Systematic Literature Review of Game-Based Learning: Objectives, Methods, Applications, Measurement Parameters, and Types of Games
Ieee account.
- Change Username/Password
- Update Address

Purchase Details
- Payment Options
- Order History
- View Purchased Documents
Profile Information
- Communications Preferences
- Profession and Education
- Technical Interests
- US & Canada: +1 800 678 4333
- Worldwide: +1 732 981 0060
- Contact & Support
- About IEEE Xplore
- Accessibility
- Terms of Use
- Nondiscrimination Policy
- Privacy & Opting Out of Cookies
A not-for-profit organization, IEEE is the world's largest technical professional organization dedicated to advancing technology for the benefit of humanity. © Copyright 2024 IEEE - All rights reserved. Use of this web site signifies your agreement to the terms and conditions.
Advertisement
Gamification and Game Based Learning for Vocational Education and Training: A Systematic Literature Review
- Published: 12 January 2023
- Volume 29 , pages 1279–1317, ( 2024 )
Cite this article
- Fazlida Dahalan ORCID: orcid.org/0000-0002-0889-1835 1 ,
- Norlidah Alias ORCID: orcid.org/0000-0002-8299-2669 1 &
- Mohd Shahril Nizam Shaharom ORCID: orcid.org/0000-0002-8213-5085 1
15k Accesses
25 Citations
Explore all metrics
Games have been used as a learning tool for centuries. Gamification and game-based learning are becoming more prominent in educational settings for several reasons. When it comes to learning, being focused and immersed can massively improve a student’s experience. The purpose of this study is to map the emerging trends of gamification and game-based learning (GBL) in the Vocational and Education Training (VET) sector. For this purpose, a Systematic Literature Review was conducted through the three most relevant scientific databases – Web of Science, Scopus, ScienceDirect, and PubMed with Google Scholar as a supporting database. The obtained sample was further selected following the PRISMA guidelines with screening and eligibility processes conducted based on inclusion criteria that were defined with consideration to the research’s aim. This review comprised seventeen studies. The findings revealed a growing interest in the Asian continents in research from 2020 onwards. Furthermore, most of the study is centred on vocational schools and colleges in the engineering and healthcare fields. According to the overview, the digital learning platform and simulation technology are the most promising tools used in the research. The findings support the conclusion that gamification and game-based learning can improve academic performance, engagement, and motivation in vocational education learners. As a result, this study suggests that more research is needed to determine the gamification strategies that are most suited for vocational education and learning.
Similar content being viewed by others
Gamification in Education: A Literature Review
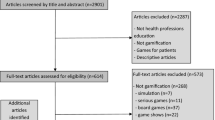
Gamification of health professions education: a systematic review
Critical Perspective on Gamification in Education
Avoid common mistakes on your manuscript.
1 Introduction
Technology is growing at a rapid pace nowadays, allowing for faster change and advancement, and those wanting to remain at the helm of innovation must adapt. The rising dependency on technology, most notably the internet (Sufian et al., 2020 ), around the world contributed to convenience, reduced cost (Dhirendra Kumar, 2015 ; Gagnon & Gagnon, 2021), and has created the illusion of a smaller world (Alghamdi et al., 2020 ). Emerging technologies like sophisticated robots, artificial intelligence, and blockchain are accelerating global transformation at a rate never seen before (Economic Planning Unit, 2014 ; Omar et al., 2022 ) and unavoidably transform the world of employment. An analysis of various National Classification of Occupations (NCO) data revealed the creation of 54 new job titles out of 2,945 (1.8 per cent) in India between 1968–2004; 114 out of 3,600 (3.2 per cent) in India between 2004–2015; 26 out of 2,338 (1.1 per cent) in Malaysia; 18 out of 498 (3.6 per cent) in the Philippines; and 16 out 506 (3.2 per cent) in Vietnam (Khatiwada & Veloso, 2019 ). These new job titles that mainly emerged in the professional, technical, and associate professional divisions demand a higher degree of skills and competence. Thus, educational systems at all levels, most notably vocational education and training, must evolve with the technology to adequately prepare current and future workers for transitioning to high-skilled jobs. However, current education systems are ill-equipped to meet the changing skill needs (Ra et al., 2019 ).
In recent years, numerous technological advancements have been made in the educational sphere, allowing for the invention and development of effective teaching and learning models capable of fulfilling the expectations of future employment requirements (Garzón-Artacho et al., 2021 ; International Labour Organization, 2020 ; Yunos et al., 2017 ; Zabolotska et al., 2021 ). Even prior to the pandemic, education was transitioning toward a digital-first environment, and it was a challenge to educational institutions. The digital transition is a convergence of digital and pedagogical technologies in order to create digital educational materials, digital didactics, and other cutting-edge pedagogical strategies with the aim of increasing student engagement and satisfaction (Martin & Bolliger, 2018 ; Sage et al., 2021 ), boost psychological needs towards motivation (Chiu & Lim, 2020 ; Reeve, 2018 ), promote inclusion, improve feedback practises, accelerate and deepen leaners' comprehension of a subject, and make educational activities more learner-centred (Shagataeva, et al., 2021 ; Zabolotska et al., 2021 ).
The migration of face-to-face instruction at schools towards online learning due to the Covid-19 pandemic has affected approximately 95% of the world’s student population, resulting in the largest education interruption in history and opening doors to student-centred learning (Engzell et al., 2021 ; United, 2020 ). The COVID-19 outbreak is swiftly illustrating why education technologies should be a critical component of teaching and learning since they play a critical role in delivering education to students outside of school as well as embracing the changes and uncertainty. Malaysia's government, without exception, promptly implemented a ‘Movement Control Order’ on 18 March 2020 and executed online learning migration as a new norm to halt the spread of disease and alleviate the burden on the health system (Sia & Adamu, 2021 ). Even though Malaysia was well prepared due to its rapid attempts to develop a policy framework, (Organisation for Economic Co-operation and Development (OECD), 2021 ), 27% of 18 digital education experts based in 10 different EU countries and Malaysia indicated that the digitalisation of offline education was the most challenging during the COVID-19 outbreak (DEL4ALL, 2020 ). It developed certain drawbacks, including restricted social connection, increased technological costs and scheduling, decreased assessment efficacy, and instructor’s competency skills (Dhirendra Kumar, 2015 ; Yasak & Alias, 2015 ; Yeap et al., 2021 ).
Despite our discussion of Education 3.0 and Education 4.0, the reality is that many countries, including those developed, lack even basic and continuous internet access (Vijayan, 2021 ). Even in affluent countries' education systems, lack of instructors' digital readiness, supporting infrastructure for online learning and low Internet connectivity or coverage have been identified as concerns (Baser et al., 2021 ; Garzón-Artacho et al., 2021 ; Shagataeva et al., 2021 ; Wardoyo et al., 2021 ). However, Dubé and Wen ( 2022 ) identified seven clusters of technology forecasts for the most prominent educational technologies from 2011 to 2021, based on data from seven Horizon Reports published between 2011 and 2017. The technologies are: (i) mobile technology, (ii) maker technology, (iii) analytics technology, (iv) games, (v) simulation technology, (vi) artificial intelligence (AI), and (vii) other technologies. The research anticipated that mobile technology would be the most prominent educational technology from 2011 through the near future. Maker technology and games, respectively, were forecasted to have an influence on education from 2015 to 2018 and 2012 to 2016. The impact of analytics technology was projected to grow and influence learning, alongside other technological advancements such as Virtual Reality (VR) and Artificial Intelligence (AI). Therefore, the aim of this study is to perform a systematic literature review of the current trends and patterns in educational gamification and GBL for vocational education, as well as to identify the most effective educational gamification or GBL projects that may be replicated in the future.
1.1 Vocational Education and Training (VET)
Vocational Education and Training (VET) encompasses formal, non-formal, and informal learning that equips individuals with the requisite information and skills for the workplace. The term “vocational education and training (VET)” refers to the education, training, and development of skills in a wide variety of occupational domains, production, services, and lifestyles (UNESCO, 2015 ). It focuses on developing a new skilled workforce that possesses both technical and interpersonal abilities, existing workers who wish to improve their employability through continued skill development, and students who intend to pursue higher education in the future which can help countries improve their economic development and remain competitive in a globalised world (OECD, 2019 ). Technical and vocational education and training (TVET) is a word that is frequently used interchangeably with vocational education and training (VET) in the Asia–Pacific area, including Malaysia (National Centre for Vocational Education Research, 2020 ). VET programmes can be either mainly school-based or work-based.
The curriculum of TVET in Malaysia is designed in accordance with the National Occupational Skills Standards (NOSS) geared toward improving the quality as well as productivity of the country’s skilled labour force (Department Skills Development, 2022 ). NOSS is a document that outlines the knowledge, skills and behavioural competencies related to occupations and is developed based on industry requirements. TVET adopt Competency-Based Education/Learning (CBE/L) principles in the vocational training system in Malaysia which entails shifting emphasis from an instructor-centred approach toward a trainee-centred approach. Statistically, Kulyk et al. ( 2022 ) discovered that the labour market's demand for competent professionals in vocational education is increasing each year. Malaysia’s Budget 2018 allotted RM4.9 billion to seven ministries and agencies involved in TVET to develop a highly trained and competitive workforce (Abdul-aziz et al., 2020 ).
However, due to the industry's emphasis on practical skills and hands-on training, online learning was not a favourable choice for the VET instructor (Arnold et al., 2021 ; Yeap et al., 2021 ). The UNESCO-UNEVOC International Centre ( 2020 ) reported that one-third of all respondents in 126 countries did not use e-learning, another third used it occasionally, 17% used it regularly, and only 11% used it very often. Hence, the complete transition to online learning due to the Covid-19 pandemic was felt most acutely in the VET sector (UNESCO-UNEVOC, 2021 ; Yeap et al., 2021 ) where most skill-based institutions were caught off guard by the lack of infrastructure and necessary resources to facilitate online learning (Sia & Adamu, 2021 ). Worse, instructors experienced mental stress because of unprepared situations arising from the preparation, presentation, application, and confirmation stages of online teaching and learning. Previous research lists several issues that plague the VET field (Gaffoor & Van der Bijl, 2019 ). Among the reasons revealed in the study were the instructor’s lack of competency and the students' heavy course load, both of which contributed to the students 'low academic performance. The report found that this might relate to courses being taught more theory-heavy than practical sessions and suggested that learning should be made more entertaining and interesting. An adequate intervention is necessary to address motivational and performance issues in vocational education.
1.2 Instructor Digital Competence
Digital competencies empower instructors to maximise digital technologies' potential. To facilitate the teaching–learning process of students and foster the development of important skills, it is required for instructors to have up-to-date training and a specific degree of digital competence. In Malaysia, concerns about the capabilities of TVET lecturers in terms of their proficiency, credentials, and skills competence have been a focus of the country's instructor education program (UNESCO, 2021 ). In recent years, the instructor education program has seen the emergence of new methodological perspectives due to technological resources and mobile connections (Gómez-Carrasco et al., 2020 ). Having a qualified instructor with a high level of digital competence and technological proficiency is substantial in VET training programmes (Kulyk et al., 2022 ; UNESCO-UNEVOC, 2020 ; Yasak & Alias, 2015 ; Zabolotska et al., 2021 ). In his systematic review related to teaching and learning during the COVID-19 pandemic, Vijayan ( 2021 ) strongly suggested that future instructor education programmes should integrate technological pedagogy as many have faced a high learning curve in giving fundamental classes during the Covid-19 pandemic period. This is supported by Alonso-García et al., ( 2019 ), Garzón-Artacho et al., ( 2021 ) and Li et al., ( 2019 ), which found that instructors must undergo hands-on experiences training involving the acquisition of new technological skills and pedagogical tactics to improve their professional competency in the educational environment, and thus direct education toward these sustainability principles.
Early evidence provided by Wardoyo et al. ( 2021 ) proves that an improvement in students learning outcomes can be achieved through a good understanding of technology by students, high educator competence and good computer skills. When compared to practical skills, the implementation of ICT among VET students is more effective at developing cognitive learning (Yasak & Alias, 2015 ). However, no correlation has been found between the cognitive dimension of learning objectives and the level of knowledge about teaching techniques (Yousef & Sumner, 2021 ). According to The Digital Competence Framework, key components of digital competence are identified in five areas: information literacy; communication and cooperation; creation of digital content; security; and problem-solving (Alessandro Brolpito, 2018 ; European Commission, 2022 ). These conditions place critical demands on VET stakeholders to train and reskill future and active VET instructors for the ever-evolving technological needs of teaching and learning.
1.3 Gamification and Game-Based Learning (GBL)
According to the International Labour Organisation ( 2020 ), six digital learning areas offer great benefits for VET: distance learning and assessment, simulation, flipped classrooms, gamification, open education (resources) and personalisation. BlueWeave Consulting reported that the global education gamification market reached $697.26 million in 2020 (Blueweave, 2021 ). By the end of 2027, it is expected to expand at a CAGR of 29.00 per cent, bringing in revenue of approximately USD 4.145 billion. The phenomenal expansion of smartphones and mobile devices has directly resulted in the creation of a massive market for gamification. Hence, VET Instructors need to improve digital competency from technologies such as gamification and next-generation digital learning environments (NGDLE) (EDUCAUSE, 2017 ) to motivate and engage students in purposeful learning activities through the design of the online or situated learning environment (Johnson et al., 2018 ; Madimabe & Omodan, 2021 ; Pittaway & Moss, 2014 ). NGDLE is envisioned as an ecosystem of learning tools and components according to common standards that may be constructed in any way a person or institution desires (EDUCAUSE, 2017 ). Game technologies are related to the way digital games can be used to facilitate learning, namely game-based learning and gamification.
Gamification is one of several innovative constructivist approaches (Roodt & Ryklief, 2019 ) to learning that has gained significant attention in recent years in a variety of discipline areas, including commerce, employment, health, the environment, and most recently, our subject of research, education (Manzano-León et al., 2021 ; Wang et al., 2021 ). It is defined as “game-based mechanics, aesthetics and game thinking to engage people, motivate action, promote learning, and solve problems” (Kapp, 2012 ). Generally, everything incorporated in the creation of a game is referred to as a "game element" (Man, 2021 ), and a wide range of game elements have been used in the design of games. However, Werbach and Hunter ( 2012 ) argue that game elements exist in a hierarchy consisting of components, mechanics, and dynamics. They analysed more than a hundred gamification implementations and discovered that a significant proportion of them incorporated points, badges, and leaderboards (PBL). There is, in fact, evidence to suggest that when digital game elements such as avatars, points, badges, and leaderboards are used to achieve specific learning goals and engage students on emotional, social, and cognitive levels, they are more likely to enjoy the learning process (Gupta & Goyal, 2022 ). However, designing successful gamification applications in education that influence positive behaviour changes is still a riddle (Dichev & Dicheva, 2017 ). For gamification to be effective, individual game elements must be linked to particular behavioural, motivational, or attitude results, which must then be related to learning outcomes (M. Ma & Oikonomou, 2017 ). A failure to establish the connection may result in unsuccessful gamification initiatives.
Game-based learning (GBL) is the union of educational learning theories, course curricula, and digital gameplay with the goal of enhancing the learning experience (Jayasinghe & Dharmaratne, 2013 ; Roodt & Ryklief, 2019 ). The concept of GBL is fun learning through doing/playing and specifically designed, structured game learning materials which can stimulate the development of thinking skills and self-learning among vocational students (Azizan et al., 2021 ). Serious games are the most common type of GBL used in education which focuses on the development of games with specific educational purposes in mind (Anastasiadis et al., 2018 ; Games & Carvalho, 2022 ), leading to increased enthusiasm for gaming and academic performance (Zhonggen, 2019 ). A serious game is a computer-based program that is designed for both entertainment and learning purposes by simulating real-world scenarios (Kapp, 2012 ) and demonstrates remarkably more effectiveness than non-serious game-based learning (Zhonggen, 2019 ). Dimitra et al. ( 2020 ) identified seven main types of GBL approaches implemented in education: (i) memory games, (ii) simulation games, (iii) interactives, (iv) quiz games, (v) puzzles, (vi) strategy games and (vii) reality testing games. There is a body of research that has focused extensively on the overall impacts of GBL, including improved motivation, engagement, satisfaction and academic achievement among vocational education students (Arnold et al., 2021 ; Balakrishnan Nair, 2021 ; Oliveira et al., 2021 ; Roodt & Ryklief, 2019 ). However, GBL is not widely used in vocational institutions’ practice (Arnold et al., 2021 ), and measures are required to enhance the skills and knowledge of VET instructors, illustrate and contextualise the influence of game components in the classroom, and make suggestions for game features.
Gamification and GBL is an innovative technology which is considered a leading trend in education. Both technologies may seem similar, but they are two distinct techniques with multidimensional relationships (Jayasinghe & Dharmaratne, 2013 ; Krath et al., 2021 ). GBL and gamification are different as GBL incorporates games seamlessly into the educational curriculum to achieve specific learning outcomes. On the other hand, GBL Gamification involves turning the whole learning process into a game using game elements, for example, levels, points, badges, leaderboards, avatars, quests, social graphs, or certificates (Krath et al., 2021 ) . Despite this, the border between GBL and gamification was rather thin at times, especially when both have relatively comparable goals. Both GBL and gamification aim to solve a problem, encourage participants, and increase learning via the use of game-based ideas and tactics.
There are a variety of publications on gamification and GBL in education and professional training nowadays. Each publication focuses on certain issues, elements and technological tools. Saleem et al. ( 2022 ), conducted a literature review to determine the advantages and challenges of gamification applications in e-learning and indicated that gamification can be a valuable tool for gaining knowledge and can improve necessary capabilities such as decision-making, cooperation, and communication. However, gamification in educational activities faces multiple difficulties, such as technological infrastructure, internet service provision, and the intention of both students and teachers to use this tool. Behl et al. ( 2022 ) used bibliometric analysis and PRISMA to present an examination of the emerging trends of gamification and e-learning for young learners and highlighted the four major future research themes of personalisation, game elements, learner styles, and learner engagement. There are reviewed studies that investigate the effect of gamification in certain fields such as mathematics (Y. Pan et al., 2022 ), computer science (Willert, 2021 ) and economics (Platz, 2022 ). In fact, companies are also turning to gamification to make work more meaningful and fun for their employees. Mahat et al. ( 2022 ); Tay et al. ( 2022 ) conducted a systematic literature review to examine the influence of gamification and digital GBL on professional training and upskilling over the past five to ten years, while Thomas et al. ( 2022 ) analysed 34 articles on gamification in Human Resource and Development and identified four areas where gamification has been studied: employee learning, task performance, employee wellness, and rising contexts.
However, the scarcity of research on gamification and GBL in the VET sector makes it difficult to demonstrate the impact of both techniques (Jayalath & Esichaikul, 2020 ). Therefore, this research will conduct a systematic review of studies on both gamification and GBL in the VET sector for the last five years. In performing this review, this study aimed to address the following research questions listed below to recognise the emerging trends and situations within the area of gamification and GBL in vocational education during the last five years, as well as to identify the most effective educational gamification or GBL projects and how to duplicate them in future research.
RQ1: What is the distribution of the studies that examine gamification and GBL for the VET sector from 2018 onwards?
RQ2: What is the educational level where gamification and GBL are most studied?
RQ3: What are the research techniques used to examine gamification and GBL for the VET sector?
RQ4: What are the geographical distributions of publication on both methods for VET programmes worldwide?
RQ5: What is the field of VET research addressed in the study?
RQ6: What are the theories and frameworks discussed in the reviewed studies?
RQ7: What is the game technology mostly used in the reviewed study?
The next section of this paper explains the methodology employed. The third section presents the results of the reviewed studies with reference to the research questions. Finally, practical applications and future research directions are suggested in the discussion section.
This paper presents our systematic literature review performed using the PRISMA guidelines (Page et al., 2021 ). We followed the PRISMA standards (Preferred Reporting Items for Systematic Reviews and Meta-Analyses) guided by the PRISMA 2020 checklist (see Fig. 1 ) to highlight scientific validity and provide an unbiased analysis to summarise the evidence on GBL and VET learners. Systematic reviews are a scientific technique of synthesising all relevant publications and documents to answer a specific research question with minimal systematic error (Mengist et al., 2020 ; Roberts, 2006 ). It concentrates knowledge on particular subjects and identifies areas of uncertainty and prospect fields where little or no relevant study has been conducted, and future additional research is required. The main purpose of our study was to investigate how far academic research on gamification and GBL in VET has progressed and to identify gaps suited for future research. In the present study, the systematic search strategy comprised three major processes: identification, screening, and eligibility.
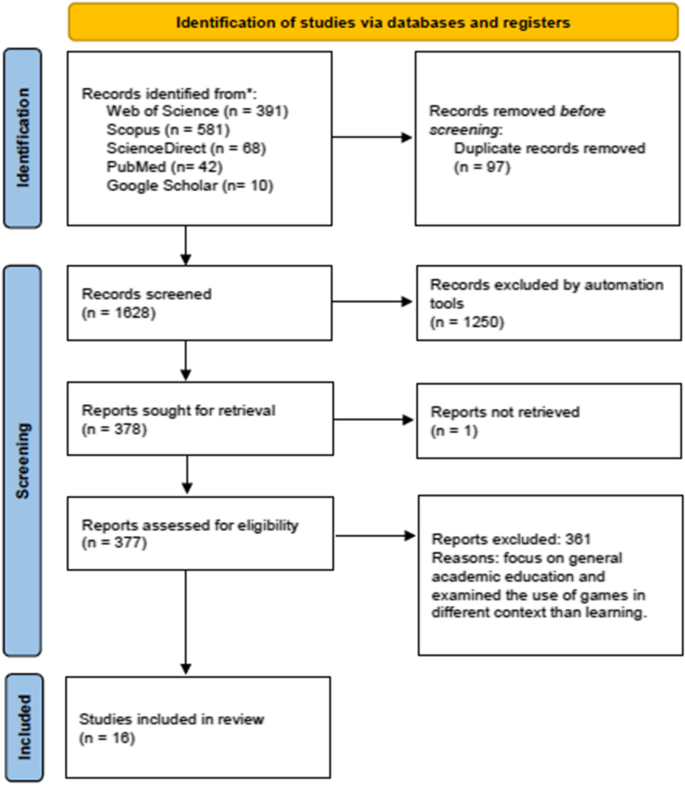
PRISMA 2020 flow diagram for systematic reviews using a template from Page et al. ( 2021 )
2.1 Identification
This phase consisted of a searching and delivery strategy, which assisted in defining a suitable search string and identifying relevant databases to compile the studies to be analysed in the systematic review. The literature search was conducted until February 2022 using four widely renowned databases: a) Scopus, b) Web of Science, c) Science Direct, and d) PubMed, as shown in Table 1 . The specific search strings were developed in accordance with each database’s search logic while preserving the same terminology. A manual search was also performed using similar keywords on Google Scholar to include citations not included in other databases which generated an additional ten articles. There is a gap in the academic literature that includes articles on gamification and GBL in VET. Thus, a total of 1092 articles were gathered in the first stage of the systematic review process. The results were then exported to a table in an Excel sheet for the record. After importing the results, a feature of MS Excel was used to eliminate duplicates automatically. Overall, 97 articles were removed due to duplication, and the remaining 995 articles were then reviewed in the screening stage.
2.2 Screening
The goal of screening is to remove irrelevant articles. The remaining 1628 articles were reviewed according to inclusion and exclusion criteria determined by the researchers (see Table 2 ). The first phase consisted of the screening of titles and abstracts of the studies to identify and label the studies as ‘included’ or ‘excluded’ in the table according to the inclusion and exclusion criteria. Educational technology has rapidly changed every facet of our society, so to avoid including outdated content in the review process, the article search was restricted to those which were published between the years 2018 and 2022. This was further validated by earlier literature reviews that concentrated on five-year review intervals to produce results relevant to the most recent research gaps (Anil Yasin & Abbas, 2021 ; Behl et al., 2022 ; Mahat et al., 2022 ; Manzano-León et al., 2021 ; Rohan et al., 2020 ). Furthermore, only the documents classified as Article and Review and limited to documents with full text available published in English-language studies were included. Moreover, to meet the research objectives, only articles published within the scope of gamification, GBL and VET were selected (see Table 1 ). The screening process resulted in 378 articles considered for the third stage.
2.3 Eligibility
A systematic review's third stage is determining eligibility, which necessitates the researcher to conduct an in-depth manual evaluation of all relevant papers based on the main topics of the research. Studies were included if they described gamification and GBL applied to VET. All studies that met the inclusion criteria were independently evaluated by three reviewers. A total of 362 articles were omitted from the database due to their emphasis on general academic education. Several articles were also omitted since the research articles examined the use of games in a variety of contexts rather than specifically for learning. To ensure the review of the field's research is comprehensive, we have opted to add theoretical works on gamification and GBL in vocational education too. This resulted in a total of 17 articles available for review in the subsequent stage (see Fig. 1 ). However, designing successful gamification apps in education that can sustain the desired behaviour changes is currently more of a guessing game than a science.
2.4 Data Extraction and Analysis
The remaining 17 articles were examined and analysed to provide answers to the posed research questions. The abstracts of the studies were examined in detail, followed by in-depth reading of the entire article to find the most relevant analysis categories. MS Excel was used to categorise key data elements, such as (1) type of publication; (2) context; (3) location; (4) field of study; (5) methodology; (6) sample size; (7) theoretical/concept framework; (8) game design; (9) technology utilised; (10) analysis, and (11) result.
This section reports the findings of 17 studies published on gamification and GBL for VET from 2018 to 2022, as systematised in Table 3 . This section provides the answers to the research questions that had been proposed earlier to identify the current progress of this study.
3.1 Publication Distribution and Educational Level of the Studies.
The study approach placed a year constraint, requiring only publications published after 2018, in order to gather the most recent research on gamification and GBL for VET programmes. Figure 2 illustrates the evolution of the number of chosen published papers over time and by educational level. The amount of research on gamification and GBL in the VET sector has expanded dramatically from 2020 and beyond, demonstrating a rising interest in this strategy for enhancing student motivation, engagement, satisfaction, and academic success. Furthermore, this finding implies that the impact of gamification and GBL in VET is a growing study area. In terms of the distribution of publications by educational level, as shown in Fig. 3 , most of the publications were discovered to focus on vocational school or college, accounting for 76 per cent of all publications, followed by 18 per cent for professional training. Only six per cent of the studies involved samples from both a vocational school/college and a working professional.
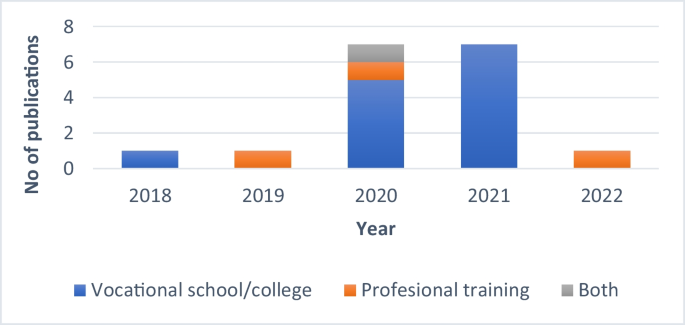
Number of selected published articles per year/education level
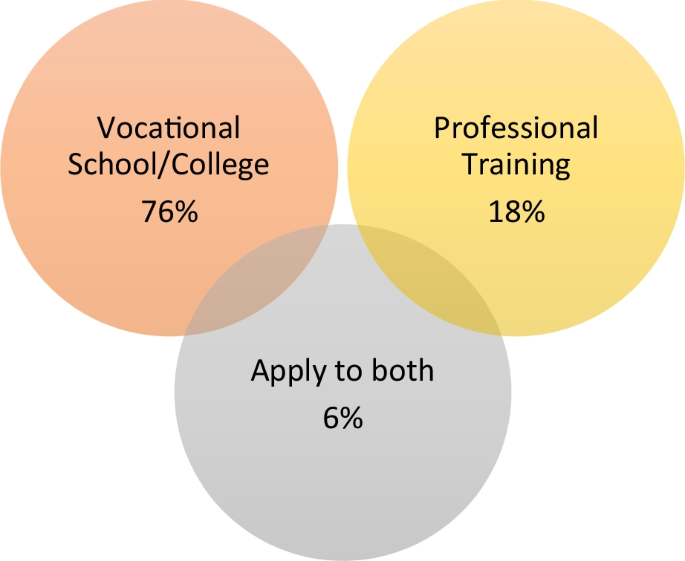
Percentage of publications distribution according to educational level
3.2 Research Techniques used to Examine Gamification and GBL for the VET sector
Scientific research is often divided into two classes: conceptual research and empirical research. There can be no meaningful research outcomes until there is an idea driving the collecting of data or "evidence", and without a concept, there can be no meaningful research results. Unlike theory-based research, empirical research relies on actual experiments. There are thirteen studies (76%) identified as empirical research, and four articles (24%) were identified as conceptual research (see Fig. 4 ).
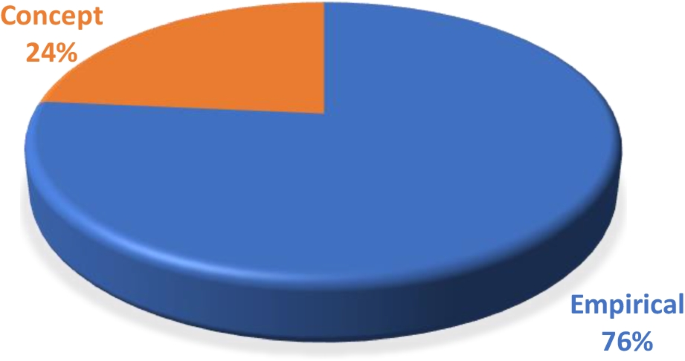
Scientific research type of reviewed studies
3.3 Geographic Distribution of Scientific Contribution
The geographical distributions of the data sources for reviewed publications are shown in Fig. 5 . Research on gamification and GBL for VET has been conducted the most in Asian countries (53%, N = 9), followed by European (29%, N = 5), South American (6%, N = 1), North American (6%, N = 1) and African (6%, N = 1). Surprisingly, no study has been conducted in Oceania. Thailand was the top country in Asia for publishing research in the context of gamification and GBL for VET (N = 4), followed by China (N = 3) and the Netherlands (N = 2). Ireland, South Korea, Germany, Malaysia, South Africa, Columbia, Finland, and the United States contributed to the literature with one study each.
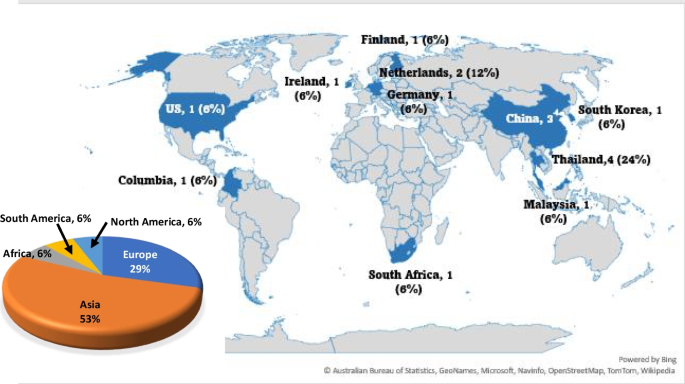
Continents and countries distributions of Gamification and GBL for VET publications
3.4 Field of Research on Gamification and GBL for VET
Notably, the data indicated (see Fig. 6 ) that the most often published articles on gamified vocational education and training (29%, N = 5) are in the fields of computer (N = 2), electrical (N = 1), electronic (N = 1) and automotive and air conditioning courses (N = 1), which are categorised as engineering. Nursing and medical-related studies, which are classified as healthcare, are the second-largest category (24%, N = 4), followed by general subjects (18%, N = 3), where English and Mathematics courses were placed. Studies in food service and tourism are in separate categories. Tourism and all other categories published 12% (N = 2) of the articles on gamified vocational education and training. All categories encompassed studies with an unspecified field, where the gamified activities can cover a wide range of professions. The least frequent domain (6%, N = 1) was food service. Considering the above findings, it is possible to verify that gamification and GBL strategies are applied to a broad range of fields and skills.
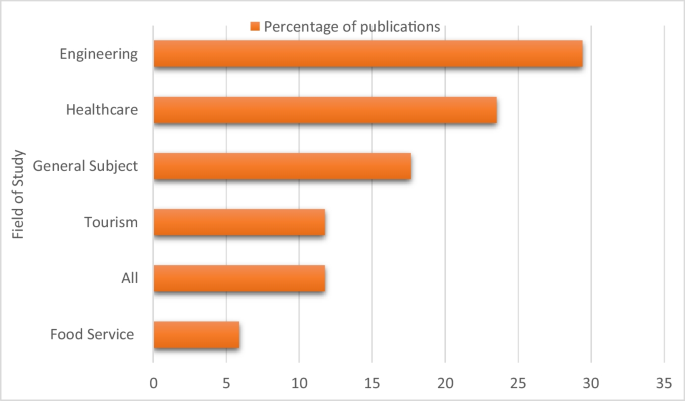
Distribution of the examined studies across the various training sectors
3.5 Theoretical Foundations or Frameworks of the Reviewed Studies
The lack of theoretical frameworks in practical studies contributes to ineffective measurement (Behl et al., 2022 ; Roodt & Ryklief, 2019 ). After synthesising some literature reviews of predecessors, this research found that there is relevant theoretical support for gamification and GBL for the VET sector. The summary of the theories is presented in Table 4 . The present literature builds upon ten theoretical perspectives and instructional design models to promote gamification and GBL in the VET sector. More than half of the studies (59%), as shown in Fig. 7 , discussed their studies from theoretical perspectives and/or were guided by frameworks. Fewer than half of the reviewed studies (41%) did not discuss any theoretical foundations or frameworks, although most reported a positive impact on motivation, engagement, and training performance. Interest-Driven Creator Theory (IDC), Self- Determination Theory (SDT) and ARCS Theory of Motivation were the most common theories found in the reviewed studies (N = 2). Sometimes multiple frameworks were integrated into the reviewed studies.
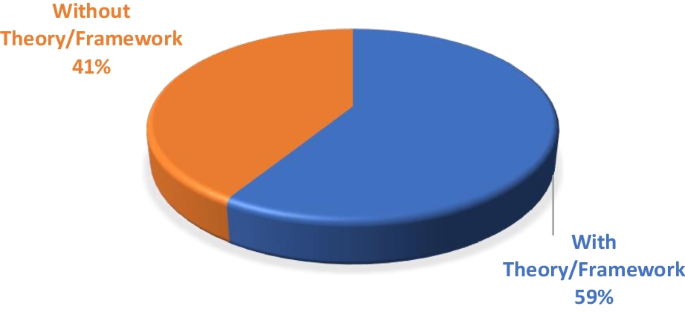
Total reviewed studies incorporating theoretical foundations/frameworks
For instance, the article by Jayalath et al. ( 2022 ) used the ARCS theory of motivation and engagement to design the operational model and gamification design of a blended electronic circuit course using gamified LMS in TVET. Similarly, a randomised controlled study by Ma et al. ( 2021 ) compared the effect of theme games and scenario simulations on the disaster nursing competence of nursing students, and used flow theory combined with the ICN framework of disaster nursing competencies as the guiding theoretical framework. Constructivism is probably the learning theory that offers propositions closest to gamification and GBL. Roodt and Ryklief ( 2019 ) chose the constructivism approach and configured a digital game application called “Millionaire Programmer” to match the motivational recommendations outlined in the ARCS Model. Glover and Bodzin ( 2021 ) developed a Hand Hygiene Serious Simulation Game (HH-SSG) for health professional clinical education by combining multiple theoretical frameworks: Expectancy-Value Theory of Achievement Motivation, Expert Performance Theory, and Self-Determination Theory; and frameworks developed through previous simulation-based learning and serious games research for first cycle coding analysis.
3.6 Game Techniques
Several combinations of technology are used during the application of gamification and GBL for the VET sector. From the reviewed literature, the author identified five game technologies used, as shown in Table 5 . Three studies (Garcia-Iruela et al., ( 2021 ); Jayalath et al., ( 2022 ); Tongpaeng et al., ( 2019 )) employed the Moodle application, which can support MOOC as their learning management system to deliver online courses, while two studies (Kladchuen and Srisomphan, ( 2021 ); Kummanee et al., ( 2020 )) designed a problem-based learning model which consisted of a digital learning platform in the early design stage. Four studies used an enriched experience in the real world and virtual settings in their educational delivery. In two studies (Wang and Khambari, ( 2020 ); Wang et al., ( 2021 )), AR technology that combines virtual information with the real world to teach English was used. Another two studies (Lerner et al., ( 2020 ); Bernal et al., ( 2022 )) developed serious games using VR and Immersive VR (IVR) technology for professional training in medical centres and power substations.
Four studies were categorised under roleplay and simulation technology. Three studies developed a serious simulation game: (1) Ma et al. ( 2021 ) developed a theme game for nursing students called “Brave the Wind and Wave” played in a simulated training room; (2) Glover and Bodzin ( 2021 ) developed the “Hand Hygiene Serious Simulation Game (HH-SSG)” for healthcare programme training played in a simulated hospital environment; (3) Hämäläinen et al., ( 2018 ) developed a virtual 3D serious game dubbed "Game Bridge" that utilises collaborative game mechanics to promote constructive knowledge production among vocational students. In a different method, Wouters and Van Der Meulen’s ( 2020 ) studies used a cartoonlike 2D game called “Zeldenrust”, which can also be characterised as a combination of a simulation game and a role-playing game.
A study by Habes et al. ( 2020 ) was classified as interactive media. The authors built an interactive video-based game and narrative experience called "Serioussoap.nl" in collaboration with two young, well-known Dutch soap opera actors in order to better understand the influence of geriatric information on home care nurses or nursing students. (Azizan et al., 2021 ; Balakrishnan Nair, 2021 ) They used online quizzes as GBL learning activities in tourism, automotive, and air-conditioning courses, whereas Roodt and Ryklief ( 2019 ) used a web application called "Millionaire Programmer" to teach and assess students' knowledge of computer programming.
Figure 8 illustrates the technology used in the reviewed studies. The majority of the research made use of LMS/MOOC-based gamification (29%). AR/VR, role-playing games, and simulation technology each contributed about 25% of the approaches used in the study of literature. Browser games technology employed 18% of the reviewed literature. The least-used technology was interactive media, which contributed to 6% of the study.
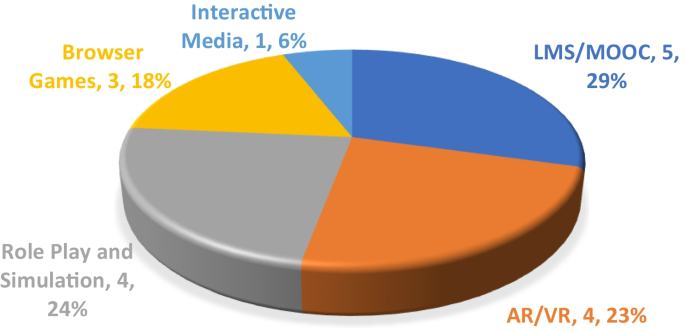
Percentage of technology employed in reviews studies
4 Discussion
The movement from traditional to digital learning has increased significantly in 21 st -century education. Traditional learning methods are being enhanced with agile, collaborative, and technology-based education. Gamification and game-based learning in vocational education is a growing trend that involves introducing game design aspects into educational environments to increase learners' motivation and engagement. The reviewed studies provide evidence to indicate that gamification and game-based learning has a promising future in vocational education and training. The systematic review identified 17 journal articles from different countries with different contexts and technology.
4.1 Publication Distributions in Time and Educational Level of the Studies
With reference to the distribution of publications on gamification and GBL for VET, the reported findings indicate that since 2020, gamification and GBL in vocational education and training have been a vibrant and rapidly rising topic of study, with an ever-increasing level of interest. We can see a surge in the number of publications between 2019 towards 2020 and onwards. This is probably because most of the current evidence supports the use of gamification and game-based learning as a powerful tool for improving and enhancing the quality of teaching and learning in vocational education due to the difficulties and disadvantages of conventional classroom instruction (Chan & Lo, 2022 ; Gupta & Goyal, 2022 ; Rodrigues et al., 2022 ). In addition, a growing number of vocational institutions including community colleges, polytechnical institutes, apprenticeship institutes, and vocational training schools are striving to expand their reach beyond geographical and social barriers where modern game-based learning platforms are specifically designed to suit all types of learning environments and provide students with access to the most favourable educational and job opportunities (The World Bank Group, 2021 ).
Competency-based education (CBE) is developing as a viable approach for educating students for global competence. However, the emergence of educational gamification approaches in the sector of vocational education was slower than in the academic field, where the topic of gamification has blossomed and expanded dramatically since 2013, with hundreds of relevant papers released each year (Charlo et al., 2022 ). The reason for this may be due to the fact that instructors in vocational institutions are only moderately prepared to use the gamification approach due to their lack of experience and time in developing gamification-based applications and preparing teaching and learning materials, and their lack of knowledge of ICT (Omar et al., 2022 ). Hence, it is crucial for TVET instructors to have the pedagogical competence to match training with the used modality. Skilled instructors/trainers are essential for assuring quality and sustaining training standards, which improve the employability of students. The graph shows a reduction during the final year (2022), but since many publications are still in the process of indexation in the databases, it cannot be said that interest in the topic has already passed its peak. In support of this, O’Neill ( 2022 ) reported that game-based learning and education have been predicted to generate revenue of more than USD24 million by 2024. This demonstrates that gamification and GBL are useful delivery strategies for vocational training and upskilling, and their use is expected to increase.
Considering the educational level, the most significant number of studies in the survey period were conducted at vocational schools or colleges (13 papers), with less attention to professional training (3 papers). Only one research makes use of both sample populations. A possible explanation for this disproportion is that there are a large variety of accessible game-based platforms and applications on the web market that integrates different engagement elements, making them easier for vocational educators to explore/experiment with their learners. With the advent of the internet, mobile applications, and gamification, educational activities have evolved into e-learning to facilitate learning and make it an entertaining experience. Some of the most popular gamified apps in the educational sector are Gimkit, BookWidgets, Edmodo, iSpring, Scratch, Kahoot, Quizlet, Plickers, Genially, Articulate 360, H5P, Thinglink and many more. While there are over thousands of educational web apps available, choosing the right ones for students will make them enjoy learning. To achieve this, the instructor must be technologically savvy or possess the requisite computer-related abilities to apply the GBL. However, there is a lack of comprehensive research devoted to the development of TVET instructors’ digital competence in using a range of educational technologies, particularly gamification and GBL, in professional activities, which allow contributions to the formation of professional competencies among students. Thus, further research on developing the digital competence of TVET instructors in various educational technologies by employing hands-on professional training, particularly in using gamification and GBL in professional activities, is necessary.
It can be acknowledged that studies including diverse demographic groups are helpful, as we cannot always generalise the results of research involving one demographic group to another. In the literature review on gamification prospects in industry 4.0, Reis et al. ( 2020 ) assume that the possibility of research limits for professional training is attributable to the fact that many firms do not transfer the outcomes of adopting gamification into the scientific database. This suggestion is backed up by the Gartner analysis, indicating that more than 70 per cent of the Global 2000 organisations have already used gamification (Softek, 2017 ). However, it remains unclear if most of the firm’s emphasis on professional training is connected to the TVET industry. Based on a review study by Mahat et al. ( 2022 ), education is not the most common field for gamification in professional training, since research on gamification in education has mostly focused on students and adults rather than instructors. Nevertheless, since the primary objective of vocational education is to prepare graduates for employment, it must include specialised training that tends to be creative-reproductive in accordance with industry requirements. In addition, it is essential to determine TVET instructors' perceptions, readiness, and competence skills to embrace the gamification and GBL strategy. Therefore, future research agendas should prioritise the development of additional empirical studies that confirm gamification and GBL's usefulness for professional training in the vocational education sector, and this should include educating TVET instructors by providing them with hands-on gamified professional training experience.
4.2 Research Techniques and Countries Distributions
From the reviewed articles, 13 studies (76%) were identified as empirical research, and four articles (24%) were identified as conceptual research (see Fig. 4 ). Since gamification and GBL are relatively new concepts in the VET sector, the amount of research is very limited, especially in experimental studies. Two conceptual research (Kladchuen and Srisomphan, ( 2021 ); Kummanee et al., ( 2020 ) synthesis of previous research documents constructed a problem-based learning management model in connection with the gamification technology in a digital learning environment to produce a vocational innovator. Meanwhile, Jayalath et al. ( 2022 ) presented a thorough design and operational model for gamified blended learning courses in TVET with the goal of increasing motivation and participation. Additionally, Glover and Bodzin ( 2021 ) reported a learner-centric design of a hand hygiene simulation game built for non-game-oriented grade 12 female CTE EHP students based on the results of two learner studies. Future empirical studies on gamification and GBL in the TVET sector should therefore be conducted to generate more results.
The presented results indicate that the Asian continent contributed the most publications on gamification and GBL for VET (53%, N = 9). Although Thailand leads the research, it is not dominated by a single country or group of countries, as published works in the research area are extremely limited, and only three countries (Thailand, China, and the Netherlands) contributed slightly more studies than the remaining thirteen countries. However, this finding is consistent with the gamification education market report, which indicates that Asia–Pacific is predicted to develop at the highest pace during the forecast period, owing to increased education sector investments in countries, which is boosting the worldwide education gamification market in APAC nations (Market Data, 2022 ). This region's fast-rising economies are also focused on closing the skilled labour supply–demand imbalance, where several initiatives are being developed to boost technical sector VET and enhance discretionary income (Meticulous Market Research, 2022 ). It is anticipated that these actions would enhance vocational education and expand market prospects for major firms in this region.
The worldwide education gamification market is classified geographically into North America, Europe, Latin America, Asia–Pacific, the Middle East and Africa, and the rest of the globe. North America accounted for nearly 37% share of the global gamification in the education market in 2018 and is expected to dominate the market throughout the forecast period (Market Data, 2022 ). Technavio analysts forecast the technical and vocational education (TVE) market in North America to grow at a CAGR of 4.13% during the period 2016–2020 (Technavio, 2016 ). However, North America contributed only one publication on the reviewed studies. This is probably because the importance is given to the region's widespread availability and penetration of digital services rather than publications on journal databases. It would be useful to investigate how developed countries embraced gamification and GBL in their vocational education sector, especially in the technology innovation of The Industrial 4.0 revolution. As a result, future research on gamification and GBL in the TVET sector should be undertaken across cultures more extensively, as various organisations and geographical regions may provide different findings.
4.3 Field of Study
The collection of papers is divided into five sections and covers a wide variety of occupational fields. Gamification and GBL can be applied in any industry, yet the engineering field seems to generate the most articles on gamification and GBL in VET. This is likely because the diverse range of engineering field courses includes subjects that are more closely related to technical abilities. In the literature review on the role of gamification in engineering education, Anil Yasin and Abbas ( 2021 ) indicated that gamification strategy using electronic-based gamification tools or digital gamification is more effective and an improvement over traditional teaching methods. However, most engineering disciplines require a minimum of seven years of formal study to achieve an average level of technical proficiency, while a vocational type of education is designed to produce “job-ready” industrial workers at all levels from entry to management. In a professional setting, students learn how to apply their knowledge in a real-world context, allowing them to step immediately into the workforce and begin their careers.
Gamification in healthcare, too, is gaining momentum as the industry is experiencing challenges that have prompted medical institutions worldwide to adopt modern technologies and become more user-centric to better cope with stakeholders’ increasing demands and needs. Three studies on healthcare (Ma et al., ( 2021 ); Lerner et al., ( 2020 ); Glover and Bodzin, ( 2021 )) used serious simulation game training to enhance the quality of healthcare training, while one study by Habes et al., ( 2020 ), conducted an explorative pilot study to examine the effect of developed gamified video storytelling on the geriatric knowledge of nursing students and home-care nurses. Another interesting observation is the use of GBL in general subjects (English and Mathematics) on vocational education learners (Wang and Khambari, ( 2020 ); Wang et al., ( 2021 ); Wouters and Van Der Meulen, ( 2020 )). Additionally, this positive finding will assist with the overcoming of numerous obstacles during this learning process since most vocational learners are more competent in practical learning. The food service and tourism industry is a service industry which emphasises experiences co-created by customers or tourists and service providers very much. From the reviewed literature (Hämäläinen et al., ( 2018 ); Tongpaeng et al., ( 2019 ); Balakrishnan Nair, ( 2021 )), it can be concluded that the food and tourism industry may also employ gamification and GBL as an external marketing, sales, and customer engagement tool, as well as an internal training and productivity tool (Pasca et al., 2021 ; Xu, 2013 ). Nonetheless, only a small number of researchers have investigated these topics in the TVET sector, although vocational education is a lifelong education comprised of a wide variety of fields that focus on developing the skills of adults and responding to the labour-market needs of the economy. Therefore, additional studies should be performed to examine the applicability of gamification and GBL in diverse domains of vocational education and to determine their effectiveness.
4.4 Theoretical Framework
This systematic literature review showed that studies on gamification and GBL for VET have so far used a variety of 12 different theories. Over half of the assessed studies employed at least one theoretical framework as a guide. Table 4 outlines all the theories mentioned in the analysed reviews, together with the total number of primary research studies conducted based on each theory. In scientific research, gamification or GBL design may be described using several theoretical foundations, including motivation, behaviour, or learning theories (L. Pan et al., 2021 ). The most recognised quality of gamification and GBL is their motivating value. Most of the studies evaluated are concerned with motivational effect, including ARCS theory of motivation, expectancy-value theory of achievement motivation, flow theory and self-determination theory. Engagement theory, interest-driven creator theory, Felder and Silverman’s learning styles model and expert performance theory describe the determinants of behavioural outcomes, while constructivist theory and cognitive load theory are theoretical foundations that deal with the processes of learning. The ICN framework of disaster nursing competencies and building information modelling propose guidelines for system design. The majority of the theoretical foundations examined in the research effectively demonstrated a beneficial effect on vocational learning through gamification and GBL. However, there is minimal consistency regarding theoretical foundations and the scope of gamification (Seaborn & Fels, 2015 ).
4.5 Game Techniques
Emerging technology can help instructors better apply gamification and GBL in the information age. Various combinations of gamification and GBL technology in vocational education and training were also identified, including the use of technological advancements in education 4.0 such as big data analytics, augmented reality (AR) /virtual reality (VR), cloud computing and simulation (Halili, 2019 ). Although the majority of the literature review (N = 5, 29%) made use of gamified LMS/MOOC technology, three studies (Jayalath et al., 2022 ; Kladchuen & Srisomphan, 2021 ; Kummanee et al., 2020 ) consist of theoretical papers with no empirical data supporting the proposed design of educational learning environment system. More studies on how LMS/MOOCs will evolve in the VET sector seem to be an interesting subject of research.
4.5.1 Learning Management System (LMS)/Massive Open Online Course (MOOC)
Recent years have seen a rise in the popularity of online learning management systems based on MOOCs, particularly during the COVID-19 pandemic period. However, there are also well-known downsides of MOOCs, such as a lack of student motivation, student involvement, and high dropout rates that call the effectiveness of MOOCs into question (Rohan et al., 2020 ; Seaborn & Fels, 2015 ). Some LMS/MOOCs just imitate traditional teaching methods by including multimedia features like video lectures in their courses. Others go a step further, seeking to maintain the interest of the exceptionally high number of course participants by combining modern technology with educational paradigms. Gamified LMS/MOOCs can raise the motivation of participants to handle the challenge tasks with game mechanics, game dynamics, and components and impact higher involvement, improve user experience, and ensure the loyalty of students, which may lead to a bigger number of proactive participants. A systematic review conducted by Muangsrinoon & Boonbrahm ( 2019 ) proves the wide variety of game elements, retrieving a total of fifteen terms of game elements from twenty-two selected papers that were screened from a total of eighty-two documents. However, only a few terms are commonly used: points, feedback, levels, leader boards, challenges, badges, avatars, competition, and cooperation. From the author’s search of the literature, four studies (Garcia-Iruela et al., 2021 ; Jayalath et al., 2022 ; Kladchuen & Srisomphan, 2021 ; Kummanee et al., 2020 ) included several game elements such as points, badges, levels, leader boards, blocked content, time limit, trophies, virtual goods and spaces feedback and progress bar in the LMS/MOOC to motivate students, increase participation, embark high interest in learning and improve satisfaction.
LMS/MOOC systems often come equipped with a selection of built-in social tools for gamification purposes. For example, Jusas et al. ( 2022 ) implemented an Object-Oriented Programming (OOP) course in a Moodle platform, where two additional plugins (H5P and Level up) for experience points and interactive content were employed. Four gamification elements (XP points, interactive content, local team, and global team), which motivate the different students’ needs, were introduced into the OOP course. Similar work was also carried out by Garcia-Iruela et al. ( 2021 ), which utilised the GameMo plug-in that expands Moodle's capabilities. She investigated three different research methodologies (teacher-centred, student-centred, and mixed approach) to address the issue of low student motivation over three different time periods and discovered that only the teacher-centred and mixed approach produced a positive result. This finding demonstrates that a problem-based learning approach in gamification design increase student engagement and the influence of gamification is context-dependent indicating that future research should focus on LMS/MOOC. Evidence from another reviewed study (Tongpaeng et al., 2019 ) suggested that implementation of shorter learning videos (below six minutes) and interactive media increased not only engagement, but also the learner's achievement in MOOCs. It should also be noted here that to increase students’ satisfaction and solve the high dropout problem in the online learning management system, or MOOCs, educational institutions, educational system designers, and instructors should design learner-centred gamification mechanisms that take students' learning requirements into consideration (Cheng, 2021 ).
4.5.2 Immersive Reality
The broad and rapid adoption of wireless networks and mobile devices has lowered the barrier to entry for new technologies like Augmented Reality (AR) and Virtual Reality (VR) and brought major benefits to technologically supported education. Two studies (Wang & Khambari, 2020 ; Wang et al., 2021 ) using AR mobile application technology for English learning aimed at vocational college students from China. This is not surprising given that China has entered the big data, network, and intelligent age known as China Education 2025, which focuses on the Industry 4.0 model (WU & SUN, 2017 ). Both studies indicate an increase in interest, motivation, and engagement because of the immersive setting and meaningful experience gained during the learning process. Additionally, students describe positive relationships and high interactions that occur throughout the educational process.
Another two studies (Bernal et al., 2022 ; Lerner et al., 2020 ) used VR and Immersive VR technology in a serious game design for professional training and are from Germany and Columbia. Both studies revealed a favourable effect with a strong sense of immersion and game flow during the session, as well as a positive influence on training effectiveness and training execution quality. Our most intriguing finding is that both VR studies used different strategies to achieve the total immersion experience and collaborative learning in the virtual world. A study by Lerner et al., ( 2020 ) used a set of head-mounted displays (HMD) while Bernal et al. ( 2022 ) used an immersive virtualisation system called the cave automatic virtual environment, a virtual reality room-sized environment where a user would be experiencing VR by the use of LCD shutter glasses. It was found that using IVR can reduce the limitations of virtual reality headsets for collaborative learning and produce higher learning gains compared to textbook study (de Back et al., 2020 ).
4.5.3 Serious Game Simulations
A serious game is a computer-based program that is designed for both entertainment and learning purposes by simulating real-world scenarios (Kapp, 2012 ). It has been experimentally demonstrated that games are indeed a learning method to enhance competency and generate innovative, collaborative learning that emerges from interactive, constructivist, cooperative, and improvisational educational techniques (Hämäläinen et al., 2018 ; D. Ma et al., 2021 ). However, a study on the influence of learning style (Wouters & Van Der Meulen, 2020 ) on games produced a null effect on motivation. Despite this, most of the current evidence supports the use of serious games in educational learning (Barbieri et al., 2021 ; Glover & Bodzin, 2021 ), and this includes the development of social and professional skills for people with intellectual disabilities (von Barnekow et al., 2017 ).
4.5.4 Interactive Media and Browser Games
Daily technology advances and inventions are rapidly being ingrained in our culture. The word "interactive media" refers to media that enables active engagement on the part of the recipient, thus the term "interactivity"(Shahzad & Khan, 2009 ). According to Habes et al. ( 2020 ), adopting interactive media technology boosted knowledge acquisition, meaningful experience, and learning impact for nursing students and home care nurses. In support of this, it has been shown (Nacional et al., 2021 ) that the usage of Digital Storytelling in conjunction with stop-motion animation techniques has been a beneficial educational and innovative experience. Alternatively, two studies (Azizan et al., 2021 ; Balakrishnan Nair, 2021 ) found that the use of online quizzes in GBL increased students' achievement, engagement and motivation while also stimulating their cognitive abilities and adding value to the learning experience. Additionally, outcomes promote diversity in education and the development of employable skills. Finally, the use of a browser game in the reviewed study increased both motivation and academic accomplishment.
A substantial amount of research has proved the benefits of using gamification in education at the university, high school, and even secondary school levels, but there has been relatively little focus in the literature on vocational education and training (VET). Based on available data, these results have led to high levels of agreement that gamification and GBL enhance good quality and effectiveness of vocational education and training. However, the beneficial effects of gamification and GBL in education also highlight the necessity of instructors’ professional development competence building. Instructors must possess knowledge as well as gaming experience, creativity, and resilience, in order to use gamification and game-based learning applications (Araújo & Carvalho, 2022 ) in an educational environment. It is important for VET instructors to be ready and digitally competent to meet the needs and challenges of today’s society by taking into consideration developing trends that are presently present in professional settings and will be present in the future. To address this issue, future research in this area should concentrate on the creation of further empirical studies on the digital pedagogy training model for TVET instructors utilising a range of emerging technologies to evaluate the efficacy of gamification and GBL in vocational education. It is possible that future studies may compare the data analysis from pre- and post-tests with the integration of various game elements and developed technology for experiments into instruction to determine the most appropriate technological pedagogy and game techniques that can be applied in the teaching and learning environment to increase students' active engagement and motivation. It is important for educational institutions, system designers, and instructors to design learner-centred gamification mechanisms that consider student learning requirements and learn from developed countries that have implemented GBL and GBL in their vocational education sector, especially in the Industrial 4.0 revolution.
5 Limitations
This study examined only conceptual and experimental academic studies conducted in selected databases (Web of Science, Scopus, PubMed and ScienceDirect) and a few from Google Scholar, excluding grey literature and book chapters. The language restriction is included, as the papers analysed were in English only, necessitating the exclusion of other publications that may have been included in the study. As a result, there was a possibility of publication bias impairing the systematic review's validity. However, the number of publications in languages other than English is extremely low (< 5), and it was not analysed to determine if it might be included in the database. The number of articles reviewed is limited, which raises concerns about the review results' generalisability. However, this evinces the need for developing more gamified experiences in this scope of training. On the other hand, research also indicates that an individual's experience with gamification may vary on the specific characteristics of users, situational relevance and possible contextual factors (Finckenhagen, 2015 ; Smiderle et al., 2020 ). There is no one-size-fits-all approach to gamifying learning. However, this study determines some implications of gamification and GBL as an active methodology, bringing together several experiences in a variety of fields.
6 Conclusion
The primary goal of this study was to obtain a better understanding of the phenomena of gamification and game-based learning in vocational education. While this new field of study is still in its infancy, it offers an exciting future. Gamification and game-based learning may be utilised as effective teaching and learning strategies to meet the requirements of 21st-century education. After the systematic literature review, it has been established that the use of gamification and game-based learning in the VET sector has been beneficial at different educational levels, from school or college to professional training. We discovered that, from 2020 forward, vocational institutions in Asia, particularly Thailand, have published the greatest number of studies on the topic. We also found that the engineering field produces the most articles on gamification and GBL in vocational education and training, followed by healthcare disciplines. From the discussion above, it is apparent that gamification and GBL technology include a wide variety of up-and-coming technologies, such as learning management systems (LMS) and massive open online courses (MOOC), immersive reality, serious game simulations, interactive media, and browser games. This means that the direction and pedagogy of the curricula, courses, and programmes must continue to adapt to meet the expanding needs of the digital economy. However, no precise objective has been set for how many vocational instructors have completely embraced the use of digital tools and technology in teaching, learning, and administrative work, as well as the related consequences. There is still a significant digital skills gap among vocational instructors, which impedes the pace of digital transformation in Malaysia, suggesting that all VET instructors must complete the technological pedagogy training programme to be competent in equipping students with the digital skills required by growth industries, well before the Covid-19 era.
The systematic study revealed various advantages of using gamified learning in vocational learners, including increased motivation, engagement, and academic accomplishment. Gamification and GBL might have a significant impact on the future of vocational education and training since they minimise the possibility of making mistakes. They may serve as a safe introduction to various dangerous occupational fields where failure is not an issue, in fact, it is anticipated while learning in a game. Although peer-reviewed research on gamification and game-based learning for vocational education found good benefits, journals in this field are scarce, and insights into the link between learners' educational game strategies and the outcomes of these learning strategies are still insufficient. However, this study examined only conceptual and experimental academic studies conducted in selected databases (Web of Science, Scopus, PubMed, ScienceDirect, and a few from Google Scholar), excluding grey literature and book chapters. Future studies should take into consideration data sources from other databases or grey literature, such as conference papers and other publications.
Data availability
The datasets supporting the conclusions of this article are included within the article.
Abdul-aziz, S. N., Zulkifli, N., Nashir, I. M., & Karim, N. A. A. (2020). Pull and Push Factors of Students’ Enrolment in the TVET Programme at Community Colleges in Malaysia . April . https://doi.org/10.30880/jtet.2020.12.01.007
Alessandro Brolpito. (2018). Digital skills and competence, and digital and online learning. In European Training Foundation . https://www.etf.europa.eu/sites/default/files/2018-10/DSC and DOL_0.pdf
Alghamdi, A., Karpinski, A. C., Lepp, A., & Barkley, J. (2020). Online and face-to-face classroom multitasking and academic performance: Moderated mediation with self-efficacy for self-regulated learning and gender. Computers in Human Behavior, 102 , 214–222. https://doi.org/10.1016/j.chb.2019.08.018
Article Google Scholar
Alonso-García, S., Aznar-Díaz, I., Cáceres-Reche, M. P., Trujillo-Torres, J. M., & Romero-Rodríguez, J. M. (2019). Systematic Review of Good Teaching Practices with ICT in Spanish Higher Education Trends and Challenges for Sustainability. Sustainability (Switzerland) , 11 (24). https://doi.org/10.3390/su11247150
Anastasiadis, T., Lampropoulos, G., & Siakas, K. (2018). Digital Game-based Learning and Serious Games in Education. International Journal of Advances in Scientific Research and Engineering , 4 (12), 139–144. https://doi.org/10.31695/ijasre.2018.33016
Anil Yasin, A., & Abbas, A. (2021). Role of gamification in engineering education: A systematic literature review. IEEE Global Engineering Education Conference, EDUCON , 2021 - April (April), 210–213. https://doi.org/10.1109/EDUCON46332.2021.9454038
Araújo, I., & Carvalho, A. A. (2022). education sciences Enablers and Difficulties in the Implementation of Gamification: A Case Study with Teachers. Education Sciences, 12 (3), 191.
Arnold, M., North, B., Fischer, H., Mueller, J., & Diab, M. (2021). Game-Based Learning in Vet Schools: A Learning Architecture for Educators in Vocational Education. INTED2021 Proceedings , 1 (April), 3297–3303. https://doi.org/10.21125/inted.2021.0692
Azizan, I. D., Alias, M., & Mustafa, M. Z. (2021). Effect of game-based learning in vehicle air-conditioning course on cognitive and affective skills of vocational students. Journal of Technical Education and Training , 13 (3), 146–154. https://doi.org/10.30880/jtet.2021.13.03.014
Balakrishnan Nair, B. (2021). Endorsing gamification pedagogy as a helpful strategy to offset the COVID-19 induced disruptions in tourism education. Journal of Hospitality, Leisure, Sport and Tourism Education , September , 100362. https://doi.org/10.1016/j.jhlste.2021.100362
Barbieri, G. G., Barbieri, R., & Capone, R. (2021). Serious Games in High School Mathematics Lessons: An Embedded Case Study in Europe. Eurasia Journal of Mathematics, Science and Technology Education , 17 (5), 1–17. https://doi.org/10.29333/ejmste/10857
Baser, D., Akkus, R., Akayoglu, S., Top, E., & Gurer, M. D. (2021). Training in-service teachers through individualized technology-related mentorship. Educational Technology Research and Development, 69 (6), 3131–3151. https://doi.org/10.1007/s11423-021-10065-w
Behl, A., Jayawardena, N., Pereira, V., Islam, N., Giudice, M. Del, & Choudrie, J. (2022). Gamification and e-learning for young learners: A systematic literature review, bibliometric analysis, and future research agenda. Technological Forecasting and Social Change , 176 (November 2021), 121445. https://doi.org/10.1016/j.techfore.2021.121445
Bernal, I. F. M., Lozano-Ramírez, N. E., Cortés, J. M. P., Valdivia, S., Muñoz, R., Aragón, J., García, R., & Hernández, G. (2022). An Immersive Virtual Reality Training Game for Power Substations Evaluated in Terms of Usability and Engagement. Applied Sciences (Switzerland) , 12 (2). https://doi.org/10.3390/app12020711
Blueweave, C. (2021). Global Education Gamification Market is Projected to Reach USD 4144.97 Million in 2027 . BlueWeave Consulting. https://www.blueweaveconsulting.com/press-release/global-education-gamification-market-is-projected-to-reach-usd-4144-97-million-in-2027 . Accessed 11 April 2022
Chan, S., & Lo, N. (2022). Teachers’ and Students’ Perception of Gamification in Online Tertiary Education Classrooms During the Pandemic. SN Computer Science, 3 (3), 1–16. https://doi.org/10.1007/s42979-022-01117-w
Charlo, J. C. P., Belova, N., Gutiérrez, E. Q., Llinares, A. Z., Arboleya-García, E., Swacha, J., López-Serentill, P., & Carmona-Medeiro, E. (2022). Preface for the Special Issue “Trends in Educational Gamification: Challenges and Learning Opportunities.” In Education Sciences (Vol. 12, Issue 3). MDPI. https://doi.org/10.3390/educsci12030179
Cheng, Y. M. (2021). Can gamification and interface design aesthetics lead to MOOCs’ success? Education and Training, 63 (9), 1346–1375. https://doi.org/10.1108/ET-09-2020-0278
Chiu, T. K. F., & Lim, C. P. (2020). Strategic Use of Technology for Inclusive Education in Hong Kong: A Content-Level Perspective. ECNU Review of Education, 3 (4), 715–734. https://doi.org/10.1177/2096531120930861
de Back, T. T., Tinga, A. M., Nguyen, P., & Louwerse, M. M. (2020). Benefits of immersive collaborative learning in CAVE-based virtual reality. International Journal of Educational Technology in Higher Education , 17 (1). https://doi.org/10.1186/s41239-020-00228-9
DEL4ALL, C. (2020). Covid-19 Effects on Higher Education Institutions: Challenges and Opportunities .
Department Skills Development . (2022). https://www.dsd.gov.my/index.php/perkhidmatan/sijil-kemahiran-malaysia-skm
Dhirendra Kumar. (2015). Pros and cons of online education. NC State Industry Expansion Splutions , 2 (1), 1–7. https://www.ies.ncsu.edu/resources/white-papers/pros-and-cons-of-online-education/
Dichev, C., & Dicheva, D. (2017). Gamifying education: what is known, what is believed and what remains uncertain: a critical review. In International Journal of Educational Technology in Higher Education (Vol. 14, Issue 1, p. 9). https://doi.org/10.1186/s41239-017-0042-5
Dimitra, K., Konstantinos, K., Christina, Z., & Katerina, T. (2020). Types of Game-Based Learning in Education: A brief state of the art and the implementation in Greece. The European Educational Researcher , 3 (2), 87–100. https://doi.org/10.31757/euer.324
Dubé, A. K., & Wen, R. (2022). Identification and evaluation of technology trends in K-12 education from 2011 to 2021. In Education and Information Technologies (Vol. 27, Issue 2). Springer US. https://doi.org/10.1007/s10639-021-10689-8
Economic Planning Unit, P. (2014). Strengthening economic growth. In Mid-Term Review of the Eleventh Malaysia Plan .
EDUCAUSE. (2017). EDUCAUSE Review: The Next Generation of Digital Learning Environments. July/August , 52 (4). http://er.educause.edu/toc/educause-review-print-edition-volume-52-number-4-july-august-2017
Engzell, P., Frey, A., & Verhagen, M. D. (2021). Learning loss due to school closures during the COVID-19 pandemic. Proceedings of the National Academy of Sciences of the United States of America , 118 (17). https://doi.org/10.1073/PNAS.2022376118
European Commission. (2022). DigComp Framework, EU Science Hub . https://joint-research-centre.ec.europa.eu/digcomp/digcompframework_en
Finckenhagen KR (2015) Context in Gamification. 2014, 1–10
Gaffoor, A., & Van der Bijl, A. (2019). Factors influencing the intention of students at a selected TVET college in the Western Cape to complete their National Certificate (Vocational) Business Studies programme. Journal of Vocational, Adult and Continuing Education and Training , 2 (2), 23–42. https://doi.org/10.14426/jovacet.v2i2.70
Games, S., & De Carvalho, C. V. (2022). Game-Based Learning, Gamification in Education and Serious Games. Computers, 11 (36), 1–4.
Google Scholar
Garcia-Iruela, M., Hijón-Neira, R., & Connolly, C. (2021). Analysis of three methodological approaches in the use of gamification in vocational training. Information (switzerland), 12 (8), 1–12. https://doi.org/10.3390/info12080300
Garzón-Artacho, E., Sola-Martínez, T., Romero-Rodríguez, J. M., & Gómez-García, G. (2021). Teachers’ perceptions of digital competence at the lifelong learning stage. Heliyon, 7 (7), e07513. https://doi.org/10.1016/j.heliyon.2021.e07513
Article PubMed PubMed Central Google Scholar
Glover, K. R., & Bodzin, A. (2021). Learner-centric Design of a Hand Hygiene Serious Simulation Game for Grade 12 Emerging Health Professional Students. TechTrends, 65 (3), 379–393. https://doi.org/10.1007/s11528-020-00577-2
Gómez-Carrasco, C. J., Monteagudo-Fernández, J., Moreno-Vera, J. R., & Sainz-Gómez, M. (2020). Evaluation of a gamification and flipped-classroom program used in teacher training: Perception of learning and outcome. PLoS ONE , 15 (7 July). https://doi.org/10.1371/journal.pone.0236083
Gupta, P., & Goyal, P. (2022). Is game-based pedagogy just a fad? A self-determination theory approach to gamification in higher education. International Journal of Educational Management, 36 (3), 341–356. https://doi.org/10.1108/IJEM-04-2021-0126
Habes, E. V., Jepma, P., Parlevliet, J. L., Bakker, A., & Buurman, B. M. (2020). Video-based tools to enhance nurses’ geriatric knowledge: A development and pilot study. Nurse Education Today, 90 (March), 104425. https://doi.org/10.1016/j.nedt.2020.104425
Article CAS PubMed Google Scholar
Halili, S. H. (2019). Technological Advancements in Education 4.0. The Online Journal of Distance Education and E-Learning , 7 (1), 63–69. http://tojdel.net/journals/tojdel/articles/v07i01/v07i01-08.pdf
Hämäläinen, R. H., Niilo-Rämä, M., Lainema, T., & Oksanen, K. (2018). How to Raise Different Game Collaboration Activities: The Association Between Game Mechanics, Players’ Roles and Collaboration Processes. Simulation and Gaming, 49 (1), 50–71. https://doi.org/10.1177/1046878117752470
International Labour Organization. (2020). The Digitization of TVET and Skills Systems .
Jayalath, J., & Esichaikul, V. (2020). Gamification to Enhance Motivation and Engagement in Blended eLearning for Technical and Vocational Education and Training. Technology, Knowledge and Learning . https://doi.org/10.1007/s10758-020-09466-2
Jayalath, J., Esichaikul, V., & ·, Esichaikul, V., Jayalath, J., & Esichaikul, V. (2022). Gamification to Enhance Motivation and Engagement in Blended eLearning for Technical and Vocational Education and Training. Technology, Knowledge and Learning, 27 (1), 91–118. https://doi.org/10.1007/s10758-020-09466-2
Jayasinghe, U., & Dharmaratne, A. (2013). Game-based learning vs. gamification from the higher education students’ perspective. Proceedings of 2013 IEEE International Conference on Teaching, Assessment and Learning for Engineering, TALE 2013 , January 2016 , 683–688. https://doi.org/10.1109/TALE.2013.6654524
Johnson, E., Morwane, R., Dada, S., Pretorius, G., & Lotriet, M. (2018). Adult Learners’ Perspectives on Their Engagement in a Hybrid Learning Postgraduate Programme. Journal of Continuing Higher Education, 66 (2), 88–105. https://doi.org/10.1080/07377363.2018.1469071
Jusas, V., Barisas, D., & Jančiukas, M. (2022). Game elements towards more sustainable learning in object-oriented programming course. Sustainability (Switzerland) , 14 (4), 2325. https://doi.org/10.3390/su14042325
Kapp, K. M. (2012). The Gamification of Learning and Instruction: Game Based Methods and Strategies for Training and Education (R. Taff (Ed.)). Pfeiffer.
Khatiwada, S., & Veloso, M. K. (2019). New Technology and Emerging Occupations: Evidence from Asia. In ADB Economics Working Paper (No. 576). https://doi.org/10.2139/ssrn.3590128
Kladchuen, R., & Srisomphan, J. (2021). The Synthesis of a Model of Problem-Based Learning with the Gamification Concept to Enhance the ProblemSolving Skills for High Vocational Certificate. International Journal of Emerging Technologies in Learning, 16 (4), 4–21. https://doi.org/10.3991/ijet.v16i14.20439
Krath, J., Schürmann, L., & von Korflesch, H. F. O. (2021). Revealing the theoretical basis of gamification: A systematic review and analysis of theory in research on gamification, serious games and game-based learning. Computers in Human Behavior, 125 (July), 106963. https://doi.org/10.1016/j.chb.2021.106963
Kulyk, Y., Kravchenko, L., Blyzniuk, M., Chystiakova, L., Orlova, N., & Bukhun, A. (2022). Pedagogical Technologies for Competent Training of Teachers in Ukrainian Professional Education. International Journal of Education and Information Technologies , 16 , 29–38. https://doi.org/10.46300/9109.2022.16.3
Kummanee, J., Nilsook, P., & Wannapiroon, P. (2020). Digital learning ecosystem involving steam gamification for a vocational innovator. International Journal of Information and Education Technology , 10 (7), 533–539. https://doi.org/10.18178/ijiet.2020.10.7.1420
Lerner, D., Mohr, S., Schild, J., Göring, M., & Luiz, T. (2020). An immersive multi-user virtual reality for emergency simulation training: Usability study. JMIR Serious Games, 8 (3), 1–10. https://doi.org/10.2196/18822
Li, S., Yamaguchi, S., Sukhbaatar, J., & Takada, J. I. (2019). The influence of teachers’ professional development activities on the factors promoting ICT integration in Primary Schools in Mongolia. Education Sciences , 9 (2). https://doi.org/10.3390/educsci9020078
Ma, D., Shi, Y., Zhang, G., & Zhang, J. (2021). Does theme game-based teaching promote better learning about disaster nursing than scenario simulation: A randomized controlled trial. Nurse Education Today, 103 (March), 104923. https://doi.org/10.1016/j.nedt.2021.104923
Article PubMed Google Scholar
Ma, M., & Oikonomou, A. (2017). Serious games and edutainment applications: Volume II. Serious Games and Edutainment Applications: Volume II , March , 1–702. https://doi.org/10.1007/978-3-319-51645-5
Madimabe, M. P., & Omodan, B. I. (2021). Investigating the Effects of E-Learning as a Method of Curriculum Dissemination for Rural TVET College Students. Research in Social Sciences and Technology , 6 (3), 82–92. https://doi.org/10.46303/ressat.2021.27
Mahat, J., Alias, N., & Yusop, F. D. (2022). Systematic literature review on gamified professional training among employees. Interactive Learning Environments , 1–21. https://doi.org/10.1080/10494820.2022.2043910
Man, C. K. (2021). Game Elements, Components, Mechanics and Dynamics: What are they? Creative Culture (MY). https://medium.com/creative-culture-my/game-elements-components-mechanics-and-dynamics-what-are-they-80c0e64d6164
Manzano-León, A., Camacho-Lazarraga, P., Guerrero, M. A., Guerrero-Puerta, L., Aguilar-Parra, J. M., Trigueros, R., & Alias, A. (2021). Between level up and game over: A systematic literature review of gamification in education. Sustainability (switzerland), 13 (4), 1–14. https://doi.org/10.3390/su13042247
Market Data, F. (2022). Gamification Education Market Size, Growth, Trends | 2022 to 2027 . Market Data Forecast. https://www.marketdataforecast.com/market-reports/gamification-education-market . Accessed 11 April 2022
Martin, F., & Bolliger, D. U. (2018). Engagement Matters: Student Perceptions on the Importance of Engagement Strategies in the Online Learning Environment. Online Learning , 22 (1), 205–222. https://doi.org/10.24059/olj.v22i1.1092
Mengist, W., Soromessa, T., & Legese, G. (2020). Method for conducting systematic literature review and meta-analysis for environmental science research. MethodsX, 7 , 100777. https://doi.org/10.1016/j.mex.2019.100777
Meticulous Market Research. (2022). Vocational Education and Training Market Worth $896.01 Billion by 2029 . Meticulous Market Research Inc. https://www.globenewswire.com/en/news-release/2022/06/06/2456915/0/en/Vocational-Education-and-Training-Market-Worth-896-01-Billion-by-2029-Exclusive-Report-by-Meticulous-Research.html . Accessed 13 Sept 2022
Muangsrinoon, S., & Boonbrahm, P. (2019). Game elements from literature review of gamification in healthcare context. Journal of Technology and Science Education, 9 (1), 20–31. https://doi.org/10.3926/jotse.556
Nacional, U., Agustin, D. S., Hurtado-mazeyra, A., Alejandro-oviedo, O. M., Núñez-pacheco, R., & Eudis, K. (2021). Digital Storytelling with Stop Motion for the Development of Competencies in University Students. 4th International Conference on Education Technology Management, ICETM 2021 , 148–154.
National Centre for Vocational Education Research. (2020). GLOSSARY of VET. In National Centre for Vocational Education Research .
O’Neill, S. (2022). Gamification in Marketing: Stats and Trends for 2022 . LXA, Learning Experience Alliance. https://www.martechalliance.com/stories/gamification-in-marketing-stats-and-trends-for-2022
Oliveira, R. P., de Souza, C. G., Reis, A. da C., & de Souza, W. M. (2021). Gamification in e-learning and sustainability: A theoretical framework. In Sustainability (Switzerland) (Vol. 13, Issue 21). https://doi.org/10.3390/su132111945
Omar, M., Ali, D. F., Md Adam @ Mohd Adnan, N. A. I., & Saari, M. A. (2022). Gamification in Vocational Teaching and Learning: Perception and Readiness among Lecturers. International Journal of Education , 14 (1), 140. https://doi.org/10.5296/ije.v14i1.19507
Organisation for Economic Co-operation and Development (OECD). (2019). What characterises upper secondary vocational education and training? OECD , 68 . https://doi.org/10.1787/a1a7e2f1-en
Organisation for Economic Co-operation and Development (OECD). (2021). Economic Survey of Malaysia (Issue August).
Page, M. J., McKenzie, J. E., Bossuyt, P. M., Boutron, I., Hoffmann, T. C., Mulrow, C. D., Shamseer, L., Tetzlaff, J. M., Akl, E. A., Brennan, S. E., Chou, R., Glanville, J., Grimshaw, J. M., Hróbjartsson, A., Lalu, M. M., Li, T., Loder, E. W., Mayo-Wilson, E., McDonald, S., … Moher, D. (2021). The PRISMA 2020 statement: An updated guideline for reporting systematic reviews. In The BMJ (Vol. 372). https://doi.org/10.1136/bmj.n71
Pan, L., Tlili, A., Li, J., Jiang, F., Shi, G., Yu, H., & Yang, J. (2021). How to Implement Game-Based Learning in a Smart Classroom? A Model Based on a Systematic Literature Review and Delphi Method. Frontiers in Psychology, 12 (December), 1–13. https://doi.org/10.3389/fpsyg.2021.749837
Pan, Y., Ke, F., & Xu, X. (2022). A systematic review of the role of learning games in fostering mathematics education in K-12 settings. Educational Research Review , 36 (April 2021), 100448. https://doi.org/10.1016/j.edurev.2022.100448
Pasca, M. G., Renzi, M. F., Di Pietro, L.. & Guglielmetti Mugion, R. (2021). Gamification in tourism and hospitality research in the era of digital platforms: a systematic literature review. Journal of Service Theory and Practice, 31 (5), 691–737. https://doi.org/10.1108/JSTP-05-2020-0094
Pittaway, S. M., & Moss, T. (2014). “Initially, we were just names on a computer screen”: Designing engagement in online teacher education. Australian Journal of Teacher Education , 39 (7), 140–156. https://doi.org/10.14221/ajte.2014v39n7.10
Platz, L. (2022). Learning with serious games in economics education a systematic review of the effectiveness of game-based learning in upper secondary and higher education. International Journal of Educational Research , 115 (October 2021), 102031. https://doi.org/10.1016/j.ijer.2022.102031
Ra, S., Shrestha, U., Khatiwada, S., Yoon, S. W., & Kwon, K. (2019). The rise of technology and impact on skills. International Journal of Training Research, 17 (sup1), 26–40. https://doi.org/10.1080/14480220.2019.1629727
Reeve, J. (2018). A Self-determination Theory Perspective on Student Engagement * . https://doi.org/10.1007/978-1-4614-2018-7
Reis, A. C. B., Júnior, E. S., Gewehr, B. B., & Torres, M. H. (2020). Prospects for using gamification in industry 4.0. Production , 30 . https://doi.org/10.1590/0103-6513.20190094
Roberts, M. P. and H. (2006). Systematic Reviews in the Social Sciences: A Practical Guide. In Blackwell Publishing .
Rodrigues, L., Pereira, F. D., Toda, A. M., Palomino, P. T., Pessoa, M., Carvalho, L. S. G., Fernandes, D., Oliveira, E. H. T., Cristea, A. I., & Isotani, S. (2022). Gamification suffers from the novelty effect but benefits from the familiarization effect: Findings from a longitudinal study. International Journal of Educational Technology in Higher Education , 19 (1). https://doi.org/10.1186/s41239-021-00314-6
Rohan, R., Pal, D., & Funilkul, S. (2020). Gamifying MOOC’s a Step in the Right Direction?: A Systematic Literature Review. ACM International Conference Proceeding Series
Roodt, S., & Ryklief, Y. (2019). Using digital game-based learning to improve the academic efficiency of vocational education students. International Journal of Game-Based Learning, 9 (4), 45–69. https://doi.org/10.4018/IJGBL.2019100104
Sage, K., Jackson, S., Fox, E., & Mauer, L. (2021). The virtual COVID-19 classroom: surveying outcomes, individual differences, and technology use in college students. Smart Learning Environments , 8 (1). https://doi.org/10.1186/s40561-021-00174-7
Saleem, A. N., Noori, N. M., & Ozdamli, F. (2022). Gamification Applications in E-learning: A Literature Review. Technology, Knowledge and Learning, 27 (1), 139–159. https://doi.org/10.1007/s10758-020-09487-x
Seaborn, K., & Fels, D. I. (2015). Gamification in theory and action: A survey. International Journal of Human Computer Studies, 74 , 14–31. https://doi.org/10.1016/j.ijhcs.2014.09.006
Shagataeva, Z. E., Sarbassov, Y. K., Seminar, E., Sydykbekova, M. A. & K. A. T. (2021). The general technological competency model for vocational teachers in kazakhstan. World Journal on Educational Technology: Current Issues , 13 (4), 574–588
Shahzad, A. H., & Khan, A. (2009). Role of interactive media in teaching learning process at higher education. Proceedings - 2009 2nd IEEE International Conference on Computer Science and Information Technology, ICCSIT 2009 , 73–78. https://doi.org/10.1109/ICCSIT.2009.5234472
Sia, J. K., & Adamu, A. A. (2021). Facing the unknown: pandemic and higher education in Malaysia . 10 (2), 263–275 https://doi.org/10.1108/AEDS-05-2020-0114
Smiderle, R., Rigo, S. J., Marques, L. B., Peçanha de Miranda Coelho, J. A., & Jaques, P. A. (2020). The impact of gamification on students’ learning, engagement and behavior based on their personality traits. Smart Learning Environments , 7 (1). https://doi.org/10.1186/s40561-019-0098-x
Softek. (2017). The 70% of the companies in the Global 2000 list already use gamification - Softtek . Softek Tech Magazine. https://www.softtek.eu/en/tech-magazine-en/software-trends-en/70-of-companies-in-the-global-2000-list-already-use-gamification/ . Accessed 11 April 2022
Sufian, S. A., Nordin, N. A., Tauji, S. S. N., Nasir, M. K. M., Sufian, S. A., Nordin, N. A., & Tauji, S. S. N. (2020). The Impact of Covid-19 on the Malaysian Education System the Impact of Covid-19 on the Malaysian Education System . 9 (2), 764–774. https://doi.org/10.6007/IJARPED/v9-i2/7659
Tay, J., Goh, Y. M., Safiena, S., & Bound, H. (2022). Designing digital game-based learning for professional upskilling: A systematic literature review. Computers and Education, 184 (April), 104518. https://doi.org/10.1016/j.compedu.2022.104518
Technavio. (2016). Technical and Vocational Education Market in North America 2016–2020 | Market Research Reports - Industry Analysis Size & Trends - Technavio . Technavio. https://www.technavio.com/report/north-america-education-technology-technical-and-vocational-education-market . Accessed 11 April 2022
The World Bank Group. (2021). Unleashing the Power of Educational Technology in TVET Systems . https://thedocs.worldbank.org/en/doc/61714f214ed04bcd6e9623ad0e215897-0400012021/related/EdTech-Report-FIN2-web.pdf
Thomas, N. J., Baral, R., & Crocco, O. S. (2022). Gamification for HRD: Systematic Review and Future Research Directions. Human Resource Development Review . https://doi.org/10.1177/15344843221074859
Tongpaeng, Y., Sureephong, P., Chernbumroong, S., Kamon, M., & Tabai, K. (2019). Vocational knowledge improvement method on massive open online course for the thai tourism worker. ECTI Transactions on Computer and Information Technology , 13 (1), 94–104. https://doi.org/10.37936/ECTI-CIT.2019131.138615
UNESCO-UNEVOC. (2020). Promoting Quality in TVET Using Technology: A practical guide .
UNESCO-UNEVOC. (2021). The Digital TVET Learning Platform Promising Practice 2021 . https://unevoc.unesco.org/home/UNEVOC+Publications/akt=detail/lang=en/qs=6471
UNESCO-UNEVOC International Centre. (2020). Skills for a Resilient Youth: Virtual Conference Report. In UNESCO-UNEVOC TVeT Forum (Issue July).
UNESCO. (2015). PROPOSAL FOR THE REVISION OF THE 2001 REVISED RECOMMENDATION CONCERNING TECHNICAL AND VOCATIONAL EDUCATION (Issue August).
UNESCO. (2021). Sub-Education Policy Review Report: Technical Vocational and Education Training (TVET). In UNESCO . https://en.unesco.org/sites/default/files/tvet_final_-_january_2021.pdf
United, N. (2020). Policy Brief: Education during COVID-19 and beyond. United Nations , 1–26. https://doi.org/10.24215/18509959.26.e12
Vijayan, R. (2021). Teaching and learning during the covid-19 pandemic: A topic modeling study. Education Sciences , 11 (7). https://doi.org/10.3390/educsci11070347
von Barnekow, A., Bonet-Codina, N., & Tost, D. (2017). Can 3D Gamified Simulations Be Valid Vocational Training Tools for Persons with Intellectual Disability? Methods of Information in Medicine, 56 (02), 162–170. https://doi.org/10.3414/me16-02-0014
Wang, D., & Khambari, M. N. M. (2020). An AR-based Gamified English Course in Vocational College through Interest-driven Approach. Universal Journal of Educational Research , 8 (1 A), 132–137. https://doi.org/10.13189/ujer.2020.081317
Wang, D., Khambari, M. N. M., Wong, S. L., & Razali, A. B. (2021). Exploring interest formation in english learning through xplorerafe+: A gamified ar mobile app. Sustainability (Switzerland) , 13 (22). https://doi.org/10.3390/su132212792
Wardoyo, C., Satrio, Y. D., Narmaditya, B. S., & Wibowo, A. (2021). Do technological knowledge and game-based learning promote student’s achievement: Lesson from Indonesia. Heliyon, 7 (11), e08467. https://doi.org/10.1016/j.heliyon.2021.e08467
Werbach, K., & Hunter, D. (2012). For the win: how game thinking can revolutionize your business. In For the win, revised and updated edition . Wharton Digital Press. https://doi.org/10.2307/j.ctv2hdrfsm.5
Willert, N. (2021). A systematic literature review of gameful feedback in computer science education. International Journal of Information and Education Technology , 11 (10), 464–470. https://doi.org/10.18178/ijiet.2021.11.10.1551
Wouters, P., & Van Der Meulen, E. S. (2020). The role of learning styles in game-based learning. International Journal of Game-Based Learning, 10 (1), 54–69. https://doi.org/10.4018/IJGBL.2020010104
WU, G., & SUN, X. (2017). From the Industry 4.0 to Intelligent Personalized Education Model. DEStech Transactions on Social Science, Education and Human Science , meit , 247–250. https://doi.org/10.12783/dtssehs/meit2017/12863
Xu, F. (2013). Information and Communication Technologies in Tourism 2014. Information and Communication Technologies in Tourism 2014 , January . https://doi.org/10.1007/978-3-319-03973-2
Yasak, Z., & Alias, M. (2015). ICT Integrations in TVET: Is it up to Expectations? Procedia - Social and Behavioral Sciences , 204 (November 2014), 88–97. https://doi.org/10.1016/j.sbspro.2015.08.120
Yeap, C. F., Suhaimi, N., & Nasir, M. K. M. (2021). Issues, Challenges, and Suggestions for Empowering Technical Vocational Education and Training Education during the COVID-19 Pandemic in Malaysia. Creative Education, 12 (08), 1818–1839. https://doi.org/10.4236/ce.2021.128138
Yousef, A. M. F., & Sumner, T. (2021). Reflections on the last decade of MOOC research. Computer Applications in Engineering Education, 29 (4), 648–665. https://doi.org/10.1002/cae.22334
Yunos, J. M., Sern, L. C., & Hamdan, N. H. (2017). Sustainability of TVET TE programme: An exploratory sequential mixed method design. Advanced Science Letters, 23 (1), 220–222. https://doi.org/10.1166/asl.2017.7138
Zabolotska, O., Zhyliak, N., Hevchuk, N., Petrenko, N., & Alieko, O. (2021). Digital competencies of teachers in the transformation of the educational environment. Journal of Optimization in Industrial Engineering , 14 (1), 43–50. https://doi.org/10.22094/JOIE.2020.677813
Zhonggen, Y. (2019). A Meta-Analysis of Use of Serious Games in Education over a Decade. International Journal of Computer Games Technology , 2019 (3). https://doi.org/10.1155/2019/4797032
Download references
Author information
Authors and affiliations.
Department of Curriculum and Instructional Technology, Faculty of Education, Jln Profesor Diraja Ungku Aziz, University Malaya, 50603, Kuala Lumpur, Malaysia
Fazlida Dahalan, Norlidah Alias & Mohd Shahril Nizam Shaharom
You can also search for this author in PubMed Google Scholar
Corresponding author
Correspondence to Norlidah Alias .
Ethics declarations
The authors have no relevant financial or non-financial interests to disclose.
Additional information
Publisher's note.
Springer Nature remains neutral with regard to jurisdictional claims in published maps and institutional affiliations.
Rights and permissions
Springer Nature or its licensor (e.g. a society or other partner) holds exclusive rights to this article under a publishing agreement with the author(s) or other rightsholder(s); author self-archiving of the accepted manuscript version of this article is solely governed by the terms of such publishing agreement and applicable law.
Reprints and permissions
About this article
Dahalan, F., Alias, N. & Shaharom, M.S.N. Gamification and Game Based Learning for Vocational Education and Training: A Systematic Literature Review. Educ Inf Technol 29 , 1279–1317 (2024). https://doi.org/10.1007/s10639-022-11548-w
Download citation
Received : 20 April 2022
Accepted : 20 December 2022
Published : 12 January 2023
Issue Date : February 2024
DOI : https://doi.org/10.1007/s10639-022-11548-w
Share this article
Anyone you share the following link with will be able to read this content:
Sorry, a shareable link is not currently available for this article.
Provided by the Springer Nature SharedIt content-sharing initiative
- Game-based learning
- Gamification
- Systematic literature review
- Find a journal
- Publish with us
- Track your research
- Open access
- Published: 19 May 2023
Effectiveness of digital educational game and game design in STEM learning: a meta-analytic review
- Yang Gui 1 , 2 ,
- Zhihui Cai 1 , 2 ,
- Yajiao Yang 1 , 2 ,
- Lingyuan Kong 1 , 2 ,
- Xitao Fan 3 &
- Robert H. Tai 4
International Journal of STEM Education volume 10 , Article number: 36 ( 2023 ) Cite this article
10k Accesses
6 Citations
2 Altmetric
Metrics details
Digital educational games exhibit substantial promise in advancing STEM education. Nevertheless, the empirical evidence on both the efficacy of digital game-based learning and its designs in STEM education is characterized by notable inconsistencies. Therefore, the current study aimed to investigate (1) the general effect of digital game-based STEM learning over STEM learning without digital game, and (2) the enhancement effect of added game-design elements against base game versions in STEM learning. Two meta-analyses were conducted in this study. Based on the 136 effect sizes extracted from 86 studies, the first meta-analysis revealed a medium to large general effect of digital game-based STEM learning over conventional STEM learning ( g = 0.624, 95% CI [0.457, 0.790]). In addition, digital game-based STEM learning appeared to be differentially effective for different learning outcome, different types of game, and different subject. A total of 44 primary studies and 81 effect sizes were identified in the second meta-analysis. The results revealed a small to medium enhancement effect of added game-design elements over base game versions ( g = 0.301, 95% CI [0.163, 0.438]). Furthermore, our results indicated that the game-design elements added for content learning were more effective than those added for gaming experience. Possible explanations for these findings, as well as the limitations and directions for future research were discussed.
Introduction
Science, technology, engineering, and mathematics (STEM) education has become an increasingly important education issue around the world and has received extensive attention from educators and other stakeholders (Kayan-Fadlelmula et al., 2022 ; The White House, 2018 ). STEM education aims to train new talents with twenty-first century skills such as computational, critical, and creative thinking (Li et al., 2016 ; Wahono et al., 2020 ). In addition, STEM education plays a unique role in addressing real-world issues such as energy, the environment and health (Martín-Páez et al., 2019 ; Struyf et al., 2019 ). Therefore, many countries regard STEM education as a national strategy to lead the reform and development of basic education (Dou, 2019 ). However, currently, STEM education faces some issues. On one hand, traditional STEM classroom can hardly attract students’ interest (Gao et al., 2020 ). On the other hand, the traditional classroom environment is difficult to provide effective practical activities to meet the educational needs of cultivating students’ complex problem-solving ability due to limited time and resources (Klopfer & Thompson, 2020 ). Therefore, new instructional methods are urgently needed to improve STEM learning.
Digital game-based learning (DGBL), which was discussed as one of the twenty-first century global pedagogical approaches (Kukulska-Hulme et al., 2021 ), has unique advantages in enhancing STEM education compared with other pedagogical strategies (Ishak et al., 2021 ). Digital educational games provide an engaging learning environment that allows learners to interact with game mechanics in a virtual world, which provides the learners with a meaningful gaming experience and also greatly enhances their learning motivation (Ball et al., 2020 ; Ishak et al., 2021 ). At the same time, digital educational game can serve as an effective learning environment, providing players with ample opportunities for simulation, real-world questions, and rich instructional support. In such an environment, learners can practice problem solving skills, develop critical thinking and foster STEM literacy (Kayan-Fadlelmula et al., 2022 ; Klopfer & Thompson, 2020 ).
Despite the great potential of digital educational games, there is no consensus among researchers on the effectiveness of DGBL in STEM education. On one hand, some researchers showed that, compared to traditional instructions, DGBL could support learners to improve learning motivation, understand STEM concepts and develop practical skills (Halpern et al., 2012 ; Johnson & Mayer, 2010 ; Masek et al., 2017 ; Wu & Anderson, 2015 ). On the other hand, some researchers believed that DGBL may not have significant advantages over traditional methods for STEM learning (Renken & Nunez, 2013 ; Riopel et al., 2019 ). Moreover, poorly designed educational games may produce extra cognitive load, which may make the learning worse compared with traditional instructional approaches (Wang, 2020 ). Therefore, several synthesis studies have pooled the findings of previous studies involving DGBL in STEM disciplines. For example, previous reviews have examined the effects of game-based science learning (Riopel et al., 2019 ; Tsai & Tsai, 2020 ), examined the effectiveness of game-based math learning (Byun & Joung, 2018 ; Tokac et al., 2019 ), or examined the effects of digital game-based STEM education on student knowledge gains (Wang et al., 2022 ). These synthesis studies, however, are limited to a single subject or single learning outcome of STEM fields, without providing an overall understanding about the effects of DGBL in STEM education.
In addition to the general question about the overall effectiveness of game-based STEM learning (e.g., comparison between game-based STEM learning vs. traditional STEM learning) as discussed above, some researchers also called for attention to the question of whether some additional gaming and learning mechanics added in an educational game would enhance the effectiveness of game-based STEM learning (Proulx et al., 2017 ; Tsai & Tsai, 2020 ). To examine this question, a study design would typically involve two conditions: one group using the basic (or base) version of a digital learning game, while the other group using an enhanced version of the same digital learning game with added game-design element(s). Thus, the current study, through systematic reviews and meta-analyses of the relevant empirical studies, would attempt to answer two questions: (1) What is the overall effect of digital game-based STEM learning compared to traditional STEM learning? (2) What is the enhancement effect of added game-design element over a base game version in game-based STEM learning?
Literature review
Effectiveness of digital game-based learning in stem education.
Mayer ( 2014 ) defines educational games as digital games designed to promote students’ academic performance. This kind of digital game contains rich learning and gaming mechanisms, providing learners with an engaging and positive learning environment (Lameras et al., 2017 ; Maheu-Cadotte et al., 2018 ). For STEM education, digital educational games are viewed as providing interactive and interesting learning environments that help to develop students’ knowledge and skills related to STEM. Why can digital educational games enhance STEM learning? The situated learning theory proposed by Lave and Wenger ( 1991 ) provides a possible explanation. From the perspective of situated learning, digital educational games can serve as a learning environment for constructing new knowledge, in which learners can learn and practice skills through interactions within the game and with other players. This game-based STEM learning environment can be viewed as a virtual community of practice that provides learners with learning context, guidelines supporting exploration, and opportunities to collaborate (Klopfer & Thompson, 2020 ). At the same time, digital educational games contain rich learning mechanisms or elements (e.g., pedagogical agents, self-explanation strategies, and adaptation) that act like cognitive apprenticeships to support learners from novice to expert. For example, Grivokostopoulou et. al. ( 2020 ) proposed a learning approach in simulation-based game, using embodied pedagogical agents to guide students to explore in virtual communities and to achieve learning goals.
As the field of DGBL evolves, Mayer ( 2015 ) advocated an evidence-based approach and proposed a new research framework, calling on researchers to conduct research in three areas: (1) value-added research, (2) cognitive consequence research, and (3) media comparison research. The value-added research compares the learning outcomes of using a games with added game-design elements against the use of base game without the added design elements. Cognitive consequences research compares cognitive skill gains between groups who play off-the-shelf games for extended time periods and those who engage in control activities. Media comparison research examines whether DGBL promotes more learning when compared to conventional instructional approach.
Many “media comparison research” studies were conducted to explore the effect of digital educational games in STEM learning relative to some conventional learning approaches. However, there is disagreement about the effectiveness of STEM digital educational games. On one hand, many researchers affirm the potential of digital games for STEM learning. The positive effects of game-based STEM learning were shown in many empirical studies (e.g., Kao et al., 2017 ; Khamparia & Pandey, 2018 ; Soflano et al., 2015 ). For example, Kao et. al. ( 2017 ) showed that game-based learning significantly enhanced scientific problem-solving performance and creativity compared to traditional STEM learning. On the other hand, insignificant or negative effects were also common among empirical studies (e.g., Beserra et al., 2014 ; Freeman & Higgins, 2016 ; Sadler et al., 2015 ) of game-based learning in STEM education. In addition, from the cognitive load perspective, poorly designed games or unnecessary game mechanics may increase extraneous cognitive load (i.e., cognitive load that are not related to learning), resulting in less efficient learning. For example, Schrader and Bastiaens ( 2012 ) compared the learning effect in immersive DGBL vs. low-immersion hypertext environments, and showed that DGBL resulted in a higher cognitive load, which reduced the retention and transfer of physical knowledge. In summary, these conflicting findings suggest that the effectiveness of game-based STEM learning is not yet certain, and there could be underlying factors and variables that could influence the effectiveness of STEM digital educational games.
In addition to “media comparison research”, more researchers are now turning their attention to the effectiveness of game design in game-based STEM education, and this direction is aligned with “value-added research” as described in Mayer ( 2015 ). As discussed in Clark et. al. ( 2016 ), the positive effects of digital educational games on learning could largely depend on game design. Plass et. al. ( 2015 ) described the elements of educational game in an integrated design framework. Game design elements consist of six parts: game mechanics, visual aesthetics, narrative, incentives, musical score, and knowledge/skills, with game mechanics and knowledge/skills being the most crucial. Games were designed and used to facilitate learners’ cognitive processing in the process of learning the content (knowledge/skills) (Mayer, 2014 ). As a result, game designers need to consider how the game content should be presented and how the learning mechanism should be designed to facilitate learners’ cognitive development.
Adams and Clark ( 2014 ) compared the differences between basic games and the games with added self-explanatory mechanisms. It was found that middle school students had higher extraneous cognitive load and worse physical test scores when playing games with added self-explaining mechanics. Based on these, the current study also intended to synthesize “value-added research” studies that compared different game mechanic design conditions.
Previous meta-analysis studies on DGBL in STEM education
Up to now, there have been several meta-analyses that attempted to integrate existing research on DGBL related to STEM disciplines, and the results from these meta-analytic reviews were inconsistent. Byun and Joung ( 2018 ) investigated the effect of digital educational games on K-12 students’ mathematics performance. This meta-analysis including 17 empirical studies found that game-based math learning produced a small to medium effect size ( d = 0.37). In addition, Tokac et. al. ( 2019 ) also examined the effect of math video games on the academic performance of PreK-12 students (pre-kindergarten through 12th grade). The meta-analysis included 24 studies and showed that DGBL had a very small effect size on math learning ( d = 0.13). Riopel et. al. ( 2019 ) focuses on the effect of digital educational games on students’ scientific knowledge compared with traditional instructions. The meta-analysis pooled 79 studies and found that digital educational games produced small to medium effect sizes on science learning ( d = 0.31–0.41). The meta-analysis conducted by Tsai and Tsai ( 2020 ) included 26 empirical studies investigating the effectiveness of gameplay design and game mechanics’ design for enhancing science learning. This meta-analysis compiled the findings from empirical studies that involved the comparison between using base game version and using the same game with added gameplay design feature or added game-mechanic design feature. The results reported a medium effect size for added gameplay design feature ( k = 14, g = 0.646) over the base version of the game, and a small effect size for added game-mechanism design ( k = 12, g = 0.270) over the base version of the game.
The meta-analysis studies described above generally indicated that digital educational games had a small to medium effect on science and math learning. Furthermore, these meta-analyses reviewed the effects of DGBL on learning in science and in mathematics separately. However, as Kelley and Knowles ( 2016 ) discussed, STEM education should be treated as a more integrated education model that uses multidisciplinary (science, technology, engineering and math) thinking and knowledge to solve real-world problems. Therefore, it is necessary to examine STEM education as a whole to develop better understanding about the application of digital educational games in STEM education. Moreover, the generalizability and robustness of the findings is also an issue due to the small numbers of studies included in these meta-analyses.
Very recently at the completion of this manuscript, Yu et. al. ( 2022 ) conducted a systematic review aimed to exploring the application of augmented reality (AR) in STEM education through digital game-based learning (DGBL). The review included a total of 46 articles published between 2010 and 2020, providing valuable insights into the potential use of AR games in STEM education. However, it is important to note that this article only provides a qualitative overview of AR in STEM education and does not address the effectiveness of AR learning games compared to other instructional approaches, nor does it explore other types of games, such as virtual reality and tablet games, in STEM education. Arztmann et. al. ( 2023 ) also conducted a meta-analysis that examined the effectiveness of game-based learning compared to traditional classroom teaching in STEM education. The meta-analysis revealed a moderate positive effect of game-based learning on cognitive ( g = 0.67), motivational ( g = 0.51), and behavioral outcomes ( g = 0.93) relative to conventional teaching methodologies. However, the definition of game-based learning used in this meta-analysis is too broad, including traditional games such as board games and card games played in non-digital environments, thereby precluding specific inquiry into the effectiveness of DGBL. In addition, the meta-analysis exclusively considered the impact of student characteristics on the effectiveness of game-based learning without due consideration of other pertinent factors, including game features. Therefore, it is imperative to undertake more comprehensive meta-analysis to ascertain the comparative effectiveness of DGBL vs. traditional teaching in STEM education, encompassing all relevant influencing factors. Such investigations will facilitate evidence-based practices and promote optimal educational outcomes for students.
In addition, Wang et. al. ( 2022 ) also published a new similar meta-analysis study about the effects of digital game-based STEM education on student learning gains. This meta-analysis, which included 33 empirical studies involving STEM subjects from 2010 to 2020, found that digital educational games showed a moderate overall effect size ( d = 0.677) compared to traditional instructing approaches. This new review study provided very useful information as a summary of empirical studies on digital game-based STEM education, but this study also left a couple of important questions unanswered, as discussed below.
First, this meta-analysis only examined the effectiveness of game-based STEM education relative to other educational approaches; in other words, this meta-analysis only synthesized the primary studies of “media comparison research” (Mayer, 2015 ). However, as the research framework by Mayer ( 2015 ) indicated, research on DGBL should not only focus on the general effect of DGBL over other STEM education approaches (i.e., media comparison research studies), but should also examine the effects of different game-design elements in enhancing STEM learning (i.e., value-added research). The large number of empirical studies for value-added research were absent in this new meta-analysis, thus leaving an obvious vacuum in our understanding about the current situation of DGBL.
Second, compared to previous similar review studies, this new meta-analysis focusing on STEM disciplines included somewhat limited number of empirical studies in STEM sub-areas, thus may not fully reflect the current status of research on game-based STEM education. For example, in terms of mathematics learning, the number ( k = 24) of empirical studies included in a previous meta-analysis (Tokac et al., 2019 ) is twice as much as that in this new meta-analysis ( k = 12). In terms of science learning, the number ( k = 79) of empirical studies included in a previous meta-analysis (Riopel et al., 2019 ) is much larger than that included in this new meta-analysis ( k = 18).
Third, methodologically, most of the included studies reported more than one effect size in Wang et. al. ( 2022 )’s meta-analysis, and the multiple effect sizes were statistically non-independent. However, the traditional meta-analytic methods used in this new meta-analysis ignored the dependence of effect sizes within a study, which could have led to potentially biased estimates (i.e., inflated Type I errors and smaller confidence intervals of estimates) (Becker, 2000 ).
In summary, previous research, including several meta-analysis studies, has provided good evidence that DGBL could promote STEM learning when compared against conventional STEM education approaches. However, our understanding about the effect of DGBL in STEM education is still limited. First, previous reviews focused on “media comparison research” studies, but the “value-added research” studies on the role of game design in DGBL in STEM education were not adequately covered or addressed. As indicated by the research framework proposed by Mayer ( 2015 ), and as discussed in Clark et. al. ( 2016 ), the positive effects of digital educational games on learning could depend on game design. With the recent focus on the role of game design in game-based STEM learning, Klopfer and Thompson ( 2020 ) called for linking game design with learning outcomes and for using learning theory as a guide to examine the effects of game design elements. Some researchers went beyond the “media comparison research”, and paid attention to the role of game design in game-based STEM learning (Clark et al., 2016 ; Tsai & Tsai, 2018 , 2020 ). However, at this time, we know relatively little about the role of game design in game-based STEM learning, and about what game design factors may affect the effectiveness of game-based STEM learning.
Second, previous reviews focused on knowledge gains as outcomes in game-based STEM education, but the question about the effect of game-based approach on promoting cognitive skills was not addressed, although there was the general belief that STEM games have good potential in promoting twenty-first century skills (Kayan-Fadlelmula et al., 2022 ; Klopfer & Thompson, 2020 ). With these important issues relevant to game-based STEM education, there is the great need to expand and deepen our understanding of the impact of digital educational games and their design elements on STEM learning.
Potential moderators
Through the review of previous empirical studies and relevant meta-analyses, we identified some game characteristics and study features that may have contributed to the inconsistent findings across the studies investigating the overall effect and also enhancement effect of game-based STEM learning. These were described below.
Game-related characteristics
Game type: Researchers examined the effect of game type on the effectiveness of game-based learning (Mao et al., 2022 ; Tsai & Tsai, 2018 ). Ke ( 2015 ) divided games into seven categories: Role-playing, Strategy, Simulation, Construction, Adventure, Action, and Puzzle game (see Table 1 ). Previous studies, however, have not reached consensus on the effects of educational game types on learning. Romero et. al. ( 2015 ) argued that strategy games could foster critical thinking better than role-playing and competitive games. In contrast, Mao et. al. ( 2022 ) found that role-playing games were the best for developing thinking skills.
Game time: Game time has been the focus of previous studies (e.g., Riopel et al., 2019 ; Tsai & Tsai, 2018 ; Wouters et al., 2013 ). There were two different views on whether the effect of DGBL depended on the length of the game time. One common-sense view was that, multiple sessions in digital educational games would provide students with more opportunities to learn for achieving higher level learning than a single intervention (Clark et al., 2016 ). For example, Wouters et. al. ( 2013 ) found that multiple interventions in digital educational games were more effective than a single intervention. A different view, however, pointed to the novelty effect in new technology-based learning (e.g., an educational game). Learners were temporarily interested in learning due to their curiosity about new technologies, but this interest would weaken with time (Poppenk et al., 2010 ). For example, Riopel et. al. ( 2019 ) found that the longer the game time, the worse the science learning gains. Therefore, it should be worthwhile to examine the potential effect of game time on DGBL.
Level of realism : The level of realism in a game is regarded as one of the important game attributes. Wilson et. al. ( 2009 ) defined the level of realism as the similarity between the game scene and the real environment. Based on previous review studies (Clark et al., 2016 ; Riopel et al., 2019 ), game realism could be divided into three levels: schematic, cartoon-like, and photorealistic. In schematic games, the game environment is mainly composed of lines, geometry, or text (e.g., Hodges et al., 2018 ). The cartoon-like game has cartoon characters, and the game environment is mainly 2D (e.g., Vanbecelaere et al., 2020 ). Photorealistic game has game character similar to human, and the game environment is close to the real environment (e.g. C. -Y. Chen et al., 2020 ). It is not clear how game-based STEM learning achievement is affected by the level of realism of a game. Some empirical studies showed that learners could achieve excellent STEM academic performances in a highly realistic game environment (Fiorella et al., 2018 ; Jong, 2015 ; Okutsu et al., 2013 ). However, some reviews found that less realistic games appeared to enhance game-based science learning more than photorealistic games (Riopel et al., 2019 ). Therefore, this study will examine the potential moderating effect of the level of realism.
Game mechanism : The game mechanism reflects the complex relationship between learning and play in game-based learning (Mayer, 2015 ). As discussed by Arnab et. al. ( 2015 ), the game mechanism consists of the learning mechanism and the gaming mechanism. Learning mechanisms (e.g., concept maps, feedback) refer to game elements related to the learning content, and the addition and absence of which can change the learning process (e.g., Hwang et al., 2013 ). In contrast, gaming mechanics (e.g., points, leaderboards) are game elements that are not related to the learning content, but instead, are designed to increase entertainment and gaming experience (e.g., Hsu & Wang, 2018 ). Some previous empirical studies showed that digital educational games with added learning mechanisms could significantly promote STEM knowledge or skills (Hwang et al., 2013 ; Khamparia & Pandey, 2018 ; Sung & Hwang, 2013 ). Some studies also indicated that games with some added gaming mechanics could be beneficial for students learning (Hsiao et al., 2014 ; Hsu & Wang, 2018 ; Nelson et al., 2014 ). Tsai and Tsai ( 2020 ), however, reviewed 12 studies on science learning and did not find beneficial effects of added gaming mechanics or learning mechanics. In general, it remains unclear how game mechanism could moderate the effects of digital educational games on STEM learning. Thus, we will examine the potential moderating effects of game mechanisms in this study.
Study features
Educational level : Whether digital educational games promote academic performance for learners at all education levels has been a topic of interest. Previous review studies involving different STEM fields have examined the moderating role of education level, but have not reached a consistent conclusion. Riopel et. al. ( 2019 ) found that high school students benefited more from game-based science learning than elementary and college students. However, the meta-analysis by Tsai and Tsai ( 2020 ) showed that there was no significant difference in science academic performance among students at different education levels. In view of these inconsistent results, this study will investigate whether there are differences in game-based STEM learning effects among students at different education levels.
Subject : STEM education includes science, technology, engineering and mathematics. The effect of digital educational games may be different among different STEM disciplines. For example, Cheng et. al. ( 2015 ) argued that digital educational games were particularly suitable for science learning because DGBL provided a simulation-based environment, where students could experience relevant phenomena that could not be experienced in traditional classroom settings. Some meta-analyses also showed that DGBL was more beneficial for science learning than for other fields (Talan et al., 2020 ; Wouters & van Oostendorp, 2013 ). Other meta-analytical reviews, however, found that digital educational games appeared to be more beneficial for students to acquire mathematical knowledge and skills (C. -H. Chen et al., 2020 ; Wouters et al., 2013 ). Furthermore, some reviews found that digital educational games did not seem to be suitable for engineering education or computer science compared to other subjects (Talan et al., 2020 ; Wouters et al., 2013 ). Based on the above findings, the current meta-analysis considers different STEM disciplines as a potential moderator.
Learning outcome : Supporting learners to achieve excellent learning outcomes has always been a core goal of digital educational games. Consistent with the previous studies (e.g., Riopel et al., 2019 ; Tsai & Tsai, 2020 ), we focus on the cognitive outcomes of learning. Wouters et. al. ( 2009 ) divided cognitive outcomes into knowledge and cognitive skills. Knowledge, including declarative knowledge and procedural knowledge, was expressed as learning gain (Hooshyar et al., 2021 ), retention (Guo & Goh, 2016 ), or recall (Hsiao et al., 2014 ). Cognitive skills involved the use of rules to solve problems or make decisions, and were measured in terms of transfer (Aladé et al., 2016 ) or problem-solving (Sarvehana, 2019 ). Digital educational games could enhance STEM learning such as STEM concepts and problem-solving skills (NRC, 2011 ). However, some empirical studies suggest that the positive effects of digital educational games seemed to vary by learning outcomes. For example, Huang et. al. ( 2020 ) found that digital educational games promoted problem-solving skills more than STEM knowledge gains. Furthermore, previous meta-analyses involving STEM fields have only found positive effects of digital educational games on knowledge acquisition, and the effects on cognitive skills have not been examined (e.g., Riopel et al., 2019 ; Tsai & Tsai, 2020 ). Therefore, we consider the learning outcomes as a potential moderator for the effect of DGBL.
Aim of the present study
Digital educational games have great potential to enhance STEM learning. However, there are inconsistent research findings about the effectiveness of game-based STEM learning, and there is also a lack of systematic review to shed light on the inconsistent findings from these empirical studies. As of now, most studies on game-based STEM learning generally fell into two categories: (1) to compare game-based STEM learning with traditional STEM instruction/learning, and (2) to examine the enhancement effect of added game-design elements through comparison with the base game version. Therefore, the current study would focus on these two types of studies by systemically synthesizing the findings of these two types of studies, and synthesizing the moderating effects of some game features and study features (reviewed above) on the results from different studies on game-based STEM learning.
Building on the findings of the previous meta-analysis, the current study would extend and refine our understanding of the effects of digital educational games and design on STEM learning. First, the current study would provide a more exhaustive quantitative review of the empirical research studies related to game-based STEM education, for the purpose of adequately reflecting the current state of affairs in this active research area. Second, the current review would extend STEM learning outcomes from knowledge gains to cognitive skills, which was not adequately addressed in previous reviews. Third, guided by the research framework of Mayer ( 2015 ), the current review would move beyond the sole focus on media comparison research studies, and the value-added research studies about the effects of game design factors on STEM learning would be synthesized. Fourth, the current quantitative review would use a cutting-edge three-level meta-analytic model so as to avoid the analytical pitfalls and biases as a result of the non-independent multiple effect sizes within one study.
Practically, the current study would perform two meta-analyses, one to focus on the effect of game-based STEM learning in comparison with traditional STEM instruction/learning (media comparison research; Mayer, 2015 ), and the other to focus on the enhancement effect of added game-design elements in STEM learning in comparison with a base game version (value-added research; Mayer, 2015 ). In each of these two meta-analyses, potential moderator variables would be examined for their roles in contributing to the inconsistent findings across the studies as observed in the research literature, the research framework is visualized in Fig. 1 . More specifically, these two meta-analyses would answer the following questions:
Meta-analysis 1: Based on the studies that compared game-based STEM learning with traditional STEM learning:
RQ1a: What is the general effect of using digital games in STEM education?
RQ1b: Are the effects of game-based learning across different studies as analyzed in RQ1a moderated by game features and study features (i.e., moderator variables)
Meta-analysis 2: Based on the studies that compared two game-based STEM learning conditions: one involving a base game version, and the other involving the same game but with some added game-design elements:
RQ2a: What is the enhancement effect of added game-design element over a base game version in game-based STEM learning?
RQ2b: Are the enhancement effects of some added game-design elements across different studies moderated by game features and study features (i.e., moderator variables)?
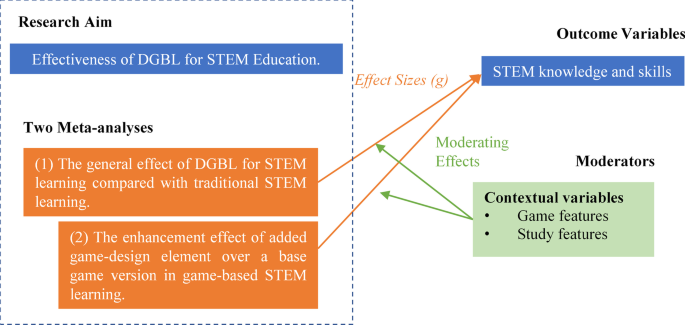
Research framework
Literature retrieval and screening
The current meta-analysis strictly followed the Preferred Reporting Items for Systematic Reviews and Meta-Analyses (PRISMA) criterion to identify relevant empirical research studies (Ziegler et al., 2011 ). We used three sets of keywords, games (“game-based learning” OR “serious game*” or “educational game*” OR “simulation game*” OR “electronic game*” OR “digital game*” “computer game*” OR “video game*”), AND STEM (“science or biology” or “physics” or “chemistry” or “math*” or “technology” or “engineering” or “STEM”), AND learning outcome (“academic achievement” OR “academic performance” OR “student performance” OR “learning outcome*” OR “learning effect*” OR “learning performance”) to screen the literature in the databases: Web of Science , EBSCOhost , and ScienceDirect. . In addition, Google Scholar , ResearchGate , and library document delivery were also used to find unpublished studies. The deadline for the literature search was August 2021. Finally, a total of 2802 articles were initially identified and imported into EndNoteX9 for further examination. After duplicated articles removed, the title and the abstract of each of the remaining 2343 entries were examined by the research team for its relevance and appropriateness for the current meta-analysis. After initial screening based on each article’s title and abstract by checking whether a study was an experimental or quasi-experimental study about the effect of DGBL in STEM education, we narrowed down the list to 467 studies for further full-text reading. The full text screening process was conducted by two authors independently first, and a very limited number of discrepancies between the two authors were later resolved by discussion in the research team. To sift these studies, we developed and used the inclusion/exclusion criteria shown in Table 2 . Eventually, 123 studies were identified to have met the inclusion criteria, thus included in one of the two meta-analyses. The flow of screening studies is shown in Fig. 2 . The inter-rater reliability coefficient (Krippendorff’s alpha) for the final screening results was greater than 0.95.
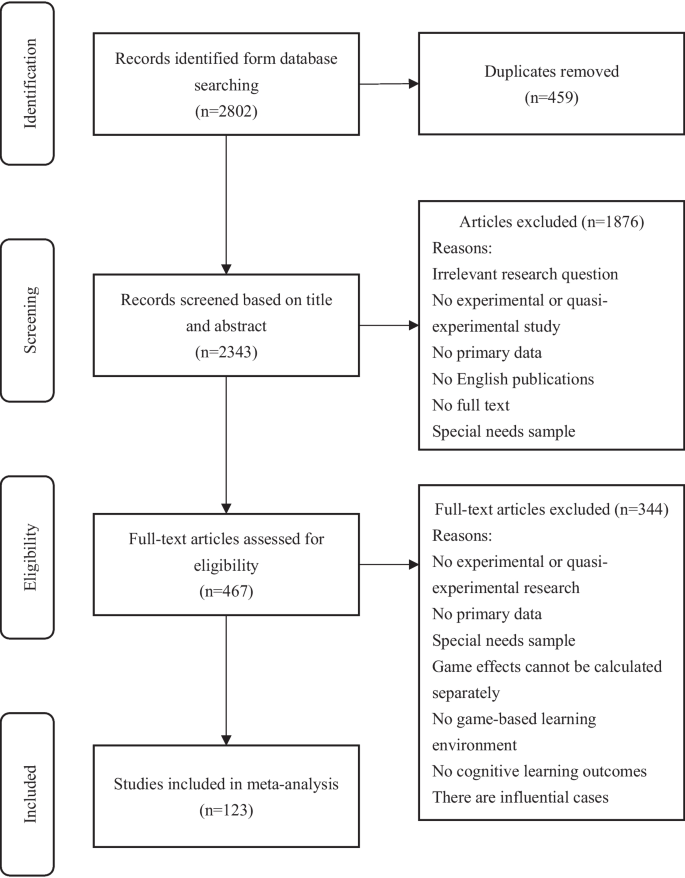
PRISMA diagram
Coding of studies
As described under “ Potential moderators ”, several salient characteristics (i.e., game features and study features) were considered potential moderators that could have influenced the findings across the primary studies. These moderator variables were coded into different levels, and the coding details of these moderator variables are presented in Table 3 . To ensure the reliability of the coding, three researchers independently coded 25 randomly selected primary studies (20% of all studies). The coding consistency coefficient among the three coders was calculated and the coding results were highly reliable ( κ = 0.81). Differences arising in the process of coding were negotiated among the three coders by consulting the original literature. Once the coding procedures established, differences among the coders resolved, and the coding reliability established, then the remaining studies were randomly divided into three groups, with each coder independently coding one group of the studies.
Quality assessment and influential effect sizes detection
We conducted quality assessment for included empirical studies using the Medical Education Study Quality Instrument (MERSQI) (Reed et al., 2007 ). This 10-item instrument was designed to assess the quality of empirical research in six domains including study design, sampling, type of data, validity of evaluation instrument, data analysis and outcomes. The full score of MERSQI is 18, with each item scoring from 0 to 3. The quality of empirical studies included in the current meta-analysis was considered adequate when the mean on the MERSQI was greater than 9 (Smith & Learman, 2017 ).
Most researchers agree that the outliers and influential case diagnostics should be examined before conducting a meta-analysis, because previous studies have showed that they could affect the validity and robustness of the conclusions from a meta-analysis (Viechtbauer & Cheung, 2010 ). In the current study, we used influential case diagnostics (e.g., Cook’s distances, DFBETAS, and studentized deleted residuals) to detect outliers (Viechtbauer & Cheung, 2010 ). When an outlier was identified, it was removed in subsequent data analyses, as this would increase the precision of the estimated average effect sizes (Viechtbauer, 2010 ).
Statistical analyses
We used R 4.0.5 (R Core Team, 2021 ) to analyze data extracted from primary studies. Some studies contained multiple effect sizes, thus leading to the issue of nested data, which, as well-known in research literature, would lead to inflated correlation between variables (Borenstein et al., 2009 ) and biased estimates in meta-analysis. To handle this issue, we performed a multilevel meta-analysis (Cheung, 2014 ). In the multilevel meta-analysis, the sources of variation are divided into sampling variance, intra-study variance, and inter-study variance, which would statistically address the issue of non-independence of the multiple effect sizes within one study.
Data extracted from a primary study, including mean, standard deviation, and sample size, were used to calculate the effect size. If mean and standard deviation were not available in a study, other statistics ( t , F , or \({{\chi}^{2}}\) ) were used to estimate an effect size (Glass, 1981 ). Then, all the obtained effect sizes were converted to Hedges’ g (Hedges, 1981 ) to minimize the impact of small sample studies.
As described previously, in this study, two meta-analyses were performed. In both meta-analyses, three-level random-effects meta-analysis model was used for statistical analysis. More specifically, in each of the two meta-analyses, the random-effects model was used to estimate the overall effect of the study, in which model parameters were obtained through Restricted Maximum Likelihood Estimation (REML), and t tests were used to calculate regression coefficients and corresponding confidence intervals (Viechtbauer, 2010 ). Subsequently, the sampling variation (level 1) in the study was calculated, and the significance of variation within studies (level 2) and variation between studies (level 3) was determined by a one-tailed log-likelihood ratio test. Finally, the omnibus F test was used to assess whether the moderating effect was significant (Gao et al., 2017 ).
Finally, publication bias is an existing and widely discussed research problem in meta-analysis. It indicates that studies with larger effect sizes or significant results are more likely to be published than those with smaller effect sizes or insignificant results (Kuppens et al., 2013 ). In the current meta-analyses, Funnel Plot (Macaskill et al., 2001 ) and Trim-and-Fill Method (Duval & Tweedie, 2000 ) were used to detect publication bias. The symmetrical distribution of the funnel plot and the small number of studies suppressed (i.e., \({L}_{0}^{+}\leq 2\) ) would suggest a lack of evidence for publication bias. Furthermore, researchers tampering with data in changing a non-significant result into a significant result ( p -hacking) could lead to the presence of publication bias (Simonsohn et al., 2014 ). p -curve, as a new method for assessing p -hacking, was used to assess the publication bias due to p- hacking, with the p -curve skewed to the right indicating that p -hacking was impossible for the given data (Simonsohn et al., 2014 ).
The quality appraisal score of the MERSQI was 13.38 (SD = 1.02), which was much higher than the prescribed threshold (Table 4 ) for adequate quality of the included studies, suggesting that the quality of the studies included in the current meta-analyses was very good.
The results of influential effect sizes detections are not presented in the paper for space consideration. The graphs of the influential case diagnostics are available in Additional file 1 , which showed that 4 outliers were flagged in the first and second meta-analyses, respectively, and these outliers varied from 2.652 to 6.618. To avoid the undue impact of these outliers on the results, the outliers were deleted in the subsequent analyses.
Descriptive characteristics
The current meta-analytic data set included 123 studies yielding 217 effect sizes from a cumulative total 11,714 participants. For the first meta-analysis (i.e., studies that compared game-based STEM learning with traditional STEM learning), the number of included studies was 86 with 136 effect sizes. For the second meta-analysis (i.e., studies in which a base game version was compared with an enhanced game version containing an added game mechanics element), the number of studies was 44 with 81 effect sizes. The included studies were published between 2005 and 2020, with the majority (97%) published after 2010. Most studies were published journal articles (89%) and involved the pre–post-test design (86%). The study samples included students of primary school and below (40%), junior high school students (22%), senior high school students (13%), and college and university students (25%). The included studies were conducted mainly in Europe (24%) and Asia (50%), and the studies involved STEM areas of science (50%), mathematics (25%) and computer courses (20%). The types of games used in the studies were mainly puzzle (38%), role-playing (27%), and simulation (14%) games. Half of the games were cartoon-like, 24% were photorealistic and 13% were schematic.

Meta-analysis 1: general effect of game-based STEM learning (RQ1a)
The first meta-analysis focused on the general effect of game-based STEM learning when compared with traditional STEM learning without using digital games. As described previously, 86 studies that compared digital game-based STEM learning with traditional STEM learning without the use of digital games were used in this meta-analysis, and a total of with 136 effect sizes from these primary studies were quantitatively synthesized. These effects sizes are shown graphically in Fig. 3 in the form of forest plot. The overall effect of digital game-based STEM learning over traditional STEM learning was shown to be larger than a medium effect ( g = 0.624, t = 7.403, p < 0.001, 95% CI [0.457, 0.790]), indicating that students using digital educational games in STEM learning significantly outperformed their counterparts who used alternative learning activities in STEM learning. The one-tailed log likelihood ratio test for the variation of the effect sizes suggested significant variations both within and between studies ( Q (135) = 2095.093, p < 0.001, \({I}_{2 }^{2}\) = 38.965%, \({I}_{3 }^{2}\) = 54.479%), indicating statistical heterogeneity among the effect sizes that warrant follow-up analysis for potential moderators (see Table 5 , upper panel).
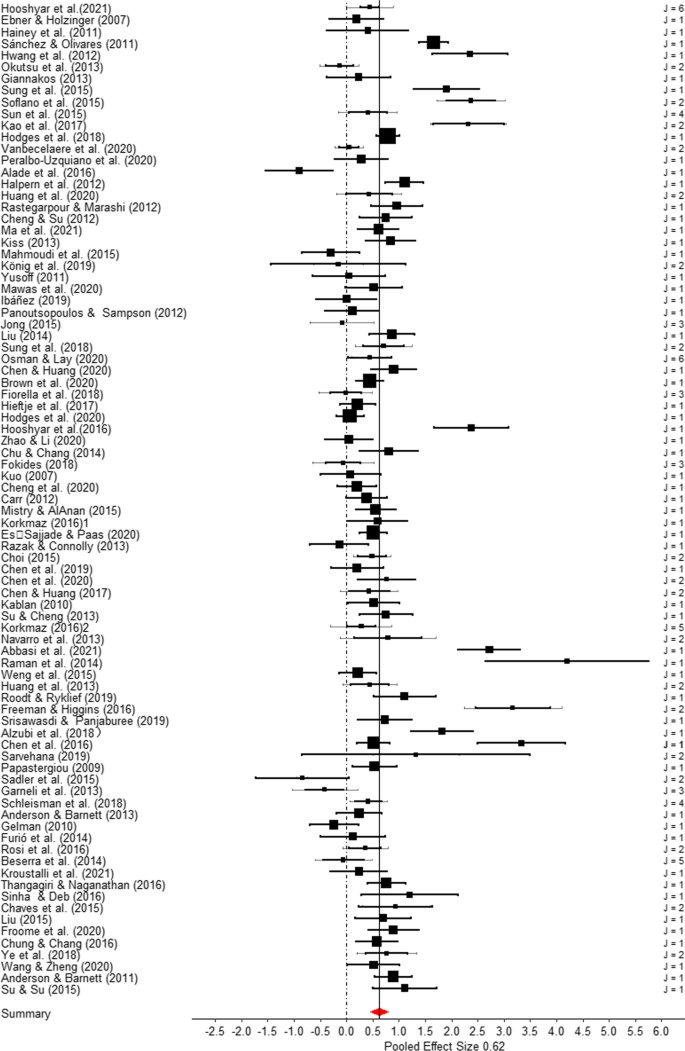
Forest plot of meta-analysis 1 (game-based STEM learning vs. alternative activities in STEM learning)
Moderator analysis for meta-analysis 1 (RQ1b)
The three-level mixed-effects model identified game type as a significant moderator ( F (6, 119) = 2.229, p < 0.05). Specifically, studies involving strategy games showed extremely large effect sizes ( g = 1.841), while those involving role-playing ( g = 0.586), puzzle ( g = 0.551) and adventure ( g = 0.701) games showed effect sizes that were medium and above, Studies involving action ( g = 0.394) and simulation ( g = 0.464) games showed effect sizes between small and medium. As too few studies involved construction games, its effect size should not be trusted or interpreted.
There were significant differences in effect sizes from the studies that involved different STEM subject areas ( F (3, 132) = 5.7, p < 0.01). Studies that used game-based learning in computer courses had very large average effect size ( g = 1.077), and the studies using game-based learning in science courses showed above medium average effect size ( g = 0.674). Studies involving game-based learning in mathematics courses ( g = 0.179) and in engineering courses ( g = 0.271) showed small average effect sizes.
The types of learning outcomes also appeared to be a significant moderator of the effect sizes from different studies ( F (2, 133) = 4.273, p < 0.05), with the studies involving cognitive skills as measured outcome showing much larger average effect size ( g = 0.91; a large effect) than the studies that measured knowledge as the learning outcome ( g = 0.538; a medium effect). Other game or study features (game time, game realism, participants’ educational level did not turn out to be statistically significant moderators for effect sizes across the studies (see Table 6 ).
Meta-analysis 2: enhancement effect of added game-design elements in STEM learning (RQ2a)
The second meta-analysis focused on the enhancement effect of some added game-design mechanics. As described above, 44 studies that compared STEM learning condition of using a base game version and the condition of using the same game but with some added game-design elements were used in this meta-analysis, and a total of with 81 effect sizes from these primary studies were obtained and quantitatively synthesized. These effects sizes are shown graphically in Fig. 4 in the form of forest plot. The three-level meta-analysis revealed that games with added game-design mechanics showed enhancement effect over the base game version condition for improving students’ STEM learning ( g = 0.301, t = 4.342, p < 0.001, 95% CI [0.163, 0.438]). Furthermore, the three-level random effect model showed that both within-study variance ( p < 0.001) and between-study heterogeneity among the effect sizes were statistically significant ( Q (80) = 357.037, p < 0.001, \({I}_{2 }^{2}\) = 36.682%, \({I}_{3 }^{2}\) = 43.160%) (see Table 5 , lower panel), indicating the need for moderator analysis to identify what game or study features could have contributed to the inconsistent effect sizes across the studies.
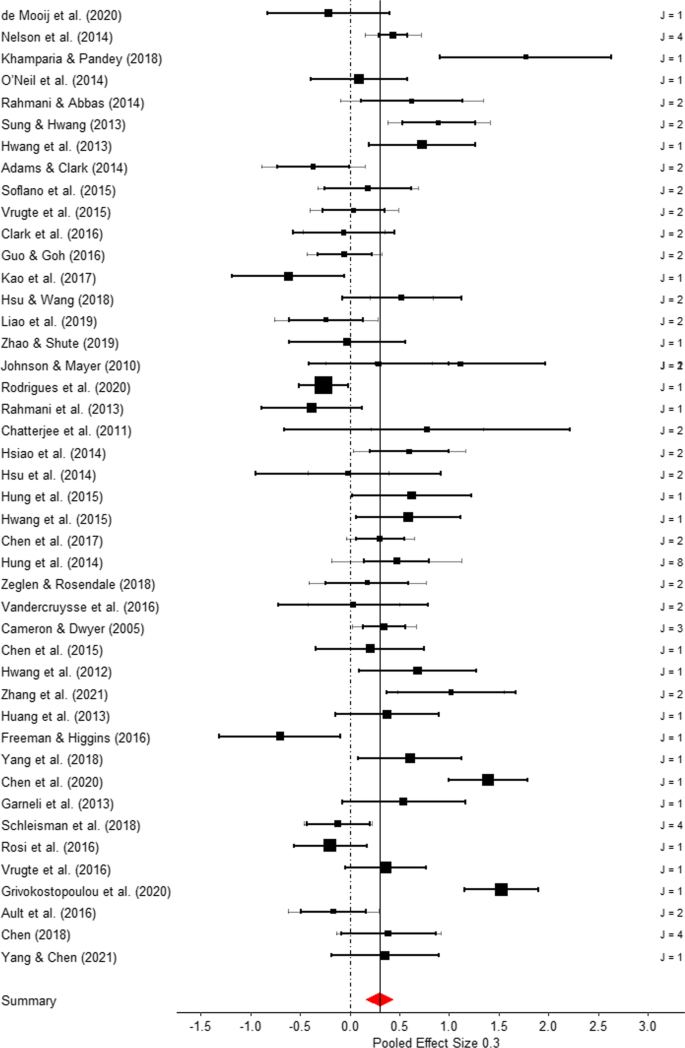
Forest plot of meta-analysis 2 (enhancement effect of added game-design element over base game version)
Moderator analysis for meta-analysis 2 (RQ2b)
The results of moderating effect analysis showed that learning outcome ( F (1, 79) = 6.055, p < 0.016) was a likely moderator for the observed inconsistent findings about the enhancement effect of added game-design element over base game for STEM learning. More specifically, with regard to the enhancement effect of added game-design element in STEM learning, in the studies that measured knowledge as learning outcome, the average effect size of such enhancement effect was 0.428 (a medium effect size), compared with a very small average effect size of 0.091 from the studies that measured cognitive skill as the learning outcome.
In this study, two types of added game-design element were coded: game element added for improving gaming experience (For Gaming), or game element added for learning content (For Learning). It turned out that, the enhancement effect of the added game-design element differed depending on which type of added game-design element: for gaming vs. for learning ( F (1, 79) = 4.315, p < 0.05). In the studies with added game-design element for gaming, the average enhancement effect is small ( g = 0.175), in contrast to the average enhancement effect of almost medium effect size ( g = 0.432) from the studies with added game-design element for learning. Other game features or study features (publication type, game type, level of realism, sample’s education level, or STEM subject content area) were statistically not significant (see Table 7 ).
Publication bias
For meta-analysis 1: The funnel plot appeared to be symmetrical, and Trim-and-fill method showed that the \({L}_{0}^{+}\) value was 0, far lower than the recommended threshold \(({L}_{0}^{+}=2)\) for publication bias. In addition, the right-skewness test of the p- curve analysis was statistically significant ( Z = − 24.664, p < 0.001), suggesting no evidence for p- hacking (Additional file 1 ).
For meta-analysis 2: The funnel plot appeared to be symmetrical, and the Trim-and-fill method showed a \({L}_{0}^{+}\) value of 0, suggesting no evidence for publication bias. In addition, the p- curve analysis showed that the curve had statistically significant right-skewness ( Z = − 8.465, p < 0.001), indicating no evidence for p- hacking (see Additional file 1 ).
Effect of DGBL in STEM learning and enhancement effect of game-design elements
DGBL, as a pedagogical strategy for STEM education, may promote learning motivation, enhance STEM knowledge and develop problem-solving skills (Klopfer & Thompson, 2020 ). While game-based STEM learning may benefit student STEM learning, previous empirical studies have not reached consistent conclusions on the effectiveness of DGBLs. To fill the research gaps, the current study, following the research framework proposed by Mayer ( 2015 , 2019 ), comprehensively and systematically reviewed two types of previous empirical studies on game-based STEM learning:
Studies that compared game-based STEM learning with traditional STEM learning with other alternatives. In the research framework proposed by Mayer ( 2015 ), this research direction was defined as “media comparison research”. The purpose of “media comparison research” is to assess the effect of game-based STEM learning over traditional STEM learning. Our first meta-analysis quantitatively summarized the findings across these studies of “media comparison research” in the literature;
Studies that compared two different conditions of game-based STEM learning: one condition involved the use of a base game version, and the other condition involved the use of the same game but with some added game-design elements. In the research framework proposed by Mayer ( 2015 ), this research direction was defined as “value-added research”. The purpose of this research direction is to assess the enhancement effect of the added game-design elements over the base game. Our second meta-analysis quantitatively summarized the findings across the studies of “value-added research” in the literature.
The findings from the first meta-analysis for the studies of “media comparison research” revealed that students with game-based STEM learning significantly outperformed their counterparts with traditional STEM learning, and the overall effect was a medium to large effect size of g = 0.624 across all the studies. Consistent with previous empirical and also meta-analysis studies (Brown et al., 2020 ; C. -L.D. Chen et al., 2016 , C. -H. Chen et al., 2016 ; Halpern et al., 2012 ), this finding provides strong support that DGBL is likely an effective pedagogical strategy for promoting STEM education. The findings also lend support to the cognitive theory of game-based learning connecting playing game and cognitive development (Mayer, 2020 ).
The findings from the second meta-analysis for the studies of “value-added research” showed an overall small to medium effect size ( g = 0.301), indicating that across all the studies that examined the enhancement effect of added game-design elements in game-based STEM learning, students using games with added game-design elements outperformed their counterparts who used the base game. This result is consistent with the findings of a previous review of game mechanic design (Tsai & Tsai, 2018 , 2020 ), in which it was suggested that adding some game mechanics in education game could improve the effect of digital game-based learning. Further, the review findings of this study responded well to the call by Mayer ( 2019 ) for more empirical evidence on the effectiveness of DGBL, and on the value of game mechanics for enhancing learning.
Factors contributing to inconsistent findings across the studies
The statistically significant heterogeneity tests for the effect sizes across the studies in both meta-analyses in this study suggested that the empirical findings about game-based STEM learning varied across the studies in the literature. To gain a better understanding of the underlying factors contributing to such inconsistent findings as observed in the research literature about DGBL, we investigated the potential moderating variables (including game characteristics and study features) in both meta-analyses conducted in this study.
Moderating variables in meta-analysis 1
In the first meta-analysis on “media comparison research” studies, which compared game-based STEM learning vs. STEM learning without the use of digital games, three game/study features (game type, subject area, and learning outcome) were shown to have statistically contributed to the variations of effect sizes across the studies, while other three game/study features (education level, game time, and level of realism) were not such statistical contributors.
For game type, seven types of games were coded for moderator analysis, and these seven types of games showed average effect sizes ranging from 0.394 to 1.841, with strategy games showing largest effect size ( g = 1.841). This finding suggests that strategy games could be more effective in the STEM learning environment than other types of games. The report by NRC ( 2011 ) discussed that an effective STEM learning environment should include handling cognitively demanding tasks, focusing on complex problem solving, and engaging in sound planning. In contrast to other types of games, a strategy game tends to involve complex tasks with an emphasis on tactics and on long-term planning (Gerber & Scott, 2011 ). Learners need to carefully evaluate and analyze problems, and actively develop critical thinking and problem-solving skills for success in a strategy game. Compared with strategy games, other game types, such as simulation, puzzle solving and role-playing, do not require learners to invest as much cognitive resources to solve problems. The review by Romero et. al. ( 2015 ) also pointed out that players in strategy games showed higher critical problem-solving ability than those playing other types of games. Therefore, a well-designed strategy game may provide students with a more effective STEM learning environment, thereby helping students achieve better learning benefits. It is worth noting that there have been relatively few empirical studies focusing on strategy games (and on some other types such as action, and building games), and more research is needed to focus on these games in the future.
With regard to STEM subject area, the results of current meta-analysis indicated that the positive effect of game-based learning was much larger from the studies involving science and computer learning as STEM areas ( g = 0.674 and 1.077 for science and computer learning, respectively), but the effect sizes were much smaller from the studies involving math and engineering learning ( g = 0.179 and 0.271 for math and engineering learning, respectively). These findings are consistent with some previous reviews (Talan et al., 2020 ; Wouters & van Oostendorp, 2013 ) suggesting that digital educational games are powerful tools to support science learning. Tsai and Tsai ( 2020 ) pointed out that the effectiveness of game-based science learning came from a well-designed game environment and strong instructional support. For computer science, DGBL could have advantages over other learning methods in multiple areas, such as learner-centricity, interactivity, and immediate feedback (Kazimoglu, 2020 ). These advantages not only could motivate students to immerse themselves in tedious programming practices, but could also support the implementation of basic programming constructs to ease the difficulty of learning to do programming. Some empirical studies on computer programming also showed that digital educational games could enhance learning more than other instructions (Chaves et al., 2015 ; Freeman & Higgins, 2016 ).
Although game-based STEM learning significantly and positively enhanced STEM knowledge and cognitive skills, the studies involving cognitive skills as learning outcome had large effect size ( g = 0.91) than those involving STEM knowledge as learning outcome ( g = 0.538). The report by NRC ( 2011 ) discussed that DGBL for STEM field involving high cognitive tasks and complex problems focused more on enhancing cognitive skills such as creative thinking and problem solving. Similarly, Klopfer and Thompson ( 2020 ) also discussed that game-based learning environment that provides students with simulation opportunities, problem-solving strategies and various instructional support could effectively improve students’ higher-order cognitive abilities. Therefore, digital educational games in the STEM field may promote students’ cognitive skills more than their knowledge acquisition (C. -Y. Chen et al., 2020 ; Huang et al., 2020 ).
Several game/study features (game time, game realism, education level) did not turn out to be statistically significant moderators for the effect sizes across the studies, despite some descriptive differences in the average effect sizes under different conditions. As these game/study features were statistically non-significant in the moderator analysis, we refrained from any further discussion on these variables.
Moderating variables in meta-analysis 2
In the second meta-analysis on “value-added research” studies, which compared two conditions of game-based STEM learning: using base game version vs. using the same game but with added game-design elements. The results of the moderator analysis showed that two game/study features (game-design element, learning outcome) were shown to have statistically contributed to the variations of effect sizes across the studies, while other game/study features (game type, game time, level of realism, educational level, and STEM subject area) were not such statistical contributors.
With regard to the statistically significant moderator of “game-design element” in Table 7 , in the “value-added research” studies, the primary focus was on the enhancement effect of added game-design elements over the same game but without such added game-design elements. Previously in Table 5 (“Results for the Overall Effects of Two Meta-Analyses”), it was already shown that the added game-design elements had positive enhancement effect ( g = 0.301) on STEM learning across all the “value-added research” studies. The moderator analysis results shown in Table 7 further informed us that the two types of added game-design elements (design elements for gaming experience, design elements for content learning) showed different enhancement effects, with game-design elements intended for content learning showing larger enhancement effect ( g = 0.432; a medium effect size) than those intended for gaming experience ( g = 0.175; a small effect size).
The cognitive theory of game-based learning could provide a possible explanation for this finding (Mayer, 2020 ), which stipulates that the effectiveness of DGBL stems from its powerful learning mechanism that minimizes irrelevant cognitive processing and promotes generative processing related to learning tasks. On the other hand, digital educational games with excessive gaming elements or mechanisms may distract learners’ attention from the learning tasks, and result in extra cognitive processing that may overburden the limited cognitive processing capacity of students. Some previous studies also showed that digital educational games with some gaming mechanic elements (e.g., narrative, multiplayers) did not perform better than the games without these gaming mechanic elements (Tsai & Tsai, 2020 ; Wouters et al., 2013 ).
With regard to learning outcome, the results of moderating effect analysis showed that the enhancement effect of added game-design elements showed larger effect ( g = 0.428) in studies with STEM knowledge as outcome, but very small enhancement effect in studies with cognitive skills as outcome ( g = 0.091). This suggests game learning with added game mechanic elements significantly enhanced STEM knowledge acquisition, but did little for cognitive skills. Previously in our first meta-analysis for “media comparison research” studies, we observed that game-based STEM learning, when compared with traditional STEM learning, could be more effective for developing cognitive skills than for STEM knowledge acquisition. Here, in the second meta-analysis, however, the comparison was between game-based STEM learning with or without added game-design elements. Thus, the foci of these two meta-analyses, as well as the findings of the moderator analyses, were entirely different, thus not to be compared or interpreted together. It is very likely that what game-design elements to add to an educational game must match what is already in the game to have meaningful enhancement effect over the original game version.
As shown in Table 7 , five game/study features (game type, game time, level of realism, educational level, and STEM subject area) were not statistically significant moderators for the effect sizes across these “value-added research” studies investigating the enhancement effect of added game-design elements. Although there appeared to be some obvious descriptive differences in the average effect sizes across different levels of some of these game/study features (e.g., game type, subject), it should be noted that the numbers of included studies/effect sizes for some levels were too small for meaningful statistical comparisons. For example, under “game type”, the numbers of studies/effect sizes for Strategy games, Action games, Adventure games, and Simulation games were all small. Similarly, under STEM “Subject”, the number of studies/effect sizes for “Engineering” was also too small. The large variations in the effect sizes across the levels appeared to have been contributed by these levels with very small number of studies/effect sizes (e.g., under “Subject”, Engineering ( n = 1) had effect size of 1.528, while others had 0.223–0.295; under “Game Type”, the four game types with small n had widely varying effect sizes, while two game types with much larger n had mean effect sizes not much different from each other). Without the levels with very small n conditions, the descriptive differences for these moderator variables would be much smaller. It was likely that this could be a reason for the statistically non-significant tests for these moderators, despite some descriptive differences being obvious. As these game/study features were statistically non-significant in the moderator analysis, we refrained from any further discussion on these variables.
Limitations and future research directions
There are some deficiencies in the current research. First, due to the limited information available from the included studies, our meta-analyses reported only cognitive outcomes. Wouters et. al. ( 2009 ) discussed that the effects of DGBL are not only manifested in cognition, but also in motivation, emotion, and motor skills. Future research in DGBL should consider and measure other types of meaningful outcomes to provide more comprehensive evidence for the effectiveness of DGBL.
Second, limited by the information available from the included studies, in our second meta-analysis on the “value-added research” studies that investigated the enhancement effect of game-design mechanic elements, we were only able to roughly divide the added game mechanic elements into those for gaming experience and those for learning. In fact, game-design mechanics include other types, such as narrative, collaboration, multiplayers, feedback, concept maps, and coaching, etc. (Plass et al., 2020 ). Wouters et. al. ( 2013 ) believed that not all game-design mechanic elements were necessary for DGBL. Future research in DGBL may examine the effect of some specific game-design mechanic elements.
Finally, this study focused primarily on the moderating effects of game characteristics and study features from a cognitive perspective. However, some sociocultural attributes such as cultural differences may have an impact on game-based STEM learning (Wahono et al., 2020 ). Future research studies in DGBL may consider including sociocultural attributes in the studies, so that the potential effects of sociocultural attributes on game-based STEM learning can be better understood.
Findings and suggestions
Built upon previous studies on DGBL effectiveness in STEM education, the current study enhanced our understanding of the positive effect of game-based STEM learning on knowledge gains. In addition to extending and updating the findings of previous reviews on the general effect of DGBL on knowledge gains in STEM disciplines, the current study further suggested that STEM digital games were more effective for developing cognitive skills than for facilitating knowledge acquisition. This finding provides empirical support for the claim that game-based STEM education is particularly suitable for developing higher-order skills. Thus, we suggest that future research efforts should focus more on the facilitative effects of digital educational games on students’ cognitive skills. National Research Council ( 2011 ) indicated that DGBL is critical for improving learners’ cognitive skills in STEM education. Previous research has also shown that educational games could promote cognitive skills (C. -Y. Chen et al., 2020 ; Huang et al., 2020 ). Therefore, educators could increase the use of DGBL in STEM education that involve high levels of cognitive tasks and complex problems to promote students’ cognitive skills.
In addition, we also found that educational games appeared to be more effective for science and computer learning, and they were suitable for students of any educational level. On the other hand, game-play time and the level of realism of game screen did not appear to have impact on the effect of game-based STEM learning. These findings suggest that educational practitioners can consider more use of educational games in science and computing classrooms without too much concern about the potential impact of playtime and the level of realism of games on student’ academic performance. Furthermore, current work suggests that strategy digital games may be more effective than other types of digital games in STEM learning. Plass et. al. ( 2020 ) argued that, as an important gameplay design, game type may have a significant impact on the effectiveness of DGBL. Consistent with our finding, Romero et. al. ( 2015 ) argued that strategy games could foster learning better than role-playing and competitive games. Therefore, research should focus on the effect of game genre on the effectiveness of DGBL for STEM education in the future work.
The current study also sheds lights on some new aspects about feedback effectiveness that were not known previously. The new findings about the enhancement effect of game-design elements in our study revealed that, in general, enhanced game design outperformed the basic game version in enhancing students’ STEM academic performance. This empirical finding strongly suggests that more attention should be given to the game design elements for game-based STEM education. This finding also provides empirical support for an integrated design framework in game-based learning from a cognitive perspective (e.g., Plass et al., 2015 ), indicating that the application of appropriate game design elements could be useful for cognitive engagement. More importantly, the current synthesis also revealed that the added elements related to learning mechanism were more effective in promoting students’ cognitive performance than those related to gaming mechanism. Therefore, for the purpose of enhancing the effectiveness of digital games in STEM education, game designers should pay more attention to the design of learning mechanisms.
In summary, this meta-analytic review strengthened that digital games as instructional medium for STEM education are effective in promoting not only student’s knowledge gains but also their cognitive skills. More importantly, the findings suggest that game design mechanism could play an important role in terms of effectiveness of game-based STEM education. Hence, the focus of future research on game-based STEM learning should shift from the proof-of-concept research (i.e., whether STEM games are effective) to the value-added research (i.e., how to design games to make them more effective), so that future educational games can be more effective in STEM education.
Conclusions
This meta-analysis study makes a valuable contribution in enhancing our understanding about the effectiveness of DGBL about STEM learning and the enhancement effect of added game-design elements in STEM learning. We conclude form this study that students with DGBL significantly outperformed their counterparts with traditional learning in STEM education. Furthermore, we also conclude from this study that students using games with added game-design elements outperformed their counterparts who used the base game. To facilitate learning in digital game-based STEM learning, designers of games are encouraged to embed appropriate game elements (e.g., pedagogical agents, self-explanation strategies, concept maps, feedback, and adaptation) into the process of game-based learning. In addition, moderator analysis reveals that the inconsistent findings about the effectiveness of DGBL in STEM education are accounted for by game type, learning subject as well as learning outcome, and the inconsistent enhancement effect of game-design elements are accounted for by types of added game-design elements.
Availability of supporting data
Additional data to this article can be found online.
Adams, D. M., & Clark, D. B. (2014). Integrating self-explanation functionality into a complex game environment: Keeping gaming in motion. Computers & Education, 73 , 149–159. https://doi.org/10.1016/j.compedu.2014.01.002
Article Google Scholar
Aladé, F., Lauricella, A. R., Beaudoin-Ryan, L., & Wartella, E. (2016). Measuring with Murray: Touchscreen technology and preschoolers’ STEM learning. Computers in Human Behavior, 62 , 433–441. https://doi.org/10.1016/j.chb.2016.03.080
Arnab, S., Lim, T., Carvalho, M. B., Bellotti, F., de Freitas, S., Louchart, S., Suttie, N., Berta, R., & De Gloria, A. (2015). Mapping learning and game mechanics for serious games analysis. British Journal of Educational Technology, 46 (2), 391–411. https://doi.org/10.1111/bjet.12113
Arztmann, M., Hornstra, L., Jeuring, J., & Kester, L. (2023). Effects of games in STEM education: A meta-analysis on the moderating role of student background characteristics. Studies in Science Education, 59 (1), 109–145. https://doi.org/10.1080/03057267.2022.2057732
Ball, C., Huang, K.-T., Cotten, S. R., & Rikard, R. V. (2020). Gaming the SySTEM: The relationship between video games and the digital and STEM divides. Games and Culture, 15 (5), 501–528. https://doi.org/10.1177/1555412018812513
Becker, B. J. (2000). Multivariate meta-analysis. In S. D. Brown & H. E. A. Tinsley (Eds.), Handbook of applied multivariate statistics and mathematical modeling (pp. 499–525). Academic Press. https://doi.org/10.1016/B978-012691360-6/50018-5 .
Beserra, V., Nussbaum, M., Zeni, R., Rodriguez, W., & Wurman, G. (2014). Practising arithmetic using educational video games with an interpersonal computer. Educational Technology & Society, 17 (3), 343–358.
Google Scholar
Borenstein, M., Hedges, L. V., Higgins, J., & Rothstein, H. R. (2009). Introduction to meta analysis . Wiley.
Book Google Scholar
Brown, F. L., Farag, A. I., Hussein Abd Alla, F., Radford, K., Miller, L., Neijenhuijs, K., Stubbé, H., de Hoop, T., Abdullatif Abbadi, A., Turner, J. S., Jetten, A., & Jordans, M. J. D. (2020). Can’t wait to learn: A quasi-experimental mixed-methods evaluation of a digital game-based learning programme for out-of-school children in Sudan. Journal of Development Effectiveness . https://doi.org/10.1080/19439342.2020.1829000
Byun, J., & Joung, E. (2018). Digital game-based learning for K-12 mathematics education: A meta-analysis. School Science and Mathematics, 118 (3–4), 113–126. https://doi.org/10.1111/ssm.12271
Chaves, R. O., von Wangenheim, C. G., Costa Furtado, J. C., Bezerra Oliveira, S. R., Santos, A., & Favero, E. L. (2015). Experimental evaluation of a serious game for teaching software process modeling. IEEE Transactions on Education, 58 (4), 289–296. https://doi.org/10.1109/te.2015.2411573
Chen, C.-L.D., Chang, C.-Y., & Yeh, T.-K. (2016). The effects of game-based learning and anticipation of a test on the learning outcomes of 10th grade geology students. Eurasia Journal of Mathematics, Science and Technology Education, 12 (5), 1379–1388. https://doi.org/10.12973/eurasia.2016.1519a
Chen, C.-Y., Huang, H.-J., Lien, C.-J., & Lu, Y.-L. (2020). Effects of multi-genre digital game-based instruction on students’ conceptual understanding, argumentation skills, and learning experiences. IEEE Access, 8 , 110643–110655. https://doi.org/10.1109/access.2020.3000659
Chen, C.-H., Liu, G.-Z., & Hwang, G.-J. (2016). Interaction between gaming and multistage guiding strategies on students’ field trip mobile learning performance and motivation. British Journal of Educational Technology, 47 (6), 1032–1050. https://doi.org/10.1111/bjet.12270
Chen, C.-H., Shih, C.-C., & Law, V. (2020). The effects of competition in digital game-based learning (DGBL): A meta-analysis. Educational Technology Research and Development, 68 (4), 1855–1873. https://doi.org/10.1007/s11423-020-09794-1
Cheng, M.-T., Chen, J.-H., Chu, S.-J., & Chen, S.-Y. (2015). The use of serious games in science education: A review of selected empirical research from 2002 to 2013. Journal of Computers in Education, 2 (3), 353–375. https://doi.org/10.1007/s40692-015-0039-9
Cheung, M. W. L. (2014). Modeling dependent effect sizes with three-level meta-analyses: A structural equation modeling approach. Psychological Methods, 19 (2), 211–229. https://doi.org/10.1037/a0032968
Clark, D. B., Tanner-Smith, E. E., & Killingsworth, S. S. (2016). Digital games, design, and learning: A systematic review and meta-analysis. Review of Educational Research, 86 (1), 79–122. https://doi.org/10.3102/0034654315582065
Dou, L. (2019). A cold thinking on the development of STEM education. In 2019 international joint conference on information, media and engineering (IJCIME) (pp. 88–92). https://doi.org/10.1109/IJCIME49369.2019.00027
Duval, S., & Tweedie, R. (2000). Trim and fill: A simple funnel-plot-based method of testing and adjusting for publication bias in meta-analysis. Biometrics, 56 (2), 455–463. https://doi.org/10.1111/j.0006-341X.2000.00455.x
Fiorella, L., Kuhlmann, S., & Vogel-Walcutt, J. J. (2018). Effects of playing an educational math game that incorporates learning by teaching. Journal of Educational Computing Research, 57 (6), 1495–1512. https://doi.org/10.1177/0735633118797133
Freeman, B., & Higgins, K. (2016). A randomised controlled trial of a digital learning game in the context of a design-based research project. International Journal of Technology Enhanced Learning, 8 (3–4), 297–317. https://doi.org/10.1504/ijtel.2016.082316
Gao, F., Li, L., & Sun, Y.-Y. (2020). A systematic review of mobile game-based learning in STEM education. Educational Technology Research and Development, 68 (4), 1791–1827. https://doi.org/10.1007/s11423-020-09787-0
Gao, S., Assink, M., Cipriani, A., & Lin, K. (2017). Associations between rejection sensitivity and mental health outcomes: A meta-analytic review. Clinical Psychology Review, 57 , 59–74. https://doi.org/10.1016/j.cpr.2017.08.007
Gerber, S., & Scott, L. (2011). Gamers and gaming context: Relationships to critical thinking. Journal of Educational Technology, 42 (5), 842–849. https://doi.org/10.1111/j.1467-8535.2010.01106.x
Giannakos, M. N. (2013). Enjoy and learn with educational games: Examining factors affecting learning performance. Computers & Education, 68 , 429–439. https://doi.org/10.1016/j.compedu.2013.06.005
Glass, G. (1981). Meta-analysis in social research . Sage. https://doi.org/10.1016/0149-7189(82)90011-8
Grivokostopoulou, F., Kovas, K., & Perikos, I. (2020). The effectiveness of embodied pedagogical agents and their impact on students learning in virtual worlds. Applied Sciences, 10 (5), 1739. https://doi.org/10.3390/app10051739
Guo, Y. R., & Goh, D.H.-L. (2016). Evaluation of affective embodied agents in an information literacy game. Computers & Education, 103 , 59–75. https://doi.org/10.1016/j.compedu.2016.09.013
Halpern, D. F., Millis, K., Graesser, A. C., Butler, H., Forsyth, C., & Cai, Z. (2012). Operation ARA: A computerized learning game that teaches critical thinking and scientific reasoning. Thinking Skills and Creativity, 7 (2), 93–100. https://doi.org/10.1016/j.tsc.2012.03.006
Hedges, L. V. (1981). Distribution theory for Glass’s estimator of effect size and related estimators. Journal of Educational Statistics, 6 (2), 107–128. https://doi.org/10.2307/1164588
Hodges, G. W., Wang, L., Lee, J., Cohen, A., & Jang, Y. (2018). An exploratory study of blending the virtual world and the laboratory experience in secondary chemistry classrooms. Computers & Education, 122 , 179–193. https://doi.org/10.1016/j.compedu.2018.03.003
Hooshyar, D., Malva, L., Yang, Y., Pedaste, M., Wang, M., & Lim, H. (2021). An adaptive educational computer game: Effects on students’ knowledge and learning attitude in computational thinking. Computers in Human Behavior, 114 , 106575. https://doi.org/10.1016/j.chb.2020.106575
Hsiao, H.-S., Chang, C.-S., Lin, C.-Y., Chang, C.-C., & Chen, J.-C. (2014). The influence of collaborative learning games within different devices on student’s learning performance and behaviours. Australasian Journal of Educational Technology, 30 (6), 652–669. https://doi.org/10.14742/ajet.347
Hsu, C.-C., & Wang, T.-I. (2018). Applying game mechanics and student-generated questions to an online puzzle-based game learning system to promote algorithmic thinking skills. Computers & Education, 121 , 73–88. https://doi.org/10.1016/j.compedu.2018.02.002
Huang, S.-Y., Kuo, Y.-H., & Chen, H.-C. (2020). Applying digital escape rooms infused with science teaching in elementary school: Learning performance, learning motivation, and problem-solving ability. Thinking Skills and Creativity, 37 , 100681. https://doi.org/10.1016/j.tsc.2020.100681
Hwang, G.-J., Yang, L.-H., & Wang, S.-Y. (2013). A concept map-embedded educational computer game for improving students’ learning performance in natural science courses. Computers & Education, 69 , 121–130. https://doi.org/10.1016/j.compedu.2013.07.008
Ishak, S. A., Din, R., & Hasran, U. A. (2021). Defining digital game-based learning for science, technology, engineering, and mathematics: A new perspective on design and developmental research. Journal of Medical Internet Research, 23 (2), e20537. https://doi.org/10.2196/20537
Johnson, C. I., & Mayer, R. E. (2010). Applying the self-explanation principle to multimedia learning in a computer-based game-like environment. Computers in Human Behavior, 26 (6), 1246–1252. https://doi.org/10.1016/j.chb.2010.03.025
Jong, M. S. Y. (2015). Does online game-based learning work in formal education at school? A case study of VISOLE. The Curriculum Journal, 26 (2), 249–267. https://doi.org/10.1080/09585176.2015.1018915
Kao, G.Y.-M., Chiang, C.-H., & Sun, C.-T. (2017). Customizing scaffolds for game-based learning in physics: Impacts on knowledge acquisition and game design creativity. Computers & Education, 113 , 294–312. https://doi.org/10.1016/j.compedu.2017.05.022
Kayan-Fadlelmula, F., Sellami, A., Abdelkader, N., & Umer, S. (2022). A systematic review of STEM education research in the GCC countries: Trends, gaps and barriers. International Journal of STEM Education, 9 , 2. https://doi.org/10.1186/s40594-021-00319-7
Kazimoglu, C. (2020). Enhancing confidence in using computational thinking skills via playing a serious game: A case study to increase motivation in learning computer programming. IEEE Access, 8 , 221831–221851. https://doi.org/10.1109/access.2020.3043278
Ke, F. (2015). Designing and integrating purposeful learning in game play: A systematic review. Educational Technology Research and Development, 64 (2), 219–244. https://doi.org/10.1007/s11423-015-9418-1
Kelley, T. R., & Knowles, J. G. (2016). A conceptual framework for integrated STEM education. International Journal of STEM Education, 3 (1), 11. https://doi.org/10.1186/s40594-016-0046-z
Khamparia, A., & Pandey, B. (2018). Effects of visual map embedded approach on students learning performance using Briggs-Myers learning style in word puzzle gaming course. Computers & Electrical Engineering, 66 , 531–540. https://doi.org/10.1016/j.compeleceng.2017.12.041
Klopfer, E., & Thompson, M. (2020). Game-based learning in science, technology, engineering, and mathematics. In J. L. Plass, R. E. Mayer, & B. D. Homer (Eds.), Handbook of GAME-BASED LEARNING (p. 387). MIT Press.
Kukulska-Hulme, A., Bossu, C., Coughlan, T., Ferguson, R., FitzGerald, E., & Gaved, M. (2021). Innovating pedagogy 2021: Open university innovation report 9 . The Open University.
Kuppens, S., Laurent, L., Heyvaert, M., & Onghena, P. (2013). Associations between parental psychological control and relational aggression in children and adolescents: A multilevel and sequential meta-analysis. Developmental Psychology, 49 (9), 1697–1712. https://doi.org/10.1037/a0030740
Lameras, P., Arnab, S., Dunwell, I., Stewart, C., Clarke, S., & Petridis, P. (2017). Essential features of serious games design in higher education: Linking learning attributes to game mechanics. British Journal of Educational Technology, 48 (4), 972–994. https://doi.org/10.1111/bjet.12467
Lave, J., & Wenger, E. (1991). Situated learning: Legitimate peripheral participation . Cambridge University Press.
Li, Y., Huang, Z., Jiang, M., & Chang, T.-W. (2016). The effect on pupils’ science performance and problem-solving ability through lego: An engineering design-based modeling approach. Educational Technology & Society, 19 (3), 143–156.
Macaskill, P., Walter, S. D., & Irwig, L. (2001). A comparison of methods to detect publication bias in meta-analysis. Statistics in Medicine, 20 (4), 641–654. https://doi.org/10.1002/sim.698
Maheu-Cadotte, M.-A., Cossette, S., Dube, V., Fontaine, G., Mailhot, T., Lavoie, P., Cournoyer, A., Balli, F., & Mathieu-Dupuis, G. (2018). Effectiveness of serious games and impact of design elements on engagement and educational outcomes in healthcare professionals and students: A systematic review and meta analysis protocol. British Medical Journal Open, 8 (3), e019871. https://doi.org/10.1136/bmjopen-2017-019871
Mao, W., Cui, Y., Chiu, M. M., & Lei, H. (2022). Effects of game-based learning on students’ critical thinking: A meta-analysis. Journal of Educational Computing Research, 59 (8), 1682–1708. https://doi.org/10.1177/07356331211007098
Martín-Páez, T., Aguilera, D., Perales-Palacios, F. J., & Vílchez-González, J. M. (2019). What are we talking about when we talk about STEM education? A review of literature. Science Education, 103 (4), 799–822. https://doi.org/10.1002/sce.21522
Masek, M., Boston, J., Lam, C. P., & Corcoran, S. (2017). Improving mastery of fractions by blending video games into the math classroom. Journal of Computer Assisted Learning, 33 (5), 486–499. https://doi.org/10.1111/jcal.12194
Mayer, R. E. (2014). Computer games for learning: An evidence-based approach . MIT Press.
Mayer, R. E. (2015). On the need for research evidence to guide the design of computer games for learning. Educational Psychologist, 50 (4), 349–353. https://doi.org/10.1080/00461520.2015.1133307
Mayer, R. E. (2019). Computer games in education. Annual Review of Psychology, 70 (1), 531–549. https://doi.org/10.1146/annurev-psych-010418-102744
Mayer, R. E. (2020). Cognitive foundations of game-based learning. In J. L. Plass, R. E. Mayer, & B. D. Homer (Eds.), Handbook of game-based learning (pp. 83–110). The MIT Press.
National Research Council. (2011). Successful K-12 STEM education: Identifying effective approaches in science, technology, engineering, and mathematics . National Academies Press.
Nelson, B. C., Kim, Y., Foshee, C., & Slack, K. (2014). Visual signaling in virtual world-based assessments: The SAVE science project. Information Sciences, 264 , 32–40. https://doi.org/10.1016/j.ins.2013.09.011
Okutsu, M., DeLaurentis, D., Brophy, S., & Lambert, J. (2013). Teaching an aerospace engineering design course via virtual worlds: A comparative assessment of learning outcomes. Computers & Education, 60 (1), 288–298. https://doi.org/10.1016/j.compedu.2012.07.012
Plass, J. L., Homer, B. D., & Kinzer, C. K. (2015). Foundations of game-based learning. Educational Psychologist, 50 (4), 258–283. https://doi.org/10.1080/00461520.2015.1122533
Plass, J. L., Mayer, R. E., & Homer, B. D. (2020). Handbook of game-based learning . MIT Press.
Poppenk, J., Kohler, S., & Moscovitch, M. (2010). Revisiting the novelty effect: When familiarity, not novelty, enhances memory. Journal of Experimental Psychology: Learning, Memory, and Cognition, 36 (5), 1321–1330. https://doi.org/10.1037/a0019900
Proulx, J.-N., Romero, M., & Arnab, S. (2017). Learning mechanics and game mechanics under the perspective of self-determination theory to foster motivation in digital game based learning. Simulation & Gaming, 48 (1), 81–97. https://doi.org/10.1177/1046878116674399
R Core Team. (2021). R: A language and environment for statistical computing . R Foundation for Statistical Computing Vienna. https://www.R-project.org/ .
Raman, R., Lal, A., & Achuthan, K. (2014). Serious games based approach to cyber security concept learning: Indian context. In 2014 international conference on green computing communication and electrical engineering (ICGCCEE) (pp. 1–5), Coimbatore, India. https://doi.org/10.1109/icgccee.2014.6921392
Reed, D. A., Cook, D. A., Beckman, T. J., Levine, R. B., Kern, D. E., & Wright, S. M. (2007). Association between funding and quality of published medical education research. JAMA—Journal of the American Medical Association, 298 (9), 1002–1009. https://doi.org/10.1001/jama.298.9.1002
Renken, M. D., & Nunez, N. (2013). Computer simulations and clear observations do not guarantee conceptual understanding. Learning and Instruction, 23 , 10–23. https://doi.org/10.1016/j.learninstruc.2012.08.006
Riopel, M., Nenciovici, L., Potvin, P., Chastenay, P., Charland, P., Sarrasin, J. B., & Masson, S. (2019). Impact of serious games on science learning achievement compared with more conventional instruction: An overview and a meta-analysis. Studies in Science Education, 55 (2), 169–214. https://doi.org/10.1080/03057267.2019.1722420
Romero, M., Usart, M., & Ott, M. (2015). Can serious games contribute to developing and sustaining 21st century skills? Games and Culture, 10 (2), 148–177. https://doi.org/10.1177/1555412014548919
Roodt, S., & Ryklief, Y. (2019). Using digital game-based learning to improve the academic efficiency of vocational education students. International Journal of Game-Based Learning, 9 (4), 45–69. https://doi.org/10.4018/ijgbl.2019100104
Sadler, T. D., Romine, W. L., Menon, D., Ferdig, R. E., & Annetta, L. (2015). Learning biology through innovative curricula: A comparison of game- and nongame-based approaches. Science Education, 99 (4), 696–720. https://doi.org/10.1002/sce.21171
Sarvehana, Z. S. J. (2019). Study on effectiveness of Hana game application on cognitive problem solving skill, attention and academic achievement linguistics and mathematics on first grade student. In 2019 international serious games symposium (pp. 109–118). IEEE. https://doi.org/10.1109/ISGS49501.2019.9047023
Schrader, C., & Bastiaens, T. (2012). Learning in educational computer games for novices: The impact of support provision types on virtual presence, cognitive load, and learning outcomes. International Review of Research in Open and Distributed Learning, 13 (3), 206–227. https://doi.org/10.19173/irrodl.v13i3.1166
Simonsohn, U., Nelson, L. D., & Simmons, J. P. (2014). P-curve: A key to the file-drawer. Journal of Experimental Psychology-General, 143 (2), 534–547. https://doi.org/10.1037/a0033242
Smith, R. P., & Learman, L. A. (2017). A plea for MERSQI the medical education research study quality instrument. Obstetrics and Gynecology, 130 (4), 686–690. https://doi.org/10.1097/aog.0000000000002091
Soflano, M., Connolly, T. M., & Hainey, T. (2015). An application of adaptive games-based learning based on learning style to teach SQL. Computers & Education, 86 , 192–211. https://doi.org/10.1016/j.compedu.2015.03.015
Struyf, A., De Loof, H., Boeve-de Pauw, J., & Van Petegem, P. (2019). Students’ engagement in different STEM learning environments: Integrated STEM education as promising practice? International Journal of Science Education, 41 (10), 1387–1407. https://doi.org/10.1080/09500693.2019.1607983
Sung, H.-Y., & Hwang, G.-J. (2013). A collaborative game-based learning approach to improving students’ learning performance in science courses. Computers & Education, 63 , 43–51. https://doi.org/10.1016/j.compedu.2012.11.019
Sung, H.-Y., Hwang, G.-J., Wu, P.-H., & Lin, D.-Q. (2018). Facilitating deep-strategy behaviors and positive learning performances in science inquiry activities with a 3D experiential gaming approach. Interactive Learning Environments, 26 (8), 1053–1073. https://doi.org/10.1080/10494820.2018.1437049
Talan, T., Dogan, Y., & Batdi, V. (2020). Efficiency of digital and non-digital educational games: A comparative meta-analysis and a meta-thematic analysis. Journal of Research on Technology in Education, 52 (4), 474–514. https://doi.org/10.1080/15391523.2020.1743798
The White House. (2018). Charting a course for success: America’s strategy for STEM education . https://www.whitehouse.gov/wp-content/uploads/2018/12/STEM-Education-Strategic-Plan-2018.pdf
Tokac, U., Novak, E., & Thompson, C. G. (2019). Effects of game-based learning on students’ mathematics achievement: A meta-analysis. Journal of Computer Assisted Learning, 35 (3), 407–420. https://doi.org/10.1111/jcal.12347
Tsai, Y.-L., & Tsai, C.-C. (2018). Digital game-based second-language vocabulary learning and conditions of research designs: A meta-analysis study. Computers & Education, 125 , 345–357. https://doi.org/10.1016/j.compedu.2018.06.020
Tsai, Y.-L., & Tsai, C.-C. (2020). A meta-analysis of research on digital game-based science learning. Journal of Computer Assisted Learning, 36 (3), 280–294. https://doi.org/10.1111/jcal.12430
Vanbecelaere, S., Van den Berghe, K., Cornillie, F., Sasanguie, D., Reynvoet, B., & Depaepe, F. (2020). The effects of two digital educational games on cognitive and non-cognitive math and reading outcomes. Computers & Education, 143 , 103680. https://doi.org/10.1016/j.compedu.2019.103680
Viechtbauer, W. (2010). Conducting meta-analyses in R with the metafor package. Journal of Statistical Software, 36 (3), 1–48. https://doi.org/10.18637/jss.v036.i03
Viechtbauer, W., & Cheung, M. W. (2010). Outlier and influence diagnostics for meta-analysis. Research Synthesis Methods, 1 (2), 112–125. https://doi.org/10.1002/jrsm.11 .
Wahono, B., Lin, P.-L., & Chang, C.-Y. (2020). Evidence of STEM enactment effectiveness in Asian student learning outcomes. International Journal of STEM Education, 7 (1), 1–18. https://doi.org/10.1186/s40594-020-00236-1
Wang, L.-H., Chen, B., Hwang, G.-J., Guan, J.-Q., & Wang, Y.-Q. (2022). Effects of digital game-based STEM education on students’ learning achievement: A meta-analysis. International Journal of STEM Education, 9 (1), 1–13. https://doi.org/10.1186/s40594-022-00344-0
Wang, Y.-H. (2020). Exploring the effects of designing a role-playing game with single and peer mode for campus learning. Educational Technology Research and Development, 68 (3), 1275–1299. https://doi.org/10.1007/s11423-019-09726-8
Weng, H. J., Chang, D. F., & Shyu, H. Y. (2015). Testing the effect of mnemonic strategy embedded in digital game. ICIC Express Letters, 9 (3), 827–833.
Wilson, K. A., Bedwell, W. L., Lazzara, E. H., Salas, E., Burke, C. S., Estock, J. L., Orvis, K. L., & Conkey, C. (2009). Relationships between game attributes and learning outcomes review and research proposals. Simulation & Gaming, 40 (2), 217–266. https://doi.org/10.1177/1046878108321866
Wouters, P., van der Spek, E. D., & van Oostendorp, H. (2009). Current practices in serious game research: a review from a learning outcomes perspective. Games-based learning advancements for multi-sensory human computer interfaces (pp. 232–250). IGI Publishing. https://doi.org/10.4018/978-1-60566-360-9.ch014
Chapter Google Scholar
Wouters, P., van Nimwegen, C., van Oostendorp, H., & van der Spek, E. (2013). A meta-analysis of the cognitive and motivational effects of serious games. Journal of Educational Psychology, 105 (2), 249–265. https://doi.org/10.1037/a0031311
Wouters, P., & van Oostendorp, H. (2013). A meta-analytic review of the role of instructional support in game-based learning. Computers & Education, 60 (1), 412–425. https://doi.org/10.1016/j.compedu.2012.07.018
Wu, Y.-T., & Anderson, O. R. (2015). Technology-enhanced stem (science, technology, engineering, and mathematics) education. Journal of Computers in Education, 2 (3), 245–249. https://doi.org/10.1007/s40692-015-0041-2
Yu, J., Denham, A. R., & Searight, E. (2022). A systematic review of augmented reality game-based learning in STEM education. Educational Technology Research and Development, 70 (4), 1169–1194. https://doi.org/10.1007/s11423-022-10122-y
Ziegler, A., Antes, G., & Koenig, I. R. (2011). Preferred report items for systematic reviews and meta-analysis: The PRISMA statement. Deutsche Medizinische Wochenschrift, 136 (8), E9–E15. https://doi.org/10.1055/s-0031-1272978
Download references
Acknowledgements
Authors would like to thank the reviewers and editors, whose comments and feedback helped us to improve the original manuscript.
This research was supported by a grant from the National Natural Science Foundation of China (No. 62107018) to Zhihui Cai. This research was also supported by Grant CCNU22QN020 and CCNU22QN013 from the Fundamental Research Funds for the Central Universities.
Author information
Authors and affiliations.
Key Laboratory of Adolescent Cyberpsychology and Behavior (CCNU), Ministry of Education, Wuhan, 430079, China
Yang Gui, Zhihui Cai, Yajiao Yang & Lingyuan Kong
School of Psychology, Central China Normal University, Wuhan, Hubei, China
Faculty of Education, The Chinese University of Hong Kong, Hong Kong, China
School of Education and Human Development, University of Virginia, Charlottesville, USA
Robert H. Tai
You can also search for this author in PubMed Google Scholar
Contributions
ZC led the study design, helped statistical analysis, led the interpretation of results, and drafted the manuscript. YG reviewed studies, extracted the data, performed the statistical analyses and draft the manuscript. YY and LK reviewed studies and extracted the data. XF participated in interpretation of results and helped to draft the manuscript. RHT contributed to manuscript revision. ZC, YG and XF read and approved the final manuscript.
Corresponding author
Correspondence to Zhihui Cai .
Ethics declarations
Competing interests.
The authors declare that they have no competing interests.
Additional information
Publisher's note.
Springer Nature remains neutral with regard to jurisdictional claims in published maps and institutional affiliations.
Supplementary Information
Additional file 1:.
Detailed results for Influential effect sizes detection and publication bias test.
Rights and permissions
Open Access This article is licensed under a Creative Commons Attribution 4.0 International License, which permits use, sharing, adaptation, distribution and reproduction in any medium or format, as long as you give appropriate credit to the original author(s) and the source, provide a link to the Creative Commons licence, and indicate if changes were made. The images or other third party material in this article are included in the article's Creative Commons licence, unless indicated otherwise in a credit line to the material. If material is not included in the article's Creative Commons licence and your intended use is not permitted by statutory regulation or exceeds the permitted use, you will need to obtain permission directly from the copyright holder. To view a copy of this licence, visit http://creativecommons.org/licenses/by/4.0/ .
Reprints and permissions
About this article
Cite this article.
Gui, Y., Cai, Z., Yang, Y. et al. Effectiveness of digital educational game and game design in STEM learning: a meta-analytic review. IJ STEM Ed 10 , 36 (2023). https://doi.org/10.1186/s40594-023-00424-9
Download citation
Received : 17 May 2022
Accepted : 01 May 2023
Published : 19 May 2023
DOI : https://doi.org/10.1186/s40594-023-00424-9
Share this article
Anyone you share the following link with will be able to read this content:
Sorry, a shareable link is not currently available for this article.
Provided by the Springer Nature SharedIt content-sharing initiative
- Digital game-based learning
- Game design
- STEM education
- Academic achievement
- Meta-analysis
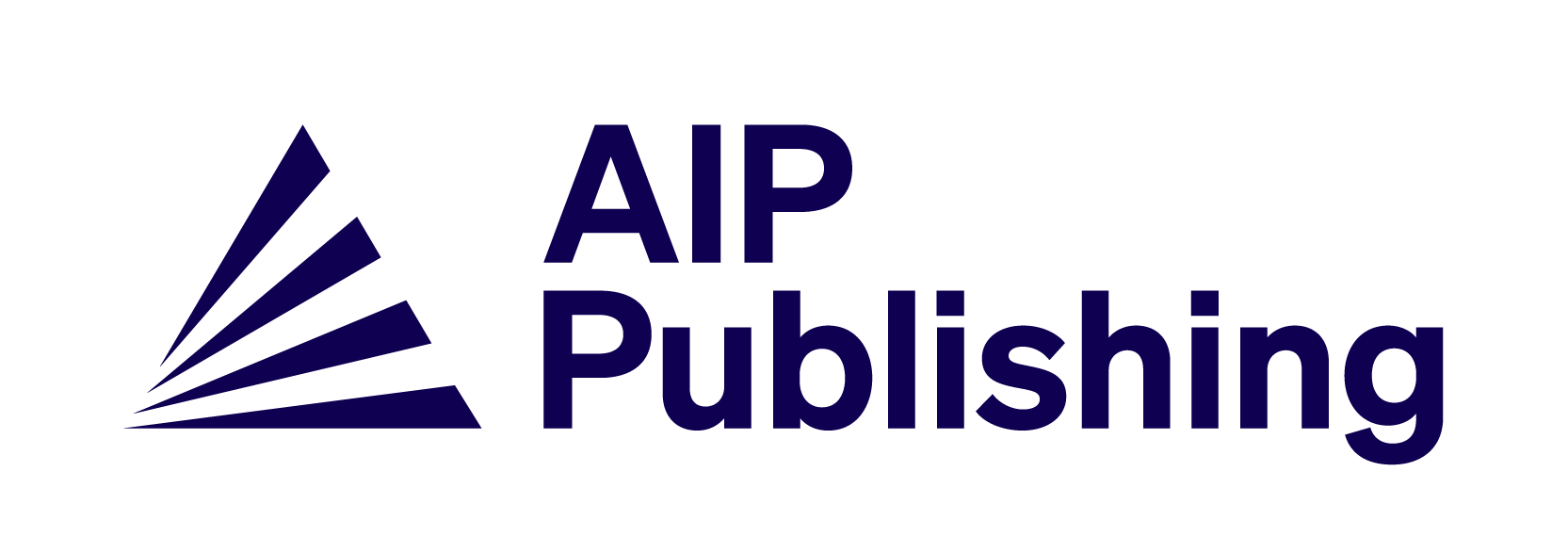
The role of game-based learning in developing students computational thinking skills: A review of the literature
- Article contents
- Figures & tables
- Supplementary Data
- Peer Review
- Reprints and Permissions
- Cite Icon Cite
- Search Site
Dimas Galang Ramadhan , Cucuk Wawan Budiyanto , Rosihan Ari Yuana; The role of game-based learning in developing students computational thinking skills: A review of the literature. AIP Conf. Proc. 27 January 2023; 2540 (1): 110019. https://doi.org/10.1063/5.0105705
Download citation file:
- Ris (Zotero)
- Reference Manager
In the 21st century, computational thinking skills are essential for solving problems around computer science and solving problems in everyday life. This belief has been recognized globally, and more and more education systems integrated computational thinking into their compulsory education in recent years. Game-based learning (GBL) is one of the pedagogical frameworks used by educational units to develop CT skills. This study was conducted by reviewing the literature from 20 studies that provide empirical evidence of the use of game-based learning to develop computational thinking. This study used a literature review method by adapting Brennan and Resnick’s framework to identify CT abilities expected to develop in game-based learning. The main finding in this study identified CT skills that can be obtained from the use of game-based learning based on the framework mentioned above. Additional CT skills not listed or explicitly stated in the framework were also included. Furthermore, this study also discusses the development of student learning motivation during learning activities using game-based learning.
Sign in via your Institution
Citing articles via, publish with us - request a quote.
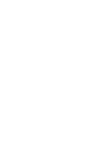
Sign up for alerts
- Online ISSN 1551-7616
- Print ISSN 0094-243X
- For Researchers
- For Librarians
- For Advertisers
- Our Publishing Partners
- Physics Today
- Conference Proceedings
- Special Topics
pubs.aip.org
- Privacy Policy
- Terms of Use
Connect with AIP Publishing
This feature is available to subscribers only.
Sign In or Create an Account
Flow experience in game based learning – a systematic literature review
- Arttu Perttula Tampere University of Technology
- Kristian Kiili Tampere University of Technology
- Antero Lindstedt Tampere University of Technology
- Pauliina Tuomi Tampere University of Technology
The entertaining elements implemented in a serious game are key factors in determining whether a player will be engaged in a play-learn process and able to achieve the desired learning outcomes. Thus, optimization of subjective playing experience is a crucial part of a game design process. Flow theory can be adopted for measuring user experience and analyzing the quality of serious game designs. In addition, flow seems to have a positive influence on performance enhancement, learning and engagement. The focus of this review is especially on examining the meaning of flow in the context of serious games as well as exploring the relationship between flow and learning, factors that influence occurrence of flow and how flow is operationalized. The review revealed that there are mainly conceptual considerations about flow in serious games, but no robust empirical evidence about the meaning of flow. This is in line with other studies. We argue that research on flow should focus on the specific aspects related to the very nature of serious games that combine enjoyment and learning. Furthermore, new methods to measure flow and analyse the data need to be developed and studied.
IJSG copyright information is provided here .
How to Cite
- Endnote/Zotero/Mendeley (RIS)
Most read articles by the same author(s)
- Kristian Juha Mikael Kiili, Keith Devlin, Arttu Perttula, Pauliina Tuomi, Antero Lindstedt, Using video games to combine learning and assessment in mathematics education , International Journal of Serious Games: Vol. 2 No. 4 (2015)
- Jannicke Madeleine Baalsrud Hauge, Ioana A. Stanescu, Sylvester Arnab, Pablo Moreno Ger, Theodore Lim, Angel Serrano-Laguna, Petros Lameras, Maurice Hendrix, Kristian Kiili, Manuel Ninaus, Sara de Freitas, Alessandro Mazzetti, Anders Dahlbom, Cristiana Degano, Learning Analytics Architecture to Scaffold Learning Experience through Technology-based Methods , International Journal of Serious Games: Vol. 2 No. 1 (2015)
- Manuel Ninaus, Korbinian Moeller, Jake McMullen, Kristian Kiili, Acceptance of Game-Based Learning and Intrinsic Motivation as Predictors for Learning Success and Flow Experience , International Journal of Serious Games: Vol. 4 No. 3 (2017)
- Kristian Kiili, Harri Ketamo, Michael D. Kickmeier-Rust, Evaluating the usefulness of Eye Tracking in Game-based Learning , International Journal of Serious Games: Vol. 1 No. 2 (2014)
- Kristian Juha Mikael Kiili, Antero Lindstedt, Antti Koskinen, Hilma Halme, Manuel Ninaus, Jake McMullen, Flow Experience and Situational Interest in Game-Based Learning: Cousins or Identical Twins , International Journal of Serious Games: Vol. 8 No. 3 (2021)
- Kristian J. M. Kiili, Arttu Perttula, Antero Lindstedt, Sylester Arnab, Marko Suominen, Flow Experience as a Quality Measure in Evaluating Physically Activating Collaborative Serious Games , International Journal of Serious Games: Vol 1, No 3 (2014)
- Kristian Kiili, Siuko Juho, Elizabeth Cloude, Muhterem Dindar, Demystifying the Relations of Motivation and Emotions in Game-Based Learning , International Journal of Serious Games: Vol. 10 No. 4 (2023)
- Simon Greipl, Korbinian Moeller, Kristian Kiili, Manuel Ninaus, Different performance, full experience: a learning game applied throughout adulthood , International Journal of Serious Games: Vol. 7 No. 3 (2020)
- Antti Koskinen, Kristian Kiili, Francesca De Rosa, Muhterem Dindar, Michael Kickmeier-Rust, Introduction to the Special Issue on GaLA Conf 2022 , International Journal of Serious Games: Vol. 10 No. 4 (2023)
Information
- For Readers
- For Authors
- For Librarians
Developed By
- Open access
- Published: 09 May 2024
Machine learning models for abstract screening task - A systematic literature review application for health economics and outcome research
- Jingcheng Du 1 ,
- Ekin Soysal 1 , 3 ,
- Dong Wang 2 ,
- Long He 1 ,
- Bin Lin 1 ,
- Jingqi Wang 1 ,
- Frank J. Manion 1 ,
- Yeran Li 2 ,
- Elise Wu 2 &
- Lixia Yao 2
BMC Medical Research Methodology volume 24 , Article number: 108 ( 2024 ) Cite this article
1 Altmetric
Metrics details
Systematic literature reviews (SLRs) are critical for life-science research. However, the manual selection and retrieval of relevant publications can be a time-consuming process. This study aims to (1) develop two disease-specific annotated corpora, one for human papillomavirus (HPV) associated diseases and the other for pneumococcal-associated pediatric diseases (PAPD), and (2) optimize machine- and deep-learning models to facilitate automation of the SLR abstract screening.
This study constructed two disease-specific SLR screening corpora for HPV and PAPD, which contained citation metadata and corresponding abstracts. Performance was evaluated using precision, recall, accuracy, and F1-score of multiple combinations of machine- and deep-learning algorithms and features such as keywords and MeSH terms.
Results and conclusions
The HPV corpus contained 1697 entries, with 538 relevant and 1159 irrelevant articles. The PAPD corpus included 2865 entries, with 711 relevant and 2154 irrelevant articles. Adding additional features beyond title and abstract improved the performance (measured in Accuracy) of machine learning models by 3% for HPV corpus and 2% for PAPD corpus. Transformer-based deep learning models that consistently outperformed conventional machine learning algorithms, highlighting the strength of domain-specific pre-trained language models for SLR abstract screening. This study provides a foundation for the development of more intelligent SLR systems.
Peer Review reports
Introduction
Systematic literature reviews (SLRs) are an essential tool in many areas of health sciences, enabling researchers to understand the current knowledge around a topic and identify future research and development directions. In the field of health economics and outcomes research (HEOR), SLRs play a crucial role in synthesizing evidence around unmet medical needs, comparing treatment options, and preparing the design and execution of future real-world evidence studies. SLRs provide a comprehensive and transparent analysis of available evidence, allowing researchers to make informed decisions and improve patient outcomes.
Conducting a SLR involves synthesizing high-quality evidence from biomedical literature in a transparent and reproducible manner, and seeks to include all available evidence on a given research question, and provides some assessment regarding quality of the evidence [ 1 , 2 ]. To conduct an SLR one or more bibliographic databases are queried based on a given research question and a corresponding set of inclusion and exclusion criteria, resulting in the selection of a relevant set of abstracts. The abstracts are reviewed, further refining the set of articles that are used to address the research question. Finally, appropriate data is systematically extracted from the articles and summarized [ 1 , 3 ].
The current approach to conducting a SLR is through manual review, with data collection, and summary done by domain experts against pre-specified eligibility criteria. This is time-consuming, labor-intensive, expensive, and non-scalable given the current more-than linear growth of the biomedical literature [ 4 ]. Michelson and Reuter estimate that each SLR costs approximately $141,194.80 and that on average major pharmaceutical companies conduct 23.36 SLRs, and major academic centers 177.32 SLRs per year, though the cost may vary based on the scope of different reviews [ 4 ]. Clearly automated methods are needed, both from a cost/time savings perspective, and for the ability to effectively scan and identify increasing amounts of literature, thereby allowing the domain experts to spend more time analyzing the data and gleaning the insights.
One major task of SLR project that involves large amounts of manual effort, is the abstract screening task. For this task, selection criteria are developed and the citation metadata and abstract for articles tentatively meeting these criteria are retrieved from one or more bibliographic databases (e.g., PubMed). The abstracts are then examined in more detail to determine if they are relevant to the research question(s) and should be included or excluded from further consideration. Consequently, the task of determining whether articles are relevant or not based on their titles, abstracts and metadata can be treated as a binary classification task, which can be addressed by natural language processing (NLP). NLP involves recognizing entities and relationships expressed in text and leverages machine-learning (ML) and deep-learning (DL) algorithms together with computational semantics to extract information. The past decade has witnessed significant advances in these areas for biomedical literature mining. A comprehensive review on how NLP techniques in particular are being applied for automatic mining and knowledge extraction from biomedical literature can be found in Zhao et al. [ 5 ].
Materials and methods
The aims of this study were to: (1) identify and develop two disease-specific corpora, one for human papillomavirus (HPV) associated diseases and the other for pneumococcal-associated pediatric diseases suitable for training the ML and DL models underlying the necessary NLP functions; (2) investigate and optimize the performance of the ML and DL models using different sets of features (e.g., keywords, Medical Subject Heading (MeSH) terms [ 6 ]) to facilitate automation of the abstract screening tasks necessary to construct a SLR. Note that these screening corpora can be used as training data to build different NLP models. We intend to freely share these two corpora with the entire scientific community so they can serve as benchmark corpora for future NLP model development in this area.
SLR corpora preparation
Two completed disease-specific SLR studies by Merck & Co., Inc., Rahway, NJ, USA were used as the basis to construct corpora for abstract-level screening. The two SLR studies were both relevant to health economics and outcome research, including one for human papillomavirus (HPV) associated diseases (referred to as the HPV corpus), and one for pneumococcal-associated pediatric diseases (which we refer to as the PAPD corpus). Both of the original SLR studies contained literature from PubMed/MEDLINE and EMBASE. Since we intended for the screening corpora to be released to the community, we only kept citations found from PubMed/MEDLINE in the finalized corpora. Because the original SLR studies did not contain the PubMed ID (PMID) for each article, we matched each article’s citation information (if available) against PubMed and then collected meta-data such as authors, journals, keywords, MeSH terms, publication types, etc., using PubMed Entrez Programming Utilities (E-utilities) Application Programming Interface (API). The detailed description of the two corpora can be seen in Table 1 . Both of the resulting corpora are publicly available at [ https://github.com/Merck/NLP-SLR-corpora ].
Machine learning algorithms
Although deep learning algorithms have demonstrated superior performance on many NLP tasks, conventional machine learning algorithms have certain advantages, such as low computation costs and faster training and prediction speed.
We evaluated four traditional ML-based document classification algorithms, XGBoost [ 7 ], Support Vector Machines (SVM) [ 8 ], Logistic regression (LR) [ 9 ], and Random Forest [ 10 ] on the binary inclusion/exclusion classification task for abstract screening. Salient characteristics of these models are as follows:
XGBoost: Short for “eXtreme Gradient Boosting”, XGBoost is a boosting-based ensemble of algorithms that turn weak learners into strong learners by focusing on where the individual models went wrong. In Gradient Boosting, individual weak models train upon the difference between the prediction and the actual results [ 7 ]. We set max_depth at 3, n_estimators at 150 and learning rate at 0.7.
Support vector machine (SVM): SVM is one of the most robust prediction methods based on statistical learning frameworks. It aims to find a hyperplane in an N-dimensional space (where N = the number of features) that distinctly classifies the data points [ 8 ]. We set C at 100, gamma at 0.005 and kernel as radial basis function.
Logistic regression (LR): LR is a classic statistical model that in its basic form uses a logistic function to model a binary dependent variable [ 9 ]. We set C at 5 and penalty as l2.
Random forest (RF): RF is a machine learning technique that utilizes ensemble learning to combine many decision trees classifiers through bagging or bootstrap aggregating [ 10 ]. We set n_estimators at 100 and max_depth at 14.
These four algorithms were trained for both the HPV screening task and the PAPD screening task using the corresponding training corpus.
For each of the four algorithms, we examined performance using (1) only the baseline feature criteria (title and abstract of each article), and (2) with five additional meta-data features (MeSH, Authors, Keywords, Journal, Publication types.) retrieved from each article using the PubMed E-utilities API. Conventionally, title and abstract are the first information a human reviewer would depend on when making a judgment for inclusion or exclusion of an article. Consequently, we used title and abstract as the baseline features to classify whether an abstract should be included at the abstract screening stage. We further evaluated the performance with additional features that can be retrieved by PubMed E-utilities API, including MeSH terms, authors, journal, keywords and publication type. For baseline evaluation, we concatenated the titles and abstracts and extracted the TF-IDF (term frequency-inverse document frequency) vector for the corpus. TF-IDF evaluates how relevant a word is to a document in a collection of documents. For additional features, we extracted TF-IDF vector using each feature respectively and then concatenated the extracted vectors with title and abstract vector. XGBoost was selected for the feature evaluation process, due to its relatively quick computational running time and robust performance.
Deep learning algorithms
Conventional ML methods rely heavily on manually designed features and suffer from the challenges of data sparsity and poor transportability when applied to new use cases. Deep learning (DL) is a set of machine learning algorithms based on deep neural networks that has advanced performance of text classification along with many other NLP tasks. Transformer-based deep learning models, such as BERT (Bidirectional encoder representations from transformers), have achieved state-of-the-art performance in many NLP tasks [ 11 ]. A Transformer is an emerging architecture of deep learning models designed to handle sequential input data such as natural language by adopting the mechanisms of attention to differentially weigh the significance of each part of the input data [ 12 ]. The BERT model and its variants (which use Transformer as a basic unit) leverage the power of transfer learning by first pre-training the models over 100’s of millions of parameters using large volumes of unlabeled textual data. The resulting model is then fine-tuned for a particular downstream NLP application, such as text classification, named entity recognition, relation extraction, etc. The following three BERT models were evaluated against both the HPV and Pediatric pneumococcal corpus using two sets of features (title and abstract versus adding all additional features into the text). For all BERT models, we used Adam optimizer with weight decay. We set learning rate at 1e-5, batch size at 8 and number of epochs at 20.
BERT base: this is the original BERT model released by Google. The BERT base model was pre-trained on textual data in the general domain, i.e., BooksCorpus (800 M words) and English Wikipedia (2500 M words) [ 11 ].
BioBERT base: as the biomedical language is different from general language, the BERT models trained on general textual data may not work well on biomedical NLP tasks. BioBERT was further pre-trained (based on original BERT models) in the large-scale biomedical corpora, including PubMed abstracts (4.5B words) and PubMed Central Full-text articles (13.5B words) [ 13 ].
PubMedBERT: PubMedBERT was pre-trained from scratch using abstracts from PubMed. This model has achieved state-of-the-art performance on several biomedical NLP tasks on Biomedical Language Understanding and Reasoning Benchmark [ 14 ].
Text pre-processing and libraries that were used
We have removed special characters and common English words as a part of text pre-processing. Default tokenizer from scikit-learn was adopted for tokenization. Scikit-learn was also used for TF-IDF feature extraction and machine learning algorithms implementation. Transformers libraries from Hugging Face were used for deep learning algorithms implementation.
Evaluation datasets were constructed from the HPV and Pediatric pneumococcal corpora and were split into training, validation and testing sets with a ratio of 8:1:1 for the two evaluation tasks: (1) ML algorithms performance assessment; and (2) DL algorithms performance assessment. Models were fitted on the training sets, and model hyperparameters were optimized on the validation sets and the performance were evaluated on the testing sets. The following major metrics are expressed by the noted calculations:
Where True positive is an outcome where the model correctly predicts the positive (e.g., “included” in our tasks) class. Similarly, a True negative is an outcome where the model correctly predicts the negative class (e.g., “excluded” in our tasks). False positive is an outcome where the model incorrectly predicts the positive class, and a False negative is an outcome where the model incorrectly predicts the negative class. We have repeated all experiments five times and reported the mean scores with standard deviation.
Table 2 shows the baseline comparison using different feature combinations for the SLR text classification tasks using XGBoost. As noted, adding additional features in addition to title and abstract was effective in further improving the classification accuracy. Specifically, using all available features for the HPV classification increased accuracy by ? ∼ 3% and F1 score by ? ∼ 3%; using all available features for Pediatric pneumococcal classification increased accuracy by ? ∼ 2% and F1 score by ? ∼ 4%. As observed, adding additional features provided a stronger boost in precision, which contributed to the overall performance improvement.
The comparison of the article inclusion/exclusion classification task for four machine learning algorithms with all features is shown in Table 3 . XGBoost achieved the highest accuracy and F-1 scores in both tasks. Table 4 shows the comparison between XGBoost and deep learning algorithms on the classification tasks for each disease. Both XGBoost and deep learning models consistently have achieved higher accuracy scores when using all features as input. Among all models, BioBERT has achieved the highest accuracy at 0.88, compared with XGBoost at 0.86. XGBoost has the highest F1 score at 0.8 and the highest recall score at 0.9 for inclusion prediction.
Discussions and conclusions
Abstract screening is a crucial step in conducting a systematic literature review (SLR), as it helps to identify relevant citations and reduces the effort required for full-text screening and data element extraction. However, screening thousands of abstracts can be a time-consuming and burdensome task for scientific reviewers. In this study, we systematically investigated the use of various machine learning and deep learning algorithms, using different sets of features, to automate abstract screening tasks. We evaluated these algorithms using disease-focused SLR corpora, including one for human papillomavirus (HPV) associated diseases and another for pneumococcal-associated pediatric diseases (PADA). The publicly available corpora used in this study can be used by the scientific community for advanced algorithm development and evaluation. Our findings suggest that machine learning and deep learning algorithms can effectively automate abstract screening tasks, saving valuable time and effort in the SLR process.
Although machine learning and deep learning algorithms trained on the two SLR corpora showed some variations in performance, there were also some consistencies. Firstly, adding additional citation features significantly improved the performance of conventional machine learning algorithms, although the improvement was not as strong in transformer-based deep learning models. This may be because transformer models were mostly pre-trained on abstracts, which do not include additional citation information like MeSH terms, keywords, and journal names. Secondly, when using only title and abstract as input, transformer models consistently outperformed conventional machine learning algorithms, highlighting the strength of subject domain-specific pre-trained language models. When all citation features were combined as input, conventional machine learning algorithms showed comparable performance to deep learning models. Given the much lower computation costs and faster training and prediction time, XGBoost or support vector machines with all citation features could be an excellent choice for developing an abstract screening system.
Some limitations remain for this study. Although we’ve evaluated cutting-edge machine learning and deep learning algorithms on two SLR corpora, we did not conduct much task-specific customization to the learning algorithms, including task-specific feature engineering and rule-based post-processing, which could offer additional benefits to the performance. As the focus of this study is to provide generalizable strategies for employing machine learning to abstract screening tasks, we leave the task-specific customization to future improvement. The corpora we evaluated in this study mainly focus on health economics and outcome research, the generalizability of learning algorithms to another domain will benefit from formal examination.
Extensive studies have shown the superiority of transformer-based deep learning models for many NLP tasks [ 11 , 13 , 14 , 15 , 16 ]. Based on our experiments, however, adding features to the pre-trained language models that have not seen these features before may not significantly boost their performance. It would be interesting to find a better way of encoding additional features to these pre-trained language models to maximize their performance. In addition, transfer learning has proven to be an effective technique to improve the performance on a target task by leveraging annotation data from a source task [ 17 , 18 , 19 ]. Thus, for a new SLR abstract screening task, it would be worthwhile to investigate the use of transfer learning by adapting our (publicly available) corpora to the new target task.
When labeled data is available, supervised machine learning algorithms can be very effective and efficient for article screening. However, as there is increasing need for explainability and transparency in NLP-assisted SLR workflow, supervised machine learning algorithms are facing challenges in explaining why certain papers fail to fulfill the criteria. The recent advances in large language models (LLMs), such as ChatGPT [ 20 ] and Gemini [ 21 ], show remarkable performance on NLP tasks and good potentials in explainablity. Although there are some concerns on the bias and hallucinations that LLMs could bring, it would be worthwhile to evaluate further how LLMs could be applied to SLR tasks and understand the performance of using LLMs to take free-text article screening criteria as the input and provide explainanation for article screening decisions.
Data availability
The annotated corpora underlying this article are available at https://github.com/Merck/NLP-SLR-corpora .
Bullers K, Howard AM, Hanson A, et al. It takes longer than you think: librarian time spent on systematic review tasks. J Med Libr Assoc. 2018;106:198–207. https://doi.org/10.5195/jmla.2018.323 .
Article PubMed PubMed Central Google Scholar
Carver JC, Hassler E, Hernandes E et al. Identifying Barriers to the Systematic Literature Review Process. In: 2013 ACM / IEEE International Symposium on Empirical Software Engineering and Measurement . 2013. 203–12. https://doi.org/10.1109/ESEM.2013.28 .
Lame G. Systematic literature reviews: an introduction. Proc Des Society: Int Conf Eng Des. 2019;1:1633–42. https://doi.org/10.1017/dsi.2019.169 .
Article Google Scholar
Michelson M, Reuter K. The significant cost of systematic reviews and meta-analyses: a call for greater involvement of machine learning to assess the promise of clinical trials. Contemp Clin Trials Commun. 2019;16:100443. https://doi.org/10.1016/j.conctc.2019.100443 .
Recent advances in. biomedical literature mining | Briefings in Bioinformatics | Oxford Academic. https://academic.oup.com/bib/article/22/3/bbaa057/5838460?login=true (accessed 30 May 2022).
Medical Subject Headings - Home Page. https://www.nlm.nih.gov/mesh/meshhome.html (accessed 30 May 2022).
Chen T, Guestrin C, XGBoost:. A Scalable Tree Boosting System. In: Proceedings of the 22nd ACM SIGKDD International Conference on Knowledge Discovery and Data Mining . New York, NY, USA: Association for Computing Machinery 2016. 785–94. https://doi.org/10.1145/2939672.2939785 .
Noble WS. What is a support vector machine? Nat Biotechnol. 2006;24:1565–7. https://doi.org/10.1038/nbt1206-1565 .
Article CAS PubMed Google Scholar
Logistic Regression . https://doi.org/10.1007/978-1-4419-1742-3 (accessed 30 May 2022).
Random forest classifier for remote sensing classification. International Journal of Remote Sensing: Vol 26, No 1. https://www.tandfonline.com/doi/abs/10.1080/01431160412331269698 (accessed 30 May 2022).
Devlin J, Chang M-W, Lee K, et al. BERT: pre-training of Deep Bidirectional transformers for Language understanding. arXiv. 2019. https://doi.org/10.48550/arXiv.1810.04805 .
Vaswani A, Shazeer N, Parmar N et al. Attention is All you Need. In: Advances in Neural Information Processing Systems . Curran Associates, Inc. 2017. https://proceedings.neurips.cc/paper/2017/hash/3f5ee243547dee91fbd053c1c4a845aa-Abstract.html (accessed 30 May 2022).
BioBERT. a pre-trained biomedical language representation model for biomedical text mining | Bioinformatics | Oxford Academic. https://academic.oup.com/bioinformatics/article/36/4/1234/5566506 (accessed 3 Jun 2020).
Gu Y, Tinn R, Cheng H, et al. Domain-specific Language Model Pretraining for Biomedical Natural Language Processing. ACM Trans Comput Healthc. 2021;3(2):1–2. https://doi.org/10.1145/3458754 .
Article CAS Google Scholar
Chen Q, Du J, Allot A, et al. LitMC-BERT: transformer-based multi-label classification of biomedical literature with an application on COVID-19 literature curation. arXiv. 2022. https://doi.org/10.48550/arXiv.2204.08649 .
Chen Q, Allot A, Leaman R, et al. Multi-label classification for biomedical literature: an overview of the BioCreative VII LitCovid Track for COVID-19 literature topic annotations. arXiv. 2022. https://doi.org/10.48550/arXiv.2204.09781 .
Kermany DS, Goldbaum M, Cai W, et al. Identifying Medical diagnoses and Treatable diseases by Image-based deep learning. Cell. 2018;172:1122–e11319. https://doi.org/10.1016/j.cell.2018.02.010 .
Howard J, Ruder S. Universal Language Model fine-tuning for text classification. arXiv. 2018. https://doi.org/10.48550/arXiv.1801.06146 .
Do CB, Ng AY. Transfer learning for text classification. In: Advances in Neural Information Processing Systems . MIT Press. 2005. https://proceedings.neurips.cc/paper/2005/hash/bf2fb7d1825a1df3ca308ad0bf48591e-Abstract.html (accessed 30 May 2022).
Achiam J et al. Gpt-4 technical report. arXiv preprint arXiv:2303.08774 (2023).
https:// gemini.google.com/app/a4dcd2e2d7672354 . (accessed 01 Feb 2024).
Download references
Acknowledgements
We thank Dr. Majid Rastegar-Mojarad for conducting some additional experiments during revision.
This research was supported by Merck Sharp & Dohme LLC, a subsidiary of Merck & Co., Inc., Rahway, NJ, USA.
Author information
Authors and affiliations.
Intelligent Medical Objects, Houston, TX, USA
Jingcheng Du, Ekin Soysal, Long He, Bin Lin, Jingqi Wang & Frank J. Manion
Merck & Co., Inc, Rahway, NJ, USA
Dong Wang, Yeran Li, Elise Wu & Lixia Yao
McWilliams School of Biomedical Informatics, University of Texas Health Science Center at Houston, Houston, TX, USA
Ekin Soysal
You can also search for this author in PubMed Google Scholar
Contributions
Study concept and design: JD and LY Corpus preparation: DW, YL and LY Experiments: JD and ES Draft of the manuscript: JD, DW, FJM and LY Acquisition, analysis, or interpretation of data: JD, ES, DW and LY Critical revision of the manuscript for important intellectual content: JD, ES, DW, LH, BL, JW, FJM, YL, EW, LY Study supervision: LY.
Corresponding author
Correspondence to Lixia Yao .
Ethics declarations
Disclaimers.
The content is the sole responsibility of the authors and does not necessarily represent the official views of Merck & Co., Inc., Rahway, NJ, USA or Intelligent Medical Objects.
Ethics approval and consent to participate
Not applicable.
Consent for publication
Competing interests.
DW is an employee of Merck Sharp & Dohme LLC, a subsidiary of Merck & Co., Inc., Rahway, NJ, USA. EW, YL, and LY were employees of Merck Sharp & Dohme LLC, a subsidiary of Merck & Co., Inc., Rahway, NJ, USA for this work. JD, LH, JW, and FJM are employees of Intelligent Medical Objects. ES was an employee of Intelligent Medical Objects during his contributions, and is currently an employee of EBSCO Information Services. All the other authors declare no competing interest.
Additional information
Publisher’s note.
Springer Nature remains neutral with regard to jurisdictional claims in published maps and institutional affiliations.
Rights and permissions
Open Access This article is licensed under a Creative Commons Attribution 4.0 International License, which permits use, sharing, adaptation, distribution and reproduction in any medium or format, as long as you give appropriate credit to the original author(s) and the source, provide a link to the Creative Commons licence, and indicate if changes were made. The images or other third party material in this article are included in the article’s Creative Commons licence, unless indicated otherwise in a credit line to the material. If material is not included in the article’s Creative Commons licence and your intended use is not permitted by statutory regulation or exceeds the permitted use, you will need to obtain permission directly from the copyright holder. To view a copy of this licence, visit http://creativecommons.org/licenses/by/4.0/ . The Creative Commons Public Domain Dedication waiver ( http://creativecommons.org/publicdomain/zero/1.0/ ) applies to the data made available in this article, unless otherwise stated in a credit line to the data.
Reprints and permissions
About this article
Cite this article.
Du, J., Soysal, E., Wang, D. et al. Machine learning models for abstract screening task - A systematic literature review application for health economics and outcome research. BMC Med Res Methodol 24 , 108 (2024). https://doi.org/10.1186/s12874-024-02224-3
Download citation
Received : 19 May 2023
Accepted : 18 April 2024
Published : 09 May 2024
DOI : https://doi.org/10.1186/s12874-024-02224-3
Share this article
Anyone you share the following link with will be able to read this content:
Sorry, a shareable link is not currently available for this article.
Provided by the Springer Nature SharedIt content-sharing initiative
- Machine learning
- Deep learning
- Text classification
- Article screening
- Systematic literature review
BMC Medical Research Methodology
ISSN: 1471-2288
- General enquiries: [email protected]

IMAGES
VIDEO
COMMENTS
The present study sought to elicit insights into pedagogical practices pertaining to the integration of digital games into teaching and learning. A review of peer-reviewed journal articles published in English over the past 10 years uncovered common pedagogical themes that were categorized into a pre-game, game, and post-game taxonomy.
A Literature Review of Game-based Learning. About the Author. Mingfong Jan is the Guest Editor of this issue. He is a Research Scientist at the Learning Sciences Lab at NIE and is interested in how new media (e.g., Flickr, mobile phones and digital cameras) restructure thinking, values, actions, community and culture.
Given the lack of consistent empirical evidence with respect to the effectiveness of game-based learning, this review aims to examine the most recent literature regarding game-based learning and seeks to further understand the influence of games on learning, with a major focus on students' 21st century skill development. 2. Literature review2.1.
A large systematic literature review of computer games at primary education. ... Games-based Learning (GBL) has developed a reputation with educationalists it is perceived as a potentially engaging form of supplementary learning that could enhance the educational process and has been used at all levels of education including primary, secondary ...
The research question for the literature review was "How has game-based learning been used in the nursing curriculum and what has been its impact on student experience and learning?" In order to identify relevant keywords, the research question was unpicked using the PICO framework (Aslam and Emmanuel, 2010). PICO was defined as the ...
Using games in education has the potential to increase students' motivation and engagement in the learning process, gathering long-lasting practical knowledge. Expanding interest in implementing a game-based approach in computer science education highlights the need for a comprehensive overview of the literature research. This scoping review aims to provide insight into current trends and ...
ABSTRACT. In recent years, Game-Based Learning (GBL) has been widely adopted in various educational settings. This paper aims to review empirical studies that adopt GBL in the field of AI education and explore its future research perspectives.
The present study sought to elicit insights into pedagogical practices pertaining to the integration of digital games into teaching and learning. A review of peer-reviewed journal articles published in English over the past 10 years uncovered common pedagogical themes that were categorized into a pre-game, game, and post-game taxonomy. The findings indicated that teachers implemented a variety ...
Game-based learning, both in the form of serious games and gamification, has developed rapidly and penetrated various fields of science and it is believed by some that it can increase the human ability to learn various things, increase the effectiveness and efficiency of a job, collaboration, and positive attitudes. To be able to choose the right game as needed, a summary of knowledge about ...
The use of educational games or some aspects of games in the educational context is known as game-based learning (GBL) or educational gamification. The objectives of this study are to assess the existing evidence about how GBL and gamification have affected the variables studied in each investigation over the past ten years; to analyse the educational levels, variables, dynamics, and types of ...
Gamification and game-based learning are becoming more prominent in educational settings for several reasons. When it comes to learning, being focused and immersed can massively improve a student's experience. ... Game elements from literature review of gamification in healthcare context. Journal of Technology and Science Education, 9(1), 20 ...
Literature retrieval and screening. The current meta-analysis strictly followed the Preferred Reporting Items for Systematic Reviews and Meta-Analyses (PRISMA) criterion to identify relevant empirical research studies (Ziegler et al., 2011).We used three sets of keywords, games ("game-based learning" OR "serious game*" or "educational game*" OR "simulation game*" OR ...
Recommendations are made for the integration of games into teaching and learning in order to maximize student engagement and learning outcomes. ABSTRACT The present study sought to elicit insights into pedagogical practices pertaining to the integration of digital games into teaching and learning. A review of peer-reviewed journal articles published in English over the past 10 years uncovered ...
ABSTRACT. The main purpose of this study is to conduct a systematic literature review of research studies on game-based learning and gamification conducted in Asian K-12 schools. We identified 22 papers based on our inclusion criteria.
Game-based learning (GBL) can allow learners to acquire and construct knowledge in a fun and focused learning atmosphere. A systematic literature review of 42 papers from 2010 to 2020 in this study showed that the current difficulties in implementing GBL in classrooms could be classified into the following categories: infrastructure, resources, theoretical guidance, teacher's capabilities ...
K, P. P., Mittal, M., Aggarwal, A. (2023) Literature Review: Learning Through Ga me-Based Technology Enhances Cognitive Skills. problem analysis in a debate, identifying the ideal solution, and ...
Gamification is closely related to two other concepts: serious games and game-based learning. Game-based learning refers to the achievement of defined learning outcomes through game content and play and enhancing learning by involving problem-solving spaces and challenges that provide learners, who are also players, with a sense of achievement ...
ABSTRACT. A new interest in the use of video games for learning has emerged and a number of claims are made. with respect to the effectiveness of games in education. These educational games are ...
DOI: 10.1016/J.COMPEDU.2019.103667 Corpus ID: 201871608; Digital game-based L2 learning outcomes for primary through high-school students: A systematic literature review @article{Acquah2020DigitalGL, title={Digital game-based L2 learning outcomes for primary through high-school students: A systematic literature review}, author={Emmanuel O. Acquah and Heidi T. Katz}, journal={Comput.
Traditional review sessions (tutorials) are mainly focused on instructor-based learning and they help to review concepts previously taught in the lecture class. The primary objective was to evaluate the cognitive learning of students in game based learning (GBL) as compared to the conventional review sessions.
review examined the effect of digital game-based learning on student learning at the K-12 level. Sixteen peer-reviewed research studies, two meta-analysis studies, and two literature reviews published between 2011-2019 were selected for analysis. The reviewed research indicated that when digital game-based learning is used that includes key ...
This study was conducted by reviewing the literature from 20 studies that provide empirical evidence of the use of game-based learning to develop computational thinking. This study used a literature review method by adapting Brennan and Resnick's framework to identify CT abilities expected to develop in game-based learning.
The entertaining elements implemented in a serious game are key factors in determining whether a player will be engaged in a play-learn process and able to achieve the desired learning outcomes. Thus, optimization of subjective playing experience is a crucial part of a game design process. Flow theory can be adopted for measuring user experience and analyzing the quality of serious game designs.
This study explores the applications of virtual scenario learning in addressing the global issue of school bullying through digital educational tools. Previous research suggests that virtual role-playing experiences can reduce bullying incidents; however, experiencing the victim role can evoke negative emotions, while the bystander role may not fully convey the severity of bullying. This study ...
Systematic literature reviews (SLRs) are critical for life-science research. However, the manual selection and retrieval of relevant publications can be a time-consuming process. This study aims to (1) develop two disease-specific annotated corpora, one for human papillomavirus (HPV) associated diseases and the other for pneumococcal-associated pediatric diseases (PAPD), and (2) optimize ...
This paper provides a systematic review of these works classified into three major categories—logic-based, classical machine learning, and deep learning-based approaches—as discussed in . Logic-based approaches use logic-based formalisms to represent and reason about the actions, plans, and goals of the observed agent [ 12 , 16 , 17 , 18 ...