

Artificial intelligence in education
Artificial Intelligence (AI) has the potential to address some of the biggest challenges in education today, innovate teaching and learning practices, and accelerate progress towards SDG 4. However, rapid technological developments inevitably bring multiple risks and challenges, which have so far outpaced policy debates and regulatory frameworks. UNESCO is committed to supporting Member States to harness the potential of AI technologies for achieving the Education 2030 Agenda, while ensuring that its application in educational contexts is guided by the core principles of inclusion and equity. UNESCO’s mandate calls inherently for a human-centred approach to AI . It aims to shift the conversation to include AI’s role in addressing current inequalities regarding access to knowledge, research and the diversity of cultural expressions and to ensure AI does not widen the technological divides within and between countries. The promise of “AI for all” must be that everyone can take advantage of the technological revolution under way and access its fruits, notably in terms of innovation and knowledge.
Furthermore, UNESCO has developed within the framework of the Beijing Consensus a publication aimed at fostering the readiness of education policy-makers in artificial intelligence. This publication, Artificial Intelligence and Education: Guidance for Policy-makers , will be of interest to practitioners and professionals in the policy-making and education communities. It aims to generate a shared understanding of the opportunities and challenges that AI offers for education, as well as its implications for the core competencies needed in the AI era

The UNESCO Courier, October-December 2023

- Plurilingual
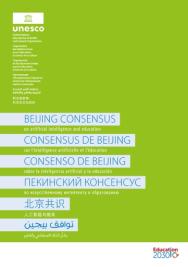
by Stefania Giannini, UNESCO Assistant Director-General for Education
International Forum on artificial intelligence and education
- More information
- Analytical report

Through its projects, UNESCO affirms that the deployment of AI technologies in education should be purposed to enhance human capacities and to protect human rights for effective human-machine collaboration in life, learning and work, and for sustainable development. Together with partners, international organizations, and the key values that UNESCO holds as pillars of their mandate, UNESCO hopes to strengthen their leading role in AI in education, as a global laboratory of ideas, standard setter, policy advisor and capacity builder. If you are interested in leveraging emerging technologies like AI to bolster the education sector, we look forward to partnering with you through financial, in-kind or technical advice contributions. 'We need to renew this commitment as we move towards an era in which artificial intelligence – a convergence of emerging technologies – is transforming every aspect of our lives (…),' said Ms Stefania Giannini, UNESCO Assistant Director-General for Education at the International Conference on Artificial Intelligence and Education held in Beijing in May 2019. 'We need to steer this revolution in the right direction, to improve livelihoods, to reduce inequalities and promote a fair and inclusive globalization.’'

Related items
- Artificial intelligence
- Open access
- Published: 19 September 2022
The application of AI technologies in STEM education: a systematic review from 2011 to 2021
- Weiqi Xu 1 &
- Fan Ouyang ORCID: orcid.org/0000-0002-4382-1381 1
International Journal of STEM Education volume 9 , Article number: 59 ( 2022 ) Cite this article
64k Accesses
51 Citations
12 Altmetric
Metrics details
The application of artificial intelligence (AI) in STEM education (AI-STEM), as an emerging field, is confronted with a challenge of integrating diverse AI techniques and complex educational elements to meet instructional and learning needs. To gain a comprehensive understanding of AI applications in STEM education, this study conducted a systematic review to examine 63 empirical AI-STEM research from 2011 to 2021, grounded upon a general system theory (GST) framework.
The results examined the major elements in the AI-STEM system as well as the effects of AI in STEM education. Six categories of AI applications were summarized and the results further showed the distribution relationships of the AI categories with other elements (i.e., information, subject, medium, environment) in AI-STEM. Moreover, the review revealed the educational and technological effects of AI in STEM education.
Conclusions
The application of AI technology in STEM education is confronted with the challenge of integrating diverse AI techniques in the complex STEM educational system. Grounded upon a GST framework, this research reviewed the empirical AI-STEM studies from 2011 to 2021 and proposed educational, technological, and theoretical implications to apply AI techniques in STEM education. Overall, the potential of AI technology for enhancing STEM education is fertile ground to be further explored together with studies aimed at investigating the integration of technology and educational system.
Introduction
Artificial intelligence in education (AIEd) is an emerging interdisciplinary field that applies AI technologies in education to transform and promote the instructional and learning design, process and assessment (Chen et al., 2020 ; Holmes et al., 2019 ; Hwang et al., 2020 ). The application of AI in STEM education (referred to AI-STEM in this paper), as a sub-branch of AIEd, focuses on the design and implementation of AI applications to support STEM education. Automated AI technologies, e.g., intelligence tutoring, automated assessment, data mining and learning analytics, have been used in STEM education to enhance the instruction and learning quality (Chen et al., 2020 ; Hwang et al., 2020 ; McLaren et al., 2010 ). STEM education is a complex system, from a system perspective, consisting of interdependent elements, including subject, information, medium, and environment (Rapoport, 1986 ; Von Bertalanffy, 1968 ). The application of AI, as a critical technology element, should take careful consideration of these complex factors, to achieve a high-quality STEM education (Byrne & Callaghan, 2014 ; Krasovskiy, 2020 ; Xu & Ouyang, 2022 ). This systematic review aims to examine the different elements, including AI technology, subject, information, medium, environment in the AI-STEM system to gain a holistic understanding of the application and integration of AI technologies in the STEM education contexts. Specifically, we collected and reviewed empirical AI-STEM research from 2011 to 2021, summarized the AI techniques and applications, the characteristics of other system elements (i.e., information, subject, medium, environment), the distribution of AI in these elements, and the effects of AI in STEM education. Based on the results, this systematic review provided educational and technological implications for the practice and research in the AI-STEM education.
Literature review
With the development of computer science and computational technologies, automatic, adaptive, and efficient AI technologies have been widely applied in various academic fields. Artificial Intelligence in Education (AIEd), as an interdisciplinary field, emphasizes applying AI to assist instructor’s instructional process, empower student’s learning process, and promote the transformation of educational system (Chen et al., 2020 ; Holmes et al., 2019 ; Hwang et al., 2020 ; Ouyang & Jiao, 2021 ). First, AIEd has potential to enhance instructional design and pedagogical development in the teaching processes, such as accessing students’ performance automatically (Wang et al., 2011 ; Zampirolli et al., 2021 ), monitoring and tracking students’ learning (Berland et al., 2015 ; Ji & Han, 2019 ), and predicting at-risk students (Hellings & Haelermans, 2020 ; Lamb et al., 2021 ). Second, AIEd is beneficial for improving student-centered learning, such as providing adaptive tutoring (Kose & Arslan, 2017 ; Myneni et al., 2013 ), recommending personalized learning resources (Ledesma & García, 2017 ; Zhang et al., 2020 ), and diagnosing students’ learning gaps (Liu et al., 2017 ). Third, AIEd also brings opportunities to transform the educational system by highlighting the essential role of technology (Hwang et al., 2020 ), enriching the mediums of knowledge delivery (Holstein et al., 2019 ; Yannier et al., 2020 ), and changing the instructor–student relationship (Xu & Ouyang, 2022 ). Overall, different AI technologies (e.g., machine learning, deep learning) have been deployed in the field of education to enhance instructional and learning process.
The development of AIEd also brought transformations to the field of science, technology, engineering and mathematics (STEM) education, as a sub-branch of AIEd named AI-STEM. STEM education aims to improve students’ interdisciplinary knowledge inquiry and application, as well as their higher-order thinking, critical thinking and problem-solving ability (Bybee, 2013 ; Pimthong & Williams, 2018 ). The application of AI in STEM education has advantages to provide adaptive and personalized learning environments or resources, and aid instructors to understand students’ learning behavioral patterns, and automatically assess STEM learning performances (Alabdulhadi & Faisal, 2021 ; Walker et al., 2014 ). However, STEM education is a complex system, consisting of interdependent elements, including subject (e.g., instructor, student), information, medium, and environment (Rapoport, 1986 ; Von Bertalanffy, 1968 ). Achieving a high quality of STEM education requires a careful consideration of the complex social, pedagogical, environmental factors, rather than merely applying AI technologies in education (Krasovskiy, 2020 ; Xu & Ouyang, 2022 ). Therefore, a major challenge in AI-STEM is how to appropriately select and apply AI techniques to adapt to the multiple elements (e.g., subject, information, environment) in STEM education with a goal of high-quality instruction and learning (Castañeda & Selwyn, 2018 ; Selwyn, 2016 ). To gain a holistic understanding of the integration of AI technologies in the STEM education contexts, it is crucial to systematically review and examine the complex elements in AI-STEM from a system perspective.
During the past decade, the emerging field of AIEd has gained great attention (Chen et al., 2020 ; Holmes et al., 2019 ; Hwang et al., 2020 ; Ouyang et al., 2022 ). But existing literature review of AIEd has mainly focused on the trends, applications, and effects of AIEd from a technological perspective (Chen et al., 2020 ; Tang et al., 2021 ; Zawacki-Richter et al., 2019 ). Specifically, we located 18 literature review articles of AIEd published from 2011 to 2021 (see Fig. 1 ). These AIEd reviews focused on different educational levels, fields, and contexts, including higher education (Zawacki-Richter et al., 2019 ), e-learning (Tang et al., 2021 ), mathematics education (Hwang & Tu, 2021 ), language education (Liang et al., 2021 ), medical education (Khandelwal et al., 2019 ; Lee et al., 2021 ), programming education (Le et al., 2013 ), and special education (Drigas & Ioannidou, 2012 ). For example, Zawacki-Richter et. al. ( 2019 ) reviewed AIEd in the higher education context and four AI technical applications were classified, namely intelligent tutoring systems, adaptive systems and personalization, profiling and prediction, and assessment and evaluation. Liang et. al. ( 2021 ) focused on the application of AI in language education and investigated the roles and research foci (e.g., research methods, research sample groups) of AI techniques in language education. Drigas and Ioannidou ( 2012 ) explored AIEd in special education and summarized AI applications based on the student’s disorders, including reading, writing and spelling difficulties, dyslexia, autistic spectrum disorder, etc.
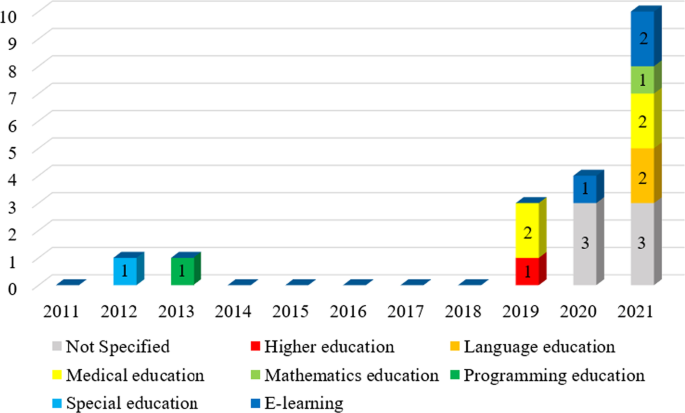
Existing literature review of AIEd articles, ranging from 2011 to 2021
Although various reviews were conducted to understand the field of AIEd, few of them focused on STEM education. Among these 18 literature review articles, we only located two works exploring the application of AI in STEM education. Le et. al. ( 2013 ) reviewed the AI-supported tutoring approaches in computer programming education and found that AI techniques were mainly applied to support feedback-based programming tutoring during the student’s individual learning. Hwang and Tu ( 2021 ) conducted a bibliometric mapping analysis to systematically review the roles of AI in mathematics education. The results clarified the role of AI in mathematics education into three main types, including intelligent tutoring systems, profiling and prediction, and adaptive systems and personalization. Although some review examined AI in computer science and mathematics education, there is a lack of literature review to investigate the application of AI in general STEM education context. More importantly, due to the complexity of AI-STEM, it is essential to systematically review multiple elements in AI-STEM as well as the effects of AI in the STEM education system.
To fill this gap, this systematic review aims to gain a comprehensive understanding of the integration of AI technologies in the STEM education contexts. Specifically, this review examined and summarized the applications and categories of AI element in the AI-STEM system, the characteristics of other system elements in AI-STEM except AI, the distribution of AI in these elements, and the effects of AI in STEM education. Three research questions (RQs) were proposed:
RQ1: What are the categories of the AI element in the AI-STEM system?
Rq2: what are the characteristics of other system elements (i.e., information, subject, medium, environment element) as well as the distribution of ai in these elements, rq3: what are the effects of ai in stem education.
In order to map the state-of-art of the application of AI techniques in STEM education, we conducted a systematic review from 2011 to 2021, following the Preferred Reporting Items for Systematic Reviews and Meta-Analyses (PRISMA) principles (Moher et al., 2009 ).
Database search
To locate the empirical studies of AI application in STEM education, the following major publisher databases were selected: Web of Science, Science Direct, Scopus, IEEE, EBSCO, ACM, Taylor & Francis, and Wiley (Guan et al., 2020 ). Filters were used to the empirical research and peer-reviewed articles in the field of education and educational research from January 2011 to December 2021. After the preliminary screening of articles, snowballing was conducted (Wohlin, 2014 ) to find the articles that were not extracted using the search strings.
Identification of search terms
Based on the specific requirements of bibliographic databases, we proposed the searching strategies. In terms of the research questions, three types of keywords were used as the search terms. First, keywords related to AIEd and specific AI applications were added (i.e., “artificial intelligence” OR “AI” OR “AIED” OR “machine learning” OR “intelligent tutoring system” OR “expert system” OR “recommended system” OR “recommendation system” OR “feedback system” OR “personalized learning” OR “adaptive learning” OR “prediction system” OR “student model” OR “learner model” OR “data mining” OR “learning analytics” OR “prediction model” OR “automated evaluation” OR “automated assessment” OR “robot” OR “virtual agent” OR “algorithm”). Second, keywords related to STEM were added (i.e., “STEM” OR “science” OR “technology” OR “math” OR “physics” OR “chemistry” OR “biology” OR “geography” OR “engineering” OR “programming” OR “lab”). Third, keywords related to education were added (i.e., “education” OR “learning” OR “course” OR “class” OR “teaching”).
Searching criteria
The search criteria were designed to locate the articles that focused on the applications of AI in STEM education. According to the research objectives, inclusion and exclusion criteria were adopted (see Table 1 ).
The screening process
The screening process involved the following procedures: (1) removing the duplicated articles; (2) reading the titles and abstracts and removing the articles according to the inclusion and exclusion criteria; (3) reading the full texts and removing the articles according to the inclusion and exclusion criteria; (4) using the snowballing to further locate the articles in Google Scholar, and (5) extracting data from the final filtered articles (see Fig. 2 ). All articles were imported into Mendeley software for screening.
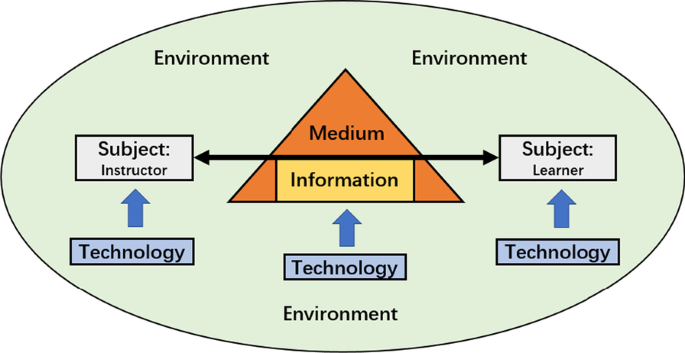
The selection flowchart used based on PRISMA (Moher et al., 2009 )
3373 articles were located as the result of the first round of searching. Among these records, 777 duplicates were removed and then 1879 records were excluded because they were not classified under Education & Educational research or journal article. By reviewing the titles and abstracts, the number of articles was reduced to 717 based on the criteria (see Table 1 ). The selected articles were examined by the first author to determine whether they were suitable for the purpose of this systematic review. The second author independently reviewed approximately 30% of the articles to confirm the reliability. The inter-rater agreement was 92%. Then, the full-text of articles were reviewed by the first author to verify that the articles met all the criteria for inclusion in the review. Finally, a total of 63 articles that met the criteria were identified for the systematic review.
Theoretical framework and analysis procedure
General system theory (GST) is a theoretical framework, arguing that the world is composed of different organic systems, which contain dynamically interacting elements and mutual relationships between them (Rapoport, 1986 ; Von Bertalanffy, 1950 ). The main principle of GST is that a system is not simply equal to the sum of its elements, but greater than the sum of its parts (Drack & Pouvreau, 2015 ; Von Bertalanffy, 1968 ). To deeply understand the complex nature and general rules of systems, GST highlights the system’s holistic principle to identify the internal elements, functional relationships of them, as well as the external influences upon a system (Crawford, 1974 ). The theoretical framework of GST has been widely applied in various fields to analyze different types of systems, such as physical, biological, social and educational systems (Drack & Pouvreau, 2015 ; Kitto, 2014 ). For example, Chen and Stroup ( 1993 ) suggested applying GST as an underpinned theoretical framework to guide the reform of science education and highlighted the integration of science curriculum to avoid the compartmentalized learning of physics, biology, and chemistry. Following this philosophy, we argue that GST can provide a new, holistic perspective to understand the integration of AI technologies and STEM education.
From the perspective of GST, AI-STEM can be viewed as an organic system, which mainly contains five basic elements, namely subject , information , medium , environment , and technology (Von Bertalanffy, 1968 ) (see Fig. 3 ). First, subject is defined as people in an educational system and different subjects of people (e.g., instructor, student) can take agency to interact with each other constantly and adaptively. Second, information refers to knowledge spread and constructed between subjects in an educational system, such as learning contents, course materials, knowledge artifacts, etc. Third, medium is the way or carrier to convey information and connect subjects in the system. Fourth, environment serves as an underlying context in an educational system, which influences the function of the whole educational system. Fifth, technology (e.g., AI techniques) is usually appeared as an external element to impact the functions of the educational system. Grounded upon GST, the integration of AI, as an external technology element, in an educational system (such as STEM) is a complex process, that has influences on other system elements (i.e., subject, information, medium, environment) and on the relationships between them. In summary, the framework of GST (see Fig. 3 ) highlights the multiple elements as well as their mutual relationships in AI-STEM system, which provides us a holistic view for applying AI technologies in STEM education.
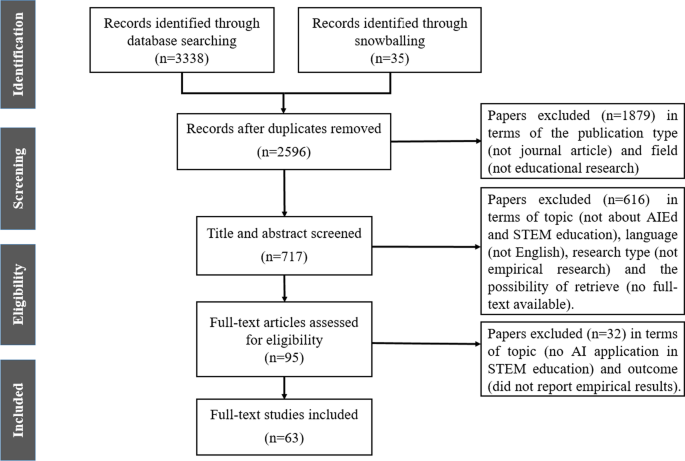
The integration of technology in an educational system from the GST perspective
We used content analysis method (Cohen et al., 2005 ; Zupic & Čater, 2015 ) to classify 63 AI-STEM articles in order to answer the research questions. Based on GST, a coding scheme of educational system elements was developed to systematically examine AI-STEM articles (see Table 2 ). This coding scheme included the subject of instructor (including instructor involvement and instructor strategy), the subject of learner (including educational level, sample size, and learning outcome), information (i.e., learning content), medium (i.e., educational medium), environment (i.e., educational context), and technology (i.e., AI technique).
63 articles were coded by two raters. The same article can be coded more than one code in one dimension. First, 20% of articles were coded by two coders independently in order to calculate coding reliability. Krippendorff’s ( 2004 ) alpha reliability was 0.91 among two raters at this phase. The remaining articles were coded independently by two raters after the reliability was ensured. Consensus was reached by two raters on conflicting coding results. We provided details and examples below to demonstrate how the coding results represented the review data (Graneheim & Lundman, 2004 ).
To answer the research questions, the results section presents the following three main topics: (1) the categories of the AI element in the AI-STEM system; (2) the characteristics of other system elements (i.e., information, subject, medium, and environment) as well as the distribution of AI in these elements, and (3) the effects and findings of the application of AI in STEM education.
Figure 4 demonstrates the trends of empirical studies by year. According to the distribution, the number of publication generally increased along the years. In addition, a majority of reviewed articles ( N = 42) were published in the last 4 years from 2018 to 2021. Only 9 of 63 reviewed articles were published in the first 4 years from 2011 to 2014.
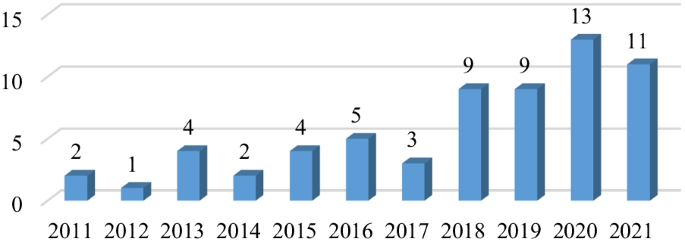
Distribution of the articles by year ( N = 63)
Regarding the element of AI technology in AI-STEM, six types of AI applications were identified, namely learning prediction ( N = 18, percentage = 29%), intelligent tutoring system ( N = 16, percentage = 25%), student behavior detection ( N = 13, percentage = 21%), automation ( N = 8, percentage = 13%), educational robots ( N = 6, percentage = 9%), and others ( N = 2, percentage = 3%) (see Fig. 5 and Table 3 ).
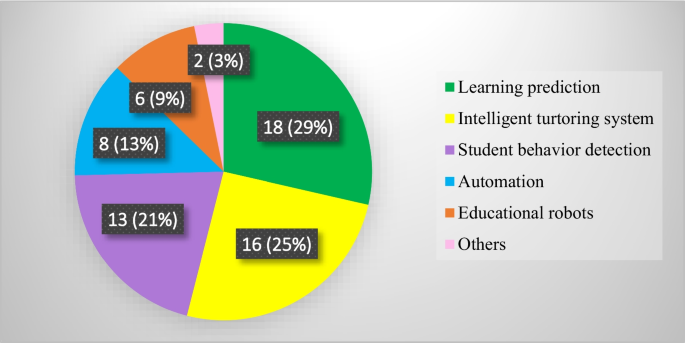
The application categories of AI techniques in STEM education ( N = 63)
Learning prediction
The first category of the AI applications in STEM education was learning prediction , illustrating should be something like systems which predict student learning performance or status in advance through AI algorithms and modeling approaches (Agrawal & Mavani, 2015 ; Lee et al., 2017 ). 18 of 63 reviewed articles (29%) focused on learning prediction in STEM education (see Fig. 5 ). Two sub-categories were summarized under learning prediction: learning performance prediction ( N = 14) and at-risk student prediction ( N = 4) (see Table 3 ). First, in the sub-category of learning performance prediction, AI algorithms and modeling techniques were employed in STEM education to help instructors adjust the instructional processes by predicting students’ learning performance (Deo et al., 2020 ; Hellings & Haelermans, 2020 ). For example, Buenaño-Fernández et. al. ( 2019 ) applied educational data mining and machine learning technique (i.e., decision tree) in computer engineering courses to predict students’ final performance based on their historical grades. Zabriskie et. al. ( 2019 ) utilized the random forest model and logistic regression model to predict the physics course outcomes. Another sub-category was predicting at-risk students and dropout factors in STEM education to help instructors intervene in student learning (Vyas et al., 2021 ; Yang et al., 2020 ). For example, Lacave et. al. ( 2018 ) used Bayesian networks techniques to investigate dropout factors of computer science students in higher education. Yang et. al. ( 2020 ) utilized random forest classification to create and examine the prediction models of identifying at-risk students in introductory physics classes. In summary, AI algorithms had been used in STEM education to help instructors or researchers predict students’ final academic performances and learning risks.
Intelligent tutoring system
The second category of the AI applications in STEM education was the intelligent tutoring system (ITS), defined as an AI-enabled system that was designed to provide customized instruction or feedback to students and promote personalized, adaptive learning (Chen et al., 2020 ; Hooshyar et al., 2015 ; Murray, 2003 ). Among the 63 reviewed articles, 16 articles (25%) focused on the applications of ITSs in promoting instruction and learning in STEM education (see Fig. 4 ). Three sub-categories were identified: instructional content delivery ( N = 9), recommendation of personalized learning path ( N = 4), and resource recommendation ( N = 3) (see Table 3 ). The first sub-category was using ITSs to deliver instructional content in STEM education. For example, Myneni et. al. ( 2013 ) introduced an interactive and intelligent learning system in physics education, where a virtual agent delivered physics concepts to students and decision algorithms were utilized to determine the support level of the virtual agent. Hooshyar et. al. ( 2018 ) proposed a novel flowchart-based ITS based on Bayesian networks techniques, which imitated a human instructor to conduct one-to-one instruction with students. The second sub-category of ITS was recommendations of personalized learning path based on student’s profile in STEM education. For example, De-Marcos et. al. ( 2015 ) combined genetic algorithm and parliamentary optimization algorithm to create personalized courseware sequencing paths in online STEM learning. Saito and Watanobe ( 2020 ) proposed an approach of recommending learning paths that applied recurrent neural network and sequential prediction model to create students’ ability charts and learning paths in programming learning based on their submission history. The third sub-category of ITS was recommending learning resource according to student’s needs in STEM education. For example, Ledesma and García ( 2017 ) introduced an expert system as a support tool to tackle mathematical topics, by recommending appropriate mathematical problems in accordance with a student’s learning style. Lin and Chen ( 2020 ) proposed a deep learning recommendation based system in programming learning that recommended learning tasks, learning missions and materials according to students’ learning processes and levels. In summary, AI technologies were widely applied in ITSs to enhance personalized and adaptive learning in STEM education through providing one-to-one tutoring and recommending personalized learning paths and resources.
Student behavior detection
The third category of the AI applications in STEM education was student behavior detection , which referred to systems to exploit and track students’ learning behaviors, patterns, and characteristics with AI-enabled data mining and learning analytics in the instructional and learning processes (Chrysafiadi & Virvou, 2013 ; Ji & Han, 2019 ; Zheng et al., 2020 ). Among the 63 reviewed articles, 13 articles (21%) focused on the applications of AI techniques to detect student behaviors in STEM education (see Fig. 5 ). Two sub-categories were summarized under student behavior detection: student behavior analysis ( N = 8) and student behavior monitoring ( N = 5) (see Table 3 ). First, the sub-category of student behavior detection, was applied in STEM education to analyze and reveal students’ latent behaviors. For example, Hsiao et. al. ( 2020 ) collected students’ learning data from programming learning platform and examined their learning behaviors through hidden Markov model, and the results revealed the reviewing patterns and reflecting strategies of students in learning programming. Pereira et. al. ( 2020 ) used data mining techniques including k-means and association rule algorithm to understand students’ behavior in introductory programming, to help novice programmers promote their learning. Another sub-category of student behavior monitoring was applied to help instructors track students’ learning in STEM education. For example, Balakrishnan ( 2018 ) helped instructors to motivate engineering students’ learning through monitoring their learning behaviors such as preferred learning materials and self-directed learning performance. Yannier et. al. ( 2020 ) introduced a mixed-reality AI system supported with computer vision algorithms to track children’s active learning behaviors in science education. In summary, student behavior detection had great potential to aid instructors and researchers to analyze, understand, and monitor students’ behaviors in STEM education.
The fourth category of the AI applications in STEM education was automation , which utilized AI technologies to automatically assess students’ performances and generate questions or tasks for instructors (Aldabe & Maritxalar, 2014 ; Wang et al., 2011 ; Zampirolli et al., 2021 ). Among 63 reviewed articles, 8 articles (13%) focused on the AI-supported automated techniques in STEM education (see Fig. 5 ). Two sub-categories were summarized under automation: automated assessment ( N = 7) and automated questions generation ( N = 1) (see Table 3 ). The first sub-category of automated assessment provided instructors and students with convenient assistance in STEM education. For example, Wang et. al. ( 2011 ) developed an automated assessment system, AutoLEP, to help novice programmers gain programming skills by providing syntactic and structural checking and immediate feedback automatically. García-Gorrostieta et. al. ( 2018 ) introduced a system for automatic argument assessment of computer engineering students’ final reports, to help them improve the abilities of statement and justification in science argumentation. Another sub-category of automated questions generation had potential to reduce instructors’ instructional burdens in STEM education. For example, Aldabe and Maritxalar ( 2014 ) proposed an approach to help instructors automatically create multiple-choice tests in science courses through the use of corpora and natural language processing techniques. In summary, AI techniques were used in STEM education to aid instructors and students through automatically generating questions and assessing academic performances.
Educational robots
The fifth category of the AI applications in STEM education was educational robots , which was the adoption of robots in STEM education to facilitate students’ learning experience as well as allow them to acquire knowledge in interactive ways (Atman Uslu et al., 2022 ; Cao et al., 2021 ; Yang & Zhang, 2019 ). It is worth noting that robots are applications that contain various techniques (e.g., mechanical manufacturing, electronic sensors, AI); therefore, considering the research topic, only AI-supported robots were included in this review. Among the 63 reviewed articles, 6 articles (9%) focused on the application of educational robots in STEM education (see Fig. 5 ). Two sub-categories were identified under educational robots: programming robots ( N = 3) and social robots ( N = 3) (see Table 3 ). The first sub-category, programming robots, were specifically designed as learning tools that engaged students to design and operate them with programming languages (Atman Uslu et al., 2022 ). For example, Rodríguez Corral et. al. ( 2016 ) applied a specific ball-shaped robot with sensing, wireless communication and output capabilities in computer courses to teach students object-oriented programming languages. Cao et. al. ( 2021 ) introduced an artificial intelligence robot called LEGO MINDSTORMS EV3, to implement instructional tasks in information technology courses to promote students’ innovation and operational ability. Another sub-category of social robots was a kind of intelligent humanoid robots, which could serve as tutors, tutees or learning companions to students and allow students to interact with them orally and physically (Belpaeme et al., 2018 ; Xu & Ouyang, 2022 ). For example, Verner et. al. ( 2020 ) employed RoboThespian, a life-size humanoid robot, as a tutor to convey science knowledge and concepts to elementary school students. In summary, AI-based educational robots were used in STEM education as instructional tools or educational subjects (e.g., tutor, tutee, companion) to convey knowledge, promote students’ operational skills, and enhance their learning experience.
Among 63 reviewed articles, 2 articles (3%) focused on other applications of AI techniques in STEM education, including AI textbook and group formation. Tehlan et. al. ( 2020 ) utilized a genetic algorithm‐based approach to form student groups in collaborative learning based on their skills and personality traits in a programming course. Koć-Januchta et. al. ( 2020 ) introduced AI-enriched textbook in biology course to improve students’ engagement by encouraging them to ask questions and receive suggested questions.
To further understand how AI techniques have been integrated in STEM education, we examined the other system elements, including information, subject (i.e., instructor, student), medium, and environment in AI-STEM research. In addition, we explored the distribution of AI categories in these elements, to reveal the relationships between AI techniques and these elements.
Information in AI-STEM research
Information (referred to learning content in this study) was described as the subject knowledge and learning contents conveyed in AI-STEM system. In the reviewed 63 studies, all of them mentioned learning content in STEM education, including science, technology, engineering, mathematics, and cross-disciplinary (i.e., more than one discipline) (see Table 4 ). Among 63 articles, 24 studies focused on technology, followed by articles that focused on science ( N = 22) and engineering ( N = 7). Mathematics ( N = 3) attracted the least attention. In addition, 7 studies contained interdisciplinary subjects, such as computer engineering (Buenaño-Fernández et al., 2019 ; Tehlan et al., 2020 ), engineering mathematics (Deo et al., 2020 ), and integrated STEM education (Suh et al., 2019 ; Wang, 2016 ).
Figure 6 shows the frequency of AI application categories in different learning contents. Among all the AI categories, student behavior detection was most frequently applied in the technology domain ( N = 8), followed by learning prediction in science ( N = 6), and learning prediction in engineering ( N = 5) (see Fig. 6 ).
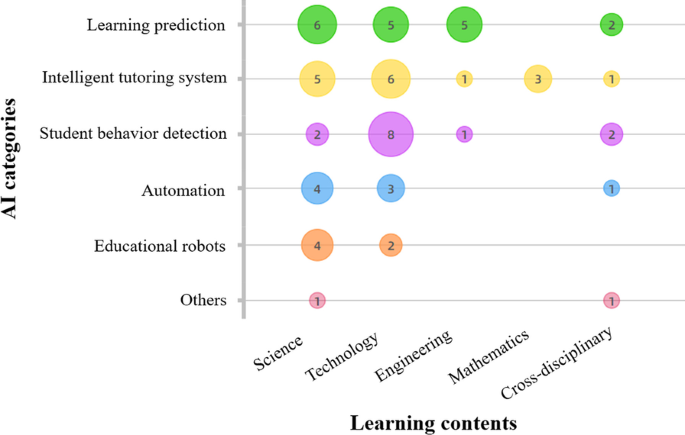
AI categories under learning content ( N = 63)
Instructor in AI-STEM research
Instructor , as a component of subject element in AI-STEM system, played a critical role in conducting instruction, conveying knowledge, and utilizing technologies. In the reviewed 63 studies, 50 of them mentioned the instructor involvement and 50 of them mentioned the instructional strategies, including traditional lecture, problem-based learning, project-based learning, game-based learning, self-learning, and collaborative learning (see Table 5 ). Regarding the instructor involvement, a majority of instructors would engage in the instructional and learning processes to support students ( N = 42), while some studies were conducted without instructors’ involvement and support ( N = 8). Additionally, among 50 articles, the traditional lecturing strategy was most frequently used by instructor ( N = 27), followed by problem-based learning ( N = 10). Also, some studies were carried out through project-based learning ( N = 5), self-learning ( N = 5), game-based learning ( N = 4), and collaborative learning ( N = 4).
All AI application categories were mainly applied with the instructor’s support, in which the most frequently used AI category were ITS ( N = 11) and learning prediction ( N = 11) (see Fig. 7 a). In addition, automation was only applied in lecture ( N = 8). Learning prediction was most frequently applied in lecture ( N = 8) and educational robots were most frequently applied in problem-based learning ( N = 4). Compared to other AI technologies, ITS and student behavior detection were integrated with more types of instructional strategies (see Fig. 7 b).
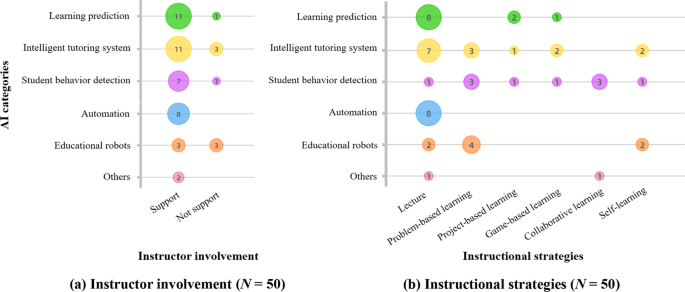
AI categories under instructor involvement and instructional strategies
Learner in AI-STEM research
Learner , as another component of subject element, could take agency to actively participate in the learning process as to influence the AI-STEM system. In the reviewed 63 studies, 59 of them mentioned the educational levels of learners, from kindergarten to higher education, and 55 of them mentioned sample sizes (see Table 6 ). Among all the educational levels, 43 focused on higher education ( N = 43), followed by elementary school ( N = 7), high school ( N = 5), and middle school ( N = 4). Only one study was conducted in kindergarten. In addition, the number of AI-STEM studies with the medium scale of learners ( N = 24) and the large scale of learners ( N = 21) were larger than the small-scale study ( N = 10).
AI application categories except educational robots were frequently applied in higher education (learning prediction: N = 13, ITS: N = 12, student behavior detection: N = 8, automation: N = 7). The educational robots were frequently applied in elementary school ( N = 3) (see Fig. 8 a). Moreover, regarding the sample size, learning prediction was most frequently used with a large scale ( N = 11), followed by ITS with a medium scale ( N = 9), and student behavior detection with a medium scale ( N = 7). Additionally, the categories of educational robots and others were not applied in large scale; the category of student behavior detection was not applied in small scale (see Fig. 8 b).
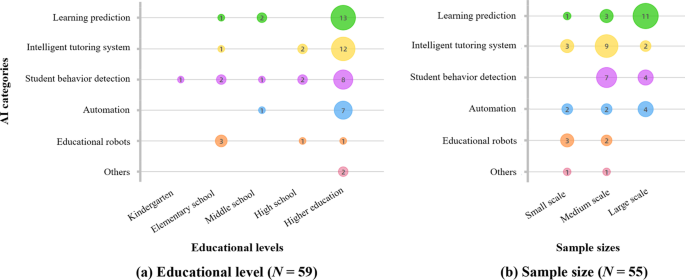
AI categories under educational levels and sample sizes
Medium in AI-STEM research
Medium (referred to educational medium in this study) was viewed as the way to convey information and connect subjects AI-STEM system. In the reviewed 63 studies, 50 of them mentioned the educational medium, including paper resource, entity resource (i.e., the material object in reality), computer system resource, web open resource, mobile phone resource and E-book resource (see Table 7 ). Among all educational mediums, computer system was the most frequently used in AI-STEM studies ( N = 28), followed by entity resource ( N = 10) and web open resource ( N = 9). Additionally, mobile phone resource ( N = 3), traditional paper resource ( N = 1), and E-book resource ( N = 1) was the infrequent medium to convey knowledge.
Among all the AI categories, ITS was most frequently used through computer system resource ( N = 15), followed by educational robots through entity resource ( N = 6), automation through computer system resource ( N = 5), and learning prediction through web open resource (see Fig. 9 ).
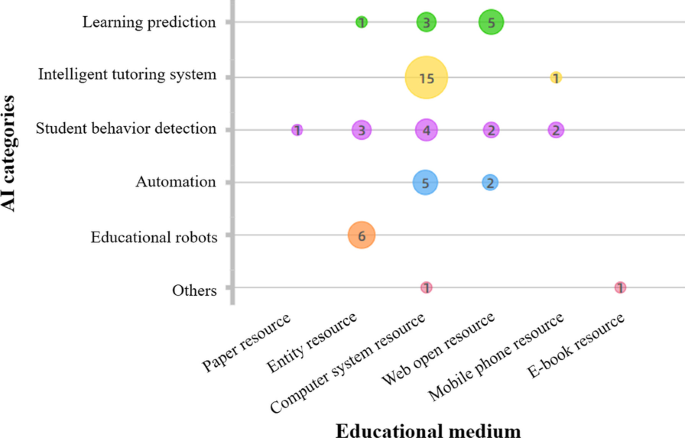
AI categories under educational medium ( N = 50)
Environment in AI-STEM research
Environment (referred to educational context in this study) served as an underlying context to influence the whole AI-STEM system. In the reviewed 63 studies, 51 of them mentioned the educational environment, including face-to-face environment, experimental learning environment, informal learning environment, web-based environment and augmented/virtual reality (see Table 8 ). Among the 51 studies, 33 studies were implemented in face-to-face environment, followed by web-based environment ( N = 11) and experimental environment ( N = 6). Two studies conducted in informal learning environment (McLurkin et al., 2013 ; Verner et al., 2020 ) and only one study conducted in augmented reality (Lin & Chen, 2020 ).
All categories of AI techniques were commonly applied in face-to-face environment, in which the most frequently used AI technology category was learning prediction ( N = 10), followed by automation ( N = 7), ITS ( N = 6), and educational robots ( N = 5). Moreover, compared to other AI categories, ITS was the most frequently used technique in the web-based environment ( N = 7) (see Fig. 10 ).
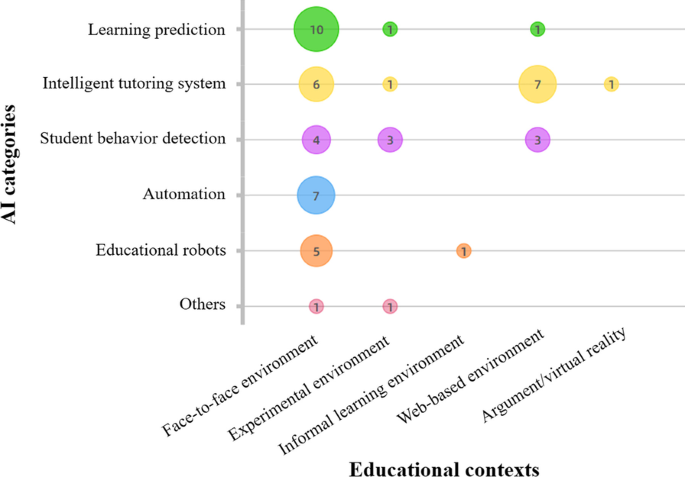
AI categories under educational contexts ( N = 51)
This review summarized the educational and technological effects of AI applications in AI-STEM research.
Educational effects and findings
From the educational perspective, 42 of the 63 reviewed articles reported the educational effects and findings when applying AI techniques in STEM education. Specifically, 30 out of the 42 articles reported the instruction and learning effects (e.g., learning performance, affective perception, higher-order thinking) of the application of AI techniques in STEM education. 12 articles out of 42 reported students’ learning behaviors and patterns by using AI-enabled data mining and learning analytics techniques.
The effect of learning performance
Among all the reviewed articles, 22 studies revealed the educational effects of AI technologies on students’ learning performance. Most of them showed significantly positive influence of AI techniques on the improvement of students’ learning performances ( N = 20). For example, Wu et. al. ( 2013 ) investigated the effect of a context-aware ubiquitous learning system in a geosciences course and the results showed that context-aware ubiquitous learning system had significantly positive effects on the learning achievements of students. Thai et. al. ( 2021 ) conducted a cluster randomized study to examine the effect of My Math Academy, a digital game-based learning environment that provided personalized content on kindergarten students; the results revealed the significant improvement of learning gains, especially for the moderate-level students. Tehlan et. al. ( 2020 ) used a quasi-experiment approach to examine the effects of genetic algorithm-supported pair programming in a programming course; the results found that the students’ learning performances were significantly higher in pair programming than individual programming. Two articles reported insignificant results of the learning performance effects. Koć-Januchta et. al. ( 2020 ) used a quasi-experiment to compare the effect of AI-enabled E-book and common E-book in students’ biology learning, and the results showed that there was no significant difference of students’ learning gains between these two types of books. Also, Hellings and Haelermans ( 2020 ) conducted a randomized experiment to examine the effect of a learning analytics dashboard with predictive function in a computer programming course, but no significant improvement was found on student performance in the final exam.
The effect of affective perception
Among all the reviewed articles, a majority of studies revealed the educational effects of AI technologies on students’ affective perception, such as attitude, interest, and motivation ( N = 17). On the one hand, students showed satisfaction and positive attitude towards the integration of AI technologies and STEM education. For example, Azcona et. al. ( 2019 ) used a questionnaire to find students’ positive feedbacks and attitudes towards the application of learning analytics in computer programming classes to detect and warn learning risks. Gavrilović et. al. ( 2018 ) evaluated student’s satisfaction of an AI-supported adaptive learning system in Java programming learning through the survey approach; the results revealed the positive feedbacks of students. On the other hand, the application of AI technologies also arouses students’ interests and motivation in STEM learning. For example, Balakrishnan ( 2018 ) used a mixed-method approach (i.e., questionnaire and interview) to examine the impact of a computer-based personalized learning environment (PLE) on engineering students’ motivation, and the results revealed the potential of PLE to engage students in learning with a strong sense of interest and motivation. Verner et. al. ( 2020 ) investigated students’ perceptions and attitudes towards an interactive robot tutor in science classes and found that the human–robot interaction fostered students’ active learning, maintain their attention and interest in the learning processes.
The effect of higher-order thinking
Among all the reviewed articles, some studies revealed the educational effects of AI technologies on students’ higher-order thinking ( N = 7), such as problem-solving ability, computational thinking, and self-regulated learning skills. For example, Hooshyar et. al. ( 2015 ) employed a quasi-experimental design to examine the impact of a flowchart-based intelligent tutoring system (FITS) on students’ programming learning and found better improvement of problem-solving abilities in the FITS group than the control group. Lin and Chen ( 2020 ) found that students who used a deep learning-based AR system performed significantly better in computational thinking than those using an AR system without deep learning recommendation. Jones and Castellano ( 2018 ) utilized adaptive robotic tutors to promote students’ self-regulated learning skills and found that when a robotic tutor provided scaffoldings adaptively, more self-regulated learning behaviors were observed from students over the control condition without scaffoldings. García-Gorrostieta et. al. ( 2018 ) used experimental evaluation to test the effect of the automatic argument assessment on students’ computer engineering writing, and the results revealed that the argument assessment system helped students improve argumentation ability in their writing.
The effect of student learning pattern and behavior
Among 42 reviewed articles that mentioned educational effects and findings, 12 articles revealed students’ learning patterns and behaviors in STEM education by using AI-enabled data mining and learning analytics approaches. For example, Sapounidis et. al. ( 2019 ) detected 48 children’s preference profiles on tangible and graphical programming through latent class modeling; results found that the graphical programming was preferred by a majority of children, especially children in younger ages. Pereira et. al. ( 2020 ) used learning analytics (i.e., k-means, association rule algorithms) in the Amazonas to understand students’ behavior in introductory programming courses and found high heterogeneity among them. Three clusters of novice programmers were detected to explain how student behaviors during programming influenced the learning outcomes. Wang ( 2016 ) utilized data mining and learning analytics techniques (i.e., association rule, decision tree) to investigate college students’ course-taking patterns in STEM learning; the results found that the most viable course-taking trajectories is taking mathematics courses after initial exposure to subject courses in STEM.
Technological effects and findings
From the technological perspective, 24 of the 63 reviewed articles reported the technological effect and findings (e.g., efficiency of technology, accuracy of algorithm) when applying AI techniques in STEM education. For example, Çınar et. al. ( 2020 ) utilized multiple machine learning algorithms, including Support Vector Machines (SVM), Gini, k-Nearest Neighbors (KNN), Breiman’s Bagging, Freund and Schapire’s Adaboost.M1 algorithms, to automatically grade open-ended physics questions; the results reported that AdaBoost.M1 had the best performance with the highest accuracy of prediction models among all machine learning algorithms. Nehm et. al. ( 2012 ) used a corpus of biology evolutionary explanations written by 565 undergraduates to test the efficacy of an automated assessment program, Summarization Integrated Development Environment (SIDE); the results showed that, compared to human expert scoring, SIDE had better performance when scoring models were built and tested at the individual item level, and the performance degraded when suites of items or entire instruments were used to build and test scoring models. Bertolini et. al. ( 2021 ) employed five machine learning methods to quantify predictive efficacy of predictive modeling in undergraduate students’ outcome in biology. Results found that individual machine learning methods, especially logistic regression achieved a poor prediction performance while ensemble machine learning methods, in particular the generalized linear model with elastic net (GLMNET), achieved the high accuracy. Deo et. al. ( 2020 ) adopted a computationally efficient AI model called extreme learning machines (ELM) to predict weighted score and the examination score in engineering mathematics courses; the results showed that ELM outperformed in prediction with respect to random forest and Volterra.
Discussion and implications
Addressing research questions.
Although AIEd has attracted wide attention in educational research and practice, few research works have investigated the applications of AI in STEM education context. To gain a comprehensive understanding of the integration of AI in STEM education, this study conducted a systematic review of AI-STEM empirical research from 2011 to 2021. Grounded upon GST, we examined the AI technologies and applications in STEM education, the characteristics of other system elements (i.e., information, subject, medium, environment), the distribution of AI in these elements, and the effects of AI applications in STEM education. To answer the first question, we found a gradually increasing trend of AI applications in STEM education in the past decade. Furthermore, six categories of AI applications were located, namely learning prediction, ITS, student behavior detection, automation, educational robots, and others (i.e., AI text book, group formation). Regarding the characteristics of elements and the distribution of AI in these elements, first, we found all categories of AI techniques, especially student behavior detection, ITS, and learning prediction, were frequently applied in the learning contents of science and technology. Second, instructors usually involved in STEM education to support students and they used lecturing strategy the most frequently, followed by problem-based learning. Automation was only applied in the lecturing instruction mode and educational robots were most frequently applied in the problem-based learning mode. Third, a majority of AI techniques (except educational robots) were applied in higher education with medium and large scale of learners. The most frequently used AI in higher education were learning prediction and ITSs. Fourth, computer system resource was the most frequently used medium to convey knowledge, particularly when it was applied in ITSs and automation, while paper, mobile phone, and E-book resources were seldom used in AI-STEM research. Fifth, the face-to-face environment was mainly utilized to support all categories of AI applications, and web-based environment was most frequently used supported with ITSs.
Regarding the third question, this review summarized the educational and technological effects and findings of AI applications in STEM. From the educational perspective, the results showed that most of the AI applications had positive effects on students’ academic performance. However, insignificant improvements of learning outcomes were also found in two empirical studies (Hellings & Haelermans, 2020 ; Koć-Januchta et al., 2020 ). Moreover, most students held positive attitudes towards the use of AI technology in STEM education, and AI technologies aroused their interest and motivation as well. In other words, the AI applications are beneficial for fostering student’s active learning in STEM education. Moreover, the applications of AI techniques also contributed to the development of students’ higher-order thinking, e.g., computational thinking, problem-solving ability. In addition, AI techniques have great potential to assist instructors by detecting students’ learning patterns and behaviors in STEM education. From the technological perspective, the reviewed articles mainly reported a good efficiency and algorithm accuracy when applying AI in STEM education. Specifically, AI algorithms, especially ensemble machine learning methods, performed well in learning prediction, automation, and personalized recommendation. Overall, underpinned by the GST framework, this review presented an overview of recent trends of the field of AI-STEM, which guided the following educational, technological, and theoretical implications.
Educational implications
The emergence of AI indirectly influences the subject elements (e.g., instructor, learner) in STEM education, which in turn would eventually influence the educational practices and effects. First, AI has potential to transform the instructor–student relationships in STEM education from the instructor-directed to student-centered learning (Cviko et al., 2014 ). When AI is applied in STEM education, the role of instructor is expected to shift from a leader to a collaborator or a facilitator under the AI-empowered, learner-as-leader paradigm (Ouyang & Jiao, 2021 ). However, this review found that the instructor-centered lecturing mode was the most frequently used instructional strategy in AI-STEM studies, while other student-centered instructional strategies (e.g., the project-based learning, collaborative learning, game-based learning) appeared infrequently. One of the reasons centers on the complexity of integrating technology and pedagogy in STEM education (Castañeda & Selwyn, 2018 ; Jiao et al., 2022 ; Loveless, 2011 ). For example, ITS and automation techniques are usually designed based on behaviorism (Skinner, 1953 ) to support instructor’s knowledge delivery and exam evaluation, which may be challenging for instructors to use when integrating it in the student-centered instructional strategies. Recent research has started to balance pedagogical design and technological application in educational practices in order to achieve the goal of AI–instructor collaboration and student-centered learning when AI is integrated (Baker & Smith, 2019 ; Holmes et al., 2019 ; Roll & Wylie, 2016 ). Furthermore, another critical question is: would AI replace instructor responsibilities and roles in STEM education (Segal, 2019 )? In this review, we found that the role of instructor was still irreplaceable, because the instructor’s involvement existed in most of the AI-STEM research. Even though AI can free instructors from redundant tasks in STEM education, it still lacks the human ability to convey social emotion, solve critical problems, and implement creative activities (Collinson, 1996 ; Gary, 2019 ; Muhisn et al., 2019 ). Therefore, although AI techniques can bring opportunities to develop STEM education (Hwang et al., 2020 ), we cannot overstate the role of technology and overlook the essential role of instructor (Selwyn, 2016 ). Overall, instructors, as important subjects in the educational system, need to take agency to promote the pedagogical designs and strategies when applying AI technologies, in order to achieve a high quality of AI-STEM education (Cantú-Ortiz et al., 2020 ).
Technological implications
Although AI has the potential to enhance the instruction and learning in STEM education (Chen et al., 2020 ; Holmes et al., 2019 ), the development of AI-STEM requires a better fit between AI technologies and other system elements in STEM education. First, regarding the relationships between AI and information element, the results showed that most of the AI applications were used in science and technology learning contents, and educational robots and automation were not applied in engineering and mathematics learning contents. Since STEM education contains interdisciplinary knowledge and learning contents from different subjects, AI is usually restricted in specific learning contents or courses (Douce et al., 2005 ). Therefore, one of the future directions is to expand the commonality and accessibility of AI techniques in different STEM subjects and courses. Second, the range of AI applications was mainly located in higher education, while few of AI techniques were applied in other educational levels, especially in kindergarten. To some extent, due to the complex function and feedback mechanisms, most of the AI techniques (e.g., ITS, learning prediction) might be appropriate for adult learners. Hence, some interactive AI techniques, e.g., social robots, AI-enabled games, can be designed and developed to support young children’s STEM learning (Belpaeme et al., 2018 ; Zapata-Cáceres & Martin-Barroso, 2021 ). Therefore, the ease of use is also one of the important considerations in future development of AI technologies (Law, 2019 ; Xu & Ouyang, 2022 ). Third, most of the AI-STEM research was conducted through the traditional mediums (e.g., computer system resource) and contexts (e.g., face-to-face learning environment). A future direction is to create AI-empowered STEM learning environment through combining the advanced educational mediums (e.g., E-book) and contexts (AR/VR), in order to better represent and convey knowledge (Mystakidis et al., 2021 ).
Theoretical implications
Due to the complexity of AI-STEM system, this research used a theoretical framework based on GST to examine the multiple elements (i.e., AI technology, information, subject, medium, environment) in AI-STEM research. Compared to previous AIEd reviews that mainly focused on the technological perspective, GST provides a holistic view for us to consider the complex human, pedagogical, environment factors when applying AI in STEM education (Kitto, 2014 ; Von Bertalanffy, 1968 ). For example, we found that sometimes instructors did not engage in STEM education to support students, especially when applying ITSs and educational robots. It might reveal a new trend that some AI technologies (e.g., social robot, virtual agent) might have the potential to replace the original role of instructor and work as a new subject to individually convey knowledge (Xu & Ouyang, 2022 ). Additionally, the results showed the different characteristics of learner’s sample size when applying different AI techniques. Learning prediction was more likely to applied with a large scale of students, and educational robots were inclined to be applied with a small scale of students. The features of AI technologies might explain this phenomenon. For example, a data training process is necessary before learning prediction, which requires the support of algorithmic modeling techniques and large data sets (Agrawal & Mavani, 2015 ; Lee et al., 2017 ), while educational robots, as human–machine interaction technologies, seem more suitable and practicable for small-scale STEM learning (Atman Uslu et al., 2022 ; Belpaeme et al., 2018 ). Overall, the current study utilized the GST framework to examine the multiple elements in the complex AI-STEM system; it is suggested that different stakeholders, e.g., educators, technical developers, and researchers, can adopt the GST framework as a guide to comprehensively consider the complex elements when applying AI techniques in STEM education (Kitto, 2014 ; Von Bertalanffy, 1950 ).
Conclusion, limitation, and future direction
The application of AI technology in STEM education is an emerging trend, which is confronted with the challenge of integrating diverse AI techniques in the complex STEM educational system. Grounded upon a GST framework, this research reviewed the empirical AI-STEM studies from 2011 to 2021. Specifically, this systematic review examined (1) the categories of the AI element in the AI-STEM system; (2) the characteristics of other system elements (i.e., information, subject, medium, and environment) as well as the distribution of AI in these elements, and (3) the effects of AI in STEM education. Based on the results, the current work proposed educational, technological, and theoretical implications for future AI-STEM research, to better aid the educators, researchers and technical developers to integrate the AI techniques and STEM education.
There are three limitations in this systematic review, which lead to future research directions. First, although we searched the best-known scholar databases with the keywords relevant to AI-STEM, some biases might exist in the searching and screening process. Since AI-STEM is a highly technology-dependent field, some studies might only highlight the technology rather than the education context. Therefore, future studies can adjust the searching criteria to solve these problems. Second, from a system perspective, we used a GST framework to examine the multiple elements in the complex AI-STEM system, but we did not investigate the mutual relationships between elements. Therefore, the complex relationships between different elements (e.g., instructor–learner, learner–learner relationship) in AI-STEM system need to be further explored in order to gain a deep understanding of the application of AI in STEM education (e.g., Xu & Ouyang, 2022 ). Third, the current study only implemented a systematic review, a meta-analysis could be conducted in the future to report the effect sizes of recent empirical studies to gain a deeper understanding of the effects of the AI-STEM integration in an educational system. Overall, the potential of AI technology for enhancing STEM education is fertile ground to be further explored together with studies aimed at investigating the integration of technology and educational system.
Availability of data and materials
The data are available upon request from the corresponding author.
*Reviewed articles ( N = 63)
Agrawal, H., & Mavani, H. (2015). Student performance prediction using machine learning. International Journal of Engineering Research and Technology, 4 (03), 111–113. https://doi.org/10.17577/IJERTV4IS030127
Article Google Scholar
Alabdulhadi, A., & Faisal, M. (2021). Systematic literature review of STEM self-study related ITSs. Education and Information Technologies, 26 , 1549–1588. https://doi.org/10.1007/s10639-020-10315-z
*Aldabe, I., & Maritxalar, M. (2014). Semantic similarity measures for the generation of science tests in Basque. IEEE Transactions on Learning Technologies, 7 (4), 375–387. https://doi.org/10.1109/TLT.2014.2355831
*Alemán, J. L. F. (2011). Automated assessment in a programming tools course. IEEE Transactions on Education, 54 (4), 576–581. https://doi.org/10.1109/TE.2010.2098442
Atman Uslu, N., Yavuz, G. Ö., & KoçakUsluel, Y. (2022). A systematic review study on educational robotics and robots. Interactive Learning Environments . https://doi.org/10.1080/10494820.2021.2023890
*Azcona, D., Hsiao, I. H., & Smeaton, A. F. (2019). Detecting students-at-risk in computer programming classes with learning analytics from students’ digital footprints. User Modeling and User-Adapted Interaction, 29 (4), 759–788. https://doi.org/10.1007/s11257-019-09234-7
Baker, T., & Smith, L. (2019). Educ-AI-tion rebooted? Exploring the future of artificial intelligence in schools and colleges. https://media.nesta.org.uk/documents/Future_of_AI_and_education_v5_WEB.Pdf
*Balakrishnan, B. (2018). Motivating engineering students learning via monitoring in personalized learning environment with tagging system. Computer Applications in Engineering Education, 26 (3), 700–710. https://doi.org/10.1002/cae.21924
Belpaeme, T., Kennedy, J., Ramachandran, A., Scassellati, B., & Tanaka, F. (2018). Social robots for education: A review. Science Robotics, 3 (21), eaat5954. https://doi.org/10.1126/scirobotics.aat5954
*Berland, M., Davis, D., & Smith, C. P. (2015). AMOEBA: Designing for collaboration in computer science classrooms through live learning analytics. International Journal of Computer-Supported Collaborative Learning, 10 (4), 425–447. https://doi.org/10.1007/s11412-015-9217-z
*Bertolini, R., Finch, S. J., & Nehm, R. H. (2021). Testing the impact of novel assessment sources and machine learning methods on predictive outcome modeling in undergraduate biology. Journal of Science Education and Technology, 30 (2), 193–209. https://doi.org/10.1007/s10956-020-09888-8
*Blikstein, P., Worsley, M., Piech, C., Sahami, M., Cooper, S., & Koller, D. (2014). Programming pluralism: Using learning analytics to detect patterns in the learning of computer programming. Journal of the Learning Sciences, 23 (4), 561–599. https://doi.org/10.1080/10508406.2014.954750
*Buenaño-Fernández, D., Gil, D., & Luján-Mora, S. (2019). Application of machine learning in predicting performance for computer engineering students: A case study. Sustainability, 11 (10), 1–18. https://doi.org/10.3390/su11102833
Bybee, R. W. (2013). The case for STEM education: Challenges and opportunities . NSTA Press.
Google Scholar
Byrne, D., & Callaghan, G. (2014). Complexity theory and the social sciences . Routledge.
Cantú-Ortiz, F. J., Galeano Sánchez, N., Garrido, L., Terashima-Marin, H., & Brena, R. F. (2020). An artificial intelligence educational strategy for the digital transformation. International Journal on Interactive Design and Manufacturing, 14 (4), 1195–1209. https://doi.org/10.1007/s12008-020-00702-8
*Cao, X., Li, Z., & Zhang, R. (2021). Analysis on academic benchmark design and teaching method improvement under artificial intelligence robot technology. International Journal of Emerging Technologies in Learning, 16 (5), 58–72. https://doi.org/10.3991/ijet.v16i05.20295
Castañeda, L., & Selwyn, N. (2018). More than tools? Making sense of the ongoing digitizations of higher education. International Journal of Educational Technology in Higher Education, 15 (1), 1–10. https://doi.org/10.1186/s41239-018-0109-y
Chen, D., & Stroup, W. (1993). General system theory: Toward a conceptual framework for science and technology education for all. Journal of Science Education and Technology, 2 (3), 447–459. https://doi.org/10.1007/BF00694427
Chen, L., Chen, P., & Lin, Z. (2020). Artificial intelligence in education: A review. IEEE Access, 8 , 75264–75278. https://doi.org/10.1109/ACCESS.2020.2988510
*Chrysafiadi, K., & Virvou, M. (2013). PeRSIVA: An empirical evaluation method of a student model of an intelligent e-learning environment for computer programming. Computers & Education, 68 , 322–333. https://doi.org/10.1016/j.compedu.2013.05.020
*Çınar, A., Ince, E., Gezer, M., & Yılmaz, Ö. (2020). Machine learning algorithm for grading open-ended physics questions in Turkish. Education and Information Technologies, 25 , 3821–3844. https://doi.org/10.1007/s10639-020-10128-0
Cohen, L., Manion, L., & Morrison, K. (2005). Research methods in education . Routledge Falmer.
Collinson, V. (1996). Reaching students: Teachers ways of knowing . Corwin Press.
Crawford, J. L. (1974). A systems approach model for the application of general systems theory principles to education [Doctoral dissertation, University of Houston]. The University of Houston Institutional Repository. https://hdl.handle.net/10657/10661
Cviko, A., McKenney, S., & Voogt, J. (2014). Teacher roles in designing technology-rich learning activities for early literacy: A cross-case analysis. Computers & Education, 72 , 68–79. https://doi.org/10.1016/j.compedu.2013.10.014
*De-Marcos, L., Garcia-Cabot, A., Garcia-Lopez, E., & Medina, J. A. (2015). Parliamentary optimization to build personalized learning paths: Case study in web engineering curriculum. International Journal of Engineering Education, 31 (4), 1092–1105.
*Deo, R. C., Yaseen, Z. M., Al-Ansari, N., Nguyen-Huy, T., Langlands, T. A. M., & Galligan, L. (2020). Modern artificial intelligence model development for undergraduate student performance prediction: An investigation on engineering mathematics courses. IEEE Access, 8 , 136697–136724. https://doi.org/10.1109/ACCESS.2020.3010938
Douce, C., Livingstone, D., & Orwell, J. (2005). Automatic test-based assessment of programming: A review. Journal on Educational Resources in Computing, 5 (3), 4-es. https://doi.org/10.1145/1163405.1163409
Drack, M., & Pouvreau, D. (2015). On the history of Ludwig von Bertalanffy’s “general systemology”, and on its relationship to cybernetics—Part III: Convergences and divergences. International Journal of General Systems, 44 (5), 523–571. https://doi.org/10.1080/03081079.2014.1000642
Drigas, A. S., & Ioannidou, R. E. (2012). Artificial intelligence in special education: A decade review. International Journal of Engineering Education, 28 (6), 1366. http://imm.demokritos.gr/publications/AI_IJEE.pdf
*Ferrarelli, P., & Iocchi, L. (2021). Learning Newtonian physics through programming robot experiments. Technology, Knowledge and Learning, 26 , 789–824. https://doi.org/10.1007/s10758-021-09508-3
*Figueiredo, M., Esteves, L., Neves, J., & Vicente, H. (2016). A data mining approach to study the impact of the methodology followed in chemistry lab classes on the weight attributed by the students to the lab work on learning and motivation. Chemistry Education Research and Practice, 17 (1), 156–171. https://doi.org/10.1039/c5rp00144g
*García-Gorrostieta, J. M., López-López, A., & González-López, S. (2018). Automatic argument assessment of final project reports of computer engineering students. Computer Applications in Engineering Education, 26 (5), 1217–1226. https://doi.org/10.1002/cae.21996
Gary, K. (2019). Pragmatic standards versus saturated phenomenon: Cultivating a love of learning. Journal of Philosophy of Education, 53 (3), 477–490. https://doi.org/10.1111/1467-9752.12377
*Gavrilović, N., Arsić, A., Domazet, D., & Mishra, A. (2018). Algorithm for adaptive learning process and improving learners’ skills in Java programming language. Computer Applications in Engineering Education, 26 (5), 1362–1382. https://doi.org/10.1002/cae.22043
Graneheim, U. H., & Lundman, B. (2004). Qualitative content analysis in nursing research: Concepts, procedures and measures to achieve trustworthiness. Nurse Education Today, 24 (2), 105–112. https://doi.org/10.1016/j.nedt.2003.10.001
Guan, C., Mou, J., & Jiang, Z. (2020). Artificial intelligence innovation in education: A twenty-year data-driven historical analysis. International Journal of Innovation Studies, 4 (4), 134–147. https://doi.org/10.1016/j.ijis.2020.09.001
*Hellings, J., & Haelermans, C. (2020). The effect of providing learning analytics on student behaviour and performance in programming: A randomised controlled experiment. Higher Education, 83 (1), 1–18. https://doi.org/10.1007/s10734-020-00560-z
Holmes, W., Bialik, M., & Fadel, C. (2019). Artificial intelligence in education: Promises and implications for teaching and learning . Center for Curriculum Redesign.
Holstein, K., McLaren, B. M., & Aleven, V. (2019). Co-designing a real-time classroom orchestration tool to support teacher—AI complementarity. Journal of Learning Analytics, 6 (2), 27–52. https://doi.org/10.18608/jla.2019.62.3
*Hooshyar, D., Ahmad, R. B., Yousefi, M., Yusop, F. D., & Horng, S. (2015). A flowchart-based intelligent tutoring system for improving problem-solving skills of novice programmers. Journal of Computer Assisted Learning, 31 , 345–361. https://doi.org/10.1111/jcal.12099
*Hooshyar, D., Binti Ahmad, R., Wang, M., Yousefi, M., Fathi, M., & Lim, H. (2018). Development and evaluation of a game-based Bayesian intelligent tutoring system for teaching programming. Journal of Educational Computing Research, 56 (6), 775–801. https://doi.org/10.1177/0735633117731872
*Hsiao, I. H., Huang, P. K., & Murphy, H. (2020). Integrating programming learning analytics across physical and digital space. IEEE Transactions on Emerging Topics in Computing, 8 (1), 206–217. https://doi.org/10.1109/TETC.2017.2701201
Hwang, G. J., & Tu, Y. F. (2021). Roles and research trends of artificial intelligence in mathematics education: A bibliometric mapping analysis and systematic review. Mathematics, 9 (6), 584. https://doi.org/10.3390/math9060584
Hwang, G. J., Xie, H., Wah, B. W., & Gašević, D. (2020). Vision, challenges, roles and research issues of artificial intelligence in education. Computers and Education: Artificial Intelligence, 1 , 100001. https://doi.org/10.1016/j.caeai.2020.100001
*Ji, Y., & Han, Y. (2019). Monitoring indicators of the flipped classroom learning process based on data mining—Taking the course of “virtual reality technology” as an example. International Journal of Emerging Technologies in Learning, 14 (3), 166–176. https://doi.org/10.3991/ijet.v14i03.10105
Jiao, P., Ouyang, F., Zhang, Q., & Alavi, A. H. (2022). Artificial intelligence-enabled prediction model of student academic performance in online engineering education. Artificial Intelligence Review . https://doi.org/10.1007/s10462-022-10155-y
*Jones, A., Bull, S., & Castellano, G. (2018). “I know that now, I’ m going to learn this next” Promoting self-regulated learning with a robotic tutor. International Journal of Social Robotics, 10 (4), 439–454. https://doi.org/10.1007/s12369-017-0430-y
*Jones, A., & Castellano, G. (2018). Adaptive robotic tutors that support self-regulated learning: A longer-term investigation with primary school children. International Journal of Social Robotics, 10 (3), 357–370. https://doi.org/10.1007/s12369-017-0458-z
*Khan, I., Ahmad, A. R., Jabeur, N., & Mahdi, M. N. (2021). Machine learning prediction and recommendation framework to support introductory programming course. International Journal of Emerging Technologies in Learning, 16 (17), 42–59. https://doi.org/10.3991/ijet.v16i17.18995
Khandelwal, P., Srinivasan, K., & Roy, S. S. (2019). Surgical education using artificial intelligence, augmented reality and machine learning: A review. In A. Sengupta & P. Eng (Eds.), 2019 IEEE international conference on consumer electronics—Taiwan (pp. 1–2). IEEE.
*Kinnebrew, J. S., Killingsworth, S. S., Clark, D. B., Biswas, G., Sengupta, P., Minstrell, J., Martinez-garza, M., & Krinks, K. (2017). Contextual markup and mining in digital games for science learning: Connecting player behaviors to learning goals. IEEE Transactions on Learning Technologies, 10 (1), 93–103. https://doi.org/10.1109/TLT.2016.2521372
Kitto, K. (2014). A contextualised general systems theory. Systems, 2 (4), 541–565. https://doi.org/10.3390/systems2040541
*Koć-Januchta, M. M., Schönborn, K. J., Tibell, L. A. E., Chaudhri, V. K., & Heller, H. C. (2020). Engaging with biology by asking questions: Investigating students’ interaction and learning with an artificial intelligence-enriched textbook. Journal of Educational Computing Research, 58 (6), 1190–1224. https://doi.org/10.1177/0735633120921581
*Kose, U., & Arslan, A. (2017). Optimization of self-learning in computer engineering courses: An intelligent software system supported by artificial neural network and vortex optimization algorithm. Computer Applications in Engineering Education, 25 (1), 142–156. https://doi.org/10.1002/cae.21787
*Krämer, N. C., Karacora, B., Lucas, G., Dehghani, M., Rüther, G., & Gratch, J. (2016). Closing the gender gap in STEM with friendly male instructors? On the effects of rapport behavior and gender of a virtual agent in an instructional interaction. Computers & Education, 99 , 1–13. https://doi.org/10.1016/j.compedu.2016.04.002
Krasovskiy, D. (2020). The challenges and benefits of adopting AI in STEM education. https://upjourney.com/the-challenges-and-benefits-of-adopting-ai-in-stem-education
Krippendorff, K. (2004). Reliability in content analysis: Some common misconceptions and recommendations. Human Communication Research, 30 (3), 411–433. https://doi.org/10.1093/hcr/30.3.411
*Lacave, C., Molina, A. I., & Cruz-Lemus, J. A. (2018). Learning analytics to identify dropout factors of computer science studies through Bayesian networks. Behaviour and Information Technology, 37 (10–11), 993–1007. https://doi.org/10.1080/0144929X.2018.1485053
*Lamb, R., Hand, B., & Kavner, A. (2021). Computational modeling of the effects of the science writing heuristic on student critical thinking in science using machine learning. Journal of Science Education and Technology, 30 (2), 283–297. https://doi.org/10.1007/s10956-020-09871-3
Law, N. W. Y. (2019). Human development and augmented intelligence. In The 20th international conference on artificial intelligence in education (AIED 2019), Chicago, IL, USA.
Le, N. T., Strickroth, S., Gross, S., & Pinkwart, N. (2013). A review of AI-supported tutoring approaches for learning programming. In N. Nguyen, T. Van Do, & H. Le Thi (Eds.), Advanced computational methods for knowledge engineering (pp. 267–279). Springer. https://doi.org/10.1007/978-3-319-00293-4_20
Chapter Google Scholar
*Ledesma, E. F. R., & García, J. J. G. (2017). Selection of mathematical problems in accordance with student’s learning style. International Journal of Advanced Computer Science and Applications, 8 (3), 101–105. https://doi.org/10.14569/IJACSA.2017.080316
Lee, J., Jang, D., & Park, S. (2017). Deep learning-based corporate performance prediction model considering technical capability. Sustainability, 9 (6), 899. https://doi.org/10.3390/su9060899
Lee, J., Wu, A. S., Li, D., & Kulasegaram, K. M. (2021). Artificial intelligence in undergraduate medical education: A scoping review. Academic Medicine, 96 (11S), S62–S70. https://doi.org/10.1097/ACM.0000000000004291
Liang, J. C., Hwang, G. J., Chen, M. R. A., & Darmawansah, D. (2021). Roles and research foci of artificial intelligence in language education: An integrated bibliographic analysis and systematic review approach. Interactive Learning Environments . https://doi.org/10.1080/10494820.2021.1958348
*Lin, P. H., & Chen, S. Y. (2020). Design and evaluation of a deep learning recommendation based augmented reality system for teaching programming and computational thinking. IEEE Access, 8 , 45689–45699. https://doi.org/10.1109/ACCESS.2020.2977679
Liu, M., Li, Y., Xu, W., & Liu, L. (2017). Automated essay feedback generation and its impact on revision. IEEE Transactions on Learning Technologies, 10 (4), 502–513. https://doi.org/10.1109/TLT.2016.2612659
Loveless, A. (2011). Technology, pedagogy and education: Reflections on the accomplishment of what teachers know, do and believe in a digital age. Technology, Pedagogy and Education, 20 (3), 301–316. https://doi.org/10.1080/1475939X.2011.610931
*Maestrales, S., Zhai, X., Touitou, I., Baker, Q., Schneider, B., & Krajcik, J. (2021). Using machine learning to score multi-dimensional assessments of chemistry and physics. Journal of Science Education and Technology, 30 (2), 239–254. https://doi.org/10.1007/s10956-020-09895-9
*Mahboob, K., Ali, S. A., & Laila, U. E. (2020). Investigating learning outcomes in engineering education with data mining. Computer Applications in Engineering Education, 28 (6), 1652–1670. https://doi.org/10.1002/cae.22345
*Matthew, F. T., Adepoju, A. I., Ayodele, O., Olumide, O., Olatayo, O., Adebimpe, E., Bolaji, O., & Funmilola, E. (2018). Development of mobile-interfaced machine learning-based predictive models for improving students’ performance in programming courses. International Journal of Advanced Computer Science and Applications, 9 (5), 105–115. https://doi.org/10.14569/IJACSA.2018.090514
McLaren, B. M., Scheuer, O., & Mikšátko, J. (2010). Supporting collaborative learning and e-discussions using artificial intelligence techniques. International Journal of Artificial Intelligence in Education, 20 (1), 1–46. https://doi.org/10.3233/JAI-2010-0001
McLurkin, J., Rykowski, J., John, M., Kaseman, Q., & Lynch, A. J. (2013). Using multi-robot systems for engineering education: Teaching and outreach with large numbers of an advanced, low-cost robot. IEEE Transactions on Education, 56 (1), 24-33. https://doi.org/10.1109/TE.2012.2222646
Moher, D., Liberati, A., Tetzlaff, J., Altman, D. G., Prisma Group. (2009). Preferred reporting items for systematic reviews and meta-analyses: The PRISMA statement. PLoS Medicine, 6 (7), e1000097. https://doi.org/10.1371/journal.pmed.1000097.t001
Muhisn, Z. A. A., Ahmad, M., Omar, M., & Muhisn, S. A. (2019). The Impact of socialization on collaborative learning method in e-learning management system (eLMS). International Journal of Emerging Technologies in Learning, 14 (20), 137–148.
Murray, T. (2003). An overview of intelligent tutoring system authoring tools: Updated analysis of the state of the art. In T. Murray, S. B. Blessing, & S. Ainsworth (Eds.), Authoring tools for advanced technology learning environments (pp. 491–544). Springer. https://doi.org/10.1007/978-94-017-0819-7_17
*Myneni, L. S., Narayanan, N. H., & Rebello, S. (2013). An interactive and intelligent learning system for physics education. IEEE Transactions on Learning Technologies, 6 (3), 228–239. https://doi.org/10.1109/TLT.2013.26
Mystakidis, S., Christopoulos, A., & Pellas, N. (2021). A systematic mapping review of augmented reality applications to support STEM learning in higher education. Education and Information Technologies, 27 , 1883–1927. https://doi.org/10.1007/S10639-021-10682-1/FIGURES/10
*Nehm, R. H., Ha, M., & Mayfield, E. (2012). Transforming biology assessment with machine learning: Automated scoring of written evolutionary explanations. Journal of Science Education and Technology, 21 (1), 183–196. https://doi.org/10.1007/s10956-011-9300-9
Ouyang, F., & Jiao, P. (2021). Artificial intelligence in education: The three paradigms. Computers and Education: Artificial Intelligence, 2 , 100020. https://doi.org/10.1016/j.caeai.2021.100020
Ouyang, F., Zheng, L., & Jiao, P. (2022). Artificial intelligence in online higher education: A systematic review of empirical research from 2011 to 2020. Education and Information Technologies . https://doi.org/10.1007/s10639-022-10925-9
*Pereira, F. D., Oliveira, E. H. T., Oliveira, D. B. F., Cristea, A. I., Carvalho, L. S. G., Fonseca, S. C., Toda, A., & Isotani, S. (2020). Using learning analytics in the Amazonas: Understanding students’ behaviour in introductory programming. British Journal of Educational Technology, 51 (4), 955–972. https://doi.org/10.1111/bjet.12953
Pimthong, P., & Williams, J. (2018). Preservice teachers’ understanding of STEM education. Kasetsart Journal of Social Sciences, 41 (2), 1–7. https://doi.org/10.1016/j.kjss.2018.07.017
Rapoport, A. (1986). General system theory: Essential concepts & applications . CRC Press.
*Rodríguez Corral, J. M., Morgado-Estévez, A., Cabrera Molina, D., Pérez-Peña, F., Amaya Rodríguez, C. A., & CivitBalcells, A. (2016). Application of robot programming to the teaching of object-oriented computer languages. International Journal of Engineering Education, 32 (4), 1823–1832.
Roll, I., & Wylie, R. (2016). Evolution and revolution in artificial intelligence in education. International Journal of Artificial Intelligence in Education, 26 (2), 582–599. https://doi.org/10.1007/s40593-016-0110-3
*Saito, T., & Watanobe, Y. (2020). Learning path recommendation system for programming education based on neural networks. International Journal of Distance Education Technologies, 18 (1), 36–64. https://doi.org/10.4018/IJDET.2020010103
*Sapounidis, T., Stamovlasis, D., & Demetriadis, S. (2019). Latent class modeling of children’s preference profiles on tangible and graphical robot programming. IEEE Transactions on Education, 62 (2), 127–133. https://doi.org/10.1109/TE.2018.2876363
Segal, M. (2019). A more human approach to artificial intelligence. Nature, 571 (7766), S18–S18. https://doi.org/10.1038/d41586-019-02213-3
Selwyn, N. (2016). Is technology good for education? Polity Press.
Skinner, B. F. (1953). Science and human behavior . Macmillan.
*Spikol, D., Ruffaldi, E., Dabisias, G., & Cukurova, M. (2018). Supervised machine learning in multimodal learning analytics for estimating success in project-based learning. Journal of Computer Assisted Learning, 34 (4), 366–377. https://doi.org/10.1111/jcal.12263
*Suh, S. C., Anusha Upadhyaya, B. N., & Ashwin Nadig, N. V. (2019). Analyzing personality traits and external factors for stem education awareness using machine learning. International Journal of Advanced Computer Science and Applications, 10 (5), 1–4. https://doi.org/10.14569/ijacsa.2019.0100501
Tang, K. Y., Chang, C. Y., & Hwang, G. J. (2021). Trends in artificial intelligence supported e-learning: A systematic review and co-citation network analysis (1998–2019). Interactive Learning Environments . https://doi.org/10.1080/10494820.2021.1875001
*Tehlan, K., Chakraverty, S., Chakraborty, P., & Khapra, S. (2020). A genetic algorithm-based approach for making pairs and assigning exercises in a programming course. Computer Applications in Engineering Education, 28 (6), 1708–1721. https://doi.org/10.1002/cae.22349
*Thai, K. P., Bang, H. J., & Li, L. (2021). Accelerating early math learning with research-based personalized learning games: A cluster randomized controlled trial. Journal of Research on Educational Effectiveness, 15 (1), 28–51. https://doi.org/10.1080/19345747.2021.1969710
*Troussas, C., Krouska, A., & Sgouropoulou, C. (2021). A novel teaching strategy through adaptive learning activities for computer programming. IEEE Transactions on Education, 64 (2), 103–109. https://doi.org/10.1109/TE.2020.3012744
*Tüfekçi, A., & Köse, U. (2013). Development of an artificial intelligence based software system on teaching computer programming and evaluation of the system. Hacettepe Üniversitesi Eğitim Fakültesi Dergisi, 28 (2), 469–481.
*Verner, I. M., Cuperman, D., Gamer, S., & Polishuk, A. (2020). Exploring affordances of robot manipulators in an introductory engineering course. International Journal of Engineering Education, 36 (5), 1691–1707.
Von Bertalanffy, L. (1950). An outline of general system theory. British Journal for the Philosophy of Science, 1 , 134–165. https://doi.org/10.1093/bjps/I.2.134
Von Bertalanffy, L. (1968). General system theory: Foundations, development, applications . George Braziller.
*Vyas, V. S., Kemp, B., & Reid, S. A. (2021). Zeroing in on the best early-course metrics to identify at-risk students in general chemistry: An adaptive learning pre-assessment vs. traditional diagnostic exam. International Journal of Science Education, 43 (4), 552–569. https://doi.org/10.1080/09500693.2021.1874071
Walker, E., Rummel, N., & Koedinger, K. R. (2014). Adaptive intelligent support to improve peer tutoring in algebra. International Journal of Artificial Intelligence in Education, 24 (1), 33–61. https://doi.org/10.1007/s40593-013-0001-9
*Wang, T., Su, X., Ma, P., Wang, Y., & Wang, K. (2011). Ability-training-oriented automated assessment in introductory programming course. Computers & Education, 56 (1), 220–226. https://doi.org/10.1016/j.compedu.2010.08.003
*Wang, X. (2016). Course-taking patterns of community college students beginning in STEM: Using data mining techniques to reveal viable STEM transfer pathways. Research in Higher Education, 57 (5), 544–569. https://doi.org/10.1007/s11162-015-9397-4
Wohlin, C. (2014). Guidelines for snowballing in systematic literature studies and a replication in software engineering. In C. Wohlin (Ed.), Proceedings of the 18th international conference on evaluation and assessment in software engineering (pp. 1–10). ACM Press. https://doi.org/10.1145/2601248.2601268
*Wu, P., Hwang, G., & Tsai, W. (2013). An expert system-based context-aware ubiquitous learning approach for conducting science learning activities. Journal of Educational Technology & Society, 16 (4), 217–230.
*Xing, W., Pei, B., Li, S., Chen, G., & Xie, C. (2019). Using learning analytics to support students’ engineering design: The angle of prediction. Interactive Learning Environments . https://doi.org/10.1080/10494820.2019.1680391
Xu, W., & Ouyang, F. (2022). A systematic review of AI role in the educational system based on a proposed conceptual framework. Education and Information Technologies, 27 , 4195–4223. https://doi.org/10.1007/s10639-021-10774-y
*Yahya, A. A., & Osman, A. (2019). A data-mining-based approach to informed decision-making in engineering education. Computer Applications in Engineering Education, 27 (6), 1402–1418. https://doi.org/10.1002/cae.22158
*Yang, J., Devore, S., Hewagallage, D., Miller, P., Ryan, Q. X., & Stewart, J. (2020). Using machine learning to identify the most at-risk students in physics classes. Physical Review Physics Education Research, 16 (2), 20130. https://doi.org/10.1103/PhysRevPhysEducRes.16.020130
Yang, J., & Zhang, B. (2019). Artificial intelligence in intelligent tutoring robots: A systematic review and design guidelines. Applied Sciences, 9 (10), 2078. https://doi.org/10.3390/app9102078
*Yannier, N., Hudson, S. E., & Koedinger, K. R. (2020). Active learning is about more than hands-on: A mixed-reality AI system to support STEM education. International Journal of Artificial Intelligence in Education, 30 (1), 74–96. https://doi.org/10.1007/s40593-020-00194-3
*Yu, Y. (2017). Teaching with a dual-channel classroom feedback system in the digital classroom environment. IEEE Transactions on Learning Technologies, 10 (3), 391–402. https://doi.org/10.1109/TLT.2016.2598167
*Zabriskie, C., Yang, J., Devore, S., & Stewart, J. (2019). Using machine learning to predict physics course outcomes. Physical Review Physics Education Research, 15 (2), 20120. https://doi.org/10.1103/PhysRevPhysEducRes.15.020120
*Zampirolli, F. A., BorovinaJosko, J. M., Venero, M. L. F., Kobayashi, G., Fraga, F. J., Goya, D., & Savegnago, H. R. (2021). An experience of automated assessment in a large-scale introduction programming course. Computer Applications in Engineering Education, 29 (5), 1284–1299. https://doi.org/10.1002/cae.22385
*Zapata-Cáceres, M., & Martin-Barroso, E. (2021). Applying game learning analytics to a voluntary video game: Intrinsic motivation, persistence, and rewards in learning to program at an early age. IEEE Access, 9 , 123588–123602. https://doi.org/10.1109/ACCESS.2021.3110475
Zawacki-Richter, O., Marín, V. I., Bond, M., & Gouverneur, F. (2019). Systematic review of research on artificial intelligence applications in higher education—Where are the educators? International Journal of Educational Technology in Higher Education, 16 (39), 1–27. https://doi.org/10.1186/s41239-019-0171-0
*Zhang, Z., Liu, H., Shu, J., Nie, H., & Xiong, N. (2020). On automatic recommender algorithm with regularized convolutional neural network and IR technology in the self-regulated learning process. Infrared Physics and Technology, 105 , 103211. https://doi.org/10.1016/j.infrared.2020.103211
Zheng, R., Jiang, F., & Shen, R. (2020). Intelligent student behavior analysis system for real classrooms. In P. C. Center (Ed.), 2020 IEEE international conference on acoustics, speech and signal processing (pp. 9244–9248). IEEE.
*Zulfiani, Z., Suwarna, I. P., & Miranto, S. (2018). Science education adaptive learning system as a computer-based science learning with learning style variations. Journal of Baltic Science Education, 17 (4), 711–727. https://doi.org/10.33225/jbse/18.17.711
Zupic, I., & Čater, T. (2015). Bibliometric methods in management and organization. Organizational Research Methods, 18 (3), 429–472. https://doi.org/10.1177/1094428114562629
Download references
Acknowledgements
The authors would like to thank Luyi Zheng for her help on preliminary data analysis.
This work was supported by National Natural Science Foundation of China, No. 62177041.
Author information
Authors and affiliations.
College of Education, Zhejiang University, #866, Yuhangtang Rd., Hangzhou, 310058, Zhejiang, China
Weiqi Xu & Fan Ouyang
You can also search for this author in PubMed Google Scholar
Contributions
WX conducted data collection and analysis as well as writing of the first draft of this manuscript. FO designed the research, designed and facilitated data analysis and revised the manuscript. Both authors read and approved the final manuscript.
Corresponding author
Correspondence to Fan Ouyang .
Ethics declarations
Competing interests.
The authors declare that they have no competing interests.
Additional information
Publisher's note.
Springer Nature remains neutral with regard to jurisdictional claims in published maps and institutional affiliations.
Rights and permissions
Open Access This article is licensed under a Creative Commons Attribution 4.0 International License, which permits use, sharing, adaptation, distribution and reproduction in any medium or format, as long as you give appropriate credit to the original author(s) and the source, provide a link to the Creative Commons licence, and indicate if changes were made. The images or other third party material in this article are included in the article's Creative Commons licence, unless indicated otherwise in a credit line to the material. If material is not included in the article's Creative Commons licence and your intended use is not permitted by statutory regulation or exceeds the permitted use, you will need to obtain permission directly from the copyright holder. To view a copy of this licence, visit http://creativecommons.org/licenses/by/4.0/ .
Reprints and permissions
About this article
Cite this article.
Xu, W., Ouyang, F. The application of AI technologies in STEM education: a systematic review from 2011 to 2021. IJ STEM Ed 9 , 59 (2022). https://doi.org/10.1186/s40594-022-00377-5
Download citation
Received : 11 June 2022
Accepted : 09 September 2022
Published : 19 September 2022
DOI : https://doi.org/10.1186/s40594-022-00377-5
Share this article
Anyone you share the following link with will be able to read this content:
Sorry, a shareable link is not currently available for this article.
Provided by the Springer Nature SharedIt content-sharing initiative
- Artificial intelligence
- Artificial intelligence in education
- STEM education
- General system theory
- Educational system
ARTIFICIAL INTELLIGENCE AT NORTHWESTERN
- Research & Applications
Education in AI Theory, Practice, and Impact
Northwestern’s core purpose as an educational institution is evident in the artificial intelligence (AI) curriculum available to learners at many levels, from undergraduates to executive and professional education. Explore the programs and variety of focus areas in the Education section .
Northwestern researchers are also looking to develop new learning methods and pathways utilizing artificial intelligence applications, and to provide opportunities for students to apply and explore their AI skillsets.
View faculty who work in this area
Featured Research
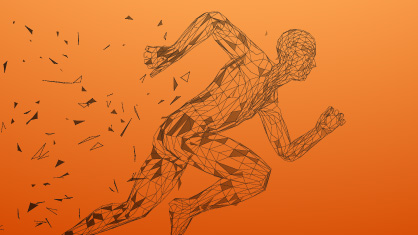
Segueing Students' Interest in Sports into an Interest in STEM and AI
Learn more about the Data in Motion project that helps students make connections between sports and technology and data analytics, showing them ways they can improve on field through their simultaneous understanding of STEM and CS concepts.
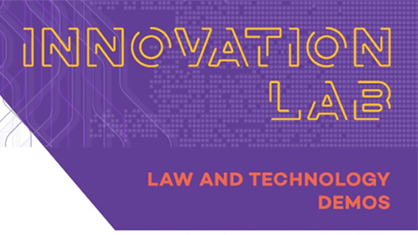
Connecting Computer Science and Law
The CS+Law Innovation Lab class brings together computer science and law students to explore real-world legal services delivery problems.
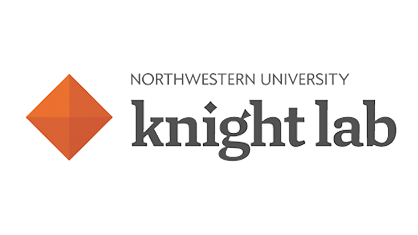
Connecting Computer Science and Journalism
The Knight Lab is a community of designers, developers, students, and educators working on experiments designed to push journalism into new spaces.
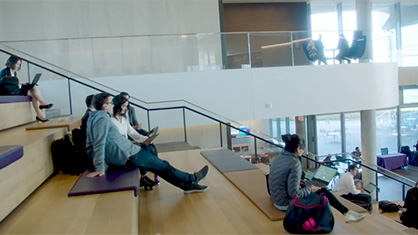
Training Business Leaders to Harness the Power of AI
Learn about how the Kellogg School of Management is incorporating AI into their curriculum to prepare future leaders to manage teams that will harness these powerful technologies.
Watch the video
Advertisement
The Intertwined Histories of Artificial Intelligence and Education
- Open access
- Published: 04 October 2022
- Volume 33 , pages 885–928, ( 2023 )
Cite this article
You have full access to this open access article
- Shayan Doroudi ORCID: orcid.org/0000-0002-0602-1406 1
16k Accesses
13 Citations
23 Altmetric
Explore all metrics
In this paper, I argue that the fields of artificial intelligence (AI) and education have been deeply intertwined since the early days of AI. Specifically, I show that many of the early pioneers of AI were cognitive scientists who also made pioneering and impactful contributions to the field of education. These researchers saw AI as a tool for thinking about human learning and used their understanding of how people learn to further AI. Furthermore, I trace two distinct approaches to thinking about cognition and learning that pervade the early histories of AI and education. Despite their differences, researchers from both strands were united in their quest to simultaneously understand and improve human and machine cognition. Today, this perspective is neither prevalent in AI nor the learning sciences. I conclude with some thoughts on how the artificial intelligence in education and learning sciences communities might reinvigorate this lost perspective.
Similar content being viewed by others
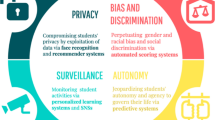
Artificial intelligence in education: Addressing ethical challenges in K-12 settings
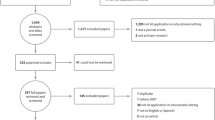
Systematic review of research on artificial intelligence applications in higher education – where are the educators?
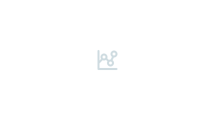
Evolution and Revolution in Artificial Intelligence in Education
Avoid common mistakes on your manuscript.
Before we embark on the substance of this essay, it is worthwhile to clarify a potential source of confusion. For many, AI is identified as a narrowly focused field directed toward the goal of programming computers in such a fashion that they acquire the appearance of intelligence. Thus it may seem paradoxical that researchers in the field have anything to say about the structure of human language or related issues in education. However, the above description is misleading. It correctly delineates the major methodology of the science, that is, the use of computers to build precise models of cognitive theories. But it mistakenly identifies this as the only purpose of the field. Although there is much practical good that can come of more intelligent machines, the fundamental theoretical goal of the discipline is understanding intelligent processes independent of their particular physical realization. (Goldstein & Papert, 1977 , pp. 84–85)
Over the past few decades, there have been numerous advances in applying artificial intelligence (AI) to educational problems. As such, when people think of the intersection of artificial intelligence and education, what likely comes to mind are the applications of AI to enhancing education (e.g., intelligent tutoring systems, automated essay scoring, and learning analytics). Indeed, this is the focus of the International Artificial Intelligence in Education Society: “It promotes rigorous research and development of interactive and adaptive learning environments for learners of all ages, across all domains” (International Artificial Intelligence in Education Society, n.d. ). In this paper, I show that historically, artificial intelligence and education have been intertwined in more principled and mutually reinforcing ways than thinking of education as just another application area of artificial intelligence would suggest.
My goal is by no means to present a complete history of the field of artificial intelligence or the field of education research. I also do not intend to provide a detailed history of the field of artificial intelligence in education (AIED). Rather, my goal is to present a narrative of how the two fields of artificial intelligence and education had intertwined histories since the 1960s, and how important figures in the development of artificial intelligence also played a significant role in the history of education research. Footnote 1 I primarily focus on some of the leading researchers in the early history of AI (1950s-1970s) in the United States and (to a lesser extent) the United Kingdom.
The focus on early pioneers in the field is to show that the very development of the field was intertwined with education research. As such, this means that I do not focus on many important leaders of the field of AIED as they are not typically recognized as major figures in the early history of AI at large; however, the history I speak to does intersect with the development of AIED, as described below. It also means this history focuses primarily on White male researchers. This is largely an artifact of who the active researchers were in the field of AI (and academic research, more broadly) at the time. It is important to acknowledge that many of these researchers worked with women and non-White researchers who may not be as well-recognized today, and that the diversity of researchers working in these areas has naturally increased over the years. Moreover, most of the early work in “sanctioned” AI history Footnote 2 was happening in the US and the UK, although there were likely AI pioneers elsewhere in the world. An exploration of whether researchers in other countries in the early days of AI were also exploring the mutual interplay between AI and education would be an interesting area of further research.
Although glimpses of this story are told in the histories of individual fields, to my knowledge, the intertwined histories of these two fields have never been fully documented previously. For example, in his insightful chapter, “A Short History of the Learning Sciences,” Lee ( 2017 ) highlights that the early days of the learning sciences had roots in artificial intelligence and cognitive science:
The so-called “cognitive revolution” led to interdisciplinary work among researchers to build new models of human knowledge. The models would enable advances in the development of artificial intelligence technologies, meaning that problem solving, text comprehension, and natural language processing figured prominently. The concern in the artificial intelligence community was on the workings of the human mind, not immediately on issues of training or education.
It is easy to read the above as suggesting that AI provided tools that were later applied by others to educational problems. While to some extent it is true that the “the concern in the artificial intelligence community was…not immediately on issues on issues of training or education,” the narrative I present below suggests that many AI pioneers were committed to advancing education. In another chapter that is also called “A Short History of the Learning Sciences,” Hoadley ( 2018 )—who, as an undergraduate, worked with AI pioneer Seymour Papert—makes only brief mention of how the birth of computing, AI, and cognitive science were some of the many seeds for the learning sciences. Moreover, Pea ( 2016 ) in his “Prehistory of the Learning Sciences,” focused on specific people and events that led to the formation of the learning sciences, but did not explicitly mention the role that artificial intelligence played at all, aside from passing mentions of the Artificial Intelligence in Education community. In her seminal history of education research, Lagemann ( 2002 ) dedicates a few pages to discussing the rise of cognitive science and as such, mentions some of the pioneers discussed in this paper (mainly Simon and Newell), but she does not explicitly connect these figures to education research. Footnote 3
Histories of artificial intelligence fare no better. Nilsson’s ( 2009 ) 700-page book on the history of AI only makes a couple of passing remarks about how education intersected with that history. Pamela McCorduck’s humanistic account of the history of AI mostly only discusses education in the context of Papert’s work with Logo in a chapter called “Applied Artificial Intelligence” (McCorduck, 2004 ). Interestingly enough, even Howard Gardner, a prominent education researcher, makes almost no mention of education in his book on the history of cognitive science (Gardner, 1987 ).
The learning sciences and artificial intelligence are both fairly new fields, having only emerged a few decades ago. Therefore, much of the history presented in this paper is still held in the individual and collective memories of individuals who either played a role in this history or who witnessed its unfolding. As such, it might seem odd that someone who was not alive for most of this history should be one to write it. Nonetheless, perhaps the story will be slightly less biased if it comes from someone who was not involved in it and who had to reconstruct this story from primary sources. Indeed, much of what is narrated here might be “obvious” to earlier generations of researchers in artificial intelligence or education, and as such, these researchers might face expert blind spots (Nathan et al., 2001 ) in constructing this narrative. If my own experience as a novice at the intersection of these two fields is telling, this rich history is not obvious to novices entering these fields. As time passes, if this history goes unwritten and untaught, I think what is obvious to current experts may be lost to the next generation of researchers in the fields of artificial intelligence, education, and AIED.
To construct this historical narrative, I used a combination of publications from the key figures involved, unpublished grey literature, historical sources, and archival material, especially from the Herbert Simon and Allen Newell Digital Collections. The paper alternates between sections focused on specific AI pioneers—describing their work in both AI and education—and sections focused on the formation of fields or subdivisions within fields that are relevant to this history. The sections on AI pioneers begin by describing their overall approach to AI research and end by discussing their direct and indirect contributions to education. The sections on fields discuss broader trends in the histories of AI and education that move beyond the specific pioneers. The majority of the paper spans work covering the 1950s-1990s. In the final section, I discuss where the relevant fields have headed since the 1990s, how the ethos present in earlier days of AI and the learning sciences has seemingly disappeared, and what we might do about that.
Overall, the historical narrative presented in this paper arrives at two overarching claims:
Early artificial intelligence pioneers were cognitive scientists who were united in the broad goal of understanding thinking and learning in machines and humans, and as such were also invested in research on education. The point is not just that they were cognitive scientists whose work had implications for education, but rather that these researchers were also at times directly involved in education research and had a significant impact on the course of education research. In this sense, such researchers differ from most AI researchers and most learning scientists today.
There were largely two different (and, at times, opposing) approaches, which manifested in various ways in both the history of AI and the history of education research.
The second claim was also made by me in another article (Doroudi, 2020 ), where I claimed that there is a “bias-variance tradeoff” (a concept drawn from machine learning) between different approaches in education research. That article drew on similar examples from the histories of AI and education to make this point. However, the present paper puts such claims in a broader historical context and more clearly describes how the “two camps” have evolved over time. Moreover, by juxtaposing the two aforementioned overarching claims, the overall picture that emerges is one in which early researchers who took different approaches in AI and education were at once united, despite their differences. The hope is that understanding and charting these historical trends can help make sense of and possibly repair ongoing fault lines in the learning sciences today, and perhaps reinvigorate this lost perspective of synergistically thinking about AI and education.
Simon and Newell: From Logic Theorist to LISP Tutor
In 1956, a workshop was held at Dartmouth College by the name of “Dartmouth Summer Research Project on Artificial Intelligence.” This event, organized by John McCarthy, along with Marvin Minsky, Nathaniel Rochester, and Claude Shannon, is widely regarded as the origin of artificial intelligence and the event that gave the field its name. Gardner ( 1987 ) singles out four of the workshop attendees—Herbert Simon, Allen Newell, Minsky, and McCarthy—as the “Dartmouth Tetrad” for their subsequent work in defining the field of artificial intelligence. After the formation of the American Association of Artificial Intelligence in 1979, Newell, Minsky, and McCarthy would all serve as three of its first five presidents.
The story I present here will begin with the work of three of the Dartmouth Tetrad (Simon, Newell, and Minsky), along with their colleagues and students. In this section, I begin by briefly describing the early pioneering work of Simon and Newell in the fields of artificial intelligence and psychology, and then discuss their contributions to education and how it related to their work in AI.
An Information-Processing Approach to AI
While the Dartmouth Workshop was a seminal event in the formation of AI, the first AI programs were being developed prior to the workshop. In 1955, Simon and Newell, professors at Carnegie Institute of Technology (now Carnegie Mellon University), along with J. C. Shaw, created the Logic Theorist, a program capable of proving logical theorems from Russell and Whitehead’s Principia Mathematica (a foundational text in mathematical logic) by manipulating “symbol structures” (Nilsson, 2009 ). Footnote 4 Simon and Newell presented this program at the Dartmouth Workshop. Shortly thereafter, in a paper titled “Elements of a Theory of Human Problem Solving,” Newell et al. ( 1958 ) describe the Logic Theorist and its links to human problem solving:
The program of LT was not fashioned directly as a theory of human behavior; it was constructed in order to get a program that would prove theorems in logic. To be sure, in constructing it the authors were guided by a firm belief that a practicable program could be constructed only if it used many of the processes that humans use. (p. 154)
In this paper, the authors laid the foundations of information-processing psychology . In a follow-up paper, “Human Problem Solving: The State of the Theory in 1970,” Simon and Newell ( 1971 ) describe the theory of information-processing psychology and their strategy for developing it over 15 years. The first three steps of their strategy culminate in the development of an artificial intelligence program like the Logic Theorist:
3. Discover and define a program, written in the language of information processes, that is capable of solving some class of problems that humans find difficult. Use whatever evidence is available to incorporate in the program processes that resemble those used by humans. (Do not admit processes, like very rapid arithmetic, that humans are known to be incapable of; p. 146)
But this was not the final destination; the next step in Newell and Simon’s strategy was to actually collect human data:
4. If the first three steps are successful, obtain data, as detailed as possible, on human behavior in solving the same problems as those tackled by the program. Search for the similarities and differences between the behavior of program and human subject. Modify the program to achieve a better approximation to the human behavior. (p. 146)
The fourth step of their procedure was carried out with extensive “think-alouds” of experts solving a variety of problem solving tasks such as cryptarithmetic, logic, chess, and algebra word problems. They followed what Ericsson and Simon ( 1980 ) would later formalize as the think-aloud protocol, which has since become a popular method for eliciting insights into human behavior in the social sciences, including education research.
Much of the theory articulated in their paper was about how experts solve problems, but how does a human learn to solve problems? Simon and Newell ( 1971 ) postulated a theory for how people might come to develop a means of solving problems in terms of what they called production systems:
In a production system, each routine has a bipartite form, consisting of a condition and an action. The condition defines some test or set of tests to be performed on the knowledge state...If the test is satisfied, the action is executed; if the test is not satisfied, no action is taken, and control is transferred to some other production. (p. 156)
Learning then becomes a matter of gradually accumulating the various production rules necessary to solve a problem. The development and analysis of production systems subsequently became an important part of information-processing psychology (Newell, 1973 ).
Overall, this 1971 paper describes a program of research that simultaneously defined information-processing psychology, a major branch of cognitive psychology, as well as the symbolic approach to artificial intelligence that dominated the early days of the field. But this work also played a role in the development of educational theory and educational technology to the present day. At the end of their paper, Simon and Newell ( 1971 ) have a section on “The Practice of Education.” This short section of their paper is very insightful on the way that Simon and Newell conceived of their work’s impact on education. They motivated their work’s impact by calling on the need to develop a science of education:
The professions always live in an uneasy relation with the basic sciences that should nourish and be nourished by them. It is really only within the present century that medicine can be said to rest solidly on the foundation of deep knowledge in the biological sciences, or the practice of engineering on modern physics and chemistry. Perhaps we should plead the recency of the dependence in those fields in mitigation of the scandal of psychology’s meager contribution to education. (p. 158)
Simon and Newell ( 1971 ) then go on to explain how information-processing psychology could answer this call to improve educational practice:
The theory of problem solving described here gives us a new basis for attacking the psychology of education and the learning process. It allows us to describe in detail the information and programs that the skilled performer possesses, and to show how they permit him to perform successfully. But the greatest opportunities for bringing the theory to bear upon the practice of education will come as we move from a theory that explains the structure of human problem-solving programs to a theory that explains how these programs develop in the face of task requirements—the kind of theory we have been discussing in the previous sections of this article [i.e., production systems]. (p. 158)
However, Simon and Newell did not just leave it to others to apply information-processing psychology to advance education; they tried to directly advance education themselves.
Forgotten Pioneers in Education
In 1967, Newell and his student, James Moore, had actually worked on developing an intelligent tutoring system, Merlin, fittingly to teach graduate artificial intelligence (Moore & Newell, 1974 ). However, for Moore and Newell ( 1974 ), this was actually a much bigger undertaking than simply creating a tutoring system; they were trying to create a system that could understand:
The task was to make it easy to construct and play with simple, laboratory-sized instances of artificial intelligence programs. Because of our direct interest in artificial intelligence, the effort transmuted into one of building a program that would understand artificial intelligence—that would be able to explain and run programs, ask and answer questions about them, and so on, at some reasonable level. The intent was to tackle a real domain of knowledge as the area for constructing a system that understood. (pp. 201–202)
In 1970, in a workshop on education and computing, Newell gave an invited talk entitled “What are the Intellectual Operations required for a Meaningful Teaching Agent?” Referring to his work on Merlin, Newell ( 1970 ) outlined 12 aspects of intelligence that they found need to be embodied in a meaningful teaching agent. Newell mentioned that there were two routes to go about automating intelligent operations in a computer: (1) automating that which is currently easy “for immediate payoff, at the price of finding that the important operations have been left untouched,” or (2) identifying “the essential intellectual operations involved” and automating those “at the price of unknown and indefinite delays in application.” Newell had opted for the second approach.
According to Laird and Rosenbloom ( 1992 ), “Merlin contained many new ideas before they became popular in mainstream AI, such as attached procedures, general mapping, indefinite context dependence, and automatic compilation” (p. 31). However, after six or so years of work, Merlin was apparently never created as a tutoring system and the various parts were not coherently put together. According to Laird and Rosenbloom ( 1992 ),
Even with all its innovations, by the end of the project, Newell regarded Merlin as a failure. It was a practical failure because it never worked well enough to be useful (possibly because of its ambitious goals), and it was a scientific failure because it had no impact on the rest of the field. Part of the scientific failure can be attributed to Newell’s belief that it was not appropriate to publish articles on incomplete systems. Many of the ideas in Merlin could have been published in the late sixties, but Newell held on, waiting until these ideas could be embedded within a complete running system that did it all. (p. 31)
In the end, he had to pay the price of “indefinite delays in application.” Merlin is virtually undocumented in the history of intelligent tutoring systems (see e.g., Nwana, 1990 ; Sleeman & Brown, 1982 ). The first intelligent tutoring system was created in 1970 by Jaime R. Carbonell. Had Newell gone with the “immediate payoff” route of automization, he might have been credited with creating the first intelligent tutoring system.
In 1966, slightly before Newell began working on Merlin, Simon ( 1967 ) coined the term “learning engineering” (Willcox et al., 2016 ) in an address titled “The Job of a College President”:
The learning engineers would have several responsibilities. The most important is that, working in collaboration with members of the faculty whose interest they can excite, they design and redesign learning experiences in particular disciplines. (p. 77)
Simon remained interested in systematic efforts in improving university education and worked on founding the Center for Innovation in Learning at CMU in 1994 (Reif & Simon, 1994 ; Simon, 1992a , 1995 ). The center was dedicated to cross-campus research in education, including supporting a PhD program in instructional science (Hayes, 1996 ). Although at least some of Simon’s interest in this area was due to his passion for teaching as a university professor, his interest in the educational implications of cognitive science played a role as well. Indeed, the effort to form the Center for Innovation in Learning seemingly started in 1992 with Simon sending a memo to the vice provost for education with the subject “Proposal for an initiative on cognitive theory in instruction” (Simon, 1992b ). The concept of learning engineering seemingly only gained widespread interest in the 2010s with the formation of campuswide learning engineering initiatives, including the Simon Initiative at CMU (named in honor of Herbert Simon), and the broader formation of the learning engineering research community, a group of researchers and practitioners with backgrounds in fields such as educational technology, instructional design, educational data mining, learning analytics, and the learning sciences interested in improving the design of learning environments in data-informed ways.
In 1975, Simon applied for and received a grant from the Alfred P. Sloan Foundation to conduct a large-scale study with other researchers at CMU on “Educational Implications of Information-Processing Psychology,” effectively drawing out the ideas first suggested by Simon and Newell ( 1971 ). This grant had several thrusts including teaching problem solving in a course at CMU and developing “computer-generated problems for individually-paced courses.” The longer term objective for the latter thrust was that it “should also be extendable into a tutoring system that can diagnose students’ specific difficulties with problems and provide appropriate hints, as well as produce the answer,” a vision that would later be largely implemented in the large body of tutoring systems coming out of Carnegie Mellon as described below. Newell had actually already embarked on some of this work. In 1971, Newell created a method for automatically generating questions in an artificial intelligence course. Ramani and Newell ( 1973 ) subsequently wrote a paper on the automated generation of computer programming problems. Although they submitted the paper to the recently formed journal Instructional Science , it was never published. The work conducted under the grant, while of relevance to education, was mostly conducted under the auspices of psychology (e.g., studying children’s thinking).
Later, Zhu and Simon ( 1987 ) tested teaching several algebra and geometry tasks using only worked examples or problem-solving exercises and showed that both could be an effective way of learning these tasks when compared to traditional lecture-style instruction. They also showed, using think-aloud protocols, that students effectively learn several production rules for an algebra factoring task. Finally, they showed that an example-based curriculum for three years of algebra and geometry in Chinese middle schools was seemingly as effective as traditional instruction and led to learning the material in two years instead of three. Zhu and Simon ( 1987 ) constructed their examples and sequenced them by postulating the underlying production rules, and therefore their claim is that carefully constructed examples based on how experts solve problems can be an efficient form of instruction. This is one of the earliest studies comparing worked examples with problem solving tasks and lecture-based instruction, and probably the earliest large-scale field experiment of the benefit of worked examples (Sweller, 1994 ). The use of worked examples was one of six evidence-based recommendations given in the What Works Clearinghouse Practice Guide on “Organizing Instruction and Study to Improve Student Learning,” which explicitly cited Zhu and Simon ( 1987 ) as one piece of evidence.
John R. Anderson joined Newell and Simon at Carnegie Mellon in 1978 and was interested in developing a cognitive architecture that could precisely and accurately simulate human cognition (American Psychological Association, 1995 ). He developed the ACT theory (standing for Adaptive Control of Thought) of human cognition, which has since evolved into ACT-R. After publishing his 1983 monograph “The Architecture of Cognition,” Anderson needed to find a way to improve his ACT theory, which seemed to be complete, so he tried to break the theory by using it to create intelligent tutoring systems (American Psychological Association, 1995 ):
The basic idea was to build into the computer a model of how ACT would solve a cognitive task like generating proofs in geometry. The tutor used ACT’s theory of skill acquisition to get the student to emulate the model. As Anderson remembers the proposal in 1983, it seemed preposterous that ACT could be right about anything so complex. It seemed certain that the enterprise would come crashing down and from the ruins a better theory would arise. However, this effort to develop cognitive tutors has been remarkably successful. While the research program had some theoretically interesting difficulties, it is often cited as the most successful intelligent tutoring effort and is making a significant impact on mathematics achievement in a number of schools in the city of Pittsburgh. It is starting to develop a life of its own and is growing substantially independent of Anderson’s involvement.
Indeed, this work led to the extensive work on intelligent tutoring systems at Carnegie Mellon and affected research on such systems worldwide. As a result of these endeavors, in 1998, Carnegie Mellon researchers, including Anderson and colleagues Kenneth Koedinger and Steve Ritter, founded Carnegie Learning Inc., which develops Cognitive Tutors for algebra and other fields that are still being used by over half a million students per year in classrooms across the United States (Bhattacharjee, 2009 ). While Newell’s pioneering work on intelligent tutoring did not see the light of day, Anderson’s became very influential.
From the above, it is clear that Simon, Newell, and Anderson made several contributions to the field of education, but their impact in the field goes far beyond these direct contributions. In the 1950s, the predominant learning theory in education was behaviorism; due to the work of Simon, Newell, and their colleagues, information-processing psychology or cognitivism offered an alternative paradigm, which became mainstream in education in the 1970s. In the 1990s, Anderson and Simon, along with Lynne Reder, wrote a sequence of articles in educational venues to dismiss new educational theories that were gaining popularity at the time, namely situated learning and constructivism, by bringing myriad evidence from information-processing psychology (Anderson et al., 1996 , 1998 , 1999 ). One of these articles, “Situated Learning and Education” (Anderson et al., 1996 ), was published in Educational Researcher , one of the most prominent journals in the field of educational research, and led to a seminal debate between Anderson, Reder, and Simon on the one hand and James Greeno on the other, who had moved from being a proponent of information-processing psychology to being an influential advocate for the situative perspective (Anderson et al., 1997 , 2000 ; Greeno, 1997 ). Based on Google Scholar, Anderson et al. ( 1996 ) is currently the 25th most cited article in Educational Researcher . The ninth most cited article in the journal was one of many articles that tried to make sense of this debate: “On Two Metaphors for Learning and the Dangers of Choosing Just One” (Sfard, 1998 ). It is important to note that Anderson, Reder, and Simon were not proposing an alternative to trendy theories of learning (situativism and constructivism); rather they were defending the predominant paradigm in educational research on learning after the heyday of behaviorism.
It should by now be clear that over the span of several decades, Simon, Newell, and Anderson simultaneously made direct contributions to education (largely as applications of their pioneering work in psychology) and helped shape the landscape of theories of learning and cognition in education for decades. But beyond that, they were committed to reminding the education community that information-processing psychology provided the science that education needed to succeed. In their paper critiquing radical constructivism, Anderson et al. ( 1999 ) made a call for bringing information-processing psychology to bear on education research, similar to the call that Simon and Newell ( 1971 ) had made earlier, but with seemingly greater concern about the “antiscience” state of education research:
Education has failed to show steady progress because it has shifted back and forth among simplistic positions such as the associationist and rationalist philosophies. Modern cognitive psychology provides a basis for genuine progress by careful scientific analysis that identifies those aspects of theoretical positions that contribute to student learning and those that do not. Radical constructivism serves as the current exemplar of simplistic extremism, and certain of its devotees exhibit an antiscience bias that, should it prevail, would destroy any hope for progress in education. (p. 231)
But the proponents of these “antiscence” positions (radical constructivism and situativism) were no strangers to cognitive science and many of them were actually originally coming from the information-processing tradition and artificial intelligence itself. They turned away from it, because, to them, it lacked something. So what was the science of Simon and Newell lacking?
The Situative Perspective as a Reaction to AI
If 1956 saw the birth of cognitive science and artificial intelligence, we might say that 1987 saw the birth of situativism, which emerged to address what its proponents saw as limitations to the information-processing approach (which also became known as cognitivism). In the 1980s, several researchers from a variety of fields independently developed related ideas around how cognition and learning are necessarily context-dependent, and not taking the situation into account can lead to gross oversimplifications. Lauren Resnick, the president of the American Educational Research Association in 1987, gave her presidential address on the topic of “Learning in School and Out” (Resnick, 1987 ), which synthesized work emerging from a variety of disciplines pointing to how learning that happens in out-of-school contexts widely differs from in-school learning. In the same year, James Greeno and John Seely Brown founded the Institute for Research on Learning (IRL) in Palo Alto, California. This organization brought together many of the researchers that were thinking about the situated nature of cognition and learning, and was highly influential in the turn that such research took over the next few years. Situativism is not really one unified theory, but a conglomerate of a variety of particular theories developed in different fields. Given the different focus of each field, the terms “situated cognition,” “situated action,” or “situated learning” are often used. However, Greeno ( 1997 ) suggested that such terms are misleading, because “all learning and cognition is situated by assumption” (p. 16), advocating for the term “situative perspective” instead.
The situative perspective is also related to and influenced by much earlier sociocultural theories drawing on the work of Vygotsky and other Russian psychologists, which gained attention in the US in the 1980s through the work of Michael Cole and others. It is also related to a number of overlapping theories that all emerged around the same time in reaction to cognitivism, such as distributed cognition (Hutchins et al., 1990 ; Salomon, 1993 ), extended mind (Clark & Chalmers, 1998 ), and embodied cognition (Johnson, 1989 ; Varela et al., 1991 ).
To those who are familiar with situativism, it is perhaps abundantly clear that it arose in reaction to the limitations of cognitivism as a theory of how people learn. What I suspect is less clear is the extent to which it arose in reaction to the broader field of artificial intelligence, and the extent to which AI influenced the thinking of the pioneers of the situativism. Indeed, many of the early proponents of the situative perspective were coming from within the AI tradition itself but had seen limitations to the traditional AI approach. John Seely Brown and Allan Collins, who wrote one of the early papers advocating for situated learning (Brown et al., 1989 )—the second most cited paper published in Educational Researcher —had worked on some of the earliest intelligent tutoring systems (Brown et al., 1975a , b ; Carbonell & Collins, 1973 ). Brown et al. ( 1975a ) explicitly proposed a tutoring system rooted in production rules. Moreover, Brown in particular conducted core AI research on various topics as well (Brown, 1973 ; De Kleer & Brown, 1984 ; Lenat & Brown, 1984 ). Etienne Wenger, who coined the concept of “communities of practice” with Jean Lave, initially wanted to write his dissertation “in the context of trying to understand the role that artificial intelligence could play in supporting learning in situ” but it “ became clear fairly early on that the field of artificial intelligence as it was conceived of was too narrow for such an enterprise” since “the traditions of information-processing theories and cognitive psychology did address questions about learning but did so in a way that seemed too out of context to be useful” (Wenger, 1990 , p. 3). Lave and Wenger ( 1991 ) wrote the second most cited book in the field of education, and Wenger’s ( 1999 ) book on communities of practice is the third most cited book in education (Green, 2016 ). Footnote 5
Moreover, despite engaging in a debate with Anderson, Reder, and Simon, James Greeno acknowledged that he “had the valuable privileges of co-authoring papers with Anderson and with Simon and of serving as a co-chair of Reder’s dissertation committee” (Greeno, 1997 , p. 5). Outside of education, another important pioneer of situated cognition, Terry Winograd, was a student of Seymour Papert and Marvin Minsky (whom we will discuss shortly) and made very important contributions to the early history of artificial intelligence. Two exceptions to this trend are Jean Lave and Lucy Suchman, who were anthropologists by training, but even they were operating in collaboration with AI researchers. For example, Suchman ( 1984 ) acknowledged John Seely Brown as having the greatest influence on her dissertation, which subsequently became an influential book in human-computer interaction and the learning sciences.
Thus, it is clear that situativism arose in reaction to the limitations of AI, but did AI have any further influence on the direction of situativist researchers? The majority of research in this tradition gravitated towards using methods of deep qualitative inquiry, such as ethnography to understand learning in situ, but some of the very pioneers of situativist theories still advocated for the use of computational methods to enhance our understanding of learning, a point I will return to in the final section of this paper. However, the use of these methods did not gain much traction as researchers turned more and more towards qualitative methods to understanding learning in context. Much of the work in the learning sciences today is rooted in situativist theories of learning, but the origins of such theories as reactions to artificial intelligence would not be apparent without taking a historical look at the field.
Different Approaches to AI: Symbolic vs. Non-symbolic and Neat vs. Scruffy
While situativism was reactionary to AI, it was not part of AI per se. Even AI researchers who adopted a situativist perspective gravitated towards other fields, such as anthropology and human-computer interaction to conduct their work. However, within the field of artificial intelligence, there were also competing approaches that challenged the one taken by Simon, Newell and their colleagues. I will now give a very high-level exposition of different approaches to AI research, in order to set the stage for how a competing approach resulted in a different line of inquiry in education as well.
The early days of AI, from the 1950s to the 1980s, was dominated by what is often called symbolic AI or good-old fashioned AI (GOFAI) (Haugeland, 1989 ), which is embodied in the work of Simon, Newell, and those influenced by their work. This approach is in stark contrast to a competing approach that has taken a number of forms throughout the history of artificial intelligence, but which may be broadly characterized as non-symbolic or subsymbolic AI. The current dominant paradigm in AI is a type of non-symbolic AI: machine learning. Within machine learning, an increasingly popular approach is deep learning, which is rooted in an early approach called connectionism. Connectionism—which involves simulating learning via artificial neural networks—actually first emerged in the 1940s (McCulloch & Pitts, 1943 ), and so it predates the birth of AI, but this approach was not taken seriously in the early days of AI by researchers who supported symbolic AI (Nilsson, 2009 ; Olazaran, 1996 ). However, neural networks made their way back into mainstream AI after advances in algorithms—such as the development of the back propagation algorithm in the 1980s—and currently dominate the field of AI.
If connectionism and machine learning are the antithesis to symbolic AI, then what was the analogous antithesis to information-processing approaches to education? This is where the story gets a little complicated. As we have already seen, the pushback to information-processing psychology came from situativism and radical constructivism. But these theories share no immediately obvious relationship with neural networks. Interestingly, some connections have been drawn between connectionism and situative and constructivist theories (Doroudi, 2020 ; Quartz, 1999 ; Shapiro & Spaulding, 2021 ; Winograd, 2006 ), but these connections have not had practical import on approaches in education. However, there was another competitor to symbolic AI, which I believe is often obscured by the distinction between symbolic and connectionist approaches. To understand this other approach, we need to examine a different dichotomy in the history of AI: neats vs. scruffies.
The distinction was first introduced by Roger Schank in the 1970s (Abelson, 1981 ; Nilsson, 2009 ; Schank, 1983 ). According to Abelson ( 1981 ), “The primary concern of the neat is that things should be orderly and predictable while the scruffy seeks the rough-and-tumble of life as it comes.” Neats are researchers that take a more precise scientific approach that favors mathematically elegant solutions, whereas scruffies are researchers that take a more ad hoc and intuition-driven engineering approach. According to Kolodner ( 2002 ), who was Roger Schank’s student,
While neats focused on the way isolated components of cognition worked, scruffies hoped to uncover the interactions between those components. Neats believed that understanding each of the components would provide us with what we needed to see how they fit together into a working system of cognition. Scruffies believed that no component of our cognitive systems was isolated, but rather, because each depends so much on the workings of the others, their interactions were the key to understanding cognition. (p. 141)
Kolodner ( 2002 ) specifically refers to Simon, Newell, and Anderson as “quintessential neats,” and Schank, Minsky, and Papert as “quintessential scruffies” in AI. Extending the definitions to education, situativist and constructivist education researchers fall largely on the scruffy side of the spectrum. Therefore, to better understand the parallels in AI and education that rejected the information-processing perspective we must now turn to the founders of AI on the scruffy side (Minsky and Papert, and in a later section, Schank).
Papert and Minsky: From Lattice Theory to Logo Turtles
As mentioned earlier, Marvin Minsky was one of the Dartmouth Tetrad. Seymour Papert was not present at the Dartmouth Conference, but joined the AI movement early on when he moved to the Massachusetts Institute of Technology (MIT) in 1964, and formed the AI Laboratory with Minsky. I believe it is common to regard Minsky as one of the founders of AI and Papert as a seminal figure in educational technology. However, this is an oversimplification; Minsky and Papert both played important roles in the field of AI and in the field of education. They coauthored Perceptrons: An Introduction to Computational Geometry , an important technical book in the history of AI. Minsky’s book The Society of Mind was originally a collaboration with Papert (Minsky, 1988 ). Moreover, Papert acknowledges in one of his seminal books on education, Mindstorms , that “Marvin Minsky was the most important person in my intellectual life during the growth of the ideas in this book” (Papert, 1980 ). A recently published book edited by Cynthia Solomon, Inventive Minds: Marvin Minsky on Education , collects six essays that Minsky has written about education (Minsky, 2019 ). Furthermore, Minsky and Papert were both associate editors of the Journal of the Learning Sciences when it formed in 1991 (Journal of the Learning Sciences, 1991 ).
Minsky and Papert’s 1969 book Perceptrons played an important role in devaluing research on connectionism in the 70 s. According to Olazaran ( 1996 ), the book did not completely end all connectionist research, but it led to the institutionalization and legitimization of symbolic AI as the mainstream. While this may very well be true, I think it obscures Minsky and Papert’s actual positions in AI research by suggesting they were proponents of symbolic AI. Indeed, Olazaran ( 1996 ) claims they were symbolic AI researchers. Perhaps, their role in “shutting down” perceptrons research was seen as so large that other researchers were naturally inclined to situate them in the symbolic camp. Indeed, Newell ( 1969 ) wrote a very positive book review of Perceptrons reinforcing the idea that he was in the same camp as the authors. Moreover, Simon and Newell have, to my knowledge, never entered into any public disputes or debates with Papert and Minsky over their approaches to AI. Perhaps, they saw each other with respect as early proponents of a new field that exhibited mathematical rigor who shared some common “foes”: connectionism and philosophical critiques against AI (Dreyfus, 1965 ; Papert, 1968 ). But in fact, their approaches were sharply different in both AI and education. This can be gauged by taking a closer look at the work of Papert and Minsky; we will begin with a look at their approach to AI research, followed by an exposition of Papert’s contributions to education (which as outlined above were developed in collaboration with Minsky).
A Piagetian Approach to AI
To understand the difference in approach, a bit of background on Papert is needed. Papert obtained two PhDs in mathematics in the 1950s, both on the topic of lattices. In 1958, he then moved to Geneva where he spent the next several years working with the famous psychologist and genetic epistemologist, Jean Piaget, who is the founder of constructivism as a psychological theory. Piaget’s influence on Papert affected his approach to AI research and education: “If Piaget had not intervened in my life I would now be a ‘real mathematician’ instead of being whatever it is that I have become” (Papert, 1980 , p. 215). In 1964, Papert moved to the Massachusetts Institute of Technology (MIT) to work with Minsky on artificial intelligence. Papert ( 1980 ) notes the reason for moving from studying children with Piaget to studying AI at MIT:
Two worlds could hardly be more different. But I made the transition because I believed that my new world of machines could provide a perspective that might lead to solutions to problems that had eluded us in the old world of children. Looking back I see that the cross-fertilization has brought benefits in both directions. For several years now Marvin Minsky and I have been working on a general theory of intelligence (called “The Society Theory of Mind”) which has emerged from a strategy of thinking simultaneously about how children do and how computers might think. (p. 208)
Minsky and Papert’s early approach to AI is well encapsulated in a 1972 progress report on their recently formed MIT AI Laboratory. After mentioning a number of projects that they were undertaking, Minsky and Papert ( 1972 ) describe their general approach:
These subjects were all closely related. The natural language project was intertwined with the commonsense meaning and reasoning study, in turn essential to the other areas, including machine vision. Our main experimental subject worlds, namely the “blocks world” robotics environment and the children’s story environment, are better suited to these studies than are the puzzle, game, and theorem-proving environments that became traditional in the early years of AI research. Our evolution of theories of Intelligence has become closely bound to the study of development of intelligence in children, so the educational methodology project is symbiotic with the other studies, both in refining older theories and in stimulating new ones; we hope this project will develop into a center like that of Piaget in Geneva.
Like Simon and Newell’s approach, Minsky and Papert were interested in studying both machine and human cognition, but some of the key differences in their approaches are apparent in the aforementioned quote. Minsky and Papert were interested in a wider range of AI tasks, like common sense reasoning, natural language processing, robotics, and computer vision, all of which are prominent areas of AI today. Moreover, they were interested in children, not experts. Relatedly, they emphasized learning and development (hence the emphasis on children) over performance, which is markedly different from Newell and Simon’s approach of emphasizing the study of performance. Indeed, according to Newell and Simon ( 1972 ),
If performance is not well understood, it is somewhat premature to study learning. Nevertheless, we pay a price for the omission of learning, for we might otherwise draw inferences about the performance system from the fact that the system must be capable of modification through learning. It is our judgment that in the present state of the art, the study of performance must be give precedence, even if the strategy is not costless. (p. 8)
Minsky ( 1977 ) later provided justification for this choice to focus on development as follows:
Minds are complex, intricate systems that evolve through elaborate developmental processes. To describe one, even at a single moment of that history, must be very difficult. On the surface, one might suppose it even harder to describe its whole developmental history. Shouldn't we content ourselves with trying to describe just the “final performance?” We think just the contrary. Only a good theory of the principles of the mind’s development can yield a manageable theory of how it finally comes to work. (p. 1085)
Later in their report, Minsky and Papert ( 1972 ) explicitly state limitations of research on “Automatic Theorem Provers” (without making explicit mention of Newell and Simon) such as the lack of emphasis on “a highly organized structure of especially appropriate facts, models, analogies, planning mechanisms, self-discipline procedures, etc.” as well as the lack of heuristics in solving proofs (e.g., mathematical insights used in solving the proof that are not part of the proof itself). They then use this to motivate the need for what they call “microworlds”:
We are dependent on having simple but highly developed models of many phenomena. Each model—or “microworld” as we shall call it—is very schematic…we talk about a fairyland in which things are so simplified that almost every statement about them would be literally false if asserted about the real world. Nevertheless, we feel they are so important that we plan to assign a large portion of our effort to developing a collection of these microworlds and finding how to embed their suggestive and predictive powers in larger systems without being misled by their incompatibility with literal truth. We see this problem—of using schematic heuristic knowledge—as a central problem in Artificial Intelligence.
Indeed, confining AI programs to tackling problems in microworlds or “toy problems”—another phrase attributed to Papert (Nilsson, 2009 )—became an important approach at MIT and in AI in general to this day. But as indicated by the quote above, Papert and Minsky’s goal was to see how to combine microworlds to develop intelligence that is meaningful in the real world. This is indicative of Papert and Minsky’s general approach to AI. Namely, they were interested in building up models of intelligence in a bottom-up fashion. Rather than positing one grand “unified theory of cognition” (Newell, 1994 ), they realized that the mind must consist of a variety of many small interacting components, and that how minds organize many pieces of localized knowledge is more important than universal general problem-solving strategies. It is the interaction of all these pieces that makes up intelligence and gives rise to learning.
This naturally leads to the question: how does the mind represent knowledge? Knowledge representation is a fundamental concern of AI (and an important but understudied concern in education as well). Minsky ( 1974 ) wrote one of the seminal papers on knowledge representation describing a representation he called “frames”:
Here is the essence of the theory: When one encounters a new situation (or makes a substantial change in one’s view of the present problem) one selects from memory a structure called a Frame . This is a remembered framework to be adapted to fit reality by changing details as necessary. A frame is a data-structure for representing a stereotyped situation, like being in a certain kind of living room, or going to a child’s birthday party. Attached to each frame are several kinds of information. Some of this information is about how to use the frame. Some is about what one can expect to happen next. Some is about what to do if these expectations are not confirmed.
Frames allow for navigating unforeseen situations in terms of situations one has seen before. It means that early on, one might make mistakes by extrapolating based on a default version of a frame, but as a situation becomes clearer, one can customize the frame (by filling in certain “terminals” or “slots”) to meet the needs of the situation. Importantly, frames were meant to be relevant to a variety of areas of artificial intelligence, including computer vision, language processing, and memory (Goldstein & Papert, 1977 ).
Frames became one component of Minsky and Papert’s broader bottom-up approach to artificial intelligence, which is outlined in Minsky’s famous book, The Society of Mind , which as mentioned earlier was jointly developed with Papert. As the name suggests, Minsky ( 1988 ) suggests the mind is a society of agents:
I’ll call Society of Mind this scheme in which each mind is made of many smaller processes. These we’ll call agents. Each mental agent by itself can only do some simple thing that needs no mind or thought at all. Yet when we join these agents in societies—in certain very special ways—this leads to true intelligence. (p. 17)
Ironically, this approach shares some commonalities with connectionism. Both posit a bottom-up process that gives rise to learning. Like Minsky’s agents, each individual neuron is not sophisticated, but it is the connections between many neurons that can result in learning to do complex tasks. Indeed, in a chapter that “grew out of many long hours of conversation with Seymour Papert” (p. 249), Turkle ( 1991 ) classified both connectionism and the society of mind theory as part of “Emergent AI,” which arose as a “romantic” response to traditional information-processing AI. But there is a clear difference—each of the agents in Minsky’s society is itself still interesting and there are several distinct kinds of agents that are designed to play conceptually different roles. In their prologue to the second edition of Perceptrons , Minsky and Papert ( 1988 ) claim that “the marvelous powers of the brain emerge not from any single, uniformly structured connectionist network but from the highly evolved arrangements of smaller, specialized networks which are interconnected in very specific ways” (p. xxiii). Minsky and Papert ( 1988 ) further admit when discussing the often dichotomized “poles of connectionist learning and symbolic reasoning”, that “it never makes any sense to choose either of those two views as one’s only model of the mind. Both are partial and manifestly useful views of a reality of which science is still far from a comprehensive understanding” (p. xxiii).
Papert: The Educational Thinker and Tinkerer
In tandem with developing this work in AI, Papert made critical advances in educational technology and educational theory. In 1966, Papert—along with Wallace Feurzeig, Cynthia Solomon, and Daniel Bobrow—conceived of the Logo programming language to introduce programming to kids (Solomon et al., 2020 ). (Bobrow was a student of Minsky’s and a prominent AI researcher in his own right who became president of AAAI in 1989.) According to Papert ( 1980 ), his goal was to design a language that would “have the power of professional programming languages, but [he] also wanted it to have easy entry routes for nonmathematical beginners.” Logo was originally a nongraphical programming language designed “for playing with words and sentences” (Solomon et al., 2020 , p. 33), but early on Papert saw the power of adding a graphical component where children write programs to move a “turtle” (either a triangle on the screen or a physical robot connected to the computer) that traces geometric patterns (Papert, 1980 ).
In 1980, Papert wrote his seminal book, Mindstorms: Children, Computers, and Powerful Ideas , which described how he envisioned the ability for computers to enact educational change (through Logo-like programs). Papert took the idea of a “microworld” that he and Minsky had earlier used in AI and repurposed it to be a core part of his educational theory. In fact, I believe those familiar with the concept of microworld in Papert’s educational thought would likely not realize the AI origins of this concept as he does not seem to explicitly link the two—to Papert, it was a natural extension. A microworld in Logo is “a little world, a little slice of reality. It’s strictly limited, completely defined by the turtle and the ways it can be made to move and draw” (Papert, 1987b ). The fact that these microworlds were not completely accurate renditions of reality was not a disadvantage, but rather a testament to the power of the approach:
So, we design microworlds that exemplify not only the “correct” Newtonian ideas, but many others as well: the historically and psychologically important Aristotelian ones, the more complex Einsteinian ones, and even a “generalized law-of-motion world” that acts as a framework for an infinite variety of laws of motion that individuals can invent for themselves. Thus learners can progress from Aristotle to Newton and even to Einstein via as many intermediate worlds as they wish. (p. 125)
To Papert, this would not confuse students but rather help them understand central concepts like motion in more intuitive ways (Papert, 1980 ). The fact that many students (including MIT undergraduates that Papert’s colleague, Andrea diSessa, studied) struggle with the concept of motion is precisely because of the way they learn the underlying mathematics and physics; they do not get the intuition they would otherwise get from experimenting with microworlds:
And I’m going to suggest that in a very general way, not only in the computer context but probably in all important learning, an essential and central mechanism is to confine yourself to a little piece of reality that is simple enough to understand. It’s by looking at little slices of reality at a time that you learn to understand the greater complexities of the whole world, the macroworld. (Papert, 1987b , p. 81)
Clearly this is a drastically different conception of learning than the one traditional information-processing psychology espouses. Here, learning is not an expert transmitting certain rules to a student, but rather the student picking up “little nuggets of knowledge” as they experiment and discover a world for themselves (Papert, 1987b ). Moreover, not every child is expected to learn the same things; each child can learn something that interests them (Papert, 1987b ): “No two people follow the same path of learnings, discoveries, and revelations. You learn in the deepest way when something happens that makes you fall in love with a particular piece of knowledge. (p. 82)”.
As such, Logo became more than a tool for children to learn programming, but also a tool to help children learn about and experience various subjects including geometry, physics, and art. However, Logo did not teach these subjects as an intelligent tutoring system would; it allowed students to discover the powerful ideas in these domains (with guidance from a teacher and peers). As described by Abelson and diSessa ( 1986 ), two of Papert’s colleagues, “The abundance of the phenomena students can investigate on their own with the aid of computer models shows that computers can foster a style of education where ‘learning through discovery’ becomes more than just a well-intentioned phrase.” (p. xiii). Moreover, Abelson and diSessa ( 1986 ) explain, “turtle geometry” not only changes the way students interact with the content, but it also changes the nature of geometric knowledge that students engage with:
Besides altering the form of a student's encounter with mathematics, we wish to emphasize the role of computation in changing the nature of the content that is taught under the rubric of mathematics….Most important in this endeavor is the expression of mathematical concepts in terms of constructive, process-oriented formulations, which can often be more assimilable and more in tune with intuitive modes of thought than the axiomatic-deductive formalisms in which these concepts are usually couched. As a consequence we are able to help students attain a working knowledge of concepts such as topological invariance and intrinsic curvature, which are usually reserved for much more advanced courses. (p. xiv)
This approach contrasts sharply with the proof-based geometry tutoring systems being developed by Anderson, Koedinger, and colleagues around the same time (Anderson et al., 1985 ; Koedinger & Anderson, 1990 ).
But what does Papert’s educational philosophy have to do with AI? In Mindstorms , Papert ( 1980 ) has a chapter titled “Logo’s Roots: Piaget and AI.” For Papert, Piaget provided the learning theory and epistemology that underpinned his endeavor, but AI allowed Papert to interpret Piaget in a richer way using computational metaphors: “The aim of AI is to give concrete form to ideas about thinking that previously might have seemed abstract, even metaphysical” (Papert, 1980 , pp. 157158). In a sense, his use of AI is similar to that of Newell and Simon: better understanding human intelligence by creating artificial intelligence. However, as we have already seen, his approach was quite different:
In artificial intelligence, researchers use computational models to gain insight into human psychology as well as reflect on human psychology as a source of ideas about how to make mechanisms emulate human intelligence. This enterprise strikes many as illogical: Even when the performance looks identical, is there any reason to think that underlying processes are the same? Others find it illicit: The line between man and machine is seen as immutable by both theology and mythology. There is a fear that we will dehumanize what is essentially human by inappropriate analogies between our “judgments” and those computer “calculations.” I take these objections very seriously, but feel that they are based on a view of artificial intelligence that is more reductionist [than] anything I myself am interested in. (Papert, 1980 , p. 164, emphasis added)
Papert ( 1980 ) then gives a particular example of how AI has influenced his and Minsky’s thinking about how people learn: how a society of agents can give rise to Piagetian conservation. Piagetian conservation refers to the concept that before the age of seven, children generally do not grasp the concept of how quantity is conserved even when it comes in different forms (e.g., the quantity of a liquid is conserved regardless of the size of the container holding it). Papert and Minsky argue that this theory could begin to be explained by a set of four simple-minded agents and their interactions (Minsky, 1988 ; Papert, 1980 ). Unlike Simon and Newell, Papert and Minsky did not actually believe they had found the exact cognitive mechanisms that explain this phenomena, but rather, they found insights into a process that could resemble it:
This model is absurdly oversimplified in suggesting that even so simple a piece of a child’s thinking (such as this conservation) can be understood in terms of interactions of four agents. Dozens or hundreds are needed to account for the complexity of the real process. But, despite its simplicity, the model accurately conveys some of the principles of the theory: in particular, that the components of the system are more like people than they are like propositions and their interactions are more like social interactions than like the operations of mathematical logic. (Papert, 1980 , pp. 168-169)
This insight in turn presumably led Papert to realize the kinds of educational experiences that students need in order to develop their “society of mind,” and thus the kind of educational experiences that Logo-like microworlds would need to support. Moreover, according to Papert ( 1980 ):
While psychologists use ideas from AI to build formal, scientific theories about mental processes, children use the same ideas in a more informal and personal way to think about themselves. And obviously I believe this to be a good thing in that the ability to articulate the processes of thinking enables us to improve them. (p. 158).
Therefore, Logo provides an environment for children to articulate and think about their own thinking (just as the programming language Lisp allowed AI researchers to concretize their theories and models). Logo did not use AI directly, but its use was designed to embody a theory of learning that was influenced by Papert and Minsky’s kind of AI.
Minsky and Papert’s approach to simultaneously studying AI and education was exemplified in a press release describing a 1970 symposium hosted by the AI Labroratory called “Teaching Children Thinking” (Minsky & Papert, 1970 ). Footnote 6 Having held this symposium prior to publishing their first AI progress report, the press release pronounced:
The meeting is the first public sign of a shift in emphasis of the program of research in the Artificial Intelligence Laboratory. In the past the principle goals have been connected with machines. These goals will not be dropped, but work on human intelligence and on education will be expanded to have equal attention....plans are being developed to create a program in graduate study in which students will be given a comprehensive exposure to all aspects of the study of thinking. This includes studying developmental psychology in the tradition of Piaget, machine intelligence, educational methods, philosophy, linguistics, and topics of mathematics that are considered to be relevant to a firm understanding of these subjects. (Minsky & Papert, 1970 )
The press release then goes on to state how “current lines of educational innovation go in exactly the wrong direction” (Minsky & Papert, 1970 ). They claimed that “The mere mention of the ‘new math’ throws them into a rage. So do most trends in the psychology of learning and in programmed instruction” (Minsky & Papert, 1970 ). Perhaps ironically, the symposium had a panel discussion led by Marvin Minsky, with Allen Newell and Patrick Suppes as two of the three panelists. Newell was working on Merlin at the time, and Suppes was pioneering efforts in computer-assisted instruction, much of which consisted of teaching elementary school students elementary logic and new math. One wonders how much rage was present in the panel discussion!
In the twenty-first century, Logo has not fundamentally changed education in K-12 schools. However, Papert ( 1980 ) did not see Logo as the solution, but rather as a model “that will contribute to the essentially social process of constructing the education of the future” (Papert, 1980 , p. 182). In a sense, Logo and Papert’s legacy have had success in this regard. Many children’s programming languages that have gained popularity in recent years were either directly or indirectly inspired by Logo. Scratch, the popular block-based programming language for kids, was developed by Papert’s student Mitchel Resnick. Lego’s popular robotics kit, Lego Mindstorms, was inspired by Papert and named after his book. However, Logo was about more than just computer science education; to reiterate, it could help students learn about topics such as geometry, physics, art, and perhaps most importantly, their own thinking.
Moreover, Papert has had an immense impact on educational theory. His theory of constructionism took Piaget’s constructivism and augmented it with the idea that a student’s constructions are best supported by having objects (whether real or digital) to build and tinker with. This has been a source of inspiration for the modern-day maker movement (Stager, 2013 ). Many of Papert’s students and colleagues who worked on Logo were or are leading figures in the learning sciences and educational technology. Footnote 7 In addition, one of Papert’s student, Terry Winograd, made important contributions to AI before becoming one of the foremost advocates for situated cognition, as mentioned earlier. In fact, it appears that seeds of situated learning and embodied cognition existed in Papert’s writings before the movement took off in the late 80 s (Papert, 1976 , 1980 ). For example, Papert ( 1980 ) describes the power of objects like gears (his childhood obsession) and the Logo turtle in learning, by connecting the body and the mind:
The gear can be used to illustrate many powerful “advanced” mathematical ideas, such as groups or relative motion. But it does more than this. As well as connecting with the formal knowledge of mathematics, it also connects with the “body knowledge,” the sensorimotor schemata of a child. You can be the gear, you can understand how it turns by projecting yourself into its place and turning with it. It is this double relationship—both abstract and sensory—that gives the gear the power to carry powerful mathematics into the mind. (p. viii)
Beyond this legacy in educational technology and the learning sciences, Papert—who was an anti-apartheid activist in his youthful days in South Africa—should also be recognized as an education revolutionary, visionary, and critic who sought to fundamentally change the nature of schools. This puts him alongside the ranks of Paulo Freire, Ivan Illich, and Neil Postman. Indeed, discussions with Freire influenced Papert’s thinking in The Children’s Machine: Rethinking School in the Age of the Computer , which Freire in turn referred to as “a thoughtful book that is important for educators and parents and essential to the future of their children” (Papert, 1993 , back cover). Footnote 8 However, unlike many technologists and entrepreneurs who want to “disrupt” education, Papert did not take a technocentric approach; in fact, he himself coined the term “technocentric” to critique it, as he recognized that technology was only secondary to “the most important components of educational situations—people and cultures” (Papert, 1987a , p. 23).
The Intertwined History of AI and Education in the UK
The narrative described so far is predominantly centered on the history of artificial intelligence and education in the United States. While the Dartmouth Tetrad are renowned for their pioneering contributions to AI, there was also early research in AI happening in the United Kingdom. In this section, I briefly show that a lot of the aspects of the intertwined history of AI and education in the US were also paralleled by AI pioneers based in the UK.
Donald Michie, who had worked with Alan Turing and others as a Bletchley Park codebreaker in World War II, was one of the earliest AI researchers in the UK (Nilsson, 2009 ). In 1960, he created the Matchbox Educable Noughts and Crosses Engine (MENACE), an arrangement of 304 matchboxes and some glass beads that (when operated by a human properly) could learn to play the game of noughts and crosses (or tic-tac-toe; Nilsson, 2009 ). In 1965, Michie established the UK’s first AI laboratory, the Experimental Programming Unit, which became the Department of Machine Intelligence and Perception a year later, at the University of Edinburgh. In 1970, the UK’s Social Science Research Council awarded a $10,000 grant to Michie “for a study of computer assisted instruction with young children” (Annett, 1976 ). In 1972, SSRC awarded Jim Howe, one of Michie's colleagues and a founding member of the Department of Machine Intelligence, a $15,000 grant to investigate “An Intelligent Teaching System” (Annett, 1976 ). Howe would receive several other grants from SSRC over the next few years in the area of educational computing, including one on “Learning through LOGO” (Annett, 1976 ). Learning mathematics through Logo programming became a large project in Howe’s group and several influential researchers in what would become the AIED community were part of that project, including Timothy O'Shea, Benedict du Boulay (who completed his PhD under Howe’s supervision), and Sylvia Weir (who joined Papert’s lab in 1978). This work included a focus on using Logo to help students with various disabilities (e.g., physical disabilities, dyslexia, and autism) to learn basic communication skills (Howe, 1978 ). From 1977 to 1996, Jim Howe was the head of the Department of Artificial Intelligence, which evolved out of the Department of Machine Intelligence and Perception.
Michie and Howe continued to pursue educational technology research throughout their careers. For example, in 1989, Michie and Bain ( 1989 ) wrote a paper advocating for the necessity of advancing machine learning for creating machines that can teach:
It is our view that the inability of computers to learn has been a principal cause of their inability to teach. It is becoming apparent from the emerging science of Machine Learning that the development of a theoretical basis for learning must be rooted in formalisms sufficiently powerful for the expression of human-type concepts. (p. 20)
That same year, Michie et al. ( 1989 ) also published a paper called “Learning by Teaching,” which advanced a relatively unexplored idea of using AI to support learning by having the student teach the computer using examples, rather than vice versa. In 1994, Michie, along with his wife and fellow AI researcher, Jean Hayes Michie, founded the Human-Computer Learning Foundation, a charity dedicated to enhancing education by designing software where “human and computer agents incrementally learn from each other through mutual interaction” (Human-Computer Learning Foundation, n.d. ).
These UK-based leaders in AI were not merely engaged in cutting-edge applications of educational technology, but like Simon, Newell, Papert, and Minsky, their interest in education was an extension of their mutual investigation of cognition and learning in humans and machines. According to Annett ( 1976 ),
the real significance of the Edinburgh work lies in its AI orientation. When the results of this project are available we may be able to reach some preliminary conclusions on the future viability of knowledge-based teaching systems, but complex problems are involved which will not be solved on the basis of these projects alone. If they are solved in technical and educational terms the question of cost still remains and even a sanguine estimate suggests it will be considerable. Nevertheless the investigation of technical and educational fesibility seems a reasonable aim not just in case implementation may be possible in the long term, but because of the light which could be thrown on some of the basic issues of the nature of “knowledge and “understanding”. This [work]…is breaking new ground in ways of conceiving the nature of the teaching/learning process. (p. 11)
As Howe ( 1978 ) described it,
the learner is viewed as a builder of mental models, erecting for each new domain a knowledge structure that can be brought to bear to solve problems in that domain. Recent research in artificial intelligence suggests that building computer programs is a powerful means of characterising and testing our understanding of cognitive tasks (see, for example, Newell & Simon, 1972; Lindsay and Norman, 1972; Howe, 1975; Longuet-Higgins, 1976). An implication of the AI approach is that teaching children to build and use computer programs to explicate and test their thinking about problems should be a valuable educational activity.
Similarly, according to a report written by a working party, which included Christopher Longuet-Higgins, who was one of the founders of the Department of Machine Intelligence and Perception at Edinburgh and who coined the name “cognitive science” (Longuet-Higgins, 1973 ) for the emerging field:
advances of our understanding of our own thought processes are also critical for improvements in education and training….Computer aided instruction is already useful, but the realization of its full potential must depend on further advances in our understanding of human cognition and on our ability to write programs that make computers function in an intelligent way. (cited in Annett, 1976 , p. 4)
Other researchers also had the same attitude towards studying AI and education in an intertwined fashion. For example, Gordon Pask was a leading cybernetician who was designing analog computer machines that could adaptively teach students as early as the 1950s; he was also doing what would aptly be called AI research at this time (but as it was under the field of cybernetics, his work is typically disregarded in the “sanctioned” history of AI). Pask ( 1972 ) tried to clarify the distinction between AI (or “computation science”) as a conceptual tool for reasoning about how people think and learn, and “computer techniques” as the infrastructure that enables computer-assisted instruction (CAI):
Computation science deals with relational networks and processes that may represent concepts; with the structure of knowledge and the activity of real and artificial minds. Computation science lies in (even is ) the kernel of CAI; it lends stature to the subject and bridges the interdisciplinary gap, between philosophy, education, psychology and the mathematical theory of organisations. Computer techniques, in contrast, bear the same relation to CAI as instrument making to physics or reagent manufacture to chemistry. (p. 236)
The idea of supporting such interdisciplinary research that bridged between AI and education was also supported in a 1973 SSRC Educational Research Board report, where “It was proposed that ‘learning science’, a field involving education, cognitive psychology and artificial intelligence, should be supported…and probably in the form of a long term interdisciplinary research unit” (Annett, 1976 , p. 4). The term “learning science,” preempted a field that would emerge in the US nearly 20 years later. Learning Science did not take off as a new field in the UK in the 1970s, but a decade later, the seeds of another new field were being sowed in the UK, and that is where we turn our attention next.
Artificial Intelligence in Education: The Field
Now that we have seen how some of the key pioneers in AI were also making contributions to education, it is worth discussing how the intersection of AI and education crystallized into a field. The first and second International Conference on Artificial Intelligence and Education were held in Exeter, UK in 1983 and 1985. The name Artificial Intelligence and Education signifies that in the 1980s, researchers saw these two fields as overlapping rather than thinking of education as yet another field where AI could be applied. Indeed, according to Yazdani and Lawler ( 1986 ):
When, in September 1985, the second international conference on Artificial Intelligence and Education was held in Exeter, it was clear that a new interest group had emerged; one which was committed neither primarily to AI nor to education matters, but to matters which fall into the overlap between them. Both subjects show an interest in knowledge acquisition (be it people or machines) and they need a theoretical framework in which to study learning and teaching processes. They can also help each other in many ways. (p. 198)
This sentiment was also shared by others, including John Self, an early AIED researcher and founding editor of the Journal of Artificial Intelligence in Education. As Self ( 2016 ) recalls:
For a brief period in the 1980s (within which AIED 83, no doubt not coincidentally, fell), AI was at the peak of a ‘hype cycle’. It became a bandwagon, with generous research funding, that it was worth trying to hitch a ride on. That, of course, was not AIED’s motivation: we were enthused by what we considered the profound association between education and AI, with its concerns for knowledge representation, reasoning and learning. (p. 5)
The first conference had a lot of emphasis on Logo (from Papert and his colleagues) and other programming languages that could be used in education (Yazdani, 1984 ). The second conference seemingly had two threads of research, one focusing on intelligent tutoring systems and another focused on computer-based learning environments like Logo (Yazdani & Lawler, 1986 ). The conference led to a publication of a book that focused on these two themes in the conference and how to integrate them. In the preface to this book, Lawler and Yazdani ( 1987 ) remarked that:
The 1985 conference ended with the exciting prospect of the ‘coming together’ of the two traditional streams of ‘tutoring systems’ and ‘learning environments’ to address common problems in the design of instructional systems from an Artificial Intelligence perspective. This volume marks the beginning of a synergy between the agendas of the various researchers which promises an interesting and productive future. (p. vii)
However, over the next few years the AIED conference seemed to lean towards the intelligent tutoring systems (Liffick, 1987 ; Sandberg, 1987 ). A comparison of paper titles in the 1985 proceedings with the 1989 proceedings shows this change of focus. Titles in the 1985 proceedings featured the word “microworld”, the word “Logo”, and “intelligent tutoring system” (or a variant) each three times. On the other hand, titles in the 1989 proceedings featured “microworld” and “Logo” only once each, but “intelligent tutoring system” (or a variant) 21 times.
But suddenly something changed. On August 4 th , 1991—the day I was born—the first International Conference of the Learning Sciences (ICLS) commenced. It was meant to be a rebranding of the AIED conference. In fact, it was initially called the Fifth International Conference of the Learning Sciences. That rebranding did not last long; I discuss why in the next section. ICLS continued biannually since 1996, but AIED also returned in 1993 and has continued biannually (and annually since 2018), but with one critical change that most would probably overlook—it has since then been called the International Conference on Artificial Intelligence in Education. Footnote 9 This change in name was likely to match the Journal of Artificial Intelligence in Education, which was founded in 1989. However, I think this change of a seemingly unimportant word reflects the change from AIED as the intersection of two interrelated research areas—AI and education—to a field concerned with applications of AI to education, which is where the status of the field is today. Footnote 10 This change is symbolic of the fact that I believe the history I am narrating here is now “forgotten” by many researchers and practitioners interested in applying artificial intelligence to education. As John Self ( 2016 ) recalls, in the 1990s:
the fact is that very few AIED researchers were able, or wished, to publish their work in the major AI journals and conferences. Not only did we not contribute much to AI, but we didn’t really borrow much from it either, in my opinion. If you looked at the AI conference proceedings of the time you’d find that almost all of it was apparently irrelevant to AIED. (p. 9)
In some ways this change reflects changes in the broader field of AI from broader questions of the nature of (human and machine) intelligence towards more technical questions that might have been less directly applicable to improving how people learn.
Recall that the change from “Artificial Intelligence and Education” to “Artificial Intelligence in Education” occurred right after there was an attempt to switch from AIED to ICLS in 1991. Why did the conference change and then quickly change back? To answer that, we need to turn our attention to another figure in early AI history: Roger Schank.
Schank: From Language Technologies to Learning Technologies
I already introduced Roger Schank as the source of the neat vs. scruffy distinction. Schank was an early pioneer in AI who joined the field as a student in the mid-1960s and made important contributions with his students at Yale (Schank, 2016 ). In 1977, he co-founded the journal Cognitive Science (which in its first two issues had contributions from Papert, Simon, and Anderson), and in 1979, he co-founded the Cognitive Science Society. Schank also made early advances in the field of natural language processing. Like the other AI pioneers we have examined, he was interested in building systems that resembled how humans think and learn. He realized it was important to model the many “scruffy” aspects of human thinking, which neat approaches tended to ignore.
A Scruffy Approach to AI
Schank’s first main contribution to AI was the development of conceptual dependency theory, which emphasized natural language understanding (Schank, 1969 , 1972 ). While Noam Chomsky and others had developed models of language based on syntax, Schank recognized that understanding language was about understanding the semantics—the concepts that underlie the actual words. In conceptual dependency theory, two sentences would share the same conceptual representation if they shared the same meaning, regardless of the language and syntax of each sentence.
Schank then made a series of other contributions to AI that built on one another, including scripts (Schank & Abelson, 1975 ), a theory of dynamic memory (Schank, 1982 ; Schank & Kolodner, 1979 ), and case-based reasoning (Riesbeck & Schank, 1989 ). Case-based reasoning provided an alternative to the “neat” rule-based reasoning, which was popular in AI. Rule-based systems (such as production systems and expert systems) use a collection of rules to deduce new information and take actions. However, Schank and his students noticed that people often do not actually reason using rules. Rather, they reason using prior experiences (i.e., cases) stored in their memory:
Certainly, experts are happy to tell knowledge engineers the rules that they use, but whether the experts actually use such rules when they reason is another question entirely. There is, after all, a difference between textbook knowledge and actual experience.…In fact, in very difficult cases, where the situation is not so clear cut, experts frequently cite previous cases that they have worked on that the current case reminds them of. (Riesbeck & Schank, 1989 , p. 10)
For example, when faced with a new patient, a doctor might consider prior patients with similar symptoms and family histories, a chef might create a new dish by considering similar recipes but using new ingredients, and a lawyer might argue for precedence based on similar prior legal cases. In short, “a case-based reasoner solves new problems by adapting solutions that were used to solve old problems” (Riesbeck & Schank, 1989 , p. 25). Moreover, while rules are useful for finding the “right answer,” case-based reasoning can be helpful when there is no clear right answer (e.g., when deciding which students to admit to a university) (Riesbeck & Schank, 1989 ). A powerful way of storing cases is as rich stories that can be applied to a variety of different situations.
Although not obvious at the surface, at a high level, Schank’s scruffy approach was similar to that of Minsky and Papert. According to Schank, “Marvin Minsky is the smartest person I’ve ever known…Marvin should have been my thesis advisor. I wouldn’t say that I’m his student, but I appreciate everything he does. His point of view is my point of view.” (quoted in Brockman, 1996 , p. 164). Indeed, Schank’s scripts were a knowledge representation that built on Minsky’s frames. Minsky similarly endorsed Schank’s approach (Brockman, 1996 ), and some of Schank’s ideas played a role in Minsky’s society of mind theory.
As with the respect that Minsky and Papert had for Simon and Newell and vice versa, Schank was also respectful of Simon and Newell’s work despite their differences in approach. In a review of Newell’s ( 1994 ) book, Schank and Jona ( 1994 ) state:
Newell has had a strong influence on our views of both psychology and AI. As AI researchers, we share many of the same opinions about the field of psychology. Our views on AI, however, while initially quite similar, have diverged. (p. 375)
Schank’s criticisms of Newell’s approach centered on issues such as the use of unrealistic tasks (e.g., cryptarithemtic and theorem proving) to develop AI and the lack of a sophisticated way of modeling the relationship between concepts in memory.
Interestingly, in his review, Schank also discusses the implications of the book for education. He comments on how Newell had little to directly state about education, and as a result seems to suggest that Newell did not have an (explicit) interest in education. As we have already shown, Newell did have an interest in education and was conducting pioneering educationally-relevant research in the 1960s-1970s, but perhaps by the 1990s, his interest in the area had died down. (Simon on the other hand was still actively committed to enhancing education and engaging in debates in the field.) Schank ended his review with a powerful call-to-action:
Newell argues that it is time for psychologists to come out of their experimental laboratories and put their heads together to build more unified theories of cognition. That is a step in the right direction, but it does not go far enough. More importantly, it is time for all cognitive scientists to realize that, by virtue of their work on learning, memory, and cognition, they have a voice in the debate on education. Good theories of cognition have a practical and important role to play in restructuring the process of education. The separation of the fields of education and cognitive science is both artificial and harmful. A unified theory of cognition must, now more than ever, be put into practical use as the cornerstone of the educational system. (p. 387)
In 1994, it was likely the case that many cognitive scientists were not actively engaged in pursuing the connections of the research on cognition to education; but as we have shown, historically researchers at the forefront of cognitive science were actually committed to education as well. As a pioneer in cognitive science and AI, Schank had joined an earlier generation of AI researchers in acknowledging the importance of their work to education, and he was practically demanding that the rest of his colleagues in cognitive science do the same. But what drove Schank to education in the first place?
Founder of the Learning Sciences
In the early 1980s, Schank gave a keynote speech at the National Reading Conference, and the support he got from the audience about the “awful stuff that [he] was complaining about in schools” led him to change his research focus from then on—his focused shifted to improving education (Schank, 2016 , p. 22). His early thinking on education can be seen in a virtually uncited defense of Papert and his work on Logo, against some critics. Schank ( 1986 ) ended his article by saying:
Right now, with the exception of LOGO and one or two other kinds of software that are also openended, nobody is doing anything very interesting with children and computers. We must learn to encourage and finance other LOGO-like attempts, not criticize the only ones we have. (p. 239)
It was not long before other such attempts would be financed. In 1989, Schank and 24 of his colleagues and students left Yale with $30 million from Anderson Consulting to start the Institute for the Learning Sciences (ILS) at Northwestern University. In 1991, Roger Schank helped form the Journal of the Learning Sciences —which was edited by his former student Janet Kolodner until 2009—and chaired the first International Conference of the Learning Sciences. With these moves, Schank played a pivotal role in the formation of a new field, the learning sciences—another term that Schank coined. But while these moves were intended to be field-building, they were also seen by many as field-fracturing.
Unbeknownst to members of the burgeoning AIED community until it was “too late,” Schank unilaterally renamed the conference and selected his colleagues and “friends” (many of whom were situativist and constructivist researchers who were not previously part of the AIED community) to make up the program committee (Self, 2016 ; see also, Kolodner, 2004 ). Footnote 11 As Self ( 2016 ) recounts, “In short, AIED 91 had been turned into an advertisement for ILS, to the exclusion of the majority of the newly-developing international AIED community” (p. 6). This event (and the feeling of betrayal that many AIED researchers felt) was likely a major force in distancing researchers who identified more with the AIED community and those who identified with the ICLS community. However, it is important to acknowledge that aside from Schank’s role, there were many factors that led up to the formation of the learning sciences, including a growing interest in situativist accounts, in contrast to traditional information-processing approaches; a desire to design microworld-based systems, which were losing popularity in the AIED community; and a growing group of education researchers interested in the interdisciplinary study of learning who were not using AI methods (Kolodner, 2004 ). As such, it appears likely that some kind of learning sciences community would have formed had Schank not initiated it (but perhaps leaving less of a sour taste).
The International Conference of the Learning Sciences has continued to have a conference every other year since 1996. The learning sciences community as embodied in ICLS, largely attracted researchers coming from situativist and constructivist traditions. While some information-processing-oriented researchers also participated in the first few ICLS conferences and published papers in the Journal of the Learning Sciences , many of these researchers found the AIED community to be more closely aligned to their work (both methodologically and theoretically).
Using case-based reasoning as a theoretical underpinning, Schank and his students developed the idea of case-based teaching, which is premised on the ideas that (1) “experts are repositories of cases” (Schank & Jona, 1991 , p. 16) and that (2) “good teaching is good story telling” (Schank, 1990 , p. 231). This work led to designing a variety of interactive software where students are put into authentic problem-solving situations that are meant to be of inherent interest. When students need support, they can seek help, at which point the receive a story which they can hopefully apply when reasoning about similar situations in the future. However, Schank and Jona ( 1991 ) did not believe that all good teaching should be confined to using cases and stories; they also developed five other teaching architectures, including “simulation-based learning by doing” and “cascaded problem sets.” This work later led to the development of goal-based scenarios, which suggested that good teaching should involve a grounded goal that the student is trying to accomplish, such as creating a TV news program (Schank et al., 1994 ), identifying if a Rembrandt-style painting is authentic (Bain, 2004 ; Riesbeck, 1998 ), and figuring out why bats are dying in a zoo (Riesbeck, 1998 ). As Schank et al. ( 1994 ) explicitly admit, the idea that learning “takes place within some authentic activity” (p. 307) was supported by the newly burgeoning theories of situated cognition and cognitive apprenticeship (Brown et al., 1989 ). Indeed, Allan Collins, one of the pioneers of situated learning and cognitive apprenticeship, was a close colleague of Schank; together they cofounded the Cognitive Science journal and the Cognitive Science Society, and Schank hired him as a faculty member of the Institute for the Learning Sciences. Despite all the sophisticated AI methods that motivated the case-based teaching architecture and goal-based systems, according to Schank et al. ( 1994 ), “perhaps the simplest way to express the fundamental principle underlying our ideas about education” is “an interest is a terrible thing to waste” (p. 305).
In the 1990s, Roger Schank and the Institute for the Learning Sciences, were impressively productive in churning out a variety of interactive learning software based on the principles outlined above. However, the particular technologies they developed are virtually unknown today, and phrases such as “case-based teaching architecture” and “goal-based scenarios” are rarely used today. “Case-based learning” is still in use, but often in the context of using cases in medicine, law, and business schools, a form of instruction that predated Schank’s use of the term. However, as per Google Books Ngram Viewer (Michel et al., 2011 ), the term became popular in the late 1980s, so it seems likely that Schank and his colleagues played a role in popularizing the broader concept. Regardless, Schank’s legacy in education far outweighs these specific contributions; he helped spearhead the learning sciences, a movement that originally brought together a group of like-minded people who took similarly “scruffy” approaches to AI and education (in contrast to the approach of Newell, Simon, and their colleagues)—a movement that continues to grow to this day.
To the Present and Beyond
The narrative I have told begins with the birth of artificial intelligence and cognitive science in 1956 and traces how pioneers of the field from its conception (i.e., Simon, Newell, Minsky, and Michie) and pioneers who joined the field a few years later (e.g., Papert and Schank) played a key role in altering the course of research on learning and education to the present day. In some cases, their work left a theoretical legacy that influenced future generations of researchers (e.g., through the development of cognitivism as the dominant learning theory for decades in the case of Simon and Newell, and through the development of constructionism in the case of Papert). In other cases, these pioneers created educational technologies and conducted educationally relevant research themselves. In yet other cases, they helped established new fields within education (e.g., the learning sciences and, in some sense, learning engineering).
Moreover, these researchers largely led two strands of work in AI and education that developed in parallel: one strand pioneered by Simon, Newell, Anderson, and their colleagues, and another strand pioneered by Papert, Minsky, Schank, and their colleagues. Figure 1 depicts some of the key events in the histories of these two strands. In 1985, there was some hope of convergence; that the work from the information-processing/tutoring system strand and the work from the constructivist/learning environment strand would “come together,” as Lawler and Yazdani ( 1987 ) stated in the aforementioned quote. After all, Simon, Newell, Papert, and Minsky seemed to get along just fine in the world of artificial intelligence. The 1985 conference seemed to bring together people who were interested in how children and machines think, and who were interested in foundational questions at the intersection of learning, knowledge representation, and technology. This is evident by scanning the proceedings’ table of contents, with papers titled “Knowledge Acquisition as a Social Phenomenon,” “The Schema Mechanism – Translating Piaget to LISP,” “The Epistemics of Computer Based Microworlds,” “The Role of Cognitive Theory in Educational Reform,” and “Observational Learning by Computer.”
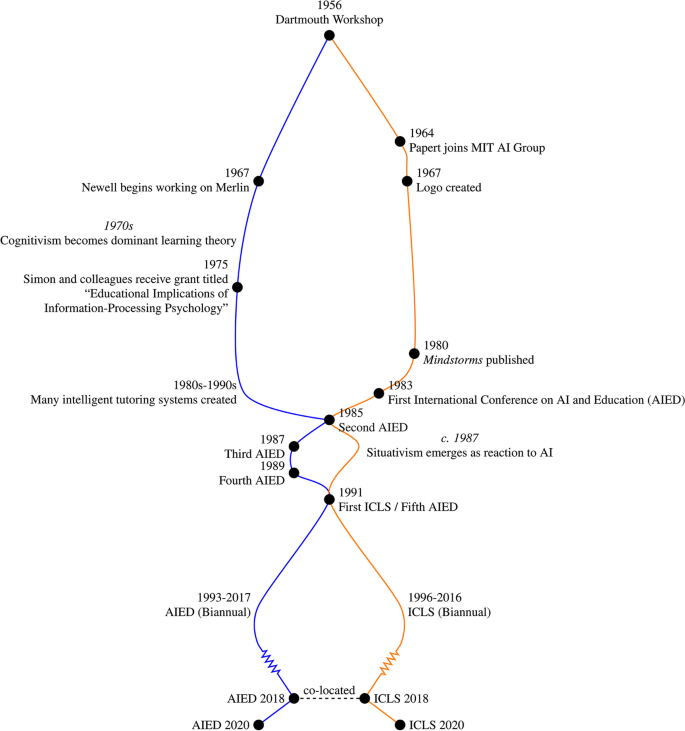
Parallel timelines for two strands within the intertwined history of AI and education. The timeline on the left shows events aligned with the information-processing strand and AIED, while the timeline on the right shows events aligned with the constructivist strand, situativism, and ICLS. The vertical axis follows a linear timescale, except for the period from 1991 to 2018, where twelve years are skipped as indicated by the zigzag patterns. The horizontal axis very loosely represents the “distance” between the two strands from each other over time; points of intersection indicates points at which the two strands were intellectually or physically in contact with one another (e.g., the 1985 Artificial Intelligence and Education conference or the 1991 ICLS/AIED conference)
But the two strands seemed to grow further apart over the next few years. In 1991, when Schank established the learning sciences and coopted the existing International Conference of Artificial Intelligence and Education to bootstrap the formation of the emerging field, there might have been hope for a convergence of the two fields again. However, at that point the two fields seemingly found that they had different interests, and Schank’s attempt to change the conference did not help. Moreover, with the emergence of situativism around 1987, many researchers became disgruntled with the inability of AI to model the kinds of learning that take place in the real world (e.g., learning that is context-dependent and inherently social). The burgeoning field of the learning sciences was more attuned to these concerns than the field of AIED (i.e., AI in Education). Footnote 12 As such, the learning sciences had to open its doors to new approaches taken by researchers coming from more situativist and sociocultural perspectives, including qualitative methods like ethnography, and over time the learning sciences started steering further away from its AI roots.
In 2018, for the first time since 1991, the two conferences of ICLS and AIED were co-located in what was called the “Festival of Learning” in London. The two conferences chose themes that would reflect the intersection of the two fields. The ICLS 2018 theme was “Rethinking learning in the digital age: Making the Learning Sciences count” and the AIED 2018 theme was “Bridging the Behavioral and the Computational: Deep Learning in Humans and Machine.” The AIED theme in particular shows a return to the original draw towards research on artificial intelligence and education: “learning in humans and machine,” but now focusing on the AI of the times—deep learning. However, a quick scan of the conference proceedings of these two conferences shows that (a) the two fields had grown further apart over the past three decades, and (b) neither conference seemed to be focusing on the intertwined and mutually reinforcing questions that fueled AI and education research in earlier decades. Despite the conference theme, most of the work presented at AIED 2018 was not trying to bridge between human and machine learning. Rather there were many papers on using machine learning, computer vision, and natural language processing in service of improving educational technologies or gaining insights on learning in particular educational settings, as well as empirical studies that evaluate the efficacy of AIED technologies. In short, by this point the community was fully invested in applying state-of-the-art AI (mostly machine learning) in service of education.
One rare exception to this trend was Tuomi’s ( 2018 ) paper that was provocatively titled, “Vygotsky Meets Backpropagation: Artificial Neural Models and the Development of Higher Forms of Thought.” This paper actually addressed the conference theme of comparing learning in humans and neural networks, showing that a particular state-of-the-art neural network could not accurately learn concepts in the way that humans do, as outlined in the work of Russian developmental psychologists like Lev Vygotsky. This work suggests that perhaps deeper connections between AI and education may still be pursued in the age of deep learning, but such work is an outlier. It appears that this paper has been largely ignored and probably thought of more as an intellectual thought experiment rather than an interesting line of inquiry to continue pursuing within AIED.
Furthermore, from 2019 to 2021, IJAIED papers have also seemingly focused on the kinds of studies mentioned above: “applying state-of-the-art AI (mostly machine learning) in service of education.” At least from the titles, I could identify almost no papers that tackle the kind of interdisciplinary approach to human and machine learning that has been the focus of this historical review. One possible exception is a paper on using Apprentice Learner models for interactively creating tutoring systems using feedback and demonstrations (MacLellan & Koedinger, 2022 ). While this paper still has an applied focus—more efficient ITS authoring—work on Apprentice Learner models in general (MacLellan, et al., 2016 ), and earlier work on SimStudent (Li et al., 2015 ; Matsuda et al., 2013 ), draw on the cognitive science tradition of using computational models of learning to develop insights on how people learn and to make practical changes in educational technology. Moreover, such work could also contribute back to the AI literature (Li et al., 2015 ).
Having attended the “Festival of Learning,” I can also anecdotally claim that the overall sentiment seemed to be that the two conferences were quite different from one another with little overlap in the questions studied and methods used. AIED researchers found ICLS to be too focused on qualitative case studies that lacked the rigor, precision, and generalizability of AIED studies. ICLS researchers likely found AIED to be too focused on technologies that target limited forms of teaching and learning. In 2020, the ICLS theme was “Interdisciplinarity in the Learning Sciences.” Yet the call for papers did not list any technical fields (such as computer science or artificial intelligence) in the list of fields that the conference was hoping to elicit contributions from.
Nonetheless, there is reason to believe that these fields could still unite. In 2016, the International Alliance to Advance Learning in the Digital Era (IAALDE) formed as an umbrella organization that encompasses ten different research societies committed to the study of learning in a technologically advanced world; ISLS and AIED are both part of this organization. IAALDE could strike meaningful dialogue across these societies, but these conversations might be more productive if points of common ground are clearly laid out. One path forward is to acknowledge the intertwined nature of early AI and education research that fueled both the early learning sciences and AIED communities.
In a talk that Papert gave in 2002, he remarked on how he “misses the good old days of ‘big ideas’ about the nature of knowledge and human learning” (as cited in Wright, 2002 ). As Papert put it,
We started with a big ‘cosmic question’: Can we make a machine to rival human intelligence? Can we make a machine so we can understand intelligence in general? But AI was a victim of its own worldly success. People discovered you could make computer programs so robots could assemble cars. Robots could do accounting! (as cited in Wright, 2002 )
Surely, Papert would agree that the learning sciences had also strayed from its roots in thinking about the “cosmic question” of understanding intelligence and learning in humans and machines. Is there still room today for a learning science that draws insights from artificial intelligence and simultaneously makes contributions to the study of thinking and learning in machines? Could the learning sciences return to their AI roots? Could the AIED community return to thinking about more central questions at the intersection of artificial intelligence and education? To answer these questions it may help to gain a better understanding of the ethos that pervaded the 1985 and 1991 AIED conferences or to take a closer look at the interdisciplinary work of the pioneers described above. That is beyond the scope of this paper. But if the AIED community is to return to its AI roots, it may depend on one of two things: either (a) the community puts a greater focus on early AI techniques, such as symbolic AI and the scruffier work of Papert, Minsky, and Schank; and/or (b) the community investigates the connections between machine learning techniques and human learning. As an example of (a), Porayska-Pomsta ( 2016 ) investigates how the use of knowledge representation and knowledge elicitation techniques from AI could inform teacher’s metacognitive reflection on their own practice. Resonant with the key idea I am presenting here, Porayska-Pomsta ( 2016 ) suggests that this work.
allows us to see AI not solely, albeit importantly, as the driver of back-end functionality of AIEd technologies (e.g. Bundy 1986), but equally as a front-end technology - of - the - mind through which educators can represent, experiment with and compare their practices at a fine-grained level of detail and engage in predictive analyses of the potential impact of their actions on individual learners. (p. 681)
One example of (b) is Tuomi’s ( 2018 ) work on comparing neural networks with Vygotksy’s theory of cognitive development. An emerging research community focused on “machine teaching” is also interested in investigating how to optimally teach machine learning algorithms and the implications that this might have on teaching human learners (Zhu, 2015 ). Whether such efforts will lead to powerful contributions to the fields of AI and education remains to be seen.
For the ISLS community to return to its AI roots, it seems like another question needs to be addressed: can researchers develop connections between AI and sociocultural theories of learning? There seems to be very little investigation in this direction in recent years, perhaps in part because most ISLS researchers are no longer typically trained in AI techniques. However, a look at the pioneers of situativism and other sociocultural theories of learning shows that this question may not be so far-fetched. Greeno, one of the foremost advocates of the situative perspective, advocated for continuing efforts on computational modeling in developing situative accounts of learning, but ones that could account for multiagent interactions (Greeno & Moore, 1993 ). Edwin Hutchins, one of the founders of distributed cognition, conducted a series of early studies on agent-based models (where each agent was described using connectionist models) that could describe learning as a cultural process (Hutchins & Hazlehurst, 1991 , 1995 ). diSessa ( 1993 ) proposed a connectionist model to describe how conceptual change can occur in terms of his popular knowledge-in-pieces framework. Social scientist Kathleen Carley’s ( 1986 ) paper “Knowledge Acquisition as a Social Phenomenon” presented a sophisticated theory and model that juxtaposed an AI-based knowledge representation (akin to frames or scripts) with a social network seeded with ethnographic data; a version of this paper was presented at the 1985 AIED conference. Despite these lines of inquiry being proposed by leaders in the field, seemingly none of them have been influential directions in the learning sciences. Perhaps following up on such lines of inquiry would be one way to bridge between the ICLS and AIED communities, by drawing on theories from the learning sciences and methods from artificial intelligence.
None of this is to suggest that existing work in AIED or the learning sciences is less important than investigating questions at the interface of AI and education. These different styles of work need not be seen as being in conflict with one another. In fact, even if the day-to-day work of most researchers in AIED and the learning sciences does not change, periodically revisiting big “cosmic questions” around how to improve our understanding of the nature of learning and how that can improve education can help ensure more incremental work is moving in the “right” direction. Footnote 13
By advancing AI models to bring in insights from how people learn in a variety of educational environments, this work can potentially advance foundational work in AI as well. This could perhaps even push AI to re-consider the relevance of older theories that have been displaced in an era of deep learning. Moreover, the focus of AI (and the history presented in this paper) has primarily been on cognition and the cognitive aspects of learning; however, as AIED researchers have emphasized in recent years, education goes beyond the cognitive, with phenomena like metacognition, affect, and motivation playing important roles in learning (see e.g., Arroyo et al., 2014 ; Poryaska-Pomsta, 2016 ; Rebolledo-Mendez et al., 2022 ; Winne, 2021 ). Motivated by human learning, recent work in machine learning has begun to design learning algorithms that include metacognition (Savitha et al., 2014 ; Zhang & Er, 2016 ) or intrinsic motivation (Baldassarre et al., 2013 ; Barto & Simsek, 2005 ; Shuvaev et al., 2021 ). However, such work is primarily motivated by psychology and neuroscience, and does not consider what education might have to say about these phenomena. The AIED community could be in a unique position to consider educationally-relevant aspects of extra-cognitive factors in the design of AI models and in the use of those models to understand and improve how people learn.
I have given pointers for what it might take for the AIED, ICLS, and AI communities to return to an interdisciplinary investigation of learning in humans and machines. But it may still be hard to imagine what a future where such work is commonplace would look like. The past has given us many examples of this work, but we cannot expect to completely return to an older ethos, given the changes in the research communities involved, advances in technology, and changes in how we think about learning and teaching. Instead, it might be worthwhile to speculate about the kind of work we might see in the future. To that end, I offer several titles of completely hypothetical research papers that reflect the kind of interdisciplinary thinking that has been at the core of this paper:
Embedding Socio-Cultural Constraints into Knowledge Spaces
Machine Teaching vs. Active Learning: Comparing the Efficacy of Learning Algorithms in a Tutorial Environment vs. a Microworld
A Montessori-Inspired Approach to Regularizing Neural Networks
A Computational Model of Distributed Cognition for a Museum Learning Environment
Can You Fool the Computer? Designing an Adversarial Teachable Agent Based on Generative Adversarial Networks
Thinking About Thinking: Using AI Models to Foster Metacognitive Reflection in an Inner-City School
Of course, the previous paragraphs presuppose that it is worthwhile to reinvigorate the role that AI once had in education research. Some might suggest that whether or not this could be done, it is not a worthwhile endeavor. Regardless, it seems reasonable to think that to give a thoughtful answer to either the question of whether these communities can return to their AI roots or the question of whether they should , we must have a more accurate picture of the history of education research, including the ways in which artificial intelligence has interacted with this history. My hope is that this paper can help researchers have a more nuanced understanding of the history of research on learning in humans and machines, in order to make more informed decisions about the directions these fields should take going forward.
On the education side, much of my focus is specifically on educational psychology, and in particular, the learning sciences, broadly conceived. However, I often use the much broader labeling of “education research” or even “education,” because the history described here at times had far-reaching consequences on education research—and, at times, even educational practice—especially to the extent that learning theories influenced broader educational thought.
The “sanctioned” history of AI is typically said to begin with the Dartmouth Workshop in 1956, discussed below. However, both prior to that time and after that time, other fields and activities have existed that were working on similar problems and/or ones that would later get adopted by mainstream AI. Cybernetics is one such field that predates AI. The interdisciplinary study of human and machine learning described here was also present in cybernetics. Although cyberneticians were also working in education, their work has not had as obvious of an impact on education as that of the AI researchers discussed here. A full treatment of the intertwined history of cybernetics and education is worthwhile, but beyond the scope of this paper.
Interestingly, Lagemann ( 2002 ) does acknowledge the influence of Herbert Simon’s earlier work (prior to AI) on educational administration. I do not discuss that here, as it is outside the scope of this paper, but it is worth keeping in mind that Simon’s work influenced other areas of education as well.
Although tangential to this history, it is interesting to note that both Russell and Whitehead, who were mathematicians and philosophers, also published texts on the philosophy of education, Russell’s On Education, Especially in Early Childhood and Whitehead’s The Aims of Education and Other Essays.
Green ( 2016 ) conducted a citation analysis of the most cited books in the social sciences according to Google Scholar in 2016, though as far as I can tell, these rankings still hold.
I thank Cynthia Solomon for sharing a draft of the "Teaching Children Thinking" symposium press release and schedule from Marvin Minsky's personal collection, courtesy of the Minsky Family.
This list includes Cynthia Solomon, Andrea diSessa, David Perkins, Barbara White, Robert Lawler, Idit Harel, Yasmin Kafai, Ricki Goldman, Mitchel Resnick, Uri Wilensky, Gary Stager, Alan Shaw, Paula Hooper, David Williamson Shaffer, Marina Umaschi Bers, and Claudia Urrea.
Paulo Freire’s Pedagogy of the Oppressed , published in Portuguese in 1968, is the most cited book in education (Green, 2016 ).
It was actually called the World Conference on Artificial Intelligence in Education until 1997 and International Conference on Artificial Intelligence in Education since 1999.
This is not to suggest that the new name was necessarily explicitly chosen for this reason. However, it is worth noting that Tim O’Shea and John Self had co-authored a book in 1983 called “Learning and Teaching with Computers: Artificial Intelligence in Education” (Self, 2016 ). Even though Self recognized the interdisciplinary interplay between AI and education (as quoted above), perhaps he (and others) had an affinity towards the phrasing “artificial intelligence in education.” This affinity likely reflected the growing interest in using AI-based technologies (like intelligent tutoring systems) to enhance education. Regardless of why the new name was chosen, the name made sense for the evolving interests of the field.
I thank three anonymous reviewers who corroborated this account (including the feeling of AIED researchers at the time) and provided additional details based on their own witnessing of the events.
This is not to say that situativism was seen as mutually incompatible with AIED by all. For example, John Seely Brown, one of the pioneers of the situated learning and an early ITS researcher, wrote a thought-provoking chapter called “Toward a new epistemology for learning” for how work on intelligent tutoring systems could (and should) embrace the situative perspective (Brown, 1990 ). Indeed, echoing one of the themes of this historical review, Brown went so far as to say:
“it is this community that is most closely coupled to—or situated in—the full blooded complexity of human learning activity. Thus if we meet this challenge correctly, it may well be that, instead of ITS being merely one subset of the overall schema of AI, we will, instead, find that it is AI that becomes one subset of the overall schema of ITS.” (p. 281).
While some researchers may have been intrigued by this call to action, it appears the ITS/AIED community did not “meet this challenge correctly.”.
I thank an anonymous reviewer for bringing this idea to my attention.
Abelson, H., & diSessa, A. (1986). Turtle geometry: The computer as a medium for exploring mathematics . MIT Press. https://doi.org/10.7551/mitpress/6933.001.0001
Abelson, R. P. (1981). Constraint, construal and cognitive science. In Proceedings of the Third Annual Conference of the Cognitive Science Society . https://cognitivesciencesociety.org/wp-content/uploads/2019/01/cogsci_3.pdf
American Psychological Association. (1995). John R. Anderson. American Psychologist , 50 , 213–215.
Anderson, J. R., Boyle, C. F., & Yost, G. (1985). The geometry tutor. In Proceedings of the Ninth International Joint Conference on Artificial Intelligence (I) (pp. 1–7). IJCAI Organization. https://www.ijcai.org/Proceedings/85-1/Papers/001.pdf
Anderson, J. R., Greeno, J. G., Reder, L. M., & Simon, H. A. (2000). Perspectives on learning, thinking, and activity. Educational Researcher , 29 (4), 11–13. https://doi.org/10.3102/0013189X029004011
Anderson, J. R., Reder, L. M., & Simon, H. A. (1996). Situated learning and education. Educational Researcher , 25 (4), 5–11. https://doi.org/10.3102/0013189X025004005
Anderson, J. R., Reder, L. M., & Simon, H. A. (1997). Situative versus cognitive perspectives: Form versus substance. Educational Researcher , 26 (1), 18–21. https://doi.org/10.3102/0013189X026001018
Anderson, J. R., Reder, L. M., & Simon, H. A. (1999). Applications and misapplications of cognitive psychology to mathematics education. http://act-r.psy.cmu.edu/papers/misapplied.html
Anderson, J. R., Reder, L. M., Simon, H. A., Ericsson, K. A., & Glaser, R. (1998). Radical constructivism and cognitive psychology. Brookings Papers on Education Policy , 1 , 227–278. http://www.jstor.org/stable/20067198
Annett, J. (1976). Computer assisted learning, 1969–1975: A report prepared for SSRC . Social Science Research Council.
Arroyo, I., Woolf, B. P., Burelson, W., Muldner, K., Rai, D., & Tai, M. (2014). A multimedia adaptive tutoring system for mathematics that addresses cognition, metacognition and affect. International Journal of Artificial Intelligence in Education , 24 (4), 387–426. https://doi.org/10.1007/s40593-014-0023-y
Bain, K. (2004). What the best college teachers do . Harvard University Press. https://doi.org/10.2307/j.ctvjnrvvb
Baldassarre, G., & Mirolli, M. (Eds.). (2013). Intrinsically motivated learning in natural and artificial systems . Springer. https://doi.org/10.1007/978-3-642-32375-1
Barto, A. G., & Simsek, O. (2005). Intrinsic motivation for reinforcement learning systems. In Proceedings of the Thirteenth Yale Workshop on Adaptive and Learning Systems (pp. 113–118).
Bhattacharjee, Y. (2009). A personal tutor for algebra. Science , 323 (5910), 64–65. https://doi.org/10.1126/science.323.5910.64
Brockman, J. (1996). Third culture: Beyond the scientific revolution . Simon and Schuster.
Brown, J. S. (1973). Steps toward automatic theory formation. In Proceedings of the Third International Joint Conference on Artificial Intelligence (pp. 121–129). IJCAI Organization. https://ijcai.org/Proceedings/73/Papers/014.pdf
Brown, J. S. (1990). Toward a new epistemology for learning. In C. Frasson & G. Gauthier. (Eds.), Intelligent tutoring systems: At the crossroad of artificial intelligence and education , 266–282. Intellect Books.
Brown, J. S., Burton, R., Miller, M., deKleer, J., Purcell, S., Hausmann, C., & Bobrow, R. (1975a). Steps toward a theoretical foundation for complex, knowledge-based CAI. ERIC. https://eric.ed.gov/?id=ED135365
Brown, J. S., Burton, R. R., & Bell, A. G. (1975b). Sophie: A step toward creating a reactive learning environment. International Journal of ManMachine Studies , 7 (5), 675–696. https://doi.org/10.1016/S00207373(75)800265
Brown, J. S., Collins, A., & Duguid, P. (1989). Situated cognition and the culture of learning. Educational Researcher , 18 (1), 32–42. https://doi.org/10.1207/s1532690xci0403_1
Carbonell, J. R., & Collins, A. M. (1973). Natural semantics in artificial intelligence. In Proceedings of the Third International Joint Conference on Artificial Intelligence (pp. 344–351). IJCAI Organization. https://www.ijcai.org/Proceedings/73/Papers/036.pdf
Carley, K. (1986). Knowledge acquisition as a social phenomenon. Instructional Science , 14 (3), 381–438. https://doi.org/10.1007/BF00051829
Clark, A., & Chalmers, D. (1998). The extended mind. Analysis , 58 (1), 7–19. https://doi.org/10.1111/14678284.00096
De Kleer, J., & Brown, J. S. (1984). A qualitative physics based on confluences. Artificial Intelligence , 24 (1–3), 7–83. https://doi.org/10.1016/00043702(84)900377
diSessa, A. A. (1993). Toward an epistemology of physics. Cognition and Instruction , 10 (2–3), 105–225. https://doi.org/10.1080/07370008.1985.9649008
Doroudi, S. (2020). The bias-variance tradeoff: How data science can inform educational debates. AERA Open , 6 (4). https://doi.org/10.1177/2332858420977208
Dreyfus, H. L. (1965). Alchemy and artificial intelligence (Tech. Rep.). RAND Corporation.
Ericsson, K. A., & Simon, H. A. (1980). Verbal reports as data. Psychological Review , 87 (3), 215. https://doi.org/10.1037/0033295X.87.3.215
Gardner, H. (1987). The mind’s new science: A history of the cognitive revolution . Basic books.
Goldstein, I., & Papert, S. (1977). Artificial intelligence, language, and the study of knowledge. Cognitive Science , 1 (1), 84–123. https://doi.org/10.1016/S03640213(77)800062
Green, E. D. (2016, May 12). What are the most-cited publications in the social sciences (according to Google Scholar)? LSE Impact Blog. https://blogs.lse.ac.uk/impactofsocialsciences/2016/05/12/what-are-the-most-cited-publications-in-the-social-sciences-according-to-google-scholar/
Greeno, J. G. (1997). On claims that answer the wrong questions. Educational Researcher , 26 (1), 5–17. https://doi.org/10.3102/0013189X026001005
Greeno, J. G., & Moore, J. L. (1993). Situativity and symbols: Response to Vera and Simon. Cognitive Science , 17 (1), 49–59. https://doi.org/10.1207/s15516709cog1701_3
Haugeland, J. (1989). Artificial intelligence: The very idea . MIT Press.
Hayes, J. R. (1996). [Letter to Herbert A. Simon] . Herbert Simon Collection (Box 23, Folder 1596), University Libraries Digital Collections, Carnegie Mellon University.
Hoadley, C. (2018). A short history of the learning sciences. In F. Fischer, C. E. HmeloSilver, S. R. Goldman, & P. Reimann (Eds.), International handbook of the learning sciences (pp. 11–23). Routledge. https://doi.org/10.4324/9781315617572
Howe, J. A. M. (1978). Artificial intelligence and computer-assisted learning: Ten years on. Programmed Learning and Educational Technology, 15 (2), 114–125. https://doi.org/10.1080/0033039780150204
Article Google Scholar
Human-Computer Learning Foundation. (n.d.). Human-computer learning foundation. Retrieved September 22, 2022, from https://www.aiai.ed.ac.uk/~dm/hclf.html
Hutchins, E., & Hazlehurst, B. (1991). Learning in the cultural process. In Artificial life II. SFI studies in the sciences of complexity (Vol. 10, pp. 689–706). Addison Wesley.
Hutchins, E., & Hazlehurst, B. (1995). How to invent a lexicon: the development of shared symbols in interaction. In Artificial societies: The computer simulation of social life (pp. 157–189). UCL Press. https://doi.org/10.4324/9780203993699
Hutchins, E., et al. (1990). The technology of team navigation. Intellectual Teamwork: Social and Technological Foundations of Cooperative Work, 1 , 191–220.
Google Scholar
International Artificial Intelligence in Education Society. (n.d.). About IAIED . Retrieved September 22, 2022, from https://iaied.org/about/
Johnson, M. (1989). Embodied knowledge. Curriculum Inquiry , 19 (4), 361–377. https://doi.org/10.1080/03626784.1989.11075338
Journal of the Learning Sciences. (1991). Front matter. The Journal of the Learning Sciences , 1 (1). http://www.jstor.org/stable/1466653
Koedinger, K. R., & Anderson, J. R. (1990, March). Theoretical and empirical motivations for the design of ANGLE: A New Geometry Learning Environment. In Working Notes of the 1990 AAAI Spring Symposia on Knowledge-Based Environments for Learning and Teaching, Stanford University, March (pp. 27–29).
Kolodner, J. L. (2002). The “neat” and the “scruffy” in promoting learning from analogy: We need to pay attention to both. The Journal of the Learning Sciences, 11 (1), 139–152. https://doi.org/10.1207/S15327809JLS1101_7
Kolodner, J. L. (2004). The learning sciences: Past, present, future. Educational Technology , 44 (3), 34–40. https://www.jstor.org/stable/44428906
Lagemann, E. C. (2002). An elusive science: The troubling history of education research . University of Chicago Press.
Laird, J. E., & Rosenbloom, P. S. (1992). In pursuit of mind: The research of Allen Newell. AI Magazine, 13 (4), 17–17. https://doi.org/10.1609/aimag.v13i4.1019
Lave, J., & Wenger, E. (1991). Situated learning: Legitimate peripheral participation . Cambridge University Press. https://doi.org/10.1017/CBO9780511815355
Lawler, R., & Yazdani, M. (1987). Artificial intelligence and education: Learning environments and tutoring systems (Vol. 1). Intellect Books.
Lee, V. (2017). A short history of the learning sciences. In R. E. West (Ed.), Foundations of learning and instructional design technology. Pressbooks. https://lidtfoundations.pressbooks.com/chapter/learning-sciences-by-victor-lee/
Lenat, D. B., & Brown, J. S. (1984). Why AM and EURISKO appear to work. Artificial Intelligence , 23 (3), 269–294. https://doi.org/10.1016/00043702(84)90016X
Li, N., Matsuda, N., Cohen, W. W., & Koedinger, K. R. (2015). Integrating representation learning and skill learning in a human-like intelligent agent. Artificial Intelligence, 219 , 67–91. https://doi.org/10.1016/j.artint.2014.11.002
Liffick, B. W. (1987). The Third International Conference on Artificial Intelligence and Education. AI Magazine , 8 (4), 97–97. https://doi.org/10.1609/aimag.v8i4.627
Longuet-Higgins, H. C. (1973). Comments on the Lighthill report and the Sutherland reply. In Artificial Intelligence: A Paper Symposium (pp. 35–37). Science Research Council. http://www.chilton-computing.org.uk/inf/literature/reports/lighthill_report/p004.htm
MacLellan, C. J., Harpstead, E., Patel, R., & Koedinger, K. R. (2016). The Apprentice Learner architecture: Closing the loop between learning theory and educational data. In T. Barnes, M. Chi, & M. Feng (Eds.), Proceedings of the 9th International Conference on Educational Data Mining (pp. 151–158). International Educational Data Mining Society.
MacLellan, C. J., & Koedinger, K. R. (2022). Domain-general tutor authoring with apprentice learner models. International Journal of Artificial Intelligence in Education , 32 (1), 76–117. https://doi.org/10.1007/s40593-020-00214-2
Matsuda, N., Yarzebinski, E., Keiser, V., Raizada, R., Cohen, W. W., Stylianides, G. J., & Koedinger, K. R. (2013). Cognitive anatomy of tutor learning: Lessons learned with SimStudent. Journal of Educational Psychology, 105 (4), 1152. https://doi.org/10.1037/a0031955
McCorduck, P. (2004). Machines who think: A personal inquiry into the history and prospects of artificial intelligence (2nd ed.). A K Peters/CRC Press. https://doi.org/10.1201/9780429258985
McCulloch, W. S., & Pitts, W. (1943). A logical calculus of the ideas immanent in nervous activity. The Bulletin of Mathematical Biophysics, 5 (4), 115–133. https://doi.org/10.1007/BF02478259
Article MathSciNet Google Scholar
Michel, J.B., Shen, Y. K., Aiden, A. P., Veres, A., Gray, M. K., Team, G. B., … et al. (2011). Quantitative analysis of culture using millions of digitized books. Science , 331 (6014), 176–182. https://doi.org/10.1126/science.1199644
Michie, D. & Bain, M. (1989) Machines that learn and machines that teach. In Jaakkola, H., & Linnainmaa, S. (Eds.), Scandinavian Conference on Artificial Intelligence 89: Proceedings of the SCAI'89 (pp. 1–25). IOS Press.
Michie, D., Paterson, A., & Michie, J. H. (1989). Learning by teaching. In Jaakkola, H., & Linnainmaa, S. (Eds.), Scandinavian Conference on Artificial Intelligence 89: Proceedings of the SCAI'89 (pp. 307–331). IOS Press.
Minsky, M. (1974). A framework for representing knowledge. MIT Artificial Intelligence Laboratory Memo , 306. http://hdl.handle.net/1721.1/6089
Minsky, M. (1977). Plain talk about neurodevelopmental epistemology. In Proceedings of the Fifth International Joint Conference on Artificial Intelligence (II) (pp. 1083–1092). IJCAI Organization. https://www.ijcai.org/Proceedings/77-2/Papers/098.pdf
Minsky, M. (1988). The society of mind . Simon and Schuster.
Minsky, M. (2019). In C. Solomon (Ed.), Inventive minds: Marvin Minsky on education . MIT Press. https://doi.org/10.7551/mitpress/11558.001.0001
Minsky, M., & Papert, S. (presumed). (1970). Teaching children thinking [Unpublished draft of symposium press release and schedule]. Copy in possession of Cynthia Solomon.
Minsky, M., & Papert, S. (1972). Artificial intelligence progress report . MIT Artificial Intelligence Laboratory Memo , 252. https://dspace.mit.edu/handle/1721.1/6087
Minsky, M., & Papert, S. (1988). Perceptrons: Introduction to computational geometry, expanded edition. MIT Press. https://doi.org/10.7551/mitpress/11301.001.0001
Moore, J., & Newell, A. (1974). How can Merlin understand? In L. W. Gregg (Ed.), Knowledge and Cognition. Psychology Press.
Nathan, M. J., Koedinger, K. R., & Alibali, M. W. (2001). Expert blind spot: When content knowledge eclipses pedagogical content knowledge. In Proceeding of the Third International Conference on Cognitive Science (pp. 644–648). USTC Press. https://website.education.wisc.edu/mnathan/Publications_files/2001_NathanEtAl_ICCS_EBS.pdf
Newell, A. (1969). A step toward the understanding of information processes. (book reviews: Perceptrons . An introduction to computational geometry). Science , 165 , 780–782. https://doi.org/10.1126/science.165.3895.780
Newell, A. (1970). What are the intellectual operations required for a meaningful teaching agent? Allen Newell Collection (Box 28, Folder 1940). University Libraries Digital Collections.
Newell, A. (1973). Production systems: Models of control structures. In W. G. Chase (Ed.), Visual information processing (pp. 463–526). Elsevier.
Chapter Google Scholar
Newell, A. (1994). Unified theories of cognition . Harvard University Press.
Newell, A., Shaw, J. C., & Simon, H. A. (1958). Elements of a theory of human problem solving. Psychological Review , 65 (3), 151. https://doi.org/10.1037/h0048495
Newell, A., & Simon, H. A. (1972). Human problem solving . Prentice Hall.
Nilsson, N. J. (2009). The quest for artificial intelligence . Cambridge University Press.
Nwana, H. S. (1990). Intelligent tutoring systems: An overview. Artificial Intelligence Review , 4 (4), 251–277. https://doi.org/10.1007/BF00168958
Olazaran, M. (1996). A sociological study of the official history of the perceptrons controversy. Social Studies of Science , 26 (3), 611–659. https://doi.org/10.1177/030631296026003005
Papert, S. (1968). The artificial intelligence of Hubert L. Dreyfus: A budget of fallacies (Tech. Rep.). https://dspace.mit.edu/bitstream/handle/1721.1/6084/AIM-154.pdf
Papert, S. (1976). Some poetic and social criteria for education design (Tech. Rep.). https://dspace.mit.edu/bitstream/handle/1721.1/6250/AIM-373.pdf
Papert, S. (1980). Mindstorms: Children, computers, and powerful ideas . Basic Books, Inc.
Papert, S. (1987a). Computer criticism vs. technocentric thinking. Educational Researcher , 16 (1), 22–30. https://doi.org/10.3102/0013189X016001022
Papert, S. (1987b). Microworlds: transforming education. In Artificial Intelligence and Education (Vol. 1, pp. 79–94).
Papert, S. (1993). The children’s machine: Rethinking school in the age of the computer. Basic Books, Inc.
Pask, G. (1972). Anti-Hodmanship: A Report on the State and Prospects of CAI. Programmed Learning and Educational Technology , 9 (5), 235–244. https://doi.org/10.1080/1355800720090502
Pea, R. (2016). The prehistory of the learning sciences . Cambridge University Press. https://doi.org/10.1017/CBO9781107707221.003
Book Google Scholar
Porayska-Pomsta, K. (2016). AI as a methodology for supporting educational praxis and teacher metacognition. International Journal of Artificial Intelligence in Education, 26 (2), 679–700. https://doi.org/10.1007/s40593-016-0101-4
Quartz, S. R. (1999). The constructivist brain. Trends in Cognitive Sciences , 3 (2), 48–57. https://doi.org/10.1016/S13646613(98)012704
Ramani, S., & Newell, A. (1973). On the generation of problems (Tech. Rep.). Carnegie Mellon University Department of Computer Science. https://kilthub.cmu.edu/articles/journal_contribution/On_the_generation_of_problems/6607970/1
Rebolledo-Mendez, G., Huerta-Pacheco, N. S., Baker, R. S., & du Boulay, B. (2022). Meta-affective behaviour within an intelligent tutoring system for mathematics. International Journal of Artificial Intelligence in Education , 32 (1), 174–195. https://doi.org/10.1007/s40593-021-00247-1
Reif, F., & Simon, H. A. (1994). [Email correspondence between Frederick Reif and Herbert A. Simon] . Herbert Simon Collection (Box 22, Folder 1548), University Libraries Digital Collections, Carnegie Mellon University.
Resnick, L. B. (1987). The 1987 presidential address: Learning in school and out. Educational Researcher , 16 (9), 13–54. https://doi.org/10.3102/0013189X016009013
Riesbeck, C. K. (1998). Indie: List of projects. Retrieved September 22, 2022, from https://users.cs.northwestern.edu/~riesbeck/indie/projects.html
Riesbeck, C. K., & Schank, R. C. (1989). Inside case-based reasoning . Psychology Press. https://doi.org/10.4324/9780203781821
Salomon, G. (1993). Distributed cognitions: Psychological and educational considerations . Cambridge University Press.
Sandberg, J. A. (1987). The third international conference on artificial intelligence and education. AI Communications , 1 , 51–53. https://doi.org/10.3233/AIC19870110
Savitha, R., Suresh, S., & Kim, H. J. (2014). A meta-cognitive learning algorithm for an extreme learning machine classifier. Cognitive Computation, 6 (2), 253–263. https://doi.org/10.1007/s12559-013-9223-2
Schank, R. C. (1969). A conceptual dependency representation for a computeroriented semantics [Doctoral dissertation, The University of Texas at Austin]. ProQuest Dissertations & Theses Global. https://www.proquest.com/pqdtglobal/docview/302479013/D26CEC566AF9466CPQ
Schank, R. C. (1972). Conceptual dependency: A theory of natural language understanding. Cognitive Psychology , 3 (4), 552–631. https://doi.org/10.1016/00100285(72)900229
Schank, R. C. (1982). Dynamic memory: A theory of reminding and learning in computers and people . Cambridge University Press.
Schank, R. C. (1983). The current state of AI: One man’s opinion. AI Magazine , 4 (1), 3. https://doi.org/10.1609/aimag.v4i1.382
Schank, R. C. (1986). Thinking about computers and thinking: A response to papert and his critics. New Ideas in Psychology , 4 (2), 231–239. https://doi.org/10.1016/0732118X(86)900140
Schank, R. C. (1990). Case-based teaching: Four experiences in educational software design. Interactive Learning Environments, 1 (4), 231–253. https://doi.org/10.1080/104948290010401
Schank, R. C. (2016). Why learning sciences? Cambridge University Press. https://doi.org/10.1017/CBO9781107707221.002
Schank, R. C., & Abelson, R. P. (1975). Scripts, plans, and knowledge. In Proceedings of the Fourth International Joint Conference on Artificial Intelligence (pp. 151–157).
Schank, R. C., Fano, A., Bell, B., & Jona, M. (1994). The design of goal-based scenarios. The Journal of the Learning Sciences, 3 (4), 305–345. https://doi.org/10.1207/s15327809jls0304_2
Schank, R. C., & Jona, M. Y. (1991). Empowering the student: New perspectives on the design of teaching systems. The Journal of the Learning Sciences , 1 (1), 7–35. https://doi.org/10.1207/s15327809jls0101_2
Schank, R. C., & Jona, M. Y. (1994). Issues for psychology, ai, and education: A review of newell’s unified theories of cognition . The MIT Press Cambridge. https://doi.org/10.1016/00043702(93)90202M
Schank, R. C., & Kolodner, J. (1979). Retrieving information from an episodic memory or why computers’ memories should be more like people’s (Tech. Rep.). Yale University Department of Computer Science.
Self, J. (2016). The birth of IJAIED. International Journal of Artificial Intelligence in Education , 26 (1), 4–12. https://doi.org/10.1007/s4059301500405
Sfard, A. (1998). On two metaphors for learning and the dangers of choosing just one. Educational Researcher , 27 (2), 4–13. https://doi.org/10.3102/0013189X027002004
Shapiro, L., & Spaulding, S. (2021). Embodied Cognition. In E. N. Zalta (Ed.), The Stanford encyclopedia of philosophy (Fall 2021 ed.). Metaphysics Research Lab, Stanford University. https://plato.stanford.edu/archives/fall2021/entries/embodied-cognition/
Shuvaev, S. A., Tran, N. B., Stephenson-Jones, M., Li, B., & Koulakov, A. A. (2021). Neural networks with motivation. Frontiers in Systems Neuroscience , 100 . https://doi.org/10.3389/fnsys.2020.609316
Simon, H. A. (1967). Job of a college president. Educational Record , 48 (1), 68–78.
Simon, H. A. (1992a). Center for innovation in learning: Proposed structure and function. Herbert Simon Collection (Box 22, Folder 1547), University Libraries Digital Collections, Carnegie Mellon University.
Simon, H. A. (1992b). Proposal for an initiative on cognitive theory in instruction. Herbert Simon Collection (Box 23, Folder 1596), University Libraries Digital Collections, Carnegie Mellon University.
Simon, H. A. (1995). [Letter to Allyson Halpern]. Herbert Simon Collection (Box 22, Folder 1548), University Libraries Digital Collections, Carnegie Mellon University.
Simon, H. A., & Newell, A. (1971). Human problem solving: The state of the theory in 1970. American Psychologist , 26 (2), 145. https://doi.org/10.1037/h0030806
Sleeman, D., & Brown, J. S. (1982). Intelligent tutoring systems . Academic Press.
Solomon, C., Harvey, B., Kahn, K., Lieberman, H., Miller, M. L., Minsky, M., … Silverman, B. (2020, June). History of Logo. Proc. ACM Program. Lang. , 4 (HOPL). https://doi.org/10.1145/3386329
Stager, G. S. (2013). Papert’s prison fab lab: implications for the maker movement and education design. In Proceedings of the 12th International Conference on Interaction Design and Children (pp. 487–490).
Suchman, L. A. (1984). Plans and situated actions: An inquiry into the idea of human-machine communication [Doctoral dissertation, University of California, Berkeley]. ProQuest Dissertations & Theses Global. https://www.proquest.com/pqdtglobal/docview/303331872/A23CFD5DC9F84671PQ
Sweller, J. (1994). Cognitive load theory, learning difficulty, and instructional design. Learning and Instruction , 4 (4), 295–312. https://doi.org/10.1016/0959-4752(94)90003-5
Tuomi, I. (2018). Vygotsky meets backgpropagation: Artificial neural models and the development of higher forms of thought. In C. P. Rosé, R. Martínez-Maldonado, H. Ulrich-Hoppe, R. Luckin, M. Mavrikis, K. Porayska-Pomsta, B. McLaren, & B. du Boulay (Eds.), Artificial Intelligence in Education. 19th International Conference, AIED 2018, London, UK, June 27–30, 2018, Proceedings, Part II (pp. 570–583). Springer. https://doi.org/10.1007/9783319938431_42
Turkle, S. (1991). Romantic reactions: Paradoxical responses to the computer presence. In J. J. Sheehan & M. Sosna (Eds.), The boundaries of humanity: Humans, animals, machines (pp. 224–252). University of California Press. https://doi.org/10.1525/9780520313118014
Varela, F. J., Thompson, E., & Rosch, E. (1991). The embodied mind: Cognitive science and human experience . MIT Press. https://doi.org/10.7551/mitpress/6730.001.0001
Wenger, E. (1990). Toward a theory of cultural transparency: Elements of a social discourse of the visible and the invisible [Doctoral dissertation, University of California, Irvine]. ProQuest Dissertations & Theses Global. https://www.proquest.com/pqdtglobal/docview/303816371/CE1A73FCBAB44A98PQ
Wenger, E. (1999). Communities of practice: Learning, meaning, and identity . Cambridge University Press. https://doi.org/10.1017/CBO9780511803932
Willcox, K. E., Sarma, S., & Lippel, P. (2016). Online education: A catalyst for higher education reforms (Tech. Rep.). Massachusetts Institute of Technology. https://oepi.mit.edu/files/2016/09/MIT-Online-Education-Policy-Initiative-April-2016.pdf
Winne, P. H. (2021). Open learner models working in symbiosis with self-regulating learners: A research agenda. International Journal of Artificial Intelligence in Education , 31 (3), 446–459. https://doi.org/10.1007/s40593-020-00212-4
Winograd, T. (2006). Shifting viewpoints: Artificial intelligence and human–computer interaction. Artificial Intelligence , 170 (18), 1256–1258. https://doi.org/10.1016/j.artint.2006.10.011
Wright, S. H. (2002). Papert misses ‘big ideas’ from early days of artificial intelligence . MIT News. https://news.mit.edu/2002/papert-misses-big-ideas-early-days-artificial-intelligence
Yazdani, M. (1984). New horizons in educational computing . Halsted Press.
Yazdani, M., & Lawler, R. W. (1986). Artificial intelligence and education: An overview. Instructional Science , 14 (3), 197–206. https://doi.org/10.1007/BF00051820
Zhang, Y., & Er, M. J. (2016). Sequential active learning using meta-cognitive extreme learning machine. Neurocomputing , 173 , 835–844. https://doi.org/10.1016/j.neucom.2015.08.037
Zhu, X. (2015). Machine teaching: An inverse problem to machine learning and an approach toward optimal education. In Proceedings of the AAAI Conference on Artificial Intelligence (Vol. 29). https://ojs.aaai.org/index.php/AAAI/article/view/9761
Zhu, X., & Simon, H. A. (1987). Learning mathematics from examples and by doing. Cognition and Instruction , 4 (3), 137–166. https://www.jstor.org/stable/3233583
Download references
Acknowledgements
I would like to thank the many individuals who gave helpful feedback on various drafts of this paper, including Yusuf Ahmad, Varun Arora, Drew Bailey, Barbara Hof, Ken Kahn, and Cynthia Solomon. I would also like to thank the editor and three anonymous reviewers who gave feedback rooted in their deep knowledge of parts of the history presented here. Much of my understanding of this history began with my graduate studies at Carnegie Mellon University and learning from mentors and colleagues there, where the impact of Simon and Newell was still in the air.
Author information
Authors and affiliations.
School of Education, University of California, Irvine, 401 E. Peltason Drive, Suite 3200, Irvine, CA, 92617, USA
Shayan Doroudi
You can also search for this author in PubMed Google Scholar
Corresponding author
Correspondence to Shayan Doroudi .

Ethics declarations
Competing interests.
The author has no relevant financial or non-financial interests to disclose.
Additional information
Publisher's note.
Springer Nature remains neutral with regard to jurisdictional claims in published maps and institutional affiliations.
Rights and permissions
Open Access This article is licensed under a Creative Commons Attribution 4.0 International License, which permits use, sharing, adaptation, distribution and reproduction in any medium or format, as long as you give appropriate credit to the original author(s) and the source, provide a link to the Creative Commons licence, and indicate if changes were made. The images or other third party material in this article are included in the article's Creative Commons licence, unless indicated otherwise in a credit line to the material. If material is not included in the article's Creative Commons licence and your intended use is not permitted by statutory regulation or exceeds the permitted use, you will need to obtain permission directly from the copyright holder. To view a copy of this licence, visit http://creativecommons.org/licenses/by/4.0/ .
Reprints and permissions
About this article
Doroudi, S. The Intertwined Histories of Artificial Intelligence and Education. Int J Artif Intell Educ 33 , 885–928 (2023). https://doi.org/10.1007/s40593-022-00313-2
Download citation
Accepted : 25 April 2022
Published : 04 October 2022
Issue Date : December 2023
DOI : https://doi.org/10.1007/s40593-022-00313-2
Share this article
Anyone you share the following link with will be able to read this content:
Sorry, a shareable link is not currently available for this article.
Provided by the Springer Nature SharedIt content-sharing initiative
- Artificial intelligence
- Learning sciences
- Cognitive science
- Information-processing psychology
- Constructivism
- Find a journal
- Publish with us
- Track your research
- LOGIN & Help
Artificial intelligence for education: Knowledge and its assessment in AI-enabled learning ecologies
- Education Policy, Organization and Leadership
- Information Trust Institute
- Coordinated Science Lab
- European Union Center
- Center for Global Studies
- National Center for Supercomputing Applications (NCSA)
- Lemann Center for Brazilian Studies
Research output : Contribution to journal › Article › peer-review
Over the past ten years, we have worked in a collaboration between educators and computer scientists at the University of Illinois to imagine futures for education in the context of what is loosely called “artificial intelligence.” Unhappy with the first generation of digital learning environments, our agenda has been to design alternatives and research their implementation. Our starting point has been to ask, what is the nature of machine intelligence, and what are its limits and potentials in education? This paper offers some tentative answers, first conceptually, and then practically in an overview of the results of a number of experimental implementations documented in greater detail elsewhere. Our key finding is that artificial intelligence—in the context of the practices of electronic computing developing over the past three quarters of a century—will never in any sense “take over” the role of teacher, because how it works and what it does are so profoundly different from human intelligence. However, within the limits that we describe in this paper, it offers the potential to transform education in ways that—counterintuitively perhaps—make education more human, not less.
- Artificial intelligence
ASJC Scopus subject areas
- History and Philosophy of Science
Online availability
- 10.1080/00131857.2020.1728732
Library availability
Related links.
- Link to publication in Scopus
- Link to the citations in Scopus
Fingerprint
- artificial intelligence Social Sciences 100%
- ecology Social Sciences 93%
- Ecology Arts & Humanities 88%
- Learning Environment Arts & Humanities 36%
- learning Social Sciences 34%
- education Social Sciences 30%
- Illinois Arts & Humanities 28%
- first generation Social Sciences 26%
T1 - Artificial intelligence for education
T2 - Knowledge and its assessment in AI-enabled learning ecologies
AU - Cope, Bill
AU - Kalantzis, Mary
AU - Searsmith, Duane
N1 - Publisher Copyright: © 2020 Philosophy of Education Society of Australasia.
N2 - Over the past ten years, we have worked in a collaboration between educators and computer scientists at the University of Illinois to imagine futures for education in the context of what is loosely called “artificial intelligence.” Unhappy with the first generation of digital learning environments, our agenda has been to design alternatives and research their implementation. Our starting point has been to ask, what is the nature of machine intelligence, and what are its limits and potentials in education? This paper offers some tentative answers, first conceptually, and then practically in an overview of the results of a number of experimental implementations documented in greater detail elsewhere. Our key finding is that artificial intelligence—in the context of the practices of electronic computing developing over the past three quarters of a century—will never in any sense “take over” the role of teacher, because how it works and what it does are so profoundly different from human intelligence. However, within the limits that we describe in this paper, it offers the potential to transform education in ways that—counterintuitively perhaps—make education more human, not less.
AB - Over the past ten years, we have worked in a collaboration between educators and computer scientists at the University of Illinois to imagine futures for education in the context of what is loosely called “artificial intelligence.” Unhappy with the first generation of digital learning environments, our agenda has been to design alternatives and research their implementation. Our starting point has been to ask, what is the nature of machine intelligence, and what are its limits and potentials in education? This paper offers some tentative answers, first conceptually, and then practically in an overview of the results of a number of experimental implementations documented in greater detail elsewhere. Our key finding is that artificial intelligence—in the context of the practices of electronic computing developing over the past three quarters of a century—will never in any sense “take over” the role of teacher, because how it works and what it does are so profoundly different from human intelligence. However, within the limits that we describe in this paper, it offers the potential to transform education in ways that—counterintuitively perhaps—make education more human, not less.
KW - Artificial intelligence
KW - assessment
KW - e-learning
KW - pedagogy
UR - http://www.scopus.com/inward/record.url?scp=85079717342&partnerID=8YFLogxK
UR - http://www.scopus.com/inward/citedby.url?scp=85079717342&partnerID=8YFLogxK
U2 - 10.1080/00131857.2020.1728732
DO - 10.1080/00131857.2020.1728732
M3 - Article
AN - SCOPUS:85079717342
SN - 0013-1857
JO - Educational Philosophy and Theory
JF - Educational Philosophy and Theory
The AI Classroom Hype Is All Wrong, Some Educators Say

- Share article
Many educators who have used generative artificial intelligence tools in their work have called the emerging technology a “game changer.”
Some say it’s been especially helpful in reducing the time it takes to do planning or administrative work , such as creating schedules, crafting lesson plans, and writing letters of recommendation for students. Teachers say they work an average of 57 hours a week , but less than half of that time is spent teaching.
“I think the use of AI has streamlined many aspects of teaching and has saved much prep time for teachers,” said a high school fine arts teacher in California in an open-ended response to an EdWeek Research Center survey conducted in March and April.
But amid all the encouragement to try the technology, there are plenty of educators who haven’t tried AI tools and don’t plan to start . These educators are more skeptical of the technology and don’t believe it should be used in K-12.
In open-ended responses to the EdWeek Research Center survey, educators shared their reasoning:
It could degrade critical thinking skills
ai is not as wonderful as you all make it out to be. how do we expect our next generation to learn to think if all we teach them is how to use ai.
— District-level administrator, Ohio
AI is driving a wedge between critical thinking and imagination.
— High school foreign language teacher, New Jersey
AI are machines. They have been trained using stolen data. Students should be learning, questioning, problem-solving, and doing their own work. Teachers should as well. I do not believe AI can ethically be used.
— High school English teacher, Louisiana
Students should not use AI until they have demonstrated some level of mastery on a subject. Students should not even use a calculator until they can do arithmetic calculations without tools. Problem solving starts in the mind, not on a keypad.
— High school math teacher, Texas
AI and use of computers in the classroom has diminished everyone's ability to think, learn and reason. It's too easy to punch in a subject and get an immediate answer, which may or may not be correct. How many times have we heard "the computer model says this or that," so therefore that's the end of the discussion. Now I hear AI says this or that. Machines do not and can never have the capabilities of the human mind and the human experience. They can never have the ability to reason. They can never have the ability to rely on "gut instinct," which is correct most of the time. They can never have the ability to say "something just isn't right here." All they can do is look at the data that is fed into them and go from there. And that data is totally dependent on the character of the human or humans feeding it into them.
— District-level administrator, Texas
I feel AI is used less as a resource and more as a crutch. I was shaken when I found out how many yearbook groups have used AI to write their entire yearbook and make the theme and set the ladder and put it together. We don't like students using AI because it's considered "plagiarism" but yet some teachers use it for everything. I don't mind AI as a brainstorming tool but when you give AI the ability to do all your work is when I have issues with it.
— Middle school teacher, Missouri
The human touch is better
i have never used ai for anything in my job. i would think we still have to follow through with the actual teaching. ai can't do what i do.
— High school math teacher, Michigan
While AI is the future, it's more important that teachers know their subject matter, and AI should only be used as a supplement to the teacher's scope of knowledge. To use it beyond that is ineffective as the presentation of the knowledge will be presented with less passion and clarity.
— Middle school physical education teacher, Virginia
While I believe AI is here to stay, I do not believe that it should be used to simply replace the human aspect of the learning experience. If AI is used by instructors or teachers heavily, then the computer is essentially doing the teachers' jobs for them and the teacher is simply the middle person who repeats what the computer tells them.
— High school career-technical education teacher, Missouri
AI concerns me in that educators need to know their "stuff" before blindly having AI create lessons, etc., to administer in class. I have tried AI and caught multiple errors in its creation. If I had used what AI created, I would have considered myself unethical in teaching students through that lesson because it contained many errors.
— District-level administrator, Alabama
Utilizing AI to develop assessments is impersonal. If the general scientific community can acknowledge that generative AI utilizes biased information to create material, why would we rely on these tools to create unbiased assessments?
— High school social studies teacher, Montana
The K-12 system isn’t prepared
i think that ai is a very dangerous phenomenon for learning and education. it seems like it is thrust upon us and unleashed without adequate preparation to handle the consequences for learning and teaching. i think this should be the number one topic for governments and academic institutions to address immediately..
— High school foreign language teacher, Pennsylvania
I fear AI is yet another trend that education professionals are running headlong into without sufficient forethought and planning.
— Elementary fine arts teacher, Virginia
I have never used AI and never will. I think it gives fuel to a fire that we won't be able to control.
— Elementary teacher, North Carolina
Concerns about how it affects their jobs
last year, i spent a lot of time talking with english teaching colleagues about how to tackle the new problem of ai generated student work. we researched apps to check for plagiarism and ai produced writing and didn't find a good source to help us. this new issue is requiring teachers to rethink the types of assignments we give and the ways we ask students to produce writing in class so we can ensure they are producing original works. it's frustrating and time consuming..
— High school English teacher, Minnesota
Artificial Intelligence will render my job unnecessary within five years. My students use Grammarly and ChatGPT to write their essays, and they even use it to email their teachers. Commercials show corporations praising their staff for using it to email each other. If humans no longer need to learn how to communicate well in writing—if AI does it for us—then what I have been teaching students for decades is no longer needed. What's more, my students already realize this and are showing it in their attitudes and efforts in writing class.
— Middle school English teacher, Massachusetts

Data analysis for this article was provided by the EdWeek Research Center. Learn more about the center’s work.
Sign Up for EdWeek Tech Leader
Edweek top school jobs.

Sign Up & Sign In

More From Forbes
Google I/O 2024 Sets Its Sights On Education With New AI LearnLM
- Share to Facebook
- Share to Twitter
- Share to Linkedin
Google I/O 2024 has emboldened the case for artificial intelligence in education.
With the unveiling of a range of groundbreaking AI tools, Google has demonstrated its unwavering commitment to integrating large language models into every facet of its ecosystem. One announcement that made educators stop in their tracks was the launch of LearnLM.
“Today marks a new chapter for learning and education at Google,” stated James Manyika , Google’s SVP of technology and society.
A Catalyst For Educational Transformation?
LearnLM potentially represents a giant leap forward for personalized learning experiences.
At the event, they showed viewers various interactive features across a wide array of Google products, from an interactive Learning Coach in the Gemini app to an immersive educational experience on YouTube. Based on these demonstrations, LearnLM could become an indispensable tool for learners worldwide. As Manyika explained, “LearnLM is grounded in educational research, making learning experiences more personal and engaging.”
Top CEO Bets On A Shock Biden Crypto Flip As Congress Hurtles Toward A Crucial Vote That Could Blow Up The Price Of Bitcoin Ethereum And XRP
Iphone 16 pro max all-new design upgrade promised, insider claims, who is ebrahim raisi iran s president nicknamed butcher of tehran dies in helicopter crash.
The integration of LearnLM into Google’s existing educational tools could signify a new era in digital learning. Sam Gibson , education sector strategy lead at The Warehouse Group in New Zealand, wrote on LinkedIn that, “The pace that companies such as Google are working at to enhance learning through AI is hugely exciting. By working towards allowing students to essentially have a personal tutor at the ready, teachers can then work in ways that allow them to provide much more targeted support and guidance to their students. It is an amazing time to work in education!”
According to Google, they are integrating LearnLM into several of their popular products, enhancing their functionality. Users will be able to employ a new feature called Circle to Search on Android to highlight math or physics problems and get step-by-step solutions. Viewers watching educational videos on YouTube will be able to ask questions of the videos and receive instant explanations. This integration across platforms could increase accessibility and provide the tools to allow students to learn anytime and anywhere.
Empowering Educators & Learners
Google have collaborated with organizations such as Columbia Teachers College and Khan Academy in the development of LearnLM. This shows a commitment to ensuring that LearnLM’s development is rooted in the teacher and learner's experiences. These tools show potential to not only save time but also provide deeper insights into student learning, helping educators tailor their approaches to meet diverse needs. Ben Whitaker , a former U.K. school leader and innovation consultant, adds, "Educators do one of the most important jobs in the world. LearnLM will allow them to do what they do best: connect with learners in authentic and informed ways. Rather than focusing on content creation, remembering individual details of student needs and maintaining manual records."
According to Google, their pilot program in Google Classroom will use LearnLM to simplify lesson planning, helping teachers discover new ideas and unique activities, find engaging materials and differentiate their lessons to meet each student's needs. They hope that this will give teachers more time to focus on student interaction.
The Rise Of AI Agents
Google’s I/O announcements placed a strong emphasis on LearnLM and its educational applications, but it also hinted at a broader shift towards an AI agent-powered future. Demis Hassabis , CEO of Google DeepMind, remarked, “We are still in the early days, and you’ll see glimpses of our approach throughout the day, but let me show you the kinds of use cases we are working hard to solve.”
Google’s comprehensive approach and focus on mass-market appeal cannot be underestimated. Integrating LearnLM and other AI innovations into its vast ecosystem of products and platforms could democratize access to cutting-edge educational tools. As Sundar Pichai, CEO of Google, passionately declared, “All of this shows the important progress we’ve made, as we take a bold and responsible approach to making AI helpful for everyone.”
After OpenAI's announcements this week and now Google's offering, the battle for AI supremacy continues. Google’s vision for the future of education and AI-powered experiences is audacious, ambitious and poised to redefine the landscape of learning for generations to come.
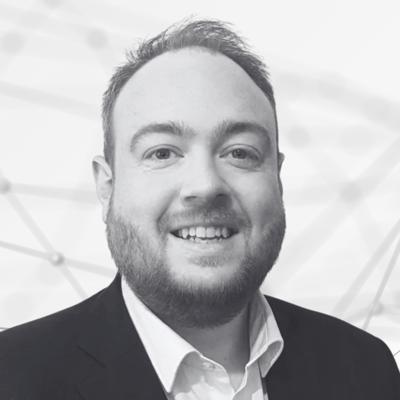
- Editorial Standards
- Reprints & Permissions
Join The Conversation
One Community. Many Voices. Create a free account to share your thoughts.
Forbes Community Guidelines
Our community is about connecting people through open and thoughtful conversations. We want our readers to share their views and exchange ideas and facts in a safe space.
In order to do so, please follow the posting rules in our site's Terms of Service. We've summarized some of those key rules below. Simply put, keep it civil.
Your post will be rejected if we notice that it seems to contain:
- False or intentionally out-of-context or misleading information
- Insults, profanity, incoherent, obscene or inflammatory language or threats of any kind
- Attacks on the identity of other commenters or the article's author
- Content that otherwise violates our site's terms.
User accounts will be blocked if we notice or believe that users are engaged in:
- Continuous attempts to re-post comments that have been previously moderated/rejected
- Racist, sexist, homophobic or other discriminatory comments
- Attempts or tactics that put the site security at risk
- Actions that otherwise violate our site's terms.
So, how can you be a power user?
- Stay on topic and share your insights
- Feel free to be clear and thoughtful to get your point across
- ‘Like’ or ‘Dislike’ to show your point of view.
- Protect your community.
- Use the report tool to alert us when someone breaks the rules.
Thanks for reading our community guidelines. Please read the full list of posting rules found in our site's Terms of Service.

Caltech Bootcamp / Blog / /
What Is Transfer Learning in Machine Learning?
- Written by John Terra
- Updated on May 21, 2024
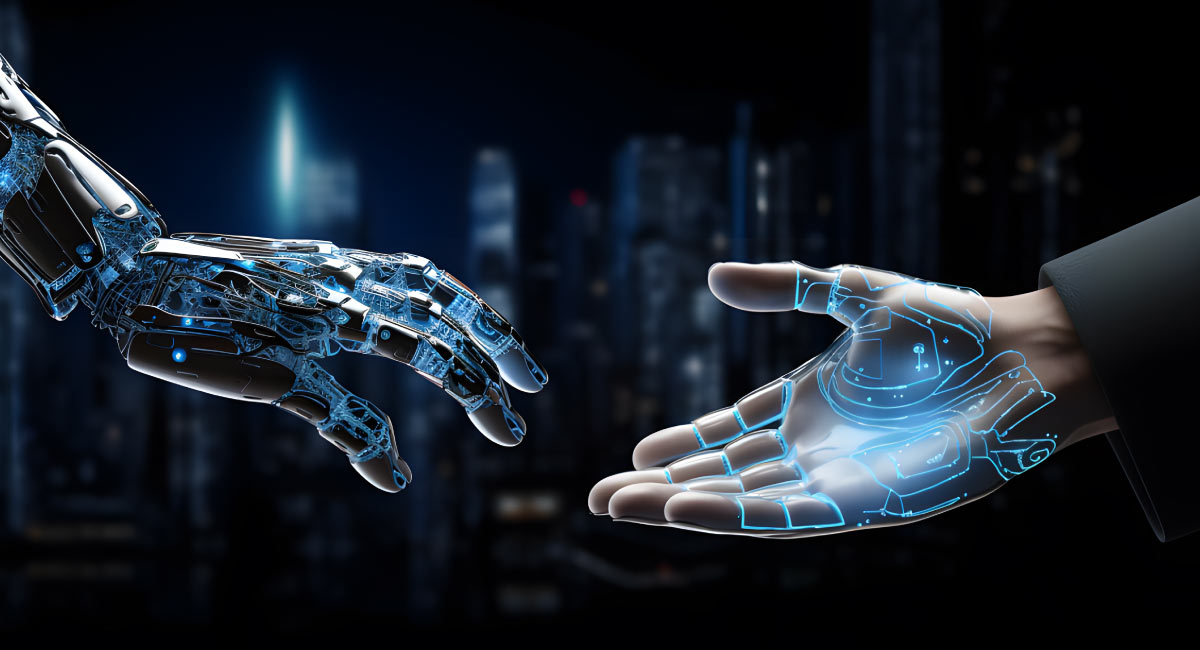
We often learn how to do new things by building off the knowledge we gained from learning how to do other things in the past. For example, if you learned how to type, that knowledge can help when using a computer keyboard. Or you could take your knowledge of how to ride a bicycle and use it to ride a moped. Well, the same principle applies when training machine learning models.
This article explores the concept of transfer learning in machine learning. We will define the term, explain its need, when to use it, and how it works. We’ll also compare it with traditional machine learning and explore some examples you can learn in an online AI and machine learning bootcamp .
What is Transfer Learning?
Transfer learning is an increasingly popular machine learning (ML) technique in which a model already created for an ML task is reused for a new task. It is also an increasingly popular deep learning approach since it enables deep neural network training using less data instead of creating a new model from scratch.
It involves a machine exploiting the knowledge it acquired from performing a previous task (also referred to as the pre-trained model) to improve the generalization of a new target task.
For instance, data scientists who train a classifier to predict whether an image contains a suitcase can use the knowledge the classifier gained during training to recognize objects usually found in a suitcase. So, the old knowledge is “transferred” to the new task to help the network “learn” to solve another problem.
Also Read: Machine Learning in Healthcare: Applications, Use Cases, and Careers
Why Do We Need Transfer Learning in ML?
Many deep neural networks trained on images have a common, curious characteristic: deep learning models try to learn a low level of features in the early layers of the network, such as detecting colors, edges, intensity variations, etc. These features don’t appear specific to any particular data set or task because no matter what image is being processed, for example, detecting a car or a cat, the low-level features must be detected. All these features occur regardless of the exact cost function or the image dataset. Therefore, learning all these features in detecting cats can be used in other tasks, such as people.
Transfer learning offers several benefits, but the primary advantages are saving training time, enhanced neural network performance of neural networks, and not requiring vast amounts of data.
Large volumes of data are typically needed to train neural networks from scratch. However, access to that amount of data is only sometimes available. This is a situation where transfer learning comes in handy. Thanks to this type of ML, a practical, reliable machine learning model can be built with relatively little training data since the model has already been pre-trained. This is particularly valuable in natural language processing because expert-level knowledge is primarily required to build large, labeled data sets. In addition, training time is reduced because it sometimes takes days or weeks to train a deep neural network from scratch on how to complete a complex task.
Explaining Transfer Learning Theory
During the transfer learning process, knowledge from a source task is used to enhance and improve the learning in a new task. However, if the transfer method decreases the new task’s performance, it’s a negative transfer. It’s a significant challenge to develop transfer methods that ensure positive transfer between two related tasks while avoiding any possible negative transfer between the less related tasks.
When applying relevant knowledge from one task to another, the characteristics of the original task are customarily mapped onto the characteristics of the other task to specify correspondence. Although people typically provide this mapping, there are evolving methods that can automatically perform the mapping.
Use the following three common indicators to measure the effectiveness of transfer learning techniques:
- First. This indicator measures if the target task can be performed using only the transferred knowledge. The question: Can we use only transferred knowledge to do this?
- Second. This indicator measures the time required to learn the target task using knowledge gained through transferred learning compared to how long it would take to learn the target task without it. The question: How long will it take to do this by using transferred knowledge?
- Third. This indicator determines if the task’s final performance learned via transfer learning is comparable to completing the original task without knowledge transfer. The question: Will the results achieved with transferred learning be as good as results achieved without transferred knowledge?
How to Approach Transfer Learning
There are three common approaches
- Train a model to reuse it. So, you want to solve a task (let’s call it Alpha) but need more data to train a deep neural network to handle the job. Fortunately, you can figure out a way around this by finding a related task (we’ll call it Beta) with abundant data. You then train the necessary deep neural network on task Beta, using the model as a starting point to solve task Alpha. Whether you wind up using the whole model or just a few layers heavily depends on the problem you’re trying to solve. If both tasks have the same input, you may have the option of reusing the model and making predictions for your new input. Otherwise, consider changing and retraining both the different task-specific layers and the output layer.
- Use a pre-trained model. The second approach entails using a pre-trained model. There are plenty of available models, so it helps to do some research. How many layers can be reused and how many need retraining depends on the problem. Numerous pre-trained models for transfer learning, feature extraction, prediction, and fine-tuning can be found. This type is most often used in deep learning.
- Use feature extraction. The final approach uses deep learning to discover the problem’s best representation, which involves finding the most essential features. This approach, also called representation learning, can frequently result in much-improved performance over what can be obtained using hand-designed representation.
Also Read: What is Machine Learning? A Comprehensive Guide for Beginners
When to Use Transfer Learning
Transfer learning is a great concept but is not a universal solution. As always happens with machine learning, forming a set of applicable rules across the board is challenging. However, here are some guidelines for when it is most useful:
- There isn’t sufficient labeled training data to train the network from scratch
- A pre-trained network already exists dedicated to a similar task, typically trained on vast amounts of data
- When the two tasks have the same input
If the original model has been trained with an open-source library such as TensorFlow, restore it and retrain the appropriate layers for your task. However, remember that it only works correctly if the features the new task learns from the first task are general, which means they can also be helpful for other related tasks. Additionally, the model’s input must be of a similar size to what it was initially trained with. If this condition doesn’t exist, you must add a pre-processing step to resize the input to the required size.
Traditional Machine Learning vs. Transfer Learning
Here’s how these two machine learning models compare.
Traditional Machine Learning Models Need Training From Scratch
This requirement is computationally expensive and demands a vast amount of data to ensure a high performance. Transfer learning is computationally efficient and uses a small data set to achieve better results.
- Traditional machine learning relies on an isolated training approach. Each model is independently trained for a particular purpose and never relies on past knowledge. On the other side, transfer learning takes advantage of knowledge acquired from the pre-trained model to carry out the task.
- Transfer learning models reach optimal performance levels faster than traditional ML models. This feature is possible because the models that leverage knowledge from previously trained models have a head start; they already understand the features. Thus, this method is faster than training neural networks from the ground up.
How Does Transfer Learning Work?
This summary explains the steps required to leverage it:
- The pre-trained model. The process begins with a model previously trained for a particular task using a large data set. This model is often trained on extensive datasets and has identified general patterns and features relevant to many related tasks.
- The base model. The base model is what we call the pre-trained model. It consists of layers that have already employed incoming data to learn hierarchical feature representations.
- The transfer layers. Looking back at the pre-trained model, we find a set of layers that capture basic, generic information relevant to both the new task and the previous one. Since both tend to learn low-level information, these layers are often found near the network’s top.
- The fine-tuning. Now, we use the dataset from the new challenge to retrain the chosen layers, a process known as fine-tuning. This step aims to preserve the knowledge from the pre-training stage while letting the model modify its parameters to best suit the current assignment’s demands.
The Pros and Cons of Transfer Learning
It has its upsides and downsides. Let’s examine them more closely.
Advantages of Transfer Learning
- It speeds up the training process . By employing pre-trained models, the model can learn more effectively and quickly on the new task since it already understands the features and patterns found in the data.
- It can work with small data sets . Suppose there is only limited data available for the second task. In that case, it helps prevent overfitting since the model will have already learned the general features most likely required for the second task.
- It creates better performances . The model often leads to a better performance on the second task since it leverages the knowledge gained from performing the first task.
Disadvantages of Transfer Learning
- There may be domain mismatches . If the two tasks or the data distribution between them are very different, the pre-trained model might not be best suited for the second task.
- Overfitting may occur. If the model is excessively fine-tuned on the second task, it may lead to overfitting. Transfer learning might learn task-specific features that don’t generalize to new data.
- The process can be complex . The pre-trained model and fine-tuning process might become computationally expensive and require specialized hardware. This, in turn, could result in additional costs and other resources.
Also Read: Machine Learning Interview Questions & Answers
Transfer Learning Examples
It has many applications in natural language processing (NLP), neural networks, and computer vision.
In machine learning, data or knowledge gained while solving a problem is stored, labeled, and applied to a different but related problem. For instance, the knowledge gained by a machine learning algorithm to recognize passenger airliners could later be used in a different machine learning model being developed to recognize other kinds of air vehicles.
Somebody could use a medical-based neural network to search through images to recognize potential illnesses or ailments. If there is insufficient data to train the network, transfer learning could help identify these conditions using pre-trained models.
Transfer learning is also valuable when deploying upgraded technology, like chatbots. If the new technology is similar to earlier deployments, it can assess which prior knowledge should be transplanted and used by the upgrades. By employing it, developers can ascertain what previous deployments’ data and knowledge can be reused and then transfer that helpful information when developing the upgraded version.
In natural language processing, an older model’s dataset that understands the vocabulary used in one area can then be used to train a new model whose function is understanding multiple dialects. This newly trained model could then be used for sentiment analysis.
Do You Want to Gain AI and Machine Learning Skills?
Machine learning is an exciting, fast-growing field transforming many aspects of our lives. If you want to be part of the AI and machine learning revolution, consider this program in artificial intelligence and machine learning.
This immersive online course delivers a practical learning experience, teaching you Python, natural language processing, machine learning, and much more.
According to Indeed.com , machine learning engineers can earn an average annual salary of $166,572. So, if you’re looking for an exciting, challenging, cutting-edge career that offers security and excellent compensation, take that first step with this AI/ML bootcamp.
You might also like to read:
How to Become an AI Architect: A Beginner’s Guide
How to Become a Robotics Engineer? A Comprehensive Guide
Machine Learning Engineer Job Description – A Beginner’s Guide
How To Start a Career in AI and Machine Learning
Career Guide: How to Become an AI Engineer
Artificial Intelligence & Machine Learning Bootcamp
- Learning Format:
Online Bootcamp
Leave a comment cancel reply.
Your email address will not be published. Required fields are marked *
Save my name, email, and website in this browser for the next time I comment.
Recommended Articles
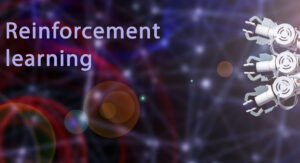
What is Reinforcement Learning in AI?
This article defines reinforcement learning in artificial intelligence, how it works, its uses, pros and cons, and its future.
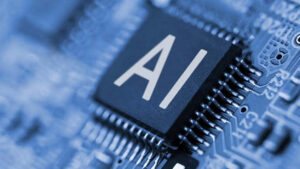
What is Sustainable AI? Definition, Significance, and Examples
This article covers sustainable AI, including its definition, importance, use cases, and more.
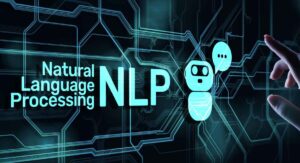
The Top 10 Natural Language Processing Applications
Every day, our society inches closer to technological innovations and devices initially found only in science fiction stories, films, and television shows. One of the
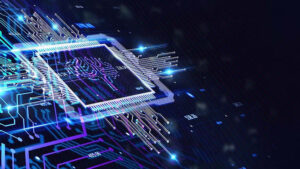
Explainable AI: Bridging the Gap Between Human Cognition and AI Models
As AI proliferates across industries, many people are worried about the veracity of something they don’t fully understand, with good reason. Enter explainable AI.
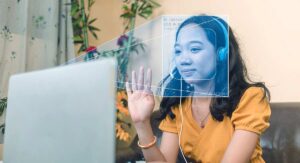
AI in Human Resources: Improving Hiring Processes with Predictive Analytics
This article discusses the role of artificial intelligence in human resources. It defines AI, shows ways AI is used in HR, and how to deploy AI in HR.
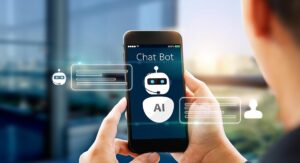
What Is an AI Chatbot, and How Do They Work?
This article discusses AI chatbots, what they are, how they work, how artificial intelligence figures into them, and their benefits.
Learning Format
Program Benefits
- 9+ top tools covered, 25+ hands-on projects
- Masterclasses by distinguished Caltech CTME instructors
- In collaboration with IBM
- Global AI and ML experts lead training
- Call us on : 1800-212-7688
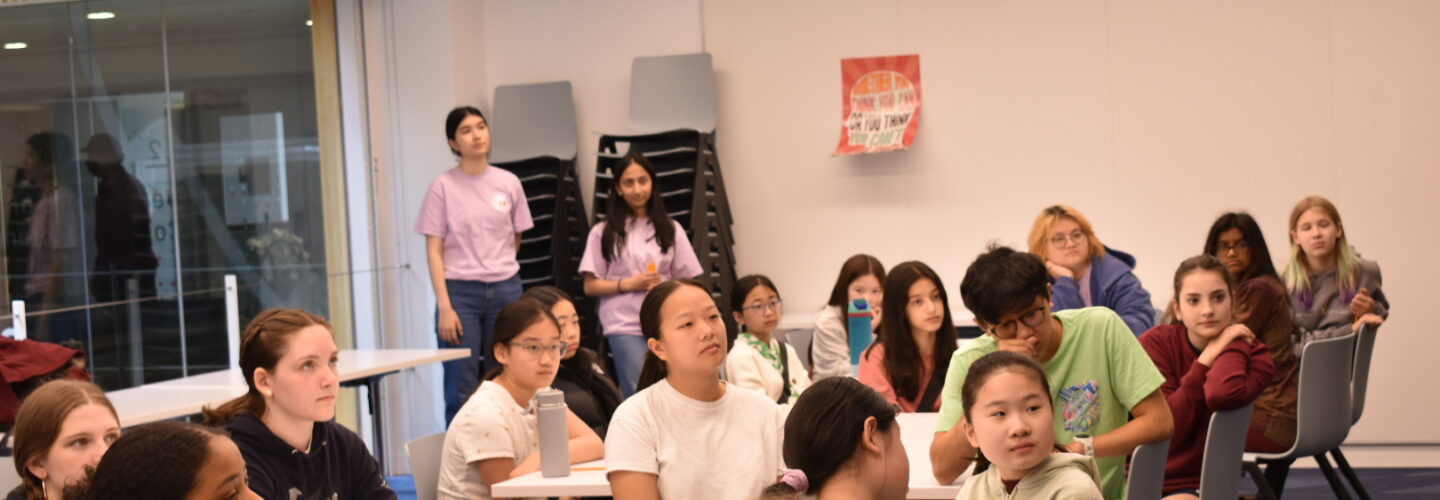
Empowering Middle School Girls in Tech: compileHER’s <prompt/HER> Capstone Event
On Saturday, May 11th, the John Crerar Library at the University of Chicago buzzed with energy as over 25 middle school girls from diverse backgrounds across Chicago convened for compileHER’s highly anticipated <prompt/HER> Capstone Project. This event, organized by compileHER , a campus organization dedicated to fostering gender equality in technology, marked a significant step in empowering young women to explore the world of artificial intelligence (AI) and its applications.
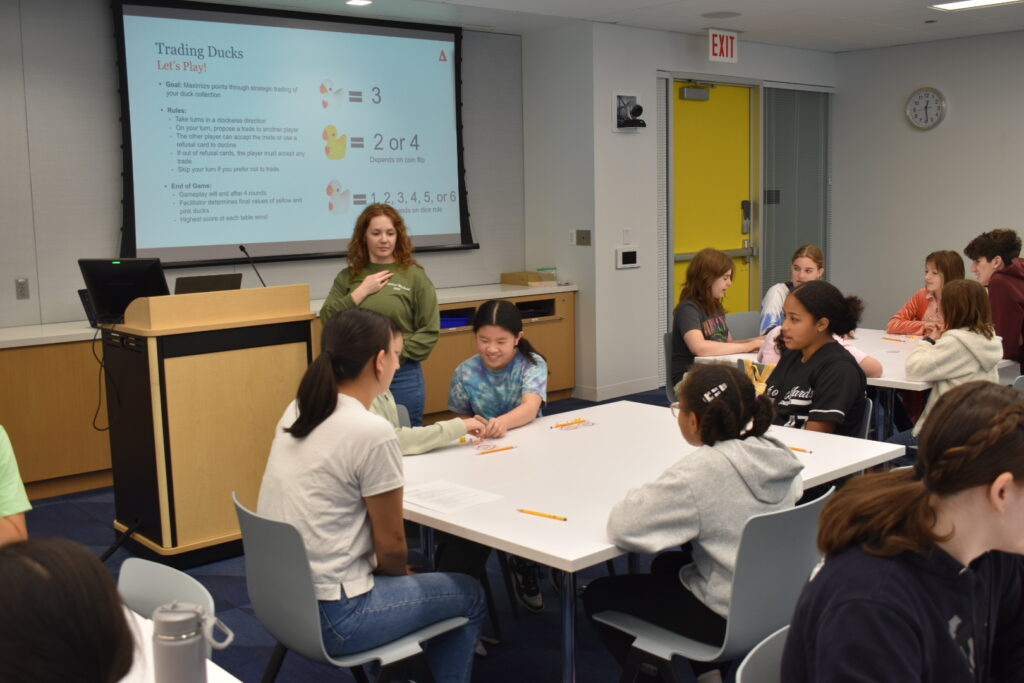
Following a brief snack break, the participants delved into the heart of the event at the CSIL Labs , where they embarked on a journey to demystify AI and explore the workings of large language models (LLMs) like ChatGPT. Through interactive sessions and hands-on activities, the girls gained a deeper understanding of AI’s fundamentals, including its applications in everyday life.
Stella Chen , president of compileHER, emphasized the organization’s commitment to empowering young women in technology. “At compileHER, we believe in providing girls with the knowledge and tools they need to thrive in the digital age,” said Chen. “Events like the <prompt/HER> Capstone Project are essential in bridging the gender gap in STEM fields and fostering a more inclusive tech community.”
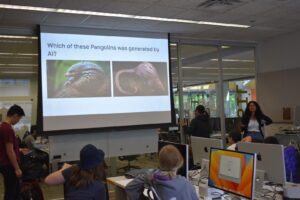
One of the highlights of the event was the hands-on creation of digital storybooks using generative AI. In collaborative groups, the participants used ChatGPT and DALL-E to craft imaginative narratives, showcasing the creative potential of AI-driven content generation. The resulting storybooks were a testament to the girls’ creativity and newfound understanding of AI’s role in storytelling.
The success of the <prompt/HER> Capstone Project underscores compileHER’s broader mission of empowering young women in tech. Through initiatives like annual hackathons, workshops, and field trips, compileHER aims to inspire the next generation of female leaders in technology. With each event, the organization seeks to break down barriers, challenge stereotypes, and create a more inclusive tech ecosystem.
Related News
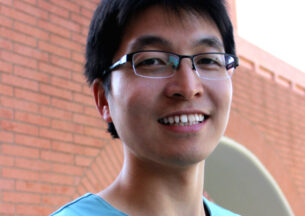
Haifeng Xu Wins Best Paper Award at Leading AI Conference for Pioneering Research on Mechanism Design for LLMs
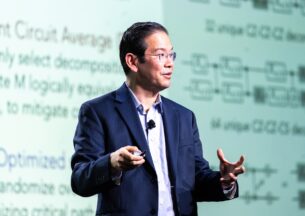
Fred Chong Receives Quantrell Award for Excellence in Teaching
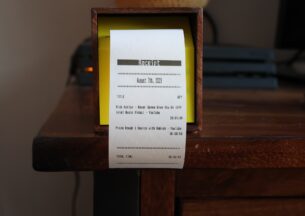
Unveiling Attention Receipts: Tangible Reflections on Digital Consumption
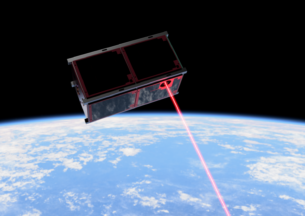
NASA to Launch UChicago Undergraduates’ Satellite
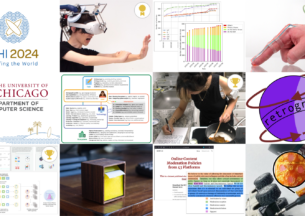
University of Chicago Computer Science Researchers To Present Ten Papers at CHI 2024
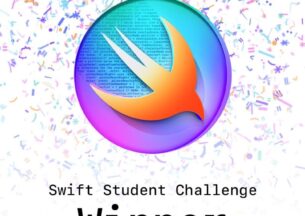
Two UChicago MPCS Students Win the Apple Swift Student Challenge
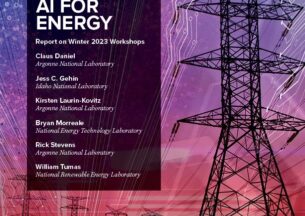
How Artificial Intelligence Can Transform U.S. Energy Infrastructure
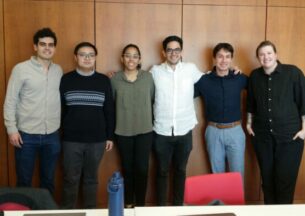
Community Data Fellow Stephania Tello Zamudio helps broaden internet access for Illinois residents

Two UChicago CS Students Awarded NSF Graduate Research Fellowship
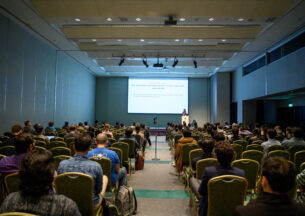
Non-Unital Noise Adds a New Wrinkle to the Quantum Supremacy Debate
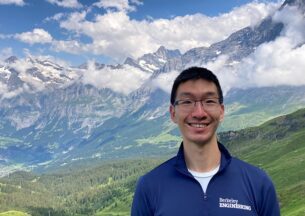
The Science of Computer Security: An Interview with Grant Ho, Assistant Professor in Computer Science
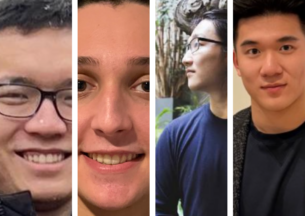
Four Students Receive Honorable Mention in CRA Undergraduate Research Awards

COMMENTS
Similarly, Rong (2022) employs constructivist learning theory and reinforcement learning theory to elucidate the impact of AI and VR technology on students' levels of concentration and creativity. ... International Journal of Artificial Intelligence in Education, 26, 701-712. 6* Turing, A. M. (1950). Computing machinery and intelligence.
1. Introduction. With the development of computing and information processing techniques, artificial intelligence (AI) has been widely applied in educational practices (Artificial Intelligence in Education; AIEd), such as intelligent tutoring systems, teaching robots, learning analytics dashboards, adaptive learning systems, human-computer interactions, etc. (Chen, Xie, & Hwang, 2020).
Rethinking learning theory for the age of artificial intelligence (AI) is needed to incorporate computational resources and capabilities into both theory and educational practices. What this paper adds ... Role of artificial intelligence in learning processes.
Artificial Intelligence in Education (AIEd) is an emerging interdisciplinary field that applies artificial intelligence technologies to transform instructional design and student learning. However, most research has investigated AIEd from the technological perspective, which cannot achieve a deep understand of the complex roles of AI in instructional and learning processes and its relationship ...
In the past few decades, technology has completely transformed the world around us. Indeed, experts believe that the next big digital transformation in how we live, communicate, work, trade and learn will be driven by Artificial Intelligence (AI) [83]. This paper presents a high-level industrial and academic overview of AI in Education (AIEd). It presents the focus of latest research in AIEd ...
2.1 Prolific countries. Artificial intelligence in education (AIEd) research has been conducted in many countries around the world. The 40 articles reported AIEd research studies in 16 countries (See Table 1).USA was so far the most prolific, with nine articles meeting all criteria applied in this study, and noticeably seven of them were conducted in K-12.
Frameworks concerning learning have been offered from several disciplines such as psychology, biology and computer science but have rarely been integrated or unified. Rethinking learning theory for the age of artificial intelligence (AI) is needed to incorporate computational resources and capabilities into both theory and educational practices.
Artificial Intelligence (AI) has the potential to address some of the biggest challenges in education today, innovate teaching and learning practices, and accelerate progress towards SDG 4. However, rapid technological developments inevitably bring multiple risks and challenges, which have so far outpaced policy debates and regulatory frameworks.
The initiative addresses the rising gap between the ultrafast development of artificial intelligence and the meticulous technological application of education. To get ready for AIED, the project advises educators, technologists, and policy makers to focus on educational leadership, and philosophy of technology in education to overcome the ...
8) assessed the future and new designs of AI in learning and education: "These design concepts expand beyond familiar ideas of technology supporting 'personalized,' 'adaptive,' or 'blended' learning. The conventional metaphors may continue to be useful, but they also may limit how we envision futures of AI in learning.".
The term "artificial intelligence" was coined in 1955 by John McCarthy, then an assistant professor at Dartmouth College, in the title of a workshop proposal for the Rockefeller Foundation. ... Educational Philosophy and Theory Volume 53, 2021 - Issue 12. Submit an article Journal homepage. 8,991 Views 113 ...
The concept of artificial intelligence (AI) was first proposed at the Dartmouth conference in 1956, discussing how to make computers simulate human intelligence [].Before the formal introduction of AI, psychology and pedagogy theory in the field of education had long been developed into a discipline system and combined with corresponding machines to form teaching equipment.
which are characterized into three paradigms in this position paper: AI-directed, learner-as-recipient, AI-. supported, learner-as-collaborator, and AI-empowered, learner-as-leader. In three ...
Theory gap. 1. Introduction. Artificial Intelligence in Education (AIEd) concerns mainly about the development of "computers which perform cognitive tasks, usually associated with human minds, particularly learning and problem-solving (p. 10)" ( Baker and Smith (2019).
The application of artificial intelligence (AI) in STEM education (AI-STEM), as an emerging field, is confronted with a challenge of integrating diverse AI techniques and complex educational elements to meet instructional and learning needs. To gain a comprehensive understanding of AI applications in STEM education, this study conducted a systematic review to examine 63 empirical AI-STEM ...
The integration of artificial intelligence [AI] into higher education is a rapidly growing field that offers transformative potential for teaching, learning and organizational processes.
The teaching of artificial intelligence (AI) topics in school curricula is an important global strategic initiative in educating the next generation. As AI technologies are new to K-12 schools, there is a lack of studies that inform schools' teachers about AI curriculum design. How to prepare and engage teachers, and which approaches are suitable for planning the curriculum for sustainable ...
Education in AI Theory, Practice, and Impact; Education in AI Theory, Practice, and Impact. Northwestern's core purpose as an educational institution is evident in the artificial intelligence (AI) curriculum available to learners at many levels, from undergraduates to executive and professional education. Explore the programs and variety of ...
This research aims to synthesize and develop a framework of an artificial intelligence learning platform for education and estimate the framework's suitability. The research is discussed into three phases: 1) synthesizing an intelligent learning platform by using Artificial Intelligence (AI), 2) developing a framework of an artificial ...
In Higher Education (HE), the study of Meade et al. identified student opinions on the use of generative artificial intelligence, particularly applications such as ChatGPT.The results revealed that over 60% of students had a basic understanding of AI tools. The study also highlighted a number of ethical and developmental concerns including standardisation, decolonisation, the reinforcement of ...
Artificial intelligence (AI) will reshape student experiences, pedagogy and how people communicate, according to dozens of higher ed and technology experts, sharing opinions in a report released Monday. AI pervaded higher education so much in the last year that Educause, a nonprofit focused on the intersection of higher ed and information technology, updated its annual Teaching and Learning ...
In this paper, I argue that the fields of artificial intelligence (AI) and education have been deeply intertwined since the early days of AI. Specifically, I show that many of the early pioneers of AI were cognitive scientists who also made pioneering and impactful contributions to the field of education. These researchers saw AI as a tool for thinking about human learning and used their ...
Artificial intelligence for education: Knowledge and its assessment in AI-enabled learning ecologies. Bill Cope, Mary Kalantzis, Duane Searsmith. Education Policy, Organization and Leadership; Information Trust Institute; ... Educational Philosophy and Theory, 53(12), 1229-1245.
Abstract. This chapter addresses several central meta-theoretical issues of AI and AGI. After analyzing the nature of the field, three criteria for desired theories are proposed: correctness ...
Many educators who have used generative artificial intelligence tools in their work have called the emerging technology a "game changer.". Some say it's been especially helpful in reducing ...
The term AI, coined by John McCarthy in 1955, is defined as a computer with the capability to perform a variety of human cognitive tasks, such as communicating, reasoning, learning, and/or problem-solving (Nilsson, 1998).Baker and Smith (2019) further explain that AI represents a generic term to describe a wide collection of different technologies and algorithms (e.g., machine learning, NLP ...
Google I/O 2024 has emboldened the case for artificial intelligence in education. With the unveiling of a range of groundbreaking AI tools, Google has demonstrated its unwavering commitment to ...
Explaining Transfer Learning Theory. During the transfer learning process, knowledge from a source task is used to enhance and improve the learning in a new task. However, if the transfer method decreases the new task's performance, it's a negative transfer. It's a significant challenge to develop transfer methods that ensure positive ...
OpenAI succeeded in making artificial intelligence (AI) accessible to the world (n.b., it is only available to the population with access to the internet) and has demonstrated how generative AI (Gen-AI), a subset of deep learning, can transform our lives [].As a result, since its launch in November 2022, the natural language model Chat Generative Pre-trained Transformer (ChatGPT) continues to ...
This event, organized by compileHER, a campus organization dedicated to fostering gender equality in technology, marked a significant step in empowering young women to explore the world of artificial intelligence (AI) and its applications. The day kicked off with an engaging icebreaker session, led by Optiver, a leading trading company.