. D. Research skills and STEM undergraduate research students’ aspirations for research careers: mediating effects of research self-efficacy. J. Res. Sci. Teach. 50 , 940–951 (2013).
Boekaerts, M. Self-regulated learning: a new concept embraced by researchers, policy makers, educators, teachers, and students. Learn. Instr. 7 , 161–186 (1997).
Honey, M., Pearson, G. & Schweingruber, H. STEM Integration in K-12 Education: Status, Prospects, and An Agenda for Research . (National Academies Press, Washington, DC, 2014).
Moote, J. K., Williams, J. M. & Sproule, J. When students take control: investigating the impact of the crest inquiry-based learning program on self-regulated processes and related motivations in young science students. J. Cogn. Educ. Psychol. 12 , 178–196 (2013).
Fantz, T. D., Siller, T. J. & Demiranda, M. A. Pre-collegiate factors influencing the self-efficacy of engineering students. J. Eng. Educ. 100 , 604–623 (2011).
Ralston, P. A., Hieb, J. L. & Rivoli, G. Partnerships and experience in building STEM pipelines. J. Professional Issues Eng. Educ. Pract. 139 , 156–162 (2012).
Kelley, T. R. & Knowles, J. G. A conceptual framework for integrated STEM education. Int. J. STEM Educ. 3 , 11 (2016).
Brown, P. L., Concannon, J. P., Marx, D., Donaldson, C. W. & Black, A. An examination of middle school students’ STEM self-efficacy with relation to interest and perceptions of STEM. J. STEM Educ.: Innov. Res. 17 , 27–38 (2016).
Bandura, A., Barbaranelli, C., Caprara, G. V. & Pastorelli, C. Self-efficacy beliefs as shapers of children’s aspirations and career trajectories. Child Dev. 72 , 187–206 (2001).
Article CAS Google Scholar
Davenport Huyer, L. et al. IBBME discovery: biomedical engineering-based iterative learning in a high school STEM curriculum (evaluation). Paper presented at ASEE Annual Conference & Exposition, Salt Lake City, UT. https://doi.org/10.18260/1-2-30591 (2018).
Abu-Faraj, Ziad O., ed. Handbook of research on biomedical engineering education and advanced bioengineering learning: interdisciplinary concepts: interdisciplinary concepts. Vol. 2. IGI Global (2012).
Johri, A. & Olds, B. M. Situated engineering learning: bridging engineering education research and the learning sciences. J. Eng. Educ. 100 , 151–185 (2011).
O’Connell, K. B., Keys, B. & Storksdieck, M. Taking stock of oregon STEM hubs: accomplishments and challenges. Corvallis: Oregon State University https://ir.library.oregonstate.edu/concern/articles/hq37vt23t (2017).
Freeman, K. E., Alston, S. T. & Winborne, D. G. Do learning communities enhance the quality of students’ learning and motivation in STEM? J. Negro Educ. 77 , 227–240 (2008).
Weaver, R. R. & Qi, J. Classroom organization and participation: college students’ perceptions. J. High. Educ. 76 , 570–601 (2005).
Chapman, K. J., Meuter, M., Toy, D. & Wright, L. Can’t we pick our own groups? The influence of group selection method on group dynamics and outcomes. J. Manag. Educ. 30 , 557–569 (2006).
Hassaskhah, J. & Mozaffari, H. The impact of group formation method (student-selected vs. teacher-assigned) on group dynamics and group outcome in EFL creative writing. J. Lang. Teach. Res. 6 , 147–156 (2015).
Ma, V. J. & Ma, X. A comparative analysis of the relationship between learning styles and mathematics performance. Int. J. STEM Educ. 1 , 3 (2014).
Weinstein, C. E. & Hume, L. M. Study Strategies for Lifelong Learning . (American Psychological Association, 1998).
Toronto District School Board. The 2017 Learning Opportunities Index: Questions and Answers. https://www.tdsb.on.ca/Portals/research/docs/reports/LOI2017v2.pdf (2017).
Download references
Acknowledgements
This study has been possible due to the support of many University of Toronto trainee volunteers, including Genevieve Conant, Sherif Ramadan, Daniel Smieja, Rami Saab, Andrew Effat, Serena Mandla, Cindy Bui, Janice Wong, Dawn Bannerman, Allison Clement, Shouka Parvin Nejad, Nicolas Ivanov, Jose Cardenas, Huntley Chang, Romario Regeenes, Dr. Henrik Persson, Ali Mojdeh, Nhien Tran-Nguyen, Ileana Co, and Jonathan Rubianto. We further acknowledge the staff and administration of George Harvey Collegiate Institute and the Institute of Biomedical Engineering (IBME), as well as Benjamin Rocheleau and Madeleine Rocheleau for contributions to data collation. Discovery has grown with continued support of Dean Christopher Yip (Faculty of Applied Science and Engineering, U of T), and the financial support of the IBME and the National Science and Engineering Research Council (NSERC) PromoScience program (PROSC 515876-2017; IBME “Igniting Youth Curiosity in STEM” initiative co-directed by DMK and Dr. Penney Gilbert). LDH and NIC were supported by Vanier Canada graduate scholarships from the Canadian Institutes of Health Research and NSERC, respectively . DMK holds a Dean’s Emerging Innovation in Teaching Professorship in the Faculty of Engineering & Applied Science, U of T.
Author information
These authors contributed equally: Locke Davenport Huyer, Neal I. Callaghan.
Authors and Affiliations
Institute of Biomedical Engineering, University of Toronto, Toronto, ON, Canada
Locke Davenport Huyer, Neal I. Callaghan, Andrey I. Shukalyuk & Dawn M. Kilkenny
Chemical Engineering and Applied Chemistry, University of Toronto, Toronto, ON, Canada
Locke Davenport Huyer
Translational Biology and Engineering Program, Ted Rogers Centre for Heart Research, University of Toronto, Toronto, ON, Canada
Neal I. Callaghan
George Harvey Collegiate Institute, Toronto District School Board, Toronto, ON, Canada
Sara Dicks, Edward Scherer & Margaret Jou
Institute for Studies in Transdisciplinary Engineering Education & Practice, University of Toronto, Toronto, ON, Canada
Dawn M. Kilkenny
You can also search for this author in PubMed Google Scholar
Contributions
LDH, NIC and DMK conceived the program structure, designed the study, and interpreted the data. LDH and NIC ideated programming, coordinated execution, and performed all data analysis. SD, ES, and MJ designed and assessed student deliverables, collected data, and anonymized data for assessment. SD assisted in data interpretation. AIS assisted in programming ideation and design. All authors provided feedback and approved the manuscript that was written by LDH, NIC and DMK.
Corresponding author
Correspondence to Dawn M. Kilkenny .
Ethics declarations
Competing interests.
The authors declare no competing interests.
Additional information
Publisher’s note Springer Nature remains neutral with regard to jurisdictional claims in published maps and institutional affiliations.
Supplementary information
Supplemental material, reporting summary, rights and permissions.
Open Access This article is licensed under a Creative Commons Attribution 4.0 International License, which permits use, sharing, adaptation, distribution and reproduction in any medium or format, as long as you give appropriate credit to the original author(s) and the source, provide a link to the Creative Commons license, and indicate if changes were made. The images or other third party material in this article are included in the article’s Creative Commons license, unless indicated otherwise in a credit line to the material. If material is not included in the article’s Creative Commons license and your intended use is not permitted by statutory regulation or exceeds the permitted use, you will need to obtain permission directly from the copyright holder. To view a copy of this license, visit http://creativecommons.org/licenses/by/4.0/ .
Reprints and permissions
About this article
Cite this article.
Davenport Huyer, L., Callaghan, N.I., Dicks, S. et al. Enhancing senior high school student engagement and academic performance using an inclusive and scalable inquiry-based program. npj Sci. Learn. 5 , 17 (2020). https://doi.org/10.1038/s41539-020-00076-2
Download citation
Received : 05 December 2019
Accepted : 08 October 2020
Published : 02 December 2020
DOI : https://doi.org/10.1038/s41539-020-00076-2
Share this article
Anyone you share the following link with will be able to read this content:
Sorry, a shareable link is not currently available for this article.
Provided by the Springer Nature SharedIt content-sharing initiative
Quick links
- Explore articles by subject
- Guide to authors
- Editorial policies
Sign up for the Nature Briefing newsletter — what matters in science, free to your inbox daily.

The challenge of change: understanding the role of habits in university students’ self-regulated learning
- Open access
- Published: 10 April 2024
Cite this article
You have full access to this open access article
- Louise David ORCID: orcid.org/0000-0003-1973-4568 1 ,
- Felicitas Biwer ORCID: orcid.org/0000-0003-4211-7234 1 ,
- Rik Crutzen ORCID: orcid.org/0000-0002-3731-6610 2 &
- Anique de Bruin ORCID: orcid.org/0000-0001-5178-0287 1
1206 Accesses
Explore all metrics
Study habits drive a large portion of how university students study. Some of these habits are not effective in fostering academic achievement. To support students in breaking old, ineffective habits and forming new, effective study habits, an in-depth understanding of what students’ study habits look like and how they are both formed and broken is needed. Therefore, in this study, we explored these aspects among first-year university students in six focus group discussions ( N = 29). Using a thematic analysis approach, we clustered the data in five themes: Goals Matter , Balancing Perceived Efficiency and Effectiveness when Studying , Navigating Student Life: from Structured Routines to Self-Regulation Challenges , the Quest for Effective Habits with Trying to Break Free From the Screen as subtheme, and the Motivation Roller Coaster . Findings suggest that students had different study habits depending on their goals. Students had quite accurate metacognitive knowledge about effective learning strategies for long-term learning, but often used other learning strategies they deemed most efficient in reaching their goals. Students indicated intentions to change, but did not prioritize change as their current habits enabled them to pass exams and change was not perceived as adding value. Fluctuations in motivation and transitioning to a self-regulated life hampered students’ intentions to form new and break old habits. Next to insights into factors affecting students’ behavioral change intentions, the findings suggest the importance of aligning assessment methods with life-long learning and supporting students in their long-term academic goal setting to prioritize study habits which target lasting learning to optimally foster their self-regulated learning.
Similar content being viewed by others
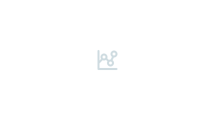
College Students’ Time Management: a Self-Regulated Learning Perspective
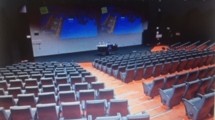
Learning environments’ influence on students’ learning experience in an Australian Faculty of Business and Economics
Exploring the factors that influence the career decision of stem students at a university in south africa.
Avoid common mistakes on your manuscript.
Introduction
In higher education, students are required to plan, monitor, and execute their learning autonomously (Dresel et al., 2015 ; Zimmerman, 1986 ). Therefore, effective self-regulated learning (SRL), facilitated by the use of effective learning strategies, is essential for students’ academic achievement and lifelong learning (e.g., Dunlosky et al., 2013 ). However, many students struggle to use effective learning strategies optimal for long-term learning, such as practice testing, and often rely on passive strategies such as re-reading (Morehead et al., 2016 ; Rea et al., 2022 ). While training programs are successful in increasing students’ knowledge regarding these effective learning strategies, many students struggle to sustainably change their behavior and apply these learning strategies (Biwer et al., 2020a , b ; Foerst et al., 2017 ; Rea et al., 2022 ). This gap between students’ knowledge and behavior is partially due to students’ strong habits of using ineffective study strategies (Blasiman et al., 2017 ; Rea et al., 2022 ). However, so far, this gap between students’ knowledge and behavior and their study habits remained largely unexplored. To explore the gap between students’ knowledge and behavior further, we deem it essential to first gain a thorough understanding of students’ current study habits, how they usually form new, and break old habits.
Using a qualitative approach, we add to the current field of research by targeting an in-depth exploration of students’ study habits, which is currently lacking. Insight into these are essential to tailor educational strategies and training to better align with students' behaviors and needs. Awareness of potential struggles students might encounter when breaking old or forming new habits provides important insights into hurdles students face when attempting to modify their study behavior and how they can be supported in achieving their goals.
Self-regulated learning in higher education
In higher education, learning is happening mostly in teacher-absent environments, which requires students to effectively self-regulate their learning (Dresel et al., 2015 ). However, many students struggle to self-regulate effectively. They monitor and control their learning inaccurately, which has a negative impact on their academic achievement (Hartwig & Dunlosky, 2012 ). One way of fostering self-regulated learning is by helping students to use learning strategies which are optimal for long-term learning (e.g., “desirably difficult” learning strategies, Bjork & Bjork, 2011 ). Examples of desirably difficult learning strategies are practice testing, interleaving items of various categories, and spacing learning sessions over time (Dunlosky et al., 2013 ). A shared commonality of these effective strategies is that they require an active learning process with repeated memory retrieval of information (Bjork & Bjork, 2011 ).
While essential for self-regulated learning, students often avoid desirable difficulties or fail to use them in the long-run, even when being aware of their benefits (Biwer et al., 2020a , b ; Rea et al., 2022 ). One reason for not using effective learning strategies is that students often have strong habits of using surface processing strategies. Instead of using desirable difficulties, students rely on passive learning strategies and mass their study sessions close to the exam instead of spacing the sessions over time (Blasiman et al., 2017 ; Dembo & Seli, 2004 ; Foerst et al., 2017 ). Often students start using these strategies in high school already (Dirkx et al., 2019 ).
Next to struggling to use effective learning strategies, students often face additional regulation issues during their self-study. Learners also need to employ resource management strategies such as effort and motivation management to optimize learning conditions (Dresel et al., 2015 ) by, for example, planning their study sessions or asking for help if necessary. While resource management strategies have been identified as an essential factor for academic performance (Grunschel et al., 2016 ; Waldeyer et al., 2020 ), many students struggle with time management (Basila, 2014 ; Thibodeaux et al., 2017 ) and encounter motivational problems leading to for example procrastination (Grunschel et al., 2016 ).
Metacognition and resource-management strategies are often indicated as important factors in self-regulated learning models (for an overview, see Panadero, 2017 ). However, these factors might not fully explain how students maintain recurring study behaviors over the long term (Fiorella, 2020 ). Instead, habits, which, once formed, are usually not guided by conscious intentions and goals, have been suggested crucial for academic behaviors that need to be repeated consistently over an extended period (Fiorella, 2020 ). Therefore, to foster students’ self-regulated learning, it has been suggested to support students in building new study habits, which, for example, incorporate desirable difficult learning strategies (Fiorella, 2020 ).
Behavioral change and study habits
In the last years, interventions have been developed to enhance students’ knowledge and initial use of effective learning strategies (e.g., Ariel & Karpicke, 2018 ; Biwer et al., 2020b ; Broeren et al., 2021 ). While showing positive effects, the actual transition of this knowledge and application to students’ self-study in the long run remain limited (Dignath & Veenman, 2021 ). The majority of these interventions focus more on increasing students’ knowledge and less on building new habits or changing the actual study behavior consistently potentially explaining the limited application. Habits, however, are an important predictor of behavior in addition to, for example, attitudinal and control beliefs (Verhoeven et al., 2012 ; Verplanken, 2018 ), and are seen as a key factor in behavior maintenance (Rothman et al., 2009 ). To ultimately support students in actually applying effective learning strategies in the long run, it is essential to first understand the factor that drives most behavior: habits (Rothman et al., 2009 ; Verplanken, 2018 ).
Habits are behaviors that reoccur in stable contexts. Once a habit forms, behavior is led by automatic, effortless actions instead of deliberate intentions (Gardner, 2015 ; Lally, et al., 2010 ). According to our conceptualization, study habits can relate to behaviors such as the usual length of a student’s study session, learning strategies used, or study timing and environment. Study habits can play an essential role in harming or helping students to achieve long-term academic goals. Irrespective of whether these habits help or hinder goal achievement, they initiate behavior automatically and effortlessly. Habits usually form when an association between a certain behavior and context is established by consistently repeating a behavior in that context (Gardner, 2015 ; Lally & Gardner, 2013 ). Due to the association, the habitual behavior is automatically and sometimes unconsciously activated (Aarts & Dijksterhuis, 2000 ) while alternative behaviors become less accessible (Danner et al., 2007 ). Often, the effort required to initiate the habitual behavior decreases over time and initiation becomes effortless (Lally et al., 2011 ). Even when intentions change, engagement in habitual behavior commonly persists (Adriaanse & Verhoeven, 2018 ).
According to the goal–habit interface model (Wood & Rünger, 2016 ), many habits are initiated by goal-directed behavior. Initially, before habit formation, goals drive individuals to repeat a certain action in a specific context. Once a habit is formed, the habitual behavior shifts from goal-dependent to goal-independent behavior. In this case, behaviors are enacted based on context cues and irrespective of goals (Mazar & Wood, 2018 ). Nevertheless, as many individuals are unaware of this habit formation process, they might misattribute their habitual behavior to goals or intentions instead of habits (Loersch & Payne, 2011 ; Mazar & Wood, 2018 ).Wood & Neal ( 2016 ) suggest various essential components for habit-based interventions that target initiation and maintenance of health behavior change. They suggest the importance of habit-forming approaches for fostering repeated engagement in healthy behaviors while also disrupting undesirable behavior by habit-breaking approaches. As main components for habit formation, they suggest frequent repetition of the desirable behavior, creating a re-occurring context and context-cues, and administering rewards randomly (Wood & Neal, 2016 ). To break undesirable habits, context-cue disruption, re-structuring of one’s environment, and monitoring one’s behavior are suggested as efficient (Wood & Neal, 2016 ).
While there has been quite some research showing the beneficial effects of habits in health behavior change (e.g., exercising habit, healthy eating habit), only a few studies have focused on how study habits could improve students’ study behavior (e.g., Galla & Duckworth, 2015 ). Students’ ineffective study habits such as ineffective learning strategy use (Biwer et al., 2020a , b ; Foerst et al., 2017 ; Rea et al., 2022 ), high smartphone use (Chen & Yan, 2016 ; Lepp et al., 2015 ), or poor time management (Basila, 2014 ; Thibodeaux et al., 2017 ) seem to play a role in their study behavior and academic achievement. Breaking these ineffective habits and forming effective study habits instead by, for example, incorporating more desirably difficult learning strategies could help students to optimize their self-regulated learning and academic achievement.
In the present study, we aim to gain a better understanding of the nature of university students’ study habits, how students usually form new habits, and how they (try) to break ineffective habits. We explored this using a qualitative approach via focus group discussions with first- and second-year university students. As our goal was to dive beyond surface-level observations and gain an in-depth understanding of students’ experiences rather than generalizing to a larger population, a qualitative approach seemed most appropriate (Morse, 2008 ). Compared to a quantitative approach, a qualitative approach via focus groups or interviews offers various benefits (for a more general overview of focus group discussions in medical education, see Stalmeijer et al., 2014 ). First, they offer a relatively time- and cost-effective access to students’ thoughts and experiences. Second, by being able to directly engage with students, it is possible to ask students for further elaboration or clarification, which would not be possible using for example questionnaires and therefore ensuring an in-depth understanding. Third, due to the flexibility of this approach and our semi-structured question guide, we were able to capture students’ opinions while limiting imposing pre-existing assumptions about their study habits. Most importantly though, we anticipated that the interactions between students would be essential when discussing study habits and focus group discussions would thus allow a more diverse and multifaceted picture of students’ study habits. While habitual behaviors could be unconscious, we expected that hearing other students’ experiences would facilitate students’ reflections on similar or dissimilar experiences. Insights from our study can help to inform potential future study habit interventions to overcome students’ knowledge-behavior gap.
Research setting
This study was conducted at the Faculty of Health, Medicine, and Life Sciences (FHML) at Maastricht University, which is a public research university in the Netherlands. At Maastricht University (UM), the academic year is divided into six different blocks (i.e., teaching periods), including different thematic courses lasting between 4 and 8 weeks. Courses usually consist of lectures and tutorial sessions and are finalized with an exam. The tutorial sessions are structured according to the problem-based learning (PBL) approach. Within this approach, authentic scenarios are central to learning (Dolmans et al., 2005 ). In small tutorial groups, ranging from 10 to 12 students and one tutor, students are introduced to these scenarios according to a seven-step model (Moust et al., 2005 ). The first five steps, (1) term clarification, (2) problem definition, (3) brainstorming explanations, (4) structuring and analyzing the identified explanations, and (5) formulation of learning goals, take place during the tutorial session. Students then individually study literature to answer their learning goals during (6) self-study. Lastly, during a next tutorial session, students integrate and discuss findings from their self-study in a (7) post-discussion.
Admission to the medical program at UM is a selective procedure, with only limited places available. Students are only eligible if having followed a certain combination of STEM courses during high school. Furthermore, potential candidates have to undergo two selection rounds. In the first selection round, students’ high school transcripts, curriculum vitae, and a written assignment are evaluated. If of sufficient quality, candidates are invited to a second selection round, which is an assessment day at the university, where potential candidates have to fulfill various assignments during which their cognitive and (inter)personal characteristics and skills are assessed. Based on these criteria, students are ranked and selected. Admission to the biomedical program at UM was not selective for the cohort participating in this study. The only eligibility criterion was that students had followed a certain combination of STEM courses during high school and were proficient enough in English.
The majority of first-year FHML students at UM are assigned to a mentor and receive a formal learning strategy training in their first weeks (“Study Smart”; Biwer & De Bruin, 2023 ) to support their self-regulated learning and professional development. The learning strategy training consists of three 90-min small-group training sessions with different goals. In the first session, the main goal is to increase students’ awareness about effective and ineffective learning strategies, why certain strategies work better, and possibly misleading experiences that students might encounter when using (in)effective learning strategies. During the second session, students are asked to try-out different effective learning strategies and think about a concrete plan, how they could incorporate these during their self-regulated learning. During the third session, which is held a few weeks after the previous sessions, students are asked to reflect on their learning strategy use during their self-regulated learning and how they could improve. Throughout the year, students meet with their mentor and write a portfolio reflecting on their competencies related to program content, professional- and study behavior.
Participants
Twenty-nine first- and second-year medicine ( n = 14) and biomedical sciences ( n = 15) bachelor students participated in one of six focus group discussions. Students were on average 20.1 years old (14% male). Students were recruited via multiple channels. The first author contacted the mentor and course coordinators of the respective bachelor programs to share the study information with their students. Additionally, students were recruited via posters and approached after tutorials and lectures. During the recruitment phase, the study goal was advertised as exploration of students’ study methods and habits. We obtained written informed consent from all participants prior to the study. Participants completed a demographic questionnaire inquiring about their age, study program and year, and grade point average (GPA). Students’ GPA ranged from 5.8 to 9, with the mean GPA being 7.4 (on a scale from 1 to 10, with 10 being the highest). Two students did not indicate their GPA. The faculty’s ethical review board (FHML-REC/2022/049) approved the study. Students received a small monetary reimbursement to compensate for their participation.
Focus groups
Students who completed the demographic questionnaire and indicated their availability were invited to the focus group discussions at university. The focus groups lasted approximately 80 min ( M = 78.4 min, SD = 4.2 min) and were moderated and observed by the first and second author. Based on a semi-structured focus group guide (see supplementary materials), students were asked which study methods they usually used during their self-study, what a typical study day looked like, and about their experiences with forming and breaking habits. The clarity of the questions within the focus group guide was piloted with two students prior to the focus group discussions. Based on their feedback, the wording of the questions was slightly adjusted to improve clarity. The first four focus groups were held at the end of students’ first academic year. Two additional focus groups were held at the beginning of students’ second bachelor year. The focus group guide was adjusted before the second part of data collection as we noticed that students had difficulties reflecting in-depth on their experiences with forming and breaking habits. Therefore, we created three vignettes describing concrete examples of how a habit was broken or formed based on students’ reflections during the first focus groups. Data was collected iteratively and occurred simultaneously with data analysis. In line with Morse ( 2015 ), as soon as we estimated our data to be sufficiently rich to gain a deep understanding of students’ study experiences and their habit-breaking and habit-forming experiences, we determined data saturation and stopped data collection. Data saturation is commonly an interpretive judgment in thematic analysis (for a critical stance regarding the concept “data saturation,” see Braun & Clarke, 2019 ). In our case, we started data analysis by descriptive line-by-line coding of the so far collected transcripts, clustering these codes, and searching for patterns, simultaneously to data collection. Based on the understanding we gained during these first analytic steps, we determined to have collected sufficient data to understand students’ experiences after six focus groups.
Data analysis and coding procedure
All focus groups were audio recorded and transcribed verbatim. Based on the thematic analysis approach (Braun & Clarke, 2006 ), we analyzed the focus group discussions inductively. Our analysis was data-driven and thus, the theme development did not mirror our question guide nor do the themes fully align with our potential pre-existing assumptions about students’ study habits (Braun & Clarke, 2006 ; Kiger & Varpio, 2020 ). We used pre-existing theories to establish our focus group guide and kept this knowledge as sensitizing concepts during the coding procedure (Bowen, 2006 ). We conducted a semantic analysis of participants’ accounts by interpreting their explicit meanings as recorded and transcribed. After thoroughly listening to the audio recordings and reading the transcripts in-depth multiple times, they were descriptively coded line-by-line using Atlas.ti (version 23.0.7.) by the first author (an extract of our codebook is included in the supplementary materials). Using these descriptive codes, the second author coded 50% of two transcripts without insights into the first coder’s line-by-line coding. The outcomes and coding alignments of these partial transcripts were discussed and definitions of codes were further specified, if necessary. Then, patterns within and across focus groups were discussed within the whole research team based on summaries of the transcripts and clustering of the descriptive codes done by the first and second author. Based on this discussion, the first and second author formulated themes, which were reviewed and refined with the entire research team. Additionally, the formulated themes were checked against the coded data and original transcripts. Throughout the analytic procedure, our research team engaged in regular discussions and asked for feedback from scholars outside of the research team during roundtable sessions and conference presentations to critically examine interpretations and findings. We followed a pragmatist approach in analyzing the data, prioritizing the practical relevance of knowledge instead of attempting to explain “reality” (Cherryholmes, 1992 ). The pragmatist approach offered us the flexibility and adaptability of addressing our research questions as well as generating practical implications from the identified themes while interpreting the data within our specific context.
Reflexivity statement
LD is a PhD student who completed a Bachelor’s degree at Maastricht University and is therefore familiar with the UM system also from a student perspective. FB is an educational psychologist with research interests in self-regulated learning and teacher professionalization and knows the PBL system in their role as mentor and tutor. RC is a cognitive psychologist with research expertise in behavior change and technology. AdB is an educational psychologist with research expertise in self-regulated learning in higher education. RC and AdB know the PBL system in various roles (student, teacher, mentor). We are aware that the findings of this study are co-created by interactions between the authors and participants. We thoroughly discussed each process step with all team members and discussed intermediate findings with educational scientists outside of the team and continuously reflected on the extent to which our individual personal experiences and beliefs shaped the research project.
We clustered students’ experiences into five themes: Goals Matter , Balancing Perceived Efficiency and Effectiveness when Studying , Navigating Student Life: from Structured Routines to Self-Regulation Challenges , the Quest for Effective Habits with Trying to Break Free From the Screen as subtheme, and the Motivation Roller Coaster .
Goals matter
Students mentioned various goals, which influenced their study behavior. Many students had a clear short-term goal of passing the exam in mind. For them, studying during the course served the goal of “collecting all the information” (student studying medicine (MED), focus group (FG) 2) to prepare for tutorial sessions. Studying for the exam served the goal of “actual studying” (student studying biomedical sciences (BMS), FG2), during which information is memorized or learned. Students experienced more pressure when studying for an exam compared to studying during the course. Approximately 1.5 weeks before an exam, students started intense studying. During this period, they dedicated extensive time to studying, often sacrificing a work-life balance and reducing time for activities like exercising, socializing, and hobbies.
Other students indicated having clear long-term academic goals such as receiving a high GPA or wanting to become a good doctor. These clear long-term goals helped them to study consistently, be less exam-oriented, and prioritize their studying also during the course, even if having to sacrifice other activities. Instead of studying solely to pass exams, students explained to be more consistent in their study behavior and tried to understand and learn the content continuously. They mentioned achieving this by planning and spreading their study load throughout the course. For example, one student mentioned:
I don’t study for exams anymore, I study because I want to be a good doctor. And I think that mindset also helped me change. […]. So it’s harder to find time around [studying], but I also prioritise [studying], university is the most important thing for me. If it means I don’t go to a party or I don’t go to the gym, which I also want to do, then that’s just how it is. (Student MED, FG5)
Balancing perceived efficiency and effectiveness when studying
Students generally had quite accurate metacognitive knowledge and were aware that active learning strategies and distributed learning sessions were more effective for long-term learning. Contrastingly, students did not necessarily use those strategies as they experienced that their own study strategies “work” (multiple students in multiple FGs) and were most efficient in reaching their short-term goal to pass the exam or having a strong habit of using them. For example, one student mentioned:
Well, I know it’s not really useful. But I still [summarize] a lot. But I know that it’s […] been proved that it’s not really effective. But I still, I don’t know, it’s just a habit. […] And it works. (Student MED, FG 3)
Students differed in which strategies they deemed to “work” (multiple students in multiple FGs) for themselves. For tutorial preparations, students usually read and summarized the provided sources. For exam preparations, students used various active and passive learning strategies such as re-reading, summarizing, creating mind maps, watching videos, recalling information, testing themselves by using past exams or creating flashcards, and explaining content to others or themselves. The amount of practice testing students used during their self-study depended on the availability of past exam questions in their study program as students experience creating practice questions themselves as too time consuming:
Some of our professors, they made […] really extensive kind of quizzes with lots of questions. And that was really helpful. I wish they did that for everything. (Student BMS, FG6)
To optimize their study efficiency, next to using cognitive learning strategies, students tried various resource management strategies, such as creating a planning for their tasks or studying with friends to stay motivated but often faced challenges in accurately managing their time and effort. Students usually started their study session by creating a planning or to-do list, but it was difficult to accurately know how long a task would take or to “really stick to it” (Student BMS, FG3). Instead of studying until a certain time, students usually studied until the task was finished or they could not focus anymore.
Navigating student life: from structured routines to self-regulation challenges
Students reported basing their study habits on what they had learned during high school, despite university being experienced as different. Next to the content being perceived as more in volume and difficulty, students also mentioned having to adjust to the change of setting. During high school, students often had a structured daily routine. When transitioning to university, they had to adjust to the lack of structure and external control, but also higher flexibility associated with student life. While students appreciated this increased freedom, it also came with the challenge of managing themselves and self-regulating various aspects of life: starting to study, moving to a new city, looking for new hobbies and friends, or managing their household.
When I lived with my parents in high school […], you have like a whole routine. […]. Now you can do things whenever you want to do it. And […] sometimes you have parties on Monday or on Wednesdays. So, it’s on random days. Yeah. And then you’re tired on Tuesdays. So, then you don’t do as much as other days. […] I think, it’s also part of student life. (Student MED, FG2)
Some students solved the lack of structure by actively creating more external structure in their daily life by joining committees, making appointments with others, or creating a clear separation between work and “personal” life. For other students, the lack of external structure seemed to negatively influence their willingness to form habits as they experienced habits as restricting flexibility. Some students appreciated the flexibility that they associated with student life and did not report actively looking for more structure. In search for more structure, students differed in their habit and preference of study location. Some students preferred to study at home to avoid distractions, whereas others preferred to study at university as they found it easier to initiate studying with others around and appreciated a separation between their studying and living space. During courses, students were more flexible in their study setting.
The quest for effective habits
Even though students experienced their current strategies to “work” for the exam, they reported intentions to change their current study behavior. These intentions targeted improving time management, such as following their planning, procrastinating less, cramming less, limiting screen time, and changing their sleeping schedule. They mentioned making study appointments with others, which helped to feel more accountable, and promoted adherence to intended behavior. Students also mentioned wanting to add steps to their current study habits such as completing their study notes or creating flashcards after each tutorial. For example, a student mentioned “I first had to […] talk about this topic, answer the question, then I was allowed, for example, to go to the bathroom” (student BMS, FG4) or working through flashcards when traveling. While students had intentions to change, they mentioned not necessarily prioritizing working on realizing their intentions since their current habits were sufficient to pass their exams but also encountering difficulties when trying to realize them. Students identified various reasons for this, for example, difficulties in creating a feasible planning, following their intention when not being motivated, or falling back into old habits when experiencing a setback. While having good intentions, students perceived change to be effortful, time-consuming, or being insecure about whether a change would actually lead to an improved outcome. For example, one student mentioned:
It’s not enough to have motivation to change that one point. Because it’s what you say, how do you make the change? By doing it daily. […]. That’s, you know, what I’m trying to do. Like be more consistent. […]. I’ll do great for the first couple of days, maybe two weeks, if I was really motivated. But then after I wake up on a bad day, I really don’t feel like it. And it’s this boring topic. I’ll push it back. […]. I’ll do something else. Then time runs out. Then we’re already kind of back in the bad cycle of procrastinating on everything. […] If there’s not the stress of a deadline, then it comes down purely to motivation every day. (Student BMS, FG3)
Trying to break free from the screen
One particularly challenging habit students tried to break was their smartphone habit. Students mentioned turning to their phones or study unrelated websites automatically when lacking focus or procrastinating. This was experienced as distracting and negatively influencing students’ studying. They tended to spend more time on their phone than anticipated, for example, if wanting to briefly check something. To reduce this automatic use, students tried to discontinue their habit by re-structuring their environment so that the distraction would be less accessible or interchanging their phone use with another activity such as reading. Instead of having their phone on the table when studying, they put their phone out of sight, blocked or deleted apps in the morning, or exchanged their phone with a friend’s phone. Even though students used various strategies to reduce the distraction from these devices, students continuously struggled in limiting their screen time.
I am so done with my phone being the hugest distraction in my life at this point, it just annoys the hell out of me. I’m actually breaking a habit right now, it’s called ‘no Instagram anymore’. Yes, it’s very bad, but I am... I’m not going to say I am improving because I am not, but I am trying to improve. (Student BMS, FG6)
The motivation roller coaster
Students described experiencing a fluctuation in motivation throughout a course and academic year, which influenced their study behavior and behavioral change intentions. With their past stressful exam preparation in mind, students reported beginning a new course with many resolutions wanting to improve what went “wrong,” such as aiming to study more consistently or to start exam preparations earlier. Students often managed to realize their resolutions for the first few weeks of a new course. However, after approximately 2 weeks, students lost motivation and started to slack with their studying (e.g., studying less consistently). They experienced less pressure to study, and thus experienced more motivational conflict when initiating studying due to tempting alternative activities, other course obligations, or interferences outside of university. For example, one student, being well aware of the consequences of procrastination, mentioned:
I can relax now and do this fun thing. And then maybe have to do like, a quick version of this assignment late at night. (Student BMS, FG3)
Three weeks prior to the exam, students felt increased pressure when realizing how close the exam and how much work was left. This pressure motivated students to catch up on the coursework and spend more time studying. Often, students focused on getting the studying done (e.g., creating summaries for each case) and cramming the content using methods that previously worked to pass the exam. For example, one student mentioned:
I’m also motivated in the beginning of a new block. […] I have this motivation over me. Like, well, now I’m going to keep up with it. And then sometimes, […] I lose track of the course that we’re in. And I’m behind again. And then I lose it a bit. So, when I get behind, and I’m like, okay, now I just do the minimal. And then two, a week and a half, before the exam, then I get my motivation back. (Student MED, FG2)
This paper outlines the findings of a study exploring university students’ study habits during self-regulated learning. We held focus groups aiming to explore university students’ current study habits, habit formation, and experiences with breaking habits. A thematic analysis of the transcribed data revealed five themes and one subtheme, which offer insights into our research aims regarding how students usually form new habits, and how they (try) to break ineffective habits. Our study offers a detailed exploration of university students’ study habits. Using a qualitative approach, we were able to dive beyond surface-level observations by exploring students’ experiences and factors, which influence these habits, their formation, and the challenges associated with breaking habits in-depth. While our chosen approach allows for a rich and nuanced understanding of the multifaceted nature of students’ study habits, the goal of a qualitative approach is commonly not to generalize findings to a larger population (Morse, 2008 ). The findings of this study are experiences from a specific student population rooted within a specific educational and cultural context. Including a thick description of this context, we try to establish transferability of our findings by indicating how they could apply to other contexts and student populations.
Students’ study habits
Students had different study habits depending on their goals. Students with clear short-term goals of passing the exam focused on collecting information during the course and started intense studying shortly before the exam. Students with long-term academic or career goals planned their study sessions continuously and focused more on learning the study content. Students differed in their preferred study setting when preparing for exams, but usually had a habit and preference of where and how to study. During courses, students were more flexible in their study setting. These findings suggest that long-term academic goal setting helps students to prioritize long-term learning by spreading out study sessions and focusing on learning the study content continuously. Long-term academic goal setting might be more evident for some study programs than for others. In study programs such as medicine, many first-year students have a clear goal of becoming a good doctor with long-term retention of knowledge and skills as important factors to reach that goal. Study programs with more diverse career paths might make it difficult for students to envision clear long-term goals. This lack of long-term goal setting might hamper students’ motivation to ensure more effortful studying in a manner that ensures long-term retention or best possible grades. Previous research indicates that students’ long-term perspectives are related to better self-regulation strategies (Bembenutty & Karabenick, 2004 ; De Bilde et al., 2011 ).
Students tried to be strategic in their learning strategy use by balancing perceived efficiency and effectiveness of the methods in reaching their goals. For tutorial preparations, students usually read and summarized the provided sources. For exam preparations, students combined active and passive learning strategies such as summarizing, watching videos, or explaining content to themselves. Unlike often assumed in the literature (e.g., Morehead et al., 2016 ; Yan et al., 2016 ), students in the current sample had quite accurate metacognitive knowledge. This could be related to the fact that they followed a formal learning strategy training at the beginning of their studies or the PBL system employed at UM, which aims to foster an active learning approach (Dolmans et al., 2005 ). Students might have been aware of effective and ineffective learning strategies and received suggestions on how to incorporate as many active learning approaches as possible within their self-regulated learning. Nevertheless, they used learning strategies, which are not the most effective for long-term learning but ones that they deemed most time-efficient to pass exams. While students were often aware of this discrepancy, assessment forms were an important driver for learning strategy choices. This is in line with prior research indicating that students adapt their learning strategies to examination requirements (Broekkamp & Van Hout-Wolters, 2007 ; Rovers et al., 2018 ). Bachelor students are often assessed using multiple choice questions, which focus on recognizing information instead of retention. They thus use learning strategies to maximize success for that assessment type. In order to nudge students to use learning strategies essential for long-term learning, it is crucial to align assessment by using methods that stimulate long-term learning and prioritize testing understanding instead of recognition (Van der Vleuten et al., 2010 ).
Furthermore, students’ willingness to incorporate active learning strategies such as retrieval practice seemed dependent on the available resources in their study program. In the medicine program, students had access to past exam questions and thus used them during exam preparations. Students in the biomedical sciences program, having no access to previous exam questions, found it difficult and time-consuming to create practice questions themselves and appreciated course coordinators who provided example questions. This suggests that more support from educators, such as providing practice questions or training sessions on formulating practice questions, might further increase students’ use of active learning strategies.
Forming and breaking habits
In line with previous research, students reported basing their study methods on what they had learned during high school (Dirkx et al., 2019 ). They updated these methods if they did not help them to achieve their goals by trying to embed additional steps in pre-existing habits (e.g., if going to bed, then reading notes). This method of specific if–then plans also called implementation intentions (Adriaanse & Verhoeven, 2018 ; Gollwitzer, 1993 ) is commonly suggested to foster habit formation and habit breaking. By pre-selecting a situation and mentally rehearsing the if–then link, individuals are more likely to automatically trigger the desired behavior when encountering the situation. Formulating these if–then plans could create new associations that compete with existing habits. If the new cue-action link is stronger than the habitual pattern, the desired action could potentially override the habitual response. While research across various domains supports the effectiveness of implementation intentions in promoting goal-directed actions (Adriaanse et al., 2011 ; Gollwitzer & Sheeran, 2006 ), it is unclear whether implementation intentions influence learners’ learning outcomes or metacognition (Hoch et al., 2020 ).
Students reported intentions to change their study behavior. While their intentions targeted mainly improving time management aspects or wanting to add steps to their current study habits, disentangling the extent to which students wanted to realize these intentions was difficult. Additionally, detailed reflections of how students broke past ineffective habits and whether they actually changed their behavior once their habits did not “work” anymore remained scarce, making it difficult to fully target our third research aim. Students did not see change as a priority because their current method “worked” to pass exams. As mentioned above, assessment was an important driver for students’ learning strategy choices but also a key factor in their behavioral change intentions. Students were successful in passing exams and thus did not perceive change as necessity or added benefit. However, they also indicated not knowing how to change, or experiencing change as effortful and time-consuming. The difference between not changing because perceiving change as having no additional value or because not knowing how to has important implications for behavioral change interventions. Students who do not perceive change as beneficial might profit from interventions that highlight the utility value of effective learning strategies (e.g., McDaniel & Einstein, 2020 ) in achieving a sufficient grade to pass a course as efficiently as possible. Students who do not know how to change might profit from interventions that provide them with information on how behavioral change could be achieved. More research is necessary to disentangle the extent to which students are willing to form new and break old habits and if so, how they could be best supported in this.
Another factor influencing students’ intention to change and breaking old habits was their fluctuating motivation throughout the course. Students began a new course with many resolutions wanting to improve what went “wrong” the block before but motivational conflict and their usual context quickly disabled them from realizing their intentions and instead continuing with unwanted behavior. In line with Verplanken and colleagues ( 2018 ), this finding suggests a potential importance of temporal considerations in behavioral change interventions in transition periods. Interventions held at the beginning of a new course might benefit from students’ increased motivation to change.
Additionally, students’ capacity to form new and break old habits seems to be influenced by their transition to a more independent environment. During this transition, they did not solely focus on establishing effective study habits but also had to navigate their flexible schedule during which they encountered other self-regulation challenges. According to the habit discontinuity hypothesis (Verplanken & Wood, 2006 ), major life events or disruptions can serve as catalysts for behavior change, indicating that the transition to university could potentially serve as a great opportunity to break existing habits and form more adaptive ones. As the transitions create a habit discontinuity, people change their habits and adopt new behaviors as old cues and routines that support existing habits are weakened or disrupted (Verplanken et al, 2018 ). As a result, individuals are more receptive to new opportunities to establish new habits or modify existing ones. This suggests that it might be valuable to support students in building effective habits as soon as they start university to make use of the discontinuity of existing habits, or, as mentioned above, at the beginning of a new course later in the semester. Contrastingly, our findings suggest that the new context and flexibility also challenge the formation of new habits, since it is difficult to “piggy-bag” new habits onto existing ones and because the needs for what makes learning effective to pass exams in the new context are not clear yet. Students solved this by actively looking for more structure. More research is necessary to investigate how the transition to university influences students’ capacity to form new habits.
Another factor, which could be important in students’ study habits and breaking and formation hereof, is co-regulation. The latter describes how learners’ cognition, emotions, and motivation during learning are influenced by interaction with others (Bransen et al., 2022 ; Hadwin & Oshige, 2011 ). Students mentioned making study appointments with others, which helped them to feel more accountable for their actions and thus promoted adherence to their intended behavior, suggesting that co-regulation could be an important factor in supporting students to break ineffective habits and form new habits. Furthermore, by observing how peers study, students could learn to model adaptive behavior, enabling them to form habits that incorporate more effective learning strategies. Furthermore, being able to exchange experiences with peers during the habit formation and breaking process, which might be associated with negative emotions such as stress and frustration, could help students to cope and stay motivated during their behavior change journey. However, more research is necessary to investigate how co-regulation could influence students’ study habits.
Strengths and limitations
Our findings highlight the central role of students’ goals in shaping their study habits. Knowing how goals influence study habits offers valuable insights into the importance of tailoring educational strategies to better align with students’ goals and to shed light onto goal-driven behavior in education. Furthermore, the discrepancy between students’ knowledge and behavior when it comes to the limited application of effective learning strategies seems to stem from the low perceived efficiency of these strategies, highlighting a need to increase students’ perceived efficiency of effective learning strategies to close the gap between knowledge and behavior. Furthermore, the identified challenges related to motivation fluctuations within and throughout a course and the transitioning from high school to a self-regulated learning environment in university present original perspectives, which so far remain underexamined in the context of self-regulated learning. Our study offers valuable insights into the dynamic hurdles that students face when attempting to modify their study habits and highlights the importance of aligning assessment methods with life-long learning goals. However, this study has various limitations. First, we conducted convenience sampling. We did not target a specific target population but all first-year biomedical sciences and medicine students were eligible to participate. As the study goal was advertised as an exploration of students’ study methods and habits, it might have been possible that especially students who were more confident in their study methods or habits participated leading to a biased perspective. While we cannot rule this out, students were openly sharing that they engaged in methods that they thought were not the most effective and also mention not currently wanting to change their habits. Furthermore, students’ self-reported GPA ranged from 5.8 to 9, suggesting a mix between low- and high-achieving students. Additionally, the students’ in our sample received a formal learning strategy training in their first weeks to support their self-regulated learning and professional development, which potentially shaped their study behaviors and perspectives and thus outcomes of this study. Next to this, we did not split students from the two different study programs but mixed them within our focus groups. As mentioned above, the structure of the study programs might have shaped the results and further research is necessary to investigate to what extent these findings transfer to other study programs. Second, we held four focus groups at the end of students’ first academic year and two focus groups at the beginning of students’ second academic year. This change in context might have influenced students’ perspectives on their study methods and habits. Future research is necessary to investigate to what extent students’ study methods and habits change throughout their study program. Third, we asked students to reflect on their habitual behavior. As habits are often unconscious, we did not explicitly ask students about their habits but instead asked them to reflect on behavior they usually engaged in. The fact that we did not offer any definition of what counts as a usual behavior might have led to different interpretations hereof. While not the goal of this study, a quantitative research design capturing students’ habitual behavior could give more systematic insights by clearly defining and operationalizing the concepts of “usual behavior” in measurable terms. For example, it could be operationalized as behaviors that participants engage in at least three times a week or behaviors that they have engaged in consistently for the past six months or using the Self-Report Habit Index (SRHI, Verplanken & Orbell, 2003 ).
Overall, this study contributes to the literature by providing a qualitative exploration of university students’ study habits, formation hereof, and difficulties they experience when breaking habits. A thematic analysis of six focus groups indicated that students had different study habits depending on their goals. While showing accurate metacognitive knowledge of what are effective learning strategies, students often used other learning strategies they deemed most efficient in reaching their goals. Students indicated intentions to change but did not prioritize change as their current habits enabled them to pass exams and change was not perceived as adding value. Fluctuations in motivation across courses and transitioning to a self-regulated life hampered students’ intentions to form new and break old habits. The findings give insights into influential factors affecting students’ behavioral change intentions and suggest the importance of aligning assessment methods with life-long learning and supporting students in their long-term academic goal setting to prioritize study habits that target lasting learning to optimally foster students’ self-regulated learning.
Data Availability
Due to the nature of the research, supporting data is not available to ensure privacy of participants.
Aarts, H., & Dijksterhuis, A. (2000). Habits as knowledge structures: Automaticity in goal-directed behavior. Journal of Personality and Social Psychology, 78 (1), 53–63. https://doi.org/10.1037/0022-3514.78.1.53
Article Google Scholar
Adriaanse, M. A., & Verhoeven, A. (2018). Breaking habits using implementation intentions. In B. Verplanken (Ed.), The Psychology of Habit (pp. 169–188). Springer International Publishing. https://doi.org/10.1007/978-3-319-97529-0_10
Adriaanse, M. A., Vinkers, C. D. W., De Ridder, D. T. D., Hox, J. J., & De Wit, J. B. F. (2011). Do implementation intentions help to eat a healthy diet? A systematic review and meta-analysis of the empirical evidence. Appetite, 56 (1), 183–193. https://doi.org/10.1016/j.appet.2010.10.012
Ariel, R., & Karpicke, J. D. (2018). Improving self-regulated learning with a retrieval practice intervention. Journal of Experimental Psychology: Applied, 24 (1), 43–56. https://doi.org/10.1037/xap0000133
Basila, C. (2014). Good time management and motivation level predict student academic success in college online courses. International Journal of Cyber Behavior Psychology and Learning, 4 (3), 45–52. https://doi.org/10.4018/ijcbpl.2014070104
Bembenutty, H., & Karabenick, S. A. (2004). Inherent association between academic delay of gratification, future time perspective, and self-regulated learning. Educational Psychology Review, 16 (1), 35–57. https://doi.org/10.1023/B:EDPR.0000012344.34008.5c
Biwer, F., & De Bruin, A. B. H. (2023). Teaching students to ‘Study Smart’ – A training program based on the science of learning. In In their own words: What scholars and teachers want you to know about why and how to apply the science of learning in your academic setting (pp. 411–425). Society for the Teaching of Psychology. https://teachpsych.org/ebooks/itow
Biwer, F., De Bruin, A. B. H., Schreurs, S., & Oude Egbrink, M. G. A. (2020a). Future steps in teaching desirably difficult learning strategies: Reflections from the study smart program. Journal of Applied Research in Memory and Cognition, 9 (4), 439–446. https://doi.org/10.1016/j.jarmac.2020.07.006
Biwer, F., Egbrink, M. G. A. O., Aalten, P., & De Bruin, A. B. H. (2020b). Fostering effective learning strategies in higher education—A mixed-methods study. Journal of Applied Research in Memory and Cognition, 9 (2), 186–203. https://doi.org/10.1016/j.jarmac.2020.03.004
Bjork, E. L., & Bjork, R. A. (2011). Making things hard on yourself, but in a good way: Creating desirable difficulties to enhance learning (pp. 56–64). Essays Illustrating Fundamental Contributions to Society.
Google Scholar
Blasiman, R. N., Dunlosky, J., & Rawson, K. A. (2017). The what, how much, and when of study strategies: Comparing intended versus actual study behaviour. Memory, 25 (6), 784–792. https://doi.org/10.1080/09658211.2016.1221974
Bowen, G. A. (2006). Grounded theory and sensitizing concepts. International Journal of Qualitative Methods, 5 (3), 12–23. https://doi.org/10.1177/160940690600500304
Bransen, D., Govaerts, M. J. B., Panadero, E., Sluijsmans, D. M. A., & Driessen, E. W. (2022). Putting self-regulated learning in context: Integrating self-, co-, and socially shared regulation of learning. Medical Education, 56 (1), 29–36. https://doi.org/10.1111/medu.14566
Braun, V., & Clarke, V. (2006). Using thematic analysis in psychology. Qualitative Research in Psychology, 3 (2), 77–101. https://doi.org/10.1191/1478088706qp063oa
Braun, V., & Clarke, V. (2019). To saturate or not to saturate? Questioning data saturation as a useful concept for thematic analysis and sample-size rationales. Qualitative Research in Sport, Exercise and Health., 13 (2), 201–216. https://doi.org/10.1080/2159676X.2019.1704846
Broekkamp, H., & Van Hout-Wolters, B. (2007). The gap between educational research and practice: A literature review, symposium, and questionnaire. Educational Research and Evaluation, 13 (3), 203–220. https://doi.org/10.1080/13803610701626127
Broeren, M., Heijltjes, A., Verkoeijen, P., Smeets, G., & Arends, L. (2021). Supporting the self-regulated use of retrieval practice: A higher education classroom experiment. Contemp Educ Psychol, 64 , Article 101939. https://doi.org/10.1016/j.cedpsych.2020.101939
Chen, Q., & Yan, Z. (2016). Does multitasking with mobile phones affect learning? A review. Computers in Human Behavior, 54 , 34–42. https://doi.org/10.1016/j.chb.2015.07.047
Cherryholmes, C. H. (1992). Notes on pragmatism and scientific realism. Educational Researcher, 21 (6), 13–17. https://doi.org/10.3102/0013189X021006013
Danner, U. N., Aarts, H., & De Vries, N. K. (2007). Habit formation and multiple means to goal attainment: Repeated retrieval of target means causes inhibited access to competitors. Personality and Social Psychology Bulletin, 33 (10), 1367–1379. https://doi.org/10.1177/0146167207303948
De Bilde, J., Vansteenkiste, M., & Lens, W. (2011). Understanding the association between future time perspective and self-regulated learning through the lens of self-determination theory. Learning and Instruction, 21 (3), 332–344. https://doi.org/10.1016/j.learninstruc.2010.03.002
Dembo, M. H., & Seli, H. P. (2004). Students’ resistance to change in learning strategies courses. Journal of Developmental Education, 27 (3), 2–11.
Dignath, C., & Veenman, M. V. J. (2021). The role of direct strategy instruction and indirect activation of self-regulated learning—Evidence from classroom observation studies. Educational Psychology Review, 33 (2), 489–533. https://doi.org/10.1007/s10648-020-09534-0
Dirkx, K. J. H., Camp, G., Kester, L., & Kirschner, P. A. (2019). Do secondary school students make use of effective study strategies when they study on their own? Applied Cognitive Psychology, 33 (5), 952–957. https://doi.org/10.1002/acp.3584
Dolmans, D. H. J. M., De Grave, W., Wolfhagen, I. H. A. P., & Van Der Vleuten, C. P. M. (2005). Problem-based learning: Future challenges for educational practice and research. Medical Education, 39 (7), 732–741. https://doi.org/10.1111/j.1365-2929.2005.02205.x
Dresel, M., Schmitz, B., Schober, B., Spiel, C., Ziegler, A., Engelschalk, T., Jöstl, G., Klug, J., Roth, A., Wimmer, B., & Steuer, G. (2015). Competencies for successful self-regulated learning in higher education: Structural model and indications drawn from expert interviews. Studies in Higher Education, 40 (3), 454–470. https://doi.org/10.1080/03075079.2015.1004236
Dunlosky, J., Rawson, K. A., Marsh, E. J., Nathan, M. J., & Willingham, D. T. (2013). Improving students’ learning with effective learning techniques: Promising directions from cognitive and educational psychology. Psychological Science in the Public Interest, 14 (1), 4–58. https://doi.org/10.1177/1529100612453266
Fiorella, L. (2020). The science of habit and its implications for student learning and well-being. Educational Psychology Review, 32 (3), 603–625. https://doi.org/10.1007/s10648-020-09525-1
Foerst, N. M., Klug, J., Jöstl, G., Spiel, C., & Schober, B. (2017). Knowledge vs. Action: Discrepancies in university students’ knowledge about and self-reported use of self-regulated learning strategies. Frontiers in Psychology , 8, Article 1288. https://www.frontiersin.org/articles/10.3389/fpsyg.2017.01288
Galla, B. M., & Duckworth, A. L. (2015). More than resisting temptation: Beneficial habits mediate the relationship between self-control and positive life outcomes. Journal of Personality and Social Psychology, 109 (3), 508–525. https://doi.org/10.1037/pspp0000026
Gardner, B. (2015). A review and analysis of the use of ‘habit’ in understanding, predicting and influencing health-related behaviour. Health Psychology Review, 9 (3), 277–295. https://doi.org/10.1080/17437199.2013.876238
Gollwitzer, P. M., & Sheeran, P. (2006). Implementation intentions and goal achievement: A meta‐analysis of effects and processes. In M.P. Zanna (Ed.), Advances in Experimental Social Psychology , Vol. 38, Elsevier Academic Press, pp. 69–119. https://doi.org/10.1016/S0065-2601(06)38002-1
Gollwitzer, P. M. (1993). Goal achievement: The role of intentions. European Review of Social Psychology, 4 (1), 141–185. https://doi.org/10.1080/14792779343000059
Grunschel, C., Schwinger, M., Steinmayr, R., & Fries, S. (2016). Effects of using motivational regulation strategies on students’ academic procrastination, academic performance, and well-being. Learning and Individual Differences, 49 , 162–170. https://doi.org/10.1016/j.lindif.2016.06.008
Hadwin, A., & Oshige, M. (2011). Self-regulation, coregulation, and socially shared regulation: Exploring perspectives of social in self-regulated learning theory. Teachers College Record, 113 (2), 240–264. https://doi.org/10.1177/016146811111300204
Hartwig, M. K., & Dunlosky, J. (2012). Study strategies of college students: Are self-testing and scheduling related to achievement? Psychonomic Bulletin & Review, 19 (1), 126–134. https://doi.org/10.3758/s13423-011-0181-y
Hoch, E., Scheiter, K., & Schüler, A. (2020). Implementation intentions related to self-regulatory processes do not enhance learning in a multimedia environment. Frontiers in Psychology, 11 , Article 46. https://doi.org/10.3389/fpsyg.2020.00046
Kiger, M. E., & Varpio, L. (2020). Thematic analysis of qualitative data: AMEE Guide No. 131. Medical Teacher, 42 (8), 846–854. https://doi.org/10.1080/0142159x.2020.1755030
Lally, P., & Gardner, B. (2013). Promoting habit formation. Health Psychology Review, 7 (1), 137–158. https://doi.org/10.1080/17437199.2011.603640
Lally, P., Van Jaarsveld, C. H. M., Potts, H. W. W., & Wardle, J. (2010). How are habits formed: Modelling habit formation in the real world. European Journal of Social Psychology, 40 (6), 998–1009. https://doi.org/10.1002/ejsp.674
Lally, P., Wardle, J., & Gardner, B. (2011). Experiences of habit formation: A qualitative study. Psychology, Health & Medicine, 16 (4), 484–489. https://doi.org/10.1080/13548506.2011.555774
Lepp, A., Barkley, J. E., & Karpinski, A. C. (2015). The relationship between cell phone use and academic performance in a sample of US college students. SAGE Open, 5 (1), Article 215824401557316. https://doi.org/10.1177/2158244015573169
Loersch, C., & Payne, B. K. (2011). The situated inference model: An integrative account of the effects of primes on perception, behavior, and motivation. Perspectives on Psychological Science, 6 (3), 234–252. https://doi.org/10.1177/1745691611406921
Mazar A., Wood W. (2018). Defining habit in psychology. In Verplanken B. (Ed.), The psychology of habit: Theory, mechanisms, change, and context (pp. 13–29). Springer. https://doi.org/10.1007/978-3-319-97529-0_2
McDaniel, M. A., & Einstein, G. O. (2020). Training learning strategies to promote self-regulation and transfer: The knowledge, belief, commitment, and planning framework. Perspectives on Psychological Science, 15 (6), 1363–1381. https://doi.org/10.1177/1745691620920723
Morehead, K., Rhodes, M. G., & DeLozier, S. (2016). Instructor and student knowledge of study strategies. Memory, 24 (2), 257–271. https://doi.org/10.1080/09658211.2014.1001992
Morse, J. M. (2008). “It’s only a qualitative study!” Considering the qualitative foundations of social sciences. Qualitative Health Research, 18 , 147–148. https://doi.org/10.1177/1049732307310262
Morse, J. M. (2015). Data Were Saturated. …. Qualitative Health Research, 25 (5), 587–588. https://doi.org/10.1177/1049732315576699
Moust, J. H. C., Van Berkel, H. J. M., & Schmidt, H. G. (2005). Signs of erosion: Reflections on three decades of problem-based learning at Maastricht University. Higher Education, 50 (4), 665–683. https://doi.org/10.1007/s10734-004-6371-z
Panadero, E. (2017). A review of self-regulated learning: Six models and four directions for research. Frontiers in Psychology , 8, Article 422. https://www.frontiersin.org/articles/10.3389/fpsyg.2017.00422
Rea, S. D., Wang, L., Muenks, K., & Yan, V. X. (2022). Students can (mostly) recognize effective learning, so why do they not do it? Journal of Intelligence, 10 (4), Article 127. https://doi.org/10.3390/jintelligence10040127
Rothman, A. J., Sheeran, P., & Wood, W. (2009). Reflective and automatic processes in the initiation and maintenance of dietary change. Annals of Behavioral Medicine, 38 (S1), 4–17. https://doi.org/10.1007/s12160-009-9118-3
Rovers, S. F. E., Stalmeijer, R. E., Van Merriënboer, J. J. G., Savelberg, H. H. C. M., & De Bruin, A. B. H. (2018). How and why do students use learning strategies? A mixed-methods study on learning strategies and desirable difficulties with effective strategy users. Frontiers in Psychology, 9 , Article 2501. https://doi.org/10.3389/fpsyg.2018.02501
Stalmeijer, R. E., McNaughton, N., & Van Mook, W. N. K. A. (2014). Using focus groups in medical education research: AMEE Guide No. 91. Medical Teacher, 36 (11), 923–939. https://doi.org/10.3109/0142159X.2014.917165
Thibodeaux, J., Deutsch, A., Kitsantas, A., & Winsler, A. (2017). First-year college students’ time use: Relations with self-regulation and GPA. Journal of Advanced Academics, 28 (1), 5–27. https://doi.org/10.1177/1932202X16676860
Van Der Vleuten, C. P. M., Schuwirth, L. W. T., Scheele, F., Driessen, E. W., & Hodges, B. (2010). The assessment of professional competence: Building blocks for theory development. Best Practice & Research Clinical Obstetrics & Gynaecology, 24 (6), 703–719. https://doi.org/10.1016/j.bpobgyn.2010.04.001
Verhoeven, A. A. C., Adriaanse, M. A., Evers, C., & De Ridder, D. T. D. (2012). The power of habits: Unhealthy snacking behaviour is primarily predicted by habit strength. British Journal of Health Psychology, 17 (4), 758–770. https://doi.org/10.1111/j.2044-8287.2012.02070.x
Verplanken, B., Roy, D., & Whitmarsh, L. (2018). Cracks in the wall: Habit discontinuities as vehicles for behaviour change. In B. Verplanken (Ed.), The Psychology of Habit (pp. 189–205). Springer International Publishing. https://doi.org/10.1007/978-3-319-97529-0_11
Verplanken, B. (2018). Introduction. In B. Verplanken (Ed.), The Psychology of Habit (pp. 1–10). Springer International Publishing. https://doi.org/10.1007/978-3-319-97529-0_1
Verplanken, B., & Orbell, S. (2003). Reflections on past behavior: A self-report index of habit strength. Journal of Applied Social Psychology, 33 (6), 1313–1330. https://doi.org/10.1111/j.1559-1816.2003.tb01951.x
Verplanken, B., & Wood, W. (2006). Interventions to break and create consumer habits. Journal of Public Policy & Marketing, 25 (1), 90–103. https://doi.org/10.1509/jppm.25.1.90
Waldeyer, J., Fleischer, J., Wirth, J., & Leutner, D. (2020). Validating the resource-management inventory (ReMI): Testing measurement invariance and predicting academic achievement in a sample of first-year university students. European Journal of Psychological Assessment, 36 (5), 777–786. https://doi.org/10.1027/1015-5759/a000557
Wood, W., & Neal, D. T. (2016). Healthy through habit: Interventions for initiating & maintaining health behavior change. Behavioral Science & Policy, 2 (1), 71–83. https://doi.org/10.1177/237946151600200109
Wood, W., & Rünger, D. (2016). Psychology of habit. Annual Review of Psychology, 67 , 289–314. https://doi.org/10.1146/annurev-psych-122414-033417
Yan, V. X., Bjork, E. L., & Bjork, R. A. (2016). On the difficulty of mending metacognitive illusions: A priori theories, fluency effects, and misattributions of the interleaving benefit. Journal of Experimental Psychology: General, 145 (7), 918–933. https://doi.org/10.1037/xge0000177
Zimmerman, B. J. (1986). Becoming a self-regulated learner: Which are the key subprocesses? Contemporary Educational Psychology, 11 (4), 307–313. https://doi.org/10.1016/0361-476X(86)90027-5
Download references
Acknowledgements
We thank our participants for their time-commitment and sharing their experiences openly. Additionally, we would like to thank the members of the EARLI Emerging Field Group 3 “Monitoring and Regulation of Effort” for the ongoing discussions on the topic of effort monitoring and regulation that contributed to this research.
This work was funded by the Dutch Research Council (NWO) (VIDI grant number VI.Vidi.195.135) awarded to Anique de Bruin.
Author information
Authors and affiliations.
Department of Educational Development and Research, School of Health Professions Education (SHE), Maastricht University, P.O. Box 616, 6200 MD, Maastricht, the Netherlands
Louise David, Felicitas Biwer & Anique de Bruin
Department of Health Promotion, School of Care and Public Health Research Institute (CAPHRI), Maastricht University, Maastricht, the Netherlands
Rik Crutzen
You can also search for this author in PubMed Google Scholar
Contributions
All authors contributed to the study concept and design. Material preparation, data collection, and analysis were performed by LD and FB in close consultation with AdB and RC. The first draft of the manuscript was written by LD. All authors commented on previous versions of the manuscript and read and approved the manuscript.
Corresponding author
Correspondence to Louise David .
Ethics declarations
The artificial intelligence tool Chat GPT 3.5 was used to refine the titles of four of the identified (sub-)themes. Based on these suggestions, the titles were adapted. We kept a list of the tools’ original title suggestions.
Conflict of interest
The authors declare no competing interests.
Additional information
Publisher's note.
Springer Nature remains neutral with regard to jurisdictional claims in published maps and institutional affiliations.
Supplementary Information
Below is the link to the electronic supplementary material.
Supplementary file1 (DOCX 20 KB)
Supplementary file2 (docx 22 kb), rights and permissions.
Open Access This article is licensed under a Creative Commons Attribution 4.0 International License, which permits use, sharing, adaptation, distribution and reproduction in any medium or format, as long as you give appropriate credit to the original author(s) and the source, provide a link to the Creative Commons licence, and indicate if changes were made. The images or other third party material in this article are included in the article's Creative Commons licence, unless indicated otherwise in a credit line to the material. If material is not included in the article's Creative Commons licence and your intended use is not permitted by statutory regulation or exceeds the permitted use, you will need to obtain permission directly from the copyright holder. To view a copy of this licence, visit http://creativecommons.org/licenses/by/4.0/ .
Reprints and permissions
About this article
David, L., Biwer, F., Crutzen, R. et al. The challenge of change: understanding the role of habits in university students’ self-regulated learning. High Educ (2024). https://doi.org/10.1007/s10734-024-01199-w
Download citation
Accepted : 12 February 2024
Published : 10 April 2024
DOI : https://doi.org/10.1007/s10734-024-01199-w

Share this article
Anyone you share the following link with will be able to read this content:
Sorry, a shareable link is not currently available for this article.
Provided by the Springer Nature SharedIt content-sharing initiative
- Desirable difficulties
- Self-regulated learning
- Behavioral change
- Higher education
- Find a journal
- Publish with us
- Track your research
ORIGINAL RESEARCH article
Relationships among students’ reading habits, study skills, and academic achievement in english at the secondary level.
- 1 Department of Education, School of Social Sciences and Humanities, University of Management and Technology, Lahore, Pakistan
- 2 Faculty of Education and Humanities, UNITAR International University, Petaling Jaya, Malaysia
- 3 Faculty of Education, Umm Al-Qura University, Mecca, Saudi Arabia
- 4 Department of English Language and Literature, Prince Sattam Bin Abdulaziz University, Al-Kharj, Saudi Arabia
Introduction: Reading is an attempt to comprehend the writer’s message for personal growth and success in the relevant fields. Thus, psychologists consider it a multifaceted cognitive process of constructing meanings from texts. The present study was conducted to determine the relationships among students’ reading habits, study skills, and academic achievement in English at the secondary level in Punjab, Pakistan.
Methods: The ( n = 1614) students enrolled in the science section for the academic year 2019–2020 participated in this descriptive correlational survey, selected from 40 high schools in Lahore, Punjab, Pakistan, through a non-proportionate stratified random sampling technique. The Reading Habits Questionnaire (RHQ) and the Study Skills Scale (SSS) were used to collect data about students’ reading habits and study skills. At the same time, academic achievement was the students’ grades obtained in the ninth class in the subject of English that were determined by the Board of Intermediate and Secondary Education (BISE) Lahore in 2019. Students’ responses were analyzed through descriptive and inferential statistics.
Results: The results indicated that students have competent reading habits and study skills. The correlational findings showed a strong positive relationship among reading habits, study skills, and academic achievement in English, while moderate positive relationships between reading habits and academic achievement in English. However, regression analysis results were significant, while reading habits and study skills moderately predicted academic achievement.
Discussion: It is implicated that teachers should plan such assignments and tasks based on reflective thinking by considering the role of study skills in academic achievement. Moreover, teachers and school administrators could mutually create timetables for library lessons to build reading habits and study skills among learners.
1. Introduction
Knowledge gained through reading is vital for the cognitive, behavioral, and attitudinal development of learners ( He, 2014 ; Baffoe and Okae-Anti, 2020 ; Hassan et al., 2021 ) because it is a person’s ability to enhance information and comprehend the words effectively ( Sabbah, 2016 ; Al-Jarf, 2019 ). An individual reads for numerous reasons, i.e., knowledge development, recreation, joy, relaxation, and so on ( Whitten et al., 2016 ). However, Erguvan (2016) and Mirza et al. (2021) directed that reading is an active part of life that is not just about pleasure when needed. However, Chotitham and Wongwanich (2014) conjectured that reading helps to develop critical and judgmental thinking abilities used to solve problems by conceptualizing context. Hence, Erdem (2015) and Pretorius and Klapwijk (2016) quantified that reading is essential to success because it starts from the commencement of school and continues throughout the lifetime.
Fischer et al. (2015) , Oyewole (2017) , Al-Jarf (2019) recognized that the importance of reading in learning could not be ignored because it is an emancipatory tool that releases students’ academic frustration, ignorance, and destitution. Palani (2012) distinguished that reading is an instrument used to exchange information, while reading habit is an academic activity that enables students to benefit from reading materials. Therefore, Walia and Sinha (2014) specified that reading habits require complex skills, such as perceiving a message, skimming and scanning information, and understanding the context. Thus, compelling reading depends on readers’ behaviors, known as study skills that enable them to conceptualize the new knowledge effectively ( DiPerna and Elliott, 2000 ; Habibu and Ejembi, 2011 ; Gormley et al., 2018 ; Naqvi et al., 2018 ; Iheakanwa et al., 2021 ). While the effective study makes one narrate in their way using the stipulated meanings of the words and terms, the researchers take up for explanation and clarity ( Biyik et al., 2017 ).
According to the available literature, students’ reading habits and study skills have been of great importance for decades; while several deficiencies were found in previous studies, thus researchers considered few of them that are related to the study context. First, the researchers mainly focused on the influence of reading habits and study skills on academic achievement separately in Western countries ( Bhan and Gupta, 2010 ; Sabbah, 2016 ). A few addressed Eastern countries restricted to the university level ( Demir et al., 2012 ; Davarci, 2013 ; Dilshad et al., 2013 ; Erguvan, 2016 ; Alzahrani et al., 2018 ; Porkaew and Fongpaiboon, 2018 ; Thamarasseri, 2018 ; Ameyaw and Anto, 2019 ; Ehsan and Sultana, 2020 ; Tonka and Bakir, 2020 ; Mirza et al., 2021 ; Nguyen Thi Thu, 2022 ). However, students’ reading habits and study skills may be initiated from the school level enabling the individuals to grow in competence, comfort, and understanding of the audience. At the same time, previous researchers focused on university level students’ reading habits. Second, there are methodological identities that lead to dubious findings not confirming the influence of reading habits and study skills on academic achievement ( Goel, 2014 ; Lawrence, 2014 ; Quadir and Chen, 2015 ; Sherafat and Murthy, 2016 ; Ameyaw and Anto, 2018 ; Silverrajoo and Hassan, 2018 ; Balan et al., 2019 ; Hassan et al., 2021 ). In general, there is a scarcity of research aiming to determine the correlation between students’ reading habits and achievement through the role of study skills at any academic level. Finally, in Pakistan, few studies could explore reading habits as a singular variable of different groups of students ( Bajwa et al., 2011 ; Hussain and Munshi, 2011 ; Rasheed, 2012 ). Numerous researchers only examined the relationship between reading habits and academic achievement ( Bashir and Mattoo, 2012 ; Bibi et al., 2020 ; Ehsan and Sultana, 2020 ). Moreover, Fazal et al. (2012) only investigated the association between study skills and achievement. Thus, this research examines the relationship among students’ reading habits, study skills, and academic achievement in English as practiced at the secondary level in Lahore, Punjab, Pakistan.
Lahore is the capital of Pakistan’s Punjab province. In terms of population, this is the second largest city in Pakistan. It is located in the northeastern part of Pakistan’s Punjab province. Lahore is one of the most cosmopolitan cities in Pakistan and is home to various cultures, traditions, and customs. Specifically, it provides researchers with opportunities to contextualize perspectives in light of academic processes and ethics.
2. Literature review
2.1. reading habits.
Rosli et al. (2018) suggested that reading is an attempt to comprehend the writer’s message, while Alnahdi and Aftab (2020) stated that it is a gateway to all other information, which may lead to understanding the world outside the text. Hence, Al-Jarf (2021) and Dadzie (2008) asserted that reading is a multifaceted cognitive process of comprehending words written in a textual form that allows readers to enhance their knowledge for personal growth and academic success. Moreover, Ogeyik and Akyay (2009) ; Erguvan (2016) , Mirza et al. (2021) stated that reading is just a method of communication between the writer and the reader. Thus, Bhan and Gupta (2010) and Baron (2017) assumed that reading is the art of decoding and interpreting messages from various written materials such as books, magazines, journals, newspapers, dictionaries, encyclopedias, pamphlets, and diaries. Hassan et al. (2021) stated that reading habits influence reading materials, activities, time duration, place of reading, and reader motivation. In this study, reading habits are considered to be the students’ reading preferences, interest in reading, attitude toward reading, and reading problems during study at the secondary level.
2.2. Study skills
Study skills are the readers’ inclination toward organizing, highlighting, reviewing, reciting, and using devices, flashcards, etc. to comprehend new knowledge effectively ( DiPerna and Elliott, 2000 ; DiPerna, 2006 ; Rozalski, 2008 ; Madhavi et al., 2014 ; Sabbah, 2016 ). While reading habit is the frequency, a reader regularly reads ( Winne, 2013 ). Moreover, study skills are the students’ intellectual practices to process new information effectively and efficiently, while reading habits are considered a psychological trait of one’s personality ( Farrington et al., 2012 ; Pillai, 2012 ; Mansor et al., 2013 ; Shahidi et al., 2014 ; Ameyaw and Anto, 2018 ; Rosli et al., 2018 ). Thus, the concept of study skills is different from reading habits. This research defines study skills as secondary school students’ approaches to comprehending new knowledge.
2.3. Reading habits and academic achievement
Horbec (2012) and Singh (2011) determined a significant positive relationship between students’ reading habits and academic achievement. Hence, Issa et al. (2012) explored that students’ reading patterns vary and have a moderately significant influence on academic success, while Bashir and Mattoo (2012) examined that academic performance is dependent on the level of students’ study habits; thus, reading habits influence on future success, which was confirmed by Owusu-Acheaw and Larson (2014) through quantitative measures. Chotitham and Wongwanich (2014) found a moderate positive relationship between students’ study habits and achievement. However, Lawrence (2014) rejected the association between students’ academic achievement and study habits, and Goel (2014) confirmed that study habits do not influence academic performance. Therefore, Schwabe et al. (2015) , Quadir and Chen (2015) concluded through a quantitative correlational study that heavy reading habits significantly impact reading efficiency; the longer the reading time, the better the results. Malik and Parveen (2016) discovered significant differences in low- and high-academic achievers’ attitudes toward study habits. They determined that high achievers are more concentrated and exhibited better study habits, good time management skills, and punctuality compared to low achievers. In the meantime, Sherafat and Murthy (2016) directed that study habits facilitate learners toward higher achievement because of their significant connections with academic achievement. Consequently, Silverrajoo and Hassan (2018) revealed divergent findings that students’ reading methods have a negative and weak relationship with academic achievement.
Ameyaw and Anto (2018) recognized the importance of reading styles in students’ learning and found that reading styles affect students’ performance. Meanwhile, Alzahrani et al. (2018) verified that students’ reading styles significantly impact their performance. Dolmaz and Kaya (2019) discovered that students’ creative writing skills are affected by their reading styles. Moreover, Balan et al. (2019) determined that students’ purpose of reading significantly affected their performance, as Annamalai and Muniandy (2013) suggested that academic performance is based on students’ reading purpose. Hence, Whitten et al. (2016) and Fatiloro et al. (2017) discovered that reading habits significantly assist students in learning and enhancing their performance. Bibi et al. (2020) examined that students’ study habits were significantly positively associated with achievement. Ehsan and Sultana (2020) predicted that reading habits significantly increase students’ performance. Moreover, Hassan et al. (2021) found a significant correlation between secondary school students’ reading habits and their reading achievement and concluded that reading habits significantly contribute to academic achievement. Thus, Nguyen Thi Thu (2022) revealed that reading habits have a significant role in the development of students writing performance.
On the other hand, by designing a correlational study, Tonka and Bakir (2020) found a negative relationship between reading anxiety and reading habits. Thus, they concluded that reading anxiety plays a role in students’ performance and reading habits. Similarly, Alnahdi and Aftab (2020) found a significant negative association between study habits and academic stress, reading habits, and academic achievement. The researchers measured all the variables through a questionnaire consisting of four scales and 43 items.
2.4. Study skills and academic achievement
Nouhi et al. (2009) determined that study skills have a significant positive association with academic success measured through a closed-ended questionnaire confirmed by Awang and Sinnadurai (2011) through an experimental study. Meanwhile, Hassanbeigi et al. (2011) and Sabbah (2016) verified that study skills are critical for academic success because they positively correlate with academic achievement found through a descriptive correlational survey using a study skills scale. Hence, Fazal et al. (2012) suggested that higher academic achievers use a wide range of study skills than low achievers, while there was a weak correlation between study skills and academic success. Furthermore, Demir et al. (2012) revealed through an experimental study that students’ study skills had a considerable influence on performance which was also confirmed by Wernersbach et al. (2014) from an experimental study. In both of the studies, researchers measured study skills through closed-ended items. Moreover, they also discovered that study skills significantly impact students’ academic self-efficacy. Nonetheless, Tahamtani et al. (2017) and Naqvi et al. (2018) revealed a weak negative link between achievement and study habits through quantitative measures that were rejected by Gormley et al. (2018) , who found a significant positive impact of study skills on achievement.
Several gaps were found in already conducted studies; first, the researchers mainly focused on the influence of reading habits and study skills on academic achievement separately in Western countries, while few addressed this phenomenon in Eastern countries. However, the investigation was restricted to university level students. Second, methodological identities lead to dubious findings not confirming this phenomenon. Finally, in Pakistan, few studies could explore reading habits as a singular variable of different groups of students. At the same time, some researchers only examined the relationship between reading habits and academic achievement. Thus, this study aimed to develop our understanding of the relationship between students’ reading habits, study skills, and academic achievement in English.
2.5. Summary
Reading is an attempt to comprehend the writer’s message for personal growth and success. Thus, psychologists consider it a multifaceted cognitive process of constructing meaning from texts. Bhan and Gupta (2010) stated that reading is the art of decoding and interpreting messages from the content of the written material that is often carried out in magazines, journals, newspapers, books, dictionaries, encyclopedias, pamphlets, diaries, and so on. While reading habits are the degree to which a reader engages in reading while studying skills to gain knowledge. Reading habits assist students in learning more, whereas study skills encourage them to understand new information effectively. Both reading habits and study skills influence students’ academic performance.
Based on literature insights, the following hypotheses are formulated:
Hypothesis (H 1 ): A significant relationship exists between students’ reading habits and their academic achievement in English language comprehension .
Hypothesis (H 2 ): A significant relationship exists between stqudents’ study skills and their academic achievement in English language comprehension.
3.1. Design
A research design is comprised of numerous elements (i.e., research paradigm, research approach, research design, and data collection method that provide guidelines for carrying out the study ( Creswell and Clark, 2017 ; Myers, 2019 ), while a correlational research design is used to determine the relationship between two or more than two variables ( Cohen et al., 2018 ). Thus, a correlational research design of a quantitative approach (positivism paradigm) was used. At the same time, a cross-sectional survey method was applied to collect data about studied variables (i.e., reading habits, study skills, and academic achievement in English).
3.2. Sample
The sample comprised 10th-grade students enrolled in district Lahore’s public sector high schools for the academic year 2019–2020. The inclusion criteria were those students who enrolled in the science section only. During the data collection, the total number of active students in both sections (i.e., science and arts) of 10th grade was 36,847 enrolled at 334 high schools in district Lahore ( Government of Punjab [GOP], 2019 ). While in the science section, the active students were 17,028, considered an accessible population of this study. A total of 1,800 (900 boys and 900 girls) were selected from 40 high schools through a non-proportionate random sampling technique that was 10.57% of the accessible population, which shows the sample was normally distributed. Out of 1,800 selected students, 1,619 participated as respondents because 181 students had not passed the subject English in the ninth-grade annual examination conducted by the Board of Intermediate and Secondary Education (BISE) Lahore. Three students declined to participate in this survey, while two could not complete the questionnaires. Therefore, the final sample consisted of n = 1,614 secondary school students.
3.3. Instruments
Two instruments were used, i.e., the Reading Habits Questionnaire (RHQ) and Study Skills Scale (SSS), to collect data about students’ reading habits and study skills.
Reading Habits Questionnaire (RHQ): The researchers developed a paper and pencil student self-report RHQ based on Ajzen’s (1991) Theory of Planned Behavior (TPB) and the social-cognitive theory of self-regulated learning strategies ( Pintrich et al., 1993 ; Duncan and McKeachie, 2005 ; Duncan et al., 2007 ). Ajzen’s (1991) TPB suggests that socio-psychological characteristics of a person’s behavior, such as reading, influence reader proximal behaviors ( Stokmans, 1999 ; Miesen, 2003 ; Van Schooten et al., 2004 ), while the social-cognitive theory of self-regulating learning strategies suggested that students’ reading habits are meta-cognitively and behaviorally active in a student’s learning process to achieve goals ( Eccles and Wigfield, 2002 ). The classical test theory model was utilized to develop RHQ, which initially consisted of 44 closed-ended items. Each item was constructed on a 5-point Likert-type agreement scale ranging from 1 (strongly disagree) to 5 (strongly agree), which means developing level reading habits to advance level reading habits. However, content validity was ensured by five education and assessment experts to validate the content coverage, language appropriateness, and usability of RHQ at the secondary level. Moreover, a pilot study was conducted on 250 students selected purposively from the target population to confirm unidimensionality among items and scales through exploratory factor analyses (EFAs) using Statistical Package for Social Sciences (SPSS) Version 23 software. Four subscales of RHQ (i.e., preferences for reading, interest in reading, attitude toward reading, and reading problems) were constructed during EFA. In contrast, nine items (two to three from each subscale) were deleted because their factor loading values (λ) were less than 0.5. In an analysis of items, reliability was also determined through Cronbach’s alpha (α = 0.821) value which was statistically acceptable. Psychometric evidence shows that RHQ was reliable for determining students’ reading habits. Improved RHQ consisted of 35 items based on four subscales, i.e., preferences of reading (10 items), interest in reading (nine items), attitude toward reading (nine items), and reading problems (seven items).
Study Skills Scale (SSS): The researchers adopted the SSS from Academic Competence and Evaluation Scale, developed by DiPerna and Elliott in 2000. The validity, as well as reliability of SSS, was confirmed by numerous researchers ( Kettler et al., 2014 ; Strunk, 2014 ; Anthony and DiPerna, 2018 ) and concluded that SSS is a standardized scale to measure study skills. The SSS consisted of 11 items that were also constructed on a 5-point Likert-type frequency scale, ranging from 1 (never) to 5 (almost always) which means developing level to advance level skills ( DiPerna and Elliott, 2000 ). The SSS was also administered to 250 students to ensure reliability through Cronbach’s alpha tests and found a value of α = 0.874 that was suitable to measure study skills in the local context (Pakistan).
Academic Achievement: Students’ marks obtained in ninth grade in the subject of English were asked them that determined by the Board of Intermediate and Secondary Education (BISE) Lahore in 2019. Their obtained scores in the subject of English were considered an academic achievement of students.
3.4. Data collection and analysis
After getting consent from the district education administration officer, the researchers personally gained permission from the selected schools’ principals and class teachers for data collection. All the selected students were informed in their classes about the study purpose and given the right to withdraw from the study at any time before data analyses. RHQ and SSS administration occurred over 8 weeks during mid of the September to mid of November 2019 academic year. Before administering the instruments, participants were informed about the confidentiality procedures. Moreover, the researchers encouraged them to respond honestly and told them to write about their obtained marks in ninth grade in English. After collecting the questionnaires, the researchers quickly scanned the participants’ responses about missing answers, and the students were asked to complete the responses in the questionnaire. A total of 1,614 participants provided valuable responses about their reading habits and study skills. The response rate was 89.6%, acceptable in social sciences research for quantitative data. Students’ responses about reading habits and study skills were analyzed by applying descriptive statistics (i.e., mean, standard deviation, skewness, and kurtosis) and inferential statistics (i.e., Pearson r test and regression test) through SPSS version 25 software.
4.1. Descriptive statistics on students’ reading habits and study skills
The Kolmogorov–Smirnov test was used to check the normality of data. At the same time, the skewness and kurtosis values indicated that data were normally distributed because skewness and kurtosis were between –2 and + 2, which was suitable for parametric statistics ( George, 2011 ; Albers, 2017 ; Mishra et al., 2019 ). Table 1 indicates that students give more preferences to reading than their attitude toward reading, interest in reading, and reading problems as M = 4.13, SD = 0.486; M = 3.91, SD = 0.616; M = 3.68, SD = 0.676; and M = 3.57, SD = 0.813, respectively. Students also thought they were facing reading problems because English was not their native language as M = 3.68; SD = 0.813. Overall, results revealed that students have permissive and desired reading habits and study skills as M = 3.88, SD = 0.455 and M = 3.85, SD = 0.602.
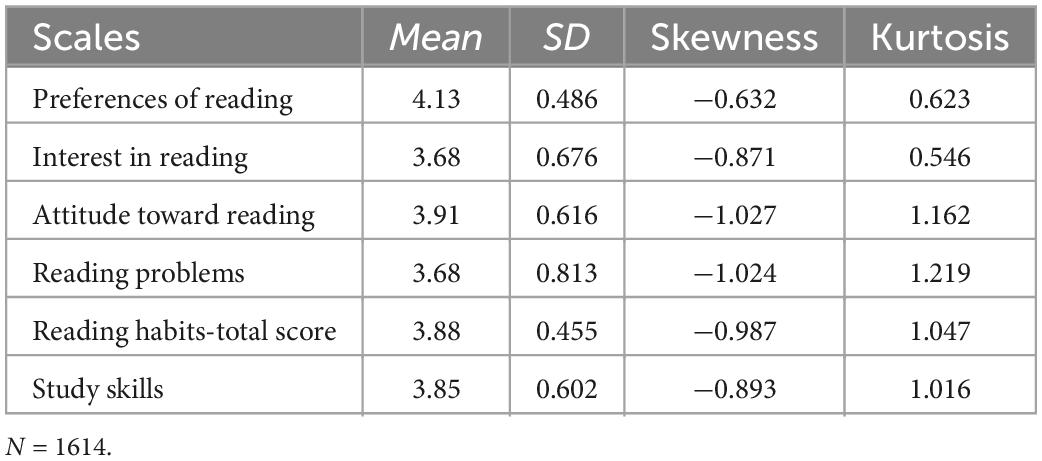
Table 1. Descriptive statistics of reading habits and study skills.
4.2. Inferential statistics on students’ reading habits, study skills, and academic achievement in English
The Pearson product–moment correlation analysis was applied to test null hypotheses, i.e., whether there are significant relationships among students’ reading habits, study skills, and academic achievement in English.
4.3. Hypotheses testing
Refer to Table 2 for the intercorrelation of variables among reading habits subscales, overall reading habits, and study skills; there were moderate-to-high positive correlations among variables. For reading habits, study skills, and academic achievement in English, students’ reading habits were significantly and positively correlated as r = 0.314–0.721. A value of r = (±) 0.3–0.7 exhibits a moderate-to-high correlation between variables ( Akoglu, 2018 ; Schober et al., 2018 ). Results also reveal higher positive correlations between reading habits and study skills while moderate positive correlations between reading habits and academic achievement in English as r = 0.848 and 0.584, respectively. Moreover, there was a higher positive correlation between study skills and academic achievement in English as r = 0.721. Thus, it is revealed that students’ reading habits and study skills are positively associated with academic achievement in English.
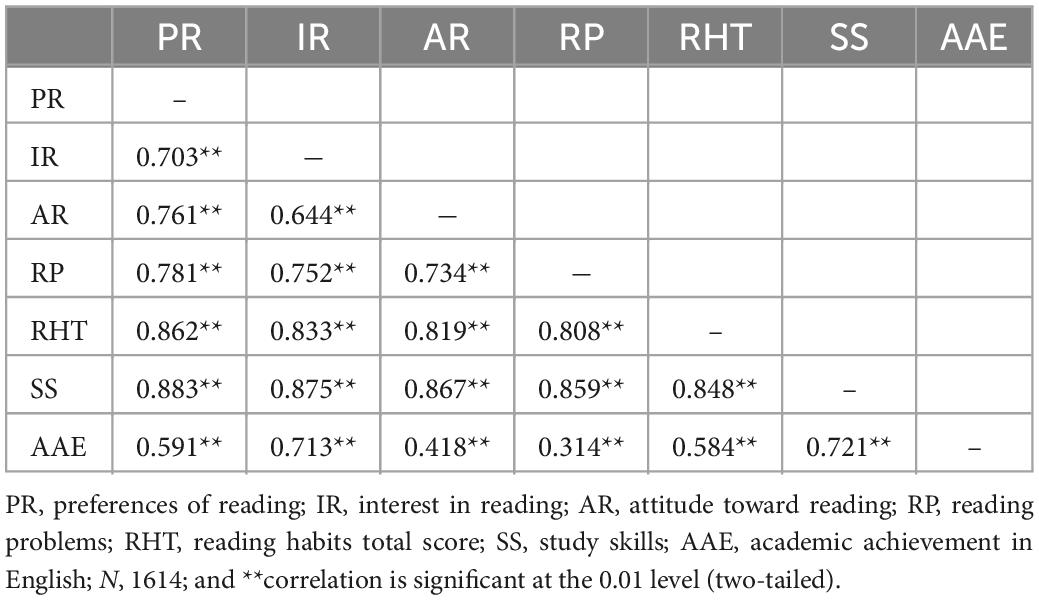
Table 2. Intercorrelations matrix and relationships among students’ reading habits, study skills, and academic achievement in English.
Since the hypotheses “there is a significant relationship between students’ reading habits and academic achievement in English, and there is a significant relationship between students’ study skills and academic achievement in English” were accepted because moderate-to-strong positive relationships were found among students’ reading habits, study skills, and academic achievement in English.
A regression analysis was conducted to explore whether students’ reading habits and study skills predict academic achievement in English. Students’ reading habits and study skills served as independent variables, while students’ academic achievement in English served as dependent variables. The regression analysis results were significant. The unique individual predictor for students’ academic achievement in English interested in reading and attitude toward reading. These two sub-factors of reading habits significantly predicted 42 and 43% of the variance, respectively.
In contrast, the numeric regression does not considerably reveal the remaining two sub-factors (preferences of reading and reading problems). However, students’ reading habits accounted for 44% of the variance, and study skills accounted for 48% of the variance. Refer to Table 3 for unstandardized betas, standard errors, standardized betas, and adjusted R 2 . The independent variables in these analyses are moderately correlated and predict academic achievement because the variance inflation factor (VIF) estimation was below 5.0 in regression.
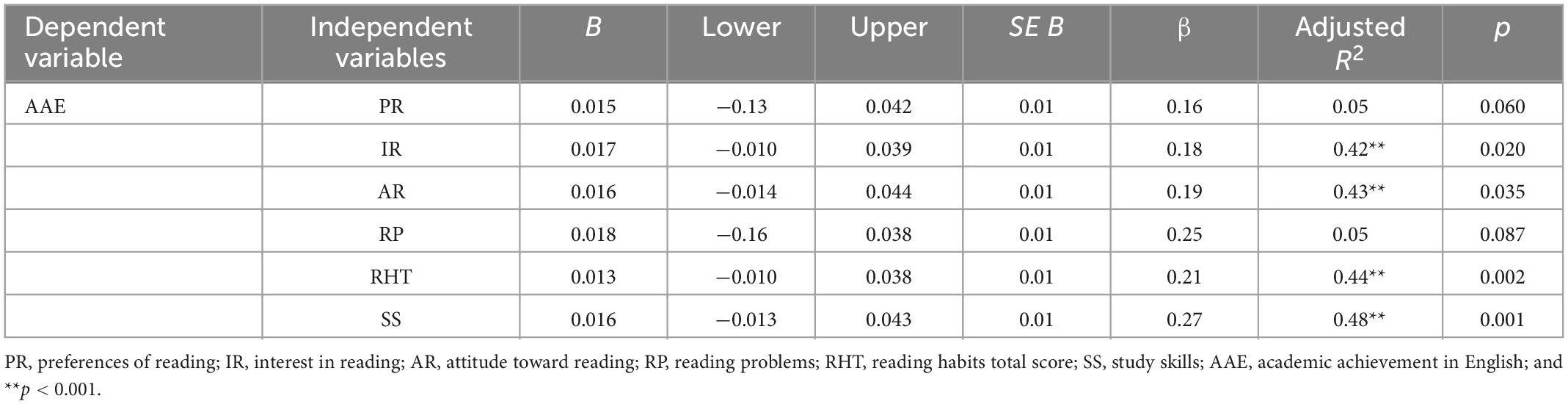
Table 3. Summary of regression analyses, with 95% confidence intervals, of students’ reading habits and study skills predicting academic achievement in English.
5. Discussion
Reading habit is a crucial aspect of creating a literate society because it helps to shape personality, develop creative and critical thinking abilities, and enhance knowledge ( Palani, 2012 ; Mansor et al., 2013 ; Fischer et al., 2015 ; Bano et al., 2018 ; Rosli et al., 2018 ; Al-Jarf, 2019 ; Wu et al., 2019 ; Hassan et al., 2021 ). At the same time, study skills are the readers’ strategies to process new information effectively ( Kuterbach, 2012 ; Anthony and DiPerna, 2018 ; Abid et al., 2021 ). Both reading habits and study skills are interdependent and influence students’ academic performance as well as future success ( Demir et al., 2012 ; Wernersbach et al., 2014 ; Tahamtani et al., 2017 ; Alzahrani et al., 2018 ; Ameyaw and Anto, 2018 ; Gormley et al., 2018 ; Balan et al., 2019 ; Dolmaz and Kaya, 2019 ; Ehsan and Sultana, 2020 ). Therefore, this study is designed to examine relationships among students’ reading habits, study skills, and academic achievement at the secondary level in Lahore, Pakistan. Lahore is one of the cosmopolitan cities of Pakistan and a hub of many cultures, traditions, and customs. Regarding the academic processes and ethics, it provides opportunities for researchers to contextualize the perspectives accordingly. Reading habits have been and are still being taught in schools, colleges, and universities through model reading by teachers, parents, or elders of the families. In addition, the reading and recitation of fold tales and poems get to gathers like at Pak Tea House, Lawrence Garden, Quaid-e-Azam Library, and so on, while formal schools books, extra reading exercises, and reading and writing competitions at the school level are prepared through a variety of book reading within the context of the particular objective. The results of normality tests indicated that the data were normality distributed and suitable to apply parametric statistics. The descriptive findings also showed that students have more preferences for reading than their attitude toward reading, interest in reading, and reading challenges. These results support the finding of numerous researchers (e.g., Pehlivan et al., 2010 ; Mansor et al., 2013 ; Owusu-Acheaw and Larson, 2014 ; Haliru et al., 2015 ; Erguvan, 2016 ; Krashen, 2016 ; Kulatunga, 2016 ; Loan and Shah, 2017 ; Ameyaw and Anto, 2018 ; Porkaew and Fongpaiboon, 2018 ; Mirza et al., 2021 ) who found that students give more preference to read academic content from textbooks and other reading materials (i.e., newspaper, storybooks, poetry, novel, magazines, cartoons, comics, sports, etc.). Rasheed (2012) determined that reading habits play a substantial essential role in developing positive attitudes toward reading. However, Maiyo and Siahi (2015) revealed that higher achievers had better reading habits than low achievers. Students prefer reading online because they can easily read content from the internet material in this technological age, so they prefer reading online ( Dollah et al., 2017 ). Thus, Molotja and Themane (2018) found that students’ reading habits may enhance through global reading strategies and problem-solving strategies. Moreover, it is found that students have competence in reading habits and study skills. These results are also in line with the findings of previous studies, e.g., Dadzie (2008) , Ogeyik and Akyay (2009) , Bhan and Gupta (2010) , and Issa et al. (2012) , and Sabbah (2016) revealed that the majority of the students read books to pass the exams that why they have good reading habits. Furthermore, it is determined that students also possess the competence level of study skills that confirmed the study conducted by numerous researchers (i.e., DiPerna, 2004 , 2006 ; Rozalski, 2008 ; Kuterbach, 2012 ; DuPaul and Stoner, 2014 ; Anthony and DiPerna, 2018 ; Abid et al., 2021 ).
Furthermore, researchers also concluded from correlational results that there were moderate-to-significant positive correlations among reading habits, study skills, and academic achievement in English. In the literature review, it is seen that these findings are consistent with the results of Singh (2011) , Horbec (2012) , Issa et al. (2012) , Sabbah (2016) , Ameyaw and Anto (2018) , Hassan et al. (2021) . They found a positive relationship between reading habits and academic achievement, while reading habits influence students’ academic performance. Moreover, some researchers determined a moderate relationship between reading habits and academic success (e.g., Chotitham and Wongwanich, 2014 ; Kutay, 2014 ; Owusu-Acheaw and Larson, 2014 ; Alzahrani et al., 2018 ; Adigun et al., 2021 ; Nguyen Thi Thu, 2022 ). Sherafat and Murthy (2016) directed that study habits facilitate learners toward higher achievement because of their significant connections with academic achievement, that confirmed by Bibi et al. (2020) . In contrast, few researchers found different results due to participants’ different selection procedures and contextual differences (i.e., purposive sample method, content, reading material, culture, etc.). For example, Lawrence (2014) ; Goel (2014) , Alnahdi and Aftab (2020) revealed no significant correlation between students’ academic achievement and study habits. At the same time, Silverrajoo and Hassan (2018) found that students’ reading styles have a negative, weak relationship with academic achievement. Findings regarding study skills: e.g., Nouhi et al. (2009) , Awang and Sinnadurai (2011) , Hassanbeigi et al. (2011) , Maiyo and Siahi (2015) , Gormley et al. (2018) revealed a significant positive connection between study skills and academic success, whereas Demir et al. (2012) and Wernersbach et al. (2014) found study skills have a considerable influence on performance that support the present study findings. However, few researchers found a negative correlation between study skills and academic performance ( Fazal et al., 2012 ; Tahamtani et al., 2017 ; Naqvi et al., 2018 ). Furthermore, researchers determined in this study students’ reading habits have positive correlations with study skills. Thus, reading habits and study skills directly correlate with their academic achievement in English. In addition, it is also revealed that reading habits and study skills moderately predict students’ academic achievement. Annamalai and Muniandy (2013) suggested that academic performance is based on students’ reading habits. Whitten et al. (2016) and Fatiloro et al. (2017) revealed that reading habits significantly help students learn more to enhance their academic performance. Ehsan and Sultana (2020) predicted that reading habits significantly improve students’ academic performance.
6. Conclusion
Reading habits and study skills differ in conceptual understanding. Reading habits are the degree to which readers regularly read, whereas study skills are the ability to comprehend new information effectively. Both reading and study habits influence students’ academic performance. It is concluded that the collected data were normally distributed. The descriptive findings about reading habit sub-constructs indicated that students give more preferences to reading than their attitude toward reading, interest in reading, and reading problems. Simultaneously, they have competent reading habits and study skills. Furthermore, it is found that there are moderate-to-strong positive correlations among reading habits, study skills, and academic achievement in English. Thus, it is concluded that reading habits and study skills directly correlate with academic achievement in English. In addition, it is also revealed that reading habits and study skills moderately predict students’ academic achievement.
7. Implications for practice
It is determined that students prefer reading to their attitude toward reading, interest in reading, and challenges and have competent reading habits and study skills. Thus, it is suggested that teachers plan such assignments and tasks based on reflective thinking ( Aslam et al., 2021 ), so students have to visit the school library to read more academic material to accomplish assigned tasks through extensive reading. Students’ reading habits and study skills have a moderate-to-strong connection with their academic achievement in English. So school administrations design a timetable by consulting with teachers, allowing students to spend at least an hour in the library regularly. In contrast, the library should have up-to-date reading material, exciting storybooks, and stock which attract students. In addition, parents can also engage their children in constant reading at home by providing related textbook materials and allowing them to watch educational television programs to gain the essence of reading habits and study skills.
8. Limitations and implications for future research
There are several limitations to this study. First, this study was conducted on secondary school students by selecting a sample from the Lahore district of Punjab, Pakistan. Therefore, future studies may include participants from other districts of Punjab and other provinces of Pakistan to increase the generalizability of results. Second, longitudinal studies are needed to explore the change in students reading habits and study skills over time. To enhance reading habits and study skills, interventional studies may build lifelong reading habits and study skills among learners to make a scholarly society. Last but not least, future researchers may explore parent’s role in developing their children’s reading habits and study skills by selecting participants from diverse populations. Cultural factors would affect students’ reading habits; thus, Pakistan’s unique culture should be considered a potential theoretical explanation in future.
Data availability statement
The raw data supporting the conclusions of this article will be made available by the authors, without undue reservation.
Ethics statement
Ethical review and approval was not required for the study on human participants in accordance with the local legislation and institutional requirements. Written informed consent to participate in this study was provided by the participants or their legal guardian/next of kin.
Author contributions
NA presented the main idea and wrote the first draft of the manuscript. SA contributed to conducting the methodology. SA, AA, and TK were involved with the revisions and proofreading. All authors contributed to the article revisions and approved the submitted version.
Acknowledgments
The authors would like to thank the Deanship of Scientific Research at Umm Al-Qura University for supporting this study by Grant Code: 22UQU4280253DSR01.
Conflict of interest
The authors declare that the research was conducted in the absence of any commercial or financial relationships that could be construed as a potential conflict of interest.
Publisher’s note
All claims expressed in this article are solely those of the authors and do not necessarily represent those of their affiliated organizations, or those of the publisher, the editors and the reviewers. Any product that may be evaluated in this article, or claim that may be made by its manufacturer, is not guaranteed or endorsed by the publisher.
Abid, N., Ali, R., and Akhter, M. (2021). Exploring gender-based difference towards academic enablers scales among secondary school students of Pakistan. Psychol. Sch. 58, 1380–1398. doi: 10.1002/pits.22538
CrossRef Full Text | Google Scholar
Adigun, I. O., Oyewusi, F. O., and Aramide, K. A. (2021). The impact of Covid-19 pandemic lockdown on reading engagement of selected secondary school students in Nigeria. Interdiscip. J. Educ. Res. 3, 45–55. doi: 10.51986/ijer-2021.vol3.01.05
Ajzen, I. (1991). The theory of planned behavior. Organ. Behav. Hum. Decis. Process. 50, 179–211. doi: 10.1016/0749-5978(91)90020-T
Akoglu, H. (2018). User’s guide to correlation coefficients. Turkish J. Emerg. Med. 18, 91–93. doi: 10.1016/j.tjem.2018.08.001
PubMed Abstract | CrossRef Full Text | Google Scholar
Albers, M. J. (2017). Introduction to quantitative data analysis in the behavioral and social sciences. Hoboken, NJ: John Wiley & Sons, doi: 10.1002/9781119290384
Al-Jarf, R. (2021). Collaborative mobile ebook reading for struggling EFL college readers. IOSR J. Res. Method Educ. 11, 32–42.
Google Scholar
Al-Jarf, R. (2019). Quality in teaching reading to high school students. Eurasian Arabic Stud. 5, 36–62.
Alnahdi, A. S., and Aftab, M. (2020). Academic stress, study habits and academic achievement among university students in Jeddah[Special Issue]. Int. J. Psychosoc. Rehabil. 24, 97–104. doi: 10.37200/IJPR/V24SP1/PR201138
Alzahrani, S. S., Soo Park, Y., and Tekian, A. (2018). Study habits and academic achievement among medical students: A comparison between male and female subjects. Med. Teach. 40, 1–9. doi: 10.1080/0142159X.2018.1464650
Ameyaw, S. K., and Anto, S. K. (2018). Read or perish: Reading habits among students and its effect on academic performance: A case study of eastbank senior high school-accra. Libr. Philos. Pract. 1–23. Available online at: https://digitalcommons.unl.edu/libphilprac/1748
Ameyaw, S., and Anto, S. K. (2019). Gender variation in reading habits in schools in Moland: A case study of Asantekwaa SDA Junior High School. Eur. J. Educ. Stud. 6, 250–264. doi: 10.5281/zenodo.3473923
Annamalai, S., and Muniandy, B. (2013). Reading habit and attitude among Malaysian polytechnic students. Int. Online J. Educ. Sci. 5, 32–41.
Anthony, C. J., and DiPerna, J. C. (2018). Piloting a short form of the academic competence evaluation scales. Sch. Ment. Health 10, 314–321. doi: 10.1007/s12310-018-9254-7
Aslam, S., Hali, A. U., Zhang, B. H., and Saleem, A. (2021). The Teacher Education Program’s Impact on Preservice Teachers’ Reflective Thinking in Pakistan. Thousand Oaks, CA: Sage Open, doi: 10.1177/21582440211055724
Awang, M., and Sinnadurai, S. K. (2011). A study on the development of strategic tools in study orientation skills towards achieving academic excellence. J. Lang. Teach. Res. 2, 60–67. doi: 10.4304/jltr.2.1.60-67
Baffoe, G. A., and Okae-Anti, A. (2020). Reading habits of selected communication educators in Ghana. J. Educ. Pract. 11, 45–51.
Bajwa, N., Gujjar, A., Shaheen, G., and Ramzan, M. (2011). A comparative study of the study habits of the students from formal and non-formal systems of education in Pakistan. Int. J. Bus. Soc. Sci. 2, 175–186.
Balan, S., Katenga, J. E., and Simon, A. (2019). Reading habits and their influence on academic achievement among students at Asia pacific international university. Abstr. Proc. Int. Scholars Conf. 7, 1490–1516. doi: 10.35974/isc.v7i1.928
Bano, J., Jabeen, Z., and Qutoshi, S. B. (2018). Perceptions of teachers about the role of parents in developing reading habits of children to improve their academic performance in schools. J. Educ. Educ. Dev. 5, 42–59. doi: 10.22555/joeed.v5i1.1445
Baron, N. S. (2017). Reading in a digital age. Phi Delta Kappan 99, 15–20. doi: 10.1177/003172171773418
Bashir, I., and Mattoo, N. H. (2012). A study on study habits and academic performance among adolescents (14-19) years. Int. J. Soc. Sci. Tomorrow 1, 1–5.
Bhan, K. S., and Gupta, R. (2010). Study habits and academic achievement among the students belonging to scheduled caste and non-scheduled caste group. J. Appl. Res. Educ. 15, 1–9.
Bibi, A., Naseer, N., and Habib, Z. (2020). Study habits of students and academic achievement: A correlational study. Glob. Educ. Stud. Rev. 5, 114–122. doi: 10.31703/gesr.2020(V-III).12
Biyik, M. A., Erdogan, T., and Yildiz, M. (2017). The examining reading motivation of primary students in the terms of some variables. Int. J. Prog. Educ. 13, 31–49.
Chotitham, S., and Wongwanich, S. (2014). The reading attitude measurement for enhancing elementary school students’ achievement. Procedia Soc. Behav. Sci. 116, 3213–3217. doi: 10.1016/j.sbspro.2014.01.737
Cohen, L., Manion, L., and Morrison, K. (2018). Research methods in education , 8th Edn. Milton Park: Routledge.
Creswell, J. W., and Clark, V. L. P. (2017). Designing and conducting mixed methods research. Thousand Oaks, CA: Sage.
Dadzie, P. S. (2008). Reading for education: The roles of libraries. Ghana Libr. J. 20, 1–14. doi: 10.4314/glj.v20i1.33978
Davarci, N. (2013). An investigation on the evaluation of the relationship between the reading habits of 8th grade elementary school students and their habits of computer-internet usage [Unpublished master thesis]. Nigeria: University of Nigeria.
Demir, S., Kilinc, M., and Dogan, A. (2012). The effect of curriculum for developing efficient studying skills on academic achievements and studying skills of learners. Int. Electron. J. Elem. Educ. 4, 427–440.
Dilshad, M., Adnan, A., and Akram, A. (2013). Gender differences in reading habits of university students: An evidence from Pakistan. Pak. J. Soc. Sci. (PJSS) 33, 311–320.
DiPerna, J. C. (2004). Structural and concurrent validity evidence for the academic competence evaluation scales-college edition. J. Coll. Couns. 7, 64–72. doi: 10.1002/j.2161-1882.2004.tb00260.x
DiPerna, J. C. (2006). Academic enablers and student achievement: Implications for assessment and intervention services in the schools. Psychol. Sch. 43, 7–17. doi: 10.1002/pits.20125
DiPerna, J. C., and Elliott, S. N. (2000). Academic competence evaluation scales. Psychol. Corp. doi: 10.1037/t14965-000
Dollah, W. A. K. W., Fakeh, S. S. K. W., Kamal Rafedzi, E. R., Ibrahim, A., Rahim, H., Masron, M. Z. A., et al. (2017). Inculcating reading habits among secondary school students. J. Sci. Eng. Res. 4, 407–421.
Dolmaz, M., and Kaya, E. (2019). The effect of 7th grade students’ reading habits and their academic achievement in social studies and Turkish courses on their creative writing skills. Int. Online J. Educ. Sci. 11, 168–183. doi: 10.15345/iojes.2019.01.012
Duncan, G. J., Dowsett, C. J., Claessens, A., Magnuson, K., Huston, A. C., Klebanov, P., et al. (2007). School readiness and later achievement. Dev. Psychol. 43, 1428–1446. doi: 10.1037/0012-1649.43.6.1428
Duncan, T. G., and McKeachie, W. J. (2005). The making of the motivated strategies for learning questionnaire. Educ. Psychol. 40, 117–128. doi: 10.1207/s15326985ep4002_6
DuPaul, G. J., and Stoner, G. (2014). ADHD in the schools: Assessment and intervention strategies , 3rd Edn. New York, NY: Guilford Press.
Eccles, J. S., and Wigfield, A. (2002). Motivational beliefs, values, and goals. Ann. Rev. Psychol. 53, 109–132. doi: 10.1146/annurev.psych.53.100901.135153
Ehsan, T., and Sultana, N. (2020). Predicting the role of study habits in academic achievement: A study of university students in Punjab. Pak. J. Educ. 37, 95–112. doi: 10.30971/pje.v37i1.1410
Erdem, A. (2015). A research on reading habits of university students: Sample of Ankara University and Erciyes University. Procedia Soc. Behav. Sci. 174, 3983–3990. doi: 10.1016/j.sbspro.2015.01.1145
Erguvan, D. (2016). ’Students’ attitudes towards extensive and intensive reading and ‘instructors’ motivational strategies. Arab World Eng. J. (AWEJ) 7, 136–150.
Farrington, C. A., Roderick, M., Allensworth, E., Nagaoka, J., Keyes, T. S., Johnson, D. W., et al. (2012). Teaching adolescents to become learners. The role of noncognitive factors in shaping school performance: A critical literature review. Chicago, IL: University of Chicago Consortium on Chicago School Research.
Fatiloro, O. F., Adesola, O. A., Hameed, B. A., and Adewumi, O. M. (2017). A survey on the reading habits among colleges of education students in the information age. J. Educ. Pract. 8, 106–110.
Fazal, S., Hussain, S., Majoka, M. I., and Masood, S. (2012). The role of study skills in academic achievement of students: A closer focus on gender. Pak. J. Psychol. Res. 27, 37–51.
Fischer, L., Hilton, J., Robinson, T. J., and Wiley, D. A. (2015). A multi-institutional study of the impact of open textbook adoption on the learning outcomes of post-secondary students. J. Comput. High. Educ. 27, 159–172. doi: 10.1007/s12528-015-9101-x
George, D. (2011). SPSS for Windows step by step: A simple study guide and reference, 17.0 update , 10th Edn. London: Pearson Education.
Goel, U. (2014). Comparative study of study habits in relation to academic achievement of senior secondary school students. Gyanodaya J. Prog. Educ. 7, 18–25. doi: 10.5958/2229-4422.2014.00004.8
Gormley, M. J., Pinho, T., Pollack, B., Puzino, K., Franklin, M. K., Busch, C., et al. (2018). Impact of study skills and parent education on first-year GPA among college students with and without ADHD: A moderated mediation model. J. Atten. Disord. 22, 334–348. doi: 10.1177/1087054715594422
Government of Punjab [GOP] (2019). Census of school education department. Lahore: Government of Punjab.
Habibu, I., and Ejembi, S. (2011). The role of schools and public libraries in promoting reading habit among children and adolescents in Nigeria. Inf. Knowl. Manag. 1, 33-40.
Haliru, R. A., Abdulkarim, M., Mohammed, A. D., and Dangani, B. U. (2015). An assessment of reading habit among secondary school students in Kaduna metropolis. J. Hum. Soc. Sci. 20, 12–17.
Hassan, I., Latiff Azmi, M. N., Muhamad, S. N., and Abdullah, A. T. H. (2021). Reading habits and their correlation with reading achievement among ESL learners in selected Malaysian secondary schools. Arab World Eng. J. (AWEJ) 12, 385–399. doi: 10.24093/awej/vol12no3.27
Hassanbeigi, A., Askari, J., Nakhjavani, M., Shirkhoda, S., Barzegar, K., Mozayyan, M. R., et al. (2011). The relationship between study skills and academic performance of university students. Procedia Soc. Behav. Sci. 30, 1416–1424. doi: 10.1016/j.sbspro.2011.10.276
He, M. (2014). “Extensive reading and students’ academic achievement: A case study,” in Exploring EFL fluency in Asia , eds T. Muller, J. Adamson, P. S. Brown, and S. Herder (Berlin: Springer), 231–243. doi: 10.1057/9781137449405_14
Horbec, D. (2012). The link between reading and academic success. Eng. Aust. 47, 58–67.
Hussain, I., and Munshi, P. (2011). Identifying reading preferences of secondary school students. Creat. Educ. 2, 429–434. doi: 10.4236/ce.2011.25062
Iheakanwa, J. U., Obro, S., and Akpochafo, W. P. (2021). Reading ability, study habits and students’ academic performance in social studies. Libr. Philos. Pract. 1–21. Available online at: https://digitalcommons.unl.edu/libphilprac/5675
Issa, A. O., Aliyu, M. B., Akangbe, R. B., and Adedeji, A. F. (2012). Reading interests and habits of the federal polytechnic, OFFA, students. Int. J. Learn. Dev. 2, 470–486. doi: 10.5296/ijld.v2i1.1470
Kettler, R. J., Elliott, S. N., DiPerna, J. C., Bolt, D. M., Reiser, D., and Resurreccion, L. (2014). Student and teacher ratings of academic competence: An examination of cross-informant agreement. J. Appl. Sch. Psychol. 30, 338–354. doi: 10.1080/15377903.2014.950442
Krashen, S. (2016). “Compelling reading and problem-solving: The easy way (and the only way) to high levels of language, literacy, and life competence,” in Proceedings of the epoch making in english language teaching and learning twenty-fifth international symposium on english teaching, english teachers , Taipei, 115–125.
Kulatunga, R. K. (2016). A study on understanding the reading habits and library usage of under graduate students (2007/2008 batch) of Uva Wellassa University of Sri Lanka. [Unpublished doctoral dissertation]. Badulla: Uva Wellassa University of Sri Lanka.
Kutay, V. (2014). A survey of the reading habits of Turkish high school students and an examination of the efforts to encourage them to read [Doctoral dissertation]. Loughborough: Loughborough University.
Kuterbach, J. M. (2012). A model of academic enablers and academic performance among postsecondary learners [Unpublished doctoral dissertation]. Pennsylvania: Penn State University.
Lawrence, A. S. (2014). Relationship between study habits and academic achievement of higher secondary school students. Online Submission 4, 143–145. doi: 10.15373/2249555X/June2014/43
Loan, F. A., and Shah, R. (2017). Survey of the literature reading habits and preferences of adolescents: A study of a public school in India. Libr. Inform. Sci. Res. Electron. J. 27, 80–96.
Madhavi, S., Naidu, S., Krishnaveni, A., and Kiran, P. (2014). Study skills assessment among medical undergraduates-where they stand. J. Dent. Med. Sci. 13, 16–19. doi: 10.9790/0853-131031619
Maiyo, J., and Siahi, E. A. (2015). Study of the relationship between study habits and academic achievement of students: A case of Spicer Higher Secondary School, India. Int. J. Educ. Admin. Pol. Stud. 7, 134–141. doi: 10.5897/IJEAPS2015.0404
Malik, M., and Parveen, N. (2016). Study habits and academic achievement: A comparative analysis of the high and low academic achievers. Bahria J. Prof. Psychol. 15, 46–54.
Mansor, A. N., Rasul, M. S., Abd Rauf, R. A., and Koh, B. L. (2013). Developing and sustaining reading habit among teenagers. Asia Pac. Educ. Res. 22, 357–365. doi: 10.1007/s40299-012-0017-1
Miesen, H. W. J. M. (2003). Predicting and explaining literary reading: An application of the theory of planned behavior. Poetics 31, 189–212. doi: 10.1016/S0304-422X(03)00030-5
Mirza, Q., Pathan, H., Khatoon, S., and Hassan, A. (2021). Digital age and reading habits: Empirical evidence from Pakistani Engineering University. TESOL Int. J. 16, 210–231.
Mishra, P., Pandey, C. M., Singh, U., Gupta, A., Sahu, C., and Keshri, A. (2019). Descriptive statistics and normality tests for statistical data. Ann. Card. Anaesth. 22, 67–72. doi: 10.4103/aca.ACA_157_18
Molotja, T. W., and Themane, M. (2018). Enhancing learners’ reading habits through reading bags at secondary schools. Read. Writ. 9, 1–9. doi: 10.4102/rw.v9i1.185
Myers, M. D. (2019). Qualitative research in business and management. Thousand Oaks, CA: Sage.
Naqvi, S., Chikwa, G., Menon, U., and Al Kharusi, D. (2018). Study skills assessment among undergraduate students at a private university college in Oman. Mediterr. J. Soc. Sci. 9, 139–147. doi: 10.2478/mjss-2018-0034
Nguyen Thi Thu, H. (2022). The effects of reading habits on writing performance: A case study at Van Lang University. Int. J. TESOL Educ. 2, 105–133. doi: 10.54855/ijte.22247
Nouhi, E., Shakoori, A., and Nakhei, N. (2009). Study habits and skills, and academic achievement of students in Kerman University of medical sciences. J. Med. Educ. 12, 77–80. doi: 10.22037/jme.v1213,4.1249
Ogeyik, M. C., and Akyay, E. (2009). Investigating reading habits and preferences of student teachers at foreign language departments. Int. J. Lang. Soc. Cult. 28, 72–78.
Owusu-Acheaw, M., and Larson, A. G. (2014). Reading habits among students and its effect on academic performance: A study of students of Koforidua polytechnic. Libr. Philos. Pract. 1, 1–22.
Oyewole, O. (2017). Impact of poor reading culture among selected secondary school students in Owo local government area of Ondo state, Nigeria. Dev. Country Stud. 7, 88–101.
Palani, K. K. (2012). Promoting reading habits and creating a literate society. J. Arts Sci. Commerce 3, 90–94.
Pehlivan, A., Serin, O., and Serin, N. B. (2010). Determining reading interests and habits of candidate teachers (TRNC Sample). Procedia Soc. Behav. Sci. 9, 869–873. doi: 10.1016/j.sbspro.2010.12.251
Pillai, S. K. (2012). An empirical study on study habits of X standard students in Nagarkovil district. Res. Expo Int. Multidiscip. Res. J. 2, 15–27.
Pintrich, P. R., Smith, D. A., Garcia, T., and McKeachie, W. J. (1993). Reliability and predictive validity of the Motivated Strategies for Learning Questionnaire (MSLQ). Educ. Psychol. Meas. 53, 801–813. doi: 10.1177/0013164493053003024
Porkaew, K., and Fongpaiboon, A. (2018). Effects of extensive reading on Thai tertiary ‘students’ reading attitudes. Arab World Eng. J. (AWEJ) 9, 207–219. doi: 10.24093/awej/vol9no1.15
Pretorius, E. J., and Klapwijk, N. M. (2016). Reading comprehension in South African schools: Are teachers getting it, and getting it right? Per Linguam 32, 1–20. doi: 10.5785/32-1-627
Quadir, B., and Chen, N. S. (2015). The effects of reading and writing habits on learning performance in a blog learning environment. Asia Pac. Educ. Res. 24, 635–644. doi: 10.1007/s40299-014-0210-5
Rasheed, S. (2012). ’ Children’s reading habits: A study of Lahore city. Pak. J. Inform. Manag. Libr. 5, 412–437.
Rosli, N. A., Razali, N. F., Zamil, Z. U. A., Noor, S. N. F. M., and Baharuddin, M. F. (2018). The determination of reading habits among students: A concept. Int. J. Acad. Res. Bus. Soc. Sci. 7, 791–798. doi: 10.6007/IJARBSS/v7-i12/3710
Rozalski, M. E. (2008). Practice, practice, practice: How to improve students’ study skills. Beyond Behav. 17, 17–23.
Sabbah, S. (2016). The effect of study habits on English language achievement. Arab World Eng. J. (AWEJ) 7, 238–257.
Schober, P., Boer, C., and Schwarte, L. A. (2018). Correlation coefficients: appropriate use and interpretation. Anesth. Analg. 126, 1763–1768. doi: 10.1213/ANE.0000000000002864
Schwabe, F., McElvany, N., and Trendtel, M. (2015). The school age gender gap in reading achievement: Examining the influences of item format and intrinsic reading motivation. Read. Res. Q. 50, 219–232. doi: 10.1002/rrq.92
Shahidi, F., Dowlatkhah, H. R., Avand, A., Musavi, S. R., and Mohammadi, E. (2014). A study on the quality of study skills of newly-admitted students of Fasa University of Medical Sciences. J. Adv. Med. Educ. Prof. 2, 45–50.
Sherafat, R., and Murthy, C. V. (2016). A study of study habits and academic achievement among secondary and senior secondary school students of Mysore city. Int. J. Indian Psychol. 3, 161–170. doi: 10.25215/0302.055
Silverrajoo, P., and Hassan, A. (2018). Relationship between study habits and academic achievement among health science students. Int. J. Acad. Res. Bus. Soc. Sci. 8, 763–780. doi: 10.6007/IJARBSS/v8-i7/4418
Singh, Y. G. (2011). Academic achievement and study habits of higher secondary students. Int. Ref. Res. J. 3, 27–42.
Stokmans, M. J. W. (1999). Reading attitude and its effect on leisure time reading. Poetics 26, 245–261. doi: 10.1016/S0304-422X(99)00005-4
Strunk, T. A. (2014). An exploration of the relationships between academic enablers and middle school achievement [Unpublished doctoral dissertation]. Pennsylvania: Penn State University.
Tahamtani, T., Jalil, K., Hosseini, M., and SoltaniArabshahi, K. (2017). Correlation of study habits with academic achievement among students attending the national medical science Olympiad. J. Adv. Med. Educ. 3, 19–23.
Thamarasseri, I. (2018). Cognitive styles, study habits and academic achievement of students of Central University of Kashmir. Stud. Home and Commun. Sci. 12, 9–20. doi: 10.31901/24566780.2018/12.1-2.335
Tonka, H., and Bakir, S. (2020). The examination of the relationship between the secondary school students’ habit of reading and their reading anxiety. J. Educ. Issues 6, 293–313. doi: 10.5296/jei.v6i1.16986
Van Schooten, E., De Glopper, K., and Stoel, R. D. (2004). Development of attitude toward reading adolescent literature and literary reading behavior. Poetics 32, 343–386. doi: 10.1016/j.poetic.2004.07.001
Walia, P. K., and Sinha, N. (2014). Changing trend in reading habits of teenagers in Delhi: An impact assessment of demographic and environmental variables. Libr Rev. 63, 125–137. doi: 10.1108/LR-03-2013-0038
Wernersbach, B. M., Crowley, S. L., Bates, S. C., and Rosenthal, C. (2014). Study skills course impact on academic self-efficacy. J. Dev. Educ. 37, 14–33.
Whitten, C., Labby, S., and Sullivan, S. L. (2016). The impact of pleasure reading on academic success. J. Multidiscip. Grad. Res. 2, 48–64.
Winne, P. H. (2013). “Learning strategies, study skills, and self-regulated learning in postsecondary education,” in Higher education: Handbook of theory and research , ed. M. Paulsen (Berlin: Springer), 377–403. doi: 10.1007/978-94-007-5836-0_8
Wu, L., Valcke, M., and Van Keer, H. (2019). Factors associated with reading comprehension of secondary school students. Educ. Sci. Theory Pract. 19, 34–47. doi: 10.12738/estp.2019.4.003
Keywords : reading, reading habits, study skills, academic achievement, secondary school students
Citation: Abid N, Aslam S, Alghamdi AA and Kumar T (2023) Relationships among students’ reading habits, study skills, and academic achievement in English at the secondary level. Front. Psychol. 14:1020269. doi: 10.3389/fpsyg.2023.1020269
Received: 16 August 2022; Accepted: 09 January 2023; Published: 27 January 2023.
Reviewed by:
Copyright © 2023 Abid, Aslam, Alghamdi and Kumar. This is an open-access article distributed under the terms of the Creative Commons Attribution License (CC BY) . The use, distribution or reproduction in other forums is permitted, provided the original author(s) and the copyright owner(s) are credited and that the original publication in this journal is cited, in accordance with accepted academic practice. No use, distribution or reproduction is permitted which does not comply with these terms.

Disclaimer: All claims expressed in this article are solely those of the authors and do not necessarily represent those of their affiliated organizations, or those of the publisher, the editors and the reviewers. Any product that may be evaluated in this article or claim that may be made by its manufacturer is not guaranteed or endorsed by the publisher.
- Open access
- Published: 01 February 2017
Examining study habits in undergraduate STEM courses from a situative perspective
- Matthew T. Hora 1 &
- Amanda K. Oleson 2
International Journal of STEM Education volume 4 , Article number: 1 ( 2017 ) Cite this article
42k Accesses
32 Citations
16 Altmetric
Metrics details
A growing body of research in cognitive psychology and education research is illuminating which study strategies are effective for optimal learning, but little descriptive research focuses on how undergraduate students in STEM courses actually study in real-world settings. Using a practice-based approach informed by situated cognition theory, we analyzed data from 61 STEM students about their study habits.
Results indicate that studying is a multi-faceted process that is initiated by instructor- or self-generated cues, followed by marshaling resources and managing distractions, and then implementing study behaviors that include selecting a social setting and specific strategies. Variations in some study behaviors are also evident according to the timing of their studying (e.g., cramming), course level, discipline, and social setting. Three cases of individual student practices reveal how studying is also shaped by how the course is designed and taught, students’ own beliefs about studying, and aspects of their personal lives.
Conclusions
The results indicate that studying involves various social, digital, and curricular resources, that many students persist in utilizing low-impact study strategies (e.g., re-reading text), and that the use of study strategies varies across different situations. We suggest that the focus on changing teaching behaviors that is dominant within STEM education be broadened to include a focus on instructional design that supports student self-regulatory behaviors and the adoption of high-impact study strategies.
Graphical abstract
The stages of studying across three cases: Brianna, Larry, and Angelica.
As concerns mount regarding the quality of undergraduate education, particularly in the science, technology, engineering, and mathematics (STEM) fields, policymakers, educators, and student affairs professionals are increasingly focusing on how to support student learning throughout their academic careers. Given that students’ academic success is shaped by a complex matrix of psychological, cultural, and organizational factors, scholars are investigating a variety of issues that may impact student success including underlying psychological attributes such as engagement (Carini et al. 2006 ) and perseverance or “grit” (Duckworth et al. 2007 ), what instructors believe about teaching and learning (Hativa and Goodyear 2002 ), and the types of teaching methods used in the classroom (Freeman et al. 2014 ). However, while these areas of research shed light on key aspects of student learning, these foci overlook a key piece of the student learning puzzle—what students actually do when they leave the classroom and study.
A considerable body of literature exists on college student study skills and habits, with foci on students’ cognitive styles and approaches to learning (Biggs 1987 ; Riding and Cheema 1991 ), the use of specific study techniques (Karpicke et al. 2009 ) and the role of study habits and time spent studying on overall student achievement (Nonis and Hudson 2010 ; Robbins et al 2004 ). Investigating the nature of study habits is important because factors related to studying such as motivation and specific study techniques have been linked to academic success. In a meta-analysis of 72, 431 students, Credé and Kuncel ( 2008 ) found that motivation and study skills (e.g., time management) were positively associated with grade point average and grades in individual courses. Furthermore, a comprehensive review of research on specific study strategies found that some (e.g., distributed practice) led to learning gains whereas others (e.g., re-reading text) did not (Dunlosky et al. 2013 ) and that many college students are not employing these study habits (Hartwig and Dunlosky 2012 ), and understanding why students persist in using ineffective study practices and how to change this state of affairs, from a situative perspective, is of particular importance to the field of STEM education. Thus, knowing whether or not (and why) students are using these practices is important information for instructors and student affairs/academic advising professionals.
Yet for the field of postsecondary education in general, and STEM education in particular, relatively little is known about student study habits, largely due to the lack of robust descriptive research that accounts for students’ behaviors in real-world settings. The gaps in the literature are twofold. First, much of the research on studying is based on survey research or experimental studies of specific study strategies, with few qualitative, descriptive studies of how students actually study in real-world situations. Such an approach to research, that focuses on descriptive accounts of naturalistic behaviors in order to inform educational programming and reforms, is becoming increasingly important in research on reform implementation in both K-12 and postsecondary contexts (Hora 2016 ; Coburn and Turner 2012 ; Spillane et al. 2002 ). Second is view of study habits as decontextualized, not shaped by social, curricular, situation; given insights from situated cognition research on how activity and learning itself is “distributed, stretched over (and) not divided among” mind, tools, and social and organizational contexts (Lave 1988 , p. 1), and that decision-making and behavior cannot be properly understood without close attention to the naturalistic settings in which they unfold (Klein 2008 ), the reliance on decontextualized survey research for insights into study habits is no longer tenable.
In this exploratory study, we utilize a practice-based approach to focus on the actual study behaviors of 61 undergraduates at three research universities in the USA and Canada who were enrolled in biology, physics, earth science, and mechanical engineering courses. Drawing upon situated cognition theory to conceptualize studying as a behavior that encompasses individual study strategies as they unfold in specific social, technological, and institutional contexts, we analyze data using inductive thematic analysis from 22 focus groups, and these students provided detailed information about their study habits that allowed us to answer the following research questions: (1) What behaviors do students taking undergraduate STEM courses engage in when studying? (2) What underlying contextual factors, if any, influence these behaviors?
We pursued this line of research because while the question “How can we teach students if we do not know how they learn?” (Coffield et al. 2004 , p. 1) is important, we also wonder “How can we best support student success if we do not understand how they study?” Insights gleaned from the data presented in this paper, which indicate that studying is a complex, multi-dimensional practice that implicates cues, social resources, artifacts, and study strategies, can provide faculty and student affairs professionals with a new way to think about studying that extends the prior focus on specific, decontextualized study strategies.
Discussions regarding the state of undergraduate education in the early twenty-first century often focus on the role of the instructor and their pedagogical acumen in the classroom (e.g., Bok 2009 ). Indeed, much of the focus in the STEM education literature is on how to affect changes in faculty teaching practices and philosophies about student learning (PCAST 2012 ). While instructors certainly play an important role in facilitating student learning by crafting experiences that engage students in these ways (or not), researchers have long questioned whether enough attention has been placed on the other actor involved in the learning enterprise—the student. As Entwistle and Tait ( 1990 , p. 170) observed, student behaviors are “part of a broader academic environment which affects learning probably as much as, if not more than, the classroom skills of the lecturer.” According to this view, the student as an agent actively engaged in his or her own learning and overall experience in college is a central, if not primary, part of the teaching and learning equation that is too often overlooked.
Psychological approaches to understanding study habits and academic success
In early research on the change processes that young people undergo while in college (Pascarella and Terenzini 2005 ) and the factors that contribute to students’ lack of persistence (Tinto 1993 ), higher education scholars have paid particularly close attention to the psychological factors that shape students’ experiences and ultimate success (or lack thereof). For instance, attributes associated with academic success such as involvement (Astin 1984 ) and engagement have been used to explain students’ relative success in their academic coursework (Carini et al. 2006 ). An underlying assumption in this literature is that students’ mental stances or psychological attributes play a major role in their academic outcomes and that higher education professionals should support them by facilitating higher degrees of involvement and engagement to increase their prospects for success.
Another line of inquiry has focused on subconscious psychological traits associated with student learning including cognitive styles and approaches to learning (see Coffield et al. 2004 for a review). For example, researchers have argued that people have stable cognitive styles or “typical or habitual mode(s) of problem solving, thinking, perceiving, and remembering” that shape how they think and learn (Riding and Cheema 1991 , p. 194). Another commonly used construct is that of approaches to studying, which refers to more elastic, changeable approaches and preferences that learners have for studying and learning (Entwistle and Tait 1990 ). Early work in this area argued for the existence of two distinct approaches to learning whose basic outlines persist to the present time: deep approaches to learning that involve searching for meaning and surface approaches that involve rote memorization (Marton and Säljö 1976 ; Biggs 1987 ). While such approaches to learning are theorized as being relatively stable within an individual, they can change over time with concerted effort. Furthermore, these psychological attributes should not be considered as operating independently from the context in which studying occurs (Ramsden 1979 ). In fact, early research in this area found that some students actively sought information in the environment (e.g., textbooks, lecture content) and then studied using what was called “cue-seeking” behavior, whereas others were more “cue-deaf” or worked to succeed without seeking hints about exams (Miller and Parlett 1974 ).
This focus on the origins of student’s motivation to initiate studying is similar to a long-standing line of inquiry that examines the degree to which learners are able and willing to assume control of their own learning process or what is known as self-regulated learning (Zimmerman and Schunk 2001 ). A self-regulated learner engages in a process of initiating the learning process on their own, setting goals, identifying appropriate strategies, and reflecting on his or her own task performance—all of which ultimately leads to a decision to enact changes in future behaviors or to maintain current practices (Cassidy 2011 ). Self-regulated learning is a particularly valuable idea in college student success, with empirical research in this area indicating that students who exhibit high degrees of self regulation have higher rates of academic achievement as measured by persistence and grades (Boekaerts and Corno 2005 ).
Research on study habits and skills
Another body of literature that examines studying focuses directly on the study habits and skills that students utilize during the act of studying itself. However, what at first glance may appear to be a straightforward, easily defined term is operationalized in a variety of ways in the literature. For instance, Robbins et al. ( 2004 , p. 276) define study skills as “activities necessary to organize and complete schoolwork tasks and to prepare for and take tests” and operationalize the construct using measures including time management, leadership skills, communication skills, and the un-defined category of “study skills and habits” (see also Credé and Kuncel 2008 ; Lotkowski et al. 2004 ). Other scholars have defined study habits in different ways, including the ability to concentrate, the scheduling of regular review sessions, and hours spend studying (Nonis and Hudson 2010 ). Conceptualizing study habits in terms of time spent studying is rather common, and a widely cited report by Babcock and Marks ( 2010 ) found that hours spent studying has declined from 24 h a week in 1961 to 14 h a week in 2003. In 2009, the picture was bleaker, with over half of freshmen who took the Your First College Year Survey and over half of seniors who took the College Senior Survey spending 10 h or less per week studying or doing homework (Ruiz et al. 2010 ; Franke et al. 2010 ).
While these studies capture important facets of studying and the role that they play in student achievement and persistence, the specific strategies and actions students actually engage in during their study sessions remain obscured. Providing more clarity on specific study habits, Karpicke et al. ( 2009 ) found that the preferred study strategy of 84% of the surveyed undergraduates was re-reading textbooks and lecture notes. Unfortunately, a study examining the utility of 10 learning techniques in the empirical literature found that habits such as these considered low utility in regard their impact on student learning, in contrast to high-utility techniques such as practice testing and distributed practice (i.e., taking tests over time), thus suggesting that many undergraduates utilize study habits that are ineffective (Dunlosky et al 2013 ).
Given the ubiquity of the Internet and digital media in many people’s lives, researchers are also investigating how these artifacts are being used as study aids. In one study exploring student utilization of digital and “traditional” resources, researchers found that 39 and 44% of students search Wikipedia and Google, respectively, if they need help with coursework, with only 36% seeking out a faculty member (Morgan et al. 2012 ). Similarly, a 2010 study of 36,950 undergraduates found that 33% used wikis, 24% used video-sharing websites, and 12% used blogging tools (Smith and Caruso 2010 ). Besides these more traditional digital media, including course websites hosted on institutional learning management systems, some argue that other tools that facilitate personalized learning (Dabbagh and Kitsantas 2012 ) and digitally mediated social learning via open Internet-based resources (Seely Brown and Adler 2008 ) are under-utilized in higher education. Researchers are also examining how digital media can inhibit studying, however, and Rosen et al. ( 2013 ) found middle-school, high-school, and undergraduate students were unable to remain on task for even 6 min before being tempted by Facebook or texting when studying at home.
However, the literature on study skills, strategies, and habits is limited by a tendency to reduce the complex and multi-faceted behaviors that comprise studying to metrics that cannot capture how and why students study (i.e., hours spent studying) or focus on strategies (e.g., re-reading) at the expense of other possible behaviors or choices students make. Perhaps the single largest limitation, however, is the lack of attention paid to the contexts within which students actually study. While some scholars have focused on the environmental contexts of studying (Kuo et al. 2004 ) and the interaction among study habits and social factors (Treisman 1992 ; Robbins et al 2004 ), few recent studies have attempted to describe studying behaviors as a multi-faceted process that includes not only study strategies but also how situations and resources are implicated in these practices.
This is important because educational practice, whether a group of undergraduates studying for a biology course or an administrator finalizing a budget, should not be thought of solely in terms of an individual making decisions in isolation, as the context of decision-making as well as tools and other artifacts utilized as part of the process is critically important. In studying the practices of principals in K-12 settings, for example, researchers have utilized theoretical frameworks from situated and distributed cognition which assert that the institutional context is not a mere backdrop for activity but is instead an integral feature of individual cognition and decision-making as well as task performance itself (Halverson 2003 ; Hora 2012 ; Spillane et al 2002 ). In this study, we draw upon these frameworks to conceptualize studying as the discrete behaviors of individuals (e.g., reviewing notes) as they unfold within specific contexts and that implicate particular artifacts and resources.
Why does the lack of descriptive research on student study habits that adopt a situative perspective matter? Because fine-grained descriptions of people’s behaviors in specific contexts and situations illuminates the specific steps people take when solving problems or performing tasks—information that can then be used by instructors and educational leaders to improve their practices and design more locally attuned interventions (Coburn and Turner 2012 ; Spillane et al. 2001 ). Educational researchers across the K-16 spectrum have argued that more practice-based research should be conducted on the various behaviors associated with teaching and learning, so that how and why educators and students make decisions in “the wild” of schools, colleges, and universities can illuminate barriers and supports to effective practice, rather than simply prescribing how people should think and act regardless of the situation (Bastedo 2012 ). With such a comprehensive and multi-faceted approach to describing studying, we set out to document the study habits of 61 undergraduates taking STEM courses as a corrective to the focus on both teaching and study strategies alone, in the hopes that such accounts could inform ways that educators can improve student learning and academic success.
Exploratory research is intended to examine poorly understood phenomena and generate new insights and hypotheses that can guide future research on the topic (Slavin 2002 ; Stebbins 2001 ). In this exploratory study, we examine the study habits of a group of STEM students, with a focus on describing the lived experiences and subjective interpretations of individuals and groups or what cultural anthropologists call an “emic” account of social life (Merriam 2014 ). The study took place at three large, public research universities in the USA and Canada that had similar undergraduate populations (approximately 25,000 students). These sites were selected due to the presence of instructional reform initiatives, which was a criterion for the larger study on STEM instructors’ data driven decision-making upon which this analysis is based. The disciplines included in this study are biology, geology, physics, and mechanical engineering based on the STEM-related focus of the larger study. For this study, a non-random purposive sampling procedure was used to identify faculty study participants. Faculty were included in the study population if they were listed as instructors in each institution’s course listings for the 2013 spring semester. We contacted 165 instructors via email requesting their participation in the study, and 59 participated (36% response rate). Thus, the faculty whose classes were recruited into the study were unique in that they were self-selected and taught undergraduate STEM courses at large research universities.
These instructors represented the initial pool of courses from which we selected student participants for the focus groups. We selected the focus group technique in order to collect a large amount of qualitative, in-depth data in a shorter amount of time than would be possible with individual interviews (Bernard 2011 ). Of the 59 faculty who participated in the larger study, we asked 30 instructors they would recruit students for participation in focus groups, of which 22 instructors agreed. The 30 courses (and instructors) selected for recruitment represented the largest courses across all four of the disciplines included in the study, which increased the prospects of recruiting sufficient numbers of students. Those instructors sent email requests to their classes, and students contacted the research team if they were interested in participation. There was a $20 incentive, and 61 students participated (see Table 1 ).
Data collection
A team of four researchers conducted the student focus group interviews using a semi-structured interview protocol, with each group led by one or two moderators depending upon scheduling constraints. The key question posed to participants in the focus groups was: “Please imagine for a moment how you typically study for this course —can you describe in as much detail as possible your study situation?” This question was followed by probes regarding the types of materials used for studying, whether participants studied alone or with others, and any additional details not yet described. While the open-ended nature of the questions resulted in detailed observations about study practices, it also led to idiosyncratic accounts that were not always comparable across individuals. We also did not provide a definition for the act of “studying” during the focus groups, which was based on our goal of capturing students’ own unique perceptions about what behaviors and situations constituted a study session. Each focus group included between two and six students and lasted approximately 45 min. These focus groups were audio recorded and transcribed.
Data analysis
Transcripts were entered into NVivo qualitative analysis software and then segmented into manageable units or discrete statements by participants that encapsulated a single thought or idea (Gee 1986 ). First, a code list was created to segment the data that aligned with the research questions guiding the analysis. We were interested in segments related to “study strategies” and “study situations,” and thus, any utterances pertaining to these two categories were sought out. Both analysts reviewed five transcripts with these two codes in mind and highlighted text fragments related to both codes and then met to ensure a common understanding of the relationship between the codes and the raw data. Upon ensuring that the codes were being applied similarly, the second author then segmented the remainder of the dataset. Second, we followed a structured approach to grounded theory that involved using a combination of a pre-existing “coding paradigm” and the inductive analysis of transcripts to develop a code list with which to analyze the entire dataset. The second author developed a preliminary code list using an inductive open-coding approach where terms or ideas mentioned by study participants themselves (e.g., re-reading textbooks) were used to create code names (Glaser and Strauss 1967 ) while the research questions and theoretical framework were also kept in mind (Strauss and Corbin 1990 ). After developing the initial code list, we met to discuss the codes and revised them while reviewing text fragments and discussing the applicability of codes to the data. During this process, we attempted to derive codes that maintained as much fidelity to participants’ own language and descriptions of study behaviors as possible.
The second author then developed the final code list using the constant comparative method, where each occurrence of a code was compared to each previous instance of that code in order to confirm or alter the code and/or its definition (Glaser and Strauss 1967 ), after which the final code list was applied to the entire dataset. At this point in the analytic process, qualitative researchers have the option of reporting recurrent themes with or without numeric counts of their prevalence. In this paper, where all study participants responded to questions in a similar fashion (e.g., specific study strategies), we elected to report the number of times a code was applied to the raw data in order to convey to readers the frequency with which a particular behavior or observation was identified in the data. In other cases where responses were more ambiguous and/or where different respondents interpreted questions differently, we report recurrent themes instead of numeric counts.
The data were also entered into a data matrix with subjects as rows and study cues, resources, and strategies as columns. These data were analyzed using exploratory data reduction methods (i.e., hierarchical cluster analysis and multi-dimensional scaling) to see if patterns across the data could be discerned. Clear patterns were not discernable, so these data were then organized to report the frequency with which particular strategies were used according to different groups of students (e.g., discipline, social situation). The results reported in this paper depict the percentage of students within each group reporting each strategy, with results weighted according to the size of each respective group.
Next, we analyzed two students and one entire focus group who provided particularly rich details about their study habits in order to depict how studying unfolds in real-world settings at the individual level. These subjects were selected because of the level of detail they provided when self-reporting their conceptions of what studying means, the contexts in which their studying occurred, and their actual study behaviors. These case studies also highlight the situated nature of studying in the influence of peers, curricular artifacts, and other features of the environment on their study habits. Finally, we examined the resulting themes to explore any patterns in the data and identified a new way of thinking about studying that is reported in this paper.
Limitations to this study include the self-selected nature of the sample, both of the participating instructors and students, that limits generalizability of the findings to broader populations of undergraduates. Such limitations to generalizability are an inherent part of research using small, non-randomly selected samples, but their strength is in illuminating behaviors at a fine-grained level. While future research involving larger samples will be necessary to assess how widespread the behaviors reported in this paper truly are, the data do raise questions and considerations about studying that can be applied to different institutions. Another limitation is that the focus group method may introduce an element of self-censoring and social desirability bias by participants due to the public nature of the setting, which can result in incomplete or inaccurate answers to the facilitator’s questions. Finally, because participants discussed their studying with varying degrees of specificity it was difficult at times to ascertain whether similar behaviors were being reported. The limitations associated with social desirability and the veracity of students’ accounts could not be overcome with the current study, though future work should consider incorporating an observational component to corroborate self-reported behaviors with actual practice.
Before reporting data addressing the research questions guiding the study, we first discuss how respondents had differing notions of what activities constituted “studying.” For some, it meant any exposure to course material such as attending a class, whereas for others, studying implied completing assigned tasks. In yet other cases studying referred to activities that were not assigned and took place outside of class. As one student said, “I see studying more as something that I do separate from any assigned material.” In addition to these task-oriented conceptions, some reported “folk” theories of the learning or ideas about phenomena that are not necessarily grounded in evidence. For example, one student stated, “Studying to me means stressing out your brain so that it realizes that the information is significant.”
Thus, for the students in this study, “studying” was not easily distilled into a set of discrete strategies such as re-reading the textbook or hours spent engaged in discrete strategies. Further, as we discuss below, students’ views of studying also implicate a variety of strategies, social and physical settings, and resources as being involved in the studying process (Greeno 1998 ; Halverson 2003 ; Robbins et al 2004 ). Future research should delve more deeply into what students consider to be studying in terms of its physical, artifactual, and temporal boundaries. To maintain a consistent definition for this analysis, however, we defined studying as any interaction with course material outside of the classroom.
Cues to initiate studying and timing of study strategies
Prior to engaging in particular study activities, students frequently discussed why they started studying, which centered on the core idea of “cues” that trigger study behaviors. These cues were either provided by the instructor or were internally generated. While students were not explicitly asked about what cued their study sessions, descriptions of the study processes for many students provided information for this analysis. Another important aspect of these preliminary stages of studying is when students choose to study—either throughout the semester, several days before an assessment, or the day before a test or exam (i.e., cramming).
Instructor-generated cues
Throughout a given semester, 40 students reported that instructors often provided cues regarding when and what they should study. The most important cue for students tended to be the announcement of an upcoming assessment, thus initiating the process of studying. For some, an impending assessment was the only reason for studying. Similarly, instructors’ discussions about assessments (e.g., topics that would be covered) served as a primary rationale for some students to attend class. One participant said, “I go to class to (hear) the professor say this week on the exam you will see this subject or that subject.” Consequently, for some students, the classroom becomes a venue in which cues pertaining to assessments are sought and then applied to their studying.
Self-generated cues
Fewer (four) participants also discussed another cue for studying, that of recognizing that they were not sufficiently prepared or familiar with the course material. One participant explained that he studied after realizing that he did not understand a concept, which then set in motion a series of study behaviors that lasted until he felt conversant with the material. He said, “…and then I realize, ‘Oh man, I don’t understand pulleys so well,’ so last week I studied pulleys until I understood them.” Others reported a strong desire to learn certain skills and material so that they could reach their career goals.
Next, we discuss findings regarding when students reported engaging in study activities. For 11 respondents studying took place several days before an exam or test, while 14 reported waiting until the last day or even night before, popularly known as “cramming.” While the literature indicates that cramming is an ineffective way to study (e.g., Kornell 2009 ), and some students recognize its limitations (e.g., one student reported that after cramming “[the information] is not still in my brain”), this mode of preparation remains a common method. Finally, 15 respondents discussed studying throughout the term. In some cases, this practice was instigated by course-specific factors such as an instructor’s use of weekly quizzes, whereas in others, the student established a regular schedule of studying on their own.
Marshaling resources for studying
After discussing cues and timing for studying, the respondents then discussed collecting and utilizing a variety of resources with which to study. In describing students’ use of resources, we included references to commonly used tools such as course websites and textbooks as well as human resources that learners draw upon when studying. This represents a broader view of resources within organizations than is commonly used but captures knowledge and capabilities of instructors and staff within an educational organization (Gamoran et al. 2003 ). Understanding the resources used during studying is important because digital, print, and human resources and tools are used to enhance or even shape the studying act itself.
The resources discussed by the respondents included digital tools and media, print resources, and human resources, and those most commonly reported are depicted in Table 2 .
Digital resources
While the most commonly reported digital tool included laptops or desktop computers, we focus here instead on the applications used by students on these now ubiquitous resources for college students. The most widely reported resource was the course website (27 students), which operated on various learning management system (LMS) platforms. These websites were developed by instructors who posted a variety of learning resources including videotaped lectures, readings, practice exams, and course syllabi. One student described her professor’s course website as such, “So basically like any way you learn you can find it on [course website name] through all her resources and find a good way to study for you.”
The next most widely used digital resource included websites for seeking out new information including Google (24) and Wikipedia (13). These websites helped students expand upon lecture notes or clarify concepts or steps in solving problems. For example, one participant noted that in lecture, he listened for key words that could be included on exams and then looked them up online, because “With the Internet and Wikipedia you just need to know a few keywords and you can learn about anything.” Other resources included Facebook (9) which was used as an organizing tool and Youtube (5) for informational purposes. These results support prior research that found college students regularly utilize these online resources, even more so than their own instructors (e.g., Morgan et al. 2012 ).
However, the evidence suggests that technology also acts as a disruptive force in some students’ study habits. Nineteen respondents reported that some digital resources, usually cell phones and Facebook, regularly disrupted their studying yet they had no strategy for managing these distractions. One student noted, “[When studying] I look up sports stuff, any excuse not to be studying….at a computer I can just click on whatever I want.” To mitigate the potential distractions of the Internet or a buzzing phone, 21 students reported having developed strategies for managing distractions, often by deliberately removing them from their study “space.” The optimal studying situation for one student was in an isolated cubicle in the library basement with no cell phone reception, and he would turn off his laptop’s wireless Internet signal. In another case, a student went to her parent’s house on the weekends for a self-imposed “no devices zone” where her phone was confiscated so she could concentrate. Thus, digital resources can both enhance and detract from an individual’s studying, and students have varying degrees of success when it comes to managing the detrimental aspect of digital devices and media.
Print-based resources
Another type of resource that respondents regularly used was print-based resources such as textbooks (34) and lecture notes (33). Lecture notes took many forms including notes taken by student in class as well as notes and/or PowerPoint slides provided by the instructor, both of which were reported as important resources for studying. Another less utilized print resource discussed by five respondents was cue cards, which were mostly used to memorize key facts and formulas.
Human resources
The last type of resource reported by students pertained to the knowledge and content-expertise of people within their courses and/or departments. These included instructors (8) as well as teaching assistants (8) and tutors (4). In some cases, the participants reported approaching instructors or teaching assistants outside of class to obtain assistance with homework, upcoming or previous exams, and challenging concepts or problems. For students who were especially struggling with the course, tutors provided expertise and one-on-one instruction that these students viewed as an especially important form of academic support.
Setting and strategies
Next, we report data that speak to the studying process itself, particularly with whom students study and the specific strategies they employ.
The social setting in which studying occurs
When describing their actual study sessions, the respondents noted whether or not they studied alone or with others. For 39 respondents, studying was often a solitary affair. Some students noted that studying alone was an explicit strategy to reduce distracting conversations with others, while others stated that it was simply a habit. In contrast, 35 students described studying in groups. In these cases, the respondents stated that group-based studying was useful because peers could provide new insights or solutions. However, because 24 students reported studying both alone and in groups, depending on the proximity to an exam or the nature of the assignment, it is clear that for some students in the sample, the social setting in which studying occurred was rather flexible and not a fixed criterion or preference.
Employing specific study strategies
The studying process next involves the selection of specific strategies or techniques. While the participants often described these strategies using imprecise or idiosyncratic terminology such that it was often not possible to align them with those discussed in the literature (e.g., Dunlosky et al. 2013 ), it was possible to identify several core strategies utilized by this group of undergraduate students. In this section, we elaborate on the most commonly referenced strategies (see Table 3 ).
Thirty-eight participants re-read or reviewed course material or notes taken in class. This strategy was discussed as both a general practice that took place throughout the term as well as an initial step in preparing for exams. For example, one participant said that he re-read all of his lecture notes before working with old test materials “to try to understand what the professor had said fully” before attempting to take practice tests.
Given the broad conception of studying used in this analysis (i.e., any interaction students have with course material outside of class), we include the strategy of “doing homework” which 25 participants reported. As one participant put it: “My method of studying is pretty much to do any homework or review questions…” Homework also provided a litmus test of understanding—one participant explained how he learned a lot in class, but it really became clear when he answered the homework questions correctly.
While students reported reviewing lecture notes from class, this particular strategy involved 22 students creating their own artifacts such as cue cards, consolidating notes from different sources (some instructor-provided, others self-procured) into one set of notes, and so on. For example, one participant explained, “I write myself notes and everything is in my notes, including the textbook material and the prof’s slides and what the prof said or the stuff I found in Wikipedia or everything.” Others created study aids (e.g., games or cheat sheets) that were used throughout the term for study sessions.
Twenty participants reported reading the textbook in some capacity, either in full or in part, either assigned or unassigned, or they consulted the book when confronted with unfamiliar material. Often, the participants did not specify if they were re-reading, reading it for the first time, or if they were skimming. Importantly, the depth with which students read textbooks appeared to vary based on their intentions. In one case, a student explained, “Sometimes I just go through the chapter we’re going to go through in class and I just read all the captions for the images (to prepare for the lecture) so I know what we’re going to talk about and then afterwards I’ll read through the chapter.” In most cases, however, students spoke more ambiguously about reading.
Nineteen participants reported working with test materials provided by the instructor or students who had previously taken the course. One participant reported her routine as taking practice exams in a simulated test-taking environment, followed by an item-by-item analysis of her performance. Another talked about reviewing tests from previous years and randomly selecting problems to complete for practice. In both cases, the materials provided the students with an opportunity to monitor their level of understanding (or lack thereof) while also becoming attuned to the test-maker’s approach.
Working on problems was a strategy reported by 17 participants. Although ambiguous, the specific nature of the term “problems” likely refers to mathematical or computational problems given that many of these participants were enrolled in science or engineering courses. As one participant said, “I just find every single practice problem that I can get my hands on and do it.”
Eleven participants reported working on a variety of questions while studying. In one class, students worked on study questions or short essay prompts that review that day’s lecture. Further, instead of relying on practice exams, one student in that class reported, “I’ve found the best way to do well on the test is not to do all of her practice exams, but do (the) study questions.” Others reported working on end-of-chapter questions and completing discussion questions as an effective study strategy.
Taking quizzes related to course material outside of class was another method of studying reported by 11 participants. Sometimes the instructor provided the quiz to test comprehension after a reading assignment. One student who takes bi-weekly extra-credit quizzes provided by her instructor said, “I take them pretty seriously, I’ll prep a little bit before them even though they’re only five questions and if I get something wrong I’ll read (about it).”
Other factors influencing the study process
In addition to specific cues, resources, and study strategies, respondents also discussed various situations or factors that influenced their study behaviors.
Role of instructor in providing resources for studying
Student’s use of resources during their studying depends, in part, on the instructor and his/her provision of particular resources within the course. For example, some instructors provided their students with a variety of modalities and tools for learning (e.g., podcasts, supplementary readings, online lecture notes) that other students might not have had access to in other courses or with other instructors. These can be offered as in-class resources, or more commonly, embedded within the course’s website or LMS. Students can then select from the resources made available by their instructors, as well as resources that they find on their own, to construct their own unique study situation.
Course characteristics and discipline
The participants described how disciplinary content and course structure also influenced the strategies and resources they used. Some students perceived that different disciplines required different approaches to studying. One participant said, “You can’t study math how you would study biology, right?” The student followed up this observation by describing how studying for a math course entailed doing numerous problem sets, while a biology course required extensive reading, memorization, and understanding laboratory assignments. Other course characteristics that influenced teaching were the assessments and teaching methods used in the course. For instance, one student explained how her approach to preparing for multiple-choice exams emphasized a surface knowledge of selected topics: “Instead of looking at a topic and being able to discuss it for paragraphs at a time in like an essay format, I’ll try to memorize details that I feel are important.” Another respondent student noted that his studying “tends to match the style of the class” so that in a class taught with PowerPoint slides, his studying entails “a lot of time looking at slides,” whereas a more interactive class involves focusing on concepts and hands-on activities. This student’s approach to studying suggests that an instructor’s teaching style may have consequences for student learning not only through in-class comprehension of material but also by sending messages to students regarding the best way to study.
Personal situations and dispositions
The participants also alluded to personal factors that influenced their studying such as the lack of time due to heavy course loads and/or work schedules, family situations, and health-related issues. Additionally, the participants brought to a course pre-existing dispositions and experiences that influenced their approach to studying. One of these pertains to historic study habits from high school, where some students attempted to alter their “old” study behaviors to fit with the “new” expectations and demands of the university, while others simply continued using what had worked for them previously. Finally, student’s personal reasons for taking a course (e.g., to satisfy degree requirement, curiosity) also shaped how participants approached their studying.
Patterns in cues, resources, and strategies
Next, we sought to explore whether or not patterns in the data existed in regard to how cues, resources, and strategies were inter-related or not. Preliminary analyses using exploratory data techniques did not reveal discernable patterns, and no clear links were evident across the three primary components of studying identified in the data (i.e., cues, resource use, strategies). Instead, we chose to examine patterns in the use of study strategies considered effective in the literature (e.g., Dunlosky et al. 2013 ) according to two aspects of study behaviors (i.e., study timing, social setting) and two variables related to subject characteristics (i.e., course level and discipline). All analyses include weighted averages.
First, when looking at when studying occurs according to three groups of students (i.e., less than 1 week prior, cramming, throughout term), some data points stand out (see Fig. 1 ). Crammers review notes more often than others (94%), while those studying less than 1 week prior to exams use more textbooks (73%), study questions (55%), and video (36%) than other groups and those studying throughout the term or semester use problem sets (53%) more often than others. These data indicate that some variation in study strategies is evident depending on when students choose to study.
Selected study strategies by timing of study practices and social setting
Second, when organizing the data according to two groups (i.e., studying alone or studying in groups) differences in study strategies are also evident (see Fig. 2 ). Note that some students reporting doing both, hence, the large numbers in both groups that do not sum to 60 (42 and 36, respectively). Students studying alone tend to review notes (64%) and textbooks (52%) and also do practice tests (24%) and quizzes (19%) more than those studying in groups. In contrast, those studying in groups create study artifacts (47%), do problems (31%) and questions (31%), and use online materials such as video (14%) and the Mastering Physics/Anatomy videos (19%).
Third, when the data are organized according to the discipline of the course students were enrolled in at the time of data collection, additional points of variation are evident (see Fig. 3 ). Again, students may or may not be majors in these fields but discussed their study habits in relation to these disciplines. Students taking biology courses (26) report reviewing notes (69%) and textbooks (46%), doing practice tests (31%) and questions (35%), and reviewing videos (27%) more than students taking courses in other fields. Students in physics courses (11) reported creating artifacts (64%), doing problem sets (82%), and mastering resources (45%) more than others. Mechanical engineers and geology students did not report any study strategies more than other groups.
Selected study strategies by discipline and course level
Finally, the data indicate that study habits vary by course level, with students in upper division courses (18) reporting using certain study strategies more than those in lower division courses (43), including practice tests (33%), questions (39%), video (28%), and mastering physics or anatomy resources (33%). The students in lower division courses reported reviewing notes (58%), creating artifacts (37%), reviewing textbooks (51%), and doing problems (42%) and quizzes (16%) more than the students in upper division courses (see Fig. 4 ).
Case examples
Finally, to illustrate how each of these sets of findings is evident in students’ own real-world experiences, we present three in-depth analyses of students’ actual studying practices. The first two cases are those of individual students—Larry and Brianna—whose study behaviors reflect different sequences of decisions that link particular cues, resource use, and strategies. The final case is that of a group of five students in a single course (i.e., upper division anatomy and physiology). These cases illustrate how studying is a complex, idiosyncratic practice, while also being shaped by the social, institutional, and technological milieu in which students operate.
Larry. When we spoke with Larry he was studying for an upper level biology course that was required for his major. He first talked about his personal view of what studying means, stating that:
Studying to me means stressing out your brain so that it realizes that the information is significant. Basically, your brain can be lazy when it doesn’t think that something’s important and stressing it is what makes it retain information. So studying for exams is a lot about stressing your brain out.
This theory of how the brain and learning work thus set the stage for Larry’s subsequent study habits, which was a process that began in the classroom. There, he “frantically” wrote on the instructor’s PowerPoint slides that he printed off before class, labeling images, drawing arrows, and identifying mechanisms for cell signaling that were being discussed in class. Larry said that he did not necessarily understand the concepts at the time but took the notes down to reference later. In fact, it is not until he finds the time to sit in the library and read the relevant sections of the textbook that “it all comes together and finally makes sense.” While reading, he writes down key terms and their definitions in a notebook. For Larry, the library represented an important resource in his education because he does not own the book because he cannot afford it. Thus, he spent a lot of time in the library reading one of two copies on reserve. He also attended the optional recitation section for the course where he was able to speak with the instructor one-on-one and earn extra credit.
While he tried to study throughout the term, with his demanding course load and work schedule, he often only had time to study 3 or 4 days prior to an exam. Describing his study habits as “messy” and comprised of “lots of big stages,” Larry first gathered his notes from classroom sessions and his review of the textbook and then made flashcards for key concepts from the course. He also completed the end-of-chapter quizzes in the text and reviewed (and retakes) any old quizzes or exams from the course. The day before the exam, he tells himself “Wow Larry, you really have to get to it now,” and he sequestered himself at the library to review his notes and difficult concepts in the textbook and to re-watch videotaped lectures from the course website. All along, he deliberately studied alone because he had to maximize the limited time available for studying, such that he “cannot afford to sit around and have people talk about other stuff.” After several hours in the library reviewing these materials, Larry generally felt ready for the exam. Altogether, in Larry’s case, studying is an act that is instigated by instructor’s cues (i.e., upcoming exam), informed by a folk theory of the mind, involves a variety of curricular resources, and is strongly influenced by his personal situation.
Brianna. Next, we consider the case of Brianna who was enrolled in a lower division physics course when we met with her group. Her general approach to studying was to hope that the instructor was direct about expectations and guidelines because then, “the ball is in (her) court and (she) either learns the material or not.” Thus, Brianna was relatively self-motivated but relied on instructors to provide cues regarding when and how hard to study. This motivation is also sparked by her aspiration to attend medical school, which requires doing well on the Medical College Admission Test (MCAT). Brianna observed that “even if I get an A on a test but have no idea what is going on, it wouldn’t set me up to be in a good place for studying the MCAT in the future.”
In addition, she relied heavily on what she called her own preferred “learning style,” which centered on reading and re-reading text, whether it be the textbook or notes taken in class. Given her reliance on text and notes, Brianna observed that, “I pretty much show up to lecture just to write down what he’s saying.” The notes she took in class then became an important artifact for later studying, as she used them to create flashcards from her notes (and the textbook) and an outline for the course that is added to throughout the term. During her actual study sessions, Brianna either studied alone, reviewing her notes, scanning various digital resources, and doing practice problems, or with a group of friends in the library where she typically had on her headphones while surrounded by classmates who intermittently helped one another out on difficult problems.
Brianna’s use of digital technology is notable because she described online videos, the course website, the Internet, and social media as the “majority” of the resources she used to study. For example, she consulted free online tutoring videos (especially videos featuring one tutor in particular at the University of California at Berkeley), which helped her fill out her notes and summaries from the class and textbook. Overall she described the Internet as a “great resource” for finding course materials (e.g., slides, notes, exams, and videos) from other instructors teaching the same course at other colleges or universities. The questions these other instructors ask their students provides insights into what Brianna perceives her professor may ask, so she values their outsider’s perspective. Further, when doing her homework problems, she looked up the solutions online, even when she was confident about her answer. She does this to ensure that she is “approaching [the problem] in the right way” or to see if there are alternative methods to solving the problem. This, in turn, gives her a more “holistic grasp of the question.” Finally, in this and other courses, social media sites such as Facebook provided a place where she and her friends posted questions and shared approaches to different problems. Notably, most of the digital resources Brianna utilized were not part of the official course materials organized by her instructor and posted on the course website.
Thus, for Brianna studying involves a process of re-reading course materials and tools such as cue cards and digital media, largely in response to instructor’s cues about upcoming exams or homework. Driven by the desire to attend medical school introduced an element of motivation that made her take studying rather seriously.
Dr. Wells’ course. The final case is that of five female students taking an upper division anatomy and physiology course with Dr. Wells. In this course, which had an enrollment of 525 students across three sections, Dr. Wells had provided a rich array of learning resources on the course website that included weekly postings of videotaped lectures and PowerPoint slides, weekly practice questions, old exams, and links to other online resources. The students in the focus group noted that Dr. Wells did not simply post these resources and let students figure out how to utilize them but instead discussed in class how to use each tool and study with them. As Angelica noted, “She just does a really great job of giving us a lot of different ways to study.” Jacquie concurred, saying that while an online course she was taking was similarly well-resourced, “Dr. Wells provides more alternative methods to study which is what makes her course stand out.” Ultimately, in providing such a variety of resources for studying, Dr. Wells had crafted a learning experience that stood out for these students. For Bailey, who had little experience with the material, this was particularly important because “it’s really hard to stick your fingers in and get going,” and if you only have a textbook to work with, the entry points to the material are limited, often inaccessible and not particularly engaging.
In many ways, Dr. Wells was running a partially flipped classroom, in that students watched videotaped lectures online and came to a class that was highly interactive and engaging. During the class, Dr. Wells was constantly in motion, using her iPad to project slides on the screen while also writing and drawing using a stylus pen. Many questions were asked of students, including peer-based activities and small group discussions. Linda noted that Dr. Wells also emphasized important ideas across various formats such as clicker questions, practice tests, and study questions, such that “the repetition is awesome…even if you’re tired or distracted, eventually you’ll still get it.”
In terms of how these students actually studied in the course, weekly study questions (i.e., short essay questions that recap entire lectures) provided by Dr. Wells played an important role. For Jacquie, who said that she essentially crams before the exam—saying “well yeah, that’s why we study, for the exams”—her lecture notes, study questions, the mastering anatomy online resources, and old test materials were all utilized during study sessions. After discussing the course with her friends, however, she concluded that the best way to succeed in the course was “not to do all of her practice exams but to do those freaking (weekly) study questions.” Angelica said that “I noticed when I don’t do the study questions I don’t do very well.” This approach is similar to the technique of distributed practice, or regularly spaced testing of material over time, which is one of the high-impact study strategies identified by Dunlosky and colleagues ( 2013 ). Robin also spent 3–4 h after each class doing the study questions, along with a variety of other tools including the mastering anatomy activities, notes, lecture videotapes, and old test materials. In fact, while the group varied on the timing of their studying, all were cued by the instructor, used a variety of digital and print resources and between four and six study strategies. This reliance on multiple resources and study strategies is unsurprising given how Dr. Wells structured her course and guided her students in regard to studying. Within this learning environment, students then developed their own approach to studying but in ways strongly shaped by the resources and strategies Dr. Wells had embedded in the course structure.
The field of higher education in general and STEM education in particular continues to grapple with how to best facilitate learning, persistence, and retention throughout students’ postsecondary careers. Does the answer lie in changing teacher behaviors alone, such as the adoption of active learning techniques, structural responses such as reducing student debt and dealing with the rising price of college, or is success also dependent upon student attributes such as engagement and motivation? What these questions reveal is that students’ experiences in college are shaped by a variety of influences and that the intersection among policy, economics, organizations, and instruction provides a more accurate frame for thinking about student success than a search for a single “magic bullet” solution.
The same idea applies to thinking about the role that effective study strategies play in student learning. While the use of high-impact practices such as distributed practice is certainly a key ingredient in leading to student learning (Dunlosky et al. 2013 ), it is important to recognize that students’ adoption of these practices requires several antecedent conditions to be in place before this can happen. These include knowledge of these methods, time to study, access to the resources required to study in this manner, and so on. Similarly, studying is not simply about using strategies such as re-reading text or doing practice problems but is a process that involves cues about when to study, the timing of their actual study sessions, which resources to utilize, where to study, and which strategies to employ. How these stages unfold in practice are also shaped by a variety of factors such as a students’ personal life, the course material, and how instructors structure courses and make learning resources available. This is not to diminish the importance of high-impact study strategies but instead to point out that there are many steps taken by students to get to the point where they can sit down and utilize them with some regularity.
In the remainder of this paper, we discuss how this exploratory study contributes to the literature on college student study habits, particularly through the articulation of a multi-dimensional conception of studying that can provide instructors and administrators with a more nuanced account of how students engage in studying. In combination with the data reported in this paper as well as developments in educational technology and research, such an account also highlights the importance of instructional design that facilitates students’ use of high-impact strategies, diversified learning tools, and self-regulatory capabilities.
A new approach for thinking about undergraduates’ study behaviors
The results reported in this paper confirm and extend prior research on college student study habits. The data reinforce prior research that some of the most dominant study strategies utilized by students include reviewing notes and re-reading textbooks (Karpicke et al. 2009 ), utilize a variety of digital resources (Smith and Caruso 2010 ), and also rely on instructors to provide cues to begin studying (Miller and Parlett 1974 ). While the study described here is limited by a small sample size and lack of data on the impact of various study habits on learning outcomes, it was designed to shed light on fine-grained behaviors among a small group of students in order to advance our understanding of decision-making and action in specific social, organizational, technological contexts. In doing so, we extend the prior literature by offering an integrative multi-stage approach for thinking about study behaviors.
When interpreting the results from this exploratory study, we observed that students discussed their studying in terms of stages that began with cues to study and ended with their use of specific strategies. Along the way, they made decisions about who to study with and which resources to use, an account consistent with a situative theory of cognition, which posits that mental activity and social action is situated within specific socio-cultural and organizational contexts while also being distributed among mind, tool, and activity (Greeno 1998 ; Spillane et al. 2001 ). In other words, studying is not solely a matter of a “mind” sequestered with a book and highlighter pen, or a behavior that could be distilled into hours spent studying or the prevalence of a particular strategy, but instead involves people interacting with one another and various tools in specific situations (Seely Brown and Adler 2008 ). This is not to diminish the value of experimental work that does hone in on specific aspects of studying such as how students self-pace their study or time spent on specific tasks (Bjork, Dunlosky and Kornell 2013 ) but instead is an argument that a broader perspective of the act of studying itself is also useful.
Consequently, based on the data presented in this paper, we suggest that a new way of thinking about studying is warranted that includes the following components: (1) recognizing the situation and detecting cues to initiate studying, (2) marshaling resources and managing distractions (or not), (3) selecting a time and social setting to study, and then selecting specific strategies, and (4) engaging in a period of self-reflection. We illustrate this approach using the three cases reported earlier in the paper (see Fig. 1 ).
Some caveats are necessary when interpreting this figure. First, while the stages of cue detection and timing, resource use, and strategies are based on data from this paper, the self-regulative period is not. Instead, it is included as a post-assessment phase of reflection and commitment that the literature indicates is an important aspect of learning (Zimmerman and Schunk 2001 ). Second, we do not claim that all 61 participants in the study progressed through each of these steps, but instead that this conception of studying captures the broad range of behaviors and experiences students reported engaging in during a recent study session. As a result, we are not suggesting that this account of studying is generalizable to all students but is a heuristic device for thinking about studying in a more multi-dimensional manner than is common at the present time.
Thus, we argue that conceptions of the act of "studying" extend beyond a focus on discrete, decontextualized factors such as hours spent studying or the use of specific strategies (e.g., re-reading text). In making this argument, we highlight the importance of ecological validity when thinking about study habits in general and interpreting laboratory-based research in particular. In other words, understanding how findings from the literature about "effective" study habits may vary according to disciplinary, social, institutional, or personal situations will be important for future work in the area. We also suggest that a more multi-dimensional conception of studying can also be a useful interpretive framework for educators, instructional designers, and administrators to begin thinking more broadly and strategically about how their courses are designed (or not) to foster effective study habits. By recognizing that studying involves multiple states, resources, strategies and actors, it becomes necessary to move beyond simply providing “how-to” guides for studying or recommendations for students to use high-impact practices to instead think about the role that cue-seeking, resource acquisition, and distraction management play in shaping students’ study habits. With a more situative view of studying in mind, it is possible to consider how the course as a whole creates an environment that prompts particular study behaviors, such as Dr. Wells’ provision of various learning tools via her LMS that prompted students to study with them. Thinking of studying in these terms, in the remainder of this paper we highlight ways that educators can facilitate or support effective studying and learning practices: fostering self-regulated learning and using principles from instructional design to encourage high-impact studying.
Fostering self-regulated learners
One of the most pressing issues facing educators is the fact that many students continue to utilize ineffective study practices, such as re-reading textbooks or cramming the night before an exam. Informing some low-impact practices are “faulty mental models” (p. 417) about how memory and learning work, such as the view that information can be recalled and played back like a recording (Bjork, Dunlosky and Kornell 2013 ). Instead, the retrieval process involves reconstructing knowledge from various stored memories, is heavily dependent on specific cues, and that upon cueing information in memory becomes reinforced. Essentially, students need to understand that in order to create a library of information in their minds that is easily accessed and retained over the long term requires a “meaningful encoding of that information” which involves integrating information into a network of connected ideas and then regularly practicing retrieval of that information (Dunlosky et al. 2013 ).
Besides becoming more sophisticated learners and theorists about how the mind works, it is clear that students can also benefit from more guidance about how to more effectively study and learn. Educational psychologists argue that becoming a more adept learner is not simply about amassing tips and strategies about how to study but is based on becoming what is known as a self-regulated learner, which is the “self-directive process by which learners transform their mental abilities into academic skills” (Zimmerman 2002 , p. 65). Self-regulation is not just a quality or personal aptitude, however, but is best thought of as a sequence of states that include forethought (i.e., plans for studying), performance, and self-reflection. Motivation to initiate studying on one’s own is important, but perhaps more critical is the self-monitoring of performance, especially the ability to scrutinize and interpret failure and make corrections (Boekaerts and Corno 2005 ). Furthermore, while considerable barriers exist for students to develop self-regulative habits, such as a belief that intelligence is “fixed” and not malleable (Yeager and Dweck 2012 ) and assumptions that learning should be simple and unproblematic (Bjork, Dunlosky and Kornell, 2013 ), helping students to develop this aptitude is critical because it is a core aspect of success not only in school but also in life and the workplace (Pellegrino and Hilton 2012 ).
So how, if at all, can STEM educators embed self-regulatory skills into their courses? Setting aside for the moment the extent to which self-control, goal setting, and responsibility should be learned in the home, grade school, or various other cultural fields during childhood, the fact remains that it is possible to teach some aspect of self-regulatory competencies in the college classroom (Nilson 2013 ). One strategy is to create a classroom environment with high expectations and a low- to zero-tolerance policy for irresponsible behavior or late assignments, thereby encouraging if not forcing students to set goals for themselves and achieve them. Another strategy is the widely used instructional wrapper, which refers to prompts for students to reflect on their performance before and after an assignment or activity, which trains students to regularly reflect on their study habits and approach to learning (Lovett 2013 ). Other ideas include modeling learning strategies such as self-monitoring and summarizing in front of students in what is known as a “cognitive apprenticeship” (Palincsar and Brown 1984 ), using small group work tasks designed to spark self-regulation (Fitch et al. 2012 ) and assigning open-ended tasks and assessments requiring students to choose strategies and take control of their learning (Boekaerts and Corno 2005 ).
We conclude this discussion about self-regulation with a note about digital media. While online resources and digital devices can play an important and productive role in facilitating student learning (Dabbagh and Kitsantas 2012 ; Smith and Caruso 2010 ), it is evident from the data that they can detract from focused study. Thus, another aspect about self-regulation is the willingness and ability to remove digital distractions when they are not serving a productive purpose, such as the students in this study who deliberately went to libraries without wireless Internet or parents’ homes where devices were confiscated. As will be discussed in the next section, technology can and should be part of educators’ instructional toolkit, but students would be well served by adopting more self-regulated stances when it comes to the presence of technology in their study sessions.
Encouraging personalized learning and high-impact studying through course structure
Next, we turn to issue of how instructors, through the deliberate design of their courses, can facilitate effective study habits. Here, we focus on two aspects of effective studying: the use of multiple representations and modalities and the use of high-impact strategies. In both cases, we can look to the example of Dr. Wells’ course where she embedded within the structure of the course itself opportunities for students to draw upon various learning tools while also imposing a high-impact study habit (i.e., distributed practice) via weekly practice questions.
First, providing students with a variety of learning resources and tools offers them a variety of entry points with which to explore the material. The rationale for doing so is not to support students’ distinct learning styles, an idea that is popular but unsupported by the empirical evidence (Pashler et al. 2008 ), but instead is based on the fact that learners that engage with varied representations of an idea or concept demonstrate improved learning outcomes (Pellegrino and Hilton 2012 ). In addition, the provision of various learning tools is useful because today’s learners are broadly proficient in developing personalized learning pathways, whether for academic or personal purposes, using online resources and social media (Dabbagh and Kitsantas 2012 ). In doing so, many create social learning environments, or what some call “participatory cultures,” where people develop online learning communities where they collectively create, share, and learn from each other (Jenkins et al. 2006 ). Essentially, the idea is to make available a repertoire of learning tools and media for students that they can then select from to approach the material from multiple perspective and according to their own unique way of engaging with different learning modalities.
The second approach for facilitating effective study habits pertains to the structure of a course, from the timing and nature of assessments to the types of learning activities students are required to do. Again, consider the example of Dr. Wells, who embedded within her course an assessment strategy that forced her students to engage in the high-impact study habit of distributed practice. Through weekly practice questions, students were required to regularly take mini-exams on different topics (Dunlosky et al. 2013 ). Spacing out study sessions on distinct topics enhances learning through the spacing effect and also by introducing comparisons or “interference” across topics, which results in higher-order representations or complex mental models that not only are repositories of information but also facilitate transfer and retention (Bjork, Dunlosky, and Kornell 2013 ).
Similarly, the role of course structure in facilitating student learning has been well documented in STEM education, where pre-class reading quizzes and weekly practice exams have been linked to improved student learning (Freeman et al. 2011 ), and even in reducing the achievement gap between white and under-represented minority students, because highly structured courses with regular practice may introduce study and learning skills to students with little experience from high school (Haak et al. 2011 ). The takeaway here is that as educators, we can design our courses and teach our classes with explicit attention towards creating (and mandating) situations for students to engage in certain study habits.
The attention currently being placed on STEM instructors and their pedagogical acumen as key facilitators of student learning is well-placed, but the relationship between teaching and learning is anything but direct, linear, and unproblematic. What students decide to do in terms of when and how to study act as critical intermediaries between what instructors do in the classroom and students’ ultimate performance in college. As Entwistle and Tait suggested over 25 years ago, ( 1990 , p. 170), students’ behaviors and strategies “affects learning probably as much as, if not more than, the classroom skills of the lecturer.” On this point, there are both promising and troubling signs. While students are increasingly utilizing varied resources and media in a deliberate and creative manner to advance their studies, some study methods that are demonstrably ineffective continue to be widely used. The exploratory study reported in this paper offers a new, multi-dimensional way to think about studying that suggests future research directions exploring undergraduate study habits including similar descriptive research with larger samples and additional disciplines, experimental research focusing on specific strategies under different conditions (e.g., resource use, cues for studying), and examining the relationship between course structure and studying.
Ultimately, students must take responsibility for their learning and strategize ways to create situations—whether in a quiet library basement or a group study session online—where they can effectively study and learn. However, postsecondary educators must also be cognizant of the pressures facing today’s college students and the fact that many have not been taught how to engage in high-impact study habits but instead rely on re-reading highlighted text. One of the guiding principles for instructional design should be the idea that it is no longer tenable to assume that students have been taught how to effectively study and learn prior to their matriculation into a college or university. While students bring a wealth of new learning habits and technological acumen to the twenty-first century classroom—whether online or face-to-face—they still need guidance in how to study. This conclusion, however, should not lead to complaints about unprepared students or a failed K-12 sector but instead needs to spark postsecondary educators to carefully design of rich and engaging learning environments that sparks self-regulatory habits of mind and encourages high-impact studying, so that students are well positioned to succeed.
Astin, A. W. (1984). Student involvement: a developmental theory for higher education. Journal of College Student Development, 40 (5), 518–529.
Google Scholar
Babcock, P., & Marks, M. (2010). Leisure college, USA: the decline in student study time. American Enterprise Institute for Public Policy Research, 7 , 1–7.
Bastedo, M. N. (2012). Organizing higher education: a manifesto. In M. N. Bastedo (Ed.), The organization of higher education: managing colleges for a new era (pp. 3–17). Baltimore: The Johns Hopkins University Press.
Bernard, H. R. (2011). Research methods in anthropology: qualitative and quantitative approaches (5th ed.). Lanham: Altamira Press.
Biggs, J. (1987). Student approaches to learning . Hawthorn: Australian Council for Educational Research.
Bjork, R. A., Dunlosky, J., & Kornell, N. (2013). Self-regulated learning: Beliefs, techniques, and illusions. Annual review of psychology, 64, 417–444.
Article Google Scholar
Boekaerts, M., & Corno, L. (2005). Self-regulation in the classroom: a perspective on assessment and intervention. Applied Psychology, 54 (2), 199–231.
Bok, D. (2009). Our underachieving colleges: a candid look at how much students learn and why they should be learning more . Princeton: Princeton University Press.
Carini, R. M., Kuh, G. D., & Klein, S. P. (2006). Student engagement and student learning: testing the linkages. Research in Higher Education, 47 (1), 1–32.
Cassidy, S. (2011). Self-regulated learning in higher education: identifying key component processes. Studies in Higher Education, 36 (8), 989–1000.
Coburn, C. E., & Turner, E. O. (2012). The practice of data use: an introduction. American Journal of Education, 118 (2), 99–111.
Coffield, F., Moseley, D., Hall, E., & Ecclestone, K. (2004). Learning styles and pedagogy in post-16 learning: a systematic and critical review . London: Learning Skills Network.
Credé, M., & Kuncel, N. R. (2008). Study habits, skills, and attitudes: the third pillar supporting collegiate academic performance. Perspectives on Psychological Science, 3 (6), 425–453.
Dabbagh, N., & Kitsantas, A. (2012). Personal learning environments, social media, and self-regulated learning: a natural formula for connecting formal and informal learning. The Internet and higher education, 15 (1), 3–8.
Duckworth, A. L., Peterson, C., Matthews, M. D., & Kelly, D. R. (2007). Grit: perseverance and passion for long-term goals. Journal of Personality and Social Psychology, 92 (6), 1087–1101.
Dunlosky, J., Rawson, K. A., Marsh, E. J., Nathan, M. J., & Willingham, D. T. (2013). Improving students’ learning with effective learning techniques: promising directions from cognitive and educational psychology. Psychological Science in the Public Interest, 14 (1), 4–58.
Entwistle, N. J., & Tait, H. (1990). Approaches to learning, evaluations of teaching, and preferences for contrasting academic environments. Higher Education, 19 (2), 169–194.
Fitch, T., Marshall, J., & McCarthy, W. (2012). The effect of solution-focused groups on self-regulated learning. Journal of College Student Development, 53 (4), 586–595.
Franke, R., Ruiz, S., Sharkness, J., DeAngelo, L., & Pryor, J. (2010). Findings from the 2009 administration of the College Senior Survey (CSS): National aggregates. Chicago: Higher Education Research Institute at the University of California Los Angeles.
Freeman, S., Haak, D., & Wenderoth, M. P. (2011). Increased course structure improves performance in introductory biology. CBE-Life Sciences Education, 10 (2), 175–186.
Freeman, S., Eddy, S. L., McDonough, M., Smith, M. K., Okoroafor, N., Jordt, H., & Wenderoth, M. P. (2014). Active learning increases student performance in science, engineering, and mathematics. Proceedings of the National Academy of Sciences, 111 (23), 8410–8415.
Gamoran, A., Anderson, C. W., Quiroz, P. A., Secada, W. G., Williams, T., & Ashman, S. (2003). Transforming teaching in math and science: how schools and districts can support change . New York: Teachers College Press.
Gee, J. P. (1986). Units in the production of narrative discourse. Discourse Processes, 9 , 391–422.
Glaser, B. G., & Strauss, A. L. (1967). The discovery of grounded theory: strategies for qualitative research . New Brunswick: Aldine Transaction.
Greeno, J. G. (1998). The situativity of knowing, learning, and research. American psychologist, 53 (1), 5–26.
Haak, D. C., HilleRisLambers, J., Pitre, E., & Freeman, S. (2011). Increased structure and active learning reduce the achievement gap in introductory biology. Science, 332 (6034), 1213–1216.
Halverson, R. (2003). Systems of practice: how leaders use artifacts to create professional community in schools. Educational Policy Analysis Archives, 11 (37), 1–35.
Hartwig, M. K., & Dunlosky, J. (2012). Study strategies of college students: are self-testing and scheduling related to achievement? Psychonomic Bulletin & Review, 19 (1), 126–134.
Hativa, N., & Goodyear, P. (Eds.). (2002). Teacher thinking, beliefs, and knowledge in higher education . Norwell: Kluwer Academic Publishers.
Hora, M. T. (2012). Organizational factors and instructional decision-making: A cognitive perspective. The Review of Higher Education, 35(2), 207–235.
Hora, M. T. (2016). Navigating the problem space of academic work: How workload and curricular affordances shape STEM faculty decisions about teaching and learning. AERA Open, 2(1), 1–19.
Jenkins, H., Clinton, K., Purushotma, R., Robison, A. J., and Weigel, M. (2006). Confronting the challenges of participatory culture: media education for the 21 st century. Chicago: The John D. and Catherine T. MacArthur Foundation.
Karpicke, J. D., Butler, A. C., & Roediger, H. L., III. (2009). Metacognitive strategies in student learning: do students practice retrieval when they study on their own? Memory, 17 (4), 471–479.
Klein, G. (2008). Naturalistic decision making. Human Factors, 50 (3), 456–460.
Kornell, N. (2009). Optimising learning using flashcards: spacing is more effective than cramming. Applied Cognitive Psychology, 23 , 1297–1317.
Kuo, J., Hagie, C., & Miller, M. T. (2004). Encouraging college student success: the instructional challenges, response strategies, and study skills of contemporary undergraduates. Journal of Instructional Psychology, 31 (1), 60–67.
Lave, J. (1988). Cognition in practice: mind, mathematics and culture in everyday life . Cambridge: Cambridge University Press.
Book Google Scholar
Lotkowski, V. A., Robbins, S. B., & Noeth, R. J. (2004). The role of academic and non-academic factors in improving college retention . ACT Policy Report, Retrieved from http://ww.cfder.org/uploads/3/0/4/9/3049955/the_role_of_academic_and_non_academic_factors_in_improving_college_retention.pdf .
Lovett, M.C. (2013). Make exams worth more than the grade: using exam wrappers to promote metacognition (pp. 18-52), in Using reflection and metacognition to improve student learning: across the disciplines, across the academy, ed. Kaplan, M, Silver, N., LaVague-Manty, D., & Meizlish, D. Sterling, VA: Stylus Publishing.
Marton, F., & Säljö, R. (1976). On qualitative differences in learning: I. Outcome and process. British Journal of Educational Psychology, 46 , 4–11.
Merriam, S. B. (2014). Qualitative research: a guide to design and implementation . San Francisco: John Wiley & Sons.
Miller, C. M., & Parlett, M. R. (1974). Up to the mark: a study of the examination game . London: SRHE.
Morgan, G., Moskal, P., Wolf, A., Dziuban, C., McMartin, F., & Morrill, J. (2012). Understanding student use of digital learning resources. [Powerpoint slides]. Retrieved from http://bit.ly/1vjZRsY
Nilson, L. (2013). Creating self-regulated learners: strategies to strengthen students self-awareness and learning skills . Sterling: Stylus Publishing.
Nonis, S. A., & Hudson, G. I. (2010). Performance of college students: impact of study time and study habits. The Journal of Education for Business, 85 , 229–238.
Palincsar, A. S., & Brown, A. L. (1984). Reciprocal teaching of comprehension-fostering and comprehension-monitoring activities. Cognition and Instruction, 1 , 117–175.
Pascarella, E., & Terenzini, P. (2005). How college affects students: a third decade of research . San Francisco: Jossey-Bass.
Pashler, H., McDaniel, M., Rohrer, D., & Bjork, R. (2008). Learning styles concepts and evidence. Psychological science in the public interest, 9 (3), 105–119.
Pellegrino, J. W., & Hilton, M. L. (Eds.). (2012). Education for life and work: developing transferable knowledge and skills in the 21 st century . Washington, DC: National Academies Press.
President’s Council of Advisors on Science and Technology (2012). Report to the President. Engage to excel: Producing one million additional college graduates with degrees in science, technology, engineering and mathematics. Washington, D.C.: Executive Office of the President.
Ramsden, P. (1979). Student learning and perceptions of the academic environment. Higher Education, 8 (4), 411–427.
Riding, R., & Cheema, I. (1991). Cognitive styles—an overview and integration. Educational Psychology, 11 (3-4), 193–215.
Robbins, S. B., Lauver, K., Le, H., Davis, D., Langley, R., & Carlstrom, A. (2004). Do psychosocial and study skill factors predict college outcomes? A meta-analysis. Psychological Bulletin, 130 (2), 261–288.
Rosen, L. D., Carrier, L. M., & Cheever, N. A. (2013). Facebook and texting made me do it: media-induced task-switching while studying. Computers in Human Behavior, 29 , 948–958.
Ruiz, S., Sharkness, J., Kelly, K., DeAngelo, L., & Pryor, J. (2010). Findings from the 2009 administration of the Your First College Year (YFCY): national aggregates. Los Angeles: Higher Education Research Institute at the University of California Los Angeles.
Seely Brown, J., & Adler, R. P. (2008). Open education, the long tail, and learning 2.0. Educause Review, 43 (1), 16–20.
Slavin, R. E. (2002). Evidence-based education policies: transforming educational practice and research. Educational researcher, 31 (7), 15–21.
Smith, S. D., & Caruso, J. B. (2010). Key findings: the ECAR study of undergraduate students and information technology . Boulder: EDUCAUSE Center for Applied Research.
Spillane, J. P., Halverson, R., & Diamond, J. B. (2001). Investigating school leadership practice: a distributed perspective. Educational Researcher, 30 (3), 23–28.
Spillane, J. P., Reiser, B. J., & Reimer, T. (2002). Policy implementation and cognition: reframing and refocusing implementation research. Review of Educational Research, 72 (3), 387–431.
Stebbins, R. A. (2001). Exploratory research in the social sciences. Qualitative research methods in the social sciences, 48 . Thousand Oaks: Sage Publications.
Strauss, A., & Corbin, J. M. (1990). Basics of qualitative research: grounded theory procedures and techniques . Thousand Oaks: Sage Publications, Inc.
Tinto, V. (1993). Leaving college: Rethinking the causes and cures of student attrition. (2nd ed.). Chicago: University of Chicago Press.
Treisman, U. (1992). Studying students studying calculus: a look at the lives of minority mathematics students in college. The College Mathematics Journal, 23 (5), 362–372.
Yeager, D. S., & Dweck, C. S. (2012). Mindsets that promote resilience: when students believe that personal characteristics can be developed. Educational Psychologist, 47 (4), 302–314.
Zimmerman, B. J. (2002). Becoming a self-regulated learner: an overview. Theory into Practice, 41 (2), 64–70.
Zimmerman, B. J., & Schunk, D. H. (Eds.). (2001). Self-regulated learning and academic achievement: theoretical perspectives . Hillsdale: Lawrence Erlbaum Associates.
Download references
Acknowledgements
The authors would also like to thank Jana Bouwma-Gearhart and Jennifer Collins for their involvement in this study and collecting data reported in this paper.
This research was supported by a grant from the National Science Foundation (DUE#1224624) for the Tracking the Processes of Data Driven Decision-Making Study ( http://tpdm.wceruw.org ).
Authors’ contributions
AO participated in the design of the study and data collection, led the data analysis, and collaborated with MH to draft the manuscript. MH conceived of the study, led the design, participated in data collection and analysis, and finalized the manuscript. Both authors read and approved the final manuscript.
Competing interests
The authors declare that they have no competing interests.
Author information
Authors and affiliations.
Department of Liberal Arts and Applied Studies, University of Wisconsin-Madison, 21 N. Park St., Madison, WI, 53715, USA
Matthew T. Hora
Wisconsin Center for Education Research, University of Wisconsin-Madison, Madison, USA
Amanda K. Oleson
You can also search for this author in PubMed Google Scholar
Corresponding author
Correspondence to Matthew T. Hora .
Rights and permissions
Open Access This article is distributed under the terms of the Creative Commons Attribution 4.0 International License ( http://creativecommons.org/licenses/by/4.0/ ), which permits unrestricted use, distribution, and reproduction in any medium, provided you give appropriate credit to the original author(s) and the source, provide a link to the Creative Commons license, and indicate if changes were made.
Reprints and permissions
About this article
Cite this article.
Hora, M.T., Oleson, A.K. Examining study habits in undergraduate STEM courses from a situative perspective. IJ STEM Ed 4 , 1 (2017). https://doi.org/10.1186/s40594-017-0055-6
Download citation
Received : 01 August 2016
Accepted : 06 January 2017
Published : 01 February 2017
DOI : https://doi.org/10.1186/s40594-017-0055-6
Share this article
Anyone you share the following link with will be able to read this content:
Sorry, a shareable link is not currently available for this article.
Provided by the Springer Nature SharedIt content-sharing initiative
- Learn Management System
- Study Strategy
- Study Session
- Digital Resource
- Medical College Admission Test
Academia.edu no longer supports Internet Explorer.
To browse Academia.edu and the wider internet faster and more securely, please take a few seconds to upgrade your browser .
Enter the email address you signed up with and we'll email you a reset link.
- We're Hiring!
- Help Center
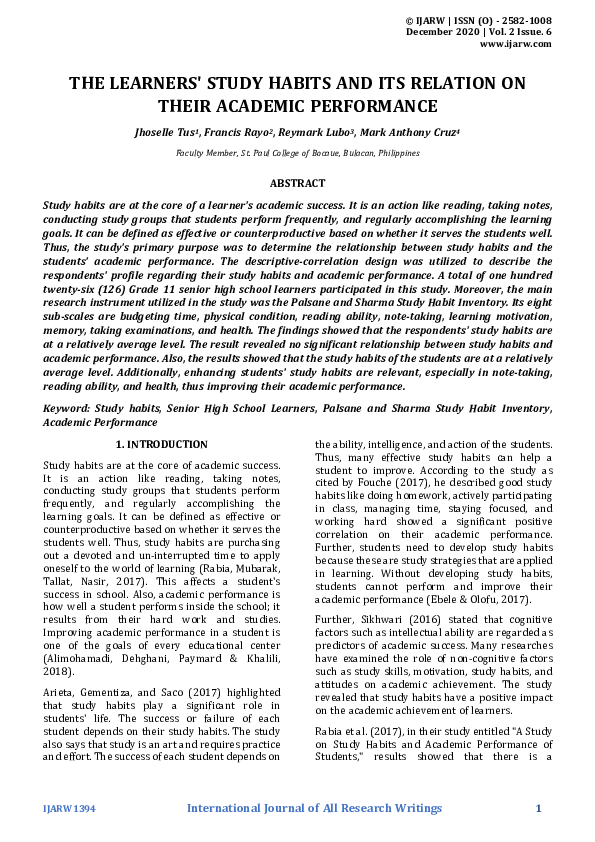
THE LEARNERS' STUDY HABITS AND ITS RELATION ON THEIR ACADEMIC PERFORMANCE

2020, International Journal of All Research Writings
Study habits are at the core of a learner's academic success. It is an action like reading, taking notes, conducting study groups that students perform frequently, and regularly accomplishing the learning goals. It can be defined as effective or counterproductive based on whether it serves the students well. Thus, the study's primary purpose was to determine the relationship between study habits and the students' academic performance. The descriptive-correlation design was utilized to describe the respondents' profile regarding their study habits and academic performance. A total of one hundred twenty-six (126) Grade 11 senior high school learners participated in this study. Moreover, the main research instrument utilized in the study was the Palsane and Sharma Study Habit Inventory. Its eight sub-scales are budgeting time, physical condition, reading ability, note-taking, learning motivation, memory, taking examinations, and health. The findings showed that the respondents' study habits are at a relatively average level. The result revealed no significant relationship between study habits and academic performance. Also, the results showed that the study habits of the students are at a relatively average level. Additionally, enhancing students' study habits are relevant, especially in note-taking, reading ability, and health, thus improving their academic performance.
Related Papers
Ellen Mariel Maslog
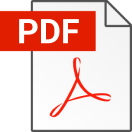
Arul Lawrence A S
The present study was probed to find the significant relationship between study habits and academic achievement of higher secondary school students with reference to the background variables. Survey method was employed. Data for the study were collected from 300 students in 13 higher secondary schools using Study Habits Inventory by V.G.Anantha (2004) and the Quarterly Achievement Test Questions. The significant difference between the means of each pair of group was computed using Standard Deviation, ‘t’ test, ANOVA and Pearson’s Co-efficient Correlation. The findings were established and tabulated from the analysed data. The finding shows that there was no significant difference between study habits and academic achievement of higher secondary school students. Finally, Interpretations were given by the investigator based on the findings.
International Journal of All Research Education and Scientific Methods (IJARESM)
praveen binjha
The aims of this study the examine the relationship between student's study habits with academic achievement. A correlational study was conducted among undergraduate students. The sample consisting sixty-four undergraduate students, age range between 17-21years randomly from Ravenshaw University, Cuttack, and North Orissa University. Study habits inventory Palsane and Sharma (2003) and academic achievement score were used in the study. ANOVA and Perasons' Correlation coefficient used to find the association between study habits with academic achievement. The findings of the study 50% of students found an average/moderate study habits while 37.5% were good and 12.5% were considered poor in study habits. There is a positive relationship between study habits and academic achievement. The mean of students' grade point was 67.12±9.9 out of 19 male and the mean of female 70.1 ± 9.4, overall students grade point was 69.21(9.63). The score of study habit includes time budgeting (6.9 out of 10), physical condition (4.9 out of 12), reading ability (3.2 out of 16), note taking (4.9 out of 6), learning motivation (5.8 out of 12), memorization (6.25 out of 8), taking the examination (3.8 out of 20) and health (3.56 out of 6) and the total score (39.34 out of 90). The results show a significant relationship between academic achievement and study habits. In conclusion, the study habits of students indicated significant relationship with students' academic achievement.
shivani kulshreshtha
International Journal of Scientific Research in Science and Technology IJSRST , charu vyas
The more than twenty online studies were reviewed to identify the relationship between study habits and academic achievement of school going children. It was found that for the last few years, that there is a significant positive relation between study habits and academic achievement of schoolers. The review of various studies revealed that the academic achievement of boys is significantly higher than the girls. The study also concluded that there is a positive correlation between various factors (such as home environment, self-esteem, socioeconomic status, parents educational level, parental involvement, school environment, peer influence, academic motivation, study facility, emotional intelligence) and academic achievement.
www.ijcrt.org
Mani Mookkiah
In this study, an attempt has been made to study the Study habits and their impact on academic performance in English of higher secondary school students in devakottai educational district. The Study Habits Inventory developed and validated by the investigator was used to collect the data from the sample of 336 students at Government and Management higher secondary schools. The survey method has been followed and the simple sampling technique was used to select the subjects for the present study. The result of the analysis reveals that the study habits and academic achievement, therefore, teachers should nurture good study habits, especially in the following areas: budgeting time, learning motivation, memorization, and examination taking because these areas had a significant correlation with English learning achievement. Furthermore, the positive study habits the students can do, such as: studying every day, attending the class every day, taking notes, asking for help if facing problems with their studies, and preparing for the examination.
Rev. Col. Bras. …
Jurandir Dias
Blessing Paul
Jonathan Story
Studia Ekonomiczne. Zeszyty Naukowe Uniwersytetu Ekonomicznego w Katowicach
Joanna Rutecka-Góra
RELATED PAPERS
Nature Genetics
EuroEconomica
Ghizlane CHAIBI
Revista Mexicana De Biodiversidad
Gabriel Barrios
Sri Supadmi
Contemporary Issues in Nig
Yusuf Danladi Mohammed
The American Journal of Human Genetics
Matthias Hammerschmidt
Journal of Natural Products
Zaheer Ahmed
Monica Bethel Garcia Aguirre
Cardiovascular Diabetology
Faadiel Essop
HIV Medicine
Birgitta Åsjö
Business & Management Studies: An International Journal
Professor Bilal Çankır
Alexandra Nobre
Australasian Plant Pathology
eddie Mwenje
Zeitschrift für Migrationsforschung
Stephan Liebscher
CONCEPCION ANGUITA OLMEDO
Journal of Applied Meteorology
Edwin X Berry
Irina Al-Khairi
Filip Ionescu
Childs Nervous System
Mario Zuccarello
Journal of Bacteriology
John Cronan
Revista Brasileira de História da Educação
Carlos Eduardo Vieira
RELATED TOPICS
- We're Hiring!
- Help Center
- Find new research papers in:
- Health Sciences
- Earth Sciences
- Cognitive Science
- Mathematics
- Computer Science
- Academia ©2024
share this!
May 6, 2024
This article has been reviewed according to Science X's editorial process and policies . Editors have highlighted the following attributes while ensuring the content's credibility:
fact-checked
peer-reviewed publication
Do good lessons promote students' attention and behavior?
by Anke Wilde, Leibniz-Institut für Bildungsforschung und Bildungsinformation
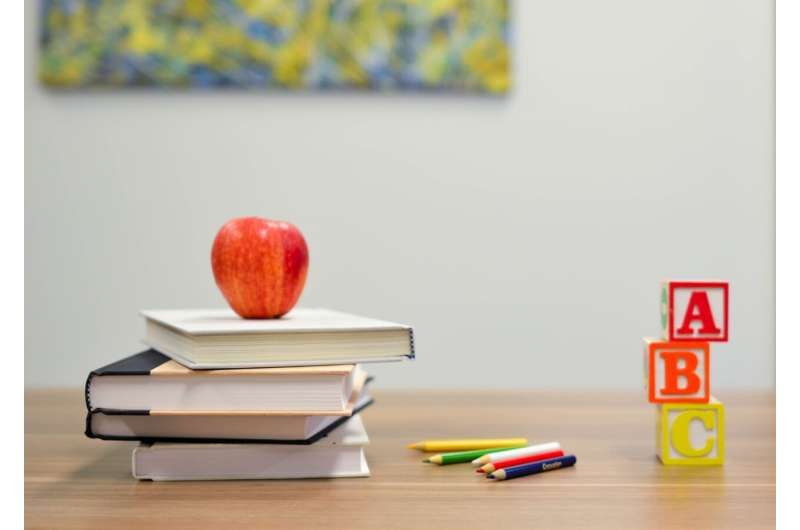
Students are better able to regulate themselves in lessons that they consider to be particularly well implemented. This is the conclusion drawn from a study by the DIPF | Leibniz Institute for Research and Information in Education, published in the journal Learning and Instruction .
The link between teaching quality and self-regulation tends to be particularly true for pupils who have problems controlling their behavior and following lessons, for example due to ADHD symptoms.
Good teaching is characterized by the teacher leading the class through the lesson without disruption, encouraging the students to think, taking an interest in them and supporting them individually. The better the teacher is at this, the better the students will be able to regulate their behavior, for example by paying attention, cooperating and adhering to the class rules.
As a result, they learn better. This link, which has already been established in research, has now been examined in more detail in this daily diary study and evaluated with the help of multilevel analyses.
It became clear that the quality of teaching has an impact not only on self-regulation overall, but also in each individual lesson, as Dr. Friederike Blume, lead author of the now published study, summarizes the results.
"When teachers are particularly good at classroom management and providing student support in a lesson, students are better able to regulate their behavior. When these two characteristics of good teaching are not working well in a lesson, students also reported that they were less able to concentrate and engage."
Cognitive activation, the third characteristic of good teaching, was hardly relevant for self-regulation. Therefore, the personal relationship between teacher and student is particularly important, emphasizes Dr. Blume.
This is especially true for students who have difficulties with self-regulation, such as those with attention deficit hyperactivity disorder (ADHD).
"Many teachers find it difficult to establish a positive relationship with children with ADHD symptoms," says the educational researcher. "However, our study showed that in lessons where children with self-regulation difficulties felt particularly supported by their teacher, they were more likely to report being able to concentrate better and follow class rules.
"It is therefore worth taking a positive approach to these children in the classroom and showing a genuine interest in them, as this can reduce the pressure on teachers in the long term and bring more calm to the classroom."
The DIPF researcher also recommends that teachers ask their students for feedback on their teaching from time to time. Although this is still a taboo for many, it can provide valuable information on how to better tailor their teaching to the needs of individual students.
A total of 64 pupils in years 5 and 6 took part in the study. They did not necessarily belong to the same school or class, but were recruited through an email appeal to music schools, sports and leisure centers, for example.
At the start of the study, the children completed a questionnaire about general information such as their grade level and type of school, as well as how they rated their self-regulation skills. Over the next three school weeks, the children answered daily questions about the last lesson of each day.
The questions related to the quality of teaching (e.g., support from the teacher, disruptions in lessons, stimulation of reflection), as well as their ability to regulate themselves in that lesson (e.g., attention, impulse control, motor activity).
The links between the individual lessons and the corresponding daily entries were evaluated using multilevel analysis. Among other things, the results were analyzed on an intrapersonal level, which allows conclusions to be drawn at the level of the individual child. In addition, interpersonal associations were examined, which allows conclusions to be drawn about all participants together.
Limitations of the study
Studies with such an elaborate design, involving daily diaries, always aim to collect data in as short time as possible. As a result, teaching quality was only measured here on the basis of only few statements, which certainly do not cover all the characteristics of good teaching.
Future studies should therefore take a closer look at classroom interaction processes to explore which features of teaching are particularly beneficial, especially for children with stronger ADHD symptoms.
Furthermore, future studies must show whether the results found here apply to all subjects or only to certain subjects, and the role of different teaching methods.
Journal information: Learning and Instruction
Provided by Leibniz-Institut für Bildungsforschung und Bildungsinformation
Explore further
Feedback to editors

A golden layer unlocks sharper imaging and faster scanning with X-rays

From roots to resilience: Investigating the vital role of microbes in coastal plant health
12 hours ago

Temperature, time and blueberry wine: Researchers examine fermentation's effects on health-promoting compounds

Heating proteins to body temperature reveals new drug targets

What fire ants can teach us about making better self-healing materials
13 hours ago

Robotic 'superlimbs' could help moonwalkers recover from falls

A novel multifunctional catalyst turns methane into valuable hydrocarbons
14 hours ago

NASA's Juno provides high-definition views of Europa's icy shell

New research addresses alleged benefits of a vegan diet for dogs

Trees on a university campus endure droughts with help from leaky pipes
15 hours ago
Relevant PhysicsForums posts
Is "college algebra" really just high school "algebra ii".
May 14, 2024
Physics education is 60 years out of date
May 13, 2024
Plagiarism & ChatGPT: Is Cheating with AI the New Normal?
Physics instructor minimum education to teach community college.
May 11, 2024
Studying "Useful" vs. "Useless" Stuff in School
Apr 30, 2024
Why are Physicists so informal with mathematics?
Apr 29, 2024
More from STEM Educators and Teaching
Related Stories

Virtual pupils make for more confident teachers
Jun 22, 2021

Small class sizes not better for pupils' grades or resilience, says study
Mar 8, 2024

Explicit socioemotional learning can have a key role in physical education lessons, study says
Mar 13, 2024

Study finds clear instruction, parental support predict students' sense of school belonging
Mar 27, 2024

How do we help students from disadvantaged backgrounds feel confident about school?
Apr 1, 2024

Positive teacher-student relationships lead to better teaching
Mar 8, 2022
Recommended for you

Investigation reveals varied impact of preschool programs on long-term school success
May 2, 2024

Training of brain processes makes reading more efficient
Apr 18, 2024

Researchers find lower grades given to students with surnames that come later in alphabetical order
Apr 17, 2024

Earth, the sun and a bike wheel: Why your high-school textbook was wrong about the shape of Earth's orbit
Apr 8, 2024

Touchibo, a robot that fosters inclusion in education through touch
Apr 5, 2024

More than money, family and community bonds prep teens for college success: Study
Let us know if there is a problem with our content.
Use this form if you have come across a typo, inaccuracy or would like to send an edit request for the content on this page. For general inquiries, please use our contact form . For general feedback, use the public comments section below (please adhere to guidelines ).
Please select the most appropriate category to facilitate processing of your request
Thank you for taking time to provide your feedback to the editors.
Your feedback is important to us. However, we do not guarantee individual replies due to the high volume of messages.
E-mail the story
Your email address is used only to let the recipient know who sent the email. Neither your address nor the recipient's address will be used for any other purpose. The information you enter will appear in your e-mail message and is not retained by Phys.org in any form.
Newsletter sign up
Get weekly and/or daily updates delivered to your inbox. You can unsubscribe at any time and we'll never share your details to third parties.
More information Privacy policy
Donate and enjoy an ad-free experience
We keep our content available to everyone. Consider supporting Science X's mission by getting a premium account.
E-mail newsletter

IMAGES
VIDEO
COMMENTS
Many first-year college students are unprepared for the academic rigors of college, with as few as 27% of American high school students demonstrating proficiency in English, reading, mathematics, and science on the ACT college entrance exam ().College students may rely on study habits they have developed throughout their elementary and secondary education which served them sufficiently in the ...
Study habits are at the core of a learner's academic success. It is an action like reading, taking notes, conducting study groups that students perform frequently, and regularly accomplishing the ...
Introduction. Academic performance of students is one of the main indicators used to evaluate the quality of education in universities. 1, 2 Academic performance is a complex process that is influenced by several factors, such as study habits. 2 Study habit is different individual behavior in relation to studying 3 and is a combination of study method and skill. 4 In other words, study habits ...
As predicted, structural equation modeling (SEM) indicated that study self-efficacy mediated the study habits—procrastination relation. The mediation effects were medium to large. We conclude that training of, and advice on, study skills and habits should be accompanied by measures that build study self-efficacy.
Students relied significantly less on low-utility strategies and significantly more on moderate and high-utility strategies at the end of the semester than at the beginning. Furthermore, students who completed this assignment outperformed their peers in a control semester of the same course, improving by about one-third of a letter grade.
This paper reports the results of a meta-analysis of 52 studies that investigated the relationship between a range of study strategies and outcomes measures.Low correlations were found between a range of different types of study skills and various outcome measures. Having many study skills (i.e. versatility), as assessed by total study skills scores, produced the largest correlations with both ...
In this study, we described students' self-reported study habits and related those habits to their performance on exams. Notably, in these analyses, we controlled for potential confounds, such as academic preparation, self-reported class absences, and self-reported total study time. First, we found that, on average, students used ...
The multi-disciplinary nature of science, technology, engineering, and math (STEM) careers often renders difficulty for high school students navigating from classroom knowledge to post-secondary ...
Habits are critical for supporting (or hindering) long-term goal attainment, including outcomes related to student learning and well-being. Building good habits can make beneficial behaviors (studying, exercise, sleep, etc.) the default choice, bypassing the need for conscious deliberation or willpower and protecting against temptations. Yet educational research and practice tends to overlook ...
Study habits drive a large portion of how university students study. Some of these habits are not effective in fostering academic achievement. To support students in breaking old, ineffective habits and forming new, effective study habits, an in-depth understanding of what students' study habits look like and how they are both formed and broken is needed. Therefore, in this study, we ...
The study utilized a descriptive-correlational design to examine the impact of study habits to the academic performance of 137 senior high school students. The study made use of the Palsane and ...
The study adopted a descriptive survey research design as its plan. The sample of the study constituted of 1050 senior secondary school students drawn from ... important to ditch bad study habits and establish good ... the study habits which make students successful in their studies after developing and applying them throughout their academic ...
RESEARCH REVIEW International Journal of Multidisciplinary 2021; 6(5):132-135 ... disruption and assigning a high importance to study. Flippo and Caverly (2000) "Study habits are the learned patterns of studying that ... (Kashinathrao, 2012). Students with right hemispherity have better study habits than students with left hemispherity ...
How do study habits affect the academic performance of senior high school students in Cagraray Island, Philippines? This quantitative study investigates this question using a survey of 120 ...
Hence, Issa et al. (2012) explored that students' reading patterns vary and have a moderately significant influence on academic success, while Bashir and Mattoo (2012) examined that academic performance is dependent on the level of students' study habits; thus, reading habits influence on future success, which was confirmed by Owusu-Acheaw ...
Study habits are at the core of a learner's academic success. It is an action like reading, taking notes, conducting study groups that students perform frequently, and regularly accomplishing the learning goals. It can be defined as effective or counterproductive based on whether it serves the students well. Thus, the study's primary purpose was to determine the relationship between study ...
Study habits are the well-planned intended methods of study, the chain of approaches in the process of memorising, systematizing, regulating, retaining novel facts and ideas related to the learning materials, which has gained the shape of consistent endeavours on the part of students, towards comprehending academic subjects and qualifying examinations. The constant practices a person utilizes ...
Exploratory research is intended to examine poorly understood phenomena and generate new insights and hypotheses that can guide future research on the topic (Slavin 2002; Stebbins 2001).In this exploratory study, we examine the study habits of a group of STEM students, with a focus on describing the lived experiences and subjective interpretations of individuals and groups or what cultural ...
Additionally, enhancing students' study habits are relevant, especially in note-taking, reading ability, and health, thus improving their academic performance. Keyword: Study habits, Senior High School Learners, Palsane and Sharma Study Habit Inventory, Academic Performance 1. INTRODUCTION Study habits are at the core of academic success.
Neal (2007) conclude that study habits are approaches applied to learning. Understandably, good study habits assist students to apply their thought processes to identify relevant bodies of knowledge and evaluation of same. Conversely, poor study habits impede students' capacity to engage in constructive intellectual exercise.
Studies conducted after the 70's make use of this construct to evaluate what habits of students at different levels have. The majority of studies have been conducted with students in primary and secondary education levels, some with junior-high school students and few with university students. 3.3 Importance of Study Habits Constructs
The link between teaching quality and self-regulation tends to be particularly true for pupils who have problems controlling their behavior and following lessons, for example due to ADHD symptoms ...
This study examined the learning style preferences, study habits and level of academic achievement of students enrolled in applied science courses of Cagayan State University at Lasam, Philippines.
AP Seminar end-of-course exams are only available to students taking AP Seminar at a school participating in the AP Capstone Diploma Program. April 30, 2024 (11:59 p.m. ET) is the deadline for: AP Seminar and AP Research students to submit performance tasks as final and their presentations to be scored by their AP Seminar or AP Research teachers.
The study employed a phenomenological qualitative research design to comprehend the live experiences of accountancy students regarding their study habits. The research was carried out at a state ...
Learning how to self-monitor: Self-monitoring helps you become more aware of what triggers you to eat in the moment and be more mindful of your food choices and portions. It also helps you stay ...
The sample was 213 undergraduate students at the University of Peshawar, Pakistan (102 day-scholars and 112 hostel living students). Student study habits are measured by the Study Habits Inventory ...