- USC Libraries
- Research Guides

Organizing Your Social Sciences Research Paper
- 3. The Abstract
- Purpose of Guide
- Design Flaws to Avoid
- Independent and Dependent Variables
- Glossary of Research Terms
- Reading Research Effectively
- Narrowing a Topic Idea
- Broadening a Topic Idea
- Extending the Timeliness of a Topic Idea
- Academic Writing Style
- Applying Critical Thinking
- Choosing a Title
- Making an Outline
- Paragraph Development
- Research Process Video Series
- Executive Summary
- The C.A.R.S. Model
- Background Information
- The Research Problem/Question
- Theoretical Framework
- Citation Tracking
- Content Alert Services
- Evaluating Sources
- Primary Sources
- Secondary Sources
- Tiertiary Sources
- Scholarly vs. Popular Publications
- Qualitative Methods
- Quantitative Methods
- Insiderness
- Using Non-Textual Elements
- Limitations of the Study
- Common Grammar Mistakes
- Writing Concisely
- Avoiding Plagiarism
- Footnotes or Endnotes?
- Further Readings
- Generative AI and Writing
- USC Libraries Tutorials and Other Guides
- Bibliography
An abstract summarizes, usually in one paragraph of 300 words or less, the major aspects of the entire paper in a prescribed sequence that includes: 1) the overall purpose of the study and the research problem(s) you investigated; 2) the basic design of the study; 3) major findings or trends found as a result of your analysis; and, 4) a brief summary of your interpretations and conclusions.
Writing an Abstract. The Writing Center. Clarion University, 2009; Writing an Abstract for Your Research Paper. The Writing Center, University of Wisconsin, Madison; Koltay, Tibor. Abstracts and Abstracting: A Genre and Set of Skills for the Twenty-first Century . Oxford, UK: Chandos Publishing, 2010;
Importance of a Good Abstract
Sometimes your professor will ask you to include an abstract, or general summary of your work, with your research paper. The abstract allows you to elaborate upon each major aspect of the paper and helps readers decide whether they want to read the rest of the paper. Therefore, enough key information [e.g., summary results, observations, trends, etc.] must be included to make the abstract useful to someone who may want to examine your work.
How do you know when you have enough information in your abstract? A simple rule-of-thumb is to imagine that you are another researcher doing a similar study. Then ask yourself: if your abstract was the only part of the paper you could access, would you be happy with the amount of information presented there? Does it tell the whole story about your study? If the answer is "no" then the abstract likely needs to be revised.
Farkas, David K. “A Scheme for Understanding and Writing Summaries.” Technical Communication 67 (August 2020): 45-60; How to Write a Research Abstract. Office of Undergraduate Research. University of Kentucky; Staiger, David L. “What Today’s Students Need to Know about Writing Abstracts.” International Journal of Business Communication January 3 (1966): 29-33; Swales, John M. and Christine B. Feak. Abstracts and the Writing of Abstracts . Ann Arbor, MI: University of Michigan Press, 2009.
Structure and Writing Style
I. Types of Abstracts
To begin, you need to determine which type of abstract you should include with your paper. There are four general types.
Critical Abstract A critical abstract provides, in addition to describing main findings and information, a judgment or comment about the study’s validity, reliability, or completeness. The researcher evaluates the paper and often compares it with other works on the same subject. Critical abstracts are generally 400-500 words in length due to the additional interpretive commentary. These types of abstracts are used infrequently.
Descriptive Abstract A descriptive abstract indicates the type of information found in the work. It makes no judgments about the work, nor does it provide results or conclusions of the research. It does incorporate key words found in the text and may include the purpose, methods, and scope of the research. Essentially, the descriptive abstract only describes the work being summarized. Some researchers consider it an outline of the work, rather than a summary. Descriptive abstracts are usually very short, 100 words or less. Informative Abstract The majority of abstracts are informative. While they still do not critique or evaluate a work, they do more than describe it. A good informative abstract acts as a surrogate for the work itself. That is, the researcher presents and explains all the main arguments and the important results and evidence in the paper. An informative abstract includes the information that can be found in a descriptive abstract [purpose, methods, scope] but it also includes the results and conclusions of the research and the recommendations of the author. The length varies according to discipline, but an informative abstract is usually no more than 300 words in length.
Highlight Abstract A highlight abstract is specifically written to attract the reader’s attention to the study. No pretense is made of there being either a balanced or complete picture of the paper and, in fact, incomplete and leading remarks may be used to spark the reader’s interest. In that a highlight abstract cannot stand independent of its associated article, it is not a true abstract and, therefore, rarely used in academic writing.
II. Writing Style
Use the active voice when possible , but note that much of your abstract may require passive sentence constructions. Regardless, write your abstract using concise, but complete, sentences. Get to the point quickly and always use the past tense because you are reporting on a study that has been completed.
Abstracts should be formatted as a single paragraph in a block format and with no paragraph indentations. In most cases, the abstract page immediately follows the title page. Do not number the page. Rules set forth in writing manual vary but, in general, you should center the word "Abstract" at the top of the page with double spacing between the heading and the abstract. The final sentences of an abstract concisely summarize your study’s conclusions, implications, or applications to practice and, if appropriate, can be followed by a statement about the need for additional research revealed from the findings.
Composing Your Abstract
Although it is the first section of your paper, the abstract should be written last since it will summarize the contents of your entire paper. A good strategy to begin composing your abstract is to take whole sentences or key phrases from each section of the paper and put them in a sequence that summarizes the contents. Then revise or add connecting phrases or words to make the narrative flow clearly and smoothly. Note that statistical findings should be reported parenthetically [i.e., written in parentheses].
Before handing in your final paper, check to make sure that the information in the abstract completely agrees with what you have written in the paper. Think of the abstract as a sequential set of complete sentences describing the most crucial information using the fewest necessary words. The abstract SHOULD NOT contain:
- A catchy introductory phrase, provocative quote, or other device to grab the reader's attention,
- Lengthy background or contextual information,
- Redundant phrases, unnecessary adverbs and adjectives, and repetitive information;
- Acronyms or abbreviations,
- References to other literature [say something like, "current research shows that..." or "studies have indicated..."],
- Using ellipticals [i.e., ending with "..."] or incomplete sentences,
- Jargon or terms that may be confusing to the reader,
- Citations to other works, and
- Any sort of image, illustration, figure, or table, or references to them.
Abstract. Writing Center. University of Kansas; Abstract. The Structure, Format, Content, and Style of a Journal-Style Scientific Paper. Department of Biology. Bates College; Abstracts. The Writing Center. University of North Carolina; Borko, Harold and Seymour Chatman. "Criteria for Acceptable Abstracts: A Survey of Abstracters' Instructions." American Documentation 14 (April 1963): 149-160; Abstracts. The Writer’s Handbook. Writing Center. University of Wisconsin, Madison; Hartley, James and Lucy Betts. "Common Weaknesses in Traditional Abstracts in the Social Sciences." Journal of the American Society for Information Science and Technology 60 (October 2009): 2010-2018; Koltay, Tibor. Abstracts and Abstracting: A Genre and Set of Skills for the Twenty-first Century. Oxford, UK: Chandos Publishing, 2010; Procter, Margaret. The Abstract. University College Writing Centre. University of Toronto; Riordan, Laura. “Mastering the Art of Abstracts.” The Journal of the American Osteopathic Association 115 (January 2015 ): 41-47; Writing Report Abstracts. The Writing Lab and The OWL. Purdue University; Writing Abstracts. Writing Tutorial Services, Center for Innovative Teaching and Learning. Indiana University; Koltay, Tibor. Abstracts and Abstracting: A Genre and Set of Skills for the Twenty-First Century . Oxford, UK: 2010; Writing an Abstract for Your Research Paper. The Writing Center, University of Wisconsin, Madison.
Writing Tip
Never Cite Just the Abstract!
Citing to just a journal article's abstract does not confirm for the reader that you have conducted a thorough or reliable review of the literature. If the full-text is not available, go to the USC Libraries main page and enter the title of the article [NOT the title of the journal]. If the Libraries have a subscription to the journal, the article should appear with a link to the full-text or to the journal publisher page where you can get the article. If the article does not appear, try searching Google Scholar using the link on the USC Libraries main page. If you still can't find the article after doing this, contact a librarian or you can request it from our free i nterlibrary loan and document delivery service .
- << Previous: Research Process Video Series
- Next: Executive Summary >>
- Last Updated: May 9, 2024 11:05 AM
- URL: https://libguides.usc.edu/writingguide
Writing an Abstract for Your Research Paper
Definition and Purpose of Abstracts
An abstract is a short summary of your (published or unpublished) research paper, usually about a paragraph (c. 6-7 sentences, 150-250 words) long. A well-written abstract serves multiple purposes:
- an abstract lets readers get the gist or essence of your paper or article quickly, in order to decide whether to read the full paper;
- an abstract prepares readers to follow the detailed information, analyses, and arguments in your full paper;
- and, later, an abstract helps readers remember key points from your paper.
It’s also worth remembering that search engines and bibliographic databases use abstracts, as well as the title, to identify key terms for indexing your published paper. So what you include in your abstract and in your title are crucial for helping other researchers find your paper or article.
If you are writing an abstract for a course paper, your professor may give you specific guidelines for what to include and how to organize your abstract. Similarly, academic journals often have specific requirements for abstracts. So in addition to following the advice on this page, you should be sure to look for and follow any guidelines from the course or journal you’re writing for.
The Contents of an Abstract
Abstracts contain most of the following kinds of information in brief form. The body of your paper will, of course, develop and explain these ideas much more fully. As you will see in the samples below, the proportion of your abstract that you devote to each kind of information—and the sequence of that information—will vary, depending on the nature and genre of the paper that you are summarizing in your abstract. And in some cases, some of this information is implied, rather than stated explicitly. The Publication Manual of the American Psychological Association , which is widely used in the social sciences, gives specific guidelines for what to include in the abstract for different kinds of papers—for empirical studies, literature reviews or meta-analyses, theoretical papers, methodological papers, and case studies.
Here are the typical kinds of information found in most abstracts:
- the context or background information for your research; the general topic under study; the specific topic of your research
- the central questions or statement of the problem your research addresses
- what’s already known about this question, what previous research has done or shown
- the main reason(s) , the exigency, the rationale , the goals for your research—Why is it important to address these questions? Are you, for example, examining a new topic? Why is that topic worth examining? Are you filling a gap in previous research? Applying new methods to take a fresh look at existing ideas or data? Resolving a dispute within the literature in your field? . . .
- your research and/or analytical methods
- your main findings , results , or arguments
- the significance or implications of your findings or arguments.
Your abstract should be intelligible on its own, without a reader’s having to read your entire paper. And in an abstract, you usually do not cite references—most of your abstract will describe what you have studied in your research and what you have found and what you argue in your paper. In the body of your paper, you will cite the specific literature that informs your research.
When to Write Your Abstract
Although you might be tempted to write your abstract first because it will appear as the very first part of your paper, it’s a good idea to wait to write your abstract until after you’ve drafted your full paper, so that you know what you’re summarizing.
What follows are some sample abstracts in published papers or articles, all written by faculty at UW-Madison who come from a variety of disciplines. We have annotated these samples to help you see the work that these authors are doing within their abstracts.
Choosing Verb Tenses within Your Abstract
The social science sample (Sample 1) below uses the present tense to describe general facts and interpretations that have been and are currently true, including the prevailing explanation for the social phenomenon under study. That abstract also uses the present tense to describe the methods, the findings, the arguments, and the implications of the findings from their new research study. The authors use the past tense to describe previous research.
The humanities sample (Sample 2) below uses the past tense to describe completed events in the past (the texts created in the pulp fiction industry in the 1970s and 80s) and uses the present tense to describe what is happening in those texts, to explain the significance or meaning of those texts, and to describe the arguments presented in the article.
The science samples (Samples 3 and 4) below use the past tense to describe what previous research studies have done and the research the authors have conducted, the methods they have followed, and what they have found. In their rationale or justification for their research (what remains to be done), they use the present tense. They also use the present tense to introduce their study (in Sample 3, “Here we report . . .”) and to explain the significance of their study (In Sample 3, This reprogramming . . . “provides a scalable cell source for. . .”).
Sample Abstract 1
From the social sciences.
Reporting new findings about the reasons for increasing economic homogamy among spouses
Gonalons-Pons, Pilar, and Christine R. Schwartz. “Trends in Economic Homogamy: Changes in Assortative Mating or the Division of Labor in Marriage?” Demography , vol. 54, no. 3, 2017, pp. 985-1005.
![dissertation abstract social sciences “The growing economic resemblance of spouses has contributed to rising inequality by increasing the number of couples in which there are two high- or two low-earning partners. [Annotation for the previous sentence: The first sentence introduces the topic under study (the “economic resemblance of spouses”). This sentence also implies the question underlying this research study: what are the various causes—and the interrelationships among them—for this trend?] The dominant explanation for this trend is increased assortative mating. Previous research has primarily relied on cross-sectional data and thus has been unable to disentangle changes in assortative mating from changes in the division of spouses’ paid labor—a potentially key mechanism given the dramatic rise in wives’ labor supply. [Annotation for the previous two sentences: These next two sentences explain what previous research has demonstrated. By pointing out the limitations in the methods that were used in previous studies, they also provide a rationale for new research.] We use data from the Panel Study of Income Dynamics (PSID) to decompose the increase in the correlation between spouses’ earnings and its contribution to inequality between 1970 and 2013 into parts due to (a) changes in assortative mating, and (b) changes in the division of paid labor. [Annotation for the previous sentence: The data, research and analytical methods used in this new study.] Contrary to what has often been assumed, the rise of economic homogamy and its contribution to inequality is largely attributable to changes in the division of paid labor rather than changes in sorting on earnings or earnings potential. Our findings indicate that the rise of economic homogamy cannot be explained by hypotheses centered on meeting and matching opportunities, and they show where in this process inequality is generated and where it is not.” (p. 985) [Annotation for the previous two sentences: The major findings from and implications and significance of this study.]](https://writing.wisc.edu/wp-content/uploads/sites/535/2019/08/Abstract-1.png)
Sample Abstract 2
From the humanities.
Analyzing underground pulp fiction publications in Tanzania, this article makes an argument about the cultural significance of those publications
Emily Callaci. “Street Textuality: Socialism, Masculinity, and Urban Belonging in Tanzania’s Pulp Fiction Publishing Industry, 1975-1985.” Comparative Studies in Society and History , vol. 59, no. 1, 2017, pp. 183-210.
![dissertation abstract social sciences “From the mid-1970s through the mid-1980s, a network of young urban migrant men created an underground pulp fiction publishing industry in the city of Dar es Salaam. [Annotation for the previous sentence: The first sentence introduces the context for this research and announces the topic under study.] As texts that were produced in the underground economy of a city whose trajectory was increasingly charted outside of formalized planning and investment, these novellas reveal more than their narrative content alone. These texts were active components in the urban social worlds of the young men who produced them. They reveal a mode of urbanism otherwise obscured by narratives of decolonization, in which urban belonging was constituted less by national citizenship than by the construction of social networks, economic connections, and the crafting of reputations. This article argues that pulp fiction novellas of socialist era Dar es Salaam are artifacts of emergent forms of male sociability and mobility. In printing fictional stories about urban life on pilfered paper and ink, and distributing their texts through informal channels, these writers not only described urban communities, reputations, and networks, but also actually created them.” (p. 210) [Annotation for the previous sentences: The remaining sentences in this abstract interweave other essential information for an abstract for this article. The implied research questions: What do these texts mean? What is their historical and cultural significance, produced at this time, in this location, by these authors? The argument and the significance of this analysis in microcosm: these texts “reveal a mode or urbanism otherwise obscured . . .”; and “This article argues that pulp fiction novellas. . . .” This section also implies what previous historical research has obscured. And through the details in its argumentative claims, this section of the abstract implies the kinds of methods the author has used to interpret the novellas and the concepts under study (e.g., male sociability and mobility, urban communities, reputations, network. . . ).]](https://writing.wisc.edu/wp-content/uploads/sites/535/2019/08/Abstract-2.png)
Sample Abstract/Summary 3
From the sciences.
Reporting a new method for reprogramming adult mouse fibroblasts into induced cardiac progenitor cells
Lalit, Pratik A., Max R. Salick, Daryl O. Nelson, Jayne M. Squirrell, Christina M. Shafer, Neel G. Patel, Imaan Saeed, Eric G. Schmuck, Yogananda S. Markandeya, Rachel Wong, Martin R. Lea, Kevin W. Eliceiri, Timothy A. Hacker, Wendy C. Crone, Michael Kyba, Daniel J. Garry, Ron Stewart, James A. Thomson, Karen M. Downs, Gary E. Lyons, and Timothy J. Kamp. “Lineage Reprogramming of Fibroblasts into Proliferative Induced Cardiac Progenitor Cells by Defined Factors.” Cell Stem Cell , vol. 18, 2016, pp. 354-367.
![dissertation abstract social sciences “Several studies have reported reprogramming of fibroblasts into induced cardiomyocytes; however, reprogramming into proliferative induced cardiac progenitor cells (iCPCs) remains to be accomplished. [Annotation for the previous sentence: The first sentence announces the topic under study, summarizes what’s already known or been accomplished in previous research, and signals the rationale and goals are for the new research and the problem that the new research solves: How can researchers reprogram fibroblasts into iCPCs?] Here we report that a combination of 11 or 5 cardiac factors along with canonical Wnt and JAK/STAT signaling reprogrammed adult mouse cardiac, lung, and tail tip fibroblasts into iCPCs. The iCPCs were cardiac mesoderm-restricted progenitors that could be expanded extensively while maintaining multipo-tency to differentiate into cardiomyocytes, smooth muscle cells, and endothelial cells in vitro. Moreover, iCPCs injected into the cardiac crescent of mouse embryos differentiated into cardiomyocytes. iCPCs transplanted into the post-myocardial infarction mouse heart improved survival and differentiated into cardiomyocytes, smooth muscle cells, and endothelial cells. [Annotation for the previous four sentences: The methods the researchers developed to achieve their goal and a description of the results.] Lineage reprogramming of adult somatic cells into iCPCs provides a scalable cell source for drug discovery, disease modeling, and cardiac regenerative therapy.” (p. 354) [Annotation for the previous sentence: The significance or implications—for drug discovery, disease modeling, and therapy—of this reprogramming of adult somatic cells into iCPCs.]](https://writing.wisc.edu/wp-content/uploads/sites/535/2019/08/Abstract-3.png)
Sample Abstract 4, a Structured Abstract
Reporting results about the effectiveness of antibiotic therapy in managing acute bacterial sinusitis, from a rigorously controlled study
Note: This journal requires authors to organize their abstract into four specific sections, with strict word limits. Because the headings for this structured abstract are self-explanatory, we have chosen not to add annotations to this sample abstract.
Wald, Ellen R., David Nash, and Jens Eickhoff. “Effectiveness of Amoxicillin/Clavulanate Potassium in the Treatment of Acute Bacterial Sinusitis in Children.” Pediatrics , vol. 124, no. 1, 2009, pp. 9-15.
“OBJECTIVE: The role of antibiotic therapy in managing acute bacterial sinusitis (ABS) in children is controversial. The purpose of this study was to determine the effectiveness of high-dose amoxicillin/potassium clavulanate in the treatment of children diagnosed with ABS.
METHODS : This was a randomized, double-blind, placebo-controlled study. Children 1 to 10 years of age with a clinical presentation compatible with ABS were eligible for participation. Patients were stratified according to age (<6 or ≥6 years) and clinical severity and randomly assigned to receive either amoxicillin (90 mg/kg) with potassium clavulanate (6.4 mg/kg) or placebo. A symptom survey was performed on days 0, 1, 2, 3, 5, 7, 10, 20, and 30. Patients were examined on day 14. Children’s conditions were rated as cured, improved, or failed according to scoring rules.
RESULTS: Two thousand one hundred thirty-five children with respiratory complaints were screened for enrollment; 139 (6.5%) had ABS. Fifty-eight patients were enrolled, and 56 were randomly assigned. The mean age was 6630 months. Fifty (89%) patients presented with persistent symptoms, and 6 (11%) presented with nonpersistent symptoms. In 24 (43%) children, the illness was classified as mild, whereas in the remaining 32 (57%) children it was severe. Of the 28 children who received the antibiotic, 14 (50%) were cured, 4 (14%) were improved, 4(14%) experienced treatment failure, and 6 (21%) withdrew. Of the 28children who received placebo, 4 (14%) were cured, 5 (18%) improved, and 19 (68%) experienced treatment failure. Children receiving the antibiotic were more likely to be cured (50% vs 14%) and less likely to have treatment failure (14% vs 68%) than children receiving the placebo.
CONCLUSIONS : ABS is a common complication of viral upper respiratory infections. Amoxicillin/potassium clavulanate results in significantly more cures and fewer failures than placebo, according to parental report of time to resolution.” (9)
Some Excellent Advice about Writing Abstracts for Basic Science Research Papers, by Professor Adriano Aguzzi from the Institute of Neuropathology at the University of Zurich:

Academic and Professional Writing
This is an accordion element with a series of buttons that open and close related content panels.
Analysis Papers
Reading Poetry
A Short Guide to Close Reading for Literary Analysis
Using Literary Quotations
Play Reviews
Writing a Rhetorical Précis to Analyze Nonfiction Texts
Incorporating Interview Data
Grant Proposals
Planning and Writing a Grant Proposal: The Basics
Additional Resources for Grants and Proposal Writing
Job Materials and Application Essays
Writing Personal Statements for Ph.D. Programs
- Before you begin: useful tips for writing your essay
- Guided brainstorming exercises
- Get more help with your essay
- Frequently Asked Questions
Resume Writing Tips
CV Writing Tips
Cover Letters
Business Letters
Proposals and Dissertations
Resources for Proposal Writers
Resources for Dissertators
Research Papers
Planning and Writing Research Papers
Quoting and Paraphrasing
Writing Annotated Bibliographies
Creating Poster Presentations
Thank-You Notes
Advice for Students Writing Thank-You Notes to Donors
Reading for a Review
Critical Reviews
Writing a Review of Literature
Scientific Reports
Scientific Report Format
Sample Lab Assignment
Writing for the Web
Writing an Effective Blog Post
Writing for Social Media: A Guide for Academics

- How to Write an Abstract for a Dissertation or Thesis
- Doing a PhD
What is a Thesis or Dissertation Abstract?
The Cambridge English Dictionary defines an abstract in academic writing as being “ a few sentences that give the main ideas in an article or a scientific paper ” and the Collins English Dictionary says “ an abstract of an article, document, or speech is a short piece of writing that gives the main points of it ”.
Whether you’re writing up your Master’s dissertation or PhD thesis, the abstract will be a key element of this document that you’ll want to make sure you give proper attention to.
What is the Purpose of an Abstract?
The aim of a thesis abstract is to give the reader a broad overview of what your research project was about and what you found that was novel, before he or she decides to read the entire thesis. The reality here though is that very few people will read the entire thesis, and not because they’re necessarily disinterested but because practically it’s too large a document for most people to have the time to read. The exception to this is your PhD examiner, however know that even they may not read the entire length of the document.
Some people may still skip to and read specific sections throughout your thesis such as the methodology, but the fact is that the abstract will be all that most read and will therefore be the section they base their opinions about your research on. In short, make sure you write a good, well-structured abstract.
How Long Should an Abstract Be?
If you’re a PhD student, having written your 100,000-word thesis, the abstract will be the 300 word summary included at the start of the thesis that succinctly explains the motivation for your study (i.e. why this research was needed), the main work you did (i.e. the focus of each chapter), what you found (the results) and concluding with how your research study contributed to new knowledge within your field.
Woodrow Wilson, the 28th President of the United States of America, once famously said:

The point here is that it’s easier to talk open-endedly about a subject that you know a lot about than it is to condense the key points into a 10-minute speech; the same applies for an abstract. Three hundred words is not a lot of words which makes it even more difficult to condense three (or more) years of research into a coherent, interesting story.
What Makes a Good PhD Thesis Abstract?
Whilst the abstract is one of the first sections in your PhD thesis, practically it’s probably the last aspect that you’ll ending up writing before sending the document to print. The reason being that you can’t write a summary about what you did, what you found and what it means until you’ve done the work.
A good abstract is one that can clearly explain to the reader in 300 words:
- What your research field actually is,
- What the gap in knowledge was in your field,
- The overarching aim and objectives of your PhD in response to these gaps,
- What methods you employed to achieve these,
- You key results and findings,
- How your work has added to further knowledge in your field of study.
Another way to think of this structure is:
- Introduction,
- Aims and objectives,
- Discussion,
- Conclusion.
Following this ‘formulaic’ approach to writing the abstract should hopefully make it a little easier to write but you can already see here that there’s a lot of information to convey in a very limited number of words.
How Do You Write a Good PhD Thesis Abstract?
The biggest challenge you’ll have is getting all the 6 points mentioned above across in your abstract within the limit of 300 words . Your particular university may give some leeway in going a few words over this but it’s good practice to keep within this; the art of succinctly getting your information across is an important skill for a researcher to have and one that you’ll be called on to use regularly as you write papers for peer review.
Keep It Concise
Every word in the abstract is important so make sure you focus on only the key elements of your research and the main outcomes and significance of your project that you want the reader to know about. You may have come across incidental findings during your research which could be interesting to discuss but this should not happen in the abstract as you simply don’t have enough words. Furthermore, make sure everything you talk about in your thesis is actually described in the main thesis.
Make a Unique Point Each Sentence
Keep the sentences short and to the point. Each sentence should give the reader new, useful information about your research so there’s no need to write out your project title again. Give yourself one or two sentences to introduce your subject area and set the context for your project. Then another sentence or two to explain the gap in the knowledge; there’s no need or expectation for you to include references in the abstract.
Explain Your Research
Some people prefer to write their overarching aim whilst others set out their research questions as they correspond to the structure of their thesis chapters; the approach you use is up to you, as long as the reader can understand what your dissertation or thesis had set out to achieve. Knowing this will help the reader better understand if your results help to answer the research questions or if further work is needed.
Keep It Factual
Keep the content of the abstract factual; that is to say that you should avoid bringing too much or any opinion into it, which inevitably can make the writing seem vague in the points you’re trying to get across and even lacking in structure.
Write, Edit and Then Rewrite
Spend suitable time editing your text, and if necessary, completely re-writing it. Show the abstract to others and ask them to explain what they understand about your research – are they able to explain back to you each of the 6 structure points, including why your project was needed, the research questions and results, and the impact it had on your research field? It’s important that you’re able to convey what new knowledge you contributed to your field but be mindful when writing your abstract that you don’t inadvertently overstate the conclusions, impact and significance of your work.
Thesis and Dissertation Abstract Examples
Perhaps the best way to understand how to write a thesis abstract is to look at examples of what makes a good and bad abstract.
Example of A Bad Abstract
Let’s start with an example of a bad thesis abstract:
In this project on “The Analysis of the Structural Integrity of 3D Printed Polymers for use in Aircraft”, my research looked at how 3D printing of materials can help the aviation industry in the manufacture of planes. Plane parts can be made at a lower cost using 3D printing and made lighter than traditional components. This project investigated the structural integrity of EBM manufactured components, which could revolutionise the aviation industry.
What Makes This a Bad Abstract
Hopefully you’ll have spotted some of the reasons this would be considered a poor abstract, not least because the author used up valuable words by repeating the lengthy title of the project in the abstract.
Working through our checklist of the 6 key points you want to convey to the reader:
- There has been an attempt to introduce the research area , albeit half-way through the abstract but it’s not clear if this is a materials science project about 3D printing or is it about aircraft design.
- There’s no explanation about where the gap in the knowledge is that this project attempted to address.
- We can see that this project was focussed on the topic of structural integrity of materials in aircraft but the actual research aims or objectives haven’t been defined.
- There’s no mention at all of what the author actually did to investigate structural integrity. For example was this an experimental study involving real aircraft, or something in the lab, computer simulations etc.
- The author also doesn’t tell us a single result of his research, let alone the key findings !
- There’s a bold claim in the last sentence of the abstract that this project could revolutionise the aviation industry, and this may well be the case, but based on the abstract alone there is no evidence to support this as it’s not even clear what the author did .
This is an extreme example but is a good way to illustrate just how unhelpful a poorly written abstract can be. At only 71 words long, it definitely hasn’t maximised the amount of information that could be presented and the what they have presented has lacked clarity and structure.
A final point to note is the use of the EBM acronym, which stands for Electron Beam Melting in the context of 3D printing; this is a niche acronym for the author to assume that the reader would know the meaning of. It’s best to avoid acronyms in your abstract all together even if it’s something that you might expect most people to know about, unless you specifically define the meaning first.
Example of A Good Abstract
Having seen an example of a bad thesis abstract, now lets look at an example of a good PhD thesis abstract written about the same (fictional) project:
Additive manufacturing (AM) of titanium alloys has the potential to enable cheaper and lighter components to be produced with customised designs for use in aircraft engines. Whilst the proof-of-concept of these have been promising, the structural integrity of AM engine parts in response to full thrust and temperature variations is not clear.
The primary aim of this project was to determine the fracture modes and mechanisms of AM components designed for use in Boeing 747 engines. To achieve this an explicit finite element (FE) model was developed to simulate the environment and parameters that the engine is exposed to during flight. The FE model was validated using experimental data replicating the environmental parameters in a laboratory setting using ten AM engine components provided by the industry sponsor. The validated FE model was then used to investigate the extent of crack initiation and propagation as the environment parameters were adjusted.
This project was the first to investigate fracture patterns in AM titanium components used in aircraft engines; the key finding was that the presence of cavities within the structures due to errors in the printing process, significantly increased the risk of fracture. Secondly, the simulations showed that cracks formed within AM parts were more likely to worsen and lead to component failure at subzero temperatures when compared to conventionally manufactured parts. This has demonstrated an important safety concern which needs to be addressed before AM parts can be used in commercial aircraft.
What Makes This a Good Abstract
Having read this ‘good abstract’ you should have a much better understand about what the subject area is about, where the gap in the knowledge was, the aim of the project, the methods that were used, key results and finally the significance of these results. To break these points down further, from this good abstract we now know that:
- The research area is around additive manufacturing (i.e. 3D printing) of materials for use in aircraft.
- The gap in knowledge was how these materials will behave structural when used in aircraft engines.
- The aim was specifically to investigate how the components can fracture.
- The methods used to investigate this were a combination of computational and lab based experimental modelling.
- The key findings were the increased risk of fracture of these components due to the way they are manufactured.
- The significance of these findings were that it showed a potential risk of component failure that could comprise the safety of passengers and crew on the aircraft.
The abstract text has a much clearer flow through these different points in how it’s written and has made much better use of the available word count. Acronyms have even been used twice in this good abstract but they were clearly defined the first time they were introduced in the text so that there was no confusion about their meaning.
The abstract you write for your dissertation or thesis should succinctly explain to the reader why the work of your research was needed, what you did, what you found and what it means. Most people that come across your thesis, including any future employers, are likely to read only your abstract. Even just for this reason alone, it’s so important that you write the best abstract you can; this will not only convey your research effectively but also put you in the best light possible as a researcher.
Browse PhDs Now
Join thousands of students.
Join thousands of other students and stay up to date with the latest PhD programmes, funding opportunities and advice.

Dissertation Structure & Layout 101: How to structure your dissertation, thesis or research project.
By: Derek Jansen (MBA) Reviewed By: David Phair (PhD) | July 2019
So, you’ve got a decent understanding of what a dissertation is , you’ve chosen your topic and hopefully you’ve received approval for your research proposal . Awesome! Now its time to start the actual dissertation or thesis writing journey.
To craft a high-quality document, the very first thing you need to understand is dissertation structure . In this post, we’ll walk you through the generic dissertation structure and layout, step by step. We’ll start with the big picture, and then zoom into each chapter to briefly discuss the core contents. If you’re just starting out on your research journey, you should start with this post, which covers the big-picture process of how to write a dissertation or thesis .
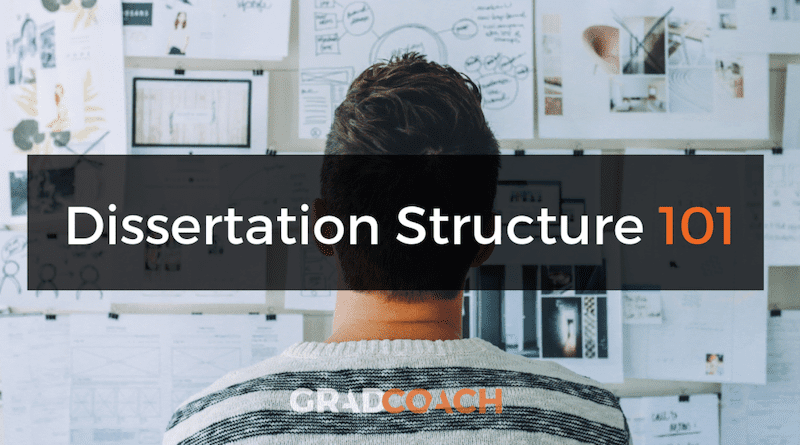
*The Caveat *
In this post, we’ll be discussing a traditional dissertation/thesis structure and layout, which is generally used for social science research across universities, whether in the US, UK, Europe or Australia. However, some universities may have small variations on this structure (extra chapters, merged chapters, slightly different ordering, etc).
So, always check with your university if they have a prescribed structure or layout that they expect you to work with. If not, it’s safe to assume the structure we’ll discuss here is suitable. And even if they do have a prescribed structure, you’ll still get value from this post as we’ll explain the core contents of each section.
Overview: S tructuring a dissertation or thesis
- Acknowledgements page
- Abstract (or executive summary)
- Table of contents , list of figures and tables
- Chapter 1: Introduction
- Chapter 2: Literature review
- Chapter 3: Methodology
- Chapter 4: Results
- Chapter 5: Discussion
- Chapter 6: Conclusion
- Reference list
As I mentioned, some universities will have slight variations on this structure. For example, they want an additional “personal reflection chapter”, or they might prefer the results and discussion chapter to be merged into one. Regardless, the overarching flow will always be the same, as this flow reflects the research process , which we discussed here – i.e.:
- The introduction chapter presents the core research question and aims .
- The literature review chapter assesses what the current research says about this question.
- The methodology, results and discussion chapters go about undertaking new research about this question.
- The conclusion chapter (attempts to) answer the core research question .
In other words, the dissertation structure and layout reflect the research process of asking a well-defined question(s), investigating, and then answering the question – see below.
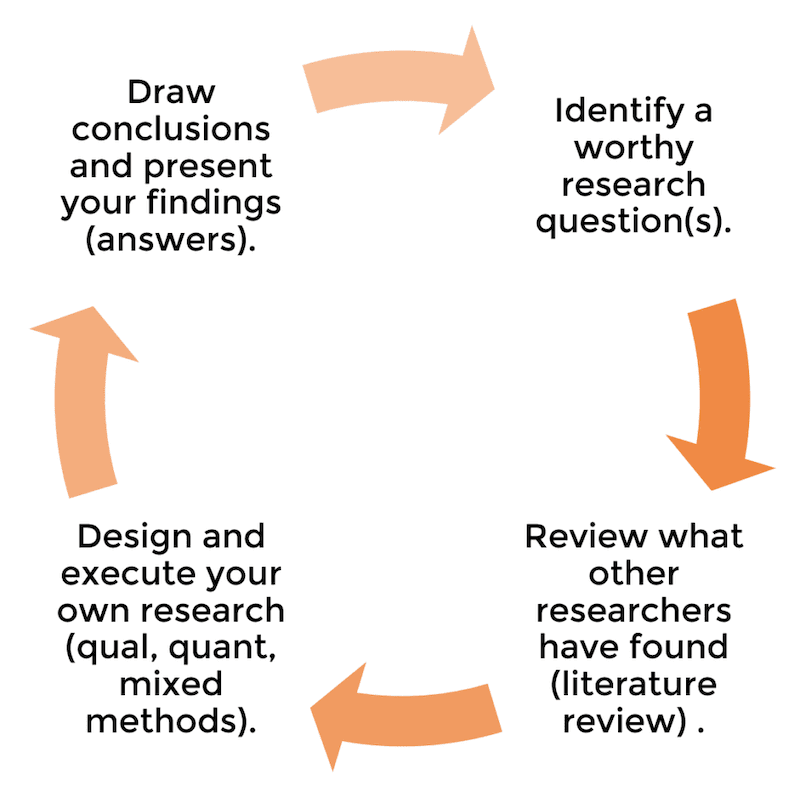
To restate that – the structure and layout of a dissertation reflect the flow of the overall research process . This is essential to understand, as each chapter will make a lot more sense if you “get” this concept. If you’re not familiar with the research process, read this post before going further.
Right. Now that we’ve covered the big picture, let’s dive a little deeper into the details of each section and chapter. Oh and by the way, you can also grab our free dissertation/thesis template here to help speed things up.
The title page of your dissertation is the very first impression the marker will get of your work, so it pays to invest some time thinking about your title. But what makes for a good title? A strong title needs to be 3 things:
- Succinct (not overly lengthy or verbose)
- Specific (not vague or ambiguous)
- Representative of the research you’re undertaking (clearly linked to your research questions)
Typically, a good title includes mention of the following:
- The broader area of the research (i.e. the overarching topic)
- The specific focus of your research (i.e. your specific context)
- Indication of research design (e.g. quantitative , qualitative , or mixed methods ).
For example:
A quantitative investigation [research design] into the antecedents of organisational trust [broader area] in the UK retail forex trading market [specific context/area of focus].
Again, some universities may have specific requirements regarding the format and structure of the title, so it’s worth double-checking expectations with your institution (if there’s no mention in the brief or study material).
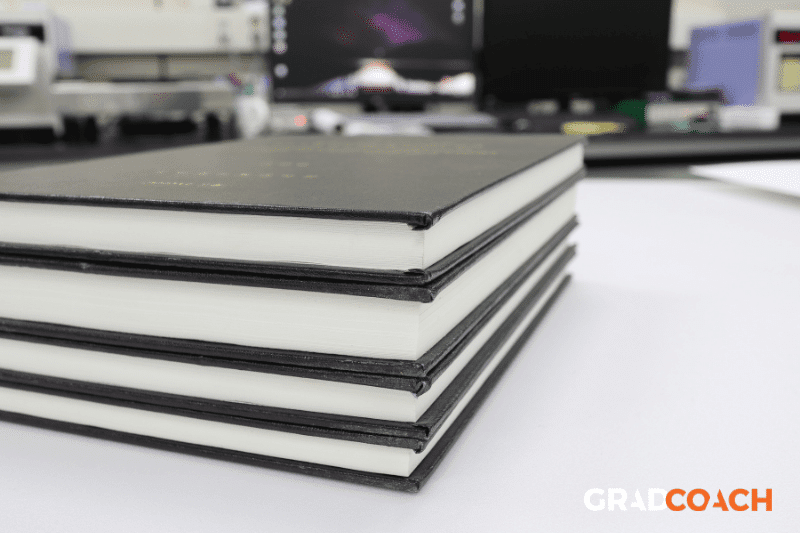
Acknowledgements
This page provides you with an opportunity to say thank you to those who helped you along your research journey. Generally, it’s optional (and won’t count towards your marks), but it is academic best practice to include this.
So, who do you say thanks to? Well, there’s no prescribed requirements, but it’s common to mention the following people:
- Your dissertation supervisor or committee.
- Any professors, lecturers or academics that helped you understand the topic or methodologies.
- Any tutors, mentors or advisors.
- Your family and friends, especially spouse (for adult learners studying part-time).
There’s no need for lengthy rambling. Just state who you’re thankful to and for what (e.g. thank you to my supervisor, John Doe, for his endless patience and attentiveness) – be sincere. In terms of length, you should keep this to a page or less.
Abstract or executive summary
The dissertation abstract (or executive summary for some degrees) serves to provide the first-time reader (and marker or moderator) with a big-picture view of your research project. It should give them an understanding of the key insights and findings from the research, without them needing to read the rest of the report – in other words, it should be able to stand alone .
For it to stand alone, your abstract should cover the following key points (at a minimum):
- Your research questions and aims – what key question(s) did your research aim to answer?
- Your methodology – how did you go about investigating the topic and finding answers to your research question(s)?
- Your findings – following your own research, what did do you discover?
- Your conclusions – based on your findings, what conclusions did you draw? What answers did you find to your research question(s)?
So, in much the same way the dissertation structure mimics the research process, your abstract or executive summary should reflect the research process, from the initial stage of asking the original question to the final stage of answering that question.
In practical terms, it’s a good idea to write this section up last , once all your core chapters are complete. Otherwise, you’ll end up writing and rewriting this section multiple times (just wasting time). For a step by step guide on how to write a strong executive summary, check out this post .
Need a helping hand?
Table of contents
This section is straightforward. You’ll typically present your table of contents (TOC) first, followed by the two lists – figures and tables. I recommend that you use Microsoft Word’s automatic table of contents generator to generate your TOC. If you’re not familiar with this functionality, the video below explains it simply:
If you find that your table of contents is overly lengthy, consider removing one level of depth. Oftentimes, this can be done without detracting from the usefulness of the TOC.
Right, now that the “admin” sections are out of the way, its time to move on to your core chapters. These chapters are the heart of your dissertation and are where you’ll earn the marks. The first chapter is the introduction chapter – as you would expect, this is the time to introduce your research…
It’s important to understand that even though you’ve provided an overview of your research in your abstract, your introduction needs to be written as if the reader has not read that (remember, the abstract is essentially a standalone document). So, your introduction chapter needs to start from the very beginning, and should address the following questions:
- What will you be investigating (in plain-language, big picture-level)?
- Why is that worth investigating? How is it important to academia or business? How is it sufficiently original?
- What are your research aims and research question(s)? Note that the research questions can sometimes be presented at the end of the literature review (next chapter).
- What is the scope of your study? In other words, what will and won’t you cover ?
- How will you approach your research? In other words, what methodology will you adopt?
- How will you structure your dissertation? What are the core chapters and what will you do in each of them?
These are just the bare basic requirements for your intro chapter. Some universities will want additional bells and whistles in the intro chapter, so be sure to carefully read your brief or consult your research supervisor.
If done right, your introduction chapter will set a clear direction for the rest of your dissertation. Specifically, it will make it clear to the reader (and marker) exactly what you’ll be investigating, why that’s important, and how you’ll be going about the investigation. Conversely, if your introduction chapter leaves a first-time reader wondering what exactly you’ll be researching, you’ve still got some work to do.
Now that you’ve set a clear direction with your introduction chapter, the next step is the literature review . In this section, you will analyse the existing research (typically academic journal articles and high-quality industry publications), with a view to understanding the following questions:
- What does the literature currently say about the topic you’re investigating?
- Is the literature lacking or well established? Is it divided or in disagreement?
- How does your research fit into the bigger picture?
- How does your research contribute something original?
- How does the methodology of previous studies help you develop your own?
Depending on the nature of your study, you may also present a conceptual framework towards the end of your literature review, which you will then test in your actual research.
Again, some universities will want you to focus on some of these areas more than others, some will have additional or fewer requirements, and so on. Therefore, as always, its important to review your brief and/or discuss with your supervisor, so that you know exactly what’s expected of your literature review chapter.
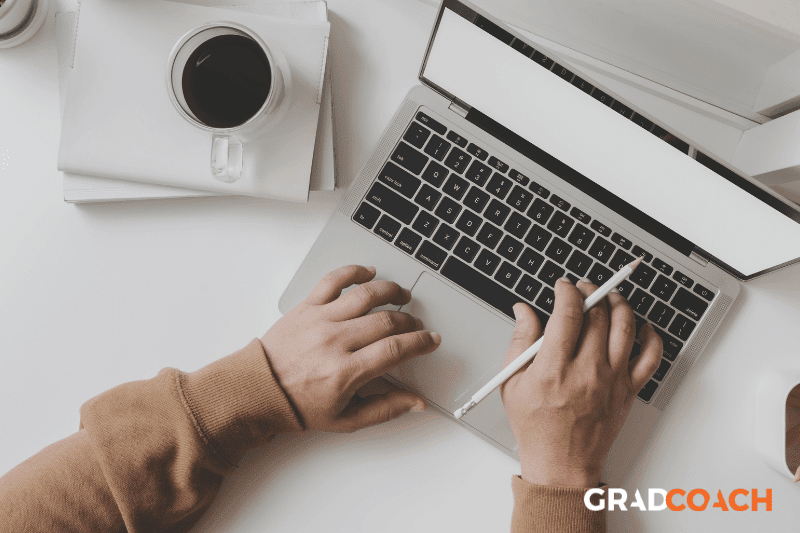
Now that you’ve investigated the current state of knowledge in your literature review chapter and are familiar with the existing key theories, models and frameworks, its time to design your own research. Enter the methodology chapter – the most “science-ey” of the chapters…
In this chapter, you need to address two critical questions:
- Exactly HOW will you carry out your research (i.e. what is your intended research design)?
- Exactly WHY have you chosen to do things this way (i.e. how do you justify your design)?
Remember, the dissertation part of your degree is first and foremost about developing and demonstrating research skills . Therefore, the markers want to see that you know which methods to use, can clearly articulate why you’ve chosen then, and know how to deploy them effectively.
Importantly, this chapter requires detail – don’t hold back on the specifics. State exactly what you’ll be doing, with who, when, for how long, etc. Moreover, for every design choice you make, make sure you justify it.
In practice, you will likely end up coming back to this chapter once you’ve undertaken all your data collection and analysis, and revise it based on changes you made during the analysis phase. This is perfectly fine. Its natural for you to add an additional analysis technique, scrap an old one, etc based on where your data lead you. Of course, I’m talking about small changes here – not a fundamental switch from qualitative to quantitative, which will likely send your supervisor in a spin!
You’ve now collected your data and undertaken your analysis, whether qualitative, quantitative or mixed methods. In this chapter, you’ll present the raw results of your analysis . For example, in the case of a quant study, you’ll present the demographic data, descriptive statistics, inferential statistics , etc.
Typically, Chapter 4 is simply a presentation and description of the data, not a discussion of the meaning of the data. In other words, it’s descriptive, rather than analytical – the meaning is discussed in Chapter 5. However, some universities will want you to combine chapters 4 and 5, so that you both present and interpret the meaning of the data at the same time. Check with your institution what their preference is.
Now that you’ve presented the data analysis results, its time to interpret and analyse them. In other words, its time to discuss what they mean, especially in relation to your research question(s).
What you discuss here will depend largely on your chosen methodology. For example, if you’ve gone the quantitative route, you might discuss the relationships between variables . If you’ve gone the qualitative route, you might discuss key themes and the meanings thereof. It all depends on what your research design choices were.
Most importantly, you need to discuss your results in relation to your research questions and aims, as well as the existing literature. What do the results tell you about your research questions? Are they aligned with the existing research or at odds? If so, why might this be? Dig deep into your findings and explain what the findings suggest, in plain English.
The final chapter – you’ve made it! Now that you’ve discussed your interpretation of the results, its time to bring it back to the beginning with the conclusion chapter . In other words, its time to (attempt to) answer your original research question s (from way back in chapter 1). Clearly state what your conclusions are in terms of your research questions. This might feel a bit repetitive, as you would have touched on this in the previous chapter, but its important to bring the discussion full circle and explicitly state your answer(s) to the research question(s).
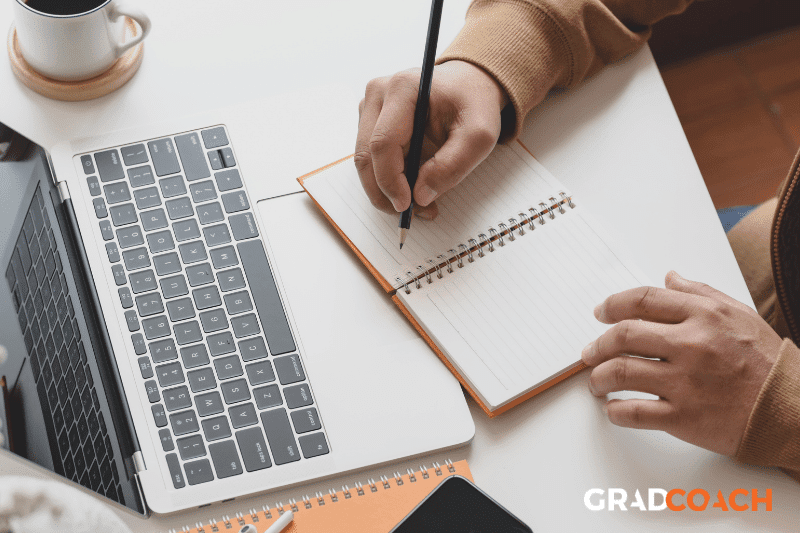
Next, you’ll typically discuss the implications of your findings? In other words, you’ve answered your research questions – but what does this mean for the real world (or even for academia)? What should now be done differently, given the new insight you’ve generated?
Lastly, you should discuss the limitations of your research, as well as what this means for future research in the area. No study is perfect, especially not a Masters-level. Discuss the shortcomings of your research. Perhaps your methodology was limited, perhaps your sample size was small or not representative, etc, etc. Don’t be afraid to critique your work – the markers want to see that you can identify the limitations of your work. This is a strength, not a weakness. Be brutal!
This marks the end of your core chapters – woohoo! From here on out, it’s pretty smooth sailing.
The reference list is straightforward. It should contain a list of all resources cited in your dissertation, in the required format, e.g. APA , Harvard, etc.
It’s essential that you use reference management software for your dissertation. Do NOT try handle your referencing manually – its far too error prone. On a reference list of multiple pages, you’re going to make mistake. To this end, I suggest considering either Mendeley or Zotero. Both are free and provide a very straightforward interface to ensure that your referencing is 100% on point. I’ve included a simple how-to video for the Mendeley software (my personal favourite) below:
Some universities may ask you to include a bibliography, as opposed to a reference list. These two things are not the same . A bibliography is similar to a reference list, except that it also includes resources which informed your thinking but were not directly cited in your dissertation. So, double-check your brief and make sure you use the right one.
The very last piece of the puzzle is the appendix or set of appendices. This is where you’ll include any supporting data and evidence. Importantly, supporting is the keyword here.
Your appendices should provide additional “nice to know”, depth-adding information, which is not critical to the core analysis. Appendices should not be used as a way to cut down word count (see this post which covers how to reduce word count ). In other words, don’t place content that is critical to the core analysis here, just to save word count. You will not earn marks on any content in the appendices, so don’t try to play the system!
Time to recap…
And there you have it – the traditional dissertation structure and layout, from A-Z. To recap, the core structure for a dissertation or thesis is (typically) as follows:
- Acknowledgments page
Most importantly, the core chapters should reflect the research process (asking, investigating and answering your research question). Moreover, the research question(s) should form the golden thread throughout your dissertation structure. Everything should revolve around the research questions, and as you’ve seen, they should form both the start point (i.e. introduction chapter) and the endpoint (i.e. conclusion chapter).
I hope this post has provided you with clarity about the traditional dissertation/thesis structure and layout. If you have any questions or comments, please leave a comment below, or feel free to get in touch with us. Also, be sure to check out the rest of the Grad Coach Blog .
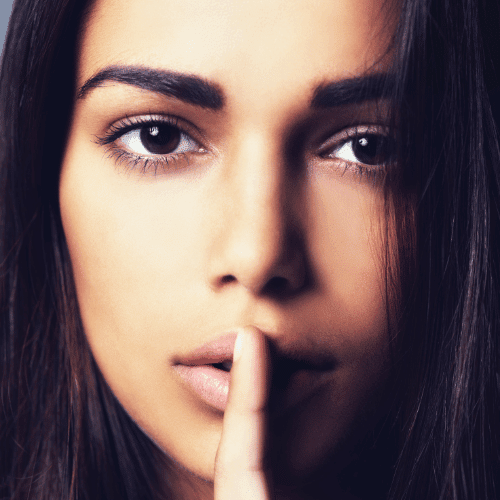
Psst... there’s more!
This post was based on one of our popular Research Bootcamps . If you're working on a research project, you'll definitely want to check this out ...
You Might Also Like:
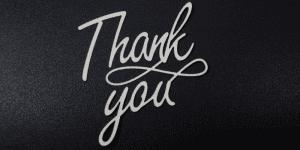
36 Comments
many thanks i found it very useful
Glad to hear that, Arun. Good luck writing your dissertation.
Such clear practical logical advice. I very much needed to read this to keep me focused in stead of fretting.. Perfect now ready to start my research!
what about scientific fields like computer or engineering thesis what is the difference in the structure? thank you very much
Thanks so much this helped me a lot!
Very helpful and accessible. What I like most is how practical the advice is along with helpful tools/ links.
Thanks Ade!
Thank you so much sir.. It was really helpful..
You’re welcome!
Hi! How many words maximum should contain the abstract?
Thank you so much 😊 Find this at the right moment
You’re most welcome. Good luck with your dissertation.
best ever benefit i got on right time thank you
Many times Clarity and vision of destination of dissertation is what makes the difference between good ,average and great researchers the same way a great automobile driver is fast with clarity of address and Clear weather conditions .
I guess Great researcher = great ideas + knowledge + great and fast data collection and modeling + great writing + high clarity on all these
You have given immense clarity from start to end.
Morning. Where will I write the definitions of what I’m referring to in my report?
Thank you so much Derek, I was almost lost! Thanks a tonnnn! Have a great day!
Thanks ! so concise and valuable
This was very helpful. Clear and concise. I know exactly what to do now.
Thank you for allowing me to go through briefly. I hope to find time to continue.
Really useful to me. Thanks a thousand times
Very interesting! It will definitely set me and many more for success. highly recommended.
Thank you soo much sir, for the opportunity to express my skills
Usefull, thanks a lot. Really clear
Very nice and easy to understand. Thank you .
That was incredibly useful. Thanks Grad Coach Crew!
My stress level just dropped at least 15 points after watching this. Just starting my thesis for my grad program and I feel a lot more capable now! Thanks for such a clear and helpful video, Emma and the GradCoach team!
Do we need to mention the number of words the dissertation contains in the main document?
It depends on your university’s requirements, so it would be best to check with them 🙂
Such a helpful post to help me get started with structuring my masters dissertation, thank you!
Great video; I appreciate that helpful information
It is so necessary or avital course
This blog is very informative for my research. Thank you
Doctoral students are required to fill out the National Research Council’s Survey of Earned Doctorates
wow this is an amazing gain in my life
This is so good
How can i arrange my specific objectives in my dissertation?
Trackbacks/Pingbacks
- What Is A Literature Review (In A Dissertation Or Thesis) - Grad Coach - […] is to write the actual literature review chapter (this is usually the second chapter in a typical dissertation or…
Submit a Comment Cancel reply
Your email address will not be published. Required fields are marked *
Save my name, email, and website in this browser for the next time I comment.
- Print Friendly

How to Write a Social Science or Humanities Thesis/Dissertation
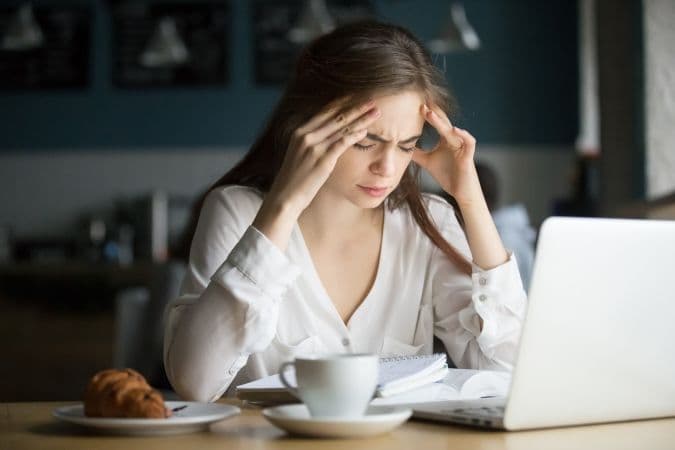
Writing a thesis/dissertation is a huge task, and it is common to feel overwhelmed at the start. A thesis and a dissertation are both long pieces of focused research written as the sum of your graduate or postgraduate course.
The difference between a thesis and a dissertation can depend on which part of the world you are in. In Europe, a dissertation is written as part of a Master’s degree, while a thesis is written by doctoral students. In the US, a thesis is generally the major research paper written by Master’s students to complete their programs, while a dissertation is written at the doctoral level.
The purpose of both types of research is generally the same: to demonstrate that you, the student, is capable of performing a degree of original, structured, long-term research. Writing a thesis/dissertation gives you experience in project planning and management, and allows you the opportunity to develop your expertise in a particular subject of interest. In that sense, a thesis/dissertation is a luxury, as you are allowed time and resources to pursue your own personal academic interest.
Writing a thesis/dissertation is a larger project than the shorter papers you likely wrote in your coursework. Therefore, the structure of a thesis/dissertation can differ from what you are used to. It may also differ based on what field you are in and what kind of research you do. In this article, we’ll look at how to structure a humanities or social science thesis/dissertation and offer some tips for writing such a big paper. Once you have a solid understanding of how your thesis/dissertation should be structured, you will be ready to begin writing.
How are humanities and social science thesis/dissertations structured?
The structure of a thesis/dissertation will vary depending on the topic, your academic discipline, methodology, and the place you are studying in. Generally, social science and humanities theses/dissertations are structured differently from those in natural sciences, as there are differences in methodologies and sources. However, some social science theses/dissertations can use the same format as natural science dissertations, especially if it heavily uses quantitative research methods. Such theses/dissertations generally follow the “IMRAD” model :
- Introduction
Social science theses/dissertations often range from 80-120 pages in length.
Humanities thesis/dissertations, on the other hand, are often structured more like long essays. This is because these theses/dissertations rely more heavily on discussions of previous literature and/or case studies. They build up an argument around a central thesis citing literature and case studies as examples. Humanities theses/dissertations tend to range from between 100-300 pages in length.
The parts of a dissertation: Starting out
Never assume what your reader knows! Explain every step of your process clearly and concisely as you write, and structure your thesis/dissertation with this goal in mind.
As you prepare your topic and structure your social science or humanities thesis/dissertation, always keep your audience in mind. Who are you writing for? Even if your topic is other experts in the field, you should aim to write in sufficient detail that someone unfamiliar with your topic could follow along. Never assume what your reader knows! Explain every step of your process clearly and concisely as you write, and structure your thesis/dissertation with this goal in mind.
While the structure of social science and humanities theses/dissertations differ somewhat, they both have some basic elements in common. Both types will typically begin with the following elements:
What is the title of your paper?
A good title is catchy and concisely indicates what your paper is about. This page also likely has your name, department and advisor information, and ID number. However, the specific information listed varies by institution.
Acknowledgments page
Many people probably helped you write your thesis/dissertation. If you want to say thank you, this is the place where it can be included.
Your abstract is a one-page summary (300 words or less) of your entire paper. Beginning with your thesis/dissertation question and a brief background information, it explains your research and findings. This is what most people will read before they decide whether to read your paper or not, so you should make it compelling and to the point.
Table of contents
This section lists the chapter and subchapter titles along with their page numbers. It should be written to help your reader easily navigate through your thesis/dissertation.
While these elements are found at the beginning of your humanities or social science thesis/dissertation, most people write them last. Otherwise, they’ll undergo a lot of needless revisions, particularly the table of contents, as you revise, edit, and proofread your thesis/dissertation.
The parts of a humanities thesis/dissertation
As we mentioned above, humanities and some social science theses/dissertations follow an essay-like structure . A typical humanities thesis/dissertation structure includes the following chapters:
- References (Bibliography)
The number of themes above was merely chosen as an example.
In a humanities thesis/dissertation, the introduction and background are often not separate chapters. The introduction and background of a humanities thesis/dissertation introduces the overall topic and provides the reader with a guide for how you will approach the issue. You can then explain why the topic is of interest, highlight the main debates in the field, and provide background information. Then you explain what you are investigating and why. You should also specifically indicate your hypothesis before moving on to the first thematic chapter.
Thematic chapters (and you can have as many of them as your thesis/dissertation guidelines allow) are generally structured as follows:
- Introduction: Briefly introduce the theme of the chapter and inform the reader what you are going to talk about.
- Argument : State the argument the chapter presents
- Material : Discuss the material you will be using
- Analysis : Provide an analysis of the materials used
- Conclusion : How does this relate to your main argument and connect to the next theme chapter?
Finally, the conclusion of your paper will bring everything together and summarize your argument clearly. This is followed by the references or bibliography section, which lists all of the sources you cited in your thesis/dissertation.
The parts of a social science thesis/dissertation
In contrast to the essay structure of a humanities thesis/dissertation, a typical social science thesis/dissertation structure includes the following chapters:
- Literature Review
- Methodology
Unlike the humanities thesis/dissertation, the introduction and literature review sections are clearly separated in a social science thesis/dissertation. The introduction tells your reader what you will talk about and presents the significance of your topic within the broader context. By the end of your introduction, it should be clear to your reader what you are doing, how you are doing it, and why.
The literature review analyzes the existing research and centres your own work within it. It should provide the reader with a clear understanding of what other people have said about the topic you are investigating. You should make it clear whether the topic you will research is contentious or not, and how much research has been done. Finally, you should explain how this thesis/dissertation will fit within the existing research and what it contributes to the literature overall.
In the methodology section of a social science thesis/dissertation, you should clearly explain how you have performed your research. Did you use qualitative or quantitative methods? How was your process structured? Why did you do it this way? What are the limitations (weaknesses) of your methodological approach?
Once you have explained your methods, it is time to provide your results . What did your research find? This is followed by the discussion , which explores the significance of your results and whether or not they were as you expected. If your research yielded the expected results, why did that happen? If not, why not? Finally, wrap up with a conclusion that reiterates what you did and why it matters, and point to future matters for research. The bibliography section lists all of the sources you cited, and the appendices list any extra information or resources such as raw data, survey questions, etc. that your reader may want to know.
In social science theses/dissertations that rely more heavily on qualitative rather than quantitative methods, the above structure can still be followed. However, sometimes the results and discussion chapters will be intertwined or combined. Certain types of social science theses/dissertations, such as public policy, history, or anthropology, may follow the humanities thesis/dissertation structure as we mentioned above.
Critical steps for writing and structuring a humanities/social science thesis/dissertation
If you are still struggling to get started, here is a checklist of steps for writing and structuring your humanities or social science thesis/dissertation.
- Choose your thesis/dissertation topic
- What is the word count/page length requirement?
- What chapters must be included?
- What chapters are optional?
- Conduct preliminary research
- Decide on your own research methodology
- Outline your proposed methods and expected results
- Use your proposed methodology to choose what chapters to include in your thesis/dissertation
- Create a preliminary table of contents to outline the structure of your thesis/dissertation
By following these steps, you should be able to organize the structure of your humanities or social science thesis/dissertation before you begin writing.
Final tips for writing and structuring a thesis/dissertation
Although writing a thesis/dissertation is a difficult project, it is also very rewarding. You will get the most out of the experience if you properly prepare yourself by carefully learning about each step. Before you decide how to structure your thesis/dissertation, you will need to decide on a thesis topic and come up with a hypothesis. You should do as much preliminary reading and notetaking as you have time for.
Since most people writing a thesis/dissertation are doing it for the first time, you should also take some time to learn about the many tools that exist to help students write better and organize their citations. Citation generators and reference managers like EndNote help you keep track of your sources and AI grammar and writing checkers are helpful as you write. You should also keep in mind that you will need to edit and proofread your thesis/dissertation once you have the bulk of the writing complete. Many thesis editing and proofreading services are available to help you with this as well.
Editor’s pick
Get free updates.
Subscribe to our newsletter for regular insights from the research and publishing industry!
What are the parts of a social science thesis/dissertation? +
A social science thesis/dissertation is usually structured as follows:
How long is a typical social science thesis/dissertation? +
What are the parts of a humanities thesis/dissertation +.
Humanities theses/dissertations are usually structured like this:
- Thematic Chapters
What is the typical structure of a thematic chapter in a humanities thesis/dissertation? +
A thematic chapter in a humanities thesis/dissertation is structured like this:
How long is a typical humanities thesis/dissertation? +
A typical humanities thesis/dissertation tends to range from 100 to 300 pages in length.
(Stanford users can avoid this Captcha by logging in.)
- Send to text email RefWorks EndNote printer
Dissertation abstracts international. A, The humanities and social sciences
Available online.
- search.proquest.com
At the library

SAL1&2 (on-campus storage)

SAL3 (off-campus storage)
More options.
- Find it at other libraries via WorldCat
- Contributors
Description
Creators/contributors, bibliographic information, browse related items.

- Stanford Home
- Maps & Directions
- Search Stanford
- Emergency Info
- Terms of Use
- Non-Discrimination
- Accessibility
© Stanford University , Stanford , California 94305 .
Next available on Monday 9 a.m.–5 p.m.

Additional Options
- smartphone Call / Text
- voice_chat Consultation Appointment
- place Visit
- email Email
Chat with a Specific library
- Business Library Offline
- College Library (Undergraduate) Offline
- Ebling Library (Health Sciences) Offline
- Gender and Women's Studies Librarian Offline
- Information School Library (Information Studies) Offline
- Law Library (Law) Offline
- Memorial Library (Humanities & Social Sciences) Offline
- MERIT Library (Education) Offline
- Steenbock Library (Agricultural & Life Sciences, Engineering) Offline
- Ask a Librarian Hours & Policy
- Library Research Tutorials
Search the for Website expand_more Articles Find articles in journals, magazines, newspapers, and more Catalog Explore books, music, movies, and more Databases Locate databases by title and description Journals Find journal titles UWDC Discover digital collections, images, sound recordings, and more Website Find information on spaces, staff, services, and more
Language website search.
Find information on spaces, staff, and services.
- ASK a Librarian
- Library by Appointment
- Locations & Hours
- Resources by Subject
book Catalog Search
Search the physical and online collections at UW-Madison, UW System libraries, and the Wisconsin Historical Society.
- Available Online
- Print/Physical Items
- Limit to UW-Madison
- Advanced Search
- Browse by...
collections_bookmark Database Search
Find databases subscribed to by UW-Madison Libraries, searchable by title and description.
- Browse by Subject/Type
- Introductory Databases
- Top 10 Databases
article Journal Search
Find journal titles available online and in print.
- Browse by Subject / Title
- Citation Search
description Article Search
Find articles in journals, magazines, newspapers, and more.
- Scholarly (peer-reviewed)
- Open Access
- Library Databases
collections UW Digital Collections Search
Discover digital objects and collections curated by the UW Digital Collections Center .
- Browse Collections
- Browse UWDC Items
- University of Wisconsin–Madison
- Email/Calendar
- Google Apps
- Loans & Requests
- Poster Printing
- Account Details
- Archives and Special Collections Requests
- Library Room Reservations
Search the UW-Madison Libraries
Catalog search.

Dissertation abstracts international. A, The humanities and social sciences
"Abstracts of dissertations available on microfilm or as xerographic reproductions."
- toc Request Options
- format_quote Citation
Physical Locations
Publication details.
- University Microfilms
- Xerox University Microfilms
- University Microfilms International
- Ann Arbor, Mich. : University Microfilms International, 1969-
- No. 12, pt. 2 of v. 30-33 contains the annual cumulated keyword title and author indexes; no. 12, pt. 2 of v. 34-54 and no. 12 of v. 55- contain the annual cumulated author index to sections A and B of Dissertation abstracts international
- Dissertation abstracts. A, Humanities and social sciences
- Issued also on microfilm.
- Also issued online.
- Issued also on CD-ROM (without abstracts, July 1969-June 1980) as part of: Dissertation abstracts ondisc.
- Humanities -- Abstracts -- Periodicals.
- Humanities -- United States -- Abstracts -- Periodicals.
- Social sciences -- Abstracts -- Periodicals.
- Social sciences -- United States -- Abstracts -- Periodicals.
- Dissertations, Academic -- Abstracts -- Periodicals.
- Dissertations, Academic -- United States -- Abstracts -- Periodicals.
- Dissertations, Academic -- Abstracts. Medical Subject Heading
- Humanities -- Abstracts. Medical Subject Heading
- Social Sciences -- Abstracts. Medical Subject Heading
Content Types
- Periodicals
Identifiers
Linking ISSN (ISSN-L): 0419-4209
URL http://newfirstsearch.oclc.org/FSIP
Google https://www.google.com/search?q=ISSN+%220419-4209%22
Bing https://www.bing.com/search?q=ISSN+%220419-4209%22
Yahoo https://search.yahoo.com/search?p=ISSN%20%220419-4209%22
Library of Congress https://catalog.loc.gov/vwebv/search?searchCode=STNO&searchArg=0419-4209&searchType=1&limitTo=none&fromYear=&toYear=&limitTo=LOCA%3Dall&limitTo=PLAC%3Dall&limitTo=TYPE%3Dall&limitTo=LANG%3Dall&recCount=25
Resource information
Title proper: Dissertation abstracts international. The humanities and social sciences.
Country: United States
Medium: Print
Record information
Last modification date: 06/02/2021
Type of record: Confirmed
ISSN Center responsible of the record: ISSN National Centre for the USA
downloads requested
Discover all the features of the complete ISSN records
Display mode x.
Labelled view
MARC21 view
UNIMARC view
- Search Menu
- Advance articles
- Author Guidelines
- Submission Site
- Open Access
- Why Submit?
- About Public Opinion Quarterly
- About the American Association for Public Opinion Research
- Editorial Board
- Advertising and Corporate Services
- Journals Career Network
- Self-Archiving Policy
- Dispatch Dates
- Journals on Oxford Academic
- Books on Oxford Academic
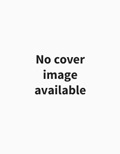
Article Contents
Anti-semitic attitudes of the mass public: estimates and explanations based on a survey of the moscow oblast.
- Article contents
- Figures & tables
- Supplementary Data
JAMES L. GIBSON, RAYMOND M. DUCH, ANTI-SEMITIC ATTITUDES OF THE MASS PUBLIC: ESTIMATES AND EXPLANATIONS BASED ON A SURVEY OF THE MOSCOW OBLAST, Public Opinion Quarterly , Volume 56, Issue 1, SPRING 1992, Pages 1–28, https://doi.org/10.1086/269293
- Permissions Icon Permissions
In this article we examine anti-Semitism as expressed by a sample of residents of the Moscow Oblast (Soviet Union). Based on a survey conducted in 1920, we begin by describing anti-Jewish prejudice and support for official discrimination against Jews. We discover a surprisingly low level of expressed anti-Semitism among these Soviet respondents and virtually no support for state policies that discriminate against Jews. At the same time, many of the conventional hypotheses predicting anti-Semitism are supported in the Soviet case. Anti-Semitism is concentrated among those with lower levels of education, those whose personal financial condition is deteriorating, and those who oppose further democratization of the Soviet Union. We do not take these findings as evidence that anti-Semitism is a trivial problem in the Soviet Union but, rather, suggest that efforts to combat anti-Jewish movements would likely receive considerable support from ordinary Soviet people.
Email alerts
Citing articles via.
- Recommend to your Library
Affiliations
- Online ISSN 1537-5331
- Copyright © 2024 American Association for Public Opinion Research
- About Oxford Academic
- Publish journals with us
- University press partners
- What we publish
- New features
- Open access
- Institutional account management
- Rights and permissions
- Get help with access
- Accessibility
- Advertising
- Media enquiries
- Oxford University Press
- Oxford Languages
- University of Oxford
Oxford University Press is a department of the University of Oxford. It furthers the University's objective of excellence in research, scholarship, and education by publishing worldwide
- Copyright © 2024 Oxford University Press
- Cookie settings
- Cookie policy
- Privacy policy
- Legal notice
This Feature Is Available To Subscribers Only
Sign In or Create an Account
This PDF is available to Subscribers Only
For full access to this pdf, sign in to an existing account, or purchase an annual subscription.
Thank you for visiting nature.com. You are using a browser version with limited support for CSS. To obtain the best experience, we recommend you use a more up to date browser (or turn off compatibility mode in Internet Explorer). In the meantime, to ensure continued support, we are displaying the site without styles and JavaScript.
- View all journals
- My Account Login
- Explore content
- About the journal
- Publish with us
- Sign up for alerts
- Open access
- Published: 08 May 2024
Estimating and modeling spontaneous mobility changes during the COVID-19 pandemic without stay-at-home orders
- Baining Zhao ORCID: orcid.org/0000-0002-9653-3316 1 , 2 na1 ,
- Xuzhe Wang 1 na1 ,
- Tianyu Zhang 1 ,
- Rongye Shi ORCID: orcid.org/0000-0003-4298-9358 3 ,
- Fengli Xu 4 ,
- Fanhang Man 1 ,
- Erbing Chen 5 ,
- Yang Li ORCID: orcid.org/0000-0002-2053-6393 1 ,
- Yong Li ORCID: orcid.org/0000-0001-5617-1659 4 ,
- Tao Sun ORCID: orcid.org/0000-0003-2570-543X 2 &
- Xinlei Chen 1 , 2 , 6
Humanities and Social Sciences Communications volume 11 , Article number: 591 ( 2024 ) Cite this article
134 Accesses
1 Altmetric
Metrics details
- Health humanities
- Information systems and information technology
- Science, technology and society
- Social anthropology
Comprehending the complex interplay among urban mobility, human behavior, and the COVID-19 pandemic could deliver vital perspectives to steer forthcoming public health endeavors. In late 2022, China lifted its "Zero-COVID" policy and rapidly abandoned nearly all interventions. It provides a unique opportunity to observe spontaneous mobility changes without government restriction throughout such a pandemic with high infection. Based on 148 million travel data from the public bus, subway, and taxi systems in Shenzhen, China, our analysis reveals discernible spatial discrepancies within mobility patterns. This phenomenon can be ascribed to the heterogeneous responses of mobility behavior tailored to specific purposes and travel modes in reaction to the pandemic. Considering both the physiological effects of virus infection and subjective willingness to travel, a dynamic model is proposed and capable of fitting fine-grained urban mobility. The analysis and model can interpret mobility data and underlying population behavior to inform policymakers when evaluating public health strategies against future large-scale infectious diseases.
Similar content being viewed by others
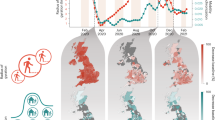
COVID-19 is linked to changes in the time–space dimension of human mobility
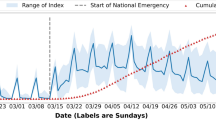
Quantifying human mobility behaviour changes during the COVID-19 outbreak in the United States
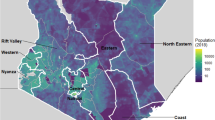
Practical geospatial and sociodemographic predictors of human mobility
Introduction.
As one of the most infectious pandemics, COVID-19 has resulted in a devastating toll of over 7 million lives lost and an estimated $8 trillion in economic damage (Clark et al., 2020 ; Taskinsoy, 2020 ; Weiss et al., 2020 ; Zhang et al., 2022 ). Research on the COVID-19 pandemic and its impact holds the potential to offer valuable insights for addressing unforeseen large-scale, highly infectious diseases in the future (Chen et al., 2022 ; Menkir et al., 2021 ; Sibley et al., 2020 ).
Due to rapid urbanization, more than 55% of the global population resides in urban areas which serve as a hotbed for infectious diseases due to dense population (United Nations, 2018 ). It is imminent to study the response and recuperation of urban mobility during the influence of COVID-19 (Arellana et al., 2020 ; Atkinson-Clement and Pigalle, 2021 ; Gkiotsalitis and Cats, 2021 ; Levin et al., 2021 ) to facilitate understanding of the spread of the pandemics (Chang et al., 2021 ; Wei et al., 2021 ). Simultaneously, the change in urban mobility profoundly influences economic dynamics and mental health outcomes, among other societal aspects (Wang et al., 2021 , 2022 ). Existing research has primarily investigated how governments proactively implement mandatory or advisory stay-at-home orders to change people’s mobility behavior and contain virus transmission (Martínez and Short, 2021 ; Shen et al., 2020 ; Tirachini and Cats, 2020 ; Wang et al., 2022 ; Zhang, 2021 ). However, there is limited knowledge regarding the spontaneous change in citizens’ mobility behavior during the COVID-19 pandemic. The spontaneous change consists of individuals’ voluntary adaption of their travel behavior concerning the infection rate of disease and perceived threats, without the restriction of government policies (Han et al., 2021 ). In the case of COVID-19, the self-driven behavior involves engaging in home-based care while infected, limiting travel to essential trips only, avoiding crowded places, shifting on-site work to remote work to reduce potential exposure to the virus, etc. (Balmford et al., 2020 ; Tisdell, 2020 ). A better understanding of these spontaneous mobility changes can provide valuable insights into the necessary mobility behavior of the citizens and in turn facilitate the design of urban mobility-related policies during pandemics.
In this paper, we investigate spontaneous mobility changes without stay-at-home orders throughout a highly infectious pandemic, from its emergence through large-scale proliferation to eventual stabilization. The mobility changes are manifested as fluctuations in the number of individuals traveling, which are microscopically composed of varying purpose-specific mobility behavior. We aim to answer the following research questions:
Q1: At the temporal and spatial scale, how does urban mobility evolve in response to a pandemic’s lifecycle?
Q2: Given distinct regions corresponding to varied functional zones and data pertaining to various modes of transportation within a city, what is the underlying mobility behavior, and how do they react to the pandemic?
Q3: How to establish a dynamic model to deduce the spatio-temporal mobility changes of the entire city based on the behavior of different travel purposes and modes?
To answer these questions, we utilize origin-destination (OD) mobility data involving 148 million occurrences before and after the cancellation of the "Zero-COVID" policy in Shenzhen subway, bus, and taxi systems, China, as shown in Fig. 1 a, b. The OD data logs the time and quantity of individuals traveling from areas proximal to the origin to regions near the destination in the urban public transport system. Such data encapsulates the spatio-temporal mobility of urban populations, focusing solely on the volume of individuals moving between two regions, while avoiding the disclosure of individual-specific information. This ensures a high degree of privacy preservation.
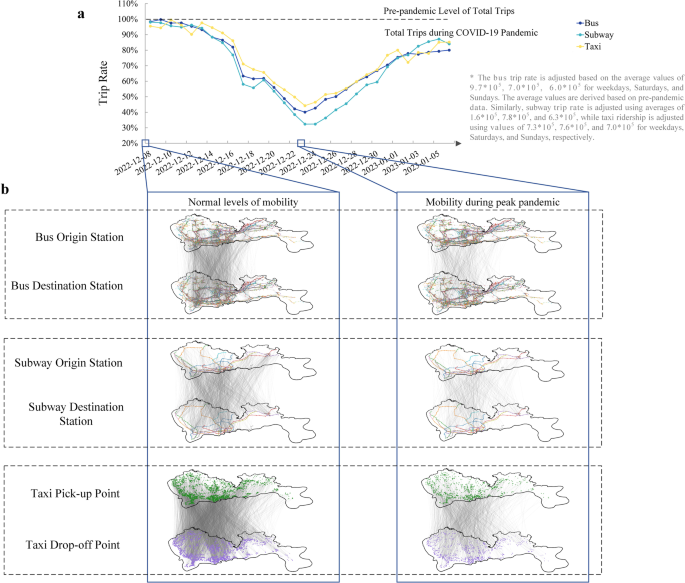
a Trips in the Shenzhen bus, subway, and taxi systems from December 8, 2022, to January 6, 2023. China adhered to a rigorous "Zero-COVID" policy and implemented strong non-pharmaceutical measures from the beginning of the COVID-19 outbreak until early Dec. 2022. As a result, over 99% of the population in China had never been infected by any variant of SARS-CoV-2. Even though China heavily distributed the COVID-19 vaccine, the vaccine merely prevents people from serious symptoms without comprehensive immunity due to the fast variability of COVID-19. On December 7, 2022, the "Zero-COVID" policy, along with the travel restrictions, was rescinded. The travel volume immediately returned to a level approximating normalcy. Thereafter, the spread of SARS-CoV-2 precipitated a substantial decrease in urban mobility. A progressive recovery would then succeed. To highlight the impact of the pandemic more effectively, we conducted normalization separately for weekdays, Saturdays, and Sundays, ultimately showcasing the trip rate. b The lines bridging the origin and destination maps symbolize the human movement between two corresponding regions. Due to the dense nature of OD data, we depict flows exceeding a daily average of 200 for clarity. Three time periods are highlighted: before the COVID-19 pandemic outbreak, during the peak impact, and during the gradual stabilization period. These periods correspond to three different levels of mobility.
The period before and after the cancellation of the "Zero-COVID" policy provides a unique opportunity to observe the spontaneous evolution of urban mobility without mobility-restricting measures, facing the pandemic spreading on a large scale in a short period. The "Zero-COVID" policy focuses on promptly identifying and containing localized outbreaks using advanced technologies such as big data analysis and nucleic acid screening (China CDC, 2022 ). Through strict quarantine measures, identification of infection sources, and high-risk group identification, China aims to swiftly end outbreaks with minimal societal and economic impacts. China’s implementation of the "Zero-COVID" policy has been highly successful. Despite its massive population of 1.4 billion people, the country has reported relatively low numbers of COVID-19 cases and deaths before the cancellation of the "Zero-COVID" policy. Impressively, China has recorded 1,655,477 cases (less than 0.2% of the population) and 13,524 deaths (less than 0.1%% of the population) (Burki, 2022 ). In late 2022, the Omicron variant of SARS-CoV-2 became predominant worldwide and it was known for its high transmissibility (basic reproduction number ≈ 9.5) and penetration rate. Yet, compared to previous variants, it also demonstrated a relatively lower infection fatality rate (<1%) (Liu et al., 2022 ). Under such a circumstance, on Dec. 7, 2022, China lifted the "Zero-COVID" policy along with the travel restrictions. However, the Omicron variant showed a high rate of immune escape from vaccines which merely prevented people from serious symptoms without delivering comprehensive immunity. Therefore, the lift of the policy led to a rapid and massive spread of the Omicron variant of SARS-CoV-2. It is estimated that within a month, the proportion of infected individuals in cities sharply escalated from nearly zero to surpassing 70% (Leung et al., 2023 ). Besides, Shenzhen is a megacity with a permanent population exceeding 17 million individuals and a population density reaching 8,800 individuals per square kilometer (Shenzhen Government, 2023 ). The research on mobility behavior during the pandemic in Shenzhen is representative and informative.
An analytic framework and a dynamic model are proposed with the following key designs. In terms of spatial distribution, mobility in central business districts (CBDs) and their adjacent areas is significantly impacted. The mobility changes can be quantitatively represented by a time series and evaluated by features about the decline and recovery of trips. To explore the spatial disparities, four mobility patterns are found among the mobility changes corresponding to various OD pairs through the K -means++ clustering algorithm (answering Q1 ). We overlay an urban land use (ULU) map on origins and destinations, thus elucidating the intent behind mobility behavior, such as commuting, recreation, schooling, and more. The differences in mobility changes among subways, buses, and taxis are also discussed (answering Q2 ). Inspired by this, we try to devise a dynamic model of changes in passenger flow, predicated on the impact of COVID-19 on different mobility behavior. We model distinct mobility behavior by combining physical infection and the willingness influence, which subsequently deduces the effects of ULU embodying travel intentions. As a result, we can infer the mobility within the geographic regions housing these lands with different uses (answering Q3 ). Our model allows us to not only fit observed changes in trips but also to conduct detailed analysis at a granularity of less than 500m. We believe the proposed insight and model could be leveraged to provide public health officials with a holistic recommendation as they decide on mobility-related policies under similar pandemic conditions.
Spatial distributions of urban mobility level
The spatial distribution of the decline and recovery in mobility levels can be approximately observed based on Fig. 2 . This observation is made at the granularity of a 1km × 1km grid level. For subways, buses, and taxis in a grid, the initial travel volumes for departures and arrivals are aggregated and compared with the passenger flow from the pre-pandemic period to assess the level of mobility. It is observed that the mobility in CBDs was more significantly impacted by COVID-19 compared to areas farther from the CBDs.
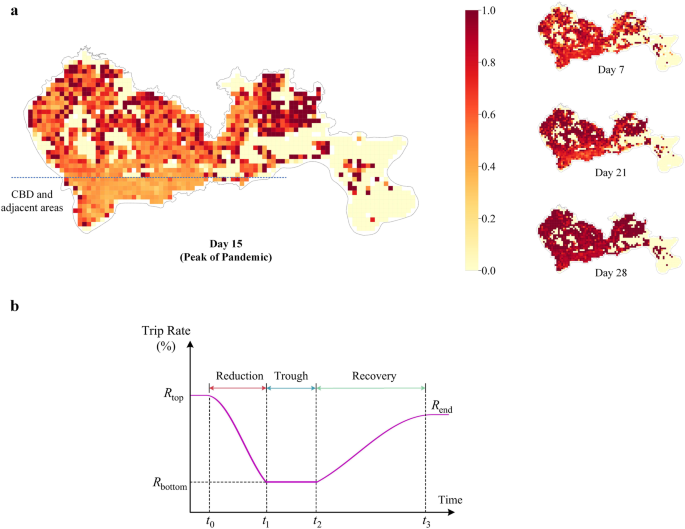
a The departure and arrival passenger flows for each region were aggregated and then divided by their pre-pandemic values to ascertain the mobility levels in each area. Notably, Day 15 was observed to have one of the most significant reductions in passenger flow. Day 7 marked a period of decline, whereas Days 21 and 28 were identified as recovery phases. Furthermore, a disparity in the impact on mobility levels was observed between the CBD and its adjacent areas compared to regions located further from the CBD. b The spontaneous mobility changes consist of the reduction, trough, and recovery stages. These three stages are quantitatively measured and a set of corresponding features are formed, as listed in Table 1 .
CBDs are typically characterized by high density, both in terms of population and infrastructure. This density leads to more congested public spaces and transportation systems. During the pandemic, such conditions increased the risk of virus transmission, leading to more stringent movement restrictions and a greater reluctance among the public to travel in these areas. Furthermore, this may be related to the attributes of land use, which will be discussed in the section “Population behavior behind mobility patterns”.
Identifying mobility patterns
To investigate mobility changes at a finer granularity (street level), bus and subway data were processed based on station information and passenger flow, while taxi data were analyzed by urban areas (see Methods for a detailed description of the data). The OD data derived from the public bus, subway, and taxi systems in Shenzhen include both spatial and temporal information on urban mobility. In the bus system, bus routes establish connectivity between two distinct urban regions corresponding to the origin station and destination station, and passenger flow is influenced by the gradual spread of the epidemic, resulting in temporal variations. This principle is similarly applicable to subway and taxi systems.
Following the large-scale outbreak of the COVID-19 pandemic (post-December 7, 2022), the mobility for almost all OD pairs swiftly plummeted from pre-pandemic levels to a markedly low volume, eventually showing signs of gradual recovery, as shown in Fig. 2 . This change in trips can be delineated via a temporal variation curve for each OD pair, as shown in Fig. 3 a. We normalize each time series of OD passenger flow using historical data (i.e., typical passenger flow levels before November 25, 2022) and subject the data to preprocessing.
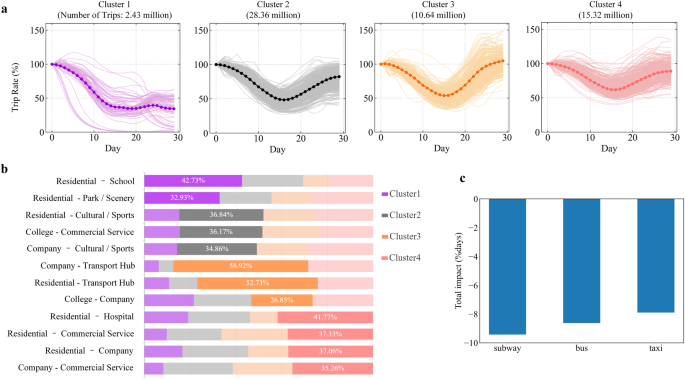
a This time series chart shows the changes in OD trip rate during the period from December 8, 2022, to January 6, 2023. The clustering algorithm identifies four distinct groups with different mobility patterns of decline and recovery. The middle line in each plot represents the average trip rate for each cluster. b Through the spatial attractiveness of ULU, we can infer prominent mobility behavior under each cluster. The changes in mobility are related to mobility behavior between different origins and destinations. c indicates the total impact on the overall passenger volumes of subway, bus, and taxi services.
To quantify the initial decline and subsequent recovery in mobility during the COVID-19 pandemic (Fig. 2 ), we establish a set of features to assess how trips evolve, as listed in Table 1 . The concept of resilience is about how the system responds to disturbances (Qian et al., 2022 ; Schwarz, 2018 ; Standish et al., 2014 ; Tabatabaei et al., 2018 ): does it withstand the shock and remain unchanged, does it adapt and transform into a new state, or does it collapse (Forzieri et al., 2022 ; Kumpfer, 2002 ; Zhao et al., 2021 ). Inspired by this, we concentrate on the resilience of mobility to the pandemic—a characteristic of mobility decreasing and rapidly recovering from large-scale infectious diseases. Features are devised to quantitatively measure the three stages—reduction, trough, and recovery— capturing the mobility changes throughout the COVID-19 pandemic.
Once the features of changes in mobility for OD pairs are extracted, the mobility trends for different modes of transportation can be uniformly analyzed. Based on the aforementioned properties, we employ unsupervised machine learning technology, i.e. K-means++ clustering algorithm (Arthur and Vassilvitskii, 2006 ), to discern the similarities and disparities in passenger flow among various OD pairs (see Methods for detail). As depicted in Fig. 3 a, the K -means++ algorithm categorizes the OD trip trends into four distinct patterns.
The OD mobility in Cluster 1 exhibits the most notable decline, with a total magnitude of impact of -13.47(% ⋅ days), as presented in Table 1 . In the initial phase of the COVID-19 pandemic, a precipitous decrease in passenger flow was observed, with the average value plummeting to less than 50% by the 12th day. While the subsequent descent in passenger flow exhibited a slower pace, a persistently low level or downward trend was maintained. Certain OD passenger flows reached a standstill till the conclusion of the statistical period. This suggests a high degree of travel flexibility within this cluster, or significant aversion to infection, implying that this demographic endeavors to avoid travel throughout the pandemic. Furthermore, the resurgence of travel intent tends to be protracted, with a near-zero recovery rate in the short term.
Cluster 2 exhibits a pronounced U-shape curve with a comparable decline rate, as shown in Fig. 3 a. The nadir of OD passenger flow appeared around the 15th day, hovering at approximately 50%. Thereafter, the travel volume exhibited a gradual resurgence, culminating in an average travel volume recovery of 82.2%. The graphical representation illustrated a significantly slower pace of travel recovery in the pandemic’s later stages, in contrast to the rapid decline observed during the initial phases. The mobility behavior characterizing Cluster 2 aligns with the epidemiological patterns of infection and recovery. During the initial phase of the COVID-19 pandemic, citizens refrained from traveling, either due to active infection or as a preventive measure against contagion. However, as the infection peaked and those infected began to recover, travel volume followed a trajectory of gradual recovery. This pattern is representative of the majority of mobility behavior.
The mobility change curve for Cluster 3 also exhibits a U-shaped pattern; however, its recovery speed significantly outpaces that of Cluster 2. Following the onset of the pandemic, there was a rapid decrease in passenger flow, averaging approximately a 48.8% reduction. Contrasting with the sluggish recovery observed in Cluster 2, the resurgence pace in Cluster 3 mirrors the speed of passenger flow decline experienced during the reduction stage. Consequently, by the conclusion of the observation period, the OD mobility level essentially reverted to its pre-epidemic benchmark. In the end, the mobility behavior corresponding to this cluster rapidly surmounted the impacts of the COVID-19 pandemic, facilitating, and even accelerating, the return to pre-epidemic levels.
The mobility patterns of OD bus stations in Cluster 4 are minimally impacted by the COVID-19 pandemic, with the total impact merely a third of that experienced by Cluster 1. The corresponding curve demonstrated minor fluctuations, with a peak declining amplitude of 38.2%. Across the tripartite stages of mobility alteration (reduction, trough, recovery), the pandemic’s impact on passenger volume was relatively insubstantial. This suggests that within this cluster, the corresponding origins and destinations exhibit robust travel demand, complemented by relatively inflexible mobility behavior.
Population behavior behind mobility patterns
The two crucial facets of population behavior are where to go and how to get there. Consequently, we delve into discussions on insights regarding travel purposes and modes during the processes of mobility decline and recovery.
Travel purpose
Mobility between two areas is aggregated from population behavior with various travel purposes. Lands with specific uses around each origin and destination have the potential to reveal the land-use characteristics of the places where passengers most visit (Chang et al., 2021 ; Sun et al., 2007 ). Therefore, the integration of OD data and the ULU map has the potential to reveal the travel purposes behind OD trips. For example, if a passenger departs from a station (or pick-up point of a taxi trip) surrounded by lands with the category of residential and alights at lands with the category of the company at night o’clock, it can be inferred that the passenger is most likely commuting to work. Therefore, the ULU categories clearly indicate people’s travel purposes.
More generally, we establish a ULU feature vector to represent the probability of departure or arrival at each nearby urban land category (Xing et al., 2020 ). We have collected nine common types of ULU information from Gaode Maps and Baidu Maps, including the residential, company, commercial service, transport hub, college, school, hospital, cultural/sport, and park/scenery. The OD pairs are mapped to OD land pairs according to the corresponding ULU feature vectors (see Methods for details). We further delineate the primary ULU visited and the key travel objectives within each cluster, as presented in Fig. 3 b.
Travel behavior to schools is mainly classified within Cluster 1 and Cluster 2. Following COVID-19’s onset, passenger flow experienced a precipitous decline, with no short-term recovery trend in sight. Firstly, the health of minors is often perceived as more vulnerable, prompting parents to exercise added caution in safeguarding their children from the COVID-19 virus (She et al., 2020 ). Parents likely prefer to refrain from sending their children to school, owing to concerns regarding viral transmission. Subsequently, as infection rates escalated, schools transitioned to online or remote learning modalities (Betthäuser et al., 2023 ). This shift obviated the need for students, teachers, and staff to commute daily, thereby perpetuating the decrease in passenger flow. The passenger volume at school-associated stations was anticipated to remain low until the pandemic stabilizes. As for colleges, numerous students were sent home ahead of the lifting of the "Zero-COVID" policy, resulting in a significant decline in passenger flow.
The majority of trips from residential, company lands to park/scenery, cultural/sports lands are concentrated within clusters with a more significant impact on mobility. Analyzing travel sentiments reveals that these journeys are deemed non-essential, and the inclination to embark on them significantly diminishes following the COVID-19 pandemic outbreak (Han et al., 2021 ). Given the increased risk of COVID-19 infection, older populations exhibit heightened caution when using buses and visiting crowded locations, resulting in highly affected resilience. After contracting COVID-19 (Wang et al., 2022 ), individuals experience compromised systemic and pulmonary functions (Mulcahey et al., 2021 ), leading to a reluctance to engage in strenuous physical activity at sports halls for a short duration. Consequently, there was a diminished recovery in passenger flow to sports halls. Although there is no lockdown ban, citizens avoid visiting by bus and maintain social distance.
Intriguingly, despite the overarching epidemic conditions, passenger counts for the transport serving major transport hubs such as airports, train stations, and ferry terminals exhibit comparatively minor reductions and rapid recovery, mainly aligning with the trends of Cluster 3 and Cluster 4. The relative stability of these numbers can largely be attributed to the inherent nature of long-distance travel across cities. Unlike short-range transit, which may be supplanted by walking, cycling, or personal vehicles, alternatives for long-haul journeys are notably limited, thereby maintaining a baseline demand for buses servicing these transport hubs even during the height of the COVID-19 pandemic.
The alterations in mobility patterns concerning residential—hospital are primarily observed within clusters characterized by a comparatively modest reduction in mobility. The OD trips exhibited fluctuation, characterized by a decline and a gradual recovery within 30 days. In the initial stages, individuals with chronic conditions are advised to minimize hospital visits to lower their exposure risk to COVID-19. However, the pandemic triggered an escalation in healthcare demand, as numerous individuals sought medical care, testing, and treatment (Birkmeyer et al., 2020 ; Peiffer-Smadja et al., 2020 ). The heightened need for infectious disease services counterbalanced the reduction in visits to other outpatient clinics in hospitals, thereby contributing to the recovery of passenger flow towards hospitals. Moreover, the surge in hospital visits necessitated that healthcare workers and other essential personnel continue to commute to and from hospitals, irrespective of the pandemic situation. Their unwavering travel patterns help maintain a basic level of passenger flow of over 40% trip rate.
The behavior disparity between residential—company exhibits various patterns, with a significant proportion found in various clusters. Overall, they are relatively less affected, serving as primary driving factors for urban mobility recovery. It is possibly related to the various industries of companies. On one hand, the characteristics of various industries significantly influence the patterns of public transport use. Employees in industries of internet technology and electric communication demonstrate greater adaptability to remote work during the COVID-19 pandemic. Due to the pressing demand for healthcare products, drugs, and research, stations located near pharmaceutical companies are expected to experience a faster recovery in passenger flow. The nature of manufacturing and factory work typically requires on-site participation, rendering remote work impractical. On the other hand, income levels within industries also influence travel patterns. Employees in the internet and telecommunications industries tend to have higher incomes and have more flexibility in choosing their transport modes, potentially opting for private vehicles over public transport during the COVID-19 pandemic to reduce exposure risks. In contrast, lower-income employees might be more reliant on public transportation to commute to their workplaces, maintaining the demand for buses. The income disparity between industries further contributes to the observed differences in passenger flow trends during the pandemic.
Travel behavior involving commercial service falls within Cluster 3 and Cluster 4. The effect on the service industry essentially aligns with the proportion of the population infected with the virus. Following about three years of "Zero-COVID" policy, the associated panic has largely dissipated. As people yearn to return to normalcy and recreational activities, customer flow at shopping malls stages a swift recovery in later phases. This observation appears comprehensible, given the fundamental nature of work activities as a pivotal component of economic endeavors. With the resumption of work activities, a consequential surge in demands for commercial activities is anticipated (Ma et al., 2023 ).
Overall, during the COVID-19 pandemic, commuting, commercial, and healthcare demand constituted the largest components of urban mobility. It is crucial to maintain and promptly restore the supply of buses among communities, transport hubs, hospitals, and companies. Integrating ULU data with OD data enables governments and transport operators to thoroughly analyze and elucidate shifts in mobility behavior during the pandemic. This comprehensive approach offers a solid foundation for well-informed policy development and implementation against unforeseen pandemics with high infection and low case fatality rates.
Travel mode
The total impact for the overall passenger volumes of subway, bus, and taxi services are computed, as presented in Fig. 3 c. The data indicates varying degrees of reduction in passenger flow, with subways (−9.42% ⋅ days) experiencing the most significant decline, followed by buses (−8.63% ⋅ days), and taxis (−7.90% ⋅ days). This trend can be primarily attributed to the perceived risk of COVID-19 transmission in different transportation environments and the adaptive responses of urban populations to the pandemic.
Subways, typically characterized by high passenger densities and closed environments, represent the apex of perceived transmission risk. The significant drop in subway usage can be attributed to people’s avoidance of crowded spaces and potential virus hotspots. Furthermore, the role of subways as connectors of various urban hubs rendered them particularly vulnerable to reduced usage as individuals sought to minimize travel and potential exposure to the virus. In contrast, buses, while also experiencing a notable decline in passenger flow, were marginally less impacted than subways. This difference might be due to the varied nature of bus routes, some of which cater to essential travel less feasible via other means, and the slightly lower passenger densities compared to subways.
Taxis, offering more individualized and controlled travel environments, demonstrated the least reduction in passenger flow. This trend suggests a public preference for modes of transportation perceived as safer and less conducive to virus spread. Nonetheless, the overall decline in taxi usage reflects broader patterns of reduced mobility, driven by lockdown measures, the shift to remote work, and heightened public health awareness. Economic factors also played a role, as the financial impacts of the pandemic might have influenced individuals’ transportation choices, with taxis being a costlier option compared to public transit. Collectively, these observations underscore the multifaceted impact of the COVID-19 pandemic on urban transportation, shaped by an interplay of health, lifestyle, and economic considerations.
Dynamic model of urban mobility
We develop a dynamic OD mobility model to quantitatively simulate the fine-grained impact of the COVID-19 pandemic on OD passenger flow. The mobility changes can be ascribed to the rate of COVID-19 infection and the willingness to travel. We employ an epidemic transmission model, the susceptible-infectious-removed (SIR) model (Cooper et al., 2020 ; Keeling and Eames, 2005 ), to simulate the rise in infection cases in the city. In general, people who got afflicted with COVID-19 would recuperate at home for days, leading to a plunge in overall city-wide human mobility flow. However, some patients might choose to crowd into the hospitals which leads to an unusual increase in travel needs between urban lands. Therefore, we establish the willingness factors to represent the emotional effects of the COVID-19 pandemic on various types of mobility behavior. The disparity in OD passenger flow under high spatial granularity can be elucidated by the travel purposes and travel modes (refer to Methods for details).
Specifically, people in the dynamic OD model have three distinct states: usual (U), infectious (I), and recovered (R). (Fig. 4 ). In contrast to the classic SIR model, the usual state in the proposed model refers to passengers who remained uninfected by the COVID-19 pandemic, with unaffected travel willingness. Infectious passengers encompass those who have contracted the COVID-19 virus or have been driven by panic to abstain from bus travel. Over time, if affected passengers decide to resume travel, they transition out of the recovered state. The model has merely four free parameters that scale: (1) transmission rate of the COVID-19 pandemic, (2) recovery rate of the COVID-19 pandemic, (3) willingness factors for transmission, and (4) willingness factors for recovery; all four parameters persist as constants over time. The first two parameters, which are determined by the infectivity and virulence of the epidemic itself, remain consistent for all OD pairs. The latter two categories of parameters are determined by travel purposes, which can be inferred by the ULU surrounding OD bus stations.
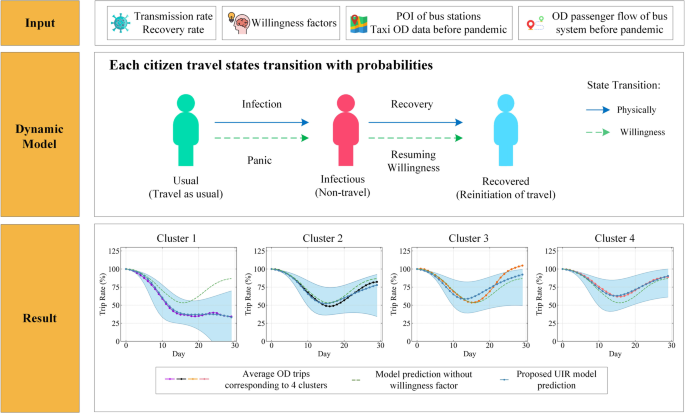
The model inputs encompass the pandemic’s transmission and recovery rates, as well as people’s willingness factors. Furthermore, the model requires the ULU information near bus stations and the pre-outbreak historical passenger flow levels. In the model, the passengers have usual (U), infectious (I), and recovered (R) states. We fit models to all OD pairs in bus, subway, and taxi systems, which shows full model fits of four clusters corresponding to Fig. 3 . The blue line represents the model predictions. As the trips of the OD passenger flow tend to have great variability, we also show the smoothed average (line of a different color from blue). Shaded regions denote the 2.5th and 97.5th percentiles across stochastic realizations. The green dashed line represents the predictions without considering willingness factors (In this case, the predictions for all clusters are consistent). We sample 100 parameter sets of willingness factors and perform stochastic realizations for each set.
The willingness factors serve as a measure of how much the panic mentality contributes to the decline and recovery in mobility. They reflect the degree of anxiety and perceived risk associated with traveling during the COVID-19 pandemic. For example, as the number of infectious passengers increases, other passengers perceive a higher risk of infection when taking the subway, which leads them to seek alternative means of transport or avoid traveling altogether. This corresponds to a negative willingness factor. Conversely, the surge in public health demand during the COVID-19 pandemic leads to positive willingness factors associated with medically related travel. The willingness factors collectively illustrate the various responses of different travel purposes to the COVID-19 pandemic.
Our model precisely matches the observed trips of OD pairs in Shenzhen from December 8, 2022, to January 6, 2023, as shown in Fig. 4 . As shown in Supplementary Fig. 1 , the average values of willingness factors correspond to the analysis of mobility patterns in section “Population behavior behind mobility patterns”. For example, the value of the willingness factor for recovery associated with the travel behavior of school-age children exhibits a significantly negative trend, indicating a slow recovery in their travel activities. This observation aligns well with the predominant mobility pattern observed within Cluster 1. The willingness factors for the recovery of “residential-transport hub” and “company-transport hub” are the two highest positive values, indicating that there are fewer viable alternatives for long-distance travel, and as a result, passenger flow is less affected. The distinctions between subway, bus, and taxi services can also be discerned from the willingness factors.
At a micro level, we can forecast the decline and recovery of mobility between any two stations or taxi-operating regions in Shenzhen. At a macro level, our model precisely captures the average mobility changes for the four clusters (Fig. 4 ). The dynamic OD model enables even a relatively straightforward UIR model to accurately fit observed passenger flow, despite mobility behavior during that period. This model offers valuable insights into urban mobility during potential future outbreaks of infectious diseases with high infection, empowering policymakers to deduce alterations in urban mobility and population behavior during the initial stages of an epidemic outbreak.
Although COVID-19 has become a familiar presence in our lives, it continues to pose a significant global threat. As we write this paper, the virus still claims a life every three minutes (WHO, 2023 ). The suffering and the painful lessons learned from the COVID-19 pandemic must not be in vain. The analytical framework and model proposed in this research contribute to the long-term management of the COVID-19 pandemic and offer tools to confront potential future viral epidemics.
Based on 148 million travel records, this paper examines the fine-grained spatio-temporal characteristics of urban mobility during the COVID-19 pandemic. It integrates these characteristics with an urban land use map to elucidate the heterogeneity in the decline and recovery of mobility. In terms of travel purposes, trips originating from schools and colleges experienced a sharp decline attributable to class suspensions and risk aversion to infections. Non-essential travel, such as visits to museums, sports halls, and parks, saw a substantial decrease and a gradual recovery. In contrast, travel associated with commuting, commercial services, and healthcare exhibited relatively modest declines and a quicker rebound. Regarding transportation modes, the most affected to least affected were subway, bus, and taxi. Subsequently, the UIR model with willingness factors is built, which comprehensively captures the influence of COVID-19 on travel willingness. In contrast to previous research that primarily focused on macro-level mobility analysis, we propose a set of indicators to measure the temporal variations in urban mobility and uncover the heterogeneity of mobility changes through clustering. There were rare urban mobility models at the block level encompassing various modes of transportation.
Our dataset has limitations as it does not encompass all modes of urban mobility. The model is also minimalist, neglecting regional differences in epidemic spread. Nevertheless, our findings are valuable for revealing spatial differences in the decline and recovery of travel. The proposed analytical framework and dynamic model are adaptable and extensible, which can be applied to different pandemics of other cities or other travel modes, capturing detailed aspects of real-world urban mobility.
This paper offers potential implications for future application and research aimed at formulating more targeted and effective public health policies and strategies. In the context of evaluating public health policies, our results can guide policymakers in assessing the opportunity cost of urban mobility limitations during large-scale pandemic outbreaks. The dynamic OD urban mobility model provides a benchmark for mobility changes without mobility-restricting intervention, enabling the precise evaluation of the impacts on the transmission rate and economic productivity. Additionally, in the realm of policy formulation, policy decisions can influence mobility behavior by altering travel willingness and emotions associated with different POI types, significantly affecting the outcome of the epidemic infection. Improved paid leave policy or income support can reduce mobility for essential staff during illness. Strengthening hygiene and disinfection, maintaining proper ventilation, and increasing routes between origins and destinations of high travel demand contribute to enhanced passenger safety. Lastly, in terms of future academic research, fusing large language models with computational, interactive agents seems to be a path to realistic simulations of human behavior (Xu et al., 2023 ). Based on emerging urban sensing technologies (e.g., drones (Wang et al., 2022 ) and crowdsensing (Chen et al., 2019 ; Guo et al., 2021 ; Xia et al., 2023 ), diverse and heterogeneous human behavioral data are continuously mined. Integrating travel behavior and large models to construct urban individual profiles for simulating travel behaviors appears to be a promising direction. Additionally, we will continue to investigate the changes and resilience of urban elements when faced with restricted urban mobility, including variations in air pollution (Chen et al., 2020 ), unmanned delivery (Chen et al., 2022 ), and emergency communication (Ren et al., 2023 ).
Origin-destination data of urban mobility
Thanks to the extensive deployment of mobile devices (Chen et al., 2020 ), the travel data are collected by a public transportation service company in Shenzhen. The original data, gathered by 663 bus routes, 16 subway routes, and 17,826 taxis during operation, total comprises approximately 148 million trips. Through advanced data collection (Li et al., 2022 ) and processing techniques (Chen et al., 2018 ), aggregated OD data can be obtained. The data collection period for bus-related information spans from October 15, 2022, to January 6, 2023, while that for subway and taxi data extends from November 1, 2022, to January 6, 2023. The OD data for buses has a shape of (3010, 3010, 98), where the first and second dimensions correspond to 3010 bus stations representing origins and destinations, respectively, and the third dimension represents dates. Similarly, the OD data for subways has a shape of (240, 240, 67) with a similar interpretation. The original data for taxis consists of individual trip records, including vehicle ID, pick-up time, drop-off time, pick–up latitude and longitude, and drop-off latitude and longitude, among other details. Based on the urban land use (ULU) map in Shenzhen (Fig. 5 a), which can be divided into 11362 regions (Gong et al., 2020 ), we perform individual matching of each order’s latitude and longitude to the corresponding urban region or the nearest urban region based on proximity to the region’s boundaries. The taxi OD data has been organized into a matrix of shapes (11362, 11362, 67). Among them, we specifically focused on the data of 56 million urban mobility records spanning from December 8, 2022, to January 6, 2023. The data preceding December 7, 2022, are utilized to calculate the baseline level of mobility and assist in obtaining the spatial attractiveness of ULU.
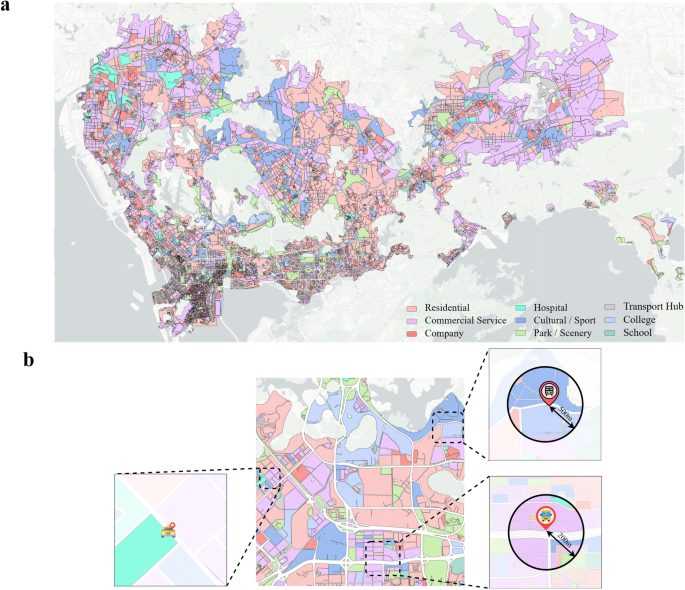
a This map shows urban land use categories in Shenzhen. b This figure illustrates the process of obtaining the urban land use feature vector.
This dataset, capturing changes in passenger flow before and after China’s cancellation of the "Zero-COVID" policy, is distinct from other datasets. China adhered to the "Zero-COVID" policy from 2020 to 2022, with over 99% of Chinese residents never having been infected with COVID-19. After the policy’s cancellation on December 7, 2022, Shenzhen experienced the entire process of large-scale COVID-19 spread without mobility-restricting intervention. As of July 23rd, 2023, China has implemented universal and free COVID-19 vaccination for its population. The reported vaccination rates are as follows (Xinhua, 2022 ): a cumulative coverage rate of 92.1% for the first dose, a completion rate of 89.7%, and an enhanced immunization rate of 71.7%. Among individuals aged 60 and above, the rates are 89.6% for at least one dose, 84.7% for completion, and 67.3% for enhanced immunization. The widespread administration of vaccines has significantly reduced the mortality rate, facilitating recovery from COVID-19. However, the transmissibility of SARS-CoV-2 remains high. This data offers insights into the effects of highly infectious and low-toxicity viruses on social mobility and public transport use under natural transmission conditions in the city. This information provides a baseline for assessing the potential impact of future infectious diseases on urban mobility in the absence of government intervention.
This dataset encompasses the entire process of trip changes, from the initial stage to a sharp decline, and ultimately to a gradual recovery during large-scale infectious disease outbreaks in cities. From November 1, 2022, to November 25, 2022, Shenzhen experienced fewer than 50 confirmed cases per day, and the daily lives of citizens remained largely unaffected (Shenzhen Government, 2022 ). The data from this period can be considered the baseline passenger volume for Shenzhen’s bus system. As the number of confirmed cases progressively increased, the government implemented stringent lockdown measures from November 25, 2022, to December 7, 2022, resulting in a substantial decrease in passenger flow. As of December 7, 2022, the cumulative number of confirmed cases constituted less than 0.1% of the total population. The official outbreak of the COVID-19 pandemic transpired after the cancellation of the "Zero-COVID" policy. The Omicron variant of the severe acute respiratory syndrome coronavirus 2 (SARS-CoV-2) spread rapidly (Leung et al., 2023 ), which is evident in the drastic reduction of passenger flow within the public transport system. Subsequently, as a majority of urban residents became infected and recovered, the public transport passenger flow gradually rebounded, as depicted in Fig. 1 .
Assuming the passenger flow from origin i to destination j on day t is denoted as f i , j , t , the entire dataset can be denoted as
where M represents the sum of the number of bus stations, subway stations, and the total number of taxi-operating urban regions. T is the number of days.
Point of interest data and urban land use data
As online social media and mobile communication continue to flourish, location-based service (LBS) systems such as Google Places, Gaode Maps (China Satellite Maps), and Facebook are gaining popularity across various sectors (Han et al., 2021 ; Xing et al., 2020 ). These LBS systems enable users to search for points of interest (POIs) to access better services and share experiences from places they have visited (Chang et al., 2021 ). Generally, a POI is a specific location that individuals may find useful or intriguing. The term is commonly used to refer to commercial services, schools, subway stations, or other categories found in digital maps (Wang et al., 2019 ; Zhu et al., 2020 ).
A detailed land use map of Chinese cities can be derived by combining POI data with 10-meter satellite imagery, OpenStreetMap, nighttime lights, and Tencent social big data (Gong et al., 2020 ). This map serves as a valuable resource for inferring travel intentions. As the data is from 2018, we complement the newly developed land use attributes based on the latest available POI data. The data sources include Gaode Maps and Baidu Maps, which are leading providers of digital map content, navigation, and location service solutions in China. Important urban land use categories for travel are selected, including residential, company, commercial service, transport hub, college, school, hospital, cultural/sport, and park/scenery.
OD data preprocessing
For each OD pair ( i , j ) in bus, subway, and taxi systems, the time series of mobility can be represented as:
Since the passenger flow at a single OD pair exhibits randomness, f i , j experiences significant fluctuations over time, as shown in Supplementary Fig. 2 . To better illustrate the impact of COVID-19 on public transport, we apply Kalman filtering to smooth the data.
Kalman filtering is a recursive algorithm utilized for estimating the state of a dynamic system by combining noisy measurements with a mathematical model of the system (Chui et al., 2017 ). When applied to noisy time series data, the Kalman filter can provide a smoothed version of the data by recursively estimating the underlying state of the system that generated the data. The filter accounts for uncertainties in both the measurements and the system’s model, rendering it particularly effective at reducing noise while preserving the true signal. Upon applying Kalman filtering, the resulting sequence for the OD station pair ( i , j ) is denoted as:
The mobility between different OD pairs exhibits significant variation, with a maximum difference spanning several orders of magnitude. To uncover the general patterns impact of large-scale infectious diseases on passenger flow, it is essential to perform normalization on the time series \({{{{{\bf{f}}}}}^{{\prime} }}_{i,j}\) . Specifically, for any OD pair ( i , j ), the passenger flow on December 8, 2022 is considered a normal level unaffected by the epidemic. The normalized time series is calculated by:
Identifying mobility patterns via clustering algorithm
Measuring of mobility changes.
The features of changes in mobility are developed to facilitate description and subsequent clustering analysis. Drawing inspiration from the resilience in the fields of biology and engineering (Schwarz, 2018 ; Standish et al., 2014 ; Tabatabaei et al., 2018 ), we develop a set of features to comprehensively quantify the decline and recovery patterns of travel before, during, and after a large-scale infectious disease outbreak. These features can characterize the temporal changes in the response of mobility behavior to the pandemic, which reflects the interplay between human behavior, public policy, and the built environment, and provides insights into the dynamics of urban mobility under extraordinary circumstances.
Firstly, for any OD time series \({{{{{\bf{f}}}}}^{{\prime}{\prime}}}_{i,j}\) , we can identify several basic features, including the maximum value R top before the passenger flow decreases, the minimum value R bottom of passenger flow, the final passenger flow level R end , and their corresponding time moments t 0 , t 1 , t 2 , t 3 . The definition and significance of the features are as follows.
Declining speed Γ: The speed at which trips decrease serves as a measure of how quickly urban populations adapt their mobility behavior in response to public health crises. It is calculated by:
Declining amplitude Λ: The maximum amplitude of the decrease in trips directly mirrors the influence of the epidemic on mobility behavior.
Trough duration Π: The third indicator of mobility changes we define pertains to the trough duration of mobility. This indicator captures the persistence of the reduced trips during the COVID-19 pandemic, reflecting the extent to which the usual travels are disrupted.
Recovery speed Θ: When infected individuals gradually recover and the overall panic levels subside, this metric captures the speed at which urban mobility bounces back to normalcy. The determination of the indicator is achieved through:
Recovery amplitude ϒ: The amplitude of mobility recovery highlights the degree to which trips rebound after the initial impact of the pandemic. As infected citizens gradually recover and no longer reject traveling, this indicator provides insights into the interplay between public health measures, human behavior, and the ability of societies to regain their normal functioning after experiencing a large-scale infectious disease outbreak.
Total impact Δ: We finally introduce a comprehensive metric that captures the decline and recovery process of the pandemic’s impact on mobility. This total impact indicator effectively represents the degree to which the pandemic affects mobility, calculated as the area between the curve and the usual level of trips using the formula:
A lower value indicates a more substantial influence of the epidemic on mobility.
Therefore, we extract features of mobility change from each OD array ( i , j ):
K-means++ clustering
In order to identify change patterns of OD mobility during the pandemic, we perform the K-means++ clustering algorithm on the OD dataset. K -means++ clustering is an unsupervised machine learning algorithm used for partitioning a given dataset into a specified number ( K ) of clusters.
K-means++ clustering aims to minimize the within-cluster sum of squared (WCSS) distances from the data points to their respective cluster centroids by iteratively updating the centroids and assigning the data points. Specifically, the input of the K-means++ clustering algorithm is
where each OD time series is represented by the features.
The algorithm first needs to initialize the cluster center. A centroid c 1 is randomly chosen from X . For each x i , j ∈ X , compute the squared distance to its nearest centroid:
where \({{{\mathcal{C}}}}={\left\{{c}_{p}\right\}}_{p = 1,...,k-1}\) represents the set of centroids chosen so far. Then, the next centroid c k is determined from the data points with probability proportional to \(D{({x}_{i})}^{2}\) :
Repeat the two steps until we have taken K centers altogether.
After selecting the K initial centroids, the algorithm proceeds with the standard iterative updates of centroids. Step 1 is assigning x i , j to the closest centroid using ( 13 ). Suppose that at iteration n , the dataset is divided into K clusters:
In step 2, calculate new centroid positions for each cluster by minimizing the WCSS distance:
The two steps are repeated until convergence is reached, i.e., when the assignment of samples to clusters no longer changes.
The elbow method is utilized to determine the optimal number of clusters, denoted as K , in K-means clustering (Bholowalia and Kumar, 2014 ). The elbow method involves plotting the variance explained or the sum of squared distances (SSE) of the data points to their cluster centroids against different values of K . The elbow method suggests selecting the value of K at the “elbow" or bend in the SSE plot (Supplementary Fig. 3a) . This point represents the optimal trade-off between minimizing SSE and avoiding excessive complexity in the clustering model.
After establishing a preliminary range for K using the elbow method, Silhouette Analysis is employed to finalize the value of K (Lleti et al., 2004 ). For each K -value, silhouette coefficient is computed for each data point, which measures the similarity between each data point and the cluster to which it is assigned, with values ranging from –1 to 1. Subsequently, the average silhouette coefficient is calculated for all data points at each K -value. This average value serves as an indicator of cluster cohesion and separation, with higher values indicating better clustering results. As depicted in Supplementary Fig. 3b , the analysis determined that the optimal value for K = 4.
Finally, we derive K clusters of OD mobility, as shown in Fig. 3 a. The labels for each OD pair have been obtained. Furthermore, the OD pairs can be associated with spatial information, which leads to subsequent analysis.
Spatial attractiveness of urban land use
Our goal is to illuminate the variation in mobility for OD pairs during a widespread infectious disease outbreak. Solely analyzing the geographical locations of the starting and ending stations of travel, however, is inadequate. As a result, we endeavor to extract travel purposes by integrating the urban land use map.
Lands in close proximity to bus stations, subway stations, and taxi pick-up and drop-off points provide insight into passengers’ potential travel motivation. For example, when a bus station is located in a residual land, there is a high probability that passengers disembarking at this station intend to commute to their residences. Accordingly, a ULU feature vector is established for each origin and destination, which is represented as:
where r = 1, 2, . . . , R represents urban land use categories. s i , r is defined as the spatial attractiveness of land use category r to the citizens boarding or disembarking from the station or urban regions i ( i = 1, 2, . . . , M ).
For the bus stations and subway stations, a common method (Xing et al., 2020 ) is to use the number of lands to determine s i , r :
where z i , r denotes the number of lands belonging to category r within a certain distance range of the stations. Taking into account the varying importance of stations, the distance range for bus stations is set at 200 meters, while the distance range for subway stations is set at 500 meters, as presented in Fig. 5 b.
As mentioned in “Origin-destination data of urban mobility”, the taxi OD data represents the mobility between 11,362 distinct regions within the city. These urban regions have been delineated with nearly homogeneous land use attributes. Therefore, s i , r can be derived directly:
For an urban region with the land use attribute r , the s i , r value corresponds to 1, while the remaining values of the land feature vector are set to 0.
As each urban land use category can be mapped to an activity, identifying the most probable land use category corresponds to determining the most likely activity to be undertaken. To further analyze the mobility behavior from the origin i to the destination j , we compute the travel transition matrix:
The elements in the matrix S i , j represent the likelihood of passengers transitioning from a land use category at the origin station (region) to a ULU category at the destination station (region). For instance, s i ,1 s j ,2 in the bus system corresponds to passengers departing from land use category 1 near bus station i and arriving at land use category 2 near bus station j . From this, we can infer the passengers’ mobility behavior and purposes. The travel transition matrix for the subway and taxi follows the same principle.
We combine the K clusters of change patterns with the spatial attractiveness of urban land use. For each cluster, we sum the corresponding S i , j values to obtain a total transition matrix. Considering daily frequency changes in passenger flow, the passenger flow from station i to station j is approximately equal to the passenger flow from station j to station i on a daily timescale. Based on the symmetry of OD passenger flow, we add the lower and upper triangular parts of the total transition matrix. Finally, we identify the top values in the matrix that correspond to the primary OD land pairs and their corresponding proportions of mobility behavior within each cluster.
Dynamic OD mobility model
We develop a dynamic model to capture the fine-grained urban mobility changes. The decline in trips can be attributed to two factors: physiological infection with viruses and emotional influence. Inspired by the classic epidemic transmission model SIR (Cooper et al., 2020 ; Keeling and Eames, 2005 ), an OD passenger flow model is proposed, comprehensively considering the spread of the COVID-19 pandemic and the willingness to travel via public transportation, as shown in Fig. 4 .
The model incorporates pandemic transmission and recovery rates, willingness factors of mobility behavior, urban land use map, and pre-pandemic OD passenger flow as input. It outputs the changes in trips for every OD pair during the COVID-19 pandemic with high spatial resolution.
Physiological infection module
Prior to the outbreak of large-scale infectious diseases, we assume that the daily number of population from station i to station j is W i , j . Following the epidemic outbreak, all passengers can be categorized into three states: usual (U), infected (I) by the virus or panic, and recovered (R). The relationship among passengers associated with the three states is shown in the subsequent ( 22 ).
Specifically, initially uninfected people may become infected with the virus through contact with infected individuals. Eventually, the infected people transition to a recovery state. For each citizen, the probability state transition process of physiological infection is as follows:
where U i , j , t , I i , j , t , R i , j , t denote passenger numbers of usual state, infected state, and recovery state, respectively on the t day. β and γ represent the transmission and recovery rates, respectively, which are inherent attributes of the pandemic spread.
Willingness influence module
During the pandemic, urban residents tend to reduce their travel activities to mitigate the risk of infection. This tendency is particularly pronounced when there is a higher reported or perceived number of infections and potential cases through news outlets, social media, or personal observations, leading to increased levels of anxiety. During the recovery phase, mobility gradually rebounds over time, and confidence in travel is steadily restored. Furthermore, the decline and recovery of different travel behaviors exhibit heterogeneity. From the clustering results in the section “Population behavior behind mobility patterns”, it is evident that the decrease and recovery of trips are associated with travel purposes.
Willingness factors are proposed to model the willingness influence. It is assumed that the willingness factors of travel from ULU type r to ULU type v follow normal distributions:
where r , v = 1, 2, , . . . , R . \({\mu }_{r,v},{\sigma }_{r,v}^{2}\) and \({{\mu }^{{\prime} }}_{r,v},{\sigma }_{r,v}^{{\prime} 2}\) are distribution parameters (mean and variance) of willingness factors for transmission and recovery, respectively.
In terms of travel mode choices, individuals tend to opt for modes associated with lower exposure to viral risks. Consequently, the willingness factor for transmission and recovery in the context of subway, bus, and taxi are denoted as \({\mu }_{q},{{\mu }^{{\prime} }}_{q}(q=1,2,3)\) .
The probability state transition process of emotional influence on travel is as follows:
Put it together
For an OD pair ( i , j ) of transport mode q , the travel transition matrix S i , j decomposes travel for a given OD pair into combinations of various trip purposes. Consequently, it enables the synthesis of willingness factors, thereby generating the willingness factor for that specific OD pair. The willingness factors can be estimated by linearly stacking the sampled willingness factors for travel purposes:
The mobility change for OD pair ( i , j ) can be determined through the following equation.
The time series of the passenger flow from origin i to destination j is:
W i , j is determined by the trips of OD ( i , j ) prior to the pandemic outbreak. α i , j and \({\alpha }_{i,j}^{{\prime} }\) denote willingness factors for transmission and recovery, respectively. These willingness factors depend on the ULU feature arrays of the origin i and the destination j , accounting for the diversity of OD passenger flow. The initial values of U i , j , t , I i , j , t , R i , j , t are determined by the initial infection rate in the city.
By obtaining the changes in passenger flow at all OD pairs, we can also derive the overall change in trips under the impact of the pandemic. This model characterizes the response of an entire city’s public transport system to the pandemic and the shifts in mobility behavior.
Model validation
The alterations in actual OD passenger flow within the Shenzhen bus, subway, and taxi systems during the COVID-19 pandemic serve to verify the effectiveness of the dynamic model. Research (Cai et al., 2022 ; Leung et al., 2023 ; Ren et al., 2022 ; Ribeiro Xavier et al., 2022 ) indicates that the transmission rate of SARS-CoV-2 ranges from approximately 0.2 to 0.4, while the recovery rate spans from approximately 0.05 to 0.15. These values are determined by the inherent transmission characteristics of COVID-19. This paper primarily concentrates on modeling the variations in mobility for different OD stations. Without loss of generality, we employ β = 0.3, γ = 0.1.
When calculating the willingness factor for recovery, we consider only the main ULU pairs depicted in Fig 3 b. Consequently, the parameter estimation target constitutes the mean values and standard deviations of willingness factors for OD ULU pairs. Based on the maximum likelihood estimation (MLE) method, we find the optimal parameter values of the willingness factor distributions, enabling the predictive changes in the mobility model to approximate the actual trend. Specifically, we employed the grid search method to identify the values of \({\alpha }_{r,v},{{\alpha }^{{\prime} }}_{r,v}\) that correspond to the minimum root mean squared error (RMSE) between the true trips and predicted trips. These values of \({\alpha }_{r,v},{{\alpha }^{{\prime} }}_{r,v}\) are treated as sampling data for the distribution of willingness factors to be estimated. Subsequently, we determined the parameter values that maximize the probability of sampling data occurrence, resulting in the optimal mean values and standard deviations of the empirical factors.
The optimization results show that the values of willingness factors for transmission are similar for all OD station pairs, whereas the willingness factors for recovery primarily contribute to the diversity observed in mobility behavior, as displayed in Supplementary Fig. 1 . Under the optimal parameters, we sample and derive 100 sets of dynamic model parameters. The predictive trips in Shenzhen during the COVID-19 pandemic are shown in Fig. 4 .
Besides, an analysis is conducted to evaluate the robustness of the UIR model. The values of β and γ vary by approximately ± 30% and observe the model performance while keeping other parameters constant. This analysis focused on calculating the average root mean square error (RMSE) between the actual and predicted values of passenger flow across various OD pairs in the city. The RMSE for each trip series provided a daily prediction error. As shown in Supplementary Fig. 4 , our findings indicate that for every 1% deviation in β , the prediction error increased by approximately 0.61%, and for every 1% deviation in γ , the prediction error increased by around 0.77%. These results suggest a certain level of sensitivity of the model to these parameters.
Data availability
Urban land use categories are available at https://data-starcloud.pcl.ac.cn/zh . Points of interest (POIs) data can be openly accessed via the APIs of Gaode Maps ( https://lbs.amap.com/ ) and Baidu Maps ( https://lbsyun.baidu.com/ ). Raw origin-destination (OD) data of buses and taxis are available with the permission of Shenzhen Bus Group Co., Ltd. ( https://en.szbus.com.cn/ ) upon request for academic cooperation. The de-identified data are available at https://github.com/trainandtest666/OD-Data-in-Public-Transport-System.git .
Arellana J, Márquez L, Cantillo V (2020) COVID-19 outbreak in Colombia: an analysis of its impacts on transport systems. J Adv Transp 2020:1–16. https://doi.org/10.1155/2020/8867316
Article Google Scholar
Arthur D, Vassilvitskii S (2007) k-means++: the advantages of careful seeding. Soda 7:1027–1035
MathSciNet Google Scholar
Atkinson-Clement C, Pigalle E (2021) What can we learn from COVID-19 pandemic’s impact on human behaviour? The case of France’s lockdown. Humanit Soc Sci Commun 8(1):81. https://doi.org/10.1057/s41599-021-00749-2
Balmford B, Annan JD, Hargreaves JC, Altoè M, Bateman IJ (2020) Cross-country comparisons of COVID-19: policy, politics and the price of life. Environ Resour Econ 76:525–551. https://doi.org/10.1007/s10640-020-00466-5
Betthäuser BA, Bach-Mortensen AM, Engzell P (2023) A systematic review and meta-analysis of the evidence on learning during the COVID-19 pandemic. Nat Hum Behav 7:375–385. https://doi.org/10.1038/s41562-022-01506-4
Article PubMed Google Scholar
Bholowalia P, Kumar A (2014) EBK-means: a clustering technique based on elbow method and k-means in WSN. Int J Comput Appl 105(9):17–24
Google Scholar
Birkmeyer JD, Barnato A, Birkmeyer N, Bessler R, Skinner J (2020) The impact of the COVID-19 pandemic on hospital admissions in the United States: study examines trends in US hospital admissions during the COVID-19 pandemic. Health Affairs 39(11):2010–2017. https://doi.org/10.1377/hlthaff.2020.00980
Burki T (2022) Dynamic zero COVID policy in the fight against COVID. Lancet Respir Med 10(6):e58–e59. https://doi.org/10.1016/S2213-2600(22)00142-4
Article CAS PubMed PubMed Central Google Scholar
Cai J, Deng X, Yang J et al. (2022) Modeling transmission of SARS-CoV-2 omicron in China. Nat Med 28(7):1468–1475. https://doi.org/10.1038/s41591-022-01855-7
Chang S, Pierson E, Koh P, Gerardin J, Redbird B, Grusky D, Leskovec J (2021) Mobility network models of COVID-19 explain inequities and inform reopening. Nature 589(7840):82–87. https://doi.org/10.1038/s41586-020-2923-3
Article ADS CAS PubMed Google Scholar
Chen L, Xu F, Han Z, Tang K, Hui P, Evans J, Li Y (2022) Strategic COVID-19 vaccine distribution can simultaneously elevate social utility and equity. Nat Hum Behav 6:1503–1514. https://doi.org/10.1038/s41562-022-01429-0
Chen X, Wang H, Li Z et al. (2022) DeliverSense: efficient delivery drone scheduling for crowdsensing with deep reinforcement learning. In: UbiComp/ISWC’22 Adjunct, Cambridge, United Kingdom, 11–15 September, pp. 403–408
Chen X, Xu S, Fu H, Joe-Wong C, Zhang L, Noh H.Y., Zhang P (2019) Asc: Actuation system for city-wide crowdsensing with ride-sharing vehicular platform. In: Proceedings of the Fourth Workshop on International Science of Smart City Operations and Platforms Engineering, Montreal, Quebec, Canada, 15 April, pp. 19–24
Chen X, Xu S, Han J et al. (2020) Pas: prediction-based actuation system for city-scale ridesharing vehicular mobile crowdsensing. IEEE Internet Things J 7(5):3719–3734. https://doi.org/10.1109/JIOT.2020.2968375
Chen X, Xu S, Liu X, Xu X, Noh HY, Zhang L, Zhang P (2020) Adaptive hybrid model-enabled sensing system (HMSS) for mobile fine-grained air pollution estimation. IEEE Trans Mobile Comput 21(6):1927–1944. https://doi.org/10.1109/TMC.2020.3034270
Chen X, Xu X, Liu X, Pan S, He J, Noh HY, Zhang L, Zhang P (2018) Pga: Physics guided and adaptive approach for mobile fine-grained air pollution estimation. In: Proceedings of the 2018 ACM International Joint Conference and 2018 International Symposium on Pervasive and Ubiquitous Computing and Wearable Computers, Singapore, 08–12 October, pp. 1321–1330
China Center for Disease Control and Prevention (2022) China Daily Perspectives: the dynamic COVID-zero strategy in China. https://doi.org/10.46234/ccdcw2022.015 . Accessed 24 May, 2023
Chui CK, Chen G et al. (2017) Kalman filtering. Springer
Clark A, Jit M, Warren-Gash C et al. (2020) Global, regional, and national estimates of the population at increased risk of severe covid-19 due to underlying health conditions in 2020: a modelling study. Lancet Glob Health 8(8):e1003–e1017. https://doi.org/10.1016/S2214-109X(20)30264-3
Cooper I, Mondal A, Antonopoulos CG (2020) A SIR model assumption for the spread of COVID-19 in different communities. Chaos Soliton Fract 139:110057. https://doi.org/10.1016/j.chaos.2020.110057
Article MathSciNet Google Scholar
Forzieri G, Dakos V, McDowell NG, Ramdane A, Cescatti A (2022) Emerging signals of declining forest resilience under climate change. Nature 608(7923):534–539. https://doi.org/10.1038/s41586-022-04959-9
Gkiotsalitis K, Cats O (2021) Public transport planning adaption under the COVID-19 pandemic crisis: literature review of research needs and directions. Transp Rev 41:374–392. https://doi.org/10.1080/01441647.2020.1857886
Gong P, Chen B, Li X et al. (2020) Mapping essential urban land use categories in China (EULUC-China): preliminary results for 2018. Sci Bull 65(3):182–187. https://doi.org/10.1016/j.scib.2019.12.007
Guo B, Wang S, Ding Y, Wang G, He S, Zhang D, He T (2021) Concurrent order dispatch for instant delivery with time-constrained actor-critic reinforcement learning. In: 2021 IEEE Real-Time Systems Symposium (RTSS), pp. 176–187
Han Z, Fu H, Xu F, Tu Z, Yu Y, Hui P, Li Y (2021) Who will survive and revive undergoing the epidemic: Analyses about POI visit behavior in Wuhan via check-in records. In: Proceedings of the ACM on Interactive, Mobile, Wearable and Ubiquitous Technologies, NY, USA, vol. 5, pp. 1–20
Keeling MJ, Eames KT (2005) Networks and epidemic models. J R Soc Interface 2(4):295–307. https://doi.org/10.1098/rsif.2005.0051
Article PubMed PubMed Central Google Scholar
Kumpfer KL (2002) Factors and processes contributing to resilience: the resilience framework. Resilience and development: positive life adaptations. 179–224. https://doi.org/10.1007/0-306-47167-1_9
Leung K, Lau EH, Wong CK, Leung GM, Wu JT (2023) Estimating the transmission dynamics of SARS-CoV-2 Omicron BF. 7 in Beijing after the adjustment of zero-COVID policy in November-December 2022. Nat Med 29:579–582. https://doi.org/10.1038/s41591-023-02212-y
Article CAS PubMed Google Scholar
Levin R, Chao DL, Wenger EA, Proctor JL (2021) Insights into population behavior during the COVID-19 pandemic from cell phone mobility data and manifold learning. Nat Comput Sci 1(9):588–597. https://doi.org/10.1038/s43588-021-00125-9
Li Z, Man F, Chen X, Zhao B, Wu C, Chen X (2022) TRACT: Towards large-scale crowdsensing with high-efficiency swarm path planning. In: UbiComp/ISWC’22 Adjunct, Cambridge, United Kingdom, 11–15 Sept, pp. 409–414. https://doi.org/10.1145/2994551.2996714
Liu Y, Yu Y, Zhao Y, He D (2022) Reduction in the infection fatality rate of Omicron variant compared with previous variants in South Africa. Int J Infect Dis 120:146–149. https://doi.org/10.1016/j.ijid.2022.04.029
Lletı R, Ortiz MC, Sarabia LA, Sánchez MS (2004) Selecting variables for k-means cluster analysis by using a genetic algorithm that optimises the silhouettes. Anal Chim Acta 515(1):87–100
Ma S, Li S, Zhang J (2023) Spatial and deep learning analyses of urban recovery from the impacts of COVID-19. Sci Rep 13(1):2447. https://doi.org/10.1038/s41598-023-29189-5
Article ADS CAS PubMed PubMed Central Google Scholar
Martínez L, Short J (2021) The pandemic city: urban issues in the time of COVID-19. Sustainability 13(6):3295. https://doi.org/10.3390/su13063295
Menkir TF, Chin T, Hay JA et al. (2021) Estimating internationally imported cases during the early COVID-19 pandemic. Nat Commun 12(1):311. https://doi.org/10.1038/s41467-020-20219-8
Mulcahey MK, Gianakos AL, Mercurio A, Rodeo S, Sutton KM (2021) Sports medicine considerations during the COVID-19 pandemic. Am J Sports Med 49(2):512–521. https://doi.org/10.1177/0363546520975186
Peiffer-Smadja N, Lucet J, Bendjelloul G et al. (2020) Challenges and issues about organizing a hospital to respond to the COVID-19 outbreak: experience from a French reference centre. Clin Microbiol Infect 26(6):669–672. https://doi.org/10.1016/j.cmi.2020.04.002
Qian T, Chen X, Xin Y, Tang W, Wang L (2022) Resilient decentralized optimization of chance constrained electricity-gas systems over lossy communication networks. Energy 239:122158. https://doi.org/10.1016/j.energy.2021.122158
Ren J, Xu Y, Li Z, Hong C, Zhang XP, Chen X (2023) Scheduling UAV swarm with attention-based graph reinforcement learning for ground-to-air heterogeneous data communication. In: UbiComp/ISWC’23 Adjunct, Cancun, Quintana Roo, Mexico, 08–12 October, pp. 670–675. https://doi.org/10.1145/3594739.3612905 (2023)
Ren SY, Wang WB, Gao RD, Zhou AM (2022) Omicron variant (B. 1.1. 529) of SARS-CoV-2: mutation, infectivity, transmission, and vaccine resistance. World J Clin Cases 10(1):1
Ribeiro XC, Sachetto OR, da FVV et al. (2022) Characterisation of Omicron variant during COVID-19 pandemic and the impact of vaccination, transmission rate, mortality, and reinfection in South Africa, Germany, and Brazil. BioTech 11(2):12. https://doi.org/10.3390/biotech11020012
Article CAS Google Scholar
Schwarz S (2018) Resilience in psychology: a critical analysis of the concept. Theory Psychol 28(4):528–541. https://doi.org/10.1177/0959354318783584
She J, Liu L, Liu W (2020) COVID-19 epidemic: disease characteristics in children. J Med Virol 92(7):747–754. https://doi.org/10.1002/jmv.25807
Shen J, Duan H, Zhang B et al. (2020) Prevention and control of COVID-19 in public transportation: experience from China. Environ Pollut 266(2):115291. https://doi.org/10.1016/j.envpol.2020.115291
Shenzhen Government (2023) Notice of the Shenzhen municipal people’s government on issuing the overall plan and three year action plan for park city construction in Shenzhen (2022–2024). http://www.sz.gov.cn/zfgb/2023/gb1272/content/post_10389162.html . Accessed 3 Aug 2023
Shenzhen Open Data Platform (2022) COVID-19 in Shenzhen—statistics of daily confirmed cases. https://opendata.sz.gov.cn . Accessed 12 Aug 2023
Sibley C, Greaves L, Satherley N et al. (2020) Effects of the COVID-19 pandemic and nationwide lockdown on trust, attitudes toward government, and well-being. Am Psychol 75(5):618–630. https://doi.org/10.1037/amp0000662
Standish RJ, Hobbs R, Mayfield M et al. (2014) Resilience in ecology: Abstraction, distraction, or where the action is? Biol Conserv 177:43–51. https://doi.org/10.1016/j.biocon.2014.06.008
Sun H, Forsythe W, Waters N (2007) Modeling urban land use change and urban sprawl: Calgary, Alberta, Canada. Netw Spat Econ 7:353–376. https://doi.org/10.1007/s11067-007-9030-y
Tabatabaei NM, Ravadanegh SN, Bizon N (2018) Power systems resilience. Springer
Taskinsoy J (2020) COVID-19: Is the great outbreak a sign of what the future has stowed for the human race? Available at SSRN 3597434. https://doi.org/10.2139/ssrn.3597434
Tirachini A, Cats O (2020) COVID-19 and public transportation: current assessment, prospects, and research needs. J Public Transp 22(1):1–21. https://doi.org/10.5038/2375-0901.22.1.1
Tisdell CA (2020) Economic, social and political issues raised by the COVID-19 pandemic. Econ Anal Policy 68:17–28. https://doi.org/10.1016/j.eap.2020.08.002
United Nations (2018) 68% of the world population projected to live in urban areas by 2050, says UN. https://www.un.org/development/desa/en/news/population/2018-revision-of-world-urbanization-prospects.html . Accessed 20 Aug 2022
Wang D, Tayarani M, He BY, Gao J, Chow JYJ, Gao HO, Ozbay K (2021) Mobility in post-pandemic economic reopening under social distancing guidelines: Congestion, emissions, and contact exposure in public transit. Transp Res Part A Policy Pract 153:151–170. https://doi.org/10.1016/j.tra.2021.09.005
Wang H, Chen X, Cheng Y et al. (2022) H-SwarmLoc: efficient scheduling for localization of heterogeneous MAV swarm with deep reinforcement learning. In: Proceedings of the 20th ACM Conference on Embedded Networked Sensor Systems, Boston, Massachusetts, US, November, pp. 1148–1154
Wang J, Huang J, Yang H, Levinson D (2022) Resilience and recovery of public transport use during COVID-19. npj Urban Sustain 2(1):18. https://doi.org/10.1038/s42949-022-00061-1
Wang S, He T, Zhang D et al. (2019) Towards efficient sharing: a usage balancing mechanism for bike sharing systems. In: WWW’19, San Francisco, CA, USA, 13-17 May, pp. 2011–2021
Wei Y, Wang J, Song W, Xiu C, Ma L, Pei T (2021) Spread of COVID-19 in China: analysis from a city-based epidemic and mobility model. Cities 110:103010. https://doi.org/10.1016/j.cities.2020.103010
Weiss M, Schwarzenberg A, Nelson R, Sutter K, Sutherland M (2020) Global economic effects of COVID-19. Congressional Research Service. pp. 21–35
World Health Organization (WHO) (2023) WHO Director-General’s opening remarks at the media briefing—5 May 2023. https://www.who.int/director-general/speeches/detail/who-director-general-s-opening-remarks-at-the-media-briefing---5-may-2023 . Accessed 22 Jun 2023
Xia K, Lin L, Wang S, Wang H, Zhang D, He T (2023) A predict-then-optimize couriers allocation framework for emergency last-mile logistics. In: Proceedings of the 29th ACM SIGKDD Conference on Knowledge Discovery and Data Mining, Long Beach, CA, USA, 6–10 August, pp. 5237–5248. https://doi.org/10.1145/3580305.3599766
Xing Y, Wang K, Lu JJ (2020) Exploring travel patterns and trip purposes of dockless bike-sharing by analyzing massive bike-sharing data in Shanghai, China. J Transp Geogr 87:102787. https://doi.org/10.1016/j.jtrangeo.2020.102787
Xinhua News Agency (2022) The overall coverage rate of COVID-19 vaccine in China. http://www.news.cn/politics/2022-07/23/c_1128857426.htm . Accessed 11 Jul 2023
Xu F, Zhang J, Gao C, Feng J, Li Y (2023) Urban generative intelligence (UGI): a foundational platform for agents in embodied city environment. https://doi.org/10.48550/arXiv.2312.11813
Zhang A, Zhang K, Li W, Wang Y, Li Y, Zhang L (2022) Optimising self-organised volunteer efforts in response to the COVID-19 pandemic. Humanit Soc Sci Commun 9(1):134. https://doi.org/10.1057/s41599-022-01127-2
Zhang J (2021) People’s responses to the COVID-19 pandemic during its early stages and factors affecting those responses. Humanit Soc Sci Commun 8:37. https://doi.org/10.1057/s41599-021-00720-1
Zhao P, Cao Z, Zeng DD, Gu C, Wang Z, Xiang Y, Qadrdan M, Chen X, Yan X, Li S (2021) Cyber-resilient multi-energy management for complex systems. IEEE Trans Industr Inform 18(3):2144–2159. https://doi.org/10.1109/TII.2021.3097760
Zhu X, Wang S, Guo B et al. (2020) SParking: A win-win data-driven contract parking sharing system. In: UbiComp/ISWC ’20 Adjunct, Virtual Event, Mexico, 12–16 September, pp. 596–604
Download references
Acknowledgements
This paper was supported by the National Key R&D Program of China No. 2022YFC3300703, the Natural Science Foundation of China under Grant No. 62371269. Guangdong Innovative and Entrepreneurial Research Team Program No. 2021ZT09L197, Shenzhen 2022 Stabilization Support Program No. WDZC20220811103500001, Tsinghua Shenzhen International Graduate School Cross-disciplinary Research and Innovation Fund Research Plan No. JC20220011, the Project from Science and Technology Innovation Committee of Shenzhen (Grant No. KCXST20221021111201002), the Major Key Project of PCL (Peng Cheng Laboratory) under Grants PCL2023A09, and Meituan.
Author information
These authors contributed equally: Baining Zhao, Xuzhe Wang.
Authors and Affiliations
Shenzhen International Graduate School, Tsinghua University, Shenzhen, China
Baining Zhao, Xuzhe Wang, Tianyu Zhang, Fanhang Man, Yang Li & Xinlei Chen
Peng Cheng Laboratory, Shenzhen, China
Baining Zhao, Tao Sun & Xinlei Chen
Institute of artificial intelligence, Beihang University, Beijing, China
Department of Electronic Engineering, Tsinghua University, Beijing, China
Fengli Xu & Yong Li
Department of Mathematics, Shenzhen University, Shenzhen, China
Erbing Chen
RISC-V International Open Source Laboratory, Shenzhen, China
Xinlei Chen
You can also search for this author in PubMed Google Scholar
Contributions
Fellow researchers and authors contributed as follows: Conceptualization: BZ, RS, YL, & XC; methodology: XW, BZ, & TS; formal analysis: RS, FX, & XC; writing/original draft preparation: BZ, XW, FM, EC, & TZ; writing/review and editing: TS, XW, XC, BZ, & YL; BZ, XW, & TS contributed equally to this work and share first authorship. Correspondence to XC.
Corresponding author
Correspondence to Xinlei Chen .
Ethics declarations
Competing interests.
The authors declare no competing interests.
Ethical approval
This article does not contain any studies with human participants performed by any of the authors.
Informed consent
Additional information.
Publisher’s note Springer Nature remains neutral with regard to jurisdictional claims in published maps and institutional affiliations.
Supplementary information
Supplementary figs, rights and permissions.
Open Access This article is licensed under a Creative Commons Attribution 4.0 International License, which permits use, sharing, adaptation, distribution and reproduction in any medium or format, as long as you give appropriate credit to the original author(s) and the source, provide a link to the Creative Commons licence, and indicate if changes were made. The images or other third party material in this article are included in the article’s Creative Commons licence, unless indicated otherwise in a credit line to the material. If material is not included in the article’s Creative Commons licence and your intended use is not permitted by statutory regulation or exceeds the permitted use, you will need to obtain permission directly from the copyright holder. To view a copy of this licence, visit http://creativecommons.org/licenses/by/4.0/ .
Reprints and permissions
About this article
Cite this article.
Zhao, B., Wang, X., Zhang, T. et al. Estimating and modeling spontaneous mobility changes during the COVID-19 pandemic without stay-at-home orders. Humanit Soc Sci Commun 11 , 591 (2024). https://doi.org/10.1057/s41599-024-03068-4
Download citation
Received : 30 August 2023
Accepted : 17 April 2024
Published : 08 May 2024
DOI : https://doi.org/10.1057/s41599-024-03068-4
Share this article
Anyone you share the following link with will be able to read this content:
Sorry, a shareable link is not currently available for this article.
Provided by the Springer Nature SharedIt content-sharing initiative
Quick links
- Explore articles by subject
- Guide to authors
- Editorial policies


The Social Science Research Council is pleased to announce the recipients of the 2024 Data Fluencies Dissertation Grants, in partnership with Simon Fraser University and with support from the Mellon Foundation. The awards will support five Ph.D. students’ research projects on just and equitable uses of information, data, and technology.
Privacy Overview
Communities of microorganisms and invertebrates in soil-like bodies of soccer fields in Moscow oblast
- Soil Biology
- Published: 06 November 2014
- Volume 47 , pages 1107–1115, ( 2014 )
Cite this article
- O. V. Kutovaya 1 ,
- I. V. Zamotaev 2 &
- V. P. Belobrov 1
76 Accesses
Explore all metrics
Artificially created soil-like technogenic formations (STFs) of soccer fields are developed under combined action of intense technogenic and natural factors and processes, which cannot but affect the structure and biological activity of their microbial communities and mesofauna. The microflora of the STFs is very similar to the microflora of the background soddy-podzolic soils of Moscow oblast with respect to the composition of the physiological groups of microorganisms. However, they are drastically different in their quantitative characteristics. The numbers of all the trophic groups of microorganisms, except for the microscopic fungi, in the STFs are much higher than those in the zonal soils. An increased biological activity of the STFs is due to regular watering, heating, application of sand and mineral fertilizers, and technogenic turbation processes. The mesofauna of the STFs is represented by several ecological groups of earthworms, including soildwelling (endogeic) earthworms ( Aporrectodea caliginosa ), epigeic earthworms dwelling at the soil-litter interface ( Lumbricus rubellus ), and litter-dwelling earthworms ( Eisenia foetida ).
This is a preview of subscription content, log in via an institution to check access.
Access this article
Price includes VAT (Russian Federation)
Instant access to the full article PDF.
Rent this article via DeepDyve
Institutional subscriptions
Similar content being viewed by others
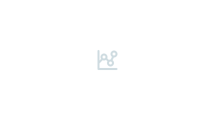
The structure of mesofauna complexes in soils of the forest-park zone of Moscow and the Prioksko-Terrasnyi Biospheric Reserve
Agrophysical assessment of alluvial calcareous soils of the çumra region of central anatolia in turkey, micromorphological and microbiological diagnostics of initial pedogenesis on the bottom of an artificial mesodepression in the northern caspian semidesert.
G. G. Abramashvili, Sports Lawns. Methodological Guidelines for Employees of Stadiums and Sports Bases Exploiting Soccer Fields (Sovetskii sport, Moscow, 1988) [in Russian].
Google Scholar
A. L. Aleksandrovskii and E. I. Aleksandrovskaya, Soil Evolution and the Geographic Environment (Nauka, Moscow, 2005) [in Russian].
V. N. Bashkin and N. S. Kasimov, Biogeochemistry (Nauchn. mir, Moscow, 2004) [in Russian].
O. S. Bezuglova, S. N. Gorbov, A. V. Gorovtsov, et al., “Agrochemical and microbiological properties of constructozems of golf courses and their impact on the state of the lawns,” Probl. Agrokhim. Ekolog., No. 4, 14–17 (2012).
O. S. Bezuglova, S. N. Gorbov, I. V. Morozov, and D. G. Nevidomskaya, Urbopedology: A Textbook (Izd. Yuzhn. Fed. Univ., Rostov-on-Don, 2012) [in Russian].
V. P. Belobrov and I. V. Zamotaev, Soils and Green Lawns of Sports and Technical Constructions (GEOS, Moscow, 2007) [in Russian].
V. P. Belobrov, I. V. Zamotaev, and A. Yu. Kulenkamp, “Pedogenic and technogenic processes in artificial recreation landscapes of Russia,” Dokl. RASKhN, No. 2, 35–38 (2002).
V. A. Bol’shakov, V. P. Belobrov, and L. L. Shishov, Terms, Their Concise Definitions, and Reference Materials on General and Soil Ecology and on the Geography and Classification of Soils (Pochven. Inst. im. V.V. Dokuchaeva RASKhN, Moscow, 2004) [in Russian].
B. A. Byzov, Zoomicrobial Interactions in Soils (GEOS, Moscow, 2005) [in Russian].
E. S. Vasilenko, I. S. Prokhorov, and A. Yu. Sementsov, “Microbiological processes upon the construction of artificial soils,” Agrokhim. Vestn., No. 5, 20–24 (2006).
T. S. Vsevolodova-Perel’, Earthworms of Russian Fauna: Cadaster and Determinative Guide (Nauka, Moscow, 1997) [in Russian].
M. I. Gerasimova, M. N. Stroganova, N. V. Mozharova, and T. V. Prokof’eva, Anthropogenic Soils (Genesis, Geography, and Reclamation) (Izd. Mosk. Gos. Univ., Moscow, 2003) [in Russian].
M. S. Gilyarov, “Dwelling conditions for invertebrates of different size groups in soil,” in Methods of Soil Zoology (Nauka, Moscow, 1975), pp. 7–12 [in Russian].
M. A. Glazovskaya, “Biotic and abiotic factors in the organization of soil biota and morphogenesis of soils,” in Soil Science: History, Sociology, and Methodology , Ed. by V. N. Kudeyarov and I. V. Ivanov (Nauka, Moscow, 2005), pp. 243–257 [in Russian].
M. I. Gol’din and K. Ya. Lyal’chenko, A Soccer Field. Construction and Exploitation (Fizkul’tura i sport, Moscow, 1971) [in Russian].
I. L. Gol’dfarb, Extended Abstract of Candidate’s Dissertation in Geography (Moscow, 2005).
I. S. Goryachkina, A. A. Rakhleeva, M. N. Stroganova, and A. V. Rappoport, “Soil mesofauna of botanical gardens (by the example of Moscow and Saint Petersburg),” Vestn. Mosk Univ., Ser. 17: Pochvoved., No. 4, 33–40 (2003).
T. G. Dobrovol’skaya, A. V. Golovchenko, T. A. Pankratov, L. V. Lysak, D. G. Zvyagintsev, “Assessment of the bacterial diversity in soils: evolution of approaches and methods,” Eur. Soil Sci. 42 (10), 1138–1147 (2009).
Article Google Scholar
I. V. Zamotaev, Extended Abstract of Doctoral Dissertation in Geography (Moscow, 2009).
I. V. Zamotaev, “Factors of soil formation on soccer fields,” Vestn. MGPU, No. 3, 15–32 (2008).
I. V. Zamotaev and V. P. Belobrov, “Technopedogenesis on artificially formed substrates of soccer fields,” Ekolog. Planirov. Upravlen., No. 3(4), 48–63 (2007).
I. V. Zamotaev, V. P. Belobrov, V. T. Dmitrieva, and D. L. Shevelev, Technopedogenesis on Soccer Fields of Russia (Media-PRESS, Moscow, 2012) [in Russian].
I. V. Zamotaev and D. L. Shevelev, “Sports technogenesis as a factor of soil formation,” Probl. Region. Ekolog., No. 6, 268–274 (2009).
D. G. Zvyagintsev, T. G. Dobrovol’skaya, I. P. Bab’eva, G. M. Zenova, L. V. Lysak, “The role of microorganisms in the biogeocenotic functions of soils,” in The Structural-Functional Role of Soils in the Biosphere (GEOS, Moscow, 1999) [in Russian].
F. A. Ivannikov, Extended Abstract of Candidate’s Dissertation in Biology (Moscow, 2012).
A. E. Ivanova, I. S. Sukhanova, and O. E. Marfenina, “Ecotrophic groups of fungi in urban soils of different ages,” Materials of the V All-Russia Soil Sci. Congr. (Rostov-on-Don, 2008), p. 436 [in Russian].
T. K. Il’ina and O. M. Fomina, USSR Inventor’s Certificate No. 1, 3328.
N. A. Karavaeva, “Agrogenic soils: environmental conditions, properties, and processes,” Eur. Soil Sci. 38 (12), 1355–1365 (2005).
N. A. Karavaeva, “Soil climate in the boreal zonal series of natural and agrolandscapes,” in Multifaceted Geography: The Development of Ideas of Innokentii Petrovich Gerasimov (on the Centennial Anniversary of the Birth) (KMK, Moscow, 2005), pp. 195–222 [in Russian].
Classification and Diagnostic System of Russian Soils (Oikumena, Smolensk, 2004) [in Russian].
O. V. Kutovaya, Extended Abstract of Candidate’s Dissertation in Agriculture (Moscow, 2012).
O. V. Kutovaya, “Transformation of the structure of microbial communities in soddy-podzolic soils under the impact of earthworms,” Agrokhim. Vestn., No. 2, 13–14 (2008).
O. V. Kutovaya, “Characterization of humic substances in agrosoddy-podzolic soils and earthworm coprolites,” Byull. Pochv. Inst. im. V.V. Docuchaeva, No. 69, 46–59 (2011).
L. V. Lysak, Extended Abstract of Doctoral Dissertation in Biology (Moscow, 2010).
L. V. Lysak, N. N. Sidorenko, O. E. Marfenina, and D. G. Zvyagintsev, “Microbial complexes in urban soils,” Eur. Soil Sci. 33 (1), 70–75 (2000).
V. G. Matveeva and T. S. Perel’, “Earthworms of the Lumbricidae family in Moscow oblast,” in Soil Invertebrates of Moscow Oblast (Nauka, Moscow, 1982), pp. 133–143 [in Russian].
A Practicum on Microbiology , Ed. by N. S. Egorov (Izd. Mosk. Gos. Univ., Moscow, 1976) [in Russian].
I. S. Prokhorov, Extended Abstract of Candidate’s Dissertation in Agriculture (Moscow, 2006).
A. V. Rappoport, A. S. Myasoedov, and L. V. Lysak, “Biological activity of some urbanozems and kulturozems in Moscow,” in Outlooks for the Development of Soil Biology (MAKS-Press, Moscow, 2001), pp. 279–282 [in Russian].
O. B. Rumyantsev, Soccer Field. Recommendations on Maintenance of Natural Soddy Soccer Fields (PFL Rossii, Moscow, 1999) [in Russian].
I. N. Skvortsova, A. V. Rappoport, T. V. Prokof’eva, and A. E. Andreeva, “Biological properties of soils in the Moscow State University botanical garden: the branch on Prospekt Mira,” Eur. Soil Sci. 39 (7), 771–778 (2006).
A. V. Smagin, Theory and Practice of Soil Construction (Izd. Mosk. Gos. Univ., Moscow, 2012) [in Russian].
N. P. Sorokina, Large-Scale Soil Maps and Their Applications. Methodological Recommendations (Pochv. inst. im. V.V. Dokuchaeva VASKhNIL, Moscow, 1977) [in Russian].
A. L. Stepanov, N. A. Manucharova, A. V. Smagin, A. S. Kurbatova, A. D. Myagkova, V. N. Bashkin, “Characterization of the biological activity of the microbial complex in urban soils,” Eur. Soil Sci. 38 (8), 864–869 (2005).
M. N. Stroganova, Extended Abstract of Doctoral Dissertation in Biology (Moscow, 1998).
E. Z. Tepper, V. K. Shil’nikova, and G. I. Pereverzeva, A Practicum on Microbiology (Drofa, Moscow, 2005) [in Russian].
D. L. Shevelev, Extended Abstract of Candidate’s Dissertation in Geography (Moscow, 2011).
Bauen mit Gruen: die Bauuund Vegetationstechnik des Landschafts und Sportplatzbaus (Parey, Berlin-Hamburg, 1989).
Grundsaetze zur Functions und Umweltgerechten Pflege von Rasensportflaechen. Teil II. Wassersparende Massnahmen , (Bundesinstitut fuer Sportwissenschaft, Hoffman, Schorndor, 1994)
Grundsaetze zur Functions und Umweltgerechten Pflege von Rasensportflaechen. Teil III. Unerwuenschte Pflanzenarten auf Rasensportflaechen , (Bundesinstitut fuer Sportwissenschaft, Verl.-Gmbh, Koeln, 1995).
Grundsaetze zur Functions und Umweltgerechten Pflege von Rasensportflaechen. Teil IY. Pflanzenkrankheiten und Schaedlinge (Bundesinstitut fuer Sportwissen-schaft, Sport und Buch Strauss, Koeln, 1995).
C. G. Matthias, Praxis des Sportplatzbaus. Fehleraufdeckung und Vermeidung (Renningen-Malmsheim, 2002).
J. Puhalla, J. Krans, and J. Goatley, Sports Fields: Design, Construction, Maintenance , (J. Wiley & Sons, 2010).
Sportrasenpflege (DEULA Rheinland Kempen, 2006)..
Urbaner Bodenschutz. Arbeitkreis Stadtboeden der Deutschen Bodenkundlichen Gesellschaft (Hrsg.) (Springer, Berlin-Heidelberg, 1996).
Download references
Author information
Authors and affiliations.
Dokuchaev Soil Science Institute, per. Pyzhevskii 7, Moscow, 119017, Russia
O. V. Kutovaya & V. P. Belobrov
Institute of Geography of the Russian Academy of Sciences, per. Staromonetnyi 29, Moscow, 119017, Russia
I. V. Zamotaev
You can also search for this author in PubMed Google Scholar
Corresponding author
Correspondence to O. V. Kutovaya .
Additional information
Original Russian Text © O.V. Kutovaya, I.V. Zamotaev, V.P. Belobrov, 2014, published in Pochvovedenie, 2014, No. 11, pp. 1315–1324.
Rights and permissions
Reprints and permissions
About this article
Kutovaya, O.V., Zamotaev, I.V. & Belobrov, V.P. Communities of microorganisms and invertebrates in soil-like bodies of soccer fields in Moscow oblast. Eurasian Soil Sc. 47 , 1107–1115 (2014). https://doi.org/10.1134/S1064229314110052
Download citation
Received : 25 February 2014
Published : 06 November 2014
Issue Date : November 2014
DOI : https://doi.org/10.1134/S1064229314110052
The Unique Burial of a Child of Early Scythian Time at the Cemetery of Saryg-Bulun (Tuva)
<< Previous page
Pages: 379-406
In 1988, the Tuvan Archaeological Expedition (led by M. E. Kilunovskaya and V. A. Semenov) discovered a unique burial of the early Iron Age at Saryg-Bulun in Central Tuva. There are two burial mounds of the Aldy-Bel culture dated by 7th century BC. Within the barrows, which adjoined one another, forming a figure-of-eight, there were discovered 7 burials, from which a representative collection of artifacts was recovered. Burial 5 was the most unique, it was found in a coffin made of a larch trunk, with a tightly closed lid. Due to the preservative properties of larch and lack of air access, the coffin contained a well-preserved mummy of a child with an accompanying set of grave goods. The interred individual retained the skin on his face and had a leather headdress painted with red pigment and a coat, sewn from jerboa fur. The coat was belted with a leather belt with bronze ornaments and buckles. Besides that, a leather quiver with arrows with the shafts decorated with painted ornaments, fully preserved battle pick and a bow were buried in the coffin. Unexpectedly, the full-genomic analysis, showed that the individual was female. This fact opens a new aspect in the study of the social history of the Scythian society and perhaps brings us back to the myth of the Amazons, discussed by Herodotus. Of course, this discovery is unique in its preservation for the Scythian culture of Tuva and requires careful study and conservation.
Keywords: Tuva, Early Iron Age, early Scythian period, Aldy-Bel culture, barrow, burial in the coffin, mummy, full genome sequencing, aDNA
Information about authors: Marina Kilunovskaya (Saint Petersburg, Russian Federation). Candidate of Historical Sciences. Institute for the History of Material Culture of the Russian Academy of Sciences. Dvortsovaya Emb., 18, Saint Petersburg, 191186, Russian Federation E-mail: [email protected] Vladimir Semenov (Saint Petersburg, Russian Federation). Candidate of Historical Sciences. Institute for the History of Material Culture of the Russian Academy of Sciences. Dvortsovaya Emb., 18, Saint Petersburg, 191186, Russian Federation E-mail: [email protected] Varvara Busova (Moscow, Russian Federation). (Saint Petersburg, Russian Federation). Institute for the History of Material Culture of the Russian Academy of Sciences. Dvortsovaya Emb., 18, Saint Petersburg, 191186, Russian Federation E-mail: [email protected] Kharis Mustafin (Moscow, Russian Federation). Candidate of Technical Sciences. Moscow Institute of Physics and Technology. Institutsky Lane, 9, Dolgoprudny, 141701, Moscow Oblast, Russian Federation E-mail: [email protected] Irina Alborova (Moscow, Russian Federation). Candidate of Biological Sciences. Moscow Institute of Physics and Technology. Institutsky Lane, 9, Dolgoprudny, 141701, Moscow Oblast, Russian Federation E-mail: [email protected] Alina Matzvai (Moscow, Russian Federation). Moscow Institute of Physics and Technology. Institutsky Lane, 9, Dolgoprudny, 141701, Moscow Oblast, Russian Federation E-mail: [email protected]
Shopping Cart Items: 0 Cart Total: 0,00 € place your order
Price pdf version
student - 2,75 € individual - 3,00 € institutional - 7,00 €

Copyright В© 1999-2022. Stratum Publishing House
Extragalactic Science with XRISM
Presentation #303.03 in the session First Results from XRISM.
XRISM, an international JAXA/NASA collaboration including participation from ESA, is an advanced X-ray observatory that will address some of the most important questions in astrophysics in the 2020s. Launched on September 5, 2022, XRISM has two instruments: Resolve, a high-resolution, non-dispersive X-ray spectrometer, providing high-resolution (~5 eV), imaging spectroscopic capability; and Xtend, a wide field imager that offers simultaneous coverage over a ~40 arcmin square field of view, with ~1′ angular resolution. This talk will highlight some of the scientific topics that XRISM will address, with emphasis on extragalactic X-ray sources: active and starburst galaxies and clusters of galaxies.

- AAS Privacy Policy
- AAS Terms of Use

Biomaterials Science
Non-viral vector-based genome editing for cancer immunotherapy.

* Corresponding authors
a Department of Biomedical Engineering, McGill University, Montreal, QC, Canada E-mail: [email protected]
b Rosalind & Morris Goodman Cancer Institute, McGill University, Montreal, QC, Canada
Despite the exciting promise of cancer immunotherapy in the clinic, immune checkpoint blockade therapy and T cell-based therapies are often associated with low response rates, intrinsic and adaptive immune resistance, and systemic side effects. CRISPR-Cas-based genome editing appears to be an effective strategy to overcome these unmet clinical needs. As a safer delivery platform for the CRISPR-Cas system, non-viral nanoformulations have been recently explored to target tumor cells and immune cells, aiming to improve cancer immunotherapy on a gene level. In this review, we summarized the efforts of non-viral vector-based CRISPR-Cas-mediated genome editing in tumor cells and immune cells for cancer immunotherapy. Their design rationale and specific applications were highlighted.
- This article is part of the themed collections: Biomaterials Science Recent Review Articles, 2024 and Biomaterials Science Emerging Investigator Series
Article information
Download citation, permissions.

T. Fang and G. Chen, Biomater. Sci. , 2024, Advance Article , DOI: 10.1039/D4BM00286E
To request permission to reproduce material from this article, please go to the Copyright Clearance Center request page .
If you are an author contributing to an RSC publication, you do not need to request permission provided correct acknowledgement is given.
If you are the author of this article, you do not need to request permission to reproduce figures and diagrams provided correct acknowledgement is given. If you want to reproduce the whole article in a third-party publication (excluding your thesis/dissertation for which permission is not required) please go to the Copyright Clearance Center request page .
Read more about how to correctly acknowledge RSC content .
Social activity
Search articles by author.
This article has not yet been cited.
Advertisements

IMAGES
VIDEO
COMMENTS
An abstract summarizes, usually in one paragraph of 300 words or less, the major aspects of the entire paper in a prescribed sequence that includes: 1) the overall purpose of the study and the research problem(s) you investigated; 2) the basic design of the study; 3) major findings or trends found as a result of your analysis; and, 4) a brief summary of your interpretations and conclusions.
Abstracts are usually around 100-300 words, but there's often a strict word limit, so make sure to check the relevant requirements. In a dissertation or thesis, include the abstract on a separate page, after the title page and acknowledgements but before the table of contents.
Usually, the length of an abstract can be anywhere between 150-250 words, so you must make sure that you include only what is necessary and relevant. Although it is written as a single paragraph, the abstract should summarize information about all sections of your study, including your thesis, goals, approach, main findings, and conclusions. It ...
Therefore, the structure of your dissertation or thesis abstract needs to reflect these four essentials, in the same order. Let's take a closer look at each of them, step by step: Step 1: Describe the purpose and value of your research. Here you need to concisely explain the purpose and value of your research.
Choosing Verb Tenses within Your Abstract. The social science sample (Sample 1) below uses the present tense to describe general facts and interpretations that have been and are currently true, including the prevailing explanation for the social phenomenon under study.That abstract also uses the present tense to describe the methods, the findings, the arguments, and the implications of the ...
Conclusion. The abstract you write for your dissertation or thesis should succinctly explain to the reader why the work of your research was needed, what you did, what you found and what it means. Most people that come across your thesis, including any future employers, are likely to read only your abstract.
Time to recap…. And there you have it - the traditional dissertation structure and layout, from A-Z. To recap, the core structure for a dissertation or thesis is (typically) as follows: Title page. Acknowledgments page. Abstract (or executive summary) Table of contents, list of figures and tables.
Writing a Doctoral Thesis or Dissertation in the Social Sciences Anne Jordan, Ph.D. Ontario Institute for Studies in Education University of Toronto ©2020 A guide for doctoral students at various stages of their doctoral theses and dissertations: Designing their thesis proposals, developing their research
Decide on your own research methodology. Outline your proposed methods and expected results. Use your proposed methodology to choose what chapters to include in your thesis/dissertation. Create a preliminary table of contents to outline the structure of your thesis/dissertation. By following these steps, you should be able to organize the ...
A practical guide for students undertaking their dissertation, Writing Successful Undergraduate Dissertations in Social Sciences uses a mixture of exercises, strategies, case study material and further reading to give hints and tips on beginning and managing a research project and working with supervisors. Providing an accessible overview of the essential steps in conducting research and ...
If so, Doing Your Undergraduate Social Science Dissertation is the book for you, covering the whole dissertation journey from project planning to submission. Using a mixture of useful information, exercises, practical strategies, case study material and further reading, it helps you through the process, giving hints and tips on beginning and ...
Dissertation Abstracts, Dissertation Abstracts International (DAI) is a bibliographic database of American dissertations published since 1938, initially by University Microfilms International ... which is also gaining acceptance in the social sciences, education and business. In it, the purpose of the study and methodological choices are ...
Issued also on CD-ROM (without abstracts, July 1969-June 1980) as part of: Dissertation abstracts ondisc. Continues Dissertation abstracts. A, Humanities and social sciences ( ISSN 0095-9154) ISSN 0419-4209 0012-3854 Key title Dissertation abstracts international. A. The humanities and social sciences
Semantic Scholar extracted view of "Dissertation Abstracts International Section A: Humanities and Social Sciences: Program Participants' Understanding about Program Rules and Its Effect on Program Outcomes: Non-Custodial Parents' Knowledge about Child Support Rules and Its Impact on Child Support Payments" by K. Nam
Dissertation Abstracts International Section A: Humanities and Social Sciences, 72(4-A), 1174. Abstract. These studies define a Buddhist conceptualization of compassion and describe the development of the Compassion Scale. The definition of compassion was adopted from Neff's (2003) model of self-compassion that proposes that the construct ...
No. 12, pt. 2 of v. 30-33 contains the annual cumulated keyword title and author indexes; no. 12, pt. 2 of v. 34-54 and no. 12 of v. 55- contain the annual cumulated author index to sections A and B of Dissertation abstracts international
Other editions - View all. Dissertation Abstracts International: The humanities and social sciences. ASnippet view - 1976. Dissertation Abstracts International: The humanities and social sciences. ASnippet view - 1977. Dissertation Abstracts International: The humanities and social sciences. ASnippet view - 1978.
ISSN 0419-4209 (Print) | Dissertation abstracts international. A. The humanities and social sciences Skip to main content. Leave this field blank . Log In; Automatic login IP ... The humanities and social sciences. Identifiers. ISSN : 0419-4209. Linking ISSN (ISSN-L): 0419-4209. Cancelled ISSN: 0012-3854.
Dissertation abstracts international. A, Humanities and social sciences 0419-4209 Former Title: Dissertation abstracts 0099-3123 Frequency: Monthly Index/Finding Aid Note: No. 21, pt. 2 of v. 27-29 contains the annual cumulated subject and author indexes to sections A and B of Dissertation abstracts. Subject: Humanities -- Abstracts -- Periodicals
Abstract. Social networking sites are integral in reshaping how we access and interact with information and others. This doctoral thesis aims to offer an in-depth understanding of engagement in an ...
Social upgrading does not inherently follow economic upgrading; rather, they present a complex interplay. This paper, focusing on China, utilizes the coupling coordination degree and a panel regression model to shed light on the intricate interaction between social upgrading and economic upgrading. It is found that the coupling coordination degree of social and economic upgrading in China has ...
Cogent Social Sciences List of Issues Volume 10, Issue 1 ... Abstract. The discourse of 'the end of ideology' put forward by Bell in 1960 was centred on the notion that an ideological consensus had been reached, especially in developed countries, and that ideologies were no longer necessary given that economic growth had replaced political ...
Abstract. In this article we examine anti-Semitism as expressed by a sample of residents of the Moscow Oblast (Soviet Union). Based on a survey conducted in 192
Humanities and Social Sciences Communications - Estimating and modeling spontaneous mobility changes during the COVID-19 pandemic without stay-at-home orders Skip to main content Thank you for ...
(1) Background: Early interventions may effectively reduce the risk of mental disorders in individuals with ultra-high risk. Specifying the health needs of individuals with ultra-high risk is crucial before the implementation of successful early intervention. This study aimed to explore the differences in lifestyles, mental risks, and physical indices among individuals with ultra-high risk ...
The Social Science Research Council is pleased to announce the recipients of the 2024 Data Fluencies Dissertation Grants, in partnership with Simon Fraser University and with support from the Mellon Foundation. The awards will support five Ph.D. students' research projects on just and equitable uses of information, data, and technology. The ...
Artificially created soil-like technogenic formations (STFs) of soccer fields are developed under combined action of intense technogenic and natural factors and processes, which cannot but affect the structure and biological activity of their microbial communities and mesofauna. The microflora of the STFs is very similar to the microflora of the background soddy-podzolic soils of Moscow oblast ...
Burial 5 was the most unique, it was found in a coffin made of a larch trunk, with a tightly closed lid. Due to the preservative properties of larch and lack of air access, the coffin contained a well-preserved mummy of a child with an accompanying set of grave goods. The interred individual retained the skin on his face and had a leather ...
Vol. 56, Issue 5 (HEAD 21 Abstracts) Abstract. Published on May 03, 2024. Extragalactic Science with XRISM ... Published on May 03, 2024. Cite. Social. Download. last released. 1 day ago. Show details. Extragalactic Science with XRISM. XRISM, an international JAXA/NASA collaboration including participation from ESA, is an advanced X-ray ...
Despite the exciting promise of cancer immunotherapy in the clinic, immune checkpoint blockade therapy and T cell-based therapies are often associated with low response rates, intrinsic and adaptive immune resistance, and systemic side effects. CRISPR-Cas-based genome editing appears to be an effective strategy to Biomaterials Science Recent Review Articles, 2024 Biomaterials Science Emerging ...